- 1Horn Point Laboratory, University of Maryland Center for Environmental Science, Cambridge, MD, United States
- 2Chesapeake Biological Laboratory, University of Maryland Center for Environmental Science, Solomons, MD, United States
- 3Facilitated Solutions, LLC, Tallahassee, FL, United States
- 4FCRC Consensus Center, Florida State University, Tallahassee, FL, United States
- 5Program Integration Office, National Oceanic and Atmospheric Administration, Silver Spring, MD, United States
- 6Batten School of Coastal and Marine Sciences, Virginia Institute of Marine Science, William & Mary, Gloucester Point, VA, United States
- 7Virginia Sea Grant, Gloucester Point, VA, United States
- 8Fishing and Boating Services, Maryland Department of Natural Resources, Annapolis, MD, United States
- 9Marketing Department, Salisbury University, Salisbury, MD, United States
- 10The Eymit Group, Baltimore, MD, United States
- 11Conflict Analysis & Dispute Resolution Department, Bosserman Center for Conflict Resolution, Salisbury University, Salisbury, MD, United States
Worldwide, enhancement of oyster populations is undertaken to achieve a variety of goals including support of food production, local economies, water quality, coastal habitat, biodiversity, and cultural heritage. Although numerous strategies for improving oyster stocks exist, enhancement efforts can be thwarted by long-standing conflict among community groups about which strategies to implement, where efforts should be focused, and how much funding should be allocated to each strategy. The objective of this paper is to compare two engagement approaches that resulted in recommendations for multi-benefit enhancements to oyster populations and the oyster industry in Maryland, U.S.A., using the Consensus Solutions process with collaborative simulation modeling. These recommendations were put forward by the OysterFutures Workgroup in 2018 and the Maryland Oyster Advisory Commission (OAC) in 2021. Notable similarities between the efforts were the basic principles of the Consensus Solutions process: neutral facilitation, a 75% agreement threshold, the presence of management agency leadership at the meetings, a scientific support team that created a management scenario model in collaboration with community group representatives, numerous opportunities for representatives to listen to each other, and a structured consensus building process for idea generation, rating, and approval of management options. To ensure meaningful representation by the most affected user groups, the goal for membership composition was 60% from industry and 40% from advocacy, agency, and academic groups in both processes. Important differences between the processes included the impetus for the process (a research program versus a legislatively-mandated process), the size of the groups, the structure of the meetings, and the clear and pervasive impact of the COVID-19 pandemic on the ability of OAC members to interact. Despite differences and challenges, both groups were able to agree on a package of recommendations, indicating that consensus-based processes with collaborative modeling offer viable paths toward coordinated cross-sector natural resource decisions with scientific basis and community support. In addition, collaborative modeling resulted in ‘myth busting’ findings that allowed participants to reassess and realign their thinking about how the coupled human-oyster system would respond to management changes.
1 Introduction
Effective natural resource management is challenging because of the multiple objectives of different community groups, a limited set of regulatory and policy options, and uncertainty or disagreement about the performance of those options. Numerous approaches have been used for making regulations and policies that allow continued use of natural resources while ensuring benefits to future generations, including collaborative governance (e.g., Ansell and Gash, 2008; Gutiérrez et al., 2011; Newig et al., 2019), Structured Decision Making (Runge et al., 2020), and participatory modeling (e.g., Goethel et al., 2019; Deith et al., 2021; Wilberg et al., 2024). The objective of this paper is to describe two successful implementations of an integration of collaborative governance and participatory modeling for Maryland’s contentious fishery on the eastern oyster (Crassostrea virginica) based on the Consensus Solutions process with collaborative modeling (Supplementary Figure S1).
The Consensus Solutions process, a type of consensus-building process (Susskind et al., 1999), was developed by co-authors Jeff Blair and Robert Jones, facilitators at Florida State University, in the 1990s and has been applied primarily in the state of Florida over the last 25 years. This formal structured process is designed to resolve highly charged disputes and has the fundamental objective of bringing a balanced group of community group representatives to agreement on a set of regulatory or policy recommendations. It has been applied to a broad suite of issues, from building codes1 to natural resources2 to water supply and quantity3, and by multiple agencies such as nonprofits and federal and state governments including the Florida State legislature4. Key elements of the process include transparency, respect, mutual trust building, equitable and balanced representation, and multiple iterative facilitated meetings (OSW, 2018) – key elements that are found in many collaborative governance processes (Ansell and Gash, 2008; Newig et al., 2019). A super-majority decision making threshold of 75% is used to develop solutions that have support across the community groups represented in the process (OSW, 2018) and to ensure that all of the groups involved support the final package of recommendations. In addition to the 75% super majority decision rule, key components include the iterative non-binding acceptability ranking of options, and the option to reconsider and revise options throughout the process which allows participants the flexibility to explore options they may not initially support on the basis that no binding vote is taken until the last meeting. The 75% agreement threshold is applied to these intermediate options and to the final vote on the overall set of recommendations at the end of the process.
Collaborative modeling, a type of participatory modeling, is a technique that brings community groups and scientists together in the co-development of scientific models that can be applied to co-design solutions to environmental problems and support decision-making (Voinov and Bousquet, 2010; Hemmerling et al., 2020; Abrami et al., 2022). Collaborative modeling has a high degree of community group participation in the co-design of the model and collaboration in the use of the model to achieve mutual benefits and make direct policy recommendations (Basco-Carrera et al., 2017). These collaborative processes have the potential to facilitate and structure deliberations among scientists and community group members surrounding scientific information and uncertainties, resulting in the development of mutual understanding, the formation of common views and an enhanced perception of legitimacy of the process and any resulting policies (Hare, 2011; Röckmann et al., 2012; Henly-Shepard et al., 2015). Collaborative modeling includes participation of community group members in applying and developing models to inform policy recommendations and model co-design (i.e., setting the objectives, deciding on options to be modeled, and identifying performance measures, but not building, running or summarizing model results). Participatory modeling may include community group members in some but not all of these aspects (i.e., not using the model for decision making).
The FishSmart program for the southeast Atlantic king mackerel (Scomberomorus cavalla) fishery is an example of a Consensus Solutions process with collaborative modeling (Wilberg et al., 2009; Miller et al., 2010; Ihde et al., 2011). The FishSmart collaborative model was a simulation-based computer model that incorporated the population dynamics of king mackerel with relationships among fishing activity, regulatory constraints and their uncertainty (Smith et al., 1999; Goethel et al., 2019). The model projected the future performance of management options in achieving the goals of both community group representatives and fishery management. The Consensus Solutions process was used to engage FishSmart workgroup members and scientists in model construction and interpretation of output using a respectful and constructive framework (Miller et al., 2010; Wilberg et al., 2024). The combined process helped FishSmart workgroup members move from visions and goals to the generation and evaluation of options and ultimately to consensus recommendations that were put forward to the South Atlantic Fishery Management Council. Although the Council did not adopt the FishSmart group’s recommendations, possibly related to fact that the fisheries managers were not part of the process, FishSmart’s clear success in producing consensus recommendations made the Consensus Solutions process with collaborative modeling a viable candidate for application to the contentious oyster fishery in Maryland.
The eastern oyster in Chesapeake Bay provides a classic example of an ecologically and commercially important species for which controversy exists on how to protect and enhance their populations. Oysters form the basis of a current and historically important fishery (MacKenzie, 1997; Rothschild et al., 1994; Wilberg et al., 2011; Tarnowski, 2022), play a role in improving water quality in Chesapeake Bay tributaries (Newell et al., 2005; Cerco and Noel, 2007; Fulford et al., 2007, 2010), and provide benefits related to reef habitat and biodiversity (Jackson et al., 2001; Luckenbach et al., 2005; Rodney and Paynter, 2006; Kellogg et al., 2019). Long-standing political intervention in the management of the oyster fishery in Maryland by the state legislature (Kennedy and Breisch, 1983) has likely contributed to, and has been a result of, conflict among community groups.
Multiple management options have been developed to restore eastern oyster populations in Chesapeake Bay since the substantial decline in abundances from historic levels. Over-harvesting and habitat degradation in the 1800s led to a decline in populations and harvests, and, starting in the 1950s, mortalities from the diseases MSX and Dermo further reduced oyster populations (Andrews and Hewatt, 1957; Andrews and Wood, 1967; Rothschild et al., 1994; Wilberg et al., 2011, 2013). Disease, habitat loss, and overharvest are common stressors of Crassostrea species in North America (Ford and Tripp, 1996; Luckenbach et al., 1999; Kirby, 2004). Considerable efforts have been made to enhance oyster stocks in the Chesapeake Bay and elsewhere along the U.S. Atlantic, Gulf and Pacific coasts (MacKenzie, 1970; Luckenbach et al., 1999; White et al., 2009; La Peyre et al., 2014). In Maryland, numerous strategies for improving oyster stocks have been tried, including harvest limits (e.g., gear restrictions, daily and seasonal limits on effort, rotational harvest closures (closing and opening bars for harvest on a specific schedule)), creating sanctuaries or marine protected areas, oyster bar replenishment programs (e.g., adding shell and juvenile oysters to harvest regions), large-scale restoration programs (e.g., building oyster reefs in sanctuaries), and artificial reef creation.
Despite the many strategies for enhancing oyster populations, disagreements have persisted for decades about which strategies to apply, where to apply them, and how to allocate effort and funding in Maryland. The many groups associated with efforts to restore and sustain oyster populations – state and federal management agencies, oyster fishers and aquaculturists, nonprofit environmental organizations, recreational anglers, boaters and Chesapeake Bay residents – have had different and sometimes conflicting goals for oysters and promoted different methods for enhancing oyster populations. While all groups supported increasing oyster populations, the rationales for improving oyster stocks varied among groups. The different rationales included maintaining access to oyster fishing, reducing loss of cultural heritage, minimizing economic disruption, promoting oysters’ role in water quality and biodiversity, reducing risk to future oyster populations, and ensuring access for future generations, with state and federal management agencies tasked with finding compromises. The designation by Maryland of oyster sanctuaries (i.e., areas closed to oyster harvest) in areas that had formerly been open to commercial fishing was particularly controversial.
In the midst of this controversy, the OysterFutures program was conducted with funding from the National Science Foundation’s Coastal Science, Engineering and Education for Sustainability (SEES) program. A goal was to implement the Consensus Solutions process with collaborative modeling to help representatives from key interest groups develop a common vision and come to agreement on recommendations for policies and regulations for the oyster fishery in the Choptank and Little Choptank Rivers (Figure 1B), one of the most active commercial oyster fishing regions of Maryland’s Chesapeake Bay. Through nine meetings held in 2016-2018, the OysterFutures Workgroup produced a collective vision for the future of oysters in the Choptank and Little Choptank Rivers, collaboratively developed a simulation model with the OysterFutures research team, and came to consensus on recommendations that they put forward to the Maryland Department of Natural Resources (MDDNR) (OSW, 2018).
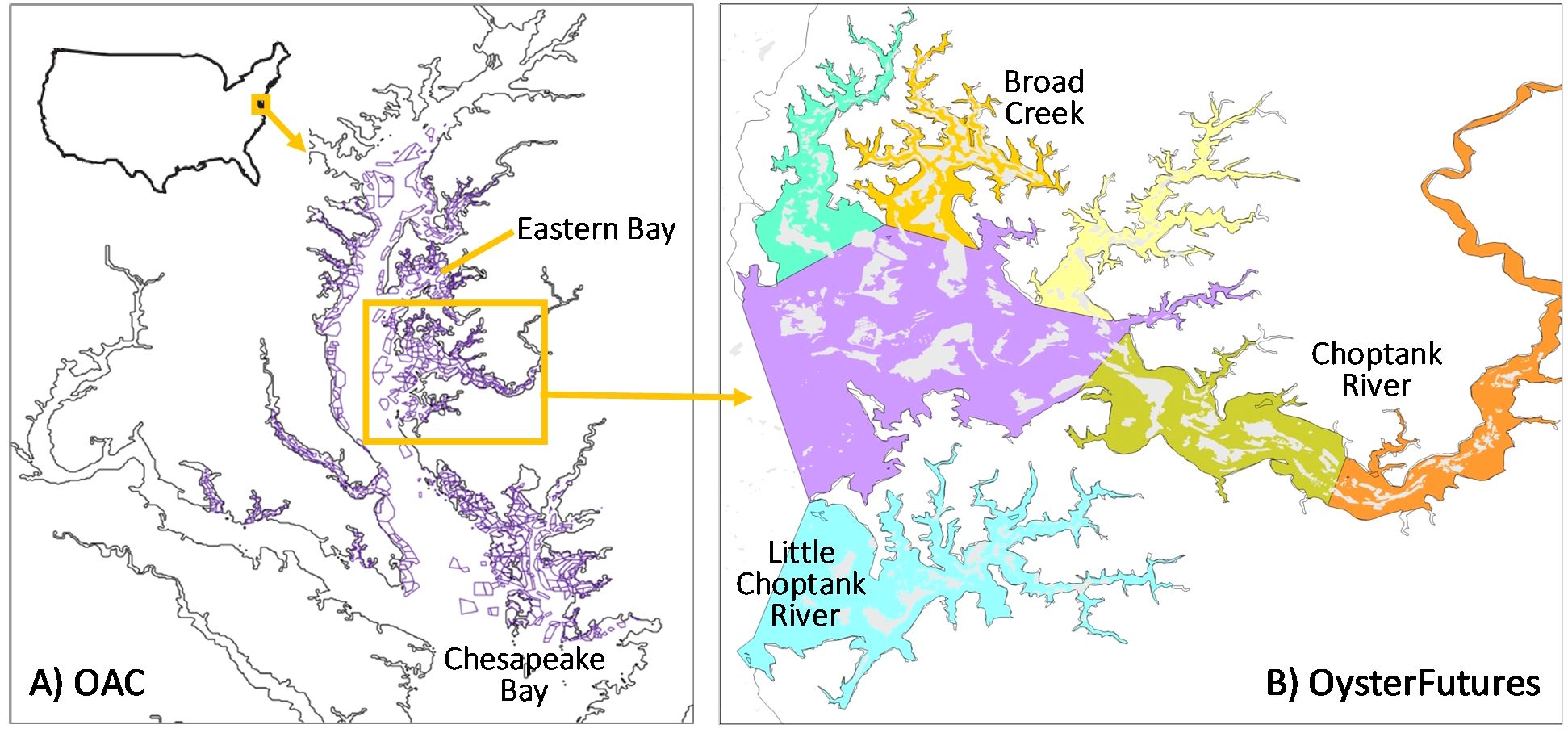
Figure 1. Study location and model spatial domains used in the Oyster Advisory Commission (OAC) and OysterFutures collaborative simulation models. (A) Model domain of the OAC simulation model. Outline of the Chesapeake Bay (black line) with oyster habitat polygons (purple lines) on which oyster populations were simulated. Eastern Bay, the location of coordinated oyster enhancement activities recommended by the OAC, is indicated. (B) Model domain of the OysterFutures simulation model. Colored regions indicate seven harvest reporting regions. Gray shaded areas are the oyster habitat polygons on which oyster populations were simulated.
The results of the OysterFutures program inspired legislation in Maryland that mandated a two-year consensus-based process with collaborative scientific modeling to enhance the fisheries management plan for oysters in Maryland waters (described in section 3.2). This legislation redefined the Maryland Oyster Advisory Commission (OAC) with prescribed membership, stipulated goals, and a requisite 75% agreement decision-making threshold. Although the intent was similar to OysterFutures, the implementation differed. Specifically, the spatial scale was statewide, and the process needed to adapt to MDDNR requirements and to the COVID-19 global pandemic. Despite the differences, the OAC process, like that of OysterFutures, achieved its goal of generating a consensus package of recommendations that were put forward to the MDDNR and the Maryland State Legislature (MDDNR, 2021).
