- 1Department of Marine Remote Sensing Technology, National Marine Environmental Monitoring Center, Dalian, China
- 2College of Environmental Science and Engineering, Dalian Maritime University, Dalian, China
The CIE hue angle has significant potential in marine ecology and environment monitoring using remote sensing methods. It is calculated from the remote sensing reflectance (Rrs) of all visible bands, which serves as a comprehensive indicator of seawater radiance information and represents one of the key quantitative parameters for assessing seawater color. In this study, a remote sensing retrieval model of total suspended matter concentration (TSM) was developed using the in situ measured data acquired in the coastal waters of Qinhuangdao from 2013 to 2021. This model, based on the CIE hue angle, achieved performance metrics of R2 = 0.68, MAPE=24.47%, and RMSE=2.72 mg/L, demonstrating better accuracy than traditional empirical models that utilize fewer bands. Based on this model, the monthly and interannual TSM in the coastal waters of Qinhuangdao from 2003 to 2023 were retrieved. Influenced by natural factors, such as the monsoons, the TSM in the coastal waters of Qinhuangdao generally exhibited a trend of first decreasing and then increasing from January to December. Except for certain anomalous years (2007, 2008, 2017, etc.), the overall variation process of TSM from 2003 to 2023 showed an initial increase followed by a decrease, closely related to the pollution reduction management requirements implemented by the Chinese government in different periods. The findings of this paper demonstrate that the coastal waters of Qinhuangdao have become clearer, and the ecological environment has been effectively improved as a result of the Chinese government’s vigorous promotion of ecological civilization construction, adhering to the development concept that ‘lucid waters and lush mountains are invaluable assets’, and by introducing the action plan for the comprehensive treatment of pollution in the Bohai Sea.
1 Introduction
Suspended matter serves as an essential carrier for pollutants in water, including nutrients and heavy metals, which significantly impacts water transparency, the underwater light field, and primary productivity (Shi et al., 2015). Consequently, suspended matter has become an important indicator for water quality monitoring and assessment. In recent years, remote sensing technology has emerged as a pivotal means for monitoring the marine ecological environment, and the types and accuracy of these monitoring products have been continuously improved (Jiang et al., 2019). Remote sensing monitoring of TSM has been in practice for many years, and the technology is relatively mature. However, the varying optical properties of waters across different seas result in the retrieval model exhibits distinct regional characteristics.
At present, the remote sensing retrieval methods of TSM mainly include empirical, semi-analytical, and analytical methods. The empirical method is one of the most widely used retrieval methods. It establishes a statistical relationship based on a large amount of measured data between TSM and Rrs, thus enabling remote sensing retrieval of TSM. Empirical methods include single-band models, band ratio models, and multiple regression models. Single-band models typically employ red or near-infrared (NIR) bands as sensitive bands, requiring high accuracy of satellite data. It is primarily used to retrieve TSM in turbid waters along coastal and estuarine areas using ocean color satellite imagery (Miller and McKee, 2004; Binding et al., 2005; Wang et al., 2012a). The band ratio model and multiple-band model are widely used because they can partly eliminate or reduce the effects of particle size and light conditions on the TSM retrieval model. Furthermore, they can reduce the impact of satellite image pre-processing, such as atmospheric correction, on the retrieval results, thereby effectively improving accuracy (Tassan, 1994; Tang et al., 2004; Zhan et al., 2019; Lei et al., 2020; Patel et al., 2022). The semi-analytical method is based on the principle of radiative transfer, which correlates the inherent and apparent optical properties of water. It establishes a statistical relationship between the backscattering coefficient of inherent optical properties and TSM, offering a certain physical basis and high retrieval accuracy. However, this method requires a large amount of measured optical data for support, which makes it difficult to be widely applied (Lee et al., 2002; Sun et al., 2013; Kong et al., 2015; Zhang et al., 2018). Finally, the analytical method uses radiative transfer equations to describe the relationship between the water spectrum and the concentrations of ocean color components. This method employs parameters with clear physical significance that are not subject to temporal and geographical constraints. However, in practical scenarios, the variability of water components and the complexity of the radiative transfer processes within the equations greatly limit the analytical method’s practical application (Wang et al., 2012b).
In addition to the above conventional retrieval methods, researchers have adopted the color space created by the Commission Internationale de l’éclairage (CIE) in 1931 with the continuous development of optical technology and have developed many new algorithms for environmental monitoring and assessment of water bodies (C.I.E, 1932). Wang et al., investigated the relationship between the Forel-Ule Index (FUI) and the water quality of inland waters (Wang et al., 2014, 2018). They further assessed the trophic states of global inland waters using the FUI, achieving high overall accuracy. Subsequently, the trophic status of additional inland and coastal waters was evaluated using the FUI (Li et al., 2016; Chen et al., 2020; Li et al., 2021; Zhou et al., 2021). The CIE xy chromaticity coordinates and CIE hue angle were used to identify algal blooms and reveal global coastal and lake bloom trends over the past decade (Hou et al., 2022; Liu et al., 2022; Dai et al., 2023). In addition, the FUI and CIE hue angle have also performed well in the retrieval of ocean color constituents, exhibiting a significant correlation with chlorophyll a concentration (Chla), TSM, CDOM, and Secchi disk depth in the studied waters (Garaba et al., 2014a, b; Wang et al., 2020a; Garaba et al., 2015; Pitarch et al., 2019; Wang et al., 2019; Woźniak and Meler, 2020; Abegaz et al., 2023; Burket et al., 2023; Ma et al., 2023).
Qinhuangdao, a famous coastal tourism destination in northern China, has seen widespread concern over its nearshore water quality. A series of studies have been conducted on ecological disasters in coastal waters of Qinhuangdao, such as brown tide, green tide, red tide (Han et al., 2019; Zhang et al., 2021; Han et al., 2022; Zhou et al., 2023; Wang et al., 2023a). Remote sensing research has mainly focused on monitoring red tides, aerosol optical properties and greenhouse gas (Xu et al., 2015; Chen et al., 2024; Wang et al., 2020b; Mustafa et al., 2021). There are relatively few reports on remote sensing for water quality retrieval, which mainly pertain to Chla and suspended particulate matter concentration (SPM) only in Chinese literature (Wang et al., 2022; Wang et al., 2023b). However, due to the complexity of TSM composition in the coastal waters of Qinhuangdao, no better TSM retrieval model has been developed for MODIS data. In this study, using 257 in situ data collected from the coastal waters of Qinhuangdao from 2013 to 2021, we aim to propose a novel TSM retrieval model based on the CIE hue angle. Afterward, the proposed model is compared with traditional model to demonstrate its superior accuracy. Finally, the hue angle-based retrieval model is applied to analyze the spatiotemporal characteristics of TSM in this sea area, using Aqua MODIS images from 2003 to 2023. The findings of this study can provide technical support for the management of marine ecology and environment.
2 Materials and methods
2.1 Data source and data pre-processing
2.1.1 In situ data
From 2013 to 2021, 257 in situ Rrs, TSM, and Chla were collected in the coastal waters of Qinhuangdao, China, all were used for model development, and 39 were used for validation (Figure 1; Table 1). Rrs was determined by the above-water measuring approach using a spectrometer from ASD company, TSM was measured by the weighing method, and Chla was determined by the fluorescence method.
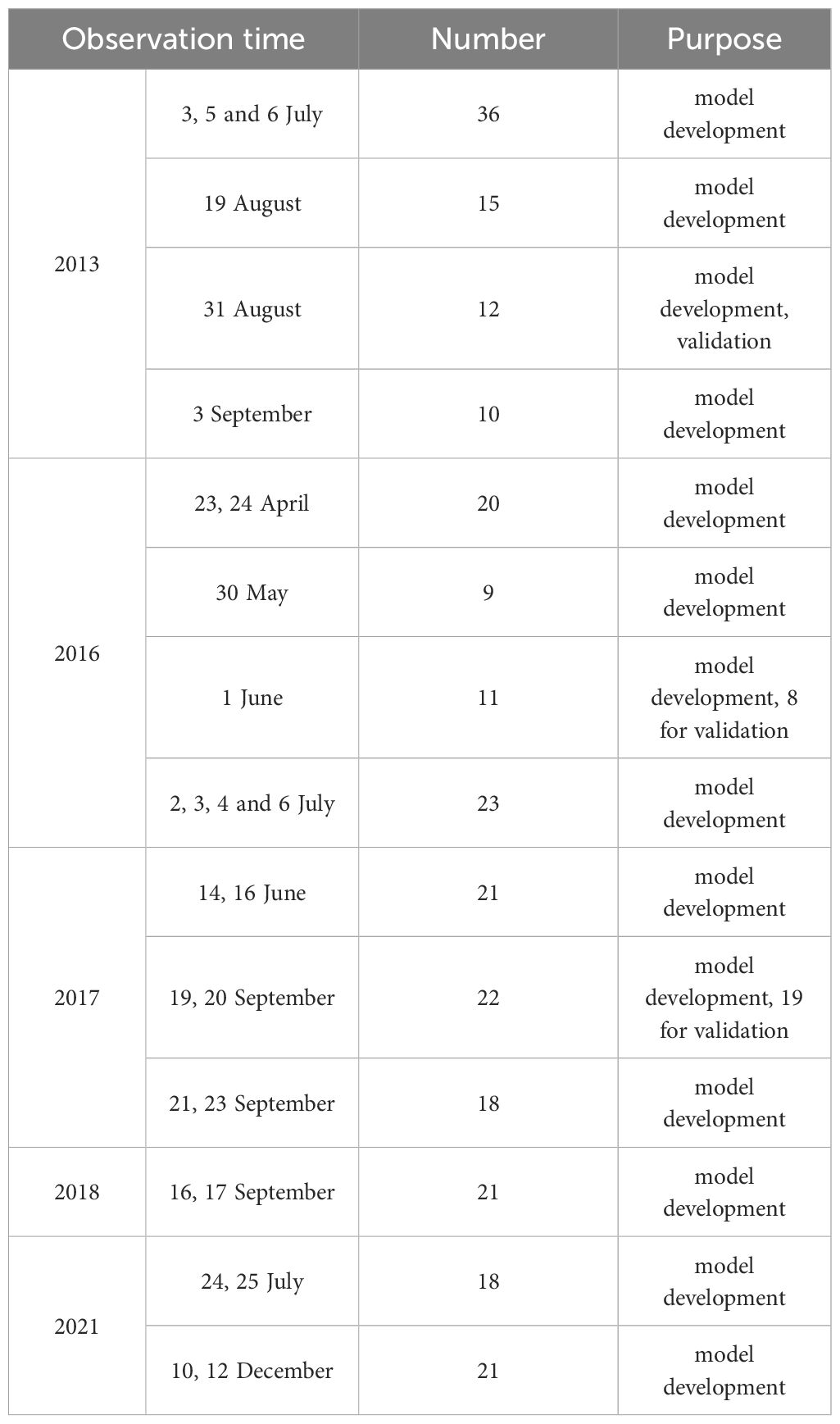
Table 1 Statistics of the in situ data collected in the coastal waters of Qinhuangdao from 2013 to 2021.
The in situ hyperspectral Rrs(λ) were used to calculate the equivalent multispectral Rrs(λi) by the MODIS spectral response function, which can be expressed as Equation 1:
where Fs(λ) represents the mean solar radiative flux at the top of the atmosphere; f(λi) represents the spectral response function at λi.
2.1.2 Satellite data
Aqua MODIS satellite data were downloaded from NASA’s open access Earth science data collections (https://www.earthdata.nasa.gov/). The daily Level 2 Aqua MODIS data for 31 August 2013, 1 June 2016, 19 and 20 September 2017 were used to validate the accuracy of TSM derived from Aqua MODIS data, and the monthly and annual Level 3 data from 2003 to 2023 were used to characterize the spatiotemporal variation of TSM in this study.
