- 1Aquatic Biotechnology Lab., Institute of Marine Research, Spanish National Research Council (IIM-CSIC), Vigo, Spain
- 2Pescanova Biomarine Center, O Grove, Spain
Over the past decade, there has been a growing interest in common octopus aquaculture, prompted by several factors such as the increase in market demand, the decline in overall fisheries and the search for more sustainable food resources. Nevertheless, this interest has raised concerns about the potential impact of large-scale production and intensified farming practices in the future. This study aims to establish a baseline understanding of the natural microbial communities that inhabit skin mucus of the common octopus, describe its core microbiota, and assess the impact of captive rearing on these communities and animal welfare. The skin microbiome of wild-captured octopuses was compared with that of reared in aquaculture using 16S ribosomal RNA metabarcoding. The findings show that the core microbiota of octopus mucosal skin is dominated by the phyla Bacteroidota and Pseudomonadota, with Aurantivirga, Pseudofulvibacter, and Rubritalea being the most abundant genera. Despite differences in abundance, the microbiota composition and diversity between wild and aquaculture octopuses were similar. Wild samples had an enrichment of Gammaproteobacteria, including potentially pathogenic species such as Vibrio spp., Photobacterium swingsii, and Lactococcus garvieae, which were less prevalent or absent in aquaculture samples. KEGG functional pathways predictions indicated a higher enrichment in functional pathways related to xenobiotic remediation in wild samples, reflecting their need to adapt to a more variable and potentially contaminated environment. This is the first study to characterize the mucosal skin microbiome of the common octopus and to compare wild and aquaculture specimens. The results suggest that current aquaculture practices support animal welfare through the use of controlled hatchery environments and high-quality water conditions. These insights are valuable for the development of sustainable and responsible aquaculture practices, with the octopus microbiome serving as a potential biomarker for health status and animal welfare.
1 Introduction
Aquaculture is a fast-growing industry that plays a significant role as a source of seafood production worldwide, accounting for 50% of the total production in 2021 (FAO, 2022). Over the past decade, there has been an increasing interest in cephalopod aquaculture research, driven by the growing market demand, the decline in overall fisheries, and the search for a more sustainable food resource (Berger, 2011; Vidal et al., 2014; Xavier et al., 2015). The common octopus (Octopus vulgaris) is considered a promising candidate for aquaculture diversification in Europe, mainly due to its fast growth rate, short life cycle, good adaptability to captive conditions and high nutritional and economic value (Vaz-Pires et al., 2004; Vidal et al., 2014). This offers a solution to meet market demand while also reducing the pressure on wild populations. Overfishing can significantly impact wild populations by reducing their numbers, disrupting ecosystems, and altering the balance of marine food chains. These practices can lead to the depletion of target species and the accidental catch of non-target species, further threatening biodiversity. Aquaculture not only helps in conserving wild species but also allows for more efficient management of aquatic resources, contributing to the overall health and stability of marine ecosystems. However, despite the significant potential of common octopus aquaculture, its development has been constrained by different factors. First, related to the complex biological and physiological characteristics of this species, particularly regarding environmental parameters and nutritional requirements (Uriarte et al., 2011, 2019). For years, the high mortality rate in the rearing phase of the paralarvae was the primary bottleneck for aquaculture (Iglesias et al., 2007). The reason for this was nutritional deficiencies, particularly the lack of lipids in the live prey that was frequently utilized as food (Navarro and Villanueva, 2000, 2003). This limitation appears to have been resolved with the creation of a patented rearing technique by scientists from the Spanish Institute of Oceanography and the Pescanova Biomarine Center (Tur et al., 2020). This accomplishment has successfully completed the life cycle of this species, allowing for large-scale industrial cultivation. However, the growing interest in octopus aquaculture has also sparked concerns about the future impact of large-scale production and intensified farming practices. In this situation, animal welfare must be a crucial aspect to ensure the development of sustainable and responsible aquaculture practices of this species. Farm animal welfare is generally assessed through measurements of physical health, immune response, behavior, and physiological indicators, with a focus on identifying stress (Fraser et al., 1997; Broom, 2010a). Stress-induced neuroendocrine responses play a critical role in the physiology and overall well-being of animals. While glucocorticoids are recognized as the key hormonal regulators of the physiological stress response in vertebrates (Cockrem, 2013), the underlying mechanisms that govern neuroendocrine responses to stressors in invertebrates, particularly in cephalopods, remain unclear (Fodor and Pirger, 2022). This hinders the evaluation of stress using corticosteroid hormones. A further limitation to the analysis of the impact of stress is the remarkable ability of octopuses and other cephalopods to edit their RNA (Birk et al., 2023; Wang et al., 2023). This capacity enables these animals to precisely regulate their gene expression in response to environmental alterations, thereby facilitating their adaptability, sophisticated behaviours, and neural plasticity. Consequently, transcriptome studies in these species are also complicated due to the high levels of RNA editing, which can obscure the direct relationship between DNA sequences and protein expression.
In recent years, researchers have suggested that the microbial communities present in the host and surrounding environment could serve as a biomarker for health and well-being, owing to their interaction with the host immune system and ability to respond to stressors (Llewellyn et al., 2014; Kraimi et al., 2019; Lorgen-Ritchie et al., 2023). In the case of aquatic animals, the microbial communities on their mucosal surfaces contribute to their individual health by serving a primarily defensive role for the host (Reverter et al., 2018). Stressful conditions in aquaculture, such as netting and transport, can disrupt this balance, leading to increased susceptibility to diseases (Boutin et al., 2013; Chiarello et al., 2015; Minniti et al., 2017; Mougin and Joyce, 2023; Rich et al., 2023). Consequently, an analysis of the composition and abundance of the bacterial microbiome of skin mucus could serve as a non-invasive indicator of the welfare and health status of the common octopus.
Recent advances in high-throughput sequencing technologies and bioinformatics tools have facilitated a more precise investigation of the composition and abundance of microbial communities. Specifically, metagenomics has emerged as the leading technique for analyzing genetic material obtained directly from an environmental sample, thereby overcoming the constrains associated with culture-based methods. These advancements have resulted in a vast scientific literature that has enhanced our comprehension of the interplay between the host, its microbiome and the environment.
Nevertheless, there is still a paucity of research on microbial communities in cephalopods. The literature only includes studies on the gut of common octopus paralarvae by Roura et al. (2017), an analysis of different tissues from Sepia officinalis by Lutz et al. (2019), and a comparative investigation of gut microbial composition in six cephalopod species by Kang et al. (2022).
In light of the growing interest in octopus aquaculture and the mounting social concern for animal welfare, our objective is to investigate the composition of the skin mucus microbiota as a tangible biomarker for evaluating and advancing animal welfare in these organisms. In order to achieve this, two main objectives have been set. The first objective is to describe, for the first time, the skin mucus microbial composition of the core microbiota in the common octopus. This will provide a fundamental understanding of the natural microbial communities present in their skin mucus. Secondly, we aim to ascertain whether captive rearing conditions exert an influence on these communities and, consequently, animal welfare. To this end, we will compare the skin microbiome of octopuses captured from the wild with that of individuals reared in aquaculture. To the best of our knowledge, this is the first study to investigate the composition of the microbiota present in the octopus skin mucus of both wild and farmed populations. These findings will provide crucial insights into the effects of captivity on these interesting organisms.
2 Materials and methods
2.1 Sampling
A total of 20 common octopuses of an average weight of approximately 1.2 ± 0.25 kg were sampled from two different sources: wild and aquaculture environments. The wild octopuses (n = 10, half of each sex) were provided by the San Bartolomé Fishermen’s Guild (Cangas, Galicia), that captured individuals in the Ría of Vigo (42°15′N 8°45′W) in 2022. The farmed octopuses (n = 10) were part of the fifth generation of domestically bred individuals raised and kept at the Pescanova Biomarine Center facilities in O Grove, Spain (42°47′N 8°86′W) in the year 2022. Samples were obtained from 12 m3 square tanks connected to a semi-open water recirculation system, maintaining specific conditions of temperature (15 ± 1°C), salinity (35 ppm), photoperiod (10 h light:14 h dark), and density (15 kg/m3) (Iglesias et al., 2000). These tanks were equipped with cognitive enrichment, incorporating diverse structures.
Skin mucus samples were collected from each specimen using sterile cotton swabs. For this purpose, the animals were previously anaesthetized with 1.5% MgCl2 and 1% ethanol. Sampling was performed by gently swabbing along the surface of the octopus. In order to obtain sufficient volumes of skin mucus for downstream analysis, two cotton swabs were taken from the same individual. The swabs were then cut and placed into individual sterile tubes, which were kept on ice until their arrival to the laboratory, where they were frozen at –80°C until processing.
2.2 DNA extraction and sequencing
Microbial DNA extraction was performed following the MasterPure Complete DNA and RNA Purification Kit (Epicentre, Southampton, Hampshire, UK) and Pathogen Lysis Tubes (QIAGEN, Hilden, Germany) protocols, with some modifications proposed by Boix-Amorós et al. (2016) to optimize DNA isolation. Total genomic DNA was extracted from the cotton swabs by adding 500 µL of lysis buffer to each tube and shaking vigorously. The resulting lysates were then transferred to the Pathogen Lysis Tubes and subjected to mechanical disruption using a TissueLyser II (QIAGEN, Hilden, Germany) at 30 Hz for 5 min. After the disruption step, samples were placed on dry ice for 3 min and incubated in a thermoblock at 65°C for 5 min.
