- 1Ecology and Evolutionary Biology, School of Biological Sciences, The University of Adelaide, Adelaide, SA, Australia
- 2South Australian Research and Development Institute (SARDI) (Aquatic Sciences), West Beach, SA, Australia
- 3Division of Aquatic Resources, Department of Land and Natural Resources, Honolulu, HI, United States
- 4Department for Environment and Water, Port Lincoln, SA, Australia
Across the world’s oceans, our knowledge of the habitats on the seabed is limited. Increasingly, video/imagery data from remotely operated underwater vehicles (ROVs) and towed and drop cameras, deployed from vessels, are providing critical new information to map unexplored benthic (seabed) habitats. However, these vessel-based surveys involve considerable time and personnel, are costly, require favorable weather conditions, and are difficult to conduct in remote, offshore, and deep marine habitats, which makes mapping and surveying large areas of the benthos challenging. In this study, we present a novel and efficient method for mapping diverse benthic habitats on the continental shelf, using animal-borne video and movement data from a benthic predator, the Australian sea lion (Neophoca cinerea). Six benthic habitats (between 5-110m depth) were identified from data collected by eight Australian sea lions from two colonies in South Australia. These habitats were macroalgae reef, macroalgae meadow, bare sand, sponge/sand, invertebrate reef and invertebrate boulder habitats. Percent cover of benthic habitats differed on the foraging paths of sea lions from both colonies. The distributions of these benthic habitats were combined with oceanographic data to build Random Forest models for predicting benthic habitats on the continental shelf. Random forest models performed well (validated models had a >98% accuracy), predicting large areas of macroalgae reef, bare sand, sponge/sand and invertebrate reef habitats on the continental shelf in southern Australia. Modelling of benthic habitats from animal-borne video data provides an effective approach for mapping extensive areas of the continental shelf. These data provide valuable new information on the seabed and complement traditional methods of mapping and surveying benthic habitats. Better understanding and preserving these habitats is crucial, amid increasing human impacts on benthic environments around the world.
1 Introduction
Across much of the marine environment, our understanding of the structure and distribution of habitats on the seabed is limited (Kostylev, 2012; Mayer et al., 2018; Menandro and Bastos, 2020). For marine habitats at depth, remotely operated underwater vehicles (ROVs), and towed and drop cameras, deployed from vessels, are gaining increasing use to collect high-resolution video and imagery data, enabling detailed mapping and surveying of benthic (seabed) environments (López-Garrido et al., 2020; Button et al., 2021; Vigo et al., 2023). However, these vessel-based surveys are costly, time and personnel-intensive, and rely on suitable weather conditions, which makes mapping large expanses of the benthos challenging (Mayer et al., 2018; Menandro and Bastos, 2020). In this study, we present a novel and effective method to map diverse benthic habitats on the continental shelf in southern Australia, using animal-borne video from a benthic foraging marine mammal, the Australian sea lion (Neophoca cinerea).
For mapping and surveying marine habitats, animal-borne video from Australian sea lions offers unique advantages. Video can be recorded across large areas of the benthos in short timeframes, deployments can be conducted from shore with reduced personnel at a relatively low cost, and deployments are less subject to weather conditions. Additionally, video can be collected from depths, habitats, and marine areas that are difficult or impossible to access using more conventional methods, such as diver surveys, and towed and drop camera deployments. Animal-borne video from Australian sea lions also provides a novel way to understand the ecological value of different benthic habitats from a predator’s perspective, complementing more traditional approaches and ecological criteria that assess habitat quality and importance (Diaz et al., 2004; Monk et al., 2010; Torn et al., 2017).
For the waters in South Australia, our knowledge of the benthos is limited and patchy. In sheltered embayments, such as the Gulf St. Vincent (Figure 1), towed camera and diver surveys have been used to map benthic habitats, highlighting large regions of bare sand plains and seagrass meadows (Shepherd and Sprigg, 1976; Tanner, 2005). Elsewhere in South Australia, in regions such as the Spencer Gulf and the Great Australian Bight, sled and grab sampling have provided some insight into sediment composition and benthic community structure (Ward et al., 2006a; Currie et al., 2007; O'Connell et al., 2016). However, for most of the state’s waters, the distribution and structure of benthic habitats is unknown. In this study, we use animal-borne video, collected from eight adult female Australian sea lions from two colonies in South Australia, to identify and map diverse benthic habitats on the continental shelf.
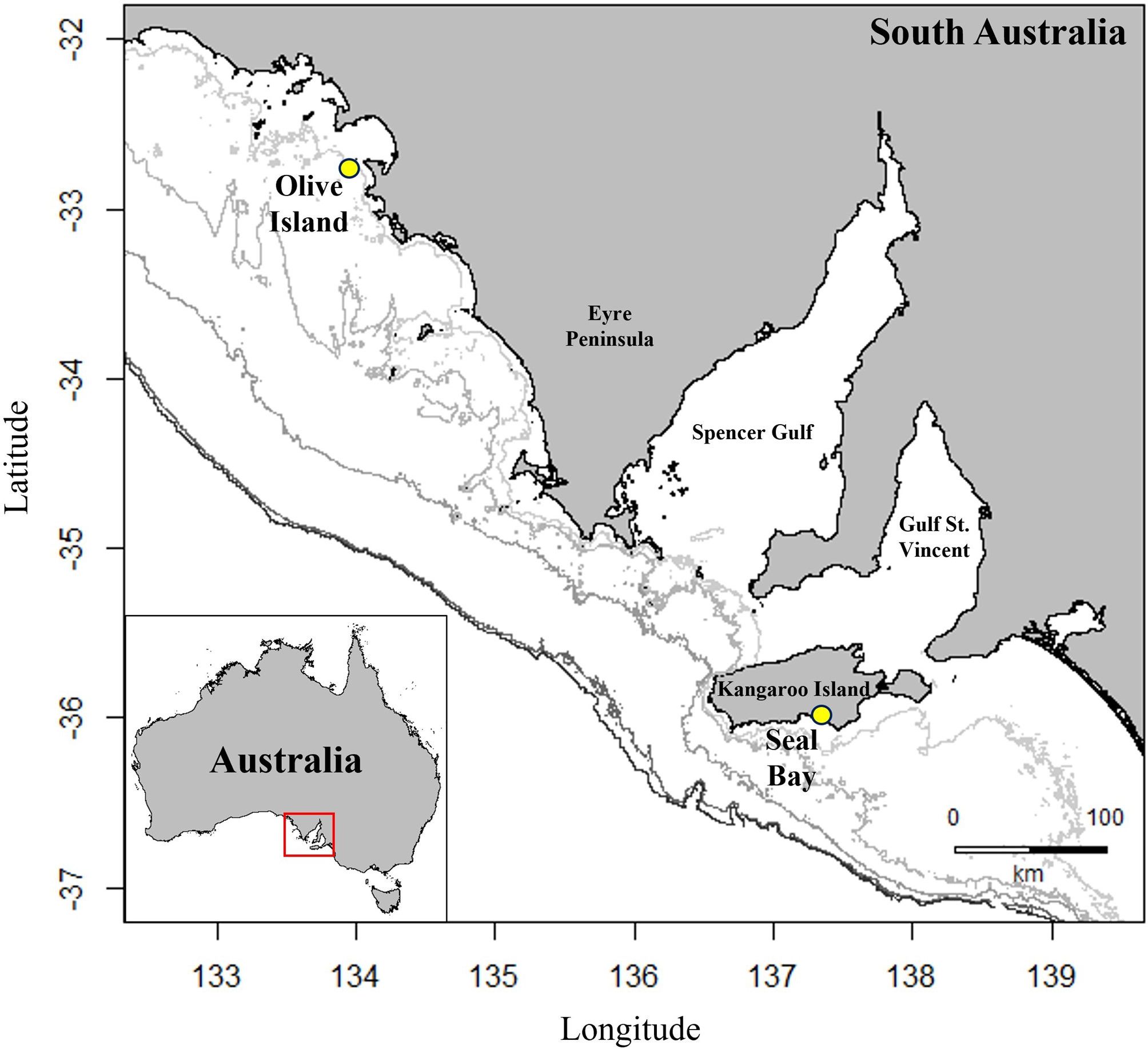
Figure 1 Location of colonies for deployment of animal-borne cameras, Argos-linked GPS loggers and accelerometers/magnetometers on eight adult female Australian sea lions from Olive Island, western Eyre Peninsula (32.721°S, 133.968°E) and Seal Bay, Kangaroo Island (35.994°S, 137.317°E) in South Australia (yellow circles). Isobaths represent depth contours at 50, 75, 100, 150 and 200m (light to dark grey).
Australian sea lions are benthic predators (Peters et al., 2015; Berry et al., 2017; Goldsworthy et al., 2019), that maximize time on the seabed (Costa and Gales, 2003; Fowler et al., 2006), restricting foraging effort to the continental shelf (Goldsworthy et al., 2007; 2022). Animal-borne video has also revealed that Australian sea lions forage across diverse benthic habitats, including sponge gardens, bare sand plains, macroalgae reefs, and seagrass meadows (Angelakis, in review). Australian sea lions are therefore an ideal platform for quantitatively assessing and mapping the distribution and structure of benthic habitats across continental shelf waters in southern Australia. Studies mapping and surveying benthic habitats from animal-borne video and imagery are limited. However, recent deployments on white sharks (Carcharodon carcharias) and grey reef sharks (Carcharhinus amblyrhynchos) have been used to map kelp forests and assess growth forms and percent cover of different corals (Jewell et al., 2019; Chapple et al., 2021), and deployments on tiger sharks (Galeocerdo cuvier) have mapped seagrass ecosystems (Gallagher et al., 2022). These approaches therefore represent an emerging area of ecological research for marine environments (Moll et al., 2007).