2.1.3 Matchup of satellite and in situ data
To validate the accuracy of the TSM retrieval model, matches between Aqua MODIS and in situ data were only included if the time interval between the in situ observations and the satellite overpass was less than 3 hours. Since the quality of satellite data is significantly affected by weather factors, such as clouds, this resulted in fewer valid satellite data entries. As a result, only 39 matches of in situ TSM and satellite-derived TSM were obtained from all the collected samples.
2.2 Calculation method of CIE hue angle
The CIE hue angle is calculated based on the CIE-XYZ standard colorimetry system, which defines the three tristimulus values X, Y, and Z, and was developed by the Commission Internationale de l’éclairage (C.I.E, 1932). For the in situ hyperspectral Rrs(λ), X, Y, and Z values can be calculated as Equations 2–5:
where S(λ) is the relative spectral energy distribution of the irradiation light source; , , and are the CIE color matching functions, all of which are constants; K is the adjustment factor.
For multispectral Rrs(λi), X, Y, and Z were calculated from Aqua MODIS data for ten visible light bands at 412, 443, 469, 488, 531, 547, 555, 645, 667, and 678 nm using a linear intercept method as Equations 6–8 (Wang et al., 2023c; van der Woerd and Wernand, 2015).
where the number of bands n of the satellite sensor is 10, xi, yi, and zi are the band linear summation coefficients, which are constants proposed by Wang et al. (Wang et al., 2023c).
The normalized chromaticity coordinates x and y were calculated from CIE tristimulus X, Y, and Z as Equations 9, 10:
Finally, using the bivariate arctangent function (arctan2) and the following Equation 11, the hue angle can be found from the chromaticity coordinates (x, y) (Wang et al., 2018):
Notably, due to the band dispersion and positional settings of multispectral satellite sensors, the true colors as represented by the hyperspectral data are different from those seen by the sensors [van der Woerd and Wernand, 2015]. Compared to the hyperspectral hue angle (αhyper), the multispectral hue angle (αmulti) exhibits a systematic bias, and Wang et al. have proposed a correction equation to adjust for this deviation (Δα) (Wang et al., 2023c).
2.3 Evaluation method
In order to evaluate the retrieval model and determine the best one, three main evaluation indexes (Equations 12–14) are selected: the coefficient of determination (R2), the mean absolute percentage error (MAPE), and the root mean square error (RMSE).
where is the i-th model retrieval value; indicates the i-th in situ value; denotes the mean of the in situ values; n is the number of samples.
3 Results
3.1 Retrieval model construction and validation
3.1.1 Applicability of classical models
The classic empirical models for remote sensing retrieval of TSM, including single-band, band ratio, and multi-band models, were selected from the previous studies to assess their applicability in the coastal waters of Qinhuangdao. The assessment results are shown in Table 2. The accuracy evaluation indexes of the single-band model are poor, rendering it inapplicable to this study area. Among the band ratio models, the power exponential model is poorly adapted, while the linear model, using 678 and 488 nm as the sensitive bands, shows higher accuracy and better applicability in the study area. The multi-band model also shows good applicability but is slightly less effective than the linear band ratio model. For highly turbid waters, researchers typically use the NIR band as a sensitive band for retrieving TSM. However, because the coastal waters of Qinhuangdao have relatively clear water and low TSM, the NIR band-associated retrieval model is not considered in this study.
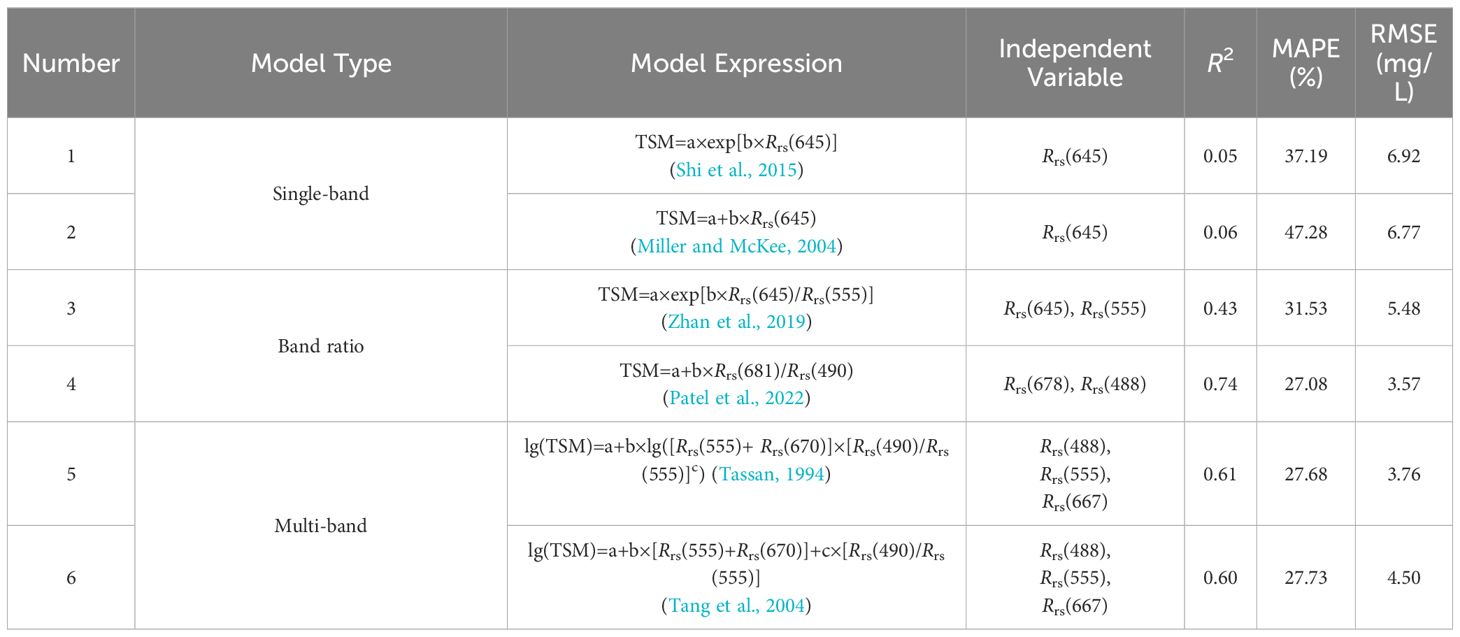
Table 2 Accuracy evaluation of classical empirical model applied to measured data in the coastal waters of Qinhuangdao.
3.1.2 Construction of the retrieval model in the research area
Based on the applicability analysis in the previous section, the band ratio and multi-band models are more applicable to the coastal waters of Qinhuangdao than other classical TSM retrieval models. Therefore, the band ratio, multi-band combinations, and CIE hue angle (α) are selected as the primary sensitivity factors for modeling and comparative analysis. The accuracy evaluation results for each fitting model (M1~M23) are shown in Table 3. It is found that the highest R2 value of the models in linear coordinates of TSM reaches 0.80, while in logarithmic coordinates, it is only 0.68. This result is attributed to the significant increase in R2 at high TSM in linear coordinates and should be comprehensively evaluated with MAPE and RMSE. In the band ratio model, using Rrs(645)/Rrs(488) as the independent variable, the fitting model under the linear TSM coordinate is optimal (M2), with an R2 of 0.77, a MAPE of 26.35%, and an RMSE of 3.34 mg/L. Among the multi-band models, the binary linear model (M22) shows superior performance in fitting TSM in logarithmic coordinates, using Rrs(488)/Rrs(555) and Rrs(645)/Rrs(555) as independent variables, with an R2 of 0.66, a MAPE of 24.99%, and an RMSE of 3.44 mg/L. However, under the logarithmic TSM coordinate, the fifth-order polynomial model (M23), with α/100 as the independent variable, shows higher model accuracy than both the band ratio and the multi-band models, with an R2 of 0.68, a MAPE of 24.47%, and an RMSE of 2.72 mg/L. The scatterplots of TSM and α/100 are shown in Figure 2. Therefore, the model based on the CIE hue angle (α) has been established as the optimal TSM retrieval model for the research area.
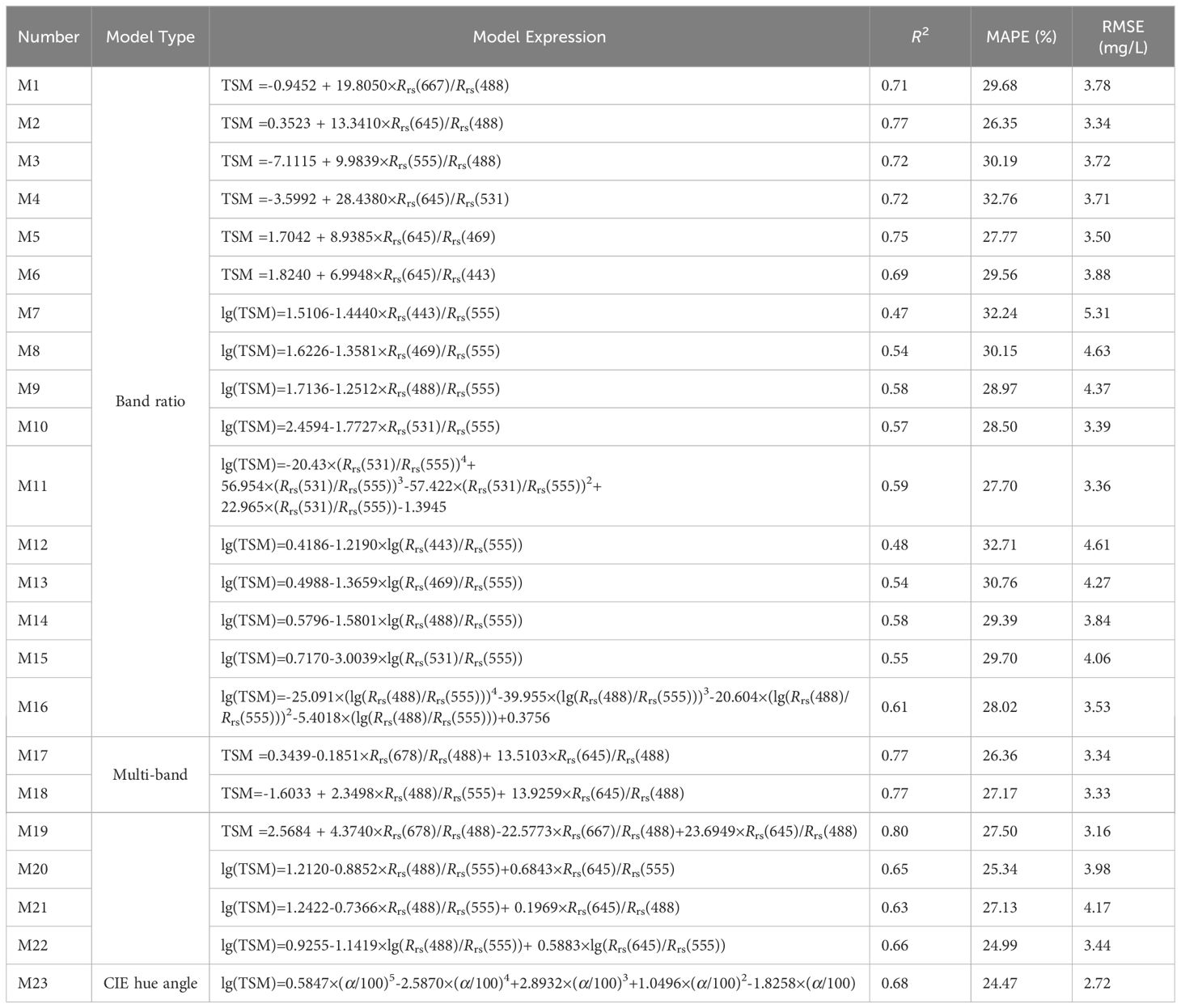
Table 3 Accuracy evaluation of remote sensing retrieval models of TSM in the Qinhuangdao coastal waters.