Although both the OysterFutures workgroup and the OAC produced regulatory and policy recommendations for enhancing oyster populations and the oyster industry in Maryland that would achieve social, economic, and ecological goals of multiple interest groups, key aspects of these processes have not yet been described and compared. While these two examples of successful community group involvement in creation of participatory models and policy recommendations are not unique (e.g., see Irwin et al., 2008, 2011; Röckmann et al., 2012), the comparison of the OysterFutures and OAC processes herein can contribute to improved understanding of the effectiveness of collaborative governance combined with participatory modeling.
2 Materials and methods
In order to compare and contrast the OysterFutures and OAC processes in a systematic framework, we used the “4P” structure: Purpose, Partnerships, Process, Products (Gray et al., 2018). The 4P components enable comparison between participatory modeling programs in an effort to advance the practice of participatory modeling (Gray et al., 2018). The Purpose section explains why each program was conducted and describes the funding, impetus and goals. In the Partnerships section, the groups – industry, nonprofit, management, and scientists – that participated in each program are explained. The integration of Consensus Solutions with collaborative modeling and the collaborative simulation model and its components are described in the Process section. For Products, policy recommendations and new knowledge that were generated are described. After explaining each process using the 4P framework, we compare the two processes in sections dedicated to key similarities, major differences, and lesson learned. The perspectives and opinions expressed herein are our own and we recognize that other participants in the OysterFutures and OAC processes may have different views.
3 Results
3.1 The OysterFutures process
3.1.1 Purpose
3.1.1.1 Impetus and funding
The overarching goal of the research project that supported the OysterFutures program was to improve the utility of predictive models for shaping natural resource policy and management. The research project was funded by a grant from the National Science Foundation’s Coastal SEES program (NSF OCE-1427019). Coastal SEES projects were “expected to lead to generalizable theoretical advances in natural sciences and engineering while, at the same time, integrating key aspects of human processes required to address issues of coastal sustainability”5. The OysterFutures research project addressed these needs by developing a novel scientific model, by applying the Consensus Solutions process with collaborative modeling for developing sustainable regulations and policies with broad community group support, and by studying the change in participants views toward scientific models during the OysterFutures program. Funds for the five-year $2M project were allocated to the development of the OysterFutures simulation model at University of Maryland Center for Environmental Science (UMCES) ($592K), facilitation provided by Florida State University’s FCRC Consensus Center ($156K), science communication at UMCES ($44K), participant support costs for the OysterFutures Workgroup meetings ($38K), supportive water quality modeling and laboratory studies at UMCES ($710K), and social science studies conducted by Virginia Institute of Marine Science ($458K). These figures included full federal overhead as well as the financial support for two Ph.D. students, three Masters students, and two postdoctoral scholars who received training through this project.
3.1.1.2 Goal
The OysterFutures Workgroup members agreed on the following goal: “The OysterFutures Stakeholder Workgroup’s ultimate goal was to ensure that the regulation and management of the oyster fishery, and oyster restoration policies, are informed by the best available science and shared stewardship values, resulting in an economically viable, healthy and sustainable Choptank and Little Choptank Rivers oyster fishery and ecosystem” (OSW, 2018).
3.1.2 Partnerships
3.1.2.1 Connection to fisheries management
Support from the Secretary of MDDNR, the agency that implements and enforces oyster regulations, played a key role in providing legitimacy to the OysterFutures program. From the beginning, MDDNR leadership expressed interest in the program, assigned the Director of Fisheries to serve as a member of the OysterFutures Workgroup, and wrote a letter to OysterFutures workgroup members stating that MDDNR would seriously consider the recommendations that resulted from the effort.
3.1.2.2 Workgroup composition
An important part of the Consensus Solutions process was ensuring equitable and credible representation from participants in the oyster industry as well as agency, recreational, restoration, and environmental groups. The relative composition of the Workgroup was determined beforehand: 60% from industry defined as oyster fishers, aquaculturists, and seafood buyers and 40% from other key groups including nonprofits and government agencies. The goals of the 60/40 composition were to demonstrate to industry members that their contributions would be valued, to ensure that the diversity of opinions within the industry could be expressed, and to give a strong voice to those who were most directly impacted by oyster regulations and policies. This composition was paired with the 75% agreement threshold, requiring collaboration among Workgroup members to develop regulation and policy options that were mutually beneficial and to ensure that no individual could veto recommendations nor could one interest group dominate the process.
3.1.2.3 Workgroup membership
The OysterFutures Workgroup had 16 members: six oyster fishers who had active licenses for harvesting oysters, one oyster buyer, two aquaculturists, five nonprofit group representatives (including environmental advocacy and recreational fishing interests), one state shellfish manager and one federal habitat restoration specialist (OSW, 2018). Commercial activities of industry members occurred in the two counties surrounding the Choptank River. Over the course of the two-year program, two of the members stepped down and were replaced with new members.
The number of workgroup members was set at 16 based on the number of impacted groups and within the optimal size range for the Consensus Solutions process based on the experience of the Florida State University facilitators. To identify suitable representatives to invite to participate, members of community groups who were active on oyster issues were asked who they thought would be acceptable and credible representatives for their interests and would be able to effectively participate in a process designed to foster collaboration and consensus building. Members of the Workgroup were asked to participate based on the results of these conversations as well as efforts to ensure diversity in perspectives, gear types, ages, gender, and geography on the Workgroup. Once community group members agreed to serve on the Workgroup, facilitators conducted a pre-meeting questionnaire. Responses to this questionnaire enabled the facilitators to draft vision themes, a goal statement, and a list of oyster resource issues for Workgroup member consideration, refinement, and approval at the first workgroup meeting.
3.1.3 Process
3.1.3.1 Implementation team
The design, planning and execution of the OysterFutures Consensus Process with collaborative modeling (Figure 2) was conducted by a leadership team that included two professional facilitators and two scientists (a fisheries scientist and a fisheries oceanographer). The facilitation team included one person to facilitate the meeting and focus on process and a second who took meeting notes, drafted and revised options proposed by Workgroup members, and tabulated ratings on a computer projected onto a large screen so that Workgroup members could see the text in real time. In addition to the two scientists on the leadership team, the scientific modeling team included members with expertise in natural resource economics, marine social scientists, nutrient biogeochemistry, and biogeochemical modeling. Additional logistical and graphic support was provided by science communicators from UMCES Integration Application Network6. Agenda packets were mailed to Workgroup members before each meeting, either by email or post based on members’ preference. As meetings progressed, additional items were sent in advance of meetings, including previous meeting summaries, model tables and graphics, and responses to requests for information from Workgroup members.
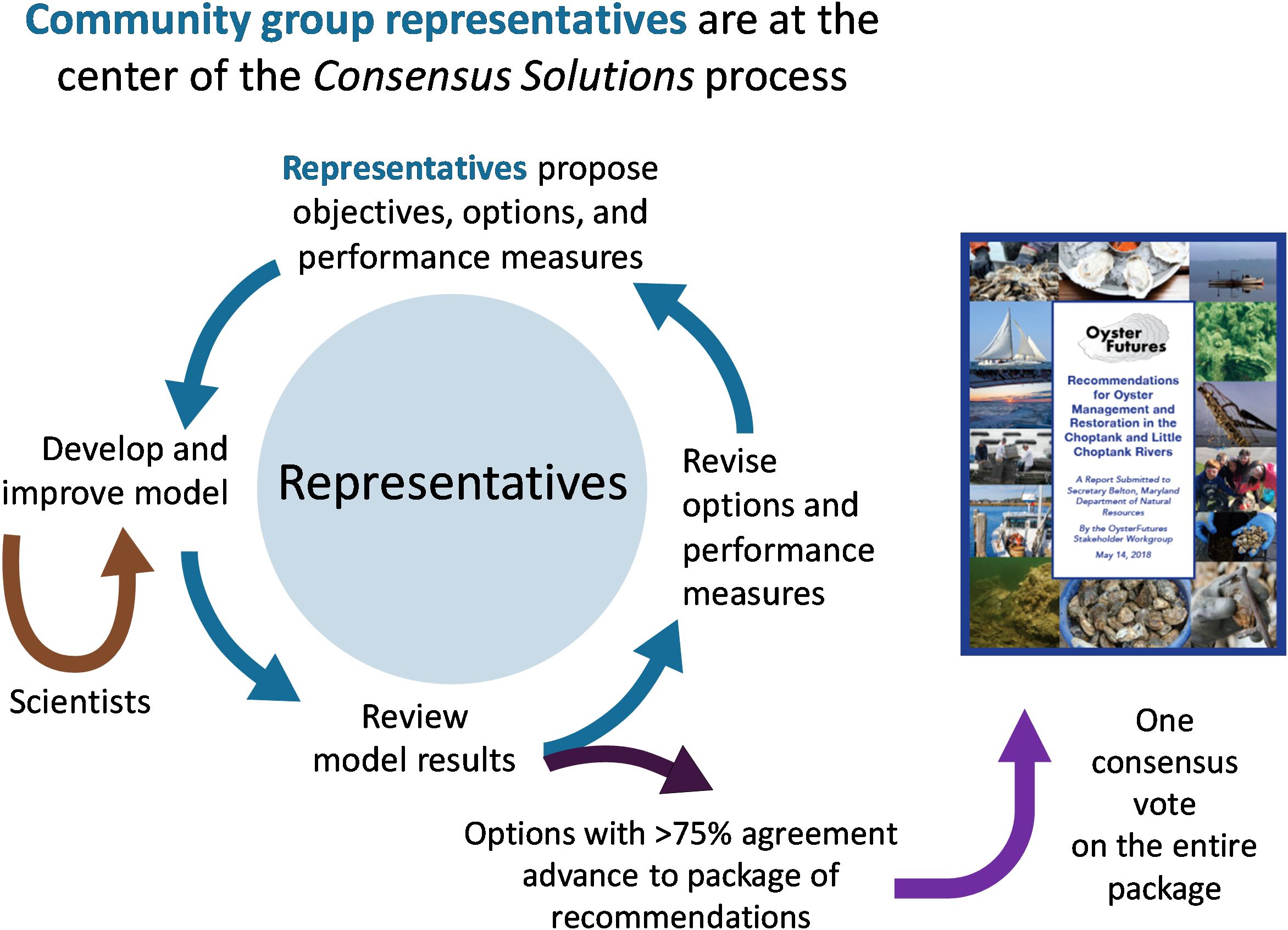
Figure 2. Schematic of the iterative Consensus Solutions process with community group representatives at the center. Representatives proposed objectives, options and outcomes; scientists collaborated with them to develop a model to forecast the effects of the options that the representatives put forward; representatives reviewed model results, revised options and performance measures. This cycle continued until a package of options that rated equal or higher than the 75% agreement threshold was generated. At the end of the process, there was one vote on the entire package of recommendations. The cover of the OysterFutures Workgroup recommendations report is shown (OSW, 2018).
3.1.3.2 Workgroup meetings
Workgroup meetings were scheduled to allow numerous opportunities for Workgroup members to speak with each other and the modeling team, both within the sessions and during breaks and meals. Initially eight 1.5-day workgroup meetings were planned and a ninth 1-day meeting was added to enable members to come to agreement. These meetings took place from February 2016 to March 2018 with the time between meetings as much as 6 months (during model development) to as little as one month (once the model was complete). Delays between meetings were due to difficulties with model development and severe weather that would have prohibited team members and Workgroup members from participating. Meetings were held on weekends (Friday and Saturday or Saturday and Sunday) to accommodate the schedules of oyster fishers and to ensure strong representation from industry. Meals and reimbursement for travel were provided. An honorarium of $200 per meeting was given to self-employed participants to help offset the costs of participating in the process. At the request of Workgroup members, meetings were closed to the public to allow for frank discussions and the security to explore the full range of options including controversial options.
At the first Workgroup meeting, clear guidelines for respectful behavior at meetings were presented by the facilation team and agreed to by all Workgroup members. The meeting agenda also included Consensus Solutions operating assumptions and principles, a shared history exercise, development of a shared vision for the Workgroup, and initial discussion of ideas for options (i.e., strategies for oyster management) and performance measures (i.e., the metrics used to evaluate the potential success of options that could be modeled). Modeling team members presented an overview of the oyster fishery and regulatory framework as well as an introduction to the collaborative simulation model (described below).
During the subsequent seven meetings, the Workgroup refined their goal statement and discussed and identified regulation and policy options, some of which could be included in a simulation model for forecasting expected outcomes (e.g., rotational harvest, oyster shell supplements, restoration in sanctuaries, artificial reef placement), and some of which could not be modeled within the confines of the process (e.g., increased education, business practices and marketing). Sitting at a U-shaped table with facilitators at the head (Supplementary Figure S2), workgroup members rated each option for its acceptability (1 = acceptable, 2 = minor reservations, 3 = major reservations, 4 = not acceptable), and members were asked to offer their perspective when they had major concerns or found an option unacceptable. This allowed the Workgroup members to learn from each other and work toward more acceptable solutions. Options and revised options that had an acceptability rating of ≥75% were carried forward as preliminary consensus recommendations. The iterative process of evaluating and revising options allowed workgroup members to explore the full range of options with the knowledge that any option could be re-evaluated and re-ranked at the request of any Workgroup member, and the status of a ranked option would not be final until the final Workgroup meeting, when a vote would be taken on the entire package of recommendations that had an acceptability rating of ≥75%.
At the final meeting on March 23-24, 2018, the results of the final model runs were presented and Workgroup members reviewed the text of options that had been rated with ≥75% acceptability, including both modeled and non-modeled options. The Workgroup voted unanimously (14 in favor, 0 not in favor) to accept the comprehensive package of consensus recommendations. One member recused themself and one member was not in attendance. Of the more than 100 options that were considered, 29 were in the final package of consensus recommendations. All Workgroup members were invited to review the report before it was submitted to the Secretary of MDDNR in May 2018.
3.1.3.3 Collaborative simulation model
Throughout the Workgroup meetings, the collaboratively-built simulation model was a central focus of presentations and discussions. Versions of the simulation model were presented during the second through fourth meetings, and Workgroup and modeling team members collaborated on adding features, refining data and functions, and otherwise adapting the model for use in evaluating the performance of alternative regulatory and policy options. The second through seventh meetings focused on developing the model to the point where Workgroup members agreed to use it to evaluate potential management options. For the final two meetings, Workgroup members focused on applying the model to evaluate and improve options and negotiating acceptable tradeoffs.
The OysterFutures simulation model forecasted oyster populations 25 years into the future starting with 2016 oyster abundance estimates. The simulations evaluated long and short-term effects of more than 100 management and policy options proposed by the Workgroup, such as rotational harvest areas, changes in enforcement of harvest regulations, changes in sanctuary boundaries, planting shell or spat on shell (hatchery-reared juveniles attached to shell), and large-scale restoration in sanctuaries. For each option, the simulation model estimated more than 25 performance measures that the Workgroup members requested and used to determine how well each option achieved the Workgroup’s goals. The performance measures included abundance and size distribution of oysters, amount of harvest, revenue generated by harvest, amount of nitrogen and suspended matter removed, the social value of nitrogen reduction, and metrics of cost effectiveness. Workgroup members adjusted, revised, and replaced options during the process in response to performance measures, resulting in a final set of options that were cost effective (e.g., annual harvest revenue > annual cost of option) and achieved positive performance for multiple performance measures (e.g., oyster abundance, harvest revenue, and nitrogen reduction) by the end of the 25-year simulation (Supplementary Table S1).
Many options that the Workgroup considered (e.g., planting shell or oysters, opening or closing oyster bars to harvest) involved actions at specific locations. To make forecasts of system responses at the spatial scale at which management options took place, the simulation model included age- and length-structured oyster population dynamics, oyster larval transport, and fisher behavior models, all implemented in AD Model Builder (Fournier et al., 2012). The model included 1,132 oyster habitat polygons with oyster dynamics modeled separately on each polygon in the Choptank and Little Choptank Rivers (Figure 1B). The habitat polygons were delineated and georeferenced (ArcGIS v.10.6.1) based on side-scan sonar surveys or Workgroup member knowledge (in areas without sonar surveys) to represent areas of oyster habitat.
