- 1Institute of Marine Sciences - Okeanos, University of the Azores, Horta, Portugal
- 2Marine Environment and Technology Laboratory, University of Azores, Praia da Vitória, Portugal
- 3Instituto do Mar - IMAR, University of the Azores, Horta, Portugal
The study of larval dispersal and connectivity between deep-sea populations is essential for the effective conservation and management of deep-sea environments and the design and implementation of Marine Protected Areas. Dense sponge aggregations, known as “sponge grounds”, are a key component of marine benthic ecosystems, by increasing the structural complexity of the sea floor and providing structure and habitat for many other species. These aggregations are characteristic of the Azores deep-sea environment. These sessile organisms rely primarily on larval dispersal for their reproduction. Connectivity between specific Pheronema carpenteri sponge aggregations in the Azores was studied using a 3-D biophysical dispersal model. Different biological trait scenarios were analyzed, considering spawning seasonality and pelagic larval duration. Model results indicate that regional circulation patterns drive larval dispersion, shaping population connectivity of P. carpenteri sponge aggregations in the Azores, particularly among aggregations in the Central Group of Azorean islands. Some areas present high retention rates, receiving larvae from several sponge aggregations while also being important larval source aggregations. In contrast, aggregations from the Eastern Group may be isolated from the others. Larval dispersal and connectivity patterns were analyzed concerning the current configuration of Marine Protected Areas (MPAs) in the Azores. The results underscored the importance of maintaining protection efforts in existing MPAs and identified stepping-stone locations and specific sites where additional measures could enhance species connectivity in the Azores.
1 Introduction
Connectivity is a fundamental process driving the persistence of marine populations and influencing the ecosystems’ structure, biodiversity, productivity, dynamics and resilience (Kenchington et al., 2019; Busch et al., 2021). In the deep-sea (the ocean below 200 meters depth), connectivity is a key element for spatial management and conservation plans including the establishment of Marine Protected Areas (MPAs) (Lima et al., 2020; Combes et al., 2021).
Many marine organisms rely on the larval phase as the primary means to colonize new areas, making this process crucial for individual survival as well as in population dynamics and persistence (Ross et al., 2020). Some species possess a pelagic larval phase, during which their larvae are transported by ocean currents, facilitating migration between geographically distant populations (Paris et al., 2013). However, despite the pivotal role of the planktonic larval stage and larval dispersal in understanding deep-sea population connectivity, knowledge gaps remain due to challenges in obtaining in-situ data (Kenchington et al., 2019). This pelagic larval dispersal phase contributes to (i) population exchanges that aid in the replenishment of populations, population connectivity and the maintenance of well-established communities, and to (ii) the colonization of new territories/habitats, possibly modifying existing communities and associated biodiversity (Adams et al., 2012).
Dense sponge aggregations, known as “sponge grounds”, are a key component of marine benthic ecosystems, enhancing, along with corals, the local biodiversity, and acting as feeding, reproductive, nursery and refuge areas for many invertebrates and fish (Pham et al., 2015; Beazley et al., 2021). Deep-sea sponges are thought to play an important role in the deep-sea nutrient cycle, recycling the nutrients, through their capacity of filtering large quantities of water (Leis, 2020). By converting dissolved organic matter (DOM) into detritus, sponges are enable to transfer the energy and nutrients in DOM to higher trophic levels, the so-called sponge loop pathway (Rix et al., 2018). They also contribute to the biogeochemical cycling of dissolved nutrients, such as carbon and nitrogen, and silicate in the case of siliceous sponges like P. carpenteri, which belongs to the class of Hexactinella (Maldonado et al., 2016; Ross et al., 2016, 2019; Taranto, 2022).
These deep-sea organisms rely upon a planktonic larval stage for dispersal; thus, studying the environmental patterns responsible for their distribution is critical to inform and support the development of appropriate conservation measures (Maldonado and Young, 1999; Cowen and Sponaugle, 2009; Xavier et al., 2015; Wang et al., 2021).
Deep-sea sponges inhabit multiple areas of the deep-sea, particularly in the North Atlantic (Samuelsen et al., 2022). The hexactinellid Pheronema carpenteri forms extensive sponge aggregations, occurring from south of Iceland and west of Scotland, across the Porcupine Seabight, Azores, northern Spain, Portugal, Canary Islands, and off Morocco at 800–1,350 m depth (Maldonado et al., 2016). Some of them are reported to have persistent spicule skeletons (Maldonado et al., 2005). These spicule mats created by the senescence and death of hexactinellid sponges, provide an important substratum that hosts diverse faunal communities (Bett and Rice, 1992) forming biodiversity hotspots (Henry and Roberts, 2014), and may even function as reducers of sediment erosion in the deep-sea (Black et al., 2003). Sponges are also known for establishing complex microbial symbioses being a reservoir of exceptional microbial diversity and major contributors to the total microbial diversity of the world’s oceans (Thomas et al., 2016).