3.1.3 Validation of the retrieval model with satellite-in situ matchup data
The Scatterplots of in situ TSM and satellite retrievals using the model based on the CIE hue angle are shown in Figure 3. The retrievals closely follow the 1:1 line, and the slope and intercept of the fitted regression line are 0.9246 and 0.7259, respectively, with an R2 of 0.69, a MAPE of 15.99%, and an RMSE of 1.49 mg/L. Additionally, the range of TSM used for validation, 3-15 mg/L, essentially covers the primary variation interval of TSM in the coastal waters of Qinhuangdao. This implies that the retrieval model showed no issues with sensitivity across different concentrations samples.
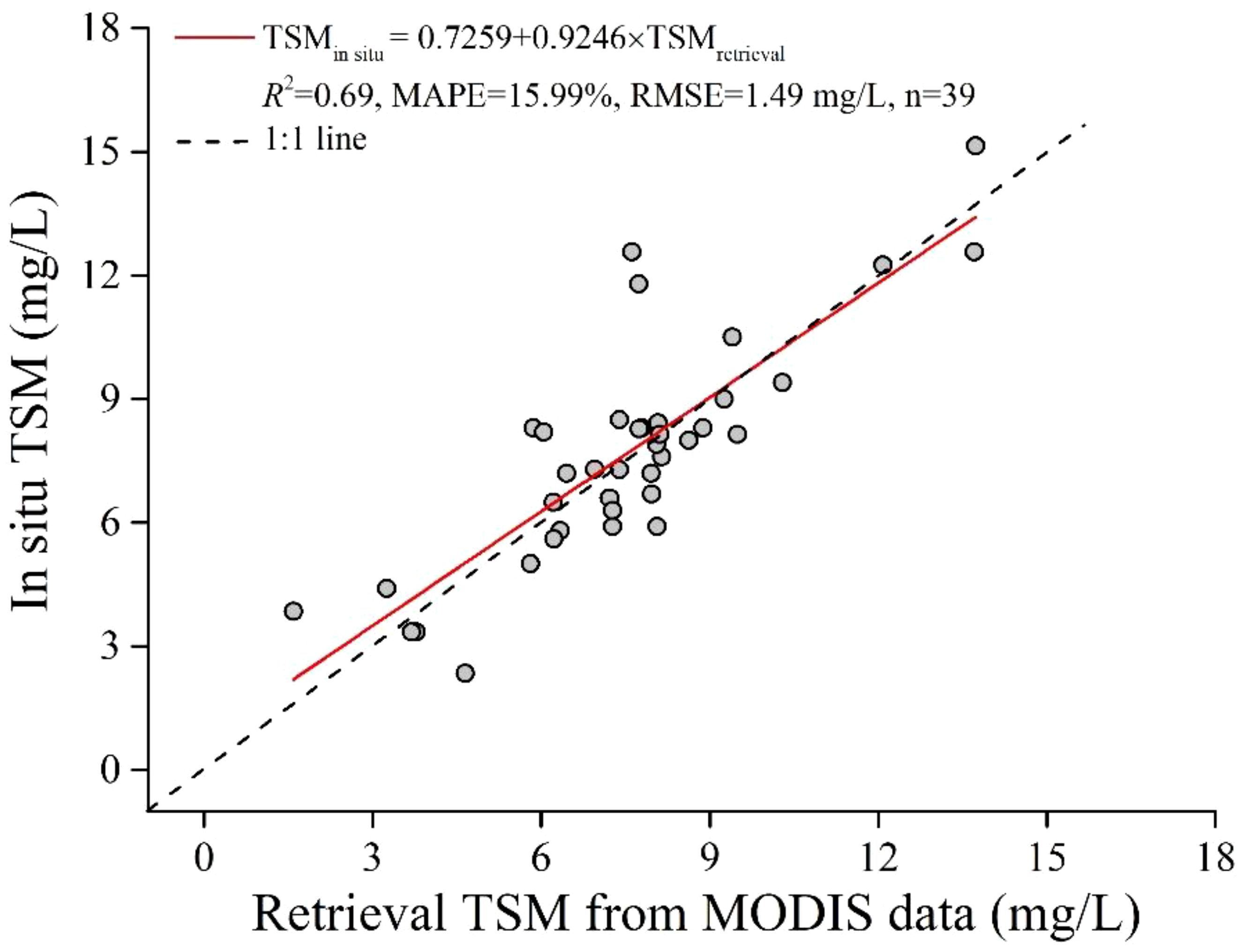
Figure 3 Scatterplot of in situ TSM and retrieval TSM from MODIS data. The red solid line shows the fitted relationship.
Based on the above validation, the CIE hue angle-based retrieval model showed very high accuracy for Aqua MODIS data, and has been used to investigate the spatiotemporal variation and remote sensing retrieval of TSM in the coastal waters of Qinhuangdao.
3.2 Spatiotemporal variation of TSM
3.2.1 Monthly spatiotemporal variation
The spatiotemporal variation characteristics of monthly TSM in the coastal waters of Qinhuangdao are significant, as shown in Figure 4. Spatially, TSM are higher near the shore, gradually decrease with increasing distance from the shore, and then increase. The overall spatial distribution is characterized by the highest concentrations in the west and north, followed by the east, and the lowest in the central and southern regions. Temporally, the highest TSM in the coastal waters of Qinhuangdao are observed from January to March and from October to December, with April, May, and September having moderate levels, and the lowest TSM occurring from June to August. Further statistical analysis reveals that the monthly mean TSM in the coastal waters of Qinhuangdao from January to December generally exhibit a ‘V’-shaped variation pattern, as depicted in Figure 5. The TSM peaks in February at a monthly mean of 8.50 mg/L, then gradually decreases to its lowest point in July at a monthly mean of 3.92 mg/L, before increasing again.
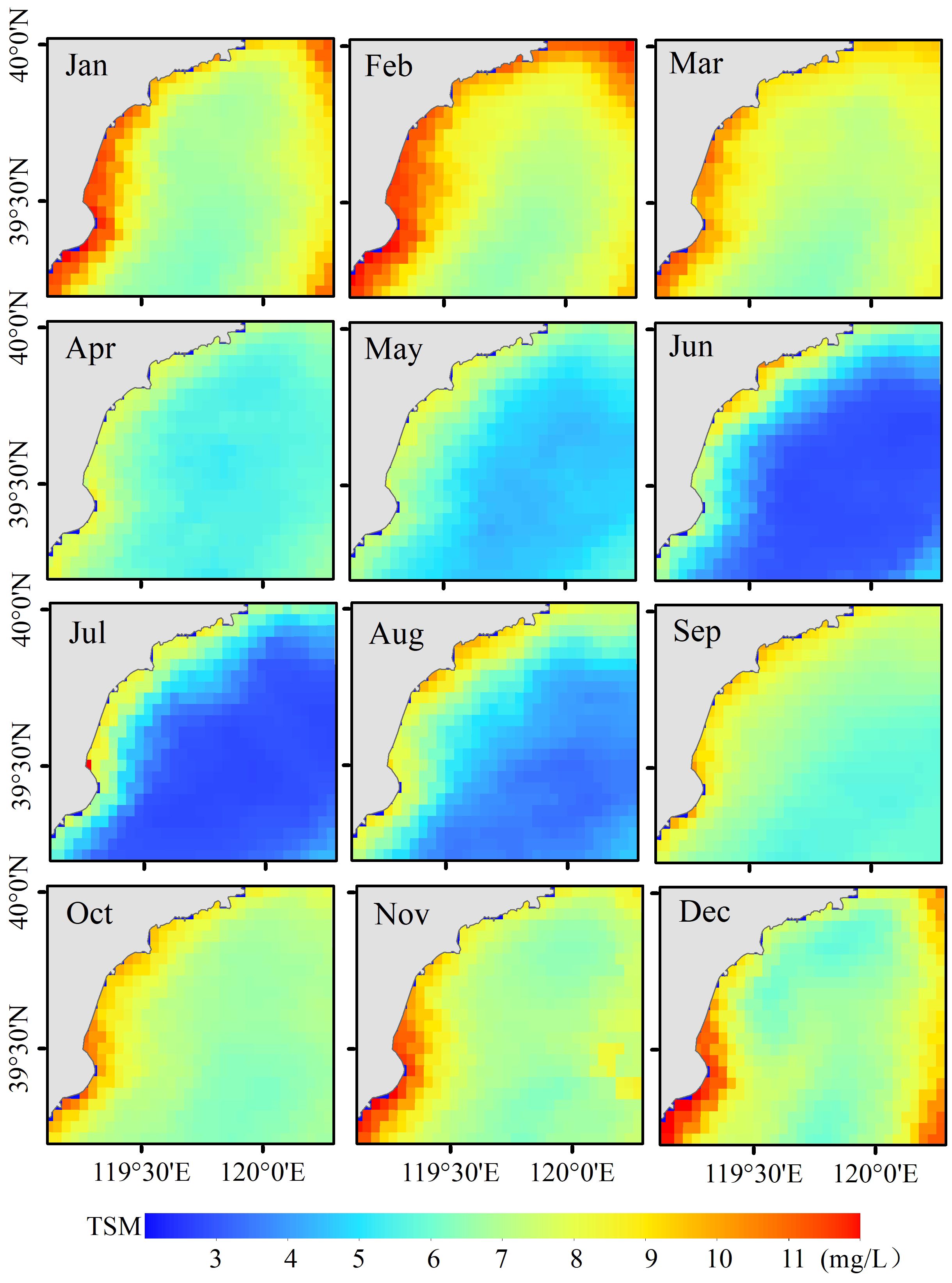
Figure 4 Spatiotemporal variation of monthly TSM in the coastal waters of Qinhuangdao from January to December over the past 21 years (2003-2023).
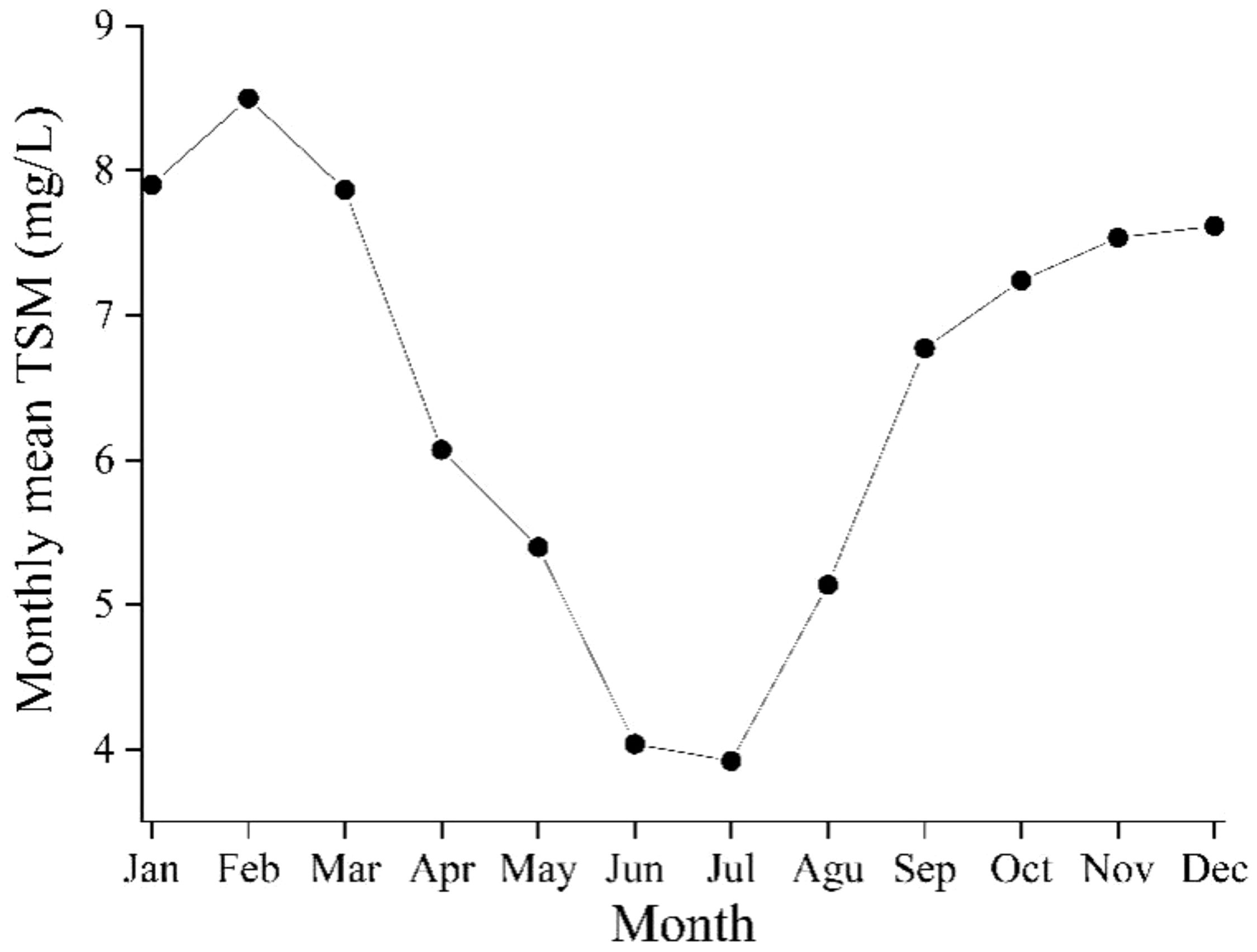
Figure 5 The monthly mean TSM in the coastal waters of Qinhuangdao from January to December over the past 21 years (2003-2023).