While the OysterFutures simulation model and some of its components were created de novo, it also integrated existing models such as oyster demographics and larval transport models that were adapted to this effort. The initial abundances of oysters in the model and the rates of growth, mortality, and reproduction in each harvest region were statistically estimated using a 25-year time series of harvest, MDDNR fall dredge survey, oyster planting data, and monitoring data from restoration sites in the Choptank and Little Choptank Rivers (Damiano and Wilberg, 2019). Fishing on each polygon was modeled assuming that oyster harvesters had perfect information about oyster abundance and that they harvested oysters on each bar until it was no longer profitable to do so. Profitability of fishing on each bar was estimated using revenue calculations for harvest and costs by gear type from a survey of oyster fishers, many of whom were Workgroup members. Fishing was also affected by enforcement options that determined the proportion of undersized (< 76.2 mm) oysters harvested and the amount of fishing in sanctuaries. A survey of watermen was used to estimate operations costs (unpublished data) and wholesale prices of oysters were publicly available (from MDDNR).
Larval transport was a key process in the system because it described connectivity between populations on separate oyster bars and so was modeled in detail. A stand-alone larval transport model [Lagrangian TRANSport model (LTRANS v.2b)] was implemented with oyster larval behavior (North et al., 2008; Spires, 2015) and with predictions from ChopROMS, a high-resolution three-dimensional hydrodynamic model of the Choptank and Little Choptank Rivers (Spires, 2015 Appendix A, Gawde et al., 2024) that used boundary conditions from ChesROMS, a hydrodynamic model of Chesapeake Bay (Xu et al., 2012). Both ChopROMS and ChesROMS were based on the Regional Ocean Modeling System (ROMS) (Shchepetkin and McWilliams, 2005). The ChopROMS model was run in high resolution and validated for 2010 with instantaneous predictions of water level, current velocities in three dimensions, salinity, and vertical diffusivity that were output every 10 min. These predictions were interpolated in time and space before being used in the advection, turbulence, and behavior sub-models of the larval transport model to calculate the movement of each larvae-like particle every 75 seconds as described in North et al. (2006, 2008), Schlag and North (2012), and Spires (2015). Three releases of particles were conducted in summer 2010 to simulate the peaks in spawning that occur in the Chotpank River (Spires, 2015). The exchange of larvae among the 1,132 habitat polygons and the advective loss of larvae out of the system was summarized in connectivity matrices for each of the three releases and then combined into one time-invariant connectivity matrix that summarized exchange between polygons (see North (2020) for matrices and model code).
The summary connectivity matrix from the larval transport model was incorporated into the OysterFutures simulation model. The simulation model predicted the number of juvenile oysters that survived on each habitat polygon depending on how many larvae arrived and the amount and quality of bottom habitat. Once settled, simulated oysters grew, and their survival depended on natural mortality, which included disease mortality, and on harvest mortality. The simulation model included stochasticity for larval and post settlement mortality to reflect the substantial year-to-year variability in recruitment and natural mortality (Doering et al., 2021).
In the OysterFutures simulation model, oyster abundance, oyster shell, harvest, harvest revenue, and nitrogen reduction due to oysters were tracked over the 25-year simulation on each habitat polygon (Figure 1B). The amount of harvest on each polygon depended on the abundance of oysters, the price of oysters, the fishers’ costs to harvest in that location, and the management options that the Workgroup members were evaluating that affected regulations or enforcement. The majority of oyster harvest in all model scenarios occurred in locations where harvest was legal. Nitrogen reduction due to oysters was parameterized as a function of oyster biomass based on Jackson et al. (2018), with the value of nitrogen (N) removal estimated as $843 USD per lb ($382 USD per kg) as the average costs per pound of N removal using government spending on stormwater and agricultural practices to remove nutrients (methods in Wainger et al., 2018). The OysterFutures simulation model was run 100 times for each option to account for parameter uncertainty and natural variability and to quantify confidence in model results. The stochastic parameters were the annual recruitments, natural mortality rates, nitrogen removal by oysters, and annual changes in habitat over time, with temporal autocorrelation in natural mortality rates (Wilberg, 2023). Model code and more information about model formulation are available (Wilberg, 2023).
A unique aspect of the OysterFutures simulation model, and part of what fulfills the Coastal SEES requirement of “generalizable theoretical advances in natural sciences and engineering”, was that options were able to be simulated on the same spatial scale as management actions. Workgroup members could view model results at the bar level to project and assess the effect of options at the spatial scale at which management, restoration, replenishment, and reef creation decisions would occur (e.g., reef scale, 0.09 km2 or greater). Modeling at the management-relevant scale was aided by the high-resolution circulation and larval transport model that allowed simulation of the transport of progeny between habitat polygons, which ultimately influenced productivity of bars and the return on investment for management options.
The modeling team incorporated local knowledge into the simulation model and presented model predictions in formats that would support decision making by the Workgroup members. In the process of model development, the modeling team explored evidence for alternative hypotheses of system function and showed that the model was able to reproduce recent trends in harvest and the performance of routinely-used management options. Expert and local knowledge was used to improve model input data, particularly related to the location of oyster habitat and the costs of fishing. When presenting model predictions, all options were compared to the base-line model of status quo management (no change in regulations or policies) to highlight the effects – either positive or negative – caused by the simulated management options. Accurate geospatial representation of oyster bars was important to Workgroup members; hence maps were created that showed the locations where management actions could occur (e.g., Figure 3). Dashboards (Supplementary Table S1) were used to summarize model output and allow Workgroup members to compare the relative merits of each option against the other options. The presentation of model results was iteratively improved with Workgroup members’ feedback on performance measures, summary graphs, and dashboard layout.
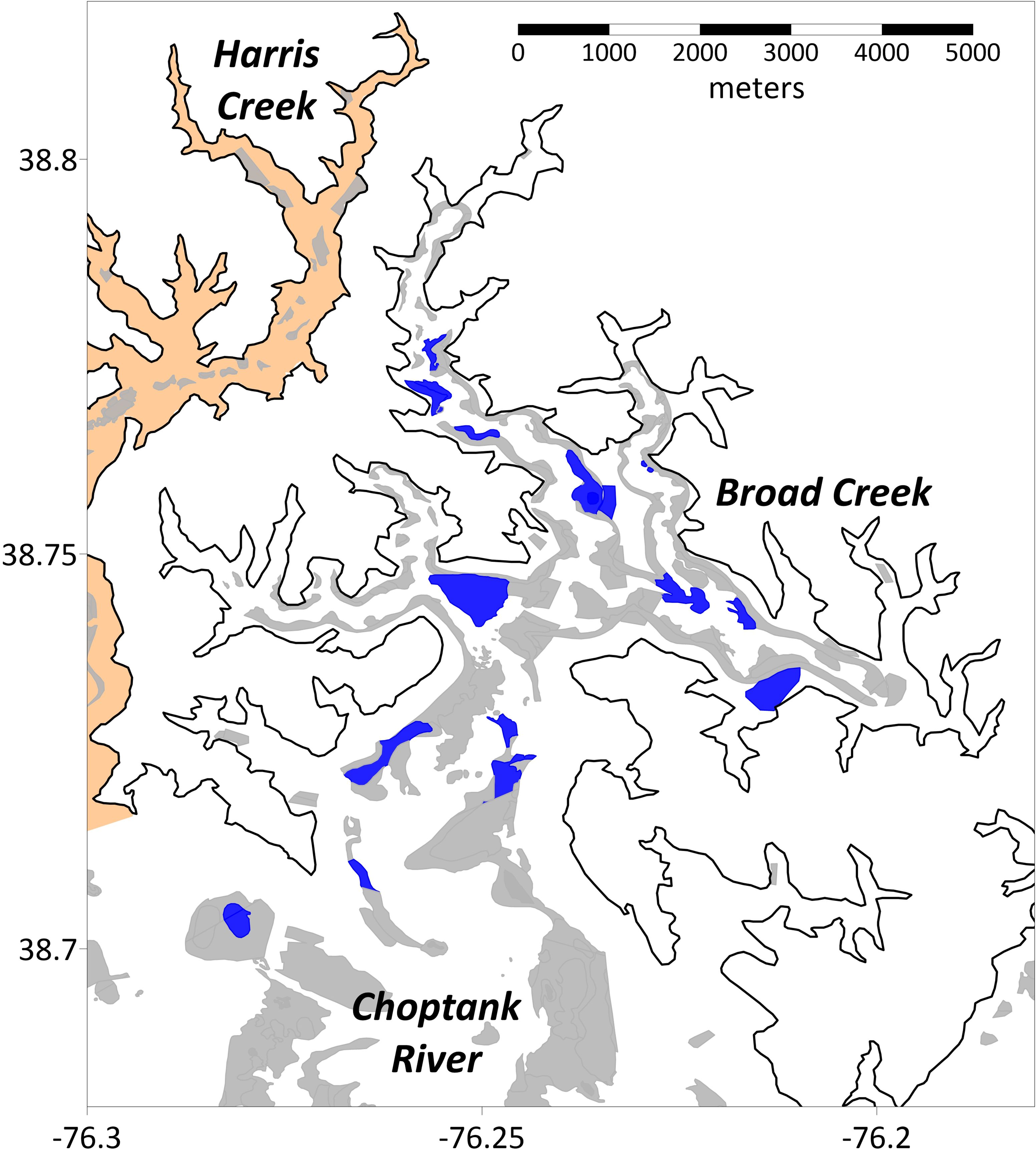
Figure 3. Map depicting one option that was simulated in the OysterFutures simulation model. The blue areas represent locations where replenishment (addition of shell every year) was simulated. Gray shapes are oyster habitat polygons and orange regions are sanctuaries closed to harvest. This figure shows the high resolution of the oyster habitat polygons and hydrodynamic model’s shorelines as well as the ability of the simulation model to evaluate options at the scale (< 1 km) at which oyster restoration and replenishment actions occur.
3.1.4 Products
3.1.4.1 Workgroup recommendations
The OysterFutures Workgroup agreed with 100% approval on a package of 29 specific recommendations to MDDNR (OSW, 2018), stating the need for change. Of the 29 recommendations, nine were directly informed by the simulation model and seven research recommendations arose through the process of model development that highlighted key knowledge gaps. The recommendations included approaches to improve enforcement, enhance habitat and restoration, bolster sources of clean shell, and extend education and training. The Workgroup also recommended considering limited entry and rotational harvest, coordinating investments in marketing strategies and development of business plans, changing and increasing oyster-fishery-related fees and taxes, and using the Consensus Solutions process in the future (OSW, 2018). Notably, the recommendations highlighted three combinations of multiple options that had high performance in the simulation model and spanned the interests of multiple community groups (see purple circles for combined options in Figure 4). The options in the combined sets included replenishment activities (planting shell and spat on shell in fisheries areas), completing restoration of two large-scale sanctuaries, ensuring full compliance with current size laws and sanctuary regulations, opening the tributaries of a large-scale sanctuary to hand tonging on a rotational basis, and planting artificial habitat structures.
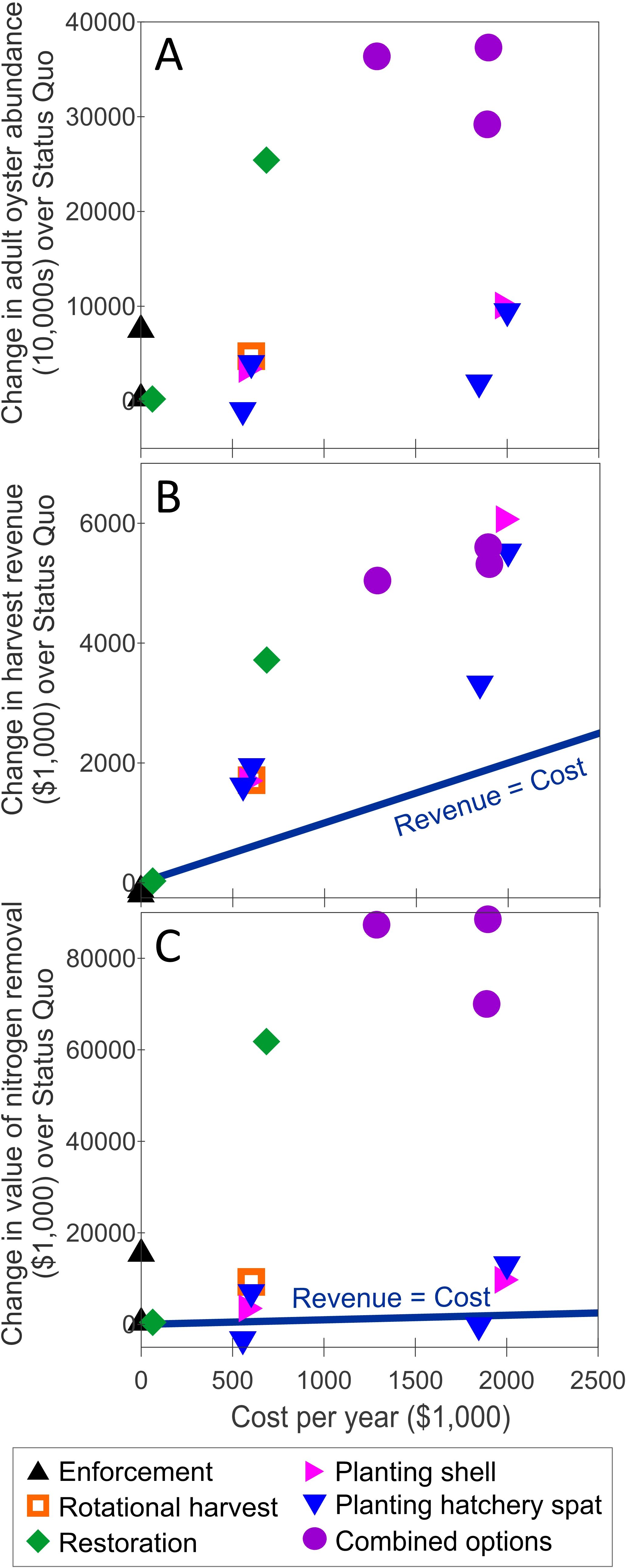
Figure 4. OysterFutures model predictions of performance metrics versus implementation costs for options that met the 75% agreement threshold. Symbols represent option category (see legend at bottom). Performance metrics shown are (A) change in oyster abundance, (B) change in harvest revenue, and (C) change in the value of nitrogen removal. These metrics were averaged over the last three years of models runs (years 22-25) and were expressed as change from the status quo with positive values indicating improved conditions. Cost estimates were based on costs for spat, shell, and artificial substrate. This plot contains the results of the final model simulations that were used by the OysterFutures Workgroup members to inform their final recommendations.
3.1.4.2 Myth-busting model results
Two major findings of the collaboratively-built OysterFutures simulation model changed the way people thought about policies and regulations surrounding the oyster. The first major ‘myth-busting’ result refuted the myth that harvest was incompatible with restoration of oyster populations and ecosystem services – rather, the model showed that “win-win-win” solutions can be found. Through the iterative modeling and discussion process, workgroup members were able to find options that were expected to improve performance compared to the status quo across all major performance measures, including increasing oyster abundance (Figure 4A), increasing harvest revenue (Figure 4B), and increasing the value of nitrogen removed (Figure 4C) while also generating net value. Importantly, combined options – that included options that were promoted by industry (e.g., planting shell) and by agencies/non-profits (e.g., complete large-scale restoration projects) – performed well across the board and were among the most cost-effective (height above the blue lines in Figures 4B, C indicates degree of cost-effectiveness). For many options, the strong positive gains that were seen at 25 years (Figure 4) did not start to be realized until about the 10th year following implementation (Figure 5). This suggests that the effects of major restoration and repletion activities may not be seen immediately, likely because they are constrained by the population growth rate of oysters.