For genomic DNA purification, samples were incubated with 2 µL of proteinase K (50 µg/mL) at 65°C for 15 min. Next, the tubes were placed on ice to stop the reaction, and 2 µL of RNAse A (5 µg/µL) were added to each sample and incubated at 37°C for 30 min. After incubation on ice for 3-5 minutes, proteins were then precipitated by adding 250 µL of MPC protein precipitation reagent, followed by vigorous shaking and centrifugation at 10,000 g for 10 minutes at 4°C. The supernatant was transferred to a clean tube, discarding the pellet. The DNA was precipitated with isopropanol and centrifugated at 4°C for 10 min at 10,000 g. Finally, the pellet containing the purified DNA was resuspended in 30 µL of TE buffer.
Extracted DNA concentration was measured in a Qubit 4.0 Fluorometer (Thermo Fisher Scientific, DE, United States) using the dsDNA High Sensitivity (HS) Assay Kit (Invitrogen, Carlsbad, California, United States). The purity and quality of the samples were assessed using a NanoDrop 2000 Spectrophotometer (Thermo Fisher Scientific, DE, United States). The microbial community was characterized using the 16S rRNA amplicon sequencing technique, targeting the V3-V4 hypervariable regions. Amplicon library preparation and sequencing were performed by an external service (Foundation for the Promotion of Health and Biomedical Research, FISABIO, Valencia, Spain) using standard protocols. Sequencing was performed using a 2×300 bp paired-end protocol on an Illumina MiSeq platform (Illumina, Inc., San Diego, CA, USA). Negative controls were included for both extraction and PCR protocols.
2.3 Bioinformatic analyses
Demultiplexed raw reads (FASTQ files) were subjected to quality control with fastp software (Chen et al., 2018) applying a minimum sequence length of 50 nucleotides (min_length 50). A quality control step was performed for each nucleotide, ensuring that the quality did not fall below 30 (trim_qual_right 30) in a 10 nucleotides window (trim_qual_window 10), using the mean quality score (trim_qual_type mean).
Subsequently, the bioinformatic analysis was conducted following a workflow that includes processing of the reads and taxonomic assignment into ASVs through the QIIME2 v2022.8 pipeline (Bolyen et al., 2019), followed by further statistical analysis using R Statistical Software v4.1.2 (R Core Team, 2020) (Additional File 1: Supplementary Figure S1). Filters for abundance or prevalence were not applied. Primer sequences were trimmed from the quality-checked paired-end reads using the Cutadapt plugin (Martin, 2011) implemented in QIIME2. Next, data were processed with the DADA2 algorithm (Callahan et al., 2016) to denoise the reads (filtering and chimera removal) and assign them to amplicon sequence variants (ASVs). Taxonomic classification of ASVs was performed using a Naïve Bayes classifier pre-trained on the hypervariable regions V3-V4 with reference sequences extracted from the SILVA database (v138) (Quast et al., 2013). Subsequently, sequences assigned to mitochondria and chloroplast, as well as those not assigned at the domain level, were filtered out from the dataset.
2.4 Statistical analyses
The ASV table, taxonomy and metadata were imported into R environment using the Phyloseq package v3.17 (McMurdie and Holmes, 2013). The diversity and abundance of the skin microbiota of the wild and aquaculture samples were assessed estimating the alpha and beta diversity.
To conduct alpha diversity analysis, the abundance data were rarefied to the minimum sequencing depth. Alpha diversity metrics, including observed richness, Chao1 index (Chao, 1987), Shannon index (Shannon, 1948) and Inverse Simpson index (Simpson, 1949), were calculated at ASV level using the estimate_richness function from Phyloseq. Statistical differences between wild and aquaculture groups, as well as possible differences between sexes were assessed using the non-parametric Wilcoxon rank sum test.
Beta diversity was estimated taking into account the compositional nature of microbiome data (Gloor et al., 2017; Quinn et al., 2018). For this purpose, count data were initially subjected to zero-replacement based on the Count Zero Multiplicative (CZM) method in zCompositions package v1.4.0-1 (Palarea-Albaladejo and Martín-Fernández, 2015) using the default parameters (threshold of 0.5 and frac = 0.65). Next, the CZM adjusted absolute abundances were transformed using the centered log-ratio (CLR) method (Aitchison et al., 2000) with the CoDaSeq package v0.99.6 (Gloor et al., 2016). These values were used to obtain an Euclidean distance matrix (Aitchison distances) that was used to perform a principal component analysis (PCA). Between-group comparisons of beta diversity were assessed using PERMANOVA (Anderson, 2017) with 999 permutations, implemented in the adonis2 function of the vegan R package v2.6-4 (Dixon, 2003).
To determine differences in the abundance levels of specific taxa, a differential abundance analysis between wild and aquaculture groups was performed with ALDEx2 R package (Fernandes et al., 2014). The input for this analysis was obtained from the CLR-transformed count data generated with the aldex.clr function using 1,000 Monte Carlo replicates from the Dirichlet distribution. Differences between groups were assessed using Wilcoxon rank sum test through the aldex.ttest function applying False Discovery Rate (FDR) multitest correction at the 5% level. Values above the significance threshold after FDR correction and with an |effect size factor| (median ratio of between- and within-group differences) ≥ 2.0 were considered for discussion. The results were illustrated in heatmaps, using the ComplexHeatmap R package version 2.14.0 (Gu et al., 2016).
2.5 Prediction of functional genes
PICRUSt2 was used to predict potential metabolic functions from 16S rRNA amplicon sequences (Douglas et al., 2020). QIIME2 denoised reads were imported into PICRUSt2 and were compared with sequences from the reference tree. Matches were filtered to exclude those ASVs with Nearest Sequenced Taxon Index (the phylogenetic distance between the ASV and the nearest sequenced reference genome) ≥ 2. Functional data were mapped and annotated at different levels of KEGG (Kyoto Encyclopedia of Genes and Genomes) category pathway (Kanehisa et al., 2016). We then used ALDEx2 to identify differentially abundant KEGG between wild and aquaculture sample groups. Pathways were defined as significantly abundant if they met the criteria of an FDR adjusted p-value ≤ 0.05 in the Wilcoxon rank sum test and an |effect size| ≥ 1.5.
3 Results
After the quality control and pre-processing steps, the final data set corresponding to the 20 skin mucus samples contained 2,283,515 sequences, which were grouped into 6,829 unique ASVs (Additional File 2: Supplementary Table S1). The mean read count was 114,175, ranging from 92,046 (sample A4) to 149,578 counts (sample A8). The rarefaction curves revealed that most of the bacterial taxa present in the samples were detected, indicating that the sequencing depth was enough to capture most of the bacterial diversity (Additional File 1: Supplementary Figure S2).
3.1 Community composition
After taxonomic assignment of ASVs, 33 phyla, 74 classes, 203 orders, 350 families and 765 genera were obtained in wild and aquaculture mucosal skin samples.
Of the 33 phyla identified, 24 were common to both groups; however, the prevalence of some phyla varied significantly between samples (Additional File 1: Supplementary Figure S3). The most dominant phyla were Bacteroidota, which accounted for 49.6% and 44% of the mean total abundance in wild and aquaculture mucosal skin samples, respectively, followed by Pseudomonadota (30.2% and 29.6%), Campylobacterota (6.8% and 10.0%), Verrucomicrobiota (6.9% and 8.9%) and Patescibacteria (2.8% in both groups) (Figures 1A, B). The relative abundances of these phyla were remarkably similar in both sample types.
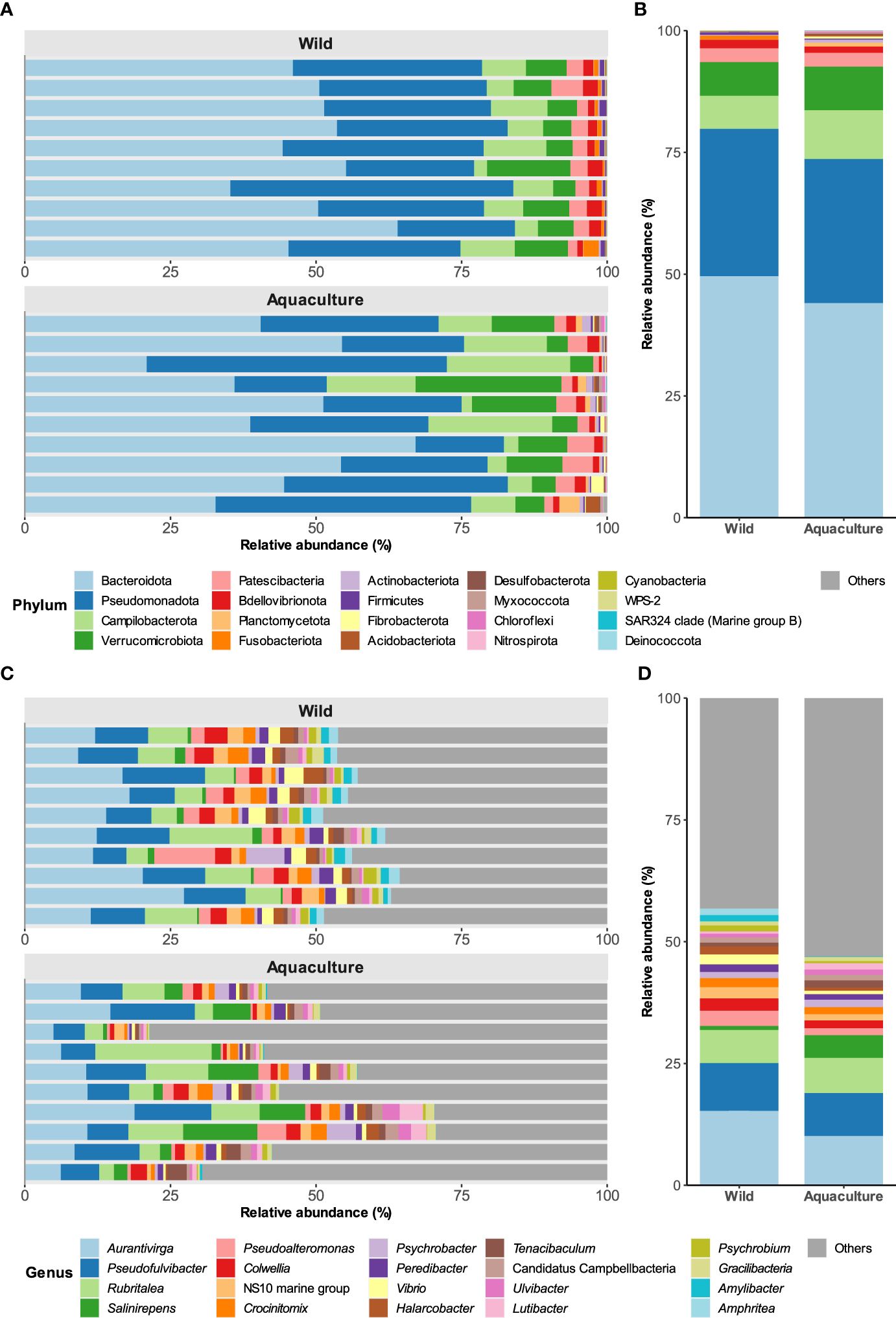
Figure 1. Taxonomic composition of bacterial populations in the skin of wild and aquaculture octopuses. (A) Comparation of the relative abundances of the 20 most prevalent phyla (x-axis) found in the mucosal skin microbiome of both wild and aquaculture octopuses. (B) Mean relative abundances of these phyla across all samples. (C, D) depict the taxonomic composition at the genus level.