3.2.2 Interannual spatiotemporal variation
The coastal waters of Qinhuangdao exhibit significant spatiotemporal variation in the interannual TSM, as depicted in Figure 6. Influenced by the terrestrial inputs from rivers flowing into the sea, nearshore TSM are typically higher than offshore TSM. In 2003, 2008, and from 2018 to 2023, the TSM in the coastal waters of Qinhuangdao are relatively low, indicating clear water. In other years, the TSM are generally higher, leading to increased water turbidity, with the period from 2010 to 2012 being the most severe.
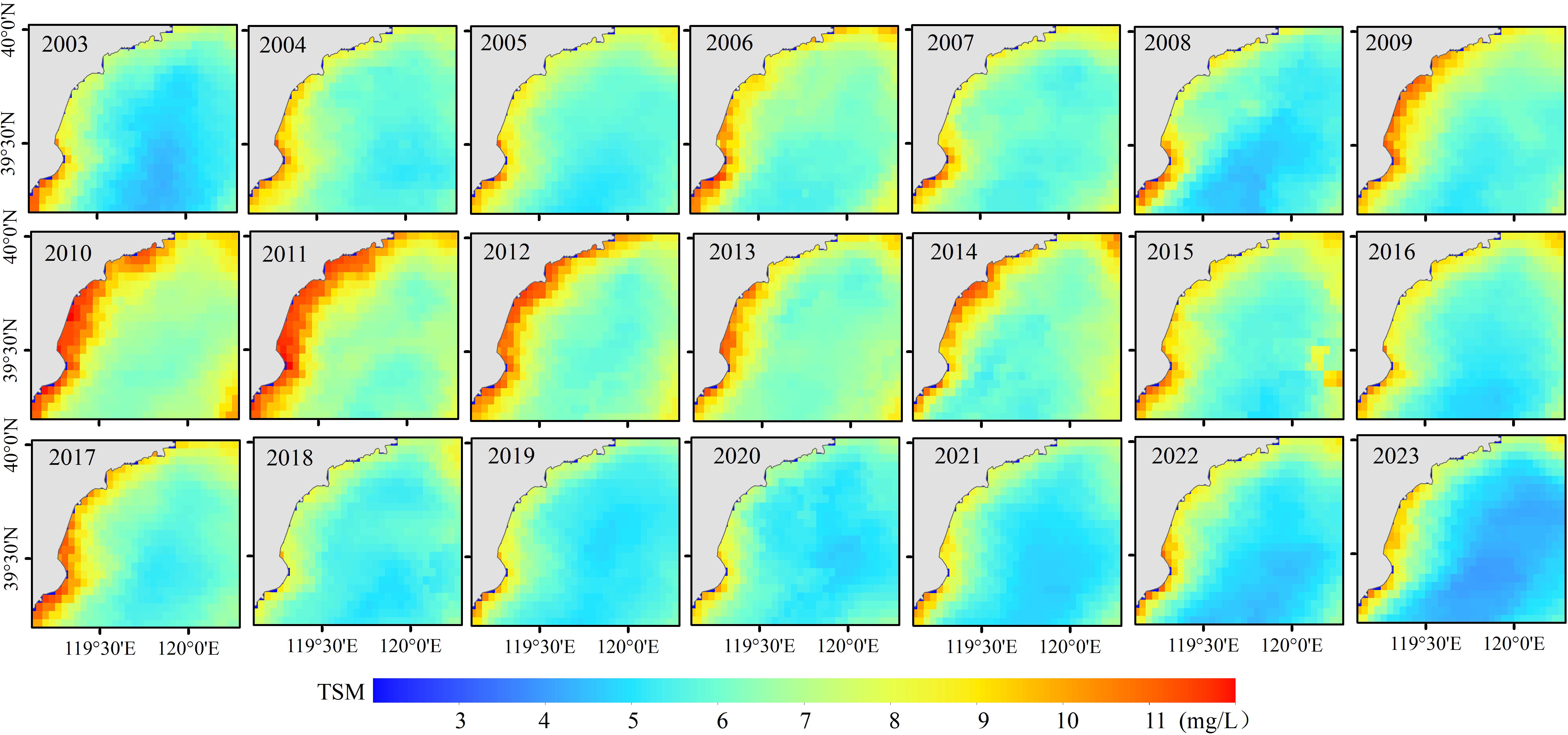
Figure 6 Spatiotemporal variation of annual TSM in the coastal waters of Qinhuangdao from 2003 to 2023.
The annual mean TSM in the coastal waters of Qinhuangdao ranged from 5.44 to 7.84 mg/L, as depicted in Figure 7. The TSM were relatively low in 2003, showing an overall increasing trend through 2006. A sudden drop occurred in 2007, reaching a low point in 2008. Subsequently, the TSM rose again, reaching a historical peak in 2010 at 7.84 mg/L. In 2011, the TSM were essentially unchanged from the previous year, with only a slight decline. From 2012, the TSM showed a general downward trend, interrupted by two rebound periods in 2017 and from 2021 to 2022. In 2023, The TSM fell to lowest historical level at 5.44 mg/L. At this time, the waters were at the clearest state in the past 21 years.
Compared to the historical annual average TSM from 2003 to 2023, Figure 8 illustrates the spatiotemporal distribution of TSM changes (ΔTSM). The TSM in the coastal waters of Qinhuangdao in 2003, 2008, and 2018 to 2023 were significantly lower. In particular, since 2019, the TSM in most sea areas have consistently been below the historical averages, marking the clearest period in the past 21 years. In other years, the TSM have consistently exceeded the historical average, with particular emphasis on the period from 2010 to 2012, identified as the most turbid phase in the past 21 years.
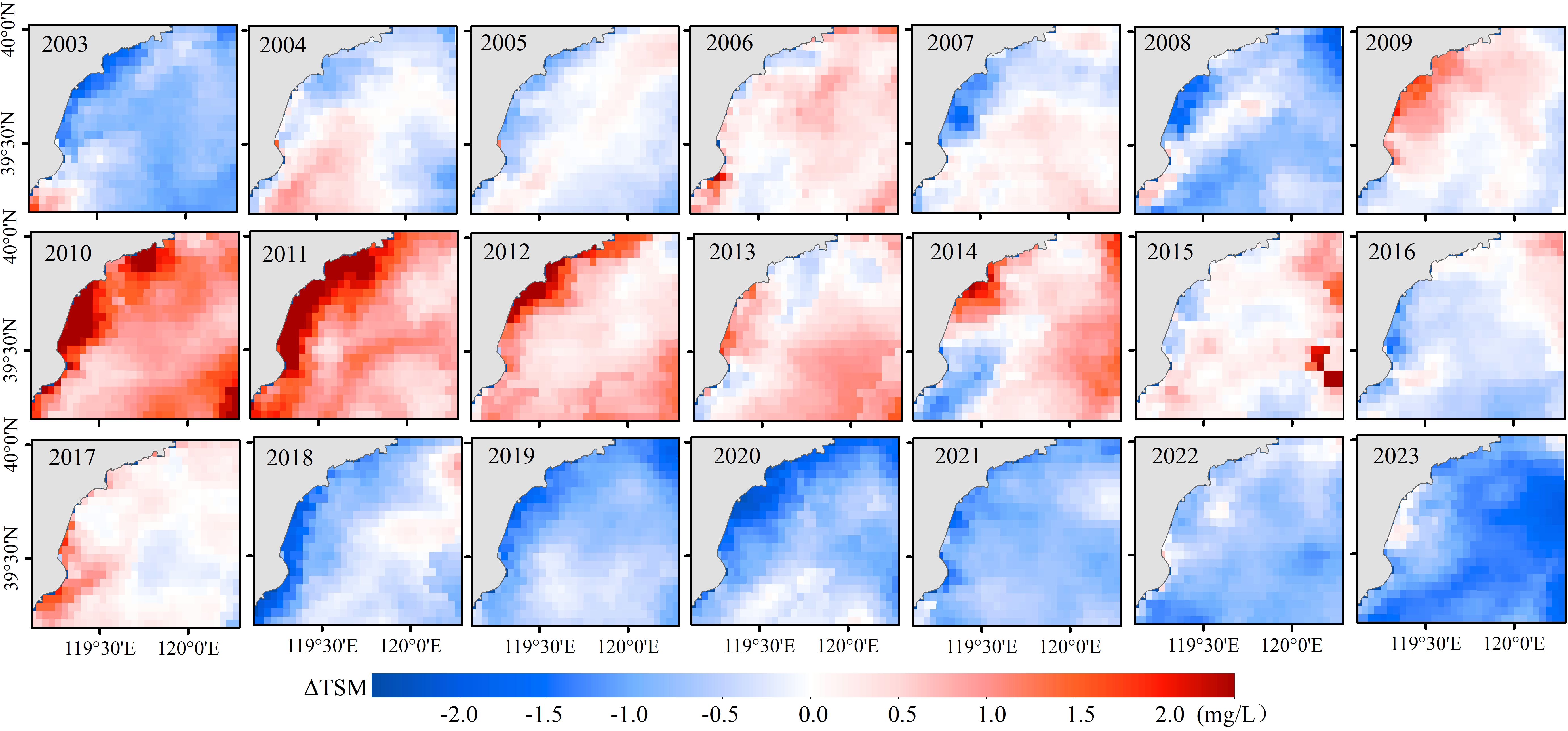
Figure 8 Spatiotemporal distribution of TSM changes (ΔTSM) in the coastal waters of Qinhuangdao from 2003 to 2023, compared to the historical annual average TSM.
4 Discussion
4.1 Impact of the material composition of TSM in the coastal waters of Qinhuangdao on the retrieval model
The sensitivity factors of the model are significantly correlated with the material composition of suspended matter. The coastal waters of Qinhuangdao, situated in the western part of the Bohai Sea, are clearer and relatively lower TSM compared to other parts of the Bohai Sea. Further analysis reveals a significant positive correlation between the in situ measured TSM and Chla in the coastal waters of Qinhuangdao, as shown in Figure 9 (R2 =0.44). This indicates that TSM in this sea area vary with the Chla of phytoplankton, suggesting a relatively high proportion of phytoplankton in the suspended matter.