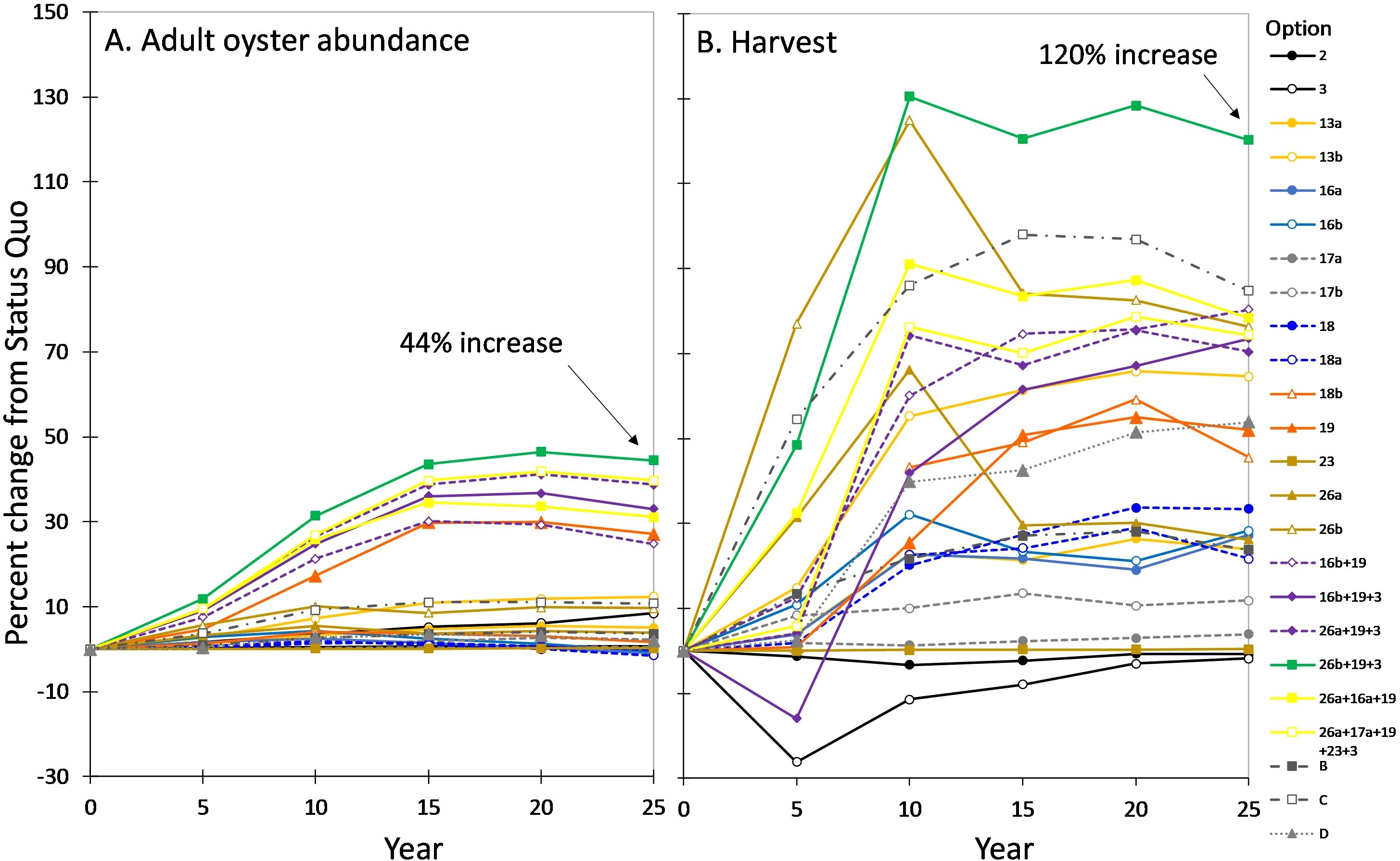
Figure 5. Percent change in (A) adult oyster abundance and (B) harvest compared to the status quo model run (i.e., no change in regulations or policies) for the final 24 options (colored lines) over the simulation time period of OysterFutures model. A description of options can be found in the first column of Supplementary Table S1 in Supplementary Materials. For example, option “26b + 19 + 3” (green line) was to place $2M worth of spat-on-shell in the middle Choptank each year, complete restoration in the Tred Avon and Little Choptank Rivers, and ensure full comliance with all oyster harvesting regulations every year.
The second “myth-busting” result of the OysterFutures simulation model was that management options had a stronger effect on harvest than on oyster abundances (rather than vice-versa). For the same set of 24 management options, adult oyster abundance was predicted to increase by as much as 44% (by 3.2 x 108 adults) over the status quo after 25 years whereas harvest could increase by as much as 120% (by 1.4 x 105 bushels) (Figure 5). Percent changes in harvest were greater than abundance across the options because harvest rates in the model increased as oyster abundance increased, which was a reflection of fisher behavior in the model. The large discrepancy in response of oyster abundance and harvest to the same options suggested that the way in which oyster populations are enhanced can have a strong positive or negative effect on fishers, and that collaboratively-built simulation models could help find solutions that improve oyster abundances and help fishing communities at the same time. Model results also suggested that current fishery management implicitly sets a maximum abundance for oysters in the Maryland portion of the Chesapeake Bay.
3.1.4.3 Social science findings
The iterative, collaborative development of the scientific model during the OysterFutures program was accompanied by research studying Workgroup member involvement in the policy formation process (Goelz et al., 2020a, 2020b) and satisfaction with the outcomes (Hayes and Wainger, 2022). The OysterFutures program presented a unique opportunity to assess the human dimensions of a collaborative decision-making process over time.
Measuring Workgroup members’ networks and their perceptions towards science, local ecological knowledge and scientific models allowed for a nuanced understanding of Workgroup member involvement in a participatory, model-based natural resources management process. One social science team used survey instruments and observations during the OysterFutures meetings to collect data on Workgroup members’ social networks (communication, mutual understanding, and advice/influence networks) and perceptions towards science, scientific models, and local ecological knowledge (Goelz et al., 2020a, 2020b). A second team used a decision science method to conduct interviews and elicit relative preferences for performance goals after the conclusion of the process to evaluate whether individuals felt the Workgroup recommendations were consistent with their individual goals (Hayes and Wainger, 2022).
A highlight of the network analysis was the change in the communication frequency network over the course of the Workgroup meetings, becoming more connected and less centrally focused from the first to the last meeting (Figure 6). The communication network at the first meeting was characterized by several people who occupied central positions in the network indicating that communication passed primarily through a few individuals (Figure 6A). In addition, the thinness of the lines connecting the nodes represent less frequent communication between and among Workgroup members. By the ninth and final meeting, node sizes were more consistent, signifying a more even distribution of degree scores, i.e., there was more even communication across and between people in the network, with fewer individual communication bottlenecks (Figure 6B). The links connecting the nodes also were thicker, indicating more frequent communication. Hence, by the end of OysterFutures, Workgroup members communicated more broadly and more frequently across the network instead of relying on a few key individuals. These changes in communication were not linear with most increases occurring between the first and second meeting and the eighth and last meeting, in response to the needs of the group during the meetings, as seen in other community group processes (Reagans and McEvily, 2003; Sandström and Carlsson, 2008). See Goelz et al. (2020a, 2020b) for more of the social science findings on perceptions of the participatory modeling and network changes.
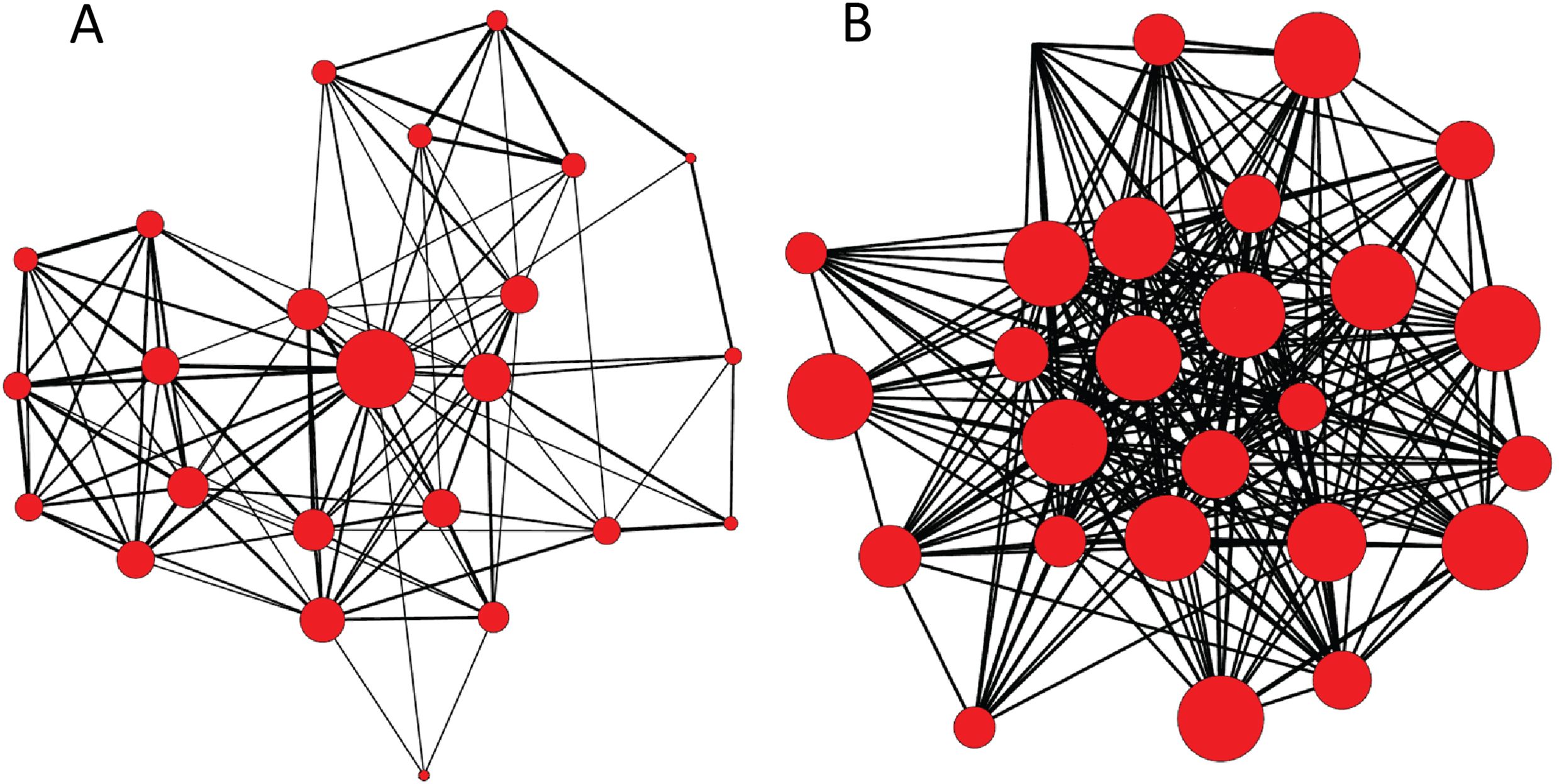
Figure 6. Communication frequency network at (A) the first OysterFutures meeting on February 26-27, 2015 and (B) the ninth and final meeting on March 23-24, 2018. Each node (red circle) represents an individual participant. Line width represents the frequency of communication between people, with thicker lines representing more frequent communication. Nodes are sized by degree score, with larger nodes indicating individuals who had more communication ties.
The decision science team tested whether the OysterFutures Workgoup’s recommendations were effective at representing all perspectives and at identifying the most cost-effective options. Negotiated group solutions can sometimes have a negative side effect of silencing some voices through peer pressure, dominant voices, or other group effects. However, group effects were not found to be evident in this case study and instead, diverse interests appeared well-aligned at the end of the process (Hayes and Wainger, 2022). Further, based on an alternative cost-effectiveness ranking of management options that applied Workgroup members’ relative preferences for individual goals, the vast majority of recommendations that were advanced to the final package of recommendations were the most cost-effective (Hayes and Wainger, 2022).
Beyond advancing our understanding of workgroup dynamics and representativeness, the social science efforts provided community group members with an outlet for their ideas about the process and clearly demonstrated that their opinions were heard and valued. Members of the OysterFutures research team also were subjects in the social network analysis. From our perspective, it was easy to forget that we were being studied – the meetings were interesting and engaging – and the fact of being included in the study helped us connect with community group members through the shared experience.
3.2 The Oyster Advisory Commission process
One of the OysterFutures Workgroup’s recommendation was foundational for the OAC process: “Based on its experience with the Consensus Solutions process, the OysterFutures Workgroup recommends that DNR invest in and support this type of process for including stakeholders in decision making. The Workgroup has found that this type of structured engagement with stakeholders and scientists on oyster resource policies and management issues can meet the needs of industry, nonprofit, and government stakeholders and will result in better decisions that have the broad support of more groups” (OSW, 2018). The Maryland state legislature adopted this recommendation: it mandated that MDDNR’s OAC use a Consensus Solutions process with collaborative modeling.
3.2.1 Purpose
3.2.1.1 Impetus and funding
The OAC consensus process was mandated by the Maryland legislature in Senate Bill 803 introduced in 2019 that became statute in the Maryland Code in 2020. The statute specified a two-year timeline for the effort with progress reports and a final report due to the Governor and Maryland legislature by December 1, 2021. The funding for the OAC process was supplied by the State of Maryland through the budget of the MDDNR with grants awarded to the scientific modeling team ($248K) at UMCES and to a facilitation team from Salisbury University ($90K). The funding to UMCES was to conduct a significant effort to develop a simulation model to represent oyster populations in the portion of the Chesapeake Bay under Maryland management. The substantial personnel commitment by MDDNR to the development and implementation of the OAC simulation model could be conservatively valued at $250K, so the cost of the OAC process with collaborative modeling was approximately $590K.
3.2.1.2 Goal
The goal of the OAC was specified in legislation directed at MDDNR: “The Department shall: In coordination with the University of Maryland Center for Environmental Science and the OAC, develop a package of consensus recommendations for enhancing and implementing the fishery management plan for oysters that will be informed by a collaboratively developed, science‐based modeling tool to quantify the long‐term impacts of identified management actions and possible combinations of management actions on: A. Oyster abundance; B. Oyster habitat; C. Oyster harvest; D. Oyster harvest revenue; and E. Nitrogen removal.” The legislation also mandated that “The Oyster Advisory Commission, with the assistance of external conflict resolution and facilitation specialists, shall: 1. Develop a package of consensus recommendations through a facilitated Consensus Solutions process, based on a 75% majority agreement level for each recommendation; 2. Recommend management actions or combinations of management actions to achieve the targets identified in the oyster stock assessment with the goal of increasing oyster abundance; and 3. Review model results for each management action or combination of management actions to inform its recommendations” (Md. Code Ann., Natural Resources §4–215 e.5.i, 2020)7.
OAC members agreed on a vision statement:
“Our goal is to increase oyster abundance/population and habitat in Maryland’s Chesapeake Bay. We will rely on science and stakeholder knowledge to work comprehensively towards:
● Shared stewardship, supporting oysters in harvest areas, aquaculture, and in sanctuaries;
● A healthy ecosystem, and
● A sustainable fishery and aquaculture industries that contribute to the economic health of the state” (MDDNR, 2021).
3.2.2 Partnerships
3.2.2.1 Connection to fisheries management
As required by statute, the OAC consensus process was convened by MDDNR with UMCES scientists and included facilitators who were chosen by MDDNR. The MDDNR Secretary during 2020–2022 convened the OAC meetings. In addition to the Secretary, there was a strong presence of MDDNR at the commission meetings, including the Deputy Secretary, the Shellfish Division Director, and the Deputy Director of the Shellfish Division.