At the family level, the most abundant were Flavobacteriaceae, representing over 25% of the mean total relative abundance in both wild and aquaculture octopuses (see Additional File 1: Supplementary Figure S4). Other families with notable abundance were Arcobacteraceae (6.7% and 10%, respectively), Rubritaleaceae (6.8% and 8.4%), Rhodobacteraceae (7.5% and 4.2%), and Saccharospirillaceae, which exhibited a considerable disparity in abundance between the wild (0.48%) and aquaculture (10%) groups.
At the genus level, no significant differences in the abundance of predominant taxa were observed between the two groups (Figures 1C, D). Among these genera, Aurantivirga, Pseudofulvibacter, and Rubritalea proved to be the most abundant, together accounting for approximately 30% of the mean total abundance in wild and aquaculture octopuses. No differences were observed between males and females (data not shown).
3.2 Diversity analysis
Results from alpha diversity at the ASV level revealed significantly higher diversity in wild octopus mucosal skin samples compared to those from aquaculture, as indicated by the Shannon and Inverse Simpson indexes (p ≤ 0.05, Wilcoxon rank sum test), but not by the observed richness and the Chao 1 index (Figure 2A). These differences disappeared when comparisons were made at the genus level (Additional File 1: Supplementary Figure S5A).
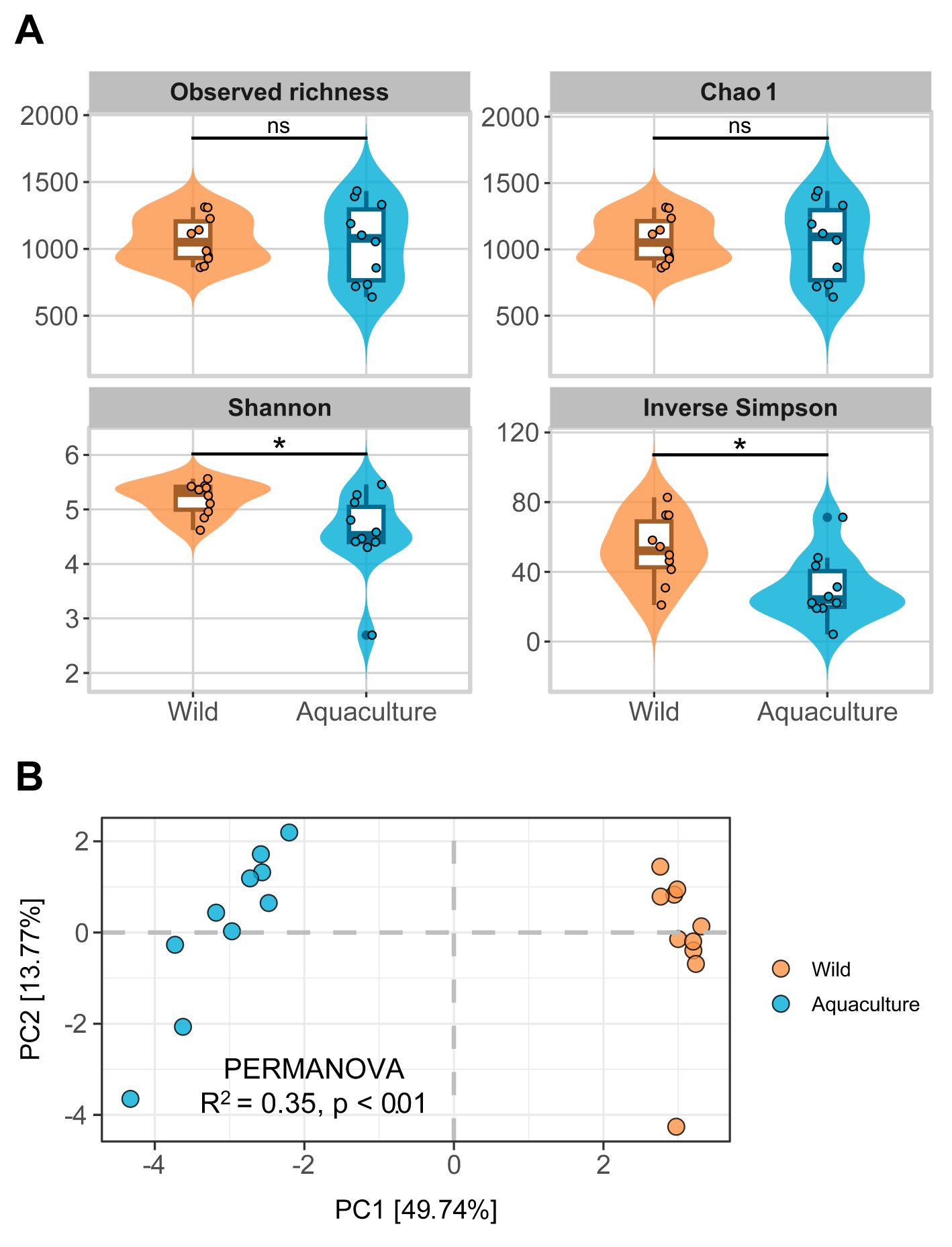
Figure 2. Analysis of diversity. (A) Alpha diversity measures for wild and aquaculture groups are represented by Violin plots that illustrate the alpha diversity indexes analyzed (Observed richness, Chao 1, Shannon and Inverse Simpson) at the ASV level in samples from wild and aquaculture groups. The Wilcoxon-Mann-Whitney test results for group comparisons are marked as ns (not significant) and asterisk (p ≤ 0.05). (B) Beta diversity is analyzed for wild and aquaculture groups. PCA plot displaying Aitchison distances at ASV level. Axes x and y represent the percentage of total variance explained by the first two components, respectively.
Regarding beta diversity, PCA on ASV data normalized according to CLR showed a clear distinction between wild and aquaculture octopuses mucosal skin samples, particularly for PC1, which explained 49.7% of the total variance (Figure 2B). This result was supported by the PERMANOVA test (R2 = 0.35, p ≤ 0.01), indicating significant differences in the microbial communities between the two groups. When the analysis was performed at the genus level, a greater dispersion was observed in the aquaculture samples (Additional File 1: Supplementary Figure S5B). Differences between males and females were not significant, as showed by PERMANOVA (R2 = 0.12, p = 0.239).
3.3 Differential abundance
At the species level, from the 500 taxa identified, 80 presented significant differential abundance between wild and aquaculture octopuses mucosal skin samples. Twenty-six out of these 80 species presented additionally an |effect size | ≥ 2.0 (Figure 3; Additional File 2: Supplementary Table S2), most of them belonging to the class Gammaproteobacteria. These taxa were predominantly enriched in the skin mucus of wild octopuses (19 in wild vs. 7 in aquaculture). Tenacibaculum todarodis, Amphritea ceti and Pseudoalteromonas marina were the top enriched (highest size-effect) species in wild samples, while Flavobacterium frigidarium, Ardenticanaceae sp. and Chryseobacterium balustinum were the top enriched species in aquaculture samples. Species previously reported as potentially pathogenic including members from genus Vibrio (V. cortegadensis, V. gallaecicus and V. tapetis), and other taxa such as Photobacterium swingsii and Lactococcus garvieae, were enriched in the wild group (FDR adjusted p ≤ 0.05, Additional File 2: Supplementary Table S2). At the genus level, the top enriched taxa in wild samples were Profundimonas, Amphritea and Kordia, while aquaculture samples were enriched in an unidentified genus of Moraxellaceae, Portibacter and Chryseobacterium. (Additional File 1: Supplementary Figure S6; Additional File 2: Supplementary Table S3).