In previous studies, the red band retrieval model for TSM has been effectively applied in numerous sea areas. However, it is entirely inapplicable to the coastal waters of Qinhuangdao. The primary reason for this can be attributed to the high abundance of phytoplankton in the suspended matter in the coastal waters of Qinhuangdao. In the red band, the strong absorption of chlorophyll a by phytoplankton creates a reflection valley. As the content of phytoplankton in the suspended matter increases, the depth of the reflectance valley deepens. Meanwhile, the backscattering effect of suspended matter in the red band strengthens increases with the rising content of suspended matter, leading to higher reflectance. This superimposed effect introduces significant uncertainty in the red band’s reflectance as the content of suspended matter varies, ultimately rendering the red band model inapplicable.
Nevertheless, the remote sensing retrieval model for TSM with single or multiple band ratios demonstrates good applicability in the coastal waters of Qinhuangdao. It is also related to the high levels of phytoplankton and their positive, significant correlations with TSM. The blue-to-green band ratio is a sensitive factor for the remote sensing retrieval of Chla in marine phytoplankton. It is also widely applied in remote sensing retrieval studies for Chla in various waters, including NASA’s standard operational algorithms like OC3 and OC4.
In particular, due to the complex material composition of suspended matter in the coastal waters of Qinhuangdao, the retrieval model based on the CIE hue angle has demonstrated unique advantages. The hue angle, calculated from the Rrs of all visible bands, serves as a comprehensive indicator of seawater radiance information and is the one of the key quantitative parameters for assessing seawater color. Furthermore, the color of seawater intuitively reflects its clarity, making the hue angle an appropriate tool for accurately estimating TSM. It has been shown that the TSM retrieval model based on the hue angle is more accurate than traditional empirical models that utilize fewer bands. Over the past decade, the hue angle derived from satellite data has been widely used in water color remote sensing, and shows great potential in water quality monitoring and assessment.
4.2 Influence of natural factors on the spatiotemporal variation of TSM in the coastal waters of Qinhuangdao
In general, the variation of TSM in coastal waters is primarily influenced by natural factors such as wind, rainfall, sea surface temperature (SST), and light intensity.
Strong winds can disturb and re-suspend sediments from the bottom layer of coastal waters, leading to increased turbidity and TSM. The study area, located in the East Asian monsoon zone, experiences distinct seasonal variations in the sea surface wind field. In winter, the prevailing wind comes from the northwest with a stable direction, strong strength, and longer duration; in summer, it comes from the southeast with an unstable direction, weaker strength, and a shorter duration. These characteristics are reflected in the retrieval results, which show higher TSM in winter and lower in summer. Moreover, a positive correlation exists between the monthly mean TSM and wind speed (Figure 10, R2 =0.37), further confirming the monsoon’s role as a primary influencing on the monthly spatiotemporal variation of TSM in the coastal waters of Qinhuangdao. In contrast, no correlation is observed between the annual mean TSM and wind speed (Figure 11, R2 =0.04), suggesting that the interannual spatiotemporal variation of TSM in the coastal waters of Qinhuangdao are not wind-related.
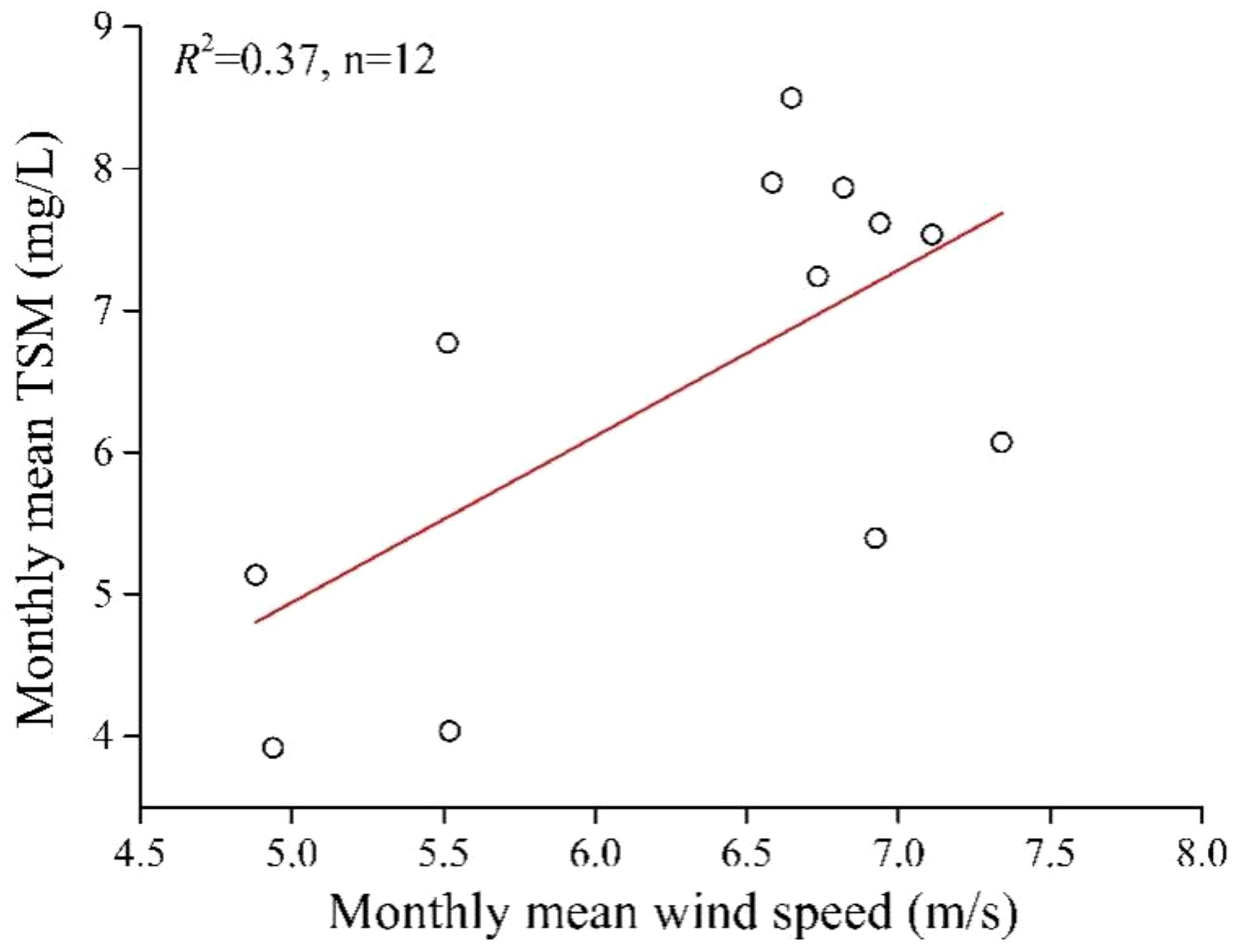
Figure 10 Relationship between monthly mean TSM derived from Aqua MODIS data and wind speed from M2IMNXLFO [Global Modeling and Assimilation Office (GMAO), 2015].
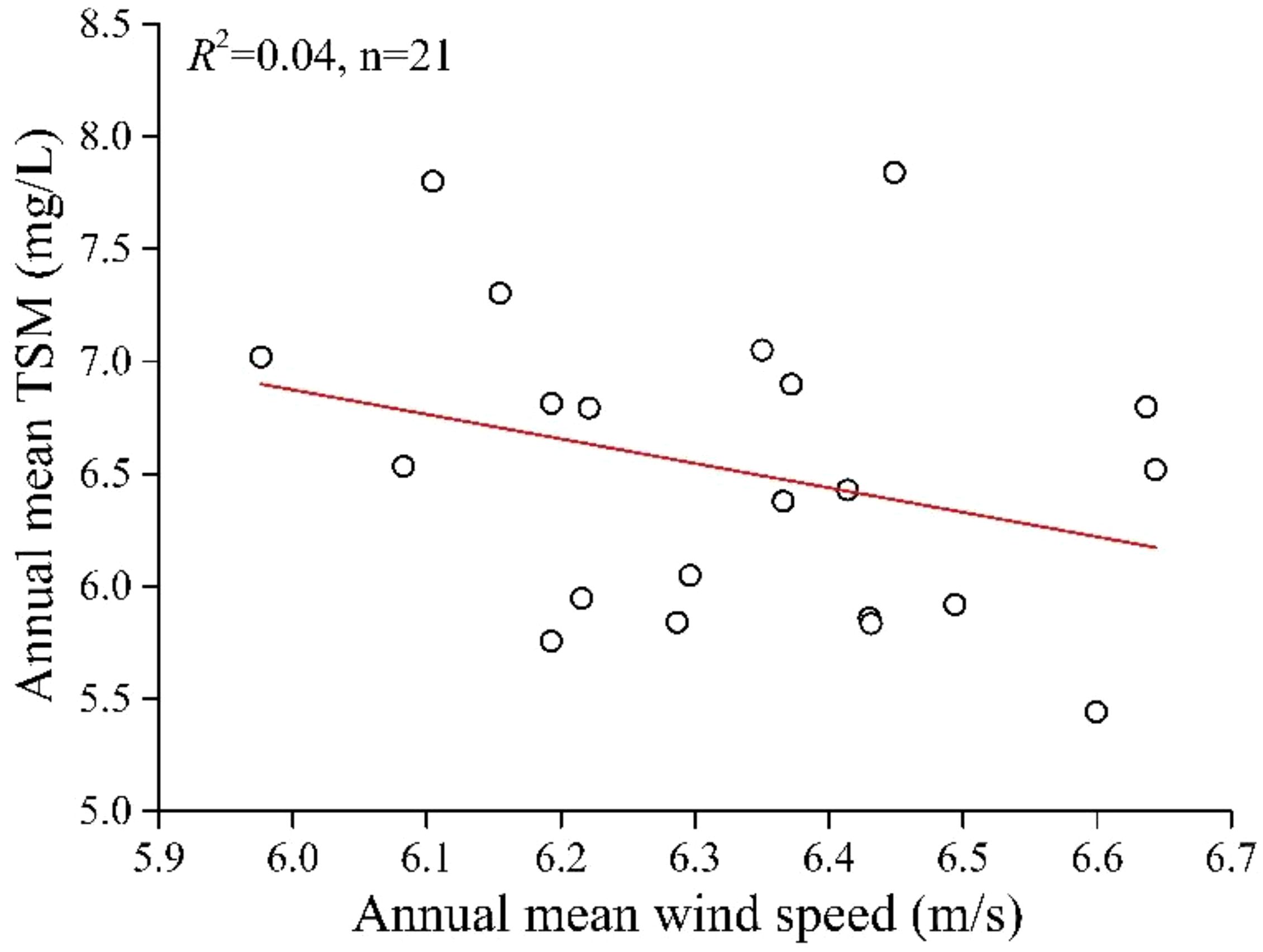
Figure 11 Relationship between annual mean TSM derived from Aqua MODIS data and wind speed from M2IMNXLFO [Global Modeling and Assimilation Office (GMAO), 2015].
Rainfall can cause a surge in the input of terrestrial turbid water, leading to a significant increase in TSM in coastal waters. However, although rainfall in the coastal area of Qinhuangdao mainly occurs in summer, the lowest TSM are observed in the coastal waters during the summer months (June to August). This observation suggests that the impact of rainfall on TSM increase in this area is transient and limited. Once the rainfall ceases, its impact dissipates quickly. Therefore, the impact of rainfall on TSM in the coastal waters of Qinhuangdao is negligible over monthly or annual timescales.