3.2.2.2 OAC composition and membership
The composition of the OAC was specified by statute that named specific organizations as members. Industry representation included one member from each of the 11 county oyster committees (most were licensed oyster fishers), two from state-wide commercial fishing organizations, an aquaculture representative, a seafood buyer, and an aquaculture nutrient credit company. Additional members comprised of nine representatives from nonprofit organizations, including environmental and recreational fishing groups, one legislative commission, and two academic science organizations. Non-voting members included representatives from state (MDDNR) and federal (ACOE, NOAA) agencies tasked with oyster management and restoration as well as four members of the Maryland State legislature. After one organization recused themselves and one organization dissolved, the composition of the 26 voting members was 62% industry and 38% other key groups from nonprofit, university, and government agencies. Four of the OAC members had participated in the OysterFutures program.
3.2.3 Process
3.2.3.1 Leadership team
The leadership team was composed of upper-level management in MDDNR (the Secretary of DNR, Deputy Secretary, Shellfish Division Director and Deputy Director), two scientists from UMCES, and two facilitators (Salisbury University). The leadership team met twice per month to coordinate agendas and follow-up from meetings, with additional meetings as needed. Three members of the leadership team had led or had participated in a Consensus Solutions process with collaborative modeling. Although the facilitators had not run a Consensus Solutions process, they had experience with facilitating group decisions including domestic and international agreements involving industry, governments, and citizens, and working with technical models in nontechnical group settings.
3.2.3.2 OAC meetings
Monthly 3-hour meetings were held from February 2020 to November 2021 for a total of 21 meetings, most held virtually. Additional 3-hr ‘listening session’ meetings were conducted to allow OAC members time to learn about the OAC simulation model (three optional sessions) and to discuss options (two optional sessions). Monthly meetings were held on weekday evenings from 6 to 9 pm to accommodate the schedules of OAC members. In accordance with Maryland’s Open Meeting Act, all monthly meetings were open to the public and included public comment periods. MDDNR also used the OAC to provide feedback on other aspects of oyster management outside of the Consensus Process with collaborative modeling. For example, two (June 2020, June 2021) of the 21 meetings were devoted to results of oyster stock assessments and associated harvest rules and did not include discussion of the consensus process model or options.
The first two OAC meetings were held in-person before the COVID-19 pandemic forced the end of in-person meetings. The first meeting focused on introductions and getting to know one another and airing issues with what had transpired during the process of developing the legislation that were contentious. At the second meeting, OAC members were divided into smaller groups and assigned tasks designed to work on seeking common ground. OAC members also discussed policies for making recommendations within and outside the Consensus Solutions process. Although initial meetings were successful at promoting interactions among OAC members, COVID forced the meetings to change format, which reduced the effectiveness of communication and slowed progress toward finding common ground.
From March 2020 to July 2021 most meetings were held virtually except for two that were hybrid during which OAC members, the leadership team, and the public either attended remotely or in-person at a single site. The final meetings from August 2021 to November 2021 were hybrid. Notably, there was a clear disadvantage to OAC members who had less experience working in a virtual environment or had poor internet connectivity at rural sites. For hybrid meetings, most of the fishing industry representatives chose to attend in-person while other OAC members tended to participate remotely. This separation reduced OAC members’ opportunities to interact with each other outside of the main meeting discussion.
The OAC consensus process (Figure 7) was similar in structure to the OysterFutures process despite differences in meeting lengths and frequency. After the introductory meetings, the agendas focused on reviewing objectives, developing the OAC members’ vision statement, and reviewing the simulation model and the data used to parameterize it. Next, OAC members focused on developing policy and management options – those that could be modeled as well as those that could not. Proposed options were rated for acceptability (1 = acceptable, 2 = minor reservations, 3 = major reservations, 4 = not acceptable), and members were asked to offer their perspective when rating with a 3 or 4 to promote discussion and collaboration toward more acceptable solutions and wording. Options that had an acceptability rating of ≥75% moved forward in the process. Questionaries were used in between virtual meetings to prioritize options for discussion at meetings to optimize use of OAC member time. Of the 104 options that were considered by the OAC members, 30 were not able to be modeled. During the period that options were being discussed and rated, model development progressed with input and guidance from OAC members.
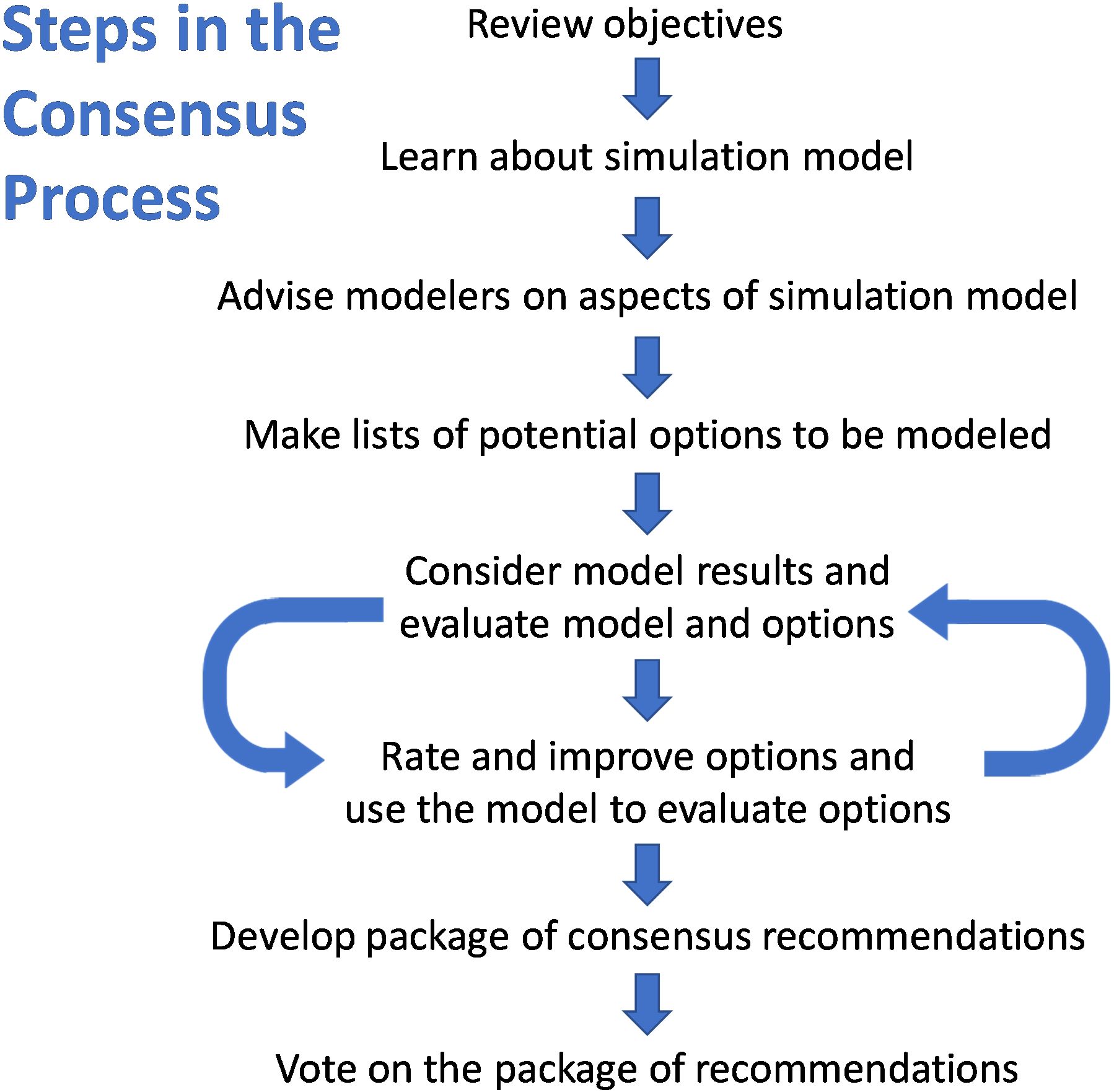
Figure 7. Schematic of the consensus process presented to members of the Oyster Advisory Commission. The looping arrows indicate that evaluation, rating, and revision of model options was intended to be an iterative process that occurred over multiple meetings.
Toward the end of the process, options with 75% or greater acceptability were collected into a package of recommendations. The process enabled the OAC members to converge on viable options with acceptable wording that would pass the 75% agreement threshold. At the last meeting on November 8, 2021, there was a final vote on the package of recommendations, with 80% of the voting members voting for it. Because the agreement level exceeded the 75% threshold, the package was considered accepted. Those that explained why they did not vote in favor of the package stated that they did not think the package fulfilled the legislative mandate or there were no recommendations in the package that emulated Virginia’s strategy for enhancing oyster abundance by moving juvenile oysters from high productivity areas to regions in need of replenishment.
3.2.3.3 Collaborative simulation model
The purpose of the OAC simulation model was to simulate outcomes of potential management options on oyster abundance, habitat, harvest, harvest revenue, and nitrogen removal and to achieve the targets identified in the oyster stock assessment with the goal of increasing oyster abundance. The OAC simulation model was developed from the ground up with input from the OAC (Mace et al., 2024). It included important aspects of the oyster life cycle such as population dynamics, habitat dynamics, and larval transport. Initial conditions (abundance in each region) and estimates of population vital rates were estimated by fitting the OAC simulation model to abundance estimates for each of the harvest reporting regions (Mace et al., 2021). Details of the OAC simulation model formulation can be found in MDDNR (2021) and Mace et al. (2024).
There were many differences between the OysterFutures simulation model and the OAC simulation model. Most notably, the spatial domain for the OAC model was extended to all natural oyster bars located in the portion of the Chesapeake Bay under Maryland management (Figure 1A). After extensive consideration of available habitat data and computational capabilities, the polygons for natural oyster bars were chosen by OAC commissioners as an appropriate representation of oyster habitat and areas where management options would be considered. Within these polygons, information on oyster habitat from a range of data sources (e.g., MDDNR fall oyster survey, side-scan sonar, Maryland Bay Bottom survey data, and MDDNR patent tong survey data) was used to estimate the volume of oyster habitat (shell) available within each polygon.
The OAC simulation model tracked the number of oysters in three stages (spat, small, and market) and the amount of available hard bottom oyster habitat on the 1,082 natural oyster bars and then projected the outcome of management options 25 years into the future starting with oyster abundance estimates from 2020. Oyster settlement depended on the number of larvae that were predicted to arrive on each habitat polygon, a stock-recruitment function for each region, available habitat, and a stochastic term for larval and post settlement mortality. Recruitment also included planting hatchery-reared oysters or wild seed (small oysters moved from one region to another). After settlement, oysters grew and were subject to natural and fishing mortality. Growth and natural mortality rates were different in each region, and natural mortality rates included stochastic variability. Fishing was represented on each bar as a function of oyster density such that a higher fraction of oysters was harvested when they were at high abundance. Separate fishing mortality rate functions were estimated for each of the five main oyster gears used in Maryland (hand tong, patent tong, power dredge, sail dredge, diver), and each bar was restricted to a single gear type (or no gear in the case of sanctuaries). Unlike the OysterFutures model, non-compliance with regulations was not included. The dynamics of hard bottom substrate included loss through dissolution, burial, and dredging and gain through planting activities and oyster growth and mortality.
Similar to the OysterFutures model, a coupled hydrodynamic and larval transport model was used to create connectivity matrices to estimate exchange of oyster larvae among habitat polygons. The Chesapeake Bay ROMS Community Model (ChesROMS; Xu et al., 2012; Scully, 2013, 2016, 2018) was used to predict circulation patterns with a spatial domain that was the entire Chesapeake Bay because these patterns determine the distribution of the larvae that have limited swimming ability. This model had a grid scale that was fine enough to resolve transport of simulated larvae within small tributaries like St. Mary’s and the Little Choptank Rivers, both of which were important sites for oyster restoration. The hydrodynamic model offered the highest possible spatial resolution ROMS model of the Chesapeake Bay that was available at the time. The LTRANS transport model was implemented with the addition of a superindividual approach that simulated the effect of pelagic duration-dependent mortality on larval transport predictions. For more information on hydrodynamic and larval transport models, see MDDNR (2021). Additional comparison of model components between the OysterFutures and OAC models can be found in Supplementary Text S1 of Supplementary Materials.
The final set of OAC model simulations totaled 74 potential options that included planting activities (shell, artificial substrate, spat on shell, and wild seed) on both sanctuary and fishing areas, opening and closing sanctuaries and harvest areas, returning to the 2018 harvest regulations, rotating harvest areas, moving wild seed, dredging buried shell, and combining options. The amount and location (i.e., oyster bar) of shell, alternate substrate, hatchery spat on shell, and wild seed plantings were specified for each option. For each option, 200 simulations were run to portray stochastic variability in natural mortality, recruitment, and the relationship of fishing mortality with oyster density. The stochastic parameters were assumed to be normally distributed on the loge scale and spatial autocorrelation was applied among regions separately for the natural mortality, fishing mortality, and recruitment processes.
The COVID pandemic caused delays in model formulation and options development and testing, including increasing the time to gather, process, and analyze data needed for the model. In addition, time was lost due to the change in meeting format from in-person to virtual, resulting in less time to review and explain model results with OAC members and to receive feedback from OAC members about model options. Also, the short (3-hr) meetings at the end of the day did not support comprehensive review and substantive discussion of the model and options. Moreover, the virtual format prevented discussion before and after the meetings. With a fixed legislatively-mandated deadline, these restrictions in time, attention, and interaction ultimately reduced the number of runs of the simulation model that were possible and the ability of the OAC members to converge on options or combinations of options that would best meet the needs of multiple community groups and achieve the spirit of the legislation to enhance oyster abundances state-wide. Despite these challenges, OAC members did use the model results to inform their decisions on three of the 19 options that were included in the consensus package of recommendations.
3.2.4 Products
3.2.4.1 OAC recommendations
OAC members made 19 specific recommendations to the Maryland State Legislature (MDDNR, 2021). Recommendations that had not been simulated included: enhance the shell and substrate resource; augment fisheries-independent monitoring of oyster resource and marking boundaries; boost cross-sector collaboration, processor capabilities and strategies to mitigate disease outbreaks; promote nutrient crediting; conduct outreach and training in minority communities; and fill knowledge gaps through science. Recommendations based on the model simulations included keeping the oyster fishery open, sustaining the fishery-led practice of planting shell and spat-on-shell on oyster fishery bottom, and:
“Over the next 25 years, a combination of replenishment, restoration and aquaculture activities should be collectively planned and undertaken in Eastern Bay, with an equal amount of funding for spat planting in sanctuaries ($1M annually adjusted for inflation) and for spat and shell planting on fishery bars ($1M annually adjusted for inflation) in addition to current replenishment and restoration activities. The effectiveness of this option should be evaluated every 5 years.”
The simulation that formed the basis of this recommendation achieved all of the goals stipulated in the legislation: rebuilding of oyster populations, enhanced harvest revenue, increased habitat, and reduced nitrogen in Maryland’s Chesapeake Bay after 25 years (Figure 8). Eastern Bay is located on the Eastern Shore of Maryland (Figure 1A) and formerly supported high oyster abundance and harvest.