P. carpenteri forms the most extensive Hexactinellid aggregations in the North-East Atlantic (Graves et al., 2023), and is a typical organisms of the deep-sea in the Azores (Pham et al., 2015). P. carpenteri, is a nest-shaped sponge that likely forms the most extensive sponge aggregations at temperate latitudes, with abundances up to 6 individuals m-2 (Maldonado et al., 2016). P. carpenteri has been recorded in the northeast Atlantic from the northern Rockall Trough at about 59°37’N north, through the Porcupine Seabight, Bay of Biscay, Portuguese coast and Moroccan coast to the Azores, at depths ranging from 650m to 1600 m (Rice et al., 1990; White, 2003; Howell et al., 2016; Creemers et al., 2019; Colaço et al., 2020; Vieira et al., 2020; Somoza et al., 2021; Taranto, 2022). In the Azores its density can vary between 2 to rarely 56 individuals per squares meter (Colaço pers. observ.).
In the Porcupine Sea Bight, this species occurs in areas with low currents but close to regions where enhanced bottom tidal currents are found (White, 2003). This corroborates the hypothesis of Rice et al. (1990), that these sponges do not tolerate enhanced currents, but may nevertheless be dependent upon the resuspended or undeposited organic matter carried to them from regions of increased tidal energy. In the Azores the same phenomena is observed, with the sponge grounds being present in areas with low intensity currents (Viegas, 2022).
The Azores deep-sea benthos includes a high diversity of sponges and cold-water corals which build rich communities (Pham et al., 2015; Gomes-Pereira et al., 2017; Creemers et al., 2019; Colaço et al., 2020; Morato et al., 2021). P. carpenteri is a common taxon in the Azores bathyal environment, forming large aggregations (Colaço et al., 2020) and is considered a vulnerable marine ecosystem (VME) since it meet several of the vulnerability criteria, such as fragility, slow growth rate and low recovery potential (FAO, 2008).
Available data about the biology of hexactinellids sponges results mainly from studies with shallow-water sponges (Barthel et al., 1996; Boury-Esnault et al., 1999), also arctic deep-sea populations (Leys and Lauzon, 1998), and other non-specific glass sponge’s case studies (Bett and Rice, 1992; Boury-Esnault et al., 1999; Guillas et al., 2019). The reproductive strategy of P. carpenteri is currently unknown (Graves et al., 2023) but Hexactinellids are assumed to reproduce both asexually and sexually with lecithotrophic larvae (Leys and Ereskovsky, 2006; Teixidó et al., 2006). During their life cycle, after hatching, pelagic larvae drifts in the water column for a limited period (Pelagic Larval Duration, PLD), before settling on the seafloor, in a recruitment area, to become sessile juvenile sponges, if the environment conditions are suitable (Maldonado, 2006). Despite their deep-water habitat, hexactinellids sponges may experience seasonality that perhaps influences their reproductive period (Leys and Lauzon, 1998). For the deep-sea species Geodia the reproductive season spans from spring to Autumn (Koutsouveli et al., 2020).
The current understanding of deep-sea larval behavior for deep-sea sponges is extremely limited (Busch et al., 2021), mainly due to the inherent difficulty of assessing deep-water habitats (Lopes, 2005), and particularly by the difficulty of collecting larvae of deep-sea organisms. Available data from the shallow-water sponges indicate that most sponge larvae are anchiplanic, with a short planktonic larval duration of minutes to a few days (usually< 2 weeks) (Maldonado, 2006; Ross et al., 2019). However, this short PLD is only reported for shallow-water species and is believed to not be representative of deep-sea water taxa such as P. carpenteri. The glass sponge V. pourtalesii has an estimated maximum pelagic larval duration of 2 weeks (Wang et al., 2021). Hilário et al. (2015) calculated that the average known PLD of a deep-sea organism is 35 days, which is longer than for their shallow-water counterparts PLD. Wang et al. (2021) used modelling tools to study the fate of glass sponge larvae in the Gulf Stream, hypothesizing a 2-week maximum PLD for deep-sea glass sponge.
Furthermore, it is suggested that sponge larvae may remain in the water column for longer periods, perhaps months (Boury-Esnault et al., 1999; Maldonado, 2006). Environmental factors like seawater temperature can influence the duration of the reproductive period (Maldonado, 2006). Therefore, two different PLDs were tested in this study, a PLD of 15 days (similar as was estimated V. pourtalesii), and a PLD of 30 days (similar as was estimated in other deep-sea larvae dispersal studies (Hilário et al., 2015; Vic et al., 2018) (Table 1).