SST and light intensity are important factors affecting the growth of marine phytoplankton, and changes in phytoplankton populations can directly affect TSM. The analysis reveals no significant positive correlation between the monthly mean TSM and Chla in the coastal waters of Qinhuangdao (Figure 12). The correlation between them is relatively low (R2 =0.09), indirectly suggesting that the SST and light intensity do not significantly affect the monthly spatiotemporal variation of TSM. However, the annual mean TSM is significantly and positively correlated with the Chla (Figure 13, R2 =0.60), and there is no significant correlation with the mean SST or light intensity (Figure 14, R2 values are very low). These results indicate that SST and light intensity do not have significant effect on the spatiotemporal variation of annual TSM.
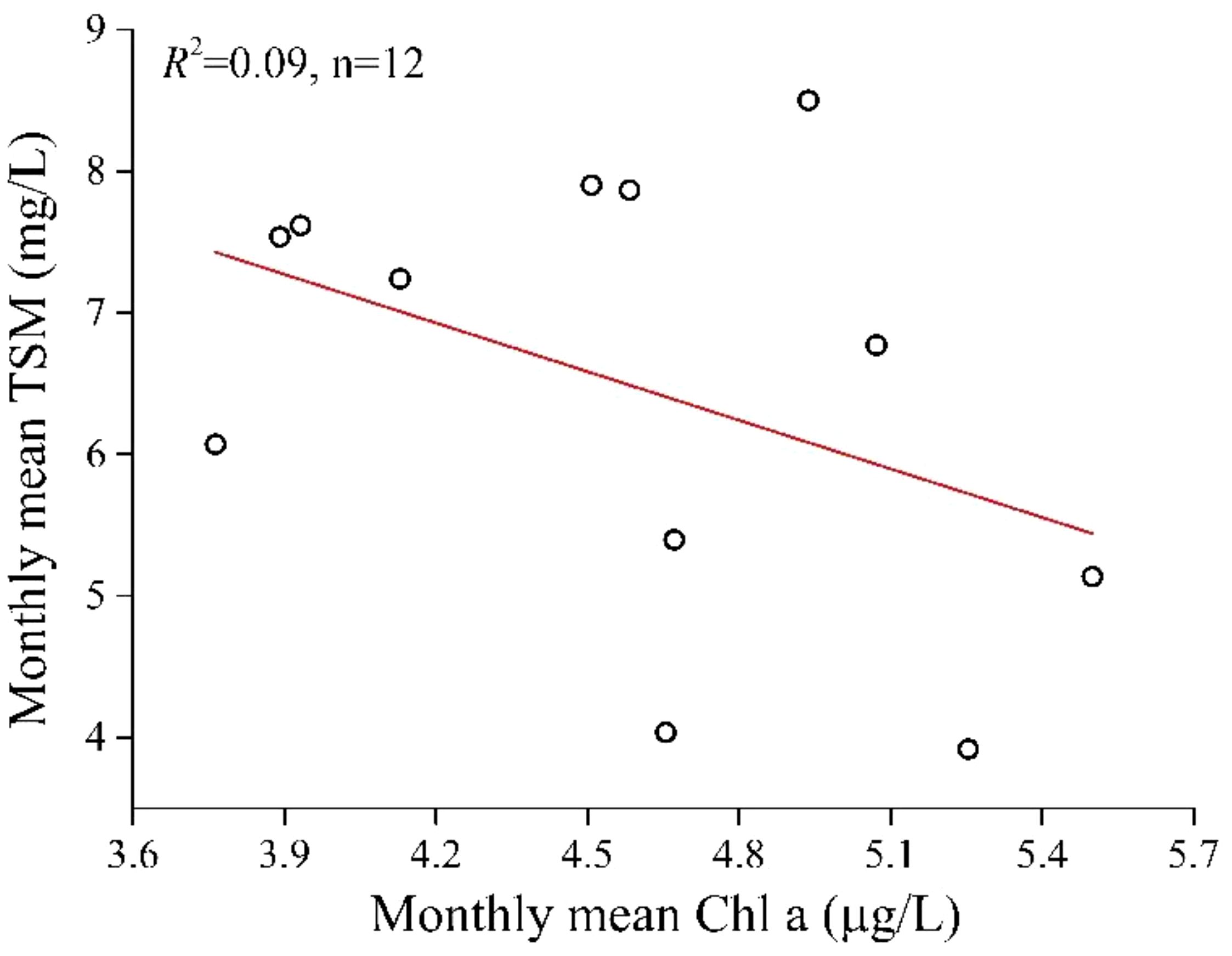
Figure 12 Relationship between monthly mean TSM and Chla, where TSM is derived from Aqua MODIS data, but Chla is downloaded from Aqua MODIS Level 3 standard product.
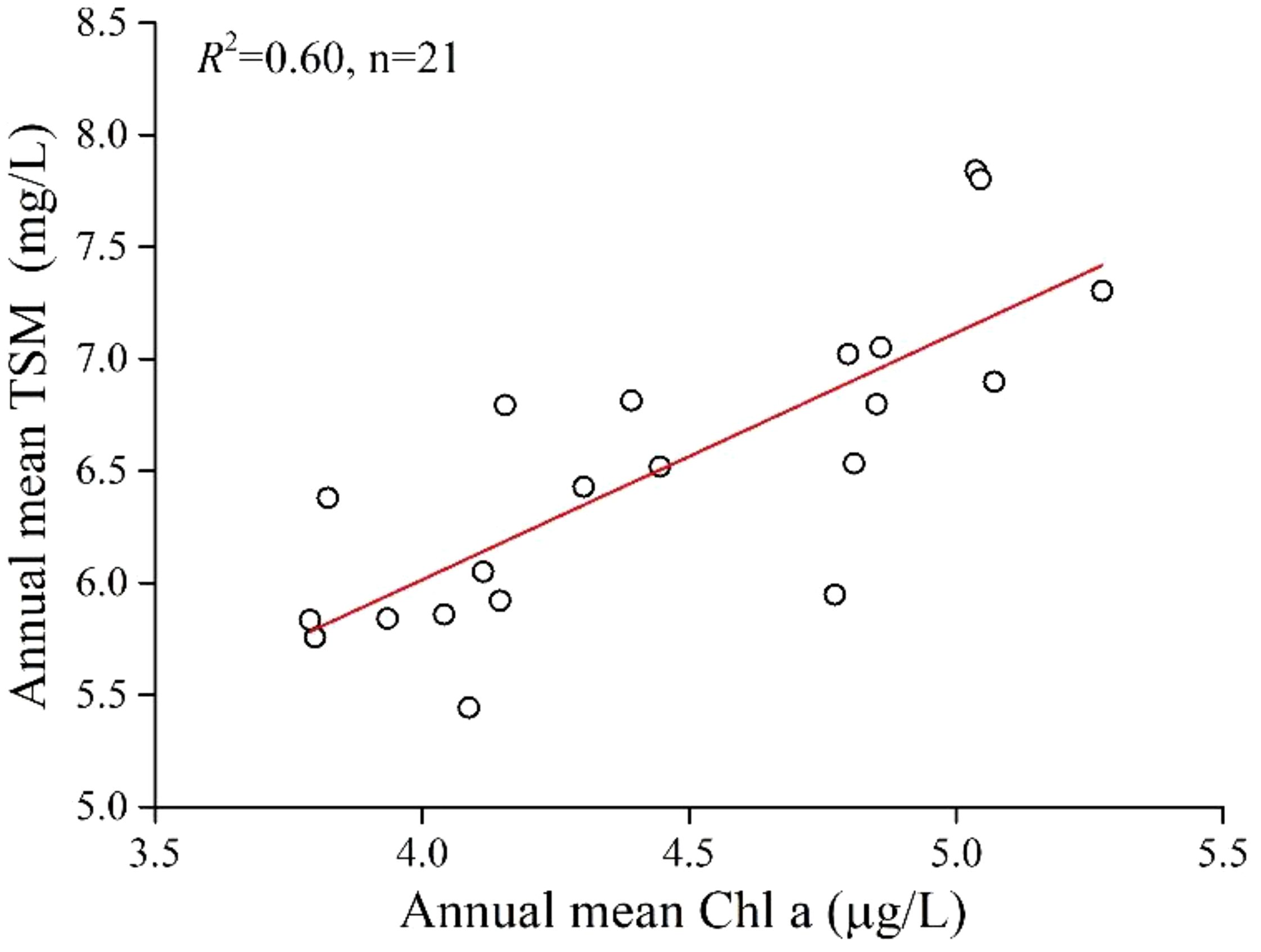
Figure 13 Relationship between annual mean TSM and Chla, where TSM is derived from Aqua MODIS data, but Chla is downloaded from Aqua MODIS Level 3 standard product.
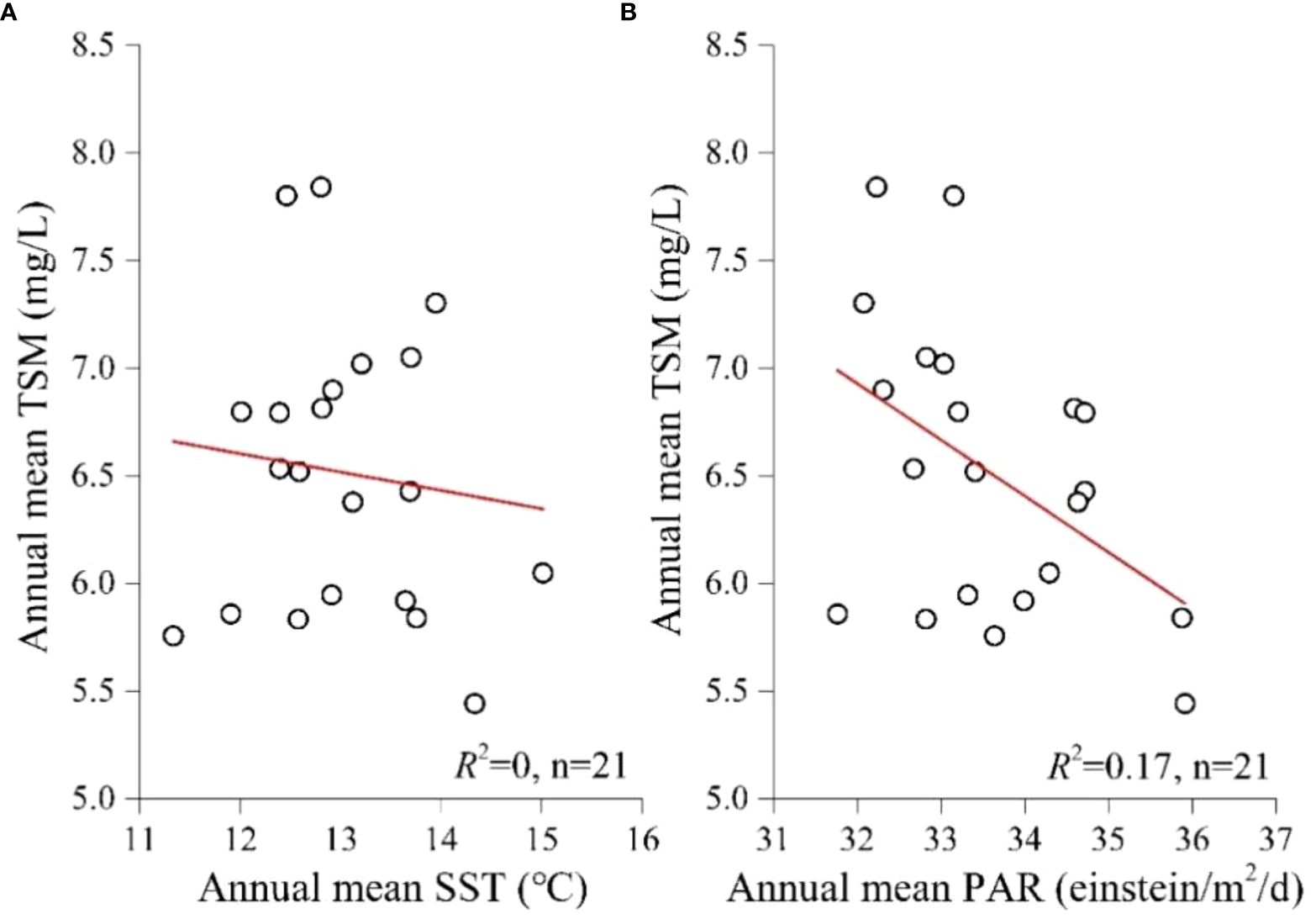
Figure 14 Relationship between annual mean TSM and (A) SST, (B) PAR, where TSM derived from Aqua MODIS data, but SST and PAR are downloaded from Aqua MODIS Level 3 standard product.