Like in many regions of the world (Brown et al., 2017; Sweetman et al., 2017; Yoklavich et al., 2018), benthic habitat surveys in South Australia have identified major changes to the marine environment, as a result of human activity (Tanner, 2005; Connell et al., 2008; Bryars and Rowling, 2009; Alleway and Connell, 2015). Critically, the documentation of these human-induced changes to habitat sparked policy developments by government and private investment for habitat restoration (McAfee et al., 2020). Such information also underpins the planning and management of marine reserve networks (Stewart et al., 2003; Thomas and Hughes, 2016). Furthermore, habitat surveys have highlighted diverse and endemic benthic communities in South Australia (Edyvane, 1999; McLeay et al., 2003; Currie et al., 2009; MacIntosh et al., 2018). As Australian sea lions utilize the continental shelf (Goldsworthy et al., 2007; 2022), the application of animal-borne video provides an efficient way to explore large areas of unmapped benthic habitats, find reefs, and locate ecologically important areas (e.g. valuable sea lion habitat), both within and outside marine reserves. Hence, this approach provides a complementary technique to existing methods for mapping benthic environments (López-Garrido et al., 2020; Vigo et al., 2023) and managing marine reserves (Stewart et al., 2003; Thomas and Hughes, 2016).
Australian sea lions are an endangered species (The International Union for Conservation of Nature IUCN Red List of Threatened Species and the Australian Environmental Protection and Biodiversity Conservation Act, 1999) (Goldsworthy, 2015), whose populations have declined by more than 60% over the last 40 years (Goldsworthy et al., 2021). The use of animal-borne video from Australian sea lions can therefore serve two major functions, providing new benthic habitat data for unknown/unmapped areas of the marine environment and identifying and mapping critical habitats for an endangered marine predator (Goldsworthy et al., 2021).
In this study, we aim to use animal-borne video and movement data to 1) calculate the percent cover of different benthic habitats on Australian sea lion foraging paths, 2) develop a model for predicting and mapping diverse benthic habitats on the continental shelf in southern Australia, and 3) assess the predicted distribution of these habitats, relative to our current understanding of benthic environments in South Australia.
2 Materials and methods
2.1 Study sites and deployment of instruments
Data were collected from eight adult female Australian sea lions from two colonies in South Australia at Olive Island Conservation Park (32.721°S, 133.968°E) on the western Eyre Peninsula (n= 4) and Seal Bay Conservation Park (35.994°S, 137.317°E) (n= 4) on Kangaroo Island (Figure 1), between December 2022 and August 2023. Olive Island and Seal Bay are two of the largest Australian sea lion colonies and are key monitoring sites for the species (Goldsworthy et al., 2021). Morphometric, condition, and reproductive history data for each sea lion are provided (Table 1).
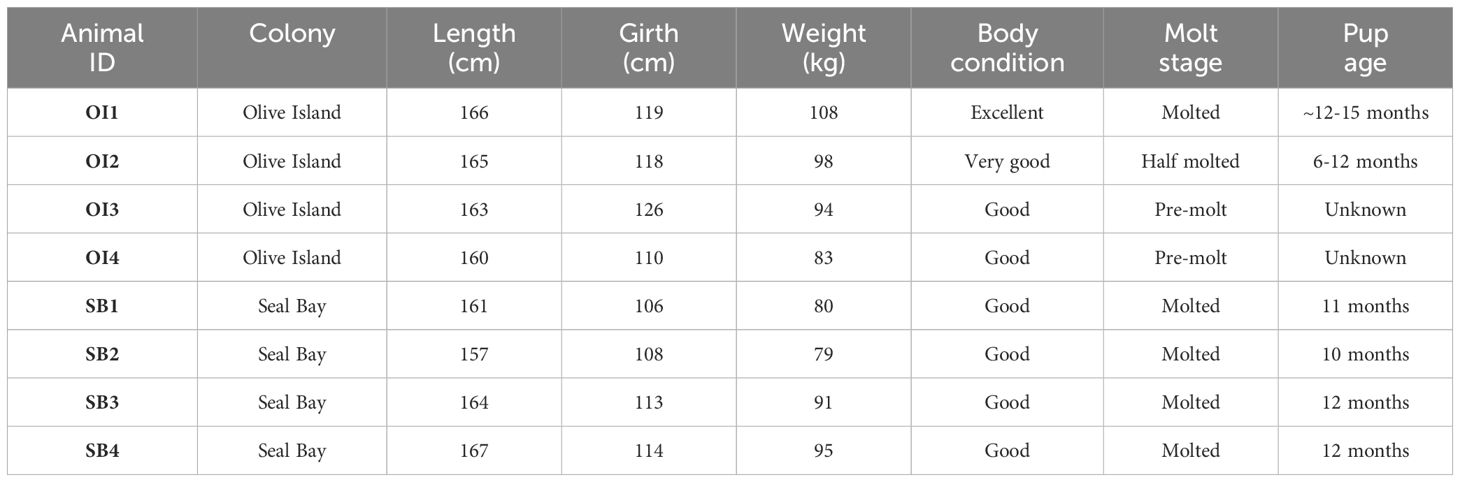
Table 1 Morphometric, condition, and reproductive data (at deployment) for eight adult female Australian sea lions from Olive Island (OI1, OI2, OI3, OI4) and Seal Bay (SB1, SB2, SB3, SB4) in South Australia.
Sea lions were sedated with Zoletil® (~1.3mg/kg, Virbac, Australia), administered intramuscularly via a syringe dart (Paxarms, 3.0ml syringe body with a 14-gauge 25mm barbed needle), delivered remotely by a dart gun (MK24c Projector, Paxarms). After a light level of sedation was attained (~10-15 minutes), sea lions could be approached, allowing application of an anesthetic mask over the muzzle. Sea lions were anesthetized using Isoflurane® (5% induction, 2-3% maintenance with medical-grade oxygen), for ~20 minutes, while instruments were attached. Isoflurane was delivered via a purpose-built gas anesthetic machine, using a Cyprane Tec III vaporizer (The Stinger™ Backpack anesthetic machine, Advanced Anaesthesia Specialists). Throughout anesthesia, vital signs of the sea lions were continuously monitored (e.g. respiratory rate, gum refill, and palpebral reflex), a pulse oximeter was also clipped to the tongue of anesthetized sea lions to monitor heart rate and blood oxygen levels. Following attachment of the instruments, sea lions were maintained on pure oxygen for several minutes until head/body movement indicated imminent recovery.
All biologging (animal-borne) instruments were pre-adhered to neoprene patches, that were then glued to the pelage (fur) on the dorsal midline of sea lions, using a two-part quick-setting epoxy (Selleys Araldite® 5 Minute Epoxy Adhesive). An archival animal-borne camera (Customized Animal Tracking Solutions, 135 x 96 x 40mm, 400g) was fitted to each sea lion, positioned at the base of the scapula, as well as an Argos-linked GPS logger with an integrated time-depth recorder (SPLASH-10, Wildlife Computers, 100 x 65 x 32mm, 200g), which was positioned posterior to the camera. In addition, a triaxial accelerometer/magnetometer (Axy-5 XS, TechnoSmArt, 28 x 12 x 9mm, 4g) was adhered to the crown of the head. Small, light, and low profile biologging instruments were used, where the combined weight of the instruments was <1% of the sea lions’ total body weight, to minimize drag impacts. Instrumented sea lions were recaptured after 2-10 days. Instruments were removed by cutting them from their neoprene patches to avoid damage to the pelage (the neoprene is shed during the subsequent molt).
2.2 Data collection and processing
High-definition color video (forward facing) was collected while sea lions were at sea, at depths greater than 5 meters, during daylight hours (0800-1800 local time). Batteries in the cameras allowed up to 12-13 hours of filming in total, which enabled the collection of video to be spread over 2-3 days of time spent at sea.
Satellite-linked GPS loggers collected Fastloc® locations when sea lions surfaced, by capturing a subsecond snapshot of signals from orbiting satellite constellations at two minute intervals (the minimum rate programmable). When dive durations exceeded two minutes, locations were sought when sea lions next surfaced. Locations obtained from four or fewer satellites were not included in analyses and erroneous locations (identified by unrealistic swimming speeds, >6ms−1) were removed, using a speed filter (McConnell et al., 1992; Sumner, 2011). Transmissions (including those of GPS location data) from the loggers were received and passed on by Argos systems on polar-orbiting satellites, allowing monitoring of each sea lion’s position in real time. Time-depth recorders measured depth every second.
Triaxial accelerometer/magnetometer data were used in combination with the GPS data to dead-reckon at-sea movement, using the methods outlined in Angelakis et al. (2023). Accelerometers measured head movement (G-force), for surge (anterior-posterior), sway (lateral) and heave (dorsal-ventral) axes at 25 Hz and 8-bit resolution (maximum and minimum acceleration value ±4G). Magnetometers measured the earth’s magnetic field in microteslas (µT) for roll (longitudinal), pitch (transverse) and yaw (vertical) axes at 2Hz.
2.3 Mapping of benthic habitats in southern Australia
Analysis of animal-borne video was conducted using the open source Behavioral Observation Research Interactive Software (version 7.12.2). A habitat key was used to classify benthic habitats (Figure 2), following the Collaborative and Annotation Tools for Analysis of Marine Imagery and Video classification scheme, which provides a national (Australian) framework for classifying marine biota and substrata (Althaus et al., 2013). The duration of time sea lions spent in different benthic habitats was recorded. All video analysis was performed by a single observer.