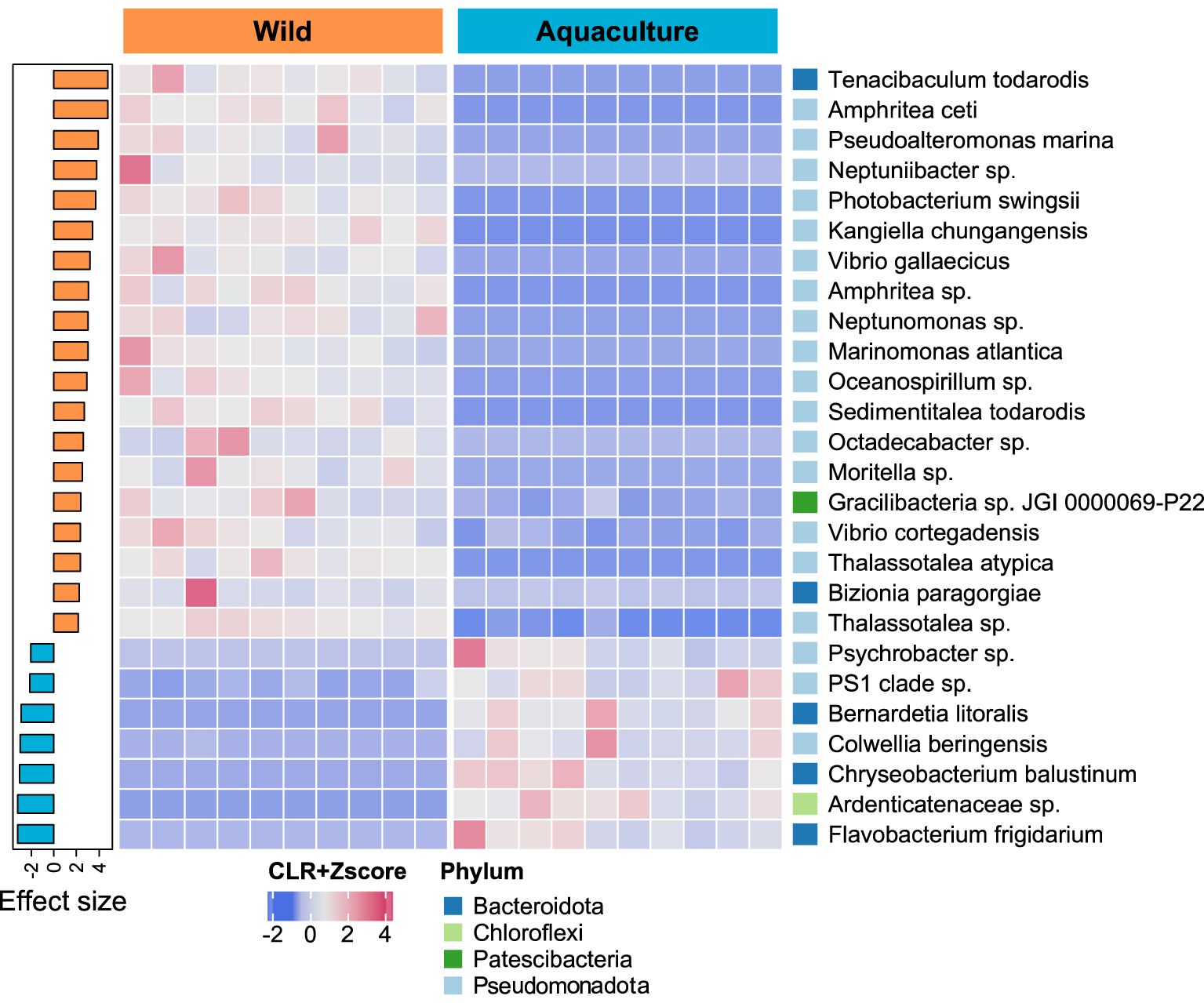
Figure 3. Comparation of abundant species between wild and aquaculture octopuses. The heatmap’s colors represent the scale-transformed relative abundance of 26 bacterial species identified as significantly differentially abundant (FDR adjusted p ≤ 0.05 and |effect size| ≥ 2.0). The sample columns are hierarchically clustered, and the bacterial species are ordered by decreasing effect size, as seen in the sidebar plot on the right. The phyla to which each species belongs are also shown on the right sidebar.
3.4 Functional profiles of skin microbiomes in wild and farmed octopuses
A total of 7,127 KEGG ortholog (KO) genes were predicted, which were annotated and collapsed into 265 KEGG pathways. From these, 28 pathways were differentially abundant between wild and aquaculture individuals when considering FDR adjusted p-values ≤ 0.05 and an |effect size| ≥ 1.5 (Figure 4). At the highest hierarchy level of classification (level 1), most of the functions were enriched in metabolic pathways, both in wild (12/28 pathways) and aquaculture (4/4 pathways) individuals (Additional File 2: Supplementary Table S4). In the wild individuals, an enrichment of functions was observed compared to the aquaculture individuals (24 vs 4 functions), several of them associated with the degradation and metabolism of organic compounds such as nitrotoluene, benzoate and geraniol, as well as the biosynthesis of other molecules such as penicillin, cephalosporin and bile acids. On the other hand, the aquaculture individuals presented a higher abundance of pathways related to caffeine metabolism and the biosynthesis of various terpenoids and polyketides.
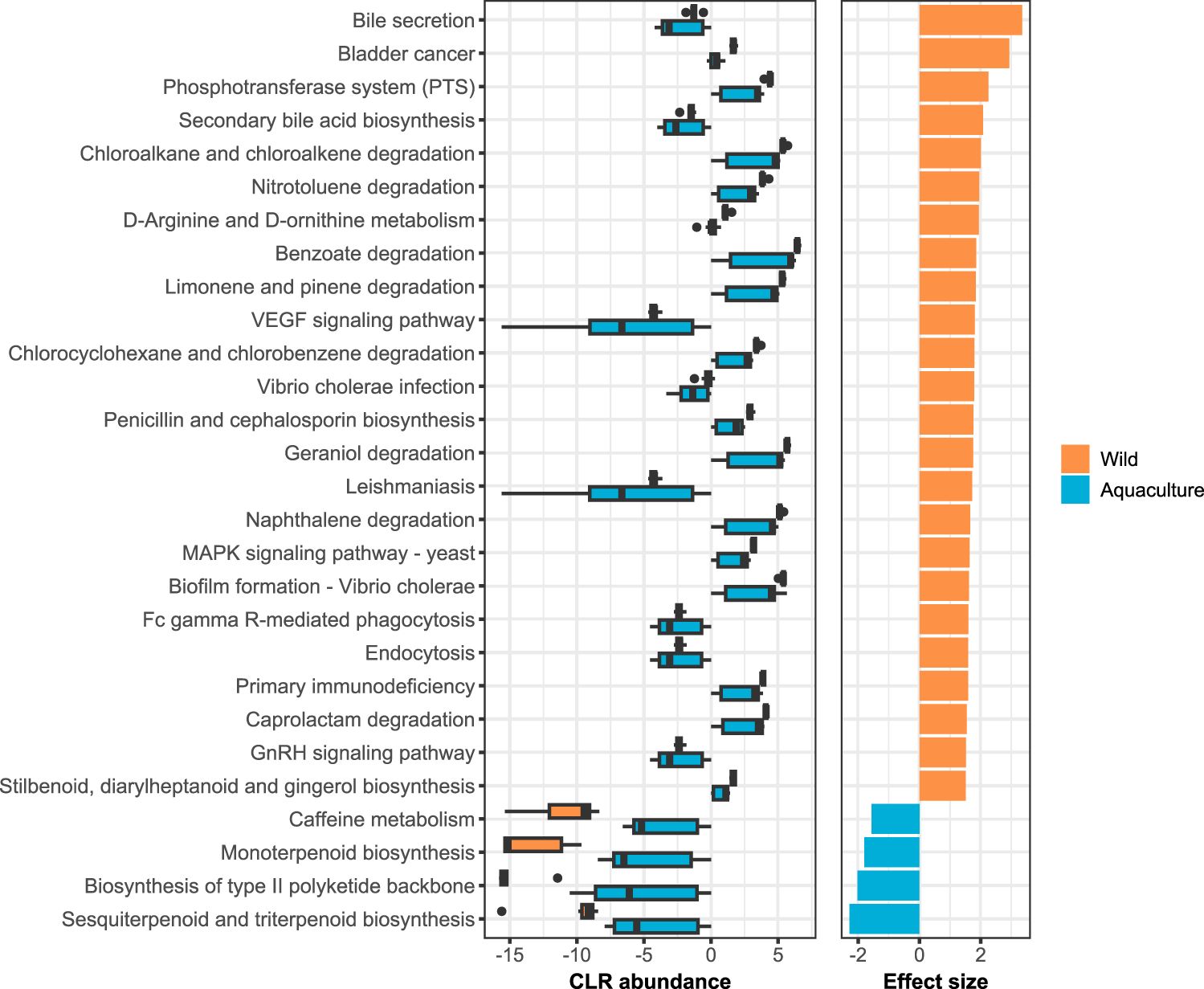
Figure 4. Predicted functional gene profiles. The left panel shows the boxplots of CLR abundances for the 28 PICRUSt2-predicted KEGG pathways identified as differentially abundant between wild and aquaculture groups by ALDEx2 analysis (FDR adjusted p ≤ 0.05 and |effect size| ≥ 1.5). Pathways are ordered by descending effect size, shown in the right panel of the graph.