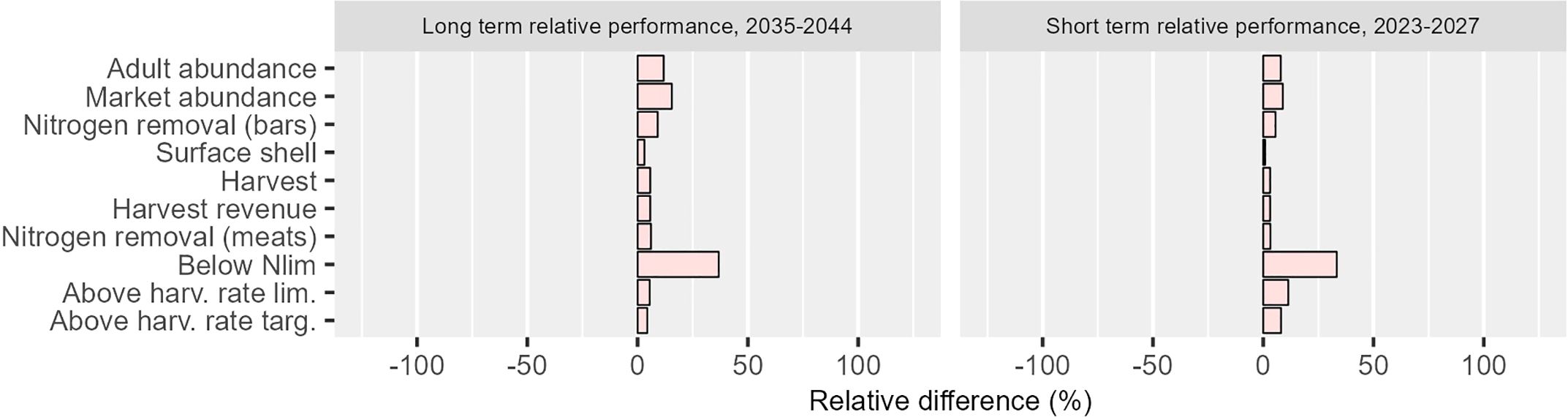
Figure 8. OAC model simulation results for the “Eastern Bay $1M for restoration (spat) and $1M fishery (shell and spat)” option with bars representing improved (positive) or reduced (negative) performance relative to the status quo. For this option, the OAC model simulated that $1,000,000 was spent each year on planting hatchery spat in sanctuaries in Eastern Bay (250 million hatchery spat planted annually at 6 million spat per acre), and $1,000,000 was spent each year on planting shell and hatchery spat in public fishery areas in Eastern Bay ($400,000 spent on planting 100 million hatchery spat at 1 million spat per acre and $600,000 spent on planting 60 acres with shell at 2,000 bushels per acre). The bottom three bars represent fishery management objectives: ‘Below Nlim’ = below the limit abundance reference point, ‘Above harv. rate lim.’ = above harvest rate limit reference point, ‘Above harv. rate targ.’ = above harvest rate target reference point. Left panels indicate model predictions for long term relative performance; right panels indicate short term relative performance.
3.2.4.2 Additional legislation
The OAC member’s recommendations were incorporated into new legislation aimed at improving the oyster resource, management, and industry. The legislation included provisions to support oyster shell retention within the state, to recycle oyster shells, to provide financing for oyster processing projects, to conduct bottom surveys of oyster habitat, to create a unified substrate plan, to conduct research on natural and artificial substrates (e.g., shell, stone) and to expand an oyster hatchery. Most of the items in the legislation stemmed directly from the OAC members’ consensus recommendations, especially this one:
“In recognition of the final report, dated December 1, 2021, of the Oyster Advisory Commission’s consensus recommendations, over the next 25 years, a combination of replenishment, restoration, and aquaculture activities should be collectively planned and undertaken in Eastern Bay, with an equal amount of funding for spat planting in sanctuaries ($1,000,000 annually adjusted for inflation) and for spat and shell planting on fishery bars ($1,000,000 annually adjusted for inflation) in addition to current replenishment and restoration activities.”8 The effectiveness of these activities “shall be evaluated every 5 years.”7
Funding for this consensus recommendation was appropriated in the 2024 Maryland budget and efforts for spat planting in fisheries areas and sanctuaries in Eastern Bay will be underway in 2024. While this does not address oyster populations in other areas of Maryland, it is a major step forward that will be guided by a Consensus Solutions process convened by the Oyster Recovery Partnership with support from the National Fish and Wildlife Foundation.
4 Discussion
Overall, the facilitated Consensus Solutions processes with collaborative modeling for both OysterFutures and the OAC resulted in consensus recommendations to improve oyster management and conservation in Maryland despite a history of conflict among participants and external confounding factors (e.g., the COVID-19 pandemic). While every process must adapt to specific topics, locations, interest groups and governance structures (Hamilton et al., 2015; Kenny and Castilla-Rho, 2022), the outcomes of these programs indicate that the Consensus Solutions process with collaborative modeling offers a framework that could aid coordinated cross-sector natural resource decisions with scientific basis and broad community support.
4.1 Key similarities
Both the OysterFutures and OAC processes were examples of successful efforts in which collaborative governance (e.g., Ansell and Gash, 2008; Gutiérrez et al., 2011; Newig et al., 2019) was combined with participatory modeling (e.g., Voinov and Bousquet, 2010; Voinov et al., 2016; Goethel et al., 2019; Deith et al., 2021; Wilberg et al., 2024) and resulted in packages of recommendations with broad community support (i.e., >75% agreement). The overall structure of both OysterFutures and OAC processes were similar (Supplementary Box S1). Combining the Consensus Solutions process with collaborative modeling met outstanding needs for participatory modeling identified by Hedelin et al. (2021), including “facilitation of a multi-value perspective within a democratic process, and the integration across organizations within a governance system.” The Consensus Solutions process included ground rules for interactions, involved experienced facilitators, incorporated the time needed to make the process as transparent as possible, and ensured key representatives were present. Van Cauwenbergh et al. (2018) also found that embedding a modeling tool within a facilitated workshop process led to transparency, trust, and knowledge generation within a workgroup.
Key to both processes were the neutral professional facilitators who led the groups through the process, the 75% agreement threshold, and a rating system that allowed ideas to be brought up, evaluated, discussed, and improved upon. The processes provided time for participants to learn from each other about their goals and constraints and to discuss with scientists the empirical evidence relevant to different ideas and options. There was a surprising difference of opinion within groups regarding different options. However, having the space to listen, clarify ideas, and offer suggestions in a respectful forum was a key aspect of the process. In addition, the 75% agreement threshold coupled with percent representation by community groups of no more than ~60% ensured that options that moved forward in the consensus process were supported by representatives from multiple community groups. This is clearly reflected in the unanimous adoption of the final package of OysterFutures recommendations.
An important similarity between OysterFutures and the OAC processes was the strong support of the natural resource agency for the consensus process and the presence of the key community group representatives. Involvement of high-level managers at MDDNR lent credibility to the processes by giving participants the confidence that their recommendations would be heard and taken seriously. In the case of the OAC, the MDDNR Secretary was a champion of the process who worked to keep the OAC members engaged and the process on track, both at meetings and behind the scenes. In addition, membership of OysterFutures and OAC groups included strong and respected leaders within community groups, including those from the commercial industry. Gutiérrez et al. (2011) found that strong leadership from within the commercial fishery was the most important factor contributing to the success of fisheries co-management efforts. Substantial commercial fishery representation was a feature of both the OysterFutures and OAC processes.
Another key similarity between the OAC and OysterFutures processes was the co-development of simulation models that led to new understandings of the response of the oyster resource and industry to management actions. These model results allowed participants to reassess and realign their thinking about how the coupled human-oyster system would respond to management changes. For example, the OysterFutures simulation model predicted that ‘win-win-win’ solutions exist that simultaneously benefit the oyster resource, the oyster industry, and water quality. This ‘myth busting’ finding provided a new goal for policy and management for the oyster fishery and management in Maryland. Importantly, both the OysterFutures and OAC models indicated that revitalization of oyster habitat would be needed for any large-scale recovery of oysters in Maryland’s Chesapeake Bay.
4.2 Major differences
Despite the overall similarities between the OysterFutures and OAC processes, there were clear differences, some of which stemmed from the impetus for the work: the OysterFutures program was funded by a research project whereas the OAC process was legislatively mandated with real-world consequences for oyster fishers’ livelihoods. In addition, participation in OysterFutures was voluntary and coordinated by the leadership team whereas organizations that participated in the OAC were specified in legislation with organizations nominating their representative. The meeting structures also differed between the two processes. The OAC process was run by MDDNR and was subject to the agency’s requirements (e.g., open meetings with public comment) and its historical process of advisory body meetings (e.g., 3-hr evening meetings). The duration and frequency of the meetings were quite different, as were the total time invested in the 2-year processes: OysterFutures Workgroup met nine times in eight 1.5-day meetings and one 1-day meeting (104 hrs total) whereas OAC members met for the consensus process in 19 3-hr meetings and two 3-hr optional listening sessions (63 hrs total).
Another clear difference was the level of support for the final package of recommendations that likely reflected the dissimilarities in the processes. For OysterFutures, 100% of the voting workgroup members supported the package of recommendations. For the OAC process, 80% of the OAC members voted in favor. This outcome may have reflected the higher stakes of the legislatively-mandated OAC process compared to those of the OysterFutures program. In addition, the differences in level of support likely reflected how much members were able to listen and speak with each other directly – OysterFutures Workgroup members met for 41 more hours than OAC members.
The quality of interactions also differed between the two processes. OysterFutures members listened to each other in person at every meeting and shared social time during breaks and meals whereas interactions by OAC members were severely hampered by the COVID pandemic and the resulting virtual meetings. OAC commissioners did not share meals, could not talk face-to-face for much of the time with those in disagreement, and were rarely able to have informal conversations. In addition, the OysterFutures process was more flexible without a fixed deadline: a final OysterFutures meeting was added to accommodate additional discussion and collaboration toward consensus. The unstructured time for participants to listen and speak with each other allowed ‘real speak’ – talking with honesty, integrity, and emotion – that inspired creativity and was an important part of finding common ground. Both the extra meeting during OysterFutures and the additional listening sessions for the OAC were process strategies that provided members with the needed time to come to agreement and, in the case of OysterFutures, to narrow down modeled options to those that were most likely to perform best. More time for discussion would have benefited the OAC process, especially because of the spatial challenge faced by OAC members – it was likely harder for OAC members to share knowledge and build relationships over a much larger area (11 counties, Figure 1A) compared to OysterFutures Workgroup members (two counties, Figure 1B). Extensions of the process were needed for other groups to achieve their transformative goals (Hare et al., 2003; Abrami et al., 2022).
Fewer iterations of model options were able to be performed for the OAC compared to OysterFutures Working group due to time constraints as well as the complexity of developing the simulation model with OAC members during the COVID pandemic. The modeling team had substantially less discussion with participants during the OAC process than during OysterFutures, which likely affected the responsiveness of the model. This reduction in the time to iteratively develop the model and run model options reduced the OAC members’ opportunity to collectively converge on multiple options that would meet legislative goals. Using questionaries in between OAC meetings partially offset the lack of time during meetings to rate options. This served to quickly identify areas of agreement and disagreement, as suggested by Hayes and Wainger (2022), allowing OAC members to focus effort on options with the most agreement in the limited time allotted.
Another challenge faced by the OAC consensus process was the need for the OAC to complete other tasks, as required by MDDNR, that were carried out under a different set of process rules (Roberts Rules and a 50% majority vote). These other tasks included review of the Maryland oyster stock assessment (MDDNR, 2020), feedback on management actions related to harvest boundaries, and response to statements in newspaper articles. The cost of this separate process, while serving MDDNR’s other needs of the OAC, was two-fold. First, OAC members needed to agree on rules and procedures for this separate process, and second, it created confusion and controversy late in the consensus process. Both resulted in a significant loss of time and trust that could have been spent building agreement on oyster management options. These factors likely contributed to the OAC’s recommendations being more general in scope compared to the OysterFutures Workgroup’s recommendations.
4.3 Lessons learned
The OysterFutures and OAC consensus efforts with collaborative modeling resulted in clear ‘lessons’ that were apparent from both processes (Supplementary Box S2). Fundamental to both was the need for facilitators as neutral parties to help plan and run the meetings (Hare, 2011; Basco-Carrera et al., 2017; Wilberg et al., 2024). The modeling team being present at meetings to clearly present the model, discuss the options, and answer questions also was important (Goethel et al., 2019).
Both the OysterFutures and OAC efforts clearly demonstrated that process is important – the process can either bring people together or create conflict. For OysterFutures, some members of the Workgroup testified against each other in the Maryland legislature during the OysterFutures program and, despite the conflict, were able to unanimously agree on recommendations that would improve the oyster resource, industry and environment. During the OAC, the portion of the process conducted under Roberts Rules was divisive while the consensus process resulted in collaborative recommendations – with the same people involved. Consensus processes create decision-making transparency and inclusiveness, and can lead to mutually beneficial outcomes, all of which are important for a process to be perceived as fair (Hearld et al., 2013). The Delaware Bay oyster fishery provides a long-standing example of a process where scientists, managers and fishers work successfully to review stock assessment results and set annual quotas (e.g., Bushek et al., 2024; Morson et al., 2023).
Implementation of consensus recommendations was more likely if the group membership included those able to implement the recommendations. For the OAC, their consensus recommendations had a clear and rapid impact: some of the 2021 recommendations were incorporated directly into bipartisan legislation in 2022 with appropriations in 2024. The rapid transition from OAC recommendations to appropriations likely was aided by the facts that 1) the MD legislature had called for the OAC consensus process and was primed to receive the OAC recommendations, 2) the convenor of the OAC consensus process, the Secretary of the MDDNR, was on the MD Governor’s Cabinet, and 3) non-voting members of the OAC included four legislators (two from the Maryland State Senate and two from the Maryland State House of Delegates) who kept apprised of the group’s progress. Hence, when a consensus process is called for by a Legislature, the results of the process can result in legislation that is backed by broad community support. In states where fishery management is strongly influenced by the legislature like in Maryland, a consensus process with collaborative modeling may be a useful avenue for thoughtful and knowledge-based change.
The collective process of sharing and combining different perspectives and knowledge enabled participants to see the many facets of a situation and design management strategies that were acceptable to multiple groups. This collective knowledge can be symbolized as a tree (Supplementary Figure S3): a person standing on one side of a tree does not see knots, while someone on the other sees many – and both people are correct. All have different views that, when combined, lead to a fuller understanding. This deepened understanding was aided by the structured Consensus Solutions process with collaborative modeling as well as the respect, active listening, and kindness of participants that helped to overcome barriers (Kenny and Castilla-Rho, 2022). The role of the modeling team as collaborators and supporters of community group representatives rather than experts tasked with decision making aided the process.
4.4 Barriers
There are barriers to the adoption of collaborative modeling for natural resource management, including substantial commitment from community group representatives, financial resources needed to support facilitation and modeling teams, the amount of time needed, and the chance that the process may not meet needed timelines (Hare, 2011; Abrami et al., 2022; Kenny and Castilla-Rho, 2022). In the case of OysterFutures and the OAC, both processes took approximately two years. The lower cost of the OAC process compared to OysterFutures was due to prior development of the OysterFutures simulation model framework, the substantial personnel commitment by MDDNR to OAC simulation model development and implementation, and the difference between federal and state indirect cost rates. In addition, the OysterFutures process was conducted within a research program that had multiple co-investigators, graduate students and postdoctoral researchers. In contrast, the OAC had a smaller budget for academic partners, with staff from MD DNR assisting with data analyses, simulation model development and implementation, and no students were supported. In both processes, lead scientists devoted considerable time and effort to both modeling and communicating results.
Risk of failure is another barrier to the adoption of collaborative modeling – the risk that the process may not come to a successful conclusion (Kolody et al., 2008; Hare, 2011). The use of the Consensus Solutions process to structure deliberations and move the groups toward agreement served to counteract the risk of failure for both OysterFutures and OAC efforts. As Goethel et al. (2019) recommend, leadership teams can mitigate the risk of failure by using a consensus-based process, by ensuring key representatives are at the table, by ensuring facilitators maintain focus on end goals and respectful discussions, and by supporting the different groups in the process equitably. Another risk is if community groups do not honor their agreement after the process ends, making the next process more difficult. From the beginning, the expectation needs to be set among the representatives that the agreement will go forward and be honored by both the individuals at the table and the organizations that they represent.