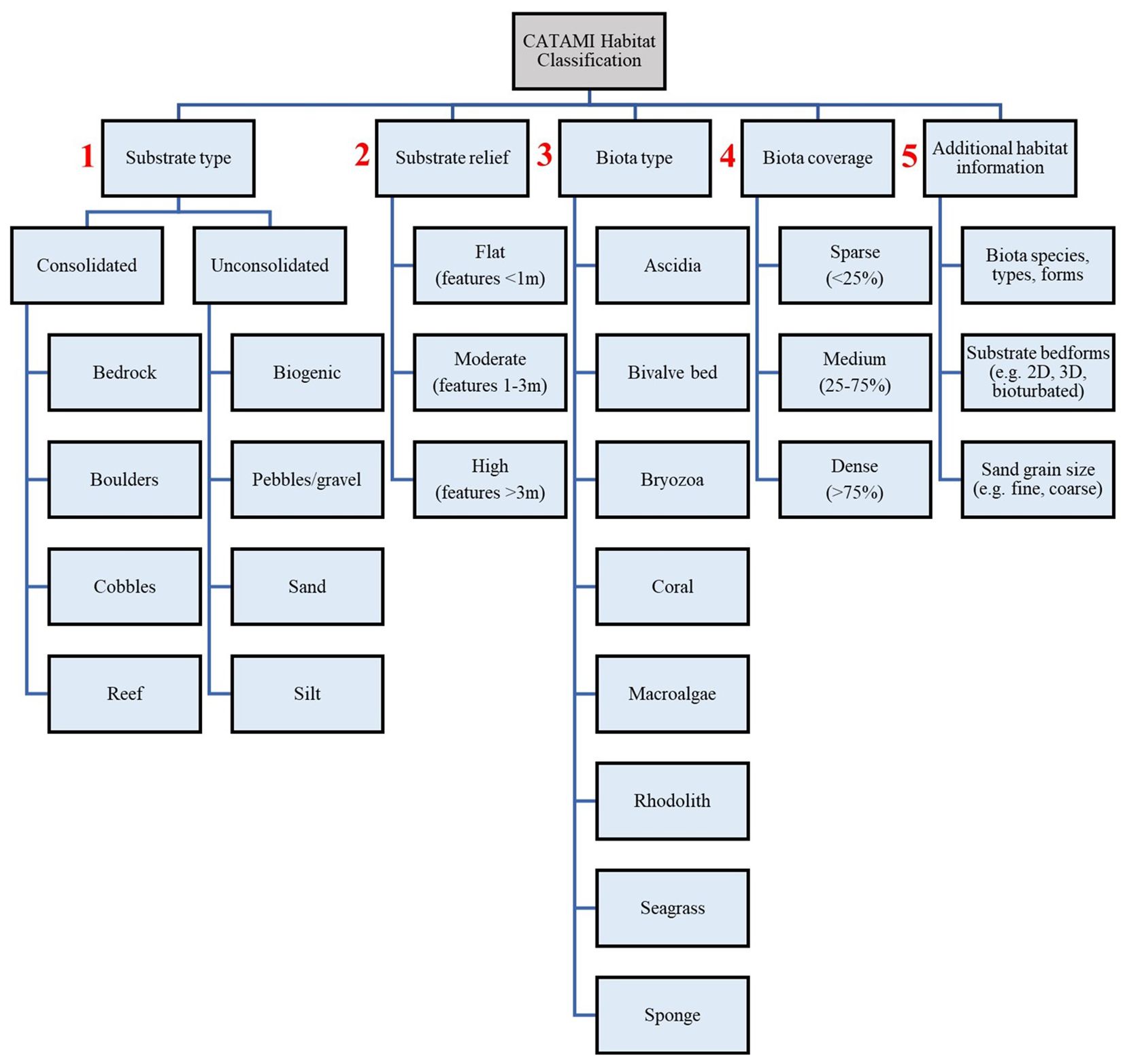
Figure 2 Habitat key used to classify benthic habitats, as identified from animal-borne video from adult female Australian sea lions. Numbers in red highlight the order of stages for habitat classification. Habitat classification was conducted in line with the Collaborative and Automated Tools for Analysis of Marine Imagery scheme.
Benthic habitat data for each sea lion were then georeferenced by time matching and amalgamation with their dead reckoned foraging paths. Georeferencing of the benthic habitat data enabled calculation of the distance travelled (km) in each habitat, from which, percent cover of different habitats could be quantified.
Georeferenced benthic habitat data were then spatially interpolated with available oceanographic/environmental data, to model benthic habitats on the continental shelf, across the sea lions’ foraging ranges from each colony, for Olive Island (32.550 to 32.850°S, 133.720 to 134.050°E, 1,023km2) and Seal Bay (35.980 to 36.800°S, 137.000 to 137.500°E, 4,004km2). The South Australian coast experiences significant coastal upwelling during the austral spring-autumn (November-May), which drives enhanced chlorophyll-a concentrations within the photic layer, leading to highly productive marine conditions (Kämpf et al., 2004; McClatchie et al., 2006; Middleton and Bye, 2007). Therefore, sea surface temperature and chlorophyll-a data were utilized to assess how they may drive the distribution of benthic habitats on the continental shelf in southern Australia. Sea surface temperature data (index), collected from polar-orbiting and geostationary satellites, were obtained from the National Aeronautics and Space Administration (NASA) Multiscale Ultrahigh Resolution Data (1km grid resolution) (Chin et al., 2017). Chlorophyll-a data (ocean color index) (Hu et al., 2012), also collected via satellites, were obtained from the National Oceanic and Atmospheric Administration (NOAA) Ocean Color Data (1km grid resolution). To model benthic habitats, we used long-term averaged sea surface temperature and chlorophyll-a data, over the previous ~21 years (between May 2002 and November 2023), across the two study regions. To assess how depth may drive the distribution of benthic habitats on the continental shelf, bathymetric data (m) were obtained from the General Bathymetric Chart of the Ocean (GEBCO) (15 arc-second grid resolution). Kriging was used to interpolate sea surface temperature, chlorophyll-a and bathymetry data across both study regions, using the gstat package in R (Pebesma and Graeler, 2015). This interpolation allowed the matching of data for each ‘presence’ location (where we had benthic habitat data), and each ‘absence’ location (where the benthic habitat was unknown) and scaled each predictor variable to the same spatial resolution, for all presences and absences. Additionally, for each presence and absence location, distance from the nearest coastline and distance from the continental slope (at the 200m depth contour), were calculated in R using the Haversine formula (Robusto, 1957), to assess how the distributions of these benthic habitats may be driven by the geomorphometry of the continental shelf.
Sea surface temperature, chlorophyll-a, bathymetry, distance from the coast and distance from the slope, were then used to predict benthic habitats for the study regions around Olive Island (1,023km2) and Seal Bay (4,004km2). Random Forest models (randomForest package in R) were chosen to predict benthic habitats, as they are suitable for modelling scenarios where variables have complex interactions and nonlinear relationships (Breiman, 2001; Liaw and Wiener, 2002) and thus are particularly useful for ecological studies. Random forests, which can be used for both regression and classification tasks, are widely used for habitat modelling (Juel et al., 2015; Rather et al., 2020; Shanley et al., 2021).
Firstly, individual random forest models for Olive Island and Seal Bay were validated by randomly subsetting the presence data into a ‘training’ dataset (using two-thirds of the presence data) and a ‘test’ dataset (using a third of the presence data). A confusion matrix was calculated to assess the predictive performance of both models (their accuracy in correctly classifying known habitats in their test datasets). Trained and tested random forest models were then used to predict benthic habitats for the absence data. The optimal number of classification trees (300), used in models, was identified by comparing mean squared error rates with an increasing number of classification trees, until error rates stabilized (Supplementary Figure 1). Random forest models were then ‘tuned’ using the tuneRF function in the randomForest package, which uses out-of-bag error estimates to find the optimal ‘mtry’ parameter entry (2), which represents the optimal number of features to consider at each ‘split’ in the model. Finally, models were cross validated, which tested their performance by iteratively reducing the number of predictor variables within the model, to find the optimal selection of parameters, determined by their mean squared error rates (Supplementary Figure 2). Variable importance in random forest models was assessed by the mean decrease in the Gini coefficient, which measured the influence of each predictor variable on the models’ ability to distinguish different benthic habitats (higher values indicating greater influence on the models’ benthic habitat predictions).
3 Results
3.1 Foraging paths and cover of benthic habitats
From the eight adult female Australian sea lions observed from Olive Island (OI1, OI2, OI3, OI4) and Seal Bay (SB1, SB2, SB3, SB4), a total of 89 hours and 9 minutes of animal-borne video from 1,935 dives was available for analysis. A summary of animal-borne video data available for each sea lion are provided in the Supplementary Material (Supplementary Table 1). Animal-borne video recorded a total of ~560km of the benthos (Olive Island= ~223km, Seal Bay= ~337km) (Figure 3) and captured benthic habitats at depths between 5-110m.
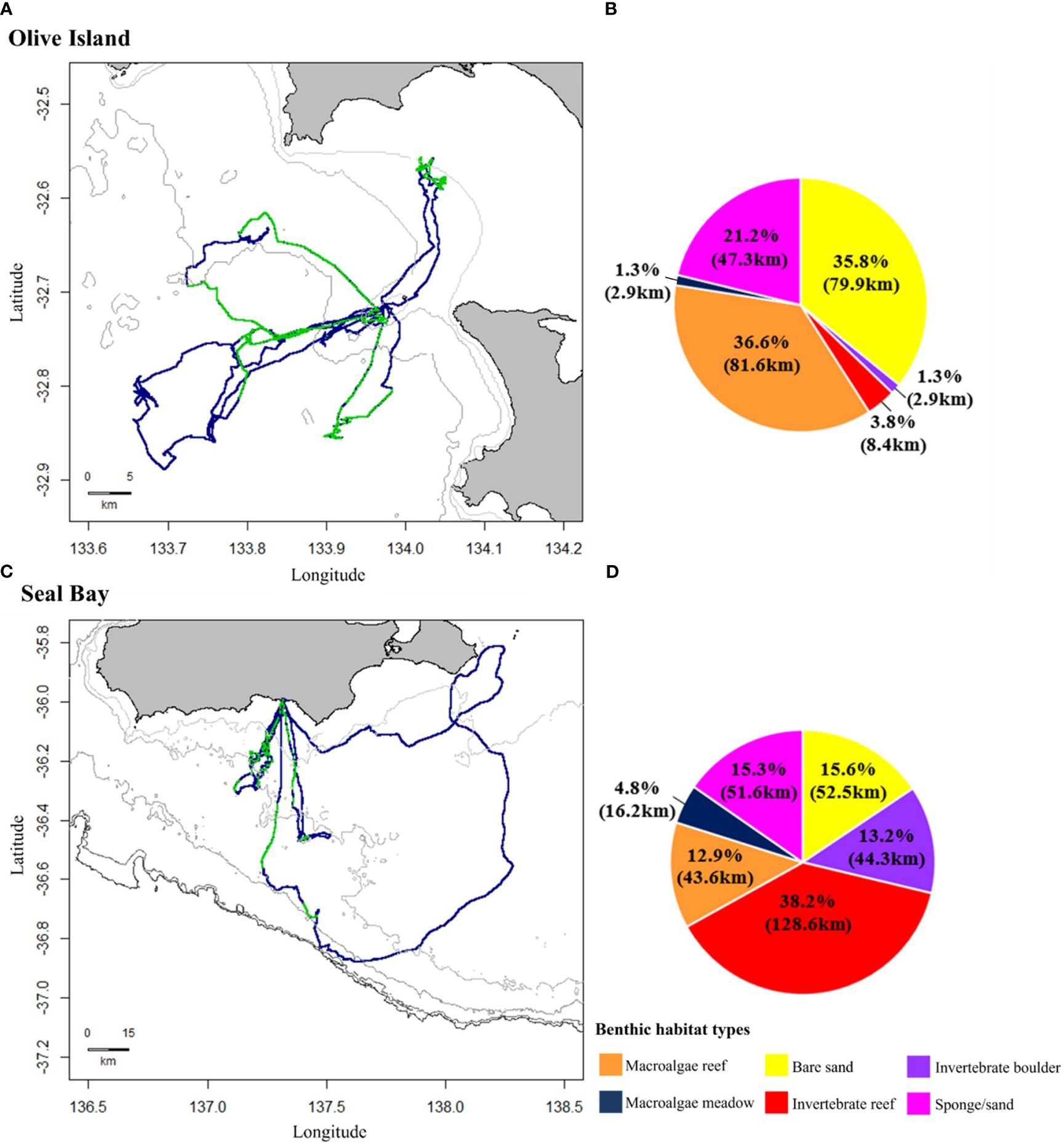
Figure 3 Movement and benthic habitat data from adult female Australian sea lions from Olive Island, western Eyre Peninsula (n= 4) and Seal Bay, Kangaroo Island (n= 4) in South Australia. Dead reckoned foraging paths represent at-sea movement in blue, with regions where animal-borne video data were available in green for sea lions from (A) Olive Island and (C) Seal Bay. Isobaths represent depth contours at 10, 25 and 50m for Olive Island (A) and 50, 75, 100, 150 and 200m for Seal Bay (C) (light to dark grey). Pie charts represent percent cover (km) of different benthic habitats on the foraging paths of sea lions from (B) Olive Island and (D) Seal Bay: macroalgae reef (orange), macroalgae meadow (navy), bare sand (yellow), invertebrate reef (red), invertebrate boulder (purple) and sponge/sand habitats (pink).