4 Discussion
In this study, we performed a comparative analysis of skin mucus microbiome between wild and aquaculture Octopus vulgaris. Our results showed that the composition of the microbiota was essentially very similar between the groups of individuals, with differences in beta diversity being mainly attributed to differential abundance. The qualitative analysis revealed a notable distinction in the prevalence of bacteria previously identified as pathogenic, including Vibrio spp., Lactococcus garvieae, and Photobacterium swingsii, in wild individuals compared to those in aquaculture. The former exhibited a higher incidence of these bacteria, while the latter demonstrated a lower abundance, which is likely attributed to the controlled conditions and superior water quality in the hatchery. To our knowledge, this is the first study where the skin microbiome of the common octopus has been characterized and compared in wild and farmed individuals as a biomarker of animal welfare.
The composition analysis demonstrated that the microbial communities comprising the common octopus mucosal skin microbiome were predominantly represented by the phylum Bacteroidota, followed by Pseudomonadota. Furthermore, the genera Aurantivirga, Pseudofulvibacter, and Rubritalea were identified as the predominant taxa. As these taxa were the most abundant in each sample (exceeding 80% for phylum, and ranging from 10-30% for genus), irrespective of their provenance, this taxonomic composition may be regarded as the core microbiota of the mucosal skin of O. vulgaris. Although our current understanding of the microbial communities inhabiting cephalopods remains limited, recent studies have begun to shed light on the core microbiota of these animals using high throughput sequencing but focusing primarily on the gut microbiota. Roura et al. (2017) provided an initial overview of the bacterial communities inhabiting the gastrointestinal tract of O. vulgaris paralarvae by comparing wild with captive-reared wild individuals using 16S rRNA amplicon sequencing (Roura et al., 2017). In their study, they found that the core gut microbiota was composed of the families Flavobacteriaceae, Comamonadaceae, Moraxellaceae and Sphingomonadaceae, which belong to the phyla Bacteroidota and Pseudomonadota, in agreement with our findings. Flavobacteriaceae was also the most abundant family in our study in all samples, followed by Arcobacteraceae and Rubritaleaceae (Additional File 1: Supplementary Figure S4, Additional File 2: Supplementary Table S1). In contrast to our results, they found important differences between wild and captive-reared wild individuals that they attributed to diet. Lutz et al. (2019) identified a highly simplified microbiota in the skin, gills and gastrointestinal tract of Sepia officinalis belonging to Vibrionaceae and Piscirickettsiaceae families, which were at low frequency or absent in our analyzed samples (Lutz et al., 2019). Comparative analysis of six free-living cephalopod species conducted by Kang et al. (2022) revealed that their gut microbiota is composed of distinctive microbes and is strongly associated with the phylogeny of individuals. In their study, they observed that the phyla Tenericutes and Pseudomonadota were the most abundant in all samples, while Mycoplasma and Photobacterium were the core taxa at the genus level, which also differs again from our results. Our findings obtained in octopuses differ significantly from the predominance of Pseudomonadota over other phyla reported in other marine organisms as described in several fish species (Chiarello et al., 2015; Gomez and Primm, 2021) and other aquatic organisms (Sehnal et al., 2021). Fish skin microbiomes typically include Firmicutes and Actinobacteriota, which in our study showed very low abundances (averaging 0.57 − 0.20% and 0.15 − 0.61%, respectively) in the wild and aquaculture groups. Thus, it is clear that the microbial communities differ markedly depending on the tissue analyzed and host genetics.
In general, the alpha diversity was comparable between wild and in aquaculture mucosal skin samples for the four indexes analyzed when the analysis was conducted at the genus level (Additional File 1: Supplementary Figure S5A). A minor discrepancy was identified when analyzing ASVs, whereby wild samples exhibited a higher diversity solely for those indexes that account for taxa evenness and abundance (Shannon and Inverse Simpson indexes). However, the observed significance was lost when these factors were ignored (observed diversity and Chao1 indexes). This indicates that although the overall diversity of microbial communities was comparable, the controlled conditions of aquaculture farms, which are characterized by regulated diets and measures to prevent pathogen proliferation, create a more stable environment. This stability may result in a relatively lower microbial diversity compared to the more variable conditions typically found in the wild. These findings align with those of previous studies examining the microbiomes of aquatic organisms, which have also observed comparable trends between wild and aquaculture individuals (Attramadal et al., 2012; Vadstein et al., 2018; Romero et al., 2022). Previous studies have evidenced a generalized tendency for alpha diversity to decrease under stress or disease conditions (Tarnecki et al., 2019; Legrand et al., 2020). Along these lines, a loss of evenness and diversity following exposure to stressors has been shown in the microbiome of different marine species (Carlson et al., 2015; Narrowe et al., 2015; He et al., 2017; Nie et al., 2017; Bagi et al., 2018; Zha et al., 2018; Zhang et al., 2018). Although this is the general trend, changes in diversity are also conditioned by the presence of specific taxa, so a decrease in alpha diversity is not always observed under detrimental conditions (Ramírez and Romero, 2017; Vasemägi et al., 2017).
Our results from beta diversity statistics revealed significant differences between wild and aquaculture individuals, which may be mainly due to differences in abundance rather than in the number of taxa detected, according to alpha diversity results. These findings could be associated with differences in the environment of wild and aquaculture individuals. Thus, while the wild environment has a heterogeneous nature modulated by different factors, aquaculture specimens were reared in a completely controlled environment, which may influence the mucosal skin microbiome (Lorgen-Ritchie et al., 2021).
To investigate whether captive rearing conditions impact welfare in the context of dysbiosis, we examined the presence and abundance of specific taxa described as potentially pathogenic in aquatic species. Previous studies in cephalopods have reported that most pathogenic bacterial infections are caused by gram-negative bacteria belonging to the genus Vibrio, particularly V. alginolyticus, V. parahaemolyticus, and V. lentus (Fiorito et al., 2015; Farto et al., 2019; Rich et al., 2023). In common octopus, V. lentus was isolated from skin lesions and the gill heart of diseased specimens captured from their natural marine environment, and it was able to induce both skin lesions and mortality in healthy octopuses maintained in laboratory conditions (Farto et al., 2003). In our study, we did not detect the presence of V. lentus but observed an enrichment of three other Vibrio species in wild individuals: V. cortegadensis, V. gallaecicus, and V. tapetis. While V. tapetis is well-known as the etiological agent of brown ring disease, which causes high mortalities in clams (Allam et al., 2002), the pathogenicity of V. cortegadensis and V. gallaecicus (both isolated from clams in Galicia, NW Spain) has not been previously reported (Beaz-Hidalgo et al., 2009; Lasa et al., 2013). We also observed an increased abundance of Photobacterium swingsii and Lactococcus garvieae in wild individuals. These species have been previously associated with a retrobulbar lesion in common octopus (Fichi et al., 2015). P. swingsii is known for its presence in marine environments and can act as an opportunistic pathogen under stress conditions or when the host’s immune system is compromised (Azizan et al., 2022). Similarly, L. garvieae is a well-documented pathogen in aquaculture, primarily affecting fish, and is known for causing septicemia (Vendrell et al., 2006). The presence of presumably pathogenic bacteria in wild octopuses, which were absent or present in lower abundance in aquaculture, indicates the impact of environmental conditions on the microbiome. This underscores the potential of using the microbiome as a biomarker for monitoring the health status of octopuses. By comparing the microbiomes of octopuses from different environments, we can gain a deeper understanding of how rearing conditions affect their health and welfare.
The functional prediction analysis revealed that mucosal skin samples from wild octopuses exhibit higher enrichment in metabolic pathways when compare to mucosal skin samples from cultured octopus. The samples from wild octopuses displayed greater enrichment in pathways involving xenobiotic remediation – consistent with their need to adapt to a more diverse and potentially contaminated environment in nature (Jokhakar et al., 2022). The skin microbiome of wild octopus exhibits greater versatility, enabling the degradation of various organic compounds and production of bioactive molecules that have ecological advantages. In contrast, cultured octopuses possess a narrower range of metabolic capacities, which may be influenced by the controlled environment of their cultivation facilities (Lorgen-Ritchie et al., 2021).
While 16S rRNA metabarcoding has limited accuracy in resolving taxa at the species level and making functional prediction (Johnson et al., 2019), it can serve as initial method to obtain details about the taxonomic composition and potential functions of a microbial community (Ortiz-Estrada et al., 2019). The utilization of this method has aided our investigation of the skin mucus microbiome of the octopus as plausible biomarker for animal welfare. Our investigation demonstrated that the managed conditions in farm facilities function as a proactive approach to thwart dysbiosis, underscoring their capacity to uphold the equilibrium of the microbiome and, subsequently, the comprehensive health of farmed octopuses.
The study of welfare in cephalopods has become a prominent area of research, driven by ethical considerations and the necessity to maintain rigorous standards in cephalopod farming (Mather and Anderson, 2007; Broom, 2010a, b). In recognition of the significance of this matter, the European Union enacted legal standards for invertebrate welfare in 2015. This was achieved by the adoption of general guidelines and the definition of specific welfare standards for their implementation (Fiorito et al., 2015). As previously stated, the lack of stress-related biomarkers in cephalopods presents a significant challenge in maintaining them under aquaculture conditions, where animal welfare is of paramount importance. Additionally, the analysis of gene expression patterns in these species is complicated by the presence of high levels of RNA editing. These gaps have driven the need to explore alternative stress biomarkers beyond the traditional glucocorticoids and gene expression markers. For example, Barragán-Méndez et al. (2019) examined the impact of acute stress on three Octopodidae species (Eledone moschata, E. cirrhosa, and O. vulgaris) captured by bottom trawling. The authors employed a methodology that involved measuring physiological and immune parameters in order to ascertain whether there were differentiated between-species responses, and to determine the rate of recovery of the parameters in question. They observed that all parameters exhibited a return to baseline within the first 24 hours following capture. A recent study by Vizcaíno et al. (2023), identified enzymatic activities in skin mucus and plasma, indicating that biomarkers from skin mucus could be a viable, minimally invasive method for evaluating and enhancing cephalopod welfare. In the context of skin mucus, recent studies have proposed that the microbial patterns found in this tissue may serve as an indicator of animal health. Thus, by comparing the bacterial composition of healthy and stressed/sick animals, a decrease in beneficial bacteria has been demonstrated, accompanied by an increase in opportunistic bacteria, leading to a decrease in the immune capacity and consequently a higher susceptibility to infections in stressed animals (Legrand et al., 2020; Mougin and Joyce, 2023; Rich et al., 2023). This is in line with our results, that indicated a widespread decrease in Gammaproteobacteria among aquaculture octopuses. This group of bacteria belongs to the phylum Pseudomonadota and encompasses various pathogens, including Pseudomonas, Salmonella, Vibrio or Yersinia (Maheshwari and Sankar, 2023). Although members of these genera typically inhabit the skin of many marine organisms, some were enriched (by 2.5-fold, Additional File 2: Supplementary Table S3) in the analyzed samples of wild octopuses.