4.5 Next steps
An enhancement to a Consensus Solutions process with collaborative modeling would be to specifically evaluate diversity within the community groups and then ensure that their representation is inclusive and diverse in terms of race, gender, age, location, gear use group, and interest in the resource. This evaluation of representativeness of the workgroup should be part of initial assessments before the consensus process begins. Additional enhancements could be undertaken once the process is underway to bridge divides between participants such as increase time for unstructured discussions and for reflection to enhance learning (Kenny and Castilla-Rho, 2022). For example, shared history exercises (as in OysterFutures) and/or field trips to participants’ workplaces would provide opportunities to listen and learn from each other. During and after the process, social science and decision science could be applied to understand which process strategies were most beneficial for enhancing collective knowledge and evaluate if consensus recommendations were consistent with individuals’ goals.
Future efforts with the Consensus Solutions process with collaborative modeling could be enhanced with careful attention to meeting timing and duration with scheduled unstructured time for participants to interact, and with support for different meeting types. Although daytime in-person meetings were more efficient than evening virtual meetings, virtual meetings were necessary during the COVID-19 pandemic. The clear difference in access and experience in the use of virtual technology among OAC members created disproportionate advantage to some community group members over others. If virtual meetings are necessary, then training, hardware, and associated staffing and funding should be part of the project expense.
The OysterFutures and OAC processes successfully produced consensus recommendations in the short term, but the long-term benefits of the processes – such as an improved oyster resource, fishery, and ecosystem services – remain to be assessed. Notably, in a state where the early history of the fisheries management in Maryland was in response to conflict over oysters (Kennedy and Breisch, 1983), the package of consensus recommendations of the OysterFutures participants was a landmark agreement. In addition, although the OAC process did not achieve all that was intended, the package of consensus recommendations was a substantial achievement considering the barriers that the process faced. The process resulted new funding for oyster fisheries replenishment and sanctuary enhancement in Eastern Bay. In the long-term, the benefits of the OysterFutures and OAC processes need to be measured against the costs of conflict among community groups and the lost opportunities that stem from lack of cooperation. Future work to quantify benefits should include the costs of these lost opportunities as well as the value of simultaneously enhancing the economies of local communities, improving habitat for the oyster resource, and promoting ecosystem services provided by oysters.
4.6 Summary
This comparison of the OysterFutures and OAC processes provides further evidence that a structured and facilitated process with participatory modeling can be applied to create marine regulations and policies with broad community support. In addition, when knowledge from the community groups, managers and scientists was integrated into the collaboratively-built simulation model, new ideas and understandings emerged, some of which changed the way people thought about the resource and its response to human actions. Many other technically complex topics around which controversy exist are candidates for a Consensus Solutions process with collaborative modeling such as, for example, pollution control, dredge disposal, and marine protected area siting. As seen here, these processes, when run at the behest of legislatures and/or management agencies, can help advance marine sustainability by developing well-thought-out regulations and policies that have the community support needed to move rapidly through regulatory and legislative processes.
Data availability statement
The original contributions presented in the study are included in the article/Supplementary Material. Further inquiries can be directed to the corresponding author.
Ethics statement
The studies involving humans were first reported in Goelz et al. (2020a, 2020b) and Hayes and Wainger (2022) and were approved by William & Mary Institutional Review Board and University of Maryland Institutional Review Board. The studies were conducted in accordance with the local legislation and institutional requirements. The participants provided their written (W&M) or oral (UMD) informed consent to participate in these studies.
Author contributions
EN: Conceptualization, Formal analysis, Funding acquisition, Investigation, Writing – review & editing, Writing – original draft, Visualization, Supervision, Software, Project administration, Methodology. MW: Writing – review & editing, Writing – original draft, Visualization, Validation, Supervision, Software, Project administration, Methodology, Investigation, Funding acquisition, Formal analysis, Data curation, Conceptualization. JB: Writing – review & editing, Writing – original draft, Supervision, Project administration, Methodology, Investigation, Funding acquisition, Conceptualization. LW: Writing – review & editing, Writing – original draft, Supervision, Project administration, Methodology, Investigation, Funding acquisition, Formal analysis, Conceptualization. JC: Conceptualization, Funding acquisition, Investigation, Methodology, Project administration, Supervision, Writing – review & editing. RJ: Writing – review & editing, Project administration, Methodology, Investigation, Funding acquisition, Conceptualization. CH: Conceptualization, Investigation, Methodology, Writing – review & editing. RG: Writing – review & editing, Visualization, Software, Methodology, Investigation. RH: Writing – review & editing, Supervision, Methodology, Investigation, Funding acquisition, Conceptualization. TG: Writing – review & editing, Writing – original draft, Visualization, Methodology, Investigation. TH: Writing – review & editing, Supervision, Project administration, Methodology, Investigation, Funding acquisition, Conceptualization. MM: Writing – review & editing, Visualization, Software, Methodology, Investigation. MD: Writing – review & editing, Project administration, Methodology, Investigation. NF: Writing – review & editing, Project administration, Methodology, Investigation. BP: Investigation, Methodology, Project administration, Writing – review & editing.
Funding
The author(s) declare financial support was received for the research, authorship, and/or publication of this article. The material in this paper is based upon work supported by the National Science Foundation (NSF) under Coastal SEES grants OCE-1427019, OCE-1427012, and OCE-1427125 that supported the OysterFutures program and by Maryland Department of Natural Resources (MDDNR) grant K00B0600131 that contracted support for the Oyster Advisory Commission. Paper preparation and publication was supported by National Oceanic and Atmospheric Administration grant 723847-71283. Any opinions, findings and conclusions or recommendations expressed in this material are those of the author(s) and do not necessarily reflect the views of the NSF, MDDNR, NOAA, or members of the OysterFutures Stakeholder Workgroup and Oyster Advisory Commission.
Acknowledgments
The authors are grateful for the efforts of the members of the OysterFutures and OAC groups and the staff of UMCES, UMCES Integration Application Network, and MDDNR. We appreciate the helpful suggestions of the editor and reviewers that strengthened this paper. This paper is University of Maryland Center for Environmental Science contribution no. 6411.
Conflict of interest
Author JB was employed by the company Facilitated Solutions, LLC. Author NF was employed by the company The Eymit Group.
The remaining authors declare that the research was conducted in the absence of any commercial or financial relationships that could be construed as a potential conflict of interest.
Publisher’s note
All claims expressed in this article are solely those of the authors and do not necessarily represent those of their affiliated organizations, or those of the publisher, the editors and the reviewers. Any product that may be evaluated in this article, or claim that may be made by its manufacturer, is not guaranteed or endorsed by the publisher.
Supplementary material
The Supplementary Material for this article can be found online at: https://www.frontiersin.org/articles/10.3389/fmars.2024.1423534/full#supplementary-material
Footnotes
- ^ Creating a Unified Building Code in Florida. https://intersector.com/case/buildingcode_florida/
- ^ Apalachicola Bay System Initiative: Community Advisory Board. https://marinelab.fsu.edu/media/4718/absi_cab_strategies_worksheet_24-feb-2021.pdf
- ^ North Florida Regional Water Supply Partnership: https://www.northfloridawater.com/
- ^ Consensus Solutions projects: https://facilitatedsolutions.org/?page_id=1179
- ^ Coastal SEES (Coastal SEES) Program Solicitation: https://www.nsf.gov/pubs/2014/nsf14502/nsf14502.htm).
- ^ UMCES Integration Application Network: https://ian.umces.edu/
- ^ Natural Resources – Fishery Management Plans – Oysters (Per Natural Resources Article § 4-215(e)(5)(iii)1, Annotated Code of Maryland, SB 808, Chapter 598 and HB 911, Chapter 597, MSAR 12769). https://mgaleg.maryland.gov/2022RS/Statute_Web/gnr/gnr.pdf
- ^ MD Senate Bill 830 of 2022. https://legiscan.com/MD/text/SB830/2022
References
Abrami G., Daré W., Ducrot R., Salliou N., Bommel P. (2022). Participatory modelling, in: The Routledge Handbook of Research Methods for Social-Ecological Systems. Routledge, 189–204. ebook ISBN: 9781003021339. doi: 10.4324/9781003021339-16
Andrews J. D., Hewatt W. G. (1957). Oyster mortality studies in Virginia. II. The fungus disease caused by Dermocystidium marinum in oysters of Chesapeake Bay. Ecol. Monogr. 27, 2–25. Available at: https://www.jstor.org/stable/1948568.
Andrews J. D., Wood J. L. (1967). Oyster mortality studies in Virginia. VI. History and distribution of Minchinia nelsoni, a pathogen of oysters, in Virginia. Chesapeake Sci. 8, 1–13. doi: 10.2307/1350351
Ansell C., Gash A. (2008). Collaborative governance in theory and practice. J. Public Administration Res. Theory 18, 543–571. doi: 10.1093/jopart/mum032
Basco-Carrera L., Warren A., van Beek E., Jonoski A., Giardino A. (2017). Collaborative modelling or participatory modelling? A framework for water resources management. Environ. Model. Software 91, 95–110. doi: 10.1016/j.envsoft.2017.01.014
Bushek D., Gius J., Morson J. (Eds.) (2024). Stock Assessment Workshop, New Jersey Delaware Bay Oyster Beds (26th SAW) (Port Norris, NJ: Haskin Shellfish Research Laboratory, Rutgers, The State University of New Jersey), 79. Available at: https://hsrl.rutgers.edu/wp-content/uploads/2024/04/SAW2024.pdf. February 1-2, 2024pp.
Cerco C. F., Noel M. R. (2007). Can oyster restoration reverse cultural eutrophication in Chesapeake Bay? Estuaries Coasts 30, 331–343. doi: 10.1007/BF02700175
Damiano M. D., Wilberg M. J. (2019). Population dynamics of eastern oysters in the Choptank River Complex, Maryland during 1989–2015. Fisheries Res. 212, 196–207. doi: 10.1016/j.fishres.2018.12.023
Deith M. C. M., Skerritt D. J., Licandeo R., Duplisea D. E., Senay C., Varkey D. A., et al. (2021). Lessons learned for collaborative approaches to management when faced with diverse stakeholder groups in a rebuilding fishery. Mar. Policy 130, 104555. doi: 10.14288/1.0434617
Doering K. L., Wilberg M. J., Liang D., Tarnowski M. (2021). Patterns in oyster natural mortality in Chesapeake Bay, Maryland using a Bayesian model. Fisheries Res. 236, 105838. doi: 10.1016/j.fishres.2020.105838
Ford S. E., Tripp M. R. (1996). Disease and defense mechanisms, in: The Eastern Oyster, Crassostrea virginica (College Park MD.: Maryland Sea Grant), 581–660, ISBN: ISBN: 9780943676616.
Fournier D. A., Skaug H. J., Ancheta J., Ianelli J., Magnusson A., Maunder M. N., et al. (2012). AD Model Builder: using automatic differentiation for statistical inference of highly parameterized complex nonlinear models. Optimization Methods Software 27, 233–249. doi: 10.1080/10556788.2011.597854
Fulford R. S., Breitburg D. L., Luckenbach M., Newell R. I. (2010). Evaluating ecosystem response to oyster restoration and nutrient load reduction with a multispecies bioenergetics model. Ecol. Appl. 20, 915–934. doi: 10.1890/08-1796.1
Fulford R. S., Breitburg D. L., Newell R. I., Kemp W. M., Luckenbach M. (2007). Effects of oyster population restoration strategies on phytoplankton biomass in Chesapeake Bay: a flexible modeling approach. Mar. Ecol. Prog. Ser. 336, 43–61. doi: 10.3354/meps336043
Gawde R., North E. W., Hood R., Long W., Wang H., Wilberg M. (2024). A high resolution hydrodynamic-biogeochemical-oyster-filtration model predicts that the presence of oysters (Crassostrea virginica) can improve, or reduce, water quality depending upon oyster abundance and location. Ecol. Model. 496, 110833. doi: 10.1016/j.ecolmodel.2024.110833
Goelz T., Hartley T., Scheld A., Carboni I. (2020a). The development of attitudes toward scientific models during a participatory modeling process–the impact of participation and social network structure. Front. Mar. Sci. 7. doi: 10.3389/fmars.2020.00644
Goelz T., Scheld A., Hartley T., Carboni I. (2020b). Understanding structural factors and actor attributes that impact the development of cohesion within a participatory modeling process. Coast. Manage. 48, 577–600. doi: 10.1080/08920753.2020.1823669
Goethel D. R., Lucey S. M., Berger A. M., Gaichas S. K., Karp M. A., Lynch P. D., et al. (2019). Closing the feedback loop: on stakeholder participation in management strategy evaluation. Can. J. Fisheries Aquat. Sci. 76, 1895–1913. doi: 10.1139/cjfas-2018-0162
Gray S., Voinov A., Paolisso M., Jordan R., BenDor T., Bommel P., et al. (2018). Purpose, processes, partnerships, and products: four Ps to advance participatory socio-environmental modeling. Ecol. Appl. 28, 46–61. doi: 10.1002/eap.1627
Gutiérrez N. L., Hilborn R., Defeo O. (2011). Leadership, social capital and incentives promote successful fisheries. Nature 470, 386–389. doi: 10.1038/nature09689
Hamilton S. H., ElSawah S., Guillaume J. H., Jakeman A. J., Pierce S. A. (2015). Integrated assessment and modelling: overview and synthesis of salient dimensions. Environ. Model. Software 64, 215–229. doi: 10.1016/j.envsoft.2014.12.005
Hare M. (2011). Forms of participatory modelling and its potential for widespread adoption in the water sector. Environ. Policy Governance 21, 386–402. doi: 10.1002/eet.590
Hare M., Letcher R. A., Jakeman A. J. (2003). Participatory modelling in natural resource management: a comparison of four case studies. Integrated Assess. 4, 62–72. doi: 10.1076/iaij.4.2.62.16706
Hayes C., Wainger L. (2022). Comparing multi-criteria decision analysis to group negotiations in fisheries co-management. Mar. Policy 138, 104997. doi: 10.1016/j.marpol.2022.104997
Hearld L. R., Alexander J. A., Bodenschatz L., Louis C. J., O'Hora J. (2013). Decision-making fairness and consensus building in multisector community health alliances: A mixed-methods analysis. Nonprofit Manage. Leadership 24, 139–161. doi: 10.1002/nml.21086
Hedelin B., Gray S., Woehlke S., BenDor T. K., Singer A., Jordan R., et al. (2021). What’s left before participatory modeling can fully support real-world environmental planning processes: A case study review. Environ. Model. Software 143, 105073. doi: 10.1016/j.envsoft.2021.105073
Hemmerling S. A., Barra M., Bienn H. C., Baustian M. M., Jung H., Meselhe E., et al. (2020). Elevating local knowledge through participatory modeling: active community engagement in restoration planning in coastal Louisiana. J. Geographical Syst. 22, 241–266. doi: 10.1007/s10109-019-00313-2
Henly-Shepard S., Gray S. A., Cox L. J. (2015). The use of participatory modeling to promote social learning and facilitate community disaster planning. Environ. Sci. Policy 45, 109–122. doi: 10.1016/j.envsci.2014.10.004
Ihde T. F., Wilberg M. J., Secor D. H., Miller. T. J. (2011). FishSmart: harnessing the knowledge of stakeholders to enhance U.S. marine recreational fisheries with application to the Atlantic king mackerel fishery, in: Proceedings of the 5th World Recreational Fisheries Conference. Am. Fisheries Soc. Symposium 73, 75–93. Available at: https://wilberglab.cbl.umces.edu/pubs/Ihde%20et%20al%202011b.pdf.