Six benthic habitats were identified from animal-borne video from Australian sea lions from Olive Island and Seal Bay: macroalgae reef, macroalgae meadow, bare sand, sponge/sand, invertebrate reef and invertebrate boulder habitats. Percent cover of these benthic habitats differed on the foraging paths of sea lions from Olive Island and Seal Bay (Figure 3). For sea lions from Olive Island, macroalgae reef (36.6%, 81.6km), bare sand (35.8%, 79.9km) and sponge/sand habitats (21.2%, 47.3km) accounted for most of the habitat cover (Figure 3B). For sea lions from Seal Bay, invertebrate reef (38.2%, 128.6km), bare sand (15.6%, 52.5km), sponge/sand (15.3%, 51.6km) and invertebrate boulder habitats (13.2%, 44.3km) accounted for most of the habitat cover (Figure 3D).
Of the macroalgae habitats, many of the reef environments were dominated by Ecklonia radiata (golden kelp) (Figure 4), other macroalgae habitats consisted of varying assemblages of different brown, red and green algae taxa, such as Sargassum, Cystophora, Plocamium and Ulva species. Sponge/sand habitats were dominated by Demospongiae sponges, such as Callyspongia and Echinodictyum species (Figure 4). Invertebrate reef and boulder habitats were also dominated by Demospongiae sponges, as well as bryozoans such as Phidoloporidae (lace coral) species, ascidians from Phlebobranchia and the Pyura genus (sea tulips) and soft corals from Alcyonacea (gorgonian species) and the Dendronephythya genus (Figure 4).
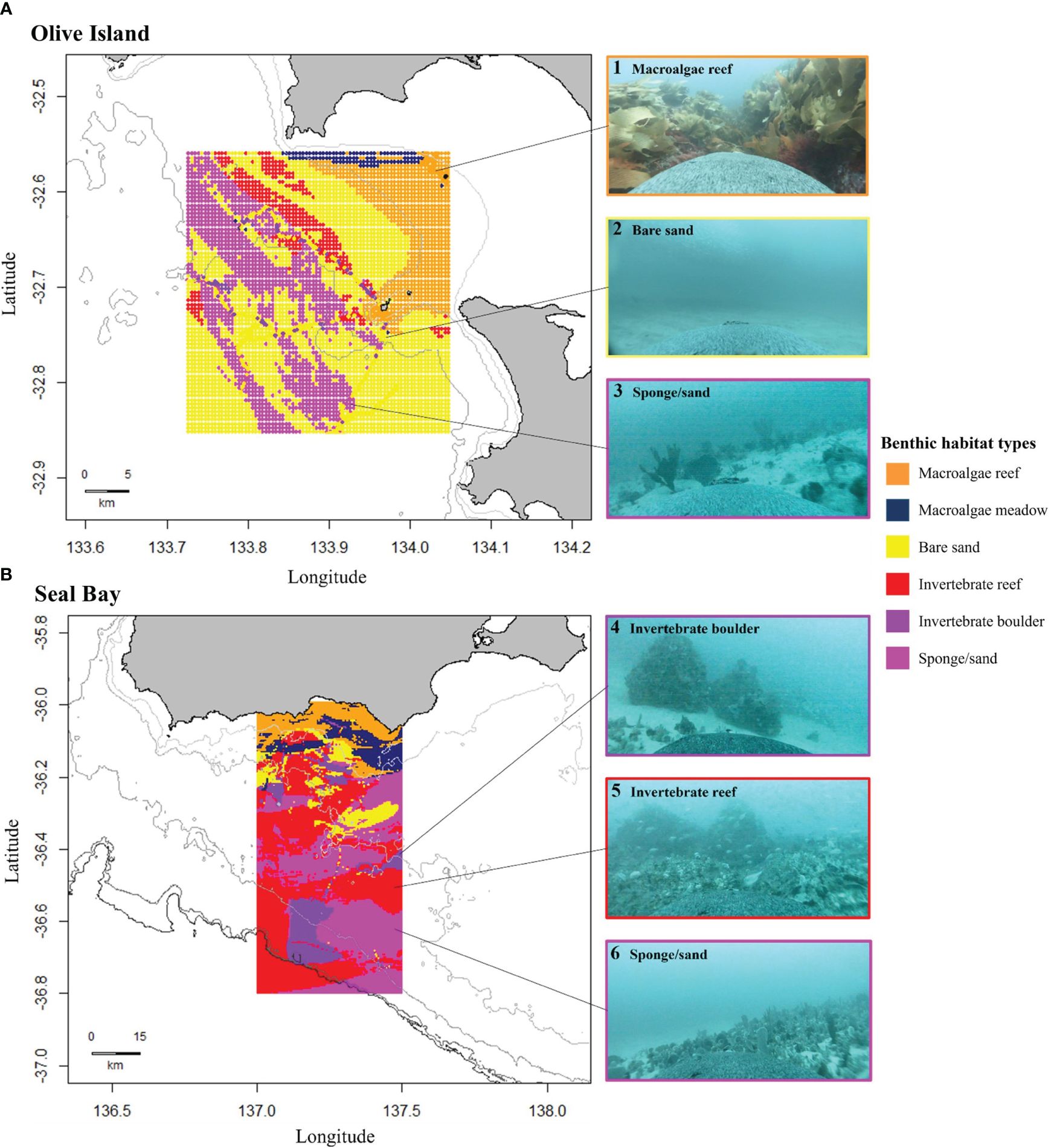
Figure 4 Modelled distributions of benthic habitats for (A) Olive Island, western Eyre Peninsula, and (B) Seal Bay, Kangaroo Island in South Australia. Maps show predicted distributions of benthic habitats from random forest modelling of animal-borne video data from adult female Australian sea lions (n= 8). Habitat distributions are: macroalgae reef (orange), macroalgae meadow (navy), bare sand (yellow), invertebrate reef (red), invertebrate boulder (purple) and sponge/sand (pink) habitats. Isobaths represent depth contours at 10, 25 and 50m for Olive Island (A) and 50, 75, 100, 150 and 200m for Seal Bay (B). Examples of captured images are: 1) macroalgae reef, 2) bare sand, 3 and 6) sponge/sand, 4) invertebrate boulder and 5) invertebrate reef habitats.
The percent cover of flat (features <1m), moderate (features 1-3m) and high relief reefs (features >3m) differed between Olive Island and Seal Bay (Supplementary Figure 3). Additionally, the percent biota cover of sparse (<25% cover), medium (25-75% cover) and dense (>75% cover) macroalgae, invertebrate and sponge habitats also differed between Olive Island and Seal Bay (Supplementary Figure 3).
3.2 Predicting distributions of benthic habitats
A trained random forest model for Olive Island predicted benthic habitats on a test dataset with a 99.5% accuracy rate (out-of-bag error rate= 0.5%) and for Seal Bay, benthic habitats were predicted at a 98.6% accuracy rate (out-of-bag error rate= 1.4%). Both random forest models for Olive Island and Seal Bay showed high precision when predicting across all identified benthic habitats (Supplementary Table 2). For Olive Island and Seal Bay, prediction accuracies were highest when all five predictor variables (sea surface temperature, chlorophyll-a, bathymetry, distance from the coast and distance from the continental slope), were included in their models (Supplementary Figure 2).
Predicted benthic habitats varied between the regions around Olive Island and Seal Bay (Figure 4). For Olive Island, macroalgae reefs were predicted for inshore waters to the northeast, constituting most of the predicted habitat at depths shallower than ~25-30m (Figure 4A). Bare sand and sponge/sand habitats were predicted as the dominant habitats at depths greater than ~25-30m, with smaller areas of invertebrate reefs, mostly predicted to the northwest of Olive Island (Figure 4A). For Seal Bay, macroalgae reef and macroalgae meadow habitats were predicted as the dominant benthic habitats at depths shallower than ~50-60m (Figure 4B). For depths greater than ~50-60m, sponge/sand and invertebrate reef habitats were the dominant predicted habitats, with smaller areas of bare sand and invertebrate boulder habitats south of Seal Bay (Figure 4B). For Olive Island, these habitats also appeared to have a distinct southeast-northwest orientation, corresponding with local bathymetry and/or the aspect of the continental slope (Figure 4A).