In light of the aforementioned findings, it is recommended that aquaculture farms continue to invest in technologies and practices that enhance water quality and control environmental variables. This encompasses the implementation of filtration systems, periodic water testing, and the maintenance of optimal stocking densities to curtail stress and the transmission of diseases. The findings of our study can inform the development or refinement of welfare standards and certification processes for octopus aquaculture. Farms that adhere to these standards can be assured that they are providing environments that promote the health and welfare of their animals.
5 Conclusions
The results of our investigation indicate that the core microbiota of the mucosal skin of common octopus is predominantly comprised of the phyla Bacteroidota and, Pseudomonadota, with Aurantivirga, Pseudofulvibacter and Rubritalea representing the most abundant genera. Although there were notable differences in abundance, the microbiota composition and diversity observed in wild and aquaculture octopuses were found to be comparable. Wild individuals exhibited a generalized increase in Gammaproteobacteria, including some potential pathogenic bacterial species that were either absent or present in small amounts in farmed individuals. In conclusion, this study provides crucial insights into the mucosal skin microbiome of the common octopus, which may serve as a potential biomarker of animal health and welfare in this species. Furthermore, the study indicates that the controlled conditions in aquaculture facilities may function as a preventive measure against dysbiosis.
Data availability statement
The datasets generated in this study have been deposited in the NCBI Sequence Read Archive (SRA) repository under BioProject accesion number PRJNA1047176.
Ethics statement
The animal studies were approved by the Bioethics Committee of The Spanish National Research Council (CSIC) under the Animal Experimentation Proyect approval number: ES360570202001/19/EDUC.FORM.07/JRM. The studies were conducted in accordance with the local legislation and institutional requirements (Spanish Authority RD53/2013). This research was conducted in accordance with the European animal directive (2010/63/UE), which is designed to protect experimental animals.
Author contributions
DC-I: Writing – review & editing, Writing – original draft, Visualization, Validation, Methodology, Investigation, Formal analysis, Data curation, Conceptualization. CC-P: Writing – review & editing, Methodology. TS: Writing – review & editing, Validation, Investigation, Formal analysis. PT: Writing – review & editing, Resources. PG-F: Writing – review & editing, Resources. RT: Writing – review & editing, Resources. DC: Writing – review & editing, Resources. MS: Writing – review & editing, Writing – original draft, Visualization, Supervision, Methodology, Formal analysis, Data curation, Conceptualization. JR: Writing – review & editing, Supervision, Resources, Project administration, Methodology, Funding acquisition, Conceptualization.
Funding
The author(s) declare financial support was received for the research, authorship, and/or publication of this article. This work was funded by Centro para el Desarrollo Tecnológico y la Innovación (CDTI-E.P.E) belonging to the Ministerio de Ciencia, Innovación y Universidades del Gobierno de España (Reference: IDI-20210907) and CSIC Special Intramural Projects (Reference: 202340E076) to JR.
Acknowledgments
The authors would like to thank Mr. Ruben Chamorro and Ms. Susana Otero for assistance during sample collection.
Conflict of interest
The authors declare that the research was conducted in the absence of any commercial or financial relationships that could be construed as a potential conflict of interest.
Publisher’s note
All claims expressed in this article are solely those of the authors and do not necessarily represent those of their affiliated organizations, or those of the publisher, the editors and the reviewers. Any product that may be evaluated in this article, or claim that may be made by its manufacturer, is not guaranteed or endorsed by the publisher.
Supplementary material
The Supplementary Material for this article can be found online at: https://www.frontiersin.org/articles/10.3389/fmars.2024.1435217/full#supplementary-material
Supplementary Table S1 | ASV table and taxonomy. Read counts per sample for all ASVs.
Supplementary Table S2 | Results from differential abundance analysis between wild and aquaculture octopuses at species level.
Supplementary Table S3 | Results from differential abundance analysis between wild and aquaculture octopuses at genus level.
Supplementary Table S4 | Differentially abundant KEGG pathways between wild and aquaculture octopuses.
References
Aitchison J., Barceló-Vidal C., Martín-Fernández J. A., Pawlowsky-Glahn V. (2000). Logratio analysis and compositional distance. Math. Geol. 32, 271–275. doi: 10.1023/a:1007529726302
Allam B., Paillard C., Ford S. E. (2002). Pathogenicity of Vibrio tapetis, the etiological agent of brown ring disease in clams. Dis. Aquat. Organ. 48, 221–231. doi: 10.3354/dao048221
Anderson M. J. (2017). “Permutational Multivariate Analysis of Variance (PERMANOVA),” in Wiley StatsRef: Statistics Reference Online. Eds. Balakrishnan N., Colton T., Everitt B., Piegorsch W., Ruggeri F., Teugels J. L. (John Wiley and Sons Ltd, Chichester), 1–15. doi: 10.1002/9781118445112.STAT07841
Attramadal K. J. K., Salvesen I., Xue R., Øie G., Størseth T. R., Vadstein O., et al. (2012). Recirculation as a possible microbial control strategy in production of marine larvae. Aquacult. Eng. 46, 27–39. doi: 10.1016/J.AQUAENG.2011.10.003
Azizan A., Alfaro A. C., Jaramillo D., Venter L., Young T., Frost E., et al. (2022). Pathogenicity and virulence of bacterial strains associated with summer mortality in marine mussels (Perna canaliculus). FEMS Microbiol. Ecol. 98, 1–14. doi: 10.1093/femsec/fiac140
Bagi A., Riiser E. S., Molland H. S., Star B., Haverkamp T. H. A., Sydnes M. O., et al. (2018). Gastrointestinal microbial community changes in Atlantic cod (Gadus morhua) exposed to crude oil. BMC Microbiol. 18, 25. doi: 10.1186/S12866-018-1171-2
Barragán-Méndez C., Sobrino I., Marín-Rincón A., Fernández-Boo S., Costas B., Mancera J. M., et al. (2019). Acute-stress biomarkers in three Octopodidae species after bottom trawling. Front. Physiol. 10. doi: 10.3389/fphys.2019.00784
Beaz-Hidalgo R., Doce A., Pascual J., Toranzo A. E., Romalde J. L. (2009). Vibrio gallaecicus sp. nov., isolated from cultured clams in north-western Spain. Syst. Appl. Microbiol. 32, 111–117. doi: 10.1016/j.syapm.2008.12.002
Berger E. (2011). Aquaculture of Octopus species: present status, problems and perspectives. Plym. Stud. Sci. 4, 384–399. Available at: https://pearl.plymouth.ac.uk/tpss/vol4/iss1/6/.