4.3 Influence of anthropogenic factors on the spatiotemporal variation of TSM in the coastal waters of Qinhuangdao
The above section shows that the TSM in the coastal waters of Qinhuangdao contains a large amount of phytoplankton, and there is a significant positive correlation between the annual mean TSM and Chla. This result indicates that changes in phytoplankton content in this area can directly affect the annual variations of TSM. However, natural factors affecting phytoplankton growth, such as SST and PAR, are not directly related to TSM. Therefore, it can be inferred that nutrients play a pivotal role in driving the annual variations of TSM (or phytoplankton) in the coastal waters of Qinhuangdao. Nutrient concentrations in the coastal waters are closely related to the discharge of terrigenous pollutants from coastal areas, and these discharges are largely influenced by anthropogenic factors.
Since the beginning of the 21st century, China’s economy has entered a phase of rapid development. However, this progress has been accompanied by a surge in industrial pollutants, exacerbating environmental pollution and creating a growing contradiction between economic development and environmental protection. The coastal waters act as the primary recipients of pollutants, and the quality of their ecological environment is closely related to the intensity of pollutant discharges from coastal regions. In 2003, the TSM in the coastal waters of Qinhuangdao remained at a relatively low level. As the local economy developed, the amount of pollutants discharged increased, causing the TSM to rise until 2006. In 2007 and 2008, there was a noticeable decline, reaching the lowest value in 2008, coinciding with the 2008 Beijing Olympics. The ‘Green Olympics’ concept was one of the three main themes of the Beijing 2008 Olympic Games. At that time, the Chinese government implemented strict pollution reduction measures in Beijing and neighboring provinces and municipalities, including Qinhuangdao, greatly reducing the impact of land-based discharges on the sea and leading to improved water quality and a significant decrease in TSM in the coastal waters of Qinhuangdao. However, since 2009, the coastal waters of Qinhuangdao have experienced persistent brown tide bloom caused by Aureococcus anophagefferens, resulting in significant economic losses and serious ecological damage (Kong et al., 2012; Zhang et al., 2012). From 2010 to 2012, this ecological disaster occurred at a high incidence. Especially in 2010, a brown tide covering 3350 km2 caused economic losses of 205 million RMB in scallop production in the Qinhuangdao coastal area, according to the MNR (2010). It has attracted the attention of local authorities. In response to this situation, the Government formulated various emission reduction measures and implemented restoration projects costing tens of billions of CNY. Additionally, since 2013, the Chinese government has vigorously promoted the ecological civilization construction, adhering to the development concept that ‘lucid waters and lush mountains are invaluable assets’. All these measures have greatly reduced pollutant emissions. As a result, the water quality in the coastal waters of Qinhuangdao has been continuously improving, with TSM decreasing gradually. The rebound in TSM in 2017 also indicates that the effectiveness of water quality improvements can be inconsistent. In 2018, the action plan for the comprehensive treatment of pollution in the Bohai Sea was fully initiated, playing a key role in further improving the coastal waters of Qinhuangdao and the ecological environment of the Bohai Sea. The TSM in the coastal waters of Qinhuangdao resumed a downward trend from 2018 to 2023, although there was a slight rebound in 2021 and 2022.
The interannual variation of TSM in the coastal waters of Qinhuangdao from 2003 to 2023 serves as a microcosm of the changing ecological environment status in China. This process perfectly encapsulates the arduous journey of China’s rapid economic development, manifested in the following five stages: (1) the increasingly serious problem of environmental pollution in the course of rapid economic growth; (2) the progressive emergence of contradictions between economic development and environmental protection; (3) the comprehensive promotion of ecological civilization construction; (4) the constant deepening of various battles against pollution; (5) the gradual improvement of the ecological environment.
5 Conclusion
(1) In this study, a remote sensing retrieval model for TSM that utilizes the CIE hue angle (R2 =0.68, MAPE=24.47%, RMSE=2.72 mg/L) was developed using in situ measured data from the coastal waters of Qinhuangdao collected between 2013 and 2021. The model demonstrates superior accuracy compared to traditional empirical models, including single-band, band ratio, and multi-band models.
(2) From January to December, the TSM are high near the shore and exhibit a trend of gradually decreasing and then increasing with increasing distance from the shore. The TSM peak in February at a monthly mean value of 8.50 mg/L, then gradually decrease to the lowest point in July at 3.92 mg/L, before gradually increasing again. It has been confirmed that the monsoon is one of the main influences on the monthly spatiotemporal variation of TSM in the coastal waters of Qinhuangdao.
(3) The TSM were relatively low in the coastal waters of Qinhuangdao in 2003, 2008, and from 2018 to 2023, but were higher in 2010 to 2012 than in other periods. The interannual variation in TSM correspond to the timing of pollution reduction management measures implemented by the government. This process perfectly encapsulates the arduous journey of China’s rapid economic development, characterized by the following five stages: 1) the increasingly serious problem of environmental pollution in the course of rapid economic growth; 2) the progressive emergence of contradictions between economic development and environmental protection; 3) the comprehensive promotion of ecological civilization construction; 4) the constant deepening of various battles against pollution; 5) the gradual improvement of the ecological environment.
(4) The TSM in the coastal waters of Qinhuangdao are relatively low, resulting in a hue angle range of 114.27° to 230.36° without saturation. However, in high-turbidity waters, such as those at the Yellow River estuary and the Yangtze River estuary sea area, hue angle saturation may occur, limiting the applicability of the hue angle for retrieving TSM. Nevertheless, the CIE hue angle is a comprehensive indicator of seawater radiance information and a key quantitative parameter for assessing seawater color. Derived from satellite data, it has been widely used in water color remote sensing and shows great potential in water quality monitoring and assessment.
Data availability statement
The original contributions presented in the study are included in the article/supplementary material, further inquiries can be directed to the corresponding author/s.
Author contributions
LW: Writing – review & editing, Writing – original draft, Validation, Supervision, Resources, Project administration, Methodology, Investigation, Funding acquisition, Data curation, Conceptualization. XAW: Writing – review & editing, Validation, Supervision, Software, Data curation. QM: Writing – review & editing, Writing – original draft, Validation, Supervision, Methodology, Investigation, Conceptualization. YC: Writing – review & editing, Visualization, Investigation, Formal analysis, Data curation. XXW: Visualization, Project administration, Methodology, Data curation, Writing – review & editing, Investigation. LJ: Resources, Investigation, Funding acquisition, Writing – review & editing. YS: Writing – review & editing, Validation, Software, Formal analysis, Data curation.
Funding
The author(s) declare financial support was received for the research, authorship, and/or publication of this article. This work was supported in part by the Ministry of Science and Technology of the People’s Republic of China (2019YFC1407904, 2018YFC1407605), and in part by the National Natural Science Foundation of China (42076186).
Acknowledgments
The authors would like to thank the National Aeronautics and Space Administration (NASA) for the distribution of Aqua MODIS data, and their colleagues at the National Marine Environmental Monitoring Center for their help in making in situ measurements, the results of which were used in this work.
Conflict of interest
The authors declare that the research was conducted in the absence of any commercial or financial relationships that could be construed as a potential conflict of interest.
Publisher’s note
All claims expressed in this article are solely those of the authors and do not necessarily represent those of their affiliated organizations, or those of the publisher, the editors and the reviewers. Any product that may be evaluated in this article, or claim that may be made by its manufacturer, is not guaranteed or endorsed by the publisher.
References
Abegaz N. T., Tsidu G. M., Arsiso B. K. (2023). Spatiotemporal variability of the lake Tana water quality derived from the MODIS-based forel–ule index: the roles of hydrometeorological and surface processes. Atmosphere 14, 289. doi: 10.1016/j.rse.2004.11.002
Binding C. E., Bowers D. G., Mitchelson-Jacob E. G. (2005). Estimating suspended sediment concentrations from ocean colour measurements in moderately turbid waters; the impact of variable particle scattering properties. Remote Sens. Environ. 94, 373–383. doi: 10.1016/j.rse.2004.11.002
Burket M. O., Olmanson L. G., Brezonik P. L. (2023). Comparison of two water color algorithms: implications for the remote sensing of water bodies with moderate to high CDOM or chlorophyll levels. Sensors 23, 1071. doi: 10.3390/s23031071
Chen C., Wang J., Bai L., Feng J., Gao X., Liu R. (2024). “Red tide monitoring in the Bohai sea based on domestic high-resolution data,” in ICIGP '24: Proceedings of the 2024 7th International Conference on Image and Graphics Processing (New York, NY, United States: Association for Computing Machinery), 455–461. doi: 10.1145/3647649.3647720
Chen Q., Huang M. T., Tang X. D. (2020). Eutrophication assessment of seasonal urban lakes in China Yangtze River Basin using Landsat 8-derived Forel-Ule index: A six-year, (2013–2018) observation. Sci. Total Environ. 745, 135392. doi: 10.1016/j.scitotenv.2019.135392
Commission Internationale de l’Eclairage (1932). Proceedings 1931 (Cambridge: Cambridge University Press).
Dai Y. H., Yang S. B., Zhao D., Hu C. M., Xu W., Anderson D. M., et al. (2023). Coastal phytoplankton blooms expand and intensify in the 21st century. Nature 615, 280–284. doi: 10.1038/s41586-023-05760-y
Garaba S. P., Badewien T. H., Braun A., Schulz A. C., Zielinski O. (2014a). Using ocean colour remote sensing products to estimate turbidity at the Wadden Sea time series station Spiekeroog. J. Eur. Opt. Soc.-Rapid Publ. 9, 14020. doi: 10.2971/jeos.2014.14020
Garaba S. P., Friedrichs A., Voß D., Zielinski O. (2015). Classifying natural waters with the Forel-Ule Colour index system: results, applications, correlations and crowdsourcing. Int. J. Environ. Res. Public Health 12, 16096–16109. doi: 10.3390/ijerph121215044
Garaba S. P., Voß D., Zielinski O. (2014b). Physical, bio-optical state and correlations in North–Western European Shelf Seas. Remote Sens. 6, 5042–5066. doi: 10.3390/rs6065042
Global Modeling and Assimilation Office (GMAO) (2015). MERRA-2 instM_2d_lfo_Nx: 2d,Monthly mean, Instantaneous, Single-Level, Assimilation, Land Surface Forcings V5.12.4 (Greenbelt, MD, USA: Goddard Earth Sciences Data and Information Services Center (GES DISC) (Accessed 2024-03-04).