For Olive Island, a random forest model showed that chlorophyll-a and distance from the continental slope were the most important variables for predicting benthic habitat (mean decrease in the Gini coefficient= 31280.28 and 23514.66 respectively) (Figure 5A). For Seal Bay, distance from the coast and chlorophyll-a were the most important variables for predicting benthic habitat (mean decrease in the Gini coefficient= 18136.47 and 18019.99 respectively) (Figure 5B).
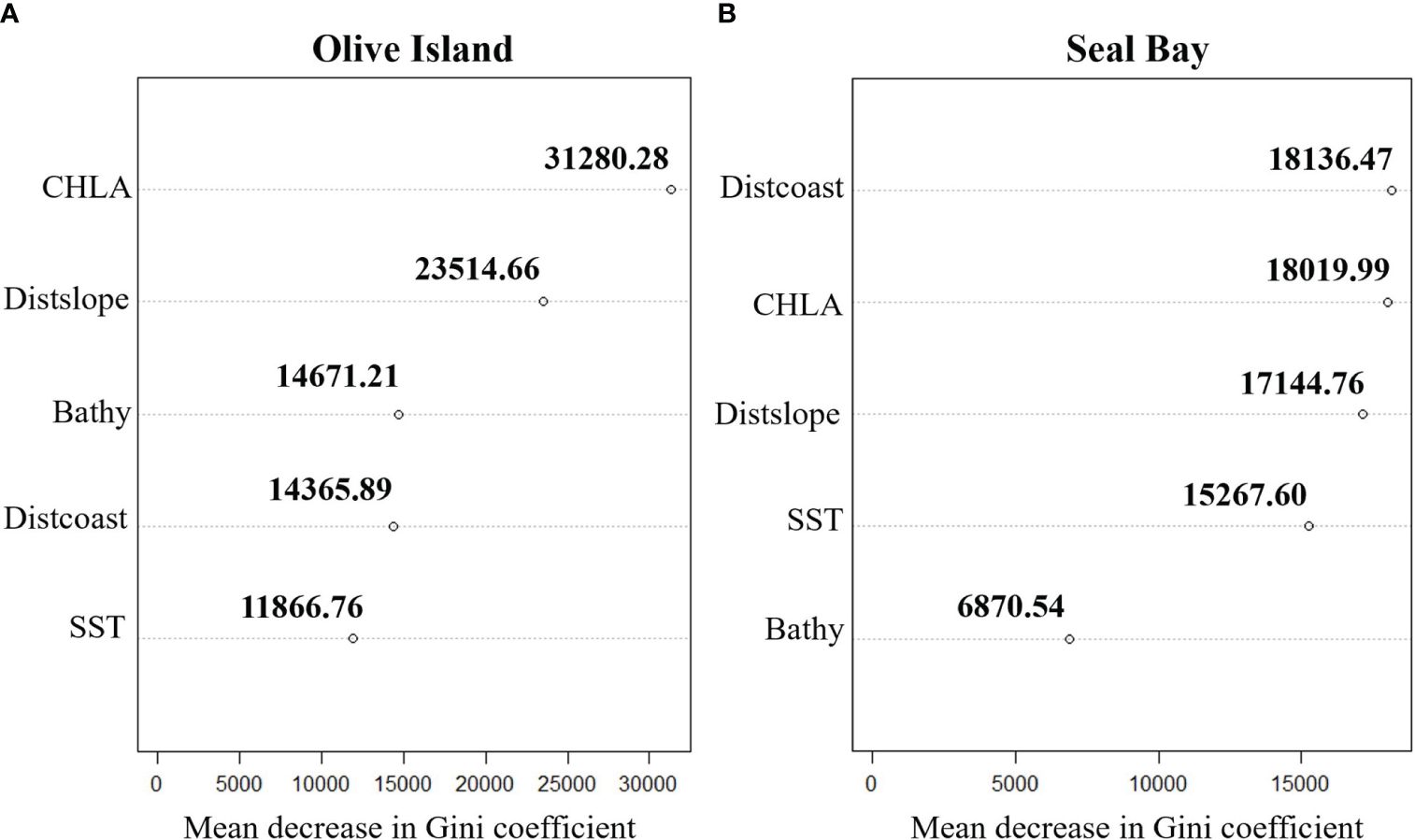
Figure 5 Cleveland dot plots highlighting the importance of predictor variables: chlorophyll-a (CHLA), distance from the continental slope (Distslope), bathymetry (Bathy), distance from the coastline (Distcoast) and sea surface temperature (SST) in random forest models for predicting benthic habitats for (A) Olive Island and (B) Seal Bay in South Australia. Mean decrease in Gini coefficient values represent the importance of each predictor variable in random forest models, where higher values indicate a greater importance in predicting benthic habitats.
4 Discussion
4.1 Distribution and structure of benthic habitats in South Australia
In this study, benthic habitat data collected from animal-borne video was used in a random forest model to predict the spatial distribution of diverse benthic habitats on the continental shelf in southern Australia. From these sea lions, six benthic habitats (between 5-110m depth) were identified at Olive Island and Seal Bay: macroalgae reef, macroalgae meadow, bare sand, sponge/sand, invertebrate reef and invertebrate boulder habitats. Random forest models predicted that large regions of the continental shelf in southern Australia are covered by invertebrate reef, bare sand and sponge/sand habitats. Animal-borne video and movement data from Australian sea lions was also useful in locating reefs, highlighting significant high relief reef systems, for example, the area at 36.100 to 36.300°S and 137.170 to 137.280°E, south of Kangaroo Island (Figure 6).
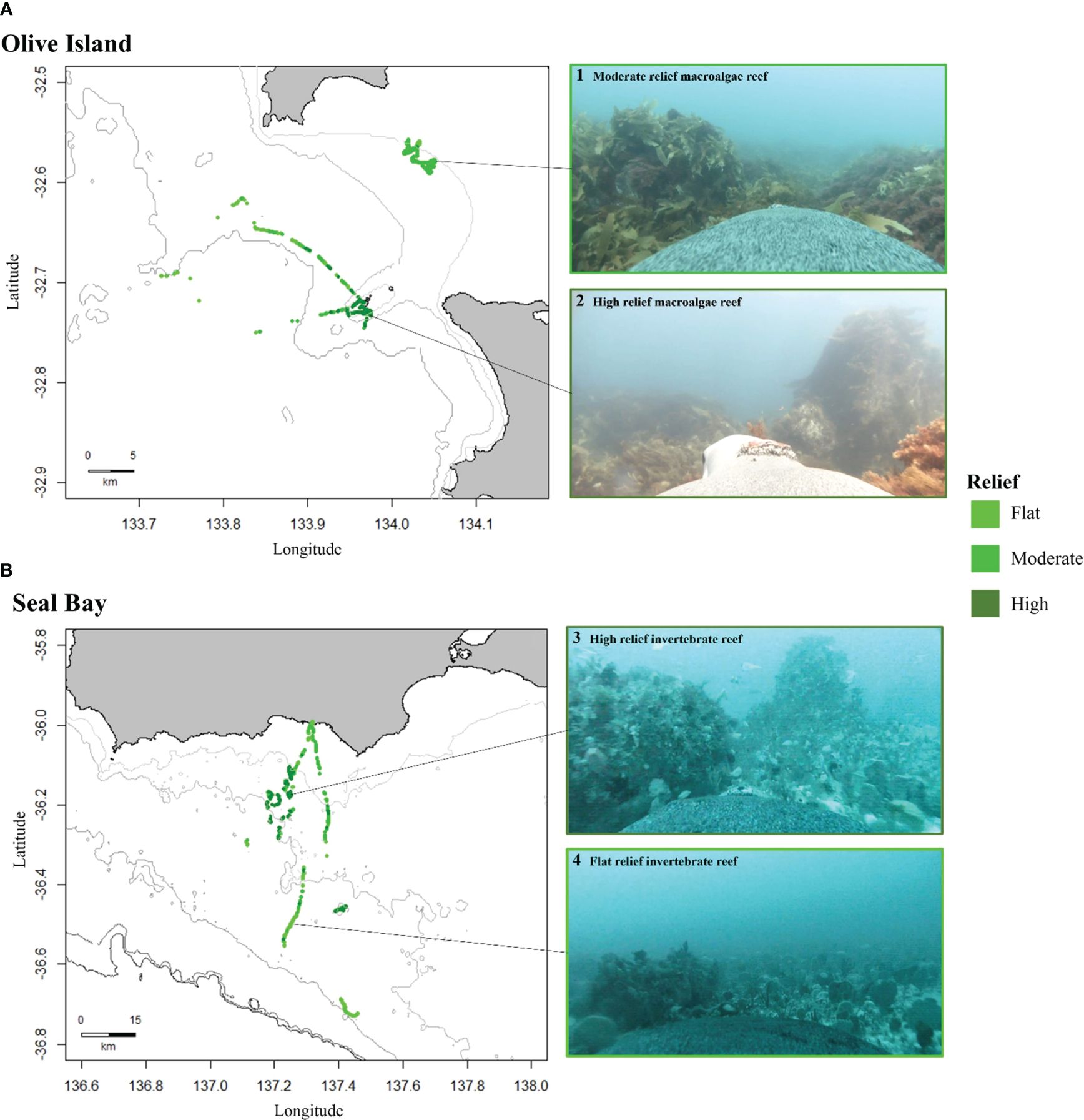
Figure 6 Distribution of reef habitats for (A) Olive Island, western Eyre Peninsula, and (B) Seal Bay, Kangaroo Island in South Australia. Maps show the distribution of flat (features <1m, light green), moderate (features 1-3m, green) and high relief reefs (features >3m, dark green), as identified from animal-borne video data from adult female Australian sea lions (n= 8). Isobaths represent depth contours at 10, 25 and 50m for Olive Island (A) and 50, 75, 100, 150 and 200m for Seal Bay (B) (light to dark grey). Examples of captured images are: 1) moderate relief macroalgae reef, 2) high relief macroalgae reef, 3) high relief invertebrate reef and 4) flat relief invertebrate reef.