Birk M. A., Liscovitch-Brauer N., Dominguez M. J., McNeme S., Yue Y., Hoff J. D., et al. (2023). Temperature-dependent RNA editing in octopus extensively recodes the neural proteome. Cell 186, 2544–2555. doi: 10.1016/j.cell.2023.05.004
Boix-Amorós A., Collado M. C., Mira A. (2016). Relationship between milk microbiota, bacterial load, macronutrients, and human cells during lactation. Front. Microbiol. 7. doi: 10.3389/FMICB.2016.00492
Bolyen E., Rideout J. R., Dillon M. R., Bokulich N. A., Abnet C. C., Al-Ghalith G. A., et al. (2019). Reproducible, interactive, scalable and extensible microbiome data science using QIIME 2. Nat. Biotechnol. 37, 852–857. doi: 10.1038/s41587-019-0209-9
Boutin S., Bernatchez L., Audet C., Derome N. (2013). Network analysis highlights complex interactions between pathogen, host and commensal microbiota. PloS One 8, e84772. doi: 10.1371/journal.pone.0084772
Broom D. M. (2010a). Animal welfare: An aspect of care, sustainability, and food quality required by the public. J. Vet. Med. Educ. 37, 83–88. doi: 10.3138/jvme.37.1.83
Broom D. M. (2010b). Cognitive ability and awareness in domestic animals and decisions about obligations to animals. Appl. Anim. Behav. Sci. 126, 1–11. doi: 10.1016/j.applanim.2010.05.001
Callahan B. J., McMurdie P. J., Rosen M. J., Han A. W., Johnson A. J. A., Holmes S. P. (2016). DADA2: high-resolution sample inference from Illumina amplicon data. Nat. Methods 13, 581–583. doi: 10.1038/nmeth.3869
Carlson J. M., Hyde E. R., Petrosino J. F., Manage A. B., Primm T. P. (2015). The host effects of Gambusia affinis with an antibiotic-disrupted microbiome. Comp. Biochem. Physiol. C Toxicol. Pharmacol. 178, 163–168. doi: 10.1016/j.cbpc.2015.10.004
Chao A. (1987). Estimating the population size for capture-recapture data with unequal catchability. Biometrics 43, 783–791. doi: 10.2307/2531532
Chen S., Zhou Y., Chen Y., Gu J. (2018). fastp: an ultra-fast all-in-one FASTQ preprocessor. Bioinformatics 34, i884–i890. doi: 10.1093/bioinformatics/bty560
Chiarello M., Villéger S., Bouvier C., Bettarel Y., Bouvier T. (2015). High diversity of skin-associated bacterial communities of marine fishes is promoted by their high variability among body parts, individuals and species. FEMS Microbiol. Ecol. 91, fiv061. doi: 10.1093/femsec/fiv061
Cockrem J. F. (2013). Individual variation in glucocorticoid stress responses in animals. Gen. Comp. Endocrinol. 181, 45–58. doi: 10.1016/j.ygcen.2012.11.025
Dixon P. (2003). VEGAN, a package of R functions for community ecology. J. Veg Sci. 14, 927–930. doi: 10.1111/j.1654-1103.2003.tb02228.x
Douglas G. M., Maffei V. J., Zaneveld J. R., Yurgel S. N., Brown J. R., Taylor C. M., et al. (2020). PICRUSt2 for prediction of metagenome functions. Nat. Biotechnol. 38, 685–688. doi: 10.1038/s41587-020-0548-6
FAO (2022). The State of World Fisheries and Aquaculture 2022. Towards Blue Transformation (Rome: FAO). doi: 10.4060/cd0683en
Farto R., Armada S. P., Montes M., Guisande J. A., Pérez M. J., Nieto T. P. (2003). Vibrio lentus associated with diseased wild octopus (Octopus vulgaris). J. Invertebr Pathol. 83, 149–156. doi: 10.1016/S0022-2011(03)00067-3
Farto R., Fichi G., Gestal C., Pascual S., Nieto T. P. (2019). “Bacteria-Affecting Cephalopods,” in Handbook of Pathogens and Diseases in Cephalopods (Springer International Publishing, Cham), 127–142. doi: 10.1007/978-3-030-11330-8_8
Fernandes A. D., Reid J. N. N. S., Macklaim J. M., McMurrough T. A., Edgell D. R., Gloor G. B. (2014). Unifying the analysis of high-throughput sequencing datasets: characterizing RNA-seq, 16S rRNA gene sequencing and selective growth experiments by compositional data analysis. Microbiome 2, 15. doi: 10.1186/2049-2618-2-15
Fichi G., Cardeti G., Perrucci S., Vanni A., Cersini A., Lenzi C., et al. (2015). Skin lesion-associated pathogens from Octopus vulgaris: first detection of Photobacterium swingsii, Lactococcus garvieae and betanodavirus. Dis. Aquat Organ. 115, 147–156. doi: 10.3354/dao02877
Fiorito G., Affuso A., Basil J., Cole A., de Girolamo P., D’Angelo L., et al. (2015). Guidelines for the care and welfare of cephalopods in research -a consensus based on an initiative by CephRes, FELASA and the Boyd Group. Lab. Anim. 49, 1–90. doi: 10.1177/0023677215580006
Fodor I., Pirger Z. (2022). From dark to light - an overview of over 70 years of endocrine disruption research on marine mollusks. Front. Endocrinol. 13. doi: 10.3389/fendo.2022.903575
Fraser D., Weary D. M., Pajor E. A., Milligan B. N. (1997). A scientific conception of animal welfare that reflects ethical concerns. Anim. Welfare 6, 187–205. Available at: https://www.wellbeingintlstudiesrepository.org/ethawel/1.
Gloor G. B., Macklaim J. M., Pawlowsky-Glahn V., Egozcue J. J. (2017). Microbiome datasets are compositional: and this is not optional. Front. Microbiol. 8. doi: 10.3389/fmicb.2017.02224
Gloor G. B., Wu J. R., Pawlowsky-Glahn V., Egozcue J. J. (2016). It’s all relative: analyzing microbiome data as compositions. Ann. Epidemiol. 26, 322–329. doi: 10.1016/j.annepidem.2016.03.003
Gomez J. A., Primm T. P. (2021). A slimy business: the future of fish skin microbiome studies. Microb. Ecol. 82, 275–287. doi: 10.1007/s00248-020-01648-w
Gu Z., Eils R., Schlesner M. (2016). Complex heatmaps reveal patterns and correlations in multidimensional genomic data. Bioinformatics 32, 2847–2849. doi: 10.1093/bioinformatics/btw313
He S., Wang Q., Li S., Ran C., Guo X., Zhang Z., et al. (2017). Antibiotic growth promoter olaquindox increases pathogen susceptibility in fish by inducing gut microbiota dysbiosis. Sci. China Life Sci. 60, 1260–1270. doi: 10.1007/s11427-016-9072-6
Iglesias J., Sanchez F. J., Bersano J. F. G., Carrasco J. F., Dhont J., Fuentes L., et al. (2007). Rearing of Octopus vulgaris paralarvae: present status, bottlenecks and trends. Aquaculture. 266, 1–15. doi: 10.1016/j.aquaculture.2007.02.019
Iglesias J., Sánchez F. J., Otero J. J., Moxica C. (2000). Culture of octopus (Octopus vulgaris, Cuvier): present knowledge, problems and perspectives. Cahiers Opt. Méditerranéennes 47, 313–321. Available at: http://om.ciheam.org/om/pdf/c47/00600632.pdf.
Johnson J. S., Spakowicz D. J., Hong B. Y., Petersen L. M., Demkowicz P., Chen L., et al. (2019). Evaluation of 16S rRNA gene sequencing for species and strain-level microbiome analysis. Nat. Commun. 10, 5029. doi: 10.1038/s41467-019-13036-1
Jokhakar P., Godhaniya M., Vaghamshi N., Patel R., Ghelani A., Dudhagara P. (2022). Comparative taxonomic and functional microbiome profiling of anthrospheric river tributary for xenobiotics degradation study. Ecol. Genet. Genom. 25, 100144. doi: 10.1016/J.EGG.2022.100144
Kanehisa M., Sato Y., Kawashima M., Furumichi M., Tanabe M. (2016). KEGG as a reference resource for gene and protein annotation. Nucleic Acids Res. 44, D457–D462. doi: 10.1093/nar/gkv1070
Kang W., Kim P. S., Tak E. J., Sung H., Shin N. R., Hyun D. W., et al. (2022). Host phylogeny, habitat, and diet are main drivers of the cephalopod and mollusk gut microbiome. Anim. Microbiome. 4, 30. doi: 10.1186/s42523-022-00184-x
Kraimi N., Dawkins M., Gebhardt-Henrich S. G., Velge P., Rychlik I., Volf J., et al. (2019). Influence of the microbiota-gut-brain axis on behaviour and welfare in farm animals: a review. Physiol. Behav. 210, 112658. doi: 10.1016/j.physbeh.2019.112658
Lasa A., Diéguez A. L., Romalde J. L. (2013). Vibrio cortegadensis sp. nov., isolated from clams. Antonie van Leeuwenhoek. 105, 335–341. doi: 10.1007/s10482-013-0078-z
Legrand T. P. R. A., Wynne J. W., Weyrich L. S., Oxley A. P. A. (2020). A microbial sea of possibilities: current knowledge and prospects for an improved understanding of the fish microbiome. Rev. Aquac. 12, 1101–1134. doi: 10.1111/raq.12375
Llewellyn M. S., Boutin S., Hoseinifar S. H., Derome N. (2014). Teleost microbiomes: the state of the art in their characterization, manipulation and importance in aquaculture and fisheries. Front. Microbiol. 5. doi: 10.3389/fmicb.2014.00207
Lorgen-Ritchie M., Clarkson M., Chalmers L., Taylor J. F., Migaud H., Martin S. A. (2021). A temporally dynamic gut microbiome in Atlantic salmon during freshwater recirculating aquaculture system (RAS) production and post-seawater transfer. Front. Mar. Sci. 8. doi: 10.3389/fmars.2021.711797
Lorgen-Ritchie M., Uren Webster T., McMurtrie J., Bass D., Tyler C. R., Rowley A., et al. (2023). Microbiomes in the context of developing sustainable intensified aquaculture. Front. Microbiol. 14. doi: 10.3389/fmicb.2023.1200997
Lutz H. L., Ramírez-Puebla S. T., Abbo L., Durand A., Schlundt C., Gottel N. R., et al. (2019). A simple microbiome in the European common cuttlefish, Sepia officinalis. mSystems. 4, e177–e119. doi: 10.1128/mSystems.00177-19
Maheshwari P., Sankar P. M. (2023). “Culture-independent and culture-dependent approaches in symbiont analysis: in Proteobacteria,” in Microbial Symbionts: Functions and Molecular Interactions on Host. (Academic Press, San Diego, CA), 743–763. doi: 10.1016/B978-0-323-99334-0.00018-9
Martin M. (2011). Cutadapt removes adapter sequences from high-throughput sequencing reads. EMBnet.journal 17, 10–12. doi: 10.14806/ej.17.1.200
Mather J. A., Anderson R. C. (2007). Ethics and invertebrates: a cephalopod perspective. Dis. Aquat. Organ. 75, 119–129. doi: 10.3354/dao075119
McMurdie P. J., Holmes S. (2013). phyloseq: an R package for reproducible interactive analysis and graphics of microbiome census data. PloS One 8, e61217. doi: 10.1111/raq.12745
Minniti G., Hagen L. H., Porcellato D., Jørgensen S. M., Pope P. B., Vaaje-Kolstad G. (2017). The skin-mucus microbial community of farmed Atlantic salmon (Salmo salar). Front. Microbiol. 8. doi: 10.3389/fmicb.2017.02043
Mougin J., Joyce A. (2023). Fish disease prevention via microbial dysbiosis-associated biomarkers in aquaculture. Rev. Aquac. 15, 579–594. doi: 10.1111/raq.12745
Narrowe A. B., Albuthi-Lantz M., Smith E. P., Bower K. J., Roane T. M., Vajda A. M., et al. (2015). Perturbation and restoration of the fathead minnow gut microbiome after low-level triclosan exposure. Microbiome 3, 6. doi: 10.1186/s40168-015-0069-6
Navarro J. C., Villanueva R. (2000). Lipid and fatty acid composition of early stages of cephalopods: an approach to their lipid requirements. Aquaculture. 183, 161–177. doi: 10.1016/S0044-8486(99)00290-2
Navarro J. C., Villanueva R. (2003). The fatty acid composition of Octopus vulgaris paralarvae reared with live and inert food: deviation from their natural fatty acid profile. Aquaculture. 219, 613–631. doi: 10.1016/S0044-8486(02)00311-3
Nie L., Zhou Q., Qiao Y., Chen J. (2017). Interplay between the gut microbiota and immune responses of ayu (Plecoglossus altivelis) during Vibrio Anguillarum infection. Fish Shellfish Immunol. 68, 479–487. doi: 10.1016/j.fsi.2017.07.054
Ortiz-Estrada Á.M., Gollas-Galván T., Martínez-Córdova L. R., Martínez-Porchas M. (2019). Predictive functional profiles using metagenomic 16S rRNA data: a novel approach to understanding the microbial ecology of aquaculture systems. Rev. Aquacult. 11, 234–245. doi: 10.1111/raq.12237
Palarea-Albaladejo J., Martín-Fernández J. A. (2015). ZCompositions – R package for multivariate imputation of left-censored data under a compositional approach. Chemom. Intell. Lab. Syst. 143, 85–96. doi: 10.1016/j.chemolab.2015.02.019
Quast C., Pruesse E., Yilmaz P., Gerken J., Schweer T., Yarza P., et al. (2013). The SILVA ribosomal RNA gene database project: improved data processing and web-based tools. Nucleic Acids Res. 41, D590–D596. doi: 10.1093/nar/gks1219
Quinn T. P., Erb I., Richardson M. F., Crowley T. M. (2018). Understanding sequencing data as compositions: an outlook and review. Bioinformatics 34, 2870–2878. doi: 10.1093/bioinformatics/bty175
R Core Team (2020). R: A language and environment for statistical computing (Vienna: R Foundation for Statistical Computing).