Irwin B. J., Wilberg M. J., Bence J. R., Jones M. L. (2008). Evaluating alternative harvest policies for yellow perch in southern Lake Michigan. Fisheries Res. 94, 267–281. doi: 10.1016/j.fishres.2008.05.009
Irwin B. J., Wilberg M. J., Jones M. L., Bence J. R. (2011). Applying structured decision making to recreational fisheries management. Fisheries 36, 113–122. doi: 10.1080/03632415.2011.10389083
Jackson J. B., Kirby M. X., Berger W. H., Bjorndal K. A., Botsford L. W., Bourque B. J., et al. (2001). Historical overfishing and the recent collapse of coastal ecosystems. Science 293, 629–637. doi: 10.1126/science.1059199
Jackson M., Owens M. S., Cornwell J. C., Kellogg M. L. (2018). Comparison of methods for determining biogeochemical fluxes from a restored oyster reef. PloS One 13, e0209799. doi: 10.1371/journal.pone.0209799
Kellogg M. L., Dreyer J. C., Turner C., Pant M., Ross P. G., Birch A., et al. (2019). Oyster reef ecosystem services: Macrofauna utilization of restored oyster reefs - Harris Creek, Maryland, USA (Virginia Institute of Marine Science, William & Mary. A final report to: NOAA Chesapeake Bay Office) (Accessed 11/5/2023).
Kennedy V. S., Breisch L. L. (1983). Sixteen decades of political management of the oyster fishery in Maryland’s Chesapeake Bay. J. Environ. Manage. 16, 153–171.
Kenny D. C., Castilla-Rho J. (2022). No stakeholder is an island: human barriers and enablers in participatory environmental modelling. Land 11, 340. doi: 10.3390/land11030340
Kirby M. X. (2004). Fishing down the coast: historical expansion and collapse of oyster fisheries along continental margins. Proc. Natl. Acad. Sci. 101, 13096–13099. doi: 10.1073/pnas.040515010
Kolody D., Polacheck T., Basson M., Davies C. (2008). Salvaged pearls: lessons learned from a floundering attempt to develop a management procedure for Southern Bluefin Tuna. Fisheries Res. 94, 339–350. doi: 10.1016/j.fishres.2008.08.016
La Peyre M., Furlong J., Brown L. A., Piazza B. P., Brown K. (2014). Oyster reef restoration in the northern Gulf of Mexico: extent, methods and outcomes. Ocean Coast. Manage. 89, 20–28. doi: 10.1016/j.ocecoaman.2013.12.002
Luckenbach M., Mann R. L., Wesson J. A. (1999). Oyster Reef Habitat Restoration : a synopsis and synthesis of approaches; proceedings from the symposium, Williamsburg, Virginia, April 1995. Virginia Institute of Marine Science, William & Mary. Available at: https://scholarworks.wm.edu/reports/22/.
Luckenbach M. W., Coen L. D., Ross J. P., Stephen J. A. (2005). Oyster reef habitat restoration: relationships between oyster abundance and community development based on two studies in Virginia and South Carolina. J. Coast. Res. SI 40, 64–78. Available at: https://www.jstor.org/stable/25736616.
Mace III, M.M., Doering K. L., Wilberg M. J., Larimer A., Marenghi F., Sharov A., et al. (2021). Spatial population dynamics of eastern oyster in the Chesapeake Bay, Maryland. Fisheries Res. 237, 105854. doi: 10.1016/j.fishres.2020.105854
Mace III, M.M., Wilberg M. J., North E. W., Gawde R., Jesse J., Wainger L., et al. (2024). Developing a fine-scale spatial operating model of eastern oyster population dynamics in Chesapeake Bay, Maryland, U.S.A. Fisheries Res. 279, 107145. doi: 10.1016/j.fishres.2024.107145
MacKenzie C. L. (1970). Oyster culture in Long Island Sound. 1966-69. Commercial Fisheries Rev. 32, 27–40. Available at: https://spo.nmfs.noaa.gov/sites/default/files/pdf-content/mfr3213.pdf.
MacKenzie C. L. (1997). “The history, present condition, and future of the molluscan fisheries of North and Central America and Europe,” in Atlantic and Gulf Coasts (No. 127), NOAA Technical Report, Silver Spring, Maryland, Vol. 1. Available at: https://spo.nmfs.noaa.gov/sites/default/files/tr127opt.pdf.
MDDNR (Maryland Department of Natural Resources) (2020). 2020 Update: Stock assessment of the eastern oyster, Crassostrea virginica, in the Maryland waters of Chesapeake Bay. Summary Rep. 13 pp. Available at: https://dnr.maryland.gov/fisheries/Documents/SummaryUpdateReport_Oysters.pdf. (accessed [September 13, 2024]).
MDDNR (Maryland Department of Natural Resources Fishing and Boating Services and the Oyster Advisory Commission in consultation with The University of Maryland Center for Environmental Science) (2021). Oyster Advisory Commission Consensus Recommendations on Oyster Management. A report to the Governor and the Maryland General Assembly, as required by Natural Resources Article §4–215 and §4-204. 478 pp. Available online at: https://dnr.maryland.gov/fisheries/Documents/Final_Report_OAC_SB0808_HB0911.pdf. (accessed [September 13, 2024]).
Miller T. J., Blair J. A., Ihde T. F., Jones R. M., Secor D. H., Wilberg M. J. (2010). FishSmart: An innovative role for science in stakeholder-centered approaches to fisheries management. Fisheries 35, 424–433. doi: 10.1577/1548-8446-35.9.422
Morson J., Gius J., Bushek D. (Eds.) (2023). 2023. Stock Assessment Workshop, New Jersey Delaware Bay Oyster Beds (25th SAW) (Port Norris, NJ: Haskin Shellfish Research Laboratory, Rutgers, The State University of New Jersey), 84. Available at: https://hsrl.rutgers.edu/wp-content/uploads/2023/12/SAW2023.pdf. pp.
Newell R. I., Fisher T. R., Holyoke R. R., Cornwell J. C. (2005). Influence of eastern oysters on nitrogen and phosphorus regeneration in Chesapeake Bay, USA. In: Dame R. F., Olenin S. (eds) The Comparative Roles of Suspension-Feeders in Ecosystems. NATO Science Series IV: Earth and Environmental Series, vol 47. Dordrecht: Springer, 93–120. doi: 10.1007/1-4020-3030-4_6
Newig J., Jager N. W., Kochskämper E., Challies E. (2019). Learning in participatory environmental governance–its antecedents and effects. Findings from a case survey meta-analysis. J. Environ. Policy Plann. 21, 213–227. doi: 10.1080/1523908X.2019.1623663
North E. (2020). OysterFutures larval transport model code, input files, output files, resulting connectivity matrices, and LTRANS User’s Guide. Biological and Chemical Oceanography Data Management Office (BCO-DMO). (Version 1) Version Date 2020-04-08 [if applicable, indicate subset used]. http://lod.bco-dmo.org/id/dataset/808650. [accessed September 15, 2024].
North E. W., Schlag Z., Hood R. R., Li M., Zhong L., Gross T., et al. (2008). Vertical swimming behavior influences the dispersal of simulated oyster larvae in a coupled particle-tracking and hydrodynamic model of Chesapeake Bay. Mar. Ecol. Prog. Ser. 359, 99–115. doi: 10.3354/meps07317
North E., Schlag Z., Hood R., Zhong L., Li M., Gross T. (2006). “Modeling dispersal of Crassostrea ariakensis oyster larvae in Chesapeake Bay,” in Final Report to Maryland Department of Natural Resources. Cambridge, MD: University of Maryland Center for Environmental Science, 55. Available at: https://northweb.hpl.umces.edu/publications/Reports/Northetal_DNR_final_report_31July06.pdf, pp.
OSW (OysterFutures Stakeholder Workgroup) (2018). “Recommendations for oyster management and restoration in the Choptank and little Choptank rivers,” in Report to Maryland Department of Natural Resources, Annapolis, MD: University of Maryland Center for Environmental Science. Available at: https://oysterfutures.wordpress.com/reports/.
Reagans R., McEvily B. (2003). Network structure and knowledge transfer: The effects of cohesion and range. Administrative Sci. Q. 48, 240–267. doi: 10.2307/3556658
Röckmann C., Ulrich C., Dreyer M., Bell E., Borodzicz E., Haapasaari P., et al. (2012). The added value of participatory modelling in fisheries management–what has been learnt? Mar. Policy 36, 1072–1085. doi: 10.1016/j.marpol.2012.02.027
Rodney W. S., Paynter K. T. (2006). Comparisons of macrofaunal assemblages on restored and non-restored oyster reefs in mesohaline regions of Chesapeake Bay in Maryland. J. Exp. Mar. Biol. Ecol. 335, 39–51. doi: 10.1016/j.jembe.2006.02.017
Rothschild B. J., Ault J. S., Goulletquer P., Heral M. (1994). Decline of the Chesapeake Bay oyster population: a century of habitat destruction and overfishing. Mar. Ecol. Prog. Ser. 111, 29–39. Available at: https://www.int-res.com/articles/meps/111/m111p029.pdf.
Runge M. C., Converse S. J., Lyons J. E. (Eds.) (2020). Structured Decision Making: Case Studies in Natural Resource Management (Baltimore, MD: Johns Hopkins University Press), 272. pp.
Sandström A., Carlsson L. (2008). The performance of policy networks: the relation between network structure and network performance. Policy Stud. J. 36, 497–524. doi: 10.1111/j.1541-0072.2008.00281.x
Schlag Z. R., North E. W. (2012). Lagrangian TRANSport model (LTRANS v.2) User’s Guide (Cambridge, MD: University of Maryland Center for Environmental Science, Horn Point Laboratory), 183. Available at: https://northweb.hpl.umces.edu/LTRANS/LTRANS-v2/LTRANSv2_UsersGuide_6Jan12.pdf. pp.
Scully M. E. (2013). Physical controls on hypoxia in Chesapeake Bay: A numerical modeling study. J. Geophysical Research: Oceans 118, 1239–1256. doi: 10.1006/jmsc.1999.0540
Scully M. E. (2016). The contribution of physical processes to inter-annual variations of hypoxia in Chesapeake Bay: A 30-yr modeling study. Limnology Oceanography 61, 2243–2260. doi: 10.1002/lno.10372
Scully M. E. (2018). A diel method of estimating gross primary production: 1. Validation with a realistic numerical model of Chesapeake Bay. J. Geophysical Research: Oceans 123, 8411–8429. doi: 10.1029/2018JC014178
Shchepetkin A. F., McWilliams J. C. (2005). The regional oceanic modeling system (ROMS): a split-explicit, free-surface, topography-following-coordinate oceanic model. Ocean Model. 9, 347–404. doi: 10.1016/j.ocemod.2004.08.002
Smith A., Sainsbury K., Stevens R. (1999). Implementing effective fisheries-management systems–management strategy evaluation and the Australian partnership approach. ICES J Mar Sci. 56, 967–979. doi: 10.1006/jmsc.1999.0540
Spires J. E. (2015). The exchange of eastern oyster (Crassostrea virginica) larvae between subpopulations in the Choptank and Little Choptank rivers: Model simulations, the influence of salinity, and implications for restoration. Masters Thesis (College Park, MD: University of Maryland, College Park), 76. Available at: http://hdl.handle.net/1903/17091. pp.
Susskind L. E., McKearnen S., Thomas-Lamar J. (1999). The consensus building handbook: A comprehensive guide to reaching agreement Vol. 1 (Thousand Oaks, California: Sage Publications), 176.
Tarnowski M. (2022). Maryland Oyster Population Status Report 2020 Fall Survey (No. DNR 17-062521-282) (Annapolis, MD: Maryland Department of Natural Resources). Available at: https://dnr.maryland.gov/fisheries/Documents/oysters/2020RptFinal.pdf.
Van Cauwenbergh N., Ciuró A. B., Ahlers R. (2018). Participatory processes and support tools for planning in complex dynamic environments. Ecol. Soc. 23, 2. doi: 10.5751/ES-09987-230202
Voinov A., Bousquet F. (2010). Modelling with stakeholders. Environ. Model. Software 25, 1268–1281. doi: 10.1016/j.envsoft.2010.03.007
Voinov A., Kolagani N., McCall M. K., Glynn P. D., Kragt M. E., Ostermann F. O., et al. (2016). Modelling with stakeholders–next generation. Environ. Model. Software 77, 196–220. doi: 10.1016/j.envsoft.2015.11.016
Wainger L. A., Gazenski K., Basenback N., Hollady T., Price E. W. (2018). “Appendix A. Estimates of potential willingness to pay for nitrogen credits in Maryland,” in Weber M. A., Wainger L. A., Parker M., Hollady T. eds. The Potential for Nutrient Credit Trading or Economic Incentives to Expand Maryland Oyster Aquaculture. Report to Maryland Sea Grant and CBL Technical Report TS-718-18. UMCES, Solomons, MD. Available at: https://repository.library.noaa.gov/view/noaa/37930.
White J. M., Buhle E. R., Ruesink J. L., Trimble A. C. (2009). Evaluation of Olympia oyster (Ostrea lurida carpenter 1864) status and restoration techniques in Puget sound, Washington, United States. J. Shellfish Res. 28, 107–112. doi: 10.2983/035.028.0101
Wilberg M. (2023). OysterFutures simulation model code, input files, and model description. Biol. Chem. Oceanography Data Manage. Office (BCO-DMO). doi: 10.26008/1912/bco-dmo.875301.1. (Version 1) Version Date 2022-06-07.
Wilberg M. J., Ihde T. F., Secor D. H., Miller T. J. (2009). “FishSmart: a stakeholder-centered approach to improve fisheries conservation and management,” International Council for Exploration of the Sea. (ICES) conference manuscript (ICES CM), Copenhagen, Denmark. Available at: https://www.ices.dk/sites/pub/CM%20Doccuments/CM-2009/O/O1509.pdf.
Wilberg M. J., Irwin B. J., Jones M. L. (2024). “Participatory modelling to support evaluation of management actions for recreational fisheries,” in Understanding Recreational Fishers: Disciplinary and Interdisciplinary Approaches for Fisheries Management. Eds. Pope K., Arlinghaus R., Hunt L., Lynch A., van Poorten B. (Springer).
Wilberg M. J., Livings M. E., Barkman J. S., Morris B. T., Robinson J. M. (2011). Overfishing, disease, habitat loss, and potential extirpation of oysters in upper Chesapeake Bay. Mar. Ecol. Prog. Ser. 436, 131–144. doi: 10.3354/meps09161
Wilberg M. J., Wiedenmann J. R., Robinson J. M. (2013). Sustainable exploitation and management of autogenic ecosystem engineers: application to oysters in Chesapeake Bay. Ecol. Appl. 23, 766–776. doi: 10.1890/12-0563.1
Keywords: collaborative governance, natural resource management (NRM), co-management, collaborative modeling, participatory modeling, oyster, Crassostrea virginica (Gemlin)
Citation: North EW, Wilberg MJ, Blair J, Wainger L, Cornwell JC, Jones R, Hayes C, Gawde R, Hood RR, Goelz T, Hartley T, Mace MM III, Diriker M, Fowler N and Polkinghorn B (2024) Two applications of the Consensus Solutions process with collaborative modeling for management of a contentious oyster fishery. Front. Mar. Sci. 11:1423534. doi: 10.3389/fmars.2024.1423534
Received: 26 April 2024; Accepted: 28 August 2024;
Published: 24 October 2024.
Edited by:
David Bushek, Rutgers, The State University of New Jersey, United StatesReviewed by:
Carolina Bourque, Louisiana Department of Wildlife and Fisheries, United StatesJohn Klinck, Old Dominion University, United States
Patrick Sullivan, Cornell University, United States
Copyright © 2024 North, Wilberg, Blair, Wainger, Cornwell, Jones, Hayes, Gawde, Hood, Goelz, Hartley, Mace, Diriker, Fowler and Polkinghorn. This is an open-access article distributed under the terms of the Creative Commons Attribution License (CC BY). The use, distribution or reproduction in other forums is permitted, provided the original author(s) and the copyright owner(s) are credited and that the original publication in this journal is cited, in accordance with accepted academic practice. No use, distribution or reproduction is permitted which does not comply with these terms.
*Correspondence: Elizabeth W. North, enorth@umces.edu
†Present address: Taylor Goelz, Energy and Environment Program, The Aspen Institute, Washington, DC, United States