The habitat assemblages identified in this study differ from other regions in South Australia where benthic habitats have been mapped across broad spatial scales, which has been restricted to the sheltered embayments of its two gulfs. Bare sand plains and seagrass meadows cover large areas of the Gulf St. Vincent (Shepherd and Sprigg, 1976; Tanner, 2005), and sediment surveys have inferred that a combination of seagrass meadows, sand and gravel plains and rhodolith pavements are prevalent in the Spencer Gulf (O'Connell et al., 2016). In this study of continental shelf waters, particular benthic habitats like seagrass meadows (such as Posidonia and Amphibolis sp.) were not observed. However, animal-borne video has previously identified seagrass meadows as foraging habitat for Australian sea lions from Dangerous Reef in the southern Spencer Gulf (Angelakis, in review). Light penetration, water depth, wave energy and turbidity in the high energy waters around Olive Island and Seal Bay, are all factors which likely explain the apparent absence of seagrass habitat in these regions (Shepherd and Sprigg, 1976; Tanner, 2005; O'Connell et al., 2016). However, some inherent spatial biases may exist with the data presented in this study, as Australian sea lions may prefer particular benthic habitats over others. Therefore, other benthic habitats may occupy these regions but were not observed in the video data if sea lions did not target them or transit over them and hence were not accounted for in random forest models.
4.2 Environmental drivers of benthic habitat
We found that invertebrate communities dominated depths where macroalgae reefs and macroalgae meadows were absent. The sponge, bryozoan, ascidian and soft coral taxa identified in this study align with those taxa previously described in the region (Sorokin et al., 2007; Sorokin and Currie, 2008; Burnell et al., 2015). In the Great Australian Bight, benthic habitat surveys have identified invertebrate communities with a diverse range of sponge, ascidian, and bryozoan species (McLeay et al., 2003; Ward et al., 2006a; Sorokin et al., 2007). The distribution and structure of these invertebrate communities is likely influenced by a range of environmental factors, including nutrient supply, bathymetry, substrate availability, seawater conditions and hydrodynamics (Ward et al., 2006; Currie et al., 2009; James and Bone, 2010; Przeslawski et al., 2011). The environmental variables used to predict benthic habitats in this study, potentially provide insights into the suite of oceanographic processes driving the distribution and structure of these invertebrate communities.
Nutrient supply is key for supporting filter-feeding benthic invertebrates (Ward et al., 2006. 2006a; Middleton et al., 2014). During the austral spring-autumn (November-May), South Australia experiences extensive coastal upwelling of cold nutrient-rich waters, which drive enhanced chlorophyll-a concentrations within the photic layer, leading to highly productive marine conditions (Kämpf et al., 2004; McClatchie et al., 2006; Middleton and Bye, 2007). Therefore, the large sponge, ascidian, bryozoan and soft coral communities identified here, may be supported in part by strong seasonal upwelling conditions, where there is an enhanced supply of nutrients to the benthos (James et al., 2001; James and Bone, 2010; Middleton et al., 2014). Changes in water circulation across southern Australia, due to temporal shifts in current patterns, outflows from gulf waters, eddies and salinity and temperature fronts, also drive nutrient transport (James et al., 2001; Middleton and Bye, 2007; van Ruth et al., 2018). The distribution of invertebrate communities across southern Australia is therefore likely influenced by a range of complex and highly dynamic oceanographic processes, that drive the supply of nutrients and trophic resources to different areas and habitats. In random forest models for both Olive Island and Seal Bay, chlorophyll-a was one of the most important variables for predicting benthic habitat, supporting the notion that supply of nutrients/trophic resources is one key determinant of benthic habitats at depth.
Conversely, some regions of the continental shelf, observed in this study, were dominated by bare sand plains. These regions may represent areas where benthic environments receive less nutrient input (Middleton et al., 2014; Menge et al., 2019), or are subject to regular swell/current impacts, compared with benthic habitats dominated by diverse sponge, ascidian, bryozoan and soft coral communities. Presumably, substrate availability (James et al., 2001), turbidity, swell/current action (Ward et al., 2006a) and seawater conditions (Middleton et al., 2014) are also critical in determining suitable areas where sessile invertebrates can establish. In this study, fine sand (no shell fragments) and coarse sand (with shell fragments) (Althaus et al., 2013), dominated sediment composition. The absence of mud/silt sediments is likely due to the high level of exposure and water movement on the continental shelf (James et al., 2001; Currie et al., 2009), at depths where the sea lions foraged at (<110m). This aligns with data for the Great Australian Bight, where coarser sediments dominated shallower waters (Ward et al., 2006a; Currie et al., 2009) and mud sediments dominated depths below 150m, where direct influences from swell and current action are much smaller (James et al., 2001; Currie et al., 2009). A better understanding of how different oceanographic and environmental processes influence the structure and distribution of benthic habitats will be key for future research and habitat assessment.
4.3 Future applications
Using animal-borne video to explore and map benthic habitats provides information that complements traditional benthic survey methods, such as ROV, towed and drop camera deployments, acoustic mapping, and sled and grab sampling (Ward et al., 2006a; Kostylev, 2012; López-Garrido et al., 2020). Animal-borne video from a benthic foraging marine mammal, such as the Australian sea lion, provides an efficient and cost-effective method for mapping and surveying benthic habitats, particularly for those at depths that are expensive, difficult, and impossible to access by more conventional survey approaches. Animal-borne video highlights the ecological value of different habitats from a predator’s perspective, valuable sea lion habitat may also highlight ecologically important areas more broadly. In future, combining animal-borne video with data from these existing survey methods will support a more comprehensive understanding of benthic habitats, and the species that use them. Furthermore, future animal-borne camera deployments on Australian sea lions, which expand the spatial extent of available benthic habitat data, will enhance the robustness and generalizability of the models developed in this study. Benthic habitats can be significantly altered by human activity (Brown et al., 2017; Sweetman et al., 2017; Yoklavich et al., 2018). In southern Australia, benthic habitats have undergone major changes, since the arrival of Europeans, from land-based release of nutrients, and from the impacts of fisheries (Tanner, 2005; Bryars and Rowling, 2009; Gorman et al., 2009; Alleway and Connell, 2015). Yet, our knowledge of benthic habitats, including how quickly they may recover from degradation, is poorly understood. The benthic habitats in southern Australia are highly diverse and endemic, with many local undescribed taxa (Edyvane, 1999; McLeay et al., 2003; Currie et al., 2009). Across southern Australia, large spatially connected temperate reef systems have been identified, which support extensive kelp and fucoid forests and diverse benthic invertebrate communities that have significant ecological, social and economic importance (Bennett et al., 2015; Coleman and Wernberg, 2017; Wong et al., 2023). Considering the gaps in our knowledge around benthic habitats (Mayer et al., 2018; Menandro and Bastos, 2020) and the human impacts on them (Tanner, 2005; Bryars and Rowling, 2009; Gorman et al., 2009; Alleway and Connell, 2015), there is a necessity to better understand their structure and distribution, throughout southern Australia and globally. Where such information has been gathered, it has led to better policies and investment to recover these habitats and the economies and social benefits derived from them (Gorman et al., 2009; McAfee et al., 2020). In South Australia, an improved knowledge of benthic habitats can support wide-ranging fields of marine science, from improving placement of marine reserves, habitat restoration planning and management of endangered species, such as the Australian sea lion.
4.4 Conclusions
This study presents novel findings on previously unmapped areas of the continental shelf in southern Australia. Random forest models demonstrated strong performance in predicting diverse benthic habitats across extensive regions of the continental shelf. To ground truth these models, predicted habitats in the absence locations could be compared with future benthic habitat data collected from various sources, such as animal-borne video, ROVs, towed and drop cameras and autonomous underwater vehicles (AUVs), provided there is spatial overlap. This research highlights the utility of random forest models in mapping and predicting habitats observed through animal-borne video, particularly those associated with benthic predators such as the Australian sea lion. Furthermore, this study highlights the value of ancillary data collected from animal-borne video, beyond solely investigating animal behavior, and illustrates how future research could repurpose such data in novel ways, to address important research objectives in the marine environment.
Data availability statement
The datasets presented in this study can be found in online repositories. The names of the repository/repositories and accession number(s) can be found below: https://metadata.imas.utas.edu.au/geonetwork/srv/eng/catalog.search#/metadata/84cb1709-a669-4f2c-b97b-5eceb7929349.
Ethics statement
The animal study was approved by The University of Adelaide Animal Ethics Committee (#S-2021-001), Primary Industries and Regions South Australia Animal Ethics Committee (#16/20), and the Department for Environment and Water (Permit/Licence to Undertake Scientific Research #A24684-22/23 and Marine Parks Permit to Undertake Scientific Research #MR00071-7-R). The study was conducted in accordance with the local legislation and institutional requirements.
Author contributions
NA: Conceptualization, Data curation, Formal analysis, Funding acquisition, Investigation, Methodology, Project administration, Validation, Visualization, Writing – original draft, Writing – review & editing. GG: Conceptualization, Formal analysis, Methodology, Visualization, Writing – review & editing, Investigation. SC: Conceptualization, Formal analysis, Methodology, Project administration, Supervision, Validation, Writing – review & editing. FB: Conceptualization, Formal analysis, Methodology, Visualization, Writing – review & editing. LD: Formal analysis, Investigation, Methodology, Validation, Visualization, Writing – review & editing. RK: Data curation, Visualization, Writing – review & editing. DH: Data curation, Investigation, Resources, Writing – review & editing. SG: Data curation, Funding acquisition, Project administration, Supervision, Writing – review & editing.
Funding
The author(s) declare financial support was received for the research, authorship, and/or publication of this article. Research for this manuscript was funded by the Australian Government under the National Environmental Science Program (NESP), Marine and Coastal Hub (Project 2.6, Mapping critical Australian sea lion habitat to assess ecological value and risks to population recovery). Additional operating costs were funded by the Ecological Society of Australia under the Holsworth Wildlife Research Endowment, awarded to Nathan Angelakis (006010901).
Acknowledgments
We would like to acknowledge Mel Stonnill, Ashleigh Wycherley and the Department for Environment and Water (DEW) staff at Seal Bay Conservation Park and the staff of the Kangaroo Island Veterinary Clinic. Thanks are also extended to Carey Kuhn (National Oceanic and Atmospheric Administration NOAA), Hugo Oliveira de Bastos (SARDI Aquatic Sciences), Dale Furley (deceased) and the staff of the Far-West Coast Aboriginal Corporation (FWCAC), Tobin Woolford (Eyrewoolf Abalone) and Bec Souter. We also thank SARDI Aquatic Sciences and The University of Adelaide for their continued support.