Ramírez C., Romero J. (2017). The microbiome of Seriola lalandi of wild and aquaculture origin reveals differences in composition and potential function. Front. Microbiol. 8. doi: 10.3389/fmicb.2017.01844
Reverter M., Tapissier-Bontemps N., Lecchini D., Banaigs B., Sasal P. (2018). Biological and ecological roles of external fish mucus: a review. Fishes 3, 41. doi: 10.3390/fishes3040041
Rich A. F., Denk D., Sangster C. R., Stidworthy M. F. (2023). A retrospective study of pathologic findings in cephalopods (extant subclasses: Coleoidea and Nautiloidea) under laboratory and aquarium management. Vet. Pathol. 60(5):578–598. doi: 10.1177/03009858231186306
Romero J., Díaz O., Miranda C. D., Rojas R. (2022). Red cusk-eel (Genypterus chilensis) gut microbiota description of wild and aquaculture specimens. Microorganisms. 10, 105. doi: 10.3390/microorganisms10010105
Roura Á., Doyle S. R., Nande M., Strugnell J. M. (2017). You are what you eat: a genomic analysis of the gut microbiome of captive and wild Octopus vulgaris paralarvae and their zooplankton prey. Front. Physiol. 8. doi: 10.3389/fphys.2017.00362
Sehnal L., Brammer-Robbins E., Wormington A. M., Blaha L., Bisesi J., Larkin I., et al. (2021). Microbiome composition and function in aquatic vertebrates: small organisms making big impacts on aquatic animal health. Front. Microbiol. 12. doi: 10.3389/fmicb.2021.567408
Shannon C. E. (1948). A mathematical theory of communication. Bell Syst. Tech. J. 27, 379–423. doi: 10.1002/j.1538-7305.1948.tb01338.x
Tarnecki A. M., Brennan N. P., Schloesser R. W., Rhody N. R. (2019). Shifts in the skin-associated microbiota of hatchery-reared common snook Centropomus undecimalis during acclimation to the wild. Microb. Ecol. 77, 770–781. doi: 10.1007/s00248-018-1252-7
Tur R., Rodrigues P. M., Almansa E., Lago M. J., García P., Pérez E. (2020). Procedimiento para el cultivo de paralarvas del pulpo común Octopus vulgaris. Patent ES 2 714 930 B2 (Spain: Oficina Española de Patentes y Marcas).
Uriarte I., Astorga M., Navarro J. C., Viana M. T., Rosas C., Molinet C., et al. (2019). Early life stage bottlenecks of carnivorous molluscs under captivity: a challenge for their farming and contribution to seafood production. Rev. Aquac. 11, 431–457. doi: 10.1111/raq.12240
Uriarte I., Iglesias J., Domingues P., Rosas C., Viana M. T., Navarro J. C., et al. (2011). Current status and bottle neck of octopod aquaculture: the case of American species. J. World Aquacult. Soc. 42, 735–752. doi: 10.1111/j.1749-7345.2011.00524.x
Vadstein O., Attramadal K. J. K., Bakke I., Olsen Y. (2018). K-selection as microbial community management strategy: a method for improved viability of larvae in aquaculture. Front. Microbiol. 9. doi: 10.3389/fmicb.2018.02730
Vasemägi A., Visse M., Kisand V. (2017). Effect of environmental factors and an emerging parasitic disease on gut microbiome of wild salmonid fish. mSphere 2, e00418–e00417. doi: 10.1128/msphere.00418-17
Vaz-Pires P., Seixas P., Barbosa A. (2004). Aquaculture potential of the common octopus (Octopus vulgaris Cuvier 1797): a review. Aquaculture 238, 221–238. doi: 10.1016/j.aquaculture.2004.05.018
Vendrell D., Balcázar J. L., Ruiz-Zarzuela I., de Blas I., Gironés O., Múzquiz J. L. (2006). Lactococcus garvieae in fish: a review. Comp. Immunol. Microbiol. Infect. Dis. 29, 177–198. doi: 10.1016/j.cimid.2006.06.003
Vidal E. A. G., Villanueva R., Andrade J. P., Gleadall I. G., Iglesias J., Koueta N., et al. (2014). Cephalopod culture: current status of main biological models and research priorities. Adv. Mar. Biol. 67, 1–98. doi: 10.1016/B978-0-12-800287-2.00001-9
Vizcaíno R., Felt S. A., Guardiola F. A., Prado-Alvarez M., MaChado M., Costas B., et al. (2023). Functional and molecular immune responses in Octopus vulgaris skin mucus and haemolymph under stressful conditions. Aquac. Rep. 29, 101484. doi: 10.1016/j.aqrep.2023.101484
Wang J., Li G., Wu M. (2023). Cephalopod RNA recoding: linking protein plasticity to thermal adaptation and catalyzing beyond-species protein characterization. Signal Transduction Target Ther. 8, 387. doi: 10.1038/s41392-023-01632-z
Xavier J. C., Allcock A. L., Cherel Y., Lipinski M. R., Pierce G. J., Rodhouse P. G. K., et al. (2015). Future challenges in cephalopod research. J. Mar. Biol. Assoc. 95, 999–1015. doi: 10.1017/S0025315414000782
Zha Y., Eiler A., Johansson F., Svanbäck R. (2018). Effects of predation stress and food ration on perch gut microbiota. Microbiome 6, 28. doi: 10.1186/s40168-018-0400-0
Keywords: aquaculture, biomarker, common octopus, domestication, dysbiosis, metagenomics, microbiome, welfare
Citation: Costas-Imbernón D, Costas-Prado C, Sequeiro T, Touriñán P, García-Fernández P, Tur R, Chavarrías D, Saura M and Rotllant J (2024) The skin microbiome as a new potential biomarker in the domestication and health status of Octopus vulgaris. Front. Mar. Sci. 11:1435217. doi: 10.3389/fmars.2024.1435217
Received: 19 May 2024; Accepted: 05 August 2024;
Published: 23 August 2024.
Edited by:
Amalia Pérez-Jiménez, University of Granada, SpainReviewed by:
Maria Del Carmen Vargas Garcia, University of Almeria, SpainMaría Concepción De La Cruz-Leyva, Universidad Juárez Autónoma de Tabasco, Mexico
Copyright © 2024 Costas-Imbernón, Costas-Prado, Sequeiro, Touriñán, García-Fernández, Tur, Chavarrías, Saura and Rotllant. This is an open-access article distributed under the terms of the Creative Commons Attribution License (CC BY). The use, distribution or reproduction in other forums is permitted, provided the original author(s) and the copyright owner(s) are credited and that the original publication in this journal is cited, in accordance with accepted academic practice. No use, distribution or reproduction is permitted which does not comply with these terms.
*Correspondence: Daniel Costas-Imbernón, ZGNvc3Rhc0BpaW0uY3NpYy5lcw==; Josep Rotllant, cm90bGxhbnRAaWltLmNzaWMuZXM=