Han H., Li Y., Ma X., Song W., Wang Z., Zhang X. (2022). Factors influencing the spatial and temporal distributions of green algae micro-propagules in the coastal waters of Jinmenghaiwan, Qinhuangdao, China. Mar. pollut. Bull. 175, 113328. doi: 10.1016/j.marpolbul.2022.113328
Han H., Song W., Wang Z., Ding D., Yuan C., Zhang X., et al. (2019). Distribution of green algae micro-propagules and their function in the formation of the green tides in the coast of Qinhuangdao, the Bohai Sea, China. Acta Oceanol. Sin. 38, 72–77. doi: 10.1007/s13131-018-1278-1
Hou X., Feng L., Dai Y., Hu C., Gibson L., Tang J., et al. (2022). Global mapping reveals increase in lacustrine algal blooms over the past decade. Nat. Geosci. 15, 130–134. doi: 10.1038/s41561-021-00887-x
Jiang X. W., He X. Q., Lin M., Gong F., Ye X. M., Pan D. L. (2019). Progresses on ocean satellite remote sensing application in China. Haiyang Xuebao 41, 113–124. doi: 10.3969/j.issn.0253-4193.2019.10.007
Kong F. Z., Yu R. C., Zhang Q. C., Yan T., Zhou M. J. (2012). Pigment characterization for the 2011 bloom in Qinhuangdao implicated “brown tide” events in China. Chin. J. Oceanol. Limnol. 30, 361–370. doi: 10.1007/s00343-012-1239-z
Kong J. L., Sun X. M., Wong D. W., Chen Y., Yang J., Yan Y., et al. (2015). A semi-analytical model for remote sensing retrieval of suspended sediment concentration in the Gulf of Bohai, China. Remote Sens. 7, 5373–5397. doi: 10.3390/rs70505373
Lee Z., Carder K. L., Arnone R. A. (2002). Deriving inherent optical properties from water color: a multiband quasi-analytical algorithm for optically deep waters. Appl. Opt. 41, 5755–5772. doi: 10.1364/AO.41.005755
Lei S. H., Xu J., Li Y. M., Du C. G., Liu G., Zheng Z. B., et al. (2020). An approach for retrieval of horizontal and vertical distribution of total suspended matter concentration from GOCI data over Lake Hongze. Sci. Total Environ. 700, 134524. doi: 10.1016/j.scitotenv.2019.134524
Li J. S., Wang S. L., Wu Y. H., Zhang B., Chen X. L., Zhang F. F., et al. (2016). MODIS observations of water color of the largest 10 lakes in China between 2000 and 2012. Int. J. Digit. Earth 9, 788–805. doi: 10.1080/17538947.2016.1139637
Li M. J., Sun Y. H., Li X. J., Cui M. Y., Huang C. (2021). An improved eutrophication assessment algorithm of estuaries and coastal waters in Liaodong Bay. Remote Sens. 13, 3867. doi: 10.3390/rs13193867
Liu R. J., Xiao Y. F., Ma Y., Cui T. W., An J. B. (2022). Red tide detection based on high spatial resolution broad band optical satellite data. ISPRS-J. Photogramm. Remote Sens. 184, 131–147. doi: 10.1016/j.isprsjprs.2021.12.009
Ma B. C., Sun D. Y., Li Z. H., Kong D. Y., Pan X. S., Wang S. Q., et al. (2023). Marine water quality environmental monitoring method based on hue-angle in CIE system. Adv. Mar. Sci. 41, 135–147. doi: 10.12362/j.issn.1671-6647.20210908001
Miller R. L., McKee B. A. (2004). Using MODIS Terra 250 m imagery to map concentrations of total suspended matter in coastal waters. Remote Sens. Environ. 93, 259–266. doi: 10.1016/j.rse.2004.07.012
Mustafa F., Wang H., Bu L., Wang Q., Shahzaman M., Bilal M., et al. (2021). Validation of gosat and oco-2 against in situ aircraft measurements and comparison with carbontracker and geos-chem over Qinhuangdao, China. Remote Sens. 13, 899. doi: 10.3390/rs13050899
Patel B., Sarangi R. K., Prajapati A., Devliya B., Patel H. (2022). Development of total suspended matter (TSM) algorithm and validation over Gujarat coastal water, the Northeast Arabian sea using in situ datasets. Mar. Geod. 45, 670–687. doi: 10.1080/01490419.2022.2116616
Pitarch J., van der Woerd H. J., Brewin R. J., Zielinski O. (2019). Optical properties of Forel-Ule water types deduced from 15 years of global satellite ocean color observations. Remote Sens. Environ. 231, 111249. doi: 10.1016/j.rse.2019.111249
Shi K., Zhang Y. L., Zhu G. W., Liu X. H., Zhou Y. Q., Xu H., et al. (2015). Long-term remote monitoring of total suspended matter concentration in Lake Taihu using 250 m MODIS-Aqua data. Remote Sens. Environ. 164, 43–56. doi: 10.1016/j.rse.2015.02.029
Sun D. Y., Li Y. M., Le C. F., Shi K., Huang C. C., Gong S. Q., et al. (2013). A semi-analytical approach for detecting suspended particulate composition in complex turbid inland waters (China). Remote Sens. Environ. 134, 92–99. doi: 10.1016/j.rse.2013.02.024
Tang J. W., Wang X. M., Song Q. J., Li T. J., Chen. Z. Z., Huang. H. H., et al. (2004). The statistic inversion algorithms of water constituents for the Huanghai Sea and the East China Sea. Acta Oceanol. Sin. 4), 617–626.
Tassan S. (1994). Local algorithms using SeaWiFS data for the retrieval of phytoplankton, pigments, suspended sediment, and yellow substance in coastal waters. Appl. Opt. 33, 2369–2378. doi: 10.1364/AO.33.002369
van der Woerd H. J., Wernand M. R. (2015). True colour classification of natural waters with medium-spectral resolution satellites: SeaWiFS, MODIS, MERIS and OLCI. Sensors 15, 25663–25680. doi: 10.3390/s151025663
Wang S. Y., Bi W. H., Li X. Y., Zhang B. J., Fu G. W., Jin W., et al. (2023a). A detection method of typical toxic mixed red tide algae in Qinhuangdao based on three-dimensional fluorescence spectroscopy. Spectrochimica Acta Part A: Mol. Biomol. Spectrosc. 298, 122704. doi: 10.1016/j.saa.2023.122704
Wang Q., Bu L., Tian L., Xu J., Zhu S., Liu J. (2020b). Validation of an airborne high spectral resolution Lidar and its measurement for aerosol optical properties over Qinhuangdao, China. Opt. Express 28, 24471–24488. doi: 10.1364/OE.397582
Wang S. L., Lee Z. P., Shang S. L., Li J. S., Zhang B., Lin G. (2019). Deriving inherent optical properties from classical water color measurements: Forel-Ule index and Secchi disk depth. Opt. Express 27, 7642–7655. doi: 10.1364/OE.27.007642
Wang S. L., Li J. S., Shen Q., Zhang B., Zhang F. F., Lu Z. Y. (2014). MODIS-based radiometric color extraction and classification of inland water with the Forel-Ule scale: A case study of Lake Taihu. IEEE J. Sel. Top. Appl. Earth Observ. Remote Sens. 8, 907–918. doi: 10.1109/JSTARS.4609443
Wang S. L., Li J. S., Zhang B., Lee Z. P., Spyrakos E., Feng L., et al. (2020a). Changes of water clarity in large lakes and reservoirs across China observed from long-term MODIS. Remote Sens. Environ. 247, 111949. doi: 10.1016/j.rse.2020.111949
Wang S. L., Li J. S., Zhang B., Spyrakos E., Tyler A. N., Shen Q., et al. (2018). Trophic state assessment of global inland waters using a MODIS-derived Forel-Ule index. Remote Sens. Environ. 217, 444–460. doi: 10.1016/j.rse.2018.08.026
Wang L., Meng Q. H., Ma Y. J., Wang X., Wang X. X., Chen Y. L. (2023b). Retrieval of chlorophyll a concentration from Sentinel-2 MSI image in Qinhuangdao coastal area. Mar. Environ. Sci. 42, 309–314. doi: 10.13634/j.cnki.mes.2023.02.015
Wang L., Meng Q. H., Wang X., Chen Y. L., Zhao S. F., Wang X. X. (2023c). Forel-Ule index extraction and spatiotemporal variation from MODIS imagery in the Bohai Sea of China. Opt. Express 31, 17861–17877. doi: 10.1364/OE.487312
Wang L., Wang X., Wang X. X., Meng Q. H., Ma Y. J., Chen Y. L. (2022). Retrieval of suspended particulate matter concentration from Sentinel-3 OLCI image in the Coastal Waters of Qinhuangdao. China Environ. Sci. 42, 3867–3875. doi: 10.19674/j.cnki.issn1000-6923.20220314.003
Wang H., Zhao D. Z., Wang L., Huang F. R. (2012b). Advance in remote sensing of water quality. Mar. Environ. Sci. 31, 285–288. doi: 10.3969/j.issn.1007-6336.2012.02.030
Wang L., Zhao D. Z., Yang J. H., Chen Y. L. (2012a). Retrieval of total suspended matter from MODIS 250 m imagery in the Bohai Sea of China. J. Oceanogr. 68, 719–725. doi: 10.1007/s10872-012-0129-5
Woźniak S. B., Meler J. (2020). Modelling water colour characteristics in an optically complex nearshore environment in the Baltic Sea; quantitative interpretation of the forel-ule scale and algorithms for the remote estimation of seawater composition. Remote Sens. 12, 2852. doi: 10.3390/rs12172852
Xu M., Gao Z., Liu C. (2015). “Detecting harmful algal blooms using Geostationary Ocean Color Imager (GOCI) data in Bohai Sea, China,” in Remote sensing and modeling of ecosystems for sustainability XII, vol. 9610. (San Diego, California, United States: SPIE Optical Engineering + Applications), 203–208. doi: 10.1117/12.2184249
Zhan W. K., Wu J., Wei X., Tang S. L., Zhan H. G. (2019). Spatio-temporal variation of the suspended sediment concentration in the Pearl River Estuary observed by MODIS during 2003–2015. Cont. Shelf Res. 172, 22–32. doi: 10.1016/j.csr.2018.11.007
Zhang Q. C., Qiu L. M., Yu R. C., Kong F. Z., Wang Y. F., Yan T., et al. (2012). Emergence of brown tides caused by Aureococcus anophagefferens Hargraves et Sieburth in China. Harmful Algae 19, 117–124. doi: 10.1016/j.hal.2012.06.007
Zhang Q. C., Yu R. C., Zhao J. Y., Kong F. Z., Chen Z. F., Niu Z., et al. (2021). Distribution of Aureococcus anophagefferens in relation to environmental factors and implications for brown tide seed sources in Qinhuangdao coastal waters, China. Harmful Algae 109, 1–10. doi: 10.1016/j.hal.2021.102105
Zhang Y. B., Shi K., Zhang Y. L., Moreno-Madrinan M. J., Li Y., Li N. (2018). A semi-analytical model for estimating total suspended matter in highly turbid waters. Opt. Express 26, 34094–34112. doi: 10.1364/OE.26.034094
Zhou Y. D., He B. Y., Fu C. J., Xiao F., Feng Q., Liu H., et al. (2021). An improved Forel–Ule index method for trophic state assessments of inland waters using Landsat 8 and sentinel archives. GISci. Remote Sens. 58, 1316–1334. doi: 10.1080/15481603.2021.1987003
Keywords: total suspended matter concentration (TSM), spatiotemporal variation, CIE hue angle, aqua MODIS, coastal waters
Citation: Wang L, Wang X, Meng Q, Chen Y, Wang X, Jiang L and Shang Y (2024) Retrieval and spatiotemporal variation of total suspended matter concentration using a MODIS-derived hue angle in the coastal waters of Qinhuangdao, China. Front. Mar. Sci. 11:1434225. doi: 10.3389/fmars.2024.1434225
Received: 17 May 2024; Accepted: 02 July 2024;
Published: 25 July 2024.
Edited by:
Jianfeng Feng, Nankai University, ChinaCopyright © 2024 Wang, Wang, Meng, Chen, Wang, Jiang and Shang. This is an open-access article distributed under the terms of the Creative Commons Attribution License (CC BY). The use, distribution or reproduction in other forums is permitted, provided the original author(s) and the copyright owner(s) are credited and that the original publication in this journal is cited, in accordance with accepted academic practice. No use, distribution or reproduction is permitted which does not comply with these terms.
*Correspondence: Qinghui Meng, cWhtZW5nQG5tZW1jLm9yZy5jbg==; Yanlong Chen, eWxjaGVuQG5tZW1jLm9yZy5jbg==