Conflict of interest
The authors declare that the research was conducted in the absence of any commercial or financial relationships that could be construed as a potential conflict of interest.
Publisher’s note
All claims expressed in this article are solely those of the authors and do not necessarily represent those of their affiliated organizations, or those of the publisher, the editors and the reviewers. Any product that may be evaluated in this article, or claim that may be made by its manufacturer, is not guaranteed or endorsed by the publisher.
Supplementary material
The Supplementary Material for this article can be found online at: https://www.frontiersin.org/articles/10.3389/fmars.2024.1425554/full#supplementary-material
References
Alleway H. K., Connell S. D. (2015). Loss of an ecological baseline through the eradication of oyster reefs from coastal ecosystems and human memory. Conserv. Biol. 29 (3), 795–804. doi: 10.1111/cobi.12452
Althaus F., Hill N., Edwards L., Ferrari R., Case M., Colquhoun J., et al. (2013). CATAMI Classification Scheme for scoring marine biota and substrata in underwater imagery—A pictorial guide to the Collaborative and Annotation Tools for Analysis of Marine Imagery and Video (CATAMI) classification scheme. Version 1.3.
Angelakis N., Goldsworthy S. D., Connell S. D., Durante L. M. (2023). A novel method for identifying fine-scale bottom-use in a benthic-foraging pinniped. Movement Ecol. 11, 1–11. doi: 10.1186/s40462-023-00386-1
Bennett S., Wernberg T., Connell S. D., Hobday A. J., Johnson C. R., Poloczanska E. S. (2015). The ‘Great Southern Reef’: social, ecological and economic value of Australia’s neglected kelp forests. Mar. Freshw. Res. 67, 47–56. doi: 10.1071/MF15232
Berry T. E., Osterrieder S. K., Murray D. C., Coghlan M. L., Richardson A. J., Grealy A. K., et al. (2017). DNA metabarcoding for diet analysis and biodiversity: A case study using the endangered Australian sea lion (Neophoca cinerea). Ecol. Evol. 7 (14), 5435–5453. doi: 10.1002/ece3.3123
Brown K. T., Bender-Champ D., Bryant D. E., Dove S., Hoegh-Guldberg O. (2017). Human activities influence benthic community structure and the composition of the coral-algal interactions in the central Maldives. J. Exp. Mar. Biol. Ecol. 497, 33–40. doi: 10.1016/j.jembe.2017.09.006
Bryars S., Rowling K. (2009). Benthic habitats of eastern Gulf St Vincent: major changes in benthic cover and composition following European settlement of Adelaide. Trans. R. Soc. S. Aust. 133 (2), 318–338.
Burnell O., Barrett S., Hooper G., Beckmann C., Sorokin S., Noell C. (2015). Report to PIRSA Fisheries and Aquaculture (South Australian Research and Development Institute (Aquatic Sciences), Adelaide. SARDI Publication No. F2015/ 000414-1. SARDI Research Report Series No. 860). Spatial and temporal reassessment of by-catch in the Spencer Gulf prawn fishery.
Button R. E., Parker D., Coetzee V., Samaai T., Palmer R. M., Sink K., et al. (2021). ROV assessment of mesophotic fish and associated habitats across the continental shelf of the Amathole region. Sci. Rep. 11 (1), 18171. doi: 10.1038/s41598-021-97369-2
Chapple T. K., Tickler D., Roche R. C., Bayley D. T., Gleiss A. C., Kanive P. E., et al. (2021). Ancillary data from animal-borne cameras as an ecological survey tool for marine communities. Mar. Biol. 168 (7), 106. doi: 10.1007/s00227-021-03916-w
Chin T. M., Vazquez-Cuervo J., Armstrong E. M. (2017). A multi-scale high-resolution analysis of global sea surface temperature. Remote Sens. Environ. 200, 154–169. doi: 10.1016/j.rse.2017.07.029
Coleman M. A., Wernberg T. (2017). Forgotten underwater forests: The key role of fucoids on Australian temperate reefs. Ecol. Evol. 7, 8406–8418. doi: 10.1002/ece3.3279
Connell S. D., Russell B. D., Turner D. J., Shepherd S. A., Kildea T., Miller D., et al. (2008). Recovering a lost baseline: missing kelp forests from a metropolitan coast. Mar. Ecol. Prog. Ser. 360, 63–72. doi: 10.3354/meps07526
Costa D. P., Gales N. J. (2003). Energetics of a benthic diver: seasonal foraging ecology of the Australian sea lion, Neophoca cinerea. Ecol. Monogr. 73 (1), 27–43. doi: 10.1890/0012-9615(2003)073[0027:EOABDS]2.0.CO;2
Currie D. R., Sorokin S. J., Ward T. M. (2009). Infaunal macroinvertebrate assemblages of the eastern Great Australian Bight: effectiveness of a marine protected area in representing the region’s benthic biodiversity. Mar. Freshw. Res. 60 (5), 459–474. doi: 10.1071/MF08239
Currie D. R., Ward T. M., Sorokin S. J. (2007). Infaunal assemblages of the eastern Great Australian Bight: Effectiveness of a Benthic Protection Zone in representing regional biodiversity (South Australian Research and Development Institute (Aquatic Sciences), Adelaide. SARDI Publication No. F2007/ 001079-1. SARDI Research Report Series No. 250).
Diaz R. J., Solan M., Valente R. M. (2004). A review of approaches for classifying benthic habitats and evaluating habitat quality. J. Environ. Manage. 73, 165–181. doi: 10.1016/j.jenvman.2004.06.004
Edyvane K. S. (1999). Conserving Marine Biodiversity in South Australia - Part 1 - Background, status and review of approach to marine biodiversity conservation in South Australia (South Australian Research and Development Institute. SARDI Research Report Series No. 38).
Fowler S. L., Costa D. P., Arnould J. P., Gales N. J., Kuhn C. E. (2006). Ontogeny of diving behaviour in the Australian sea lion: trials of adolescence in a late bloomer. J. Anim. Ecol. 75 (2), 358–367. doi: 10.1111/j.1365-2656.2006.01055.x
Gallagher A. J., Brownscombe J. W., Alsudairy N. A., Casagrande A. B., Fu C., Harding L., et al. (2022). Tiger sharks support the characterization of the world’s largest seagrass ecosystem. Nat. Commun. 13, 6328. doi: 10.1038/s41467-022-33926-1
Goldsworthy S. D. (2015). “Neophoca cinerea,” in The IUCN Red List of Threatened Species. doi: 10.2305/IUCN.UK.2015-2.RLTS.T14549A45228341.en
Goldsworthy S. D., Bailleul F., Nursey-Bray M., Mackay A. I., Oxley A., Reinhold S.-L., et al. (2019). Assessment of the impacts of seal populations on the seafood industry in South Australia (South Australian Research and Development Institute (Aquatic Sciences), Adelaide, June).
Goldsworthy S. D., Hamer D. J., Page B. (2007). Assessment of the implications of interactions between fur seals and sea lions and the southern rock lobster and gillnet sector of the Southern and Eastern Scalefish and Shark Fishery (SESSF) in South Australia (South Australian Research and Development Institute (Aquatic Sciences), Adelaide. SARDI Publication No. F2007/000711. SARDI Research Report Series No. 225).
Goldsworthy S. D., Page B., Hamer D. J., Lowther A. D., Shaughnessy P. D., Hindell M. A., et al. (2022). Assessment of Australian sea lion bycatch mortality in a gillnet fishery, and implementation and evaluation of an effective mitigation strategy. Front. Mar. Sci. 9, 799102. doi: 10.3389/fmars.2022.799102
Goldsworthy S. D., Shaughnessy P. D., Mackay A. I., Bailleul F., Holman D., Lowther A. D., et al. (2021). Assessment of the status and trends in abundance of a coastal pinniped, the Australian sea lion. Neophoca cinerea. Endang. Species Res. 44, 421–437. doi: 10.3354/esr01118
Gorman D., Russell B. D., Connell S. D. (2009). Land-to-sea connectivity: linking human-derived terrestrial subsidies to subtidal habitat change on open rocky coasts. Ecol. Appl. 19, 1114–1126. doi: 10.1890/08-0831.1
Hu C., Lee Z., Franz B. (2012). Chlorophyll a algorithms for oligotrophic oceans: A novel approach based on three-band reflectance difference. J. Geophys. Res. Oceans 117, C1. doi: 10.1029/2011JC007395
James N. P., Bone Y. (2010). Neritic carbonate sediments in a temperate realm: Southern Australia. Springer Science & Business Media. doi: 10.1007/978-90-481-9289-2
James N. P., Bone Y., Collins L. B., Kyser T. K. (2001). Surficial sediments of the Great Australian Bight: facies dynamics and oceanography on a vast cool-water carbonate shelf. J. Sediment. Res. 71 (4), 549–567. doi: 10.1306/102000710549
Jewell O. J., Gleiss A. C., Jorgensen S. J., Andrzejaczek S., Moxley J. H., Beatty S. J., et al. (2019). Cryptic habitat use of white sharks in kelp forest revealed by animal-borne video. Biol. Lett. 15 (4), 20190085. doi: 10.1098/rsbl.2019.0085
Juel A., Groom G. B., Svenning J.-C., Ejrnaes R. (2015). Spatial application of Random Forest models for fine-scale coastal vegetation classification using object based analysis of aerial orthophoto and DEM data. Int. J. Appl. Earth Obs. Geoinf. 42, 106–114. doi: 10.1016/j.jag.2015.05.008
Kämpf J., Doubell M., Griffin D., Matthews R. L., Ward T. M. (2004). Evidence of a large seasonal coastal upwelling system along the southern shelf of Australia. Geophys. Res. Lett. 31, 9. doi: 10.1029/2003GL019221
Kostylev V. E. (2012). Benthic habitat mapping from seabed acoustic surveys: do implicit assumptions hold? Sediments Morphology Sedimentary Processes Continental Shelves: Adv. Technologies Research Appl. 44, 405–416. doi: 10.1002/9781118311172.ch20
López-Garrido P. H., Barry J. P., González-Gordillo J. I., Escobar-Briones E. (2020). ROV’s video recordings as a tool to estimate variation in megabenthic epifauna diversity and community composition in the Guaymas Basin. Front. Mar. Sci. 7, 154. doi: 10.3389/fmars.2020.00154
MacIntosh H., Althaus F., Williams A., Tanner J. E., Alderslade P., Ahyong S. T., et al. (2018). Invertebrate diversity in the deep Great Australian Bight (200–5000 m). Mar. Biodiversity Records 11, 1–21. doi: 10.1186/s41200-018-0158-x
Mayer L., Jakobsson M., Allen G., Dorschel B., Falconer R., Ferrini V., et al. (2018). The Nippon Foundation—GEBCO seabed 2030 project: The quest to see the world’s oceans completely mapped by 2030. Geosciences 8 (2), 63. doi: 10.3390/geosciences8020063
McAfee D., Alleway H. K., Connell S. D. (2020). Environmental solutions sparked by environmental history. Conserv. Biol. 34, 386–394. doi: 10.1111/cobi.13403
McClatchie S., Middleton J. F., Ward T. M. (2006). Water mass analysis and alongshore variation in upwelling intensity in the eastern Great Australian Bight. J. Geophys. Res. Oceans 111 (C8). doi: 10.1029/2004JC002699
McConnell B., Chambers C., Fedak M. (1992). Foraging ecology of southern elephant seals in relation to the bathymetry and productivity of the Southern Ocean. Antarct. Sci. 4 (4), 393–398. doi: 10.1017/S0954102092000580
McLeay L. J., Sorokin S. J., Rogers P. J., Ward T. M. (2003). Benthic Protection Zone of the Great Australian Bight Marine Park: 1. Literature Review. Final Report to National Parks and Wildlife South Australia and the Commonwealth Department of the Environment and Heritage (South Australian Research and Development Institute (Aquatic Sciences), Adelaide).
Menandro P. S., Bastos A. C. (2020). Seabed mapping: A brief history from meaningful words. Geosciences 10 (7), 273. doi: 10.3390/geosciences10070273
Menge B. A., Caselle J. E., Milligan K., Gravem S. A., Gouhier T. C., White J. W., et al. (2019). Integrating coastal oceanic and benthic ecological approaches for understanding large-scale meta-ecosystem dynamics. Oceanography 32, 38–49. doi: 10.5670/oceanog.2019.309
Middleton J. F., Bye J. A. (2007). A review of the shelf-slope circulation along Australia’s southern shelves: Cape Leeuwin to Portland. Prog. Oceanogr. 75 (1), 1–41. doi: 10.1016/j.pocean.2007.07.001
Middleton J. F., James N. P., James C., Bone Y. (2014). Cross-shelf seawater exchange controls the distribution of temperature, salinity, and neritic carbonate sediments in the Great Australian Bight. J. Geophys. Res. Oceans 119 (4), 2539–2549. doi: 10.1002/2013JC009420
Moll R. J., Millspaugh J. J., Beringer J., Sartwell J., He Z. (2007). A new ‘view’ of ecology and conservation through animal-borne video systems. Trends Ecol. Evol. 22, 660–668. doi: 10.1016/j.tree.2007.09.007
Monk J., Ierodiaconou D., Versace V. L., Bellgrove A., Harvey E., Rattray A., et al. (2010). Habitat suitability for marine fishes using presence-only modelling and multibeam sonar. Mar. Ecol. Prog. Ser. 420, 157–174. doi: 10.3354/meps08858
O'Connell L. G., James N. P., Doubell M., Middleton J. F., Luick J., Currie D. R., et al. (2016). Oceanographic controls on shallow-water temperate carbonate sedimentation: Spencer Gulf, South Australia. Sedimentology 63 (1), 105–135. doi: 10.1111/sed.12226
Peters K. J., Ophelkeller K., Bott N. J., Deagle B. E., Jarman S. N., Goldsworthy S. D. (2015). Fine-scale diet of the Australian sea lion (Neophoca cinerea) using DNA-based analysis of faeces. Mar. Ecol. 36 (3), 347–367. doi: 10.1111/maec.12145
Przeslawski R., Currie D. R., Sorokin S. J., Ward T. M., Althaus F., Williams A. (2011). Utility of a spatial habitat classification system as a surrogate of marine benthic community structure for the Australian margin. ICES J. Mar. Sci. 68 (9), 1954–1962. doi: 10.1093/icesjms/fsr106
Rather T. A., Kumar S., Khan J. A. (2020). Multi-scale habitat modelling and predicting change in the distribution of tiger and leopard using random forest algorithm. Sci. Rep. 10 (1), 11473. doi: 10.1038/s41598-020-68167-z
Robusto C. C. (1957). The cosine-haversine formula. Am. Math. Monthly 64, 38–40. doi: 10.2307/2309088
Shanley C. S., Eacker D. R., Reynolds C. P., Bennetsen B. M., Gilbert S. L. (2021). Using LiDAR and Random Forest to improve deer habitat models in a managed forest landscape. Forest Ecol Manag. 499, 119580. doi: 10.1016/j.foreco.2021.119580
Shepherd S. A., Sprigg R. (1976). “Substrate, sediments and subtidal ecology of Gulf St. Vincent and Investigator Strait” in Natural History of the Adelaide Region, 161–174.
Sorokin S. J., Currie D. R. (2008). Report to Nature Foundation SA Inc, 68. The distribution and diversity of sponges in Spencer Gulf.
Sorokin S., Fromont J., Currie D. (2007). Demosponge biodiversity in the benthic protection zone of the Great Australian Bight. Trans. R. Soc. S. Aust. 131 (2), 192–204. doi: 10.1080/03721426.2007.10887083
Stewart R. R., Noyce T., Possingham H. P. (2003). Opportunity cost of ad hoc marine reserve design decisions: an example from South Australia. Mar. Ecol. Prog. Ser. 253, 25–38. doi: 10.2254/meps253025
Sweetman A. K., Thurber A. R., Smith C. R., Levin L. A., Mora C., Wei C.-L., et al. (2017). Major impacts of climate change on deep-sea benthic ecosystems. Elementa: Science of the Anthropocene 5, 4. doi: 10.1525/elementa.203
Tanner J. E. (2005). Three decades of habitat change in Gulf St. Vincent, South Australia. Habitat Modification and its Influence on Prawn and Crab Fisheries. Final Report to the Fisheries Research and Development Corporation (South Australian Research and Development Institute (Aquatic Sciences), Adelaide).
Thomas C., Hughes V. (2016). “South Australia’s experience: establishing a network of nineteen marine parks,” in Big, Bold and Blue: Lessons from Australia’s Marine Protected Areas, 139–152.
Torn K., Herkül K., Martin G., Oganjan K. (2017). Assessment of quality of three marine benthic habitat types in northern Baltic Sea. Ecol. Indic. 73, 772–783. doi: 10.1016/j.ecolind.2016.10.037
van Ruth P. D., Patten N. L., Doubell M. J., Chapman P., Rodriguez A. R., Middleton J. F. (2018). Seasonal-and event-scale variations in upwelling, enrichment and primary productivity in the eastern Great Australian Bight. Deep Sea Res. Part II: Topical Stud. Oceanography 157, 36–45. doi: 10.1016/j.dsr2.2018.09.008
Vigo M., Navarro J., Aguzzi J., Bahamón N., García J. A., Rotllant G., et al. (2023). ROV-based monitoring of passive ecological recovery in a deep-sea no-take fishery reserve. Sci. Total Environ. 883, 163339. doi: 10.1016/j.scitotenv.2023.163339
Ward T. M., McLeay L. J., Dimmlich W. F., Rogers P. J., McClatchie S., Matthews R., et al. (2006). Pelagic ecology of a northern boundary current system: effects of upwelling on the production and distribution of sardine (Sardinops sagax), anchovy (Engraulis australis) and southern bluefin tuna (Thunnus maccoyii) in the Great Australian Bight. Fish. Oceanogr. 15 (3), 191–207. doi: 10.1111/j.1365-2419.2006.00353.x
Ward T. M., Sorokin S. J., Currie D. R., Rogers P. J., McLeay L. J. (2006a). Epifaunal assemblages of the eastern Great Australian Bight: Effectiveness of a benthic protection zone in representing regional biodiversity. Cont. Shelf Res. 26 (1), 25–40. doi: 10.1016/j.csr.2005.09.006
Wong R. H., Monk J., Perkins N. R., Barrett N. S. (2023). A systematic review on the anthropogenic stressors on sessile benthic mesophotic reef communities: implications for temperate reef management in Australia. Front. Mar. Sci. 10. doi: 10.3389/fmars.2023.1276072
Yoklavich M. M., Laidig T. E., Graiff K., Clarke M. E., Whitmire C. E. (2018). Incidence of disturbance and damage to deep-sea corals and sponges in areas of high trawl bycatch near the California and Oregon border. Deep Sea Res. Part II: Top. Stud. Oceanogr. 150, 156–163. doi: 10.1016/j.dsr2.2017.08.005
Keywords: habitat mapping, benthic, pinniped, animal-borne video, southern Australia, continental shelf, mesophotic reefs, biologging
Citation: Angelakis N, Grammer GL, Connell SD, Bailleul F, Durante LM, Kirkwood R, Holman D and Goldsworthy SD (2024) Using sea lion-borne video to map diverse benthic habitats in southern Australia. Front. Mar. Sci. 11:1425554. doi: 10.3389/fmars.2024.1425554
Received: 30 April 2024; Accepted: 24 June 2024;
Published: 07 August 2024.
Edited by:
Tim Wilhelm Nattkemper, Bielefeld University, GermanyReviewed by:
Lonny J. Lundsten, Monterey Bay Aquarium Research Institute (MBARI), United StatesPeriyadan K. Krishnakumar, King Fahd University of Petroleum and Minerals, Saudi Arabia
Copyright © 2024 Angelakis, Grammer, Connell, Bailleul, Durante, Kirkwood, Holman and Goldsworthy. This is an open-access article distributed under the terms of the Creative Commons Attribution License (CC BY). The use, distribution or reproduction in other forums is permitted, provided the original author(s) and the copyright owner(s) are credited and that the original publication in this journal is cited, in accordance with accepted academic practice. No use, distribution or reproduction is permitted which does not comply with these terms.
*Correspondence: Nathan Angelakis, nathan.angelakis@adelaide.edu.au