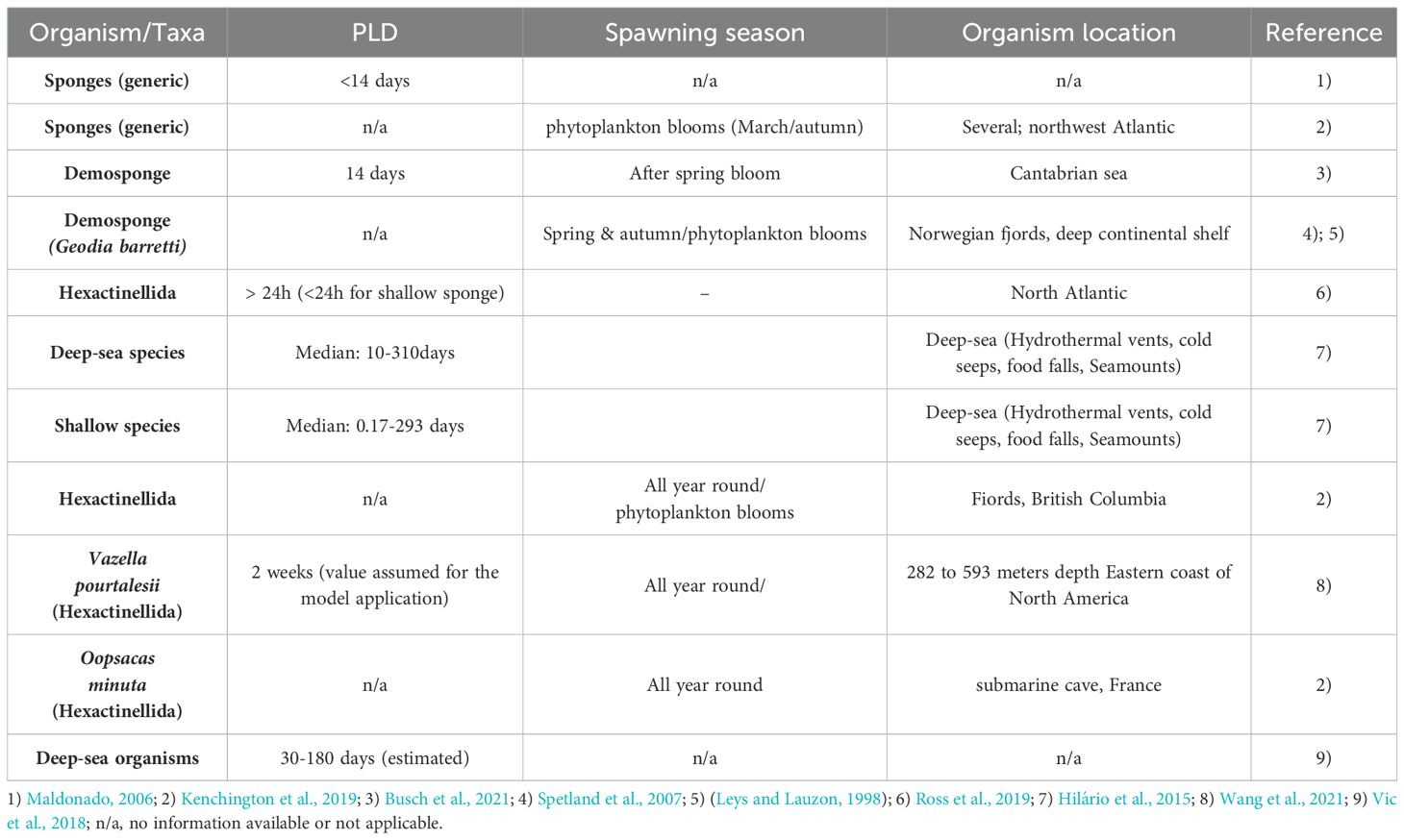
Table 1. Literature review for Pelagic larval duration, spawning seasonality, of deep-sea sponges, from different locations and depths, used/obtained in other studies.
In this work, the potential larval dispersal of P. carpenteri deep-sea sponge aggregations in the Azores, it was modeled, as a proxy for the potential larval transport around Azores and evaluate the probability of the current MPAs to maintaining the connectivity of these benthic organism populations.
2 Methodology
2.1 Study area
The Azores archipelago is composed of nine islands, spanning 600 km in the Northeast Atlantic, between 23°W and 33°W and 37°N and 40°N (Figure 1) and divided into three geographical groups. The Mid Atlantic Ridge (MAR) crosses the archipelago. Two islands are located west of the MAR, on the American plate, forming the Western Group (WG), while the other islands are located on the Eurasian plate with 5 islands positioned in the Central Group (CG) of the Archipelago and two islands situated further east (Eastern Group: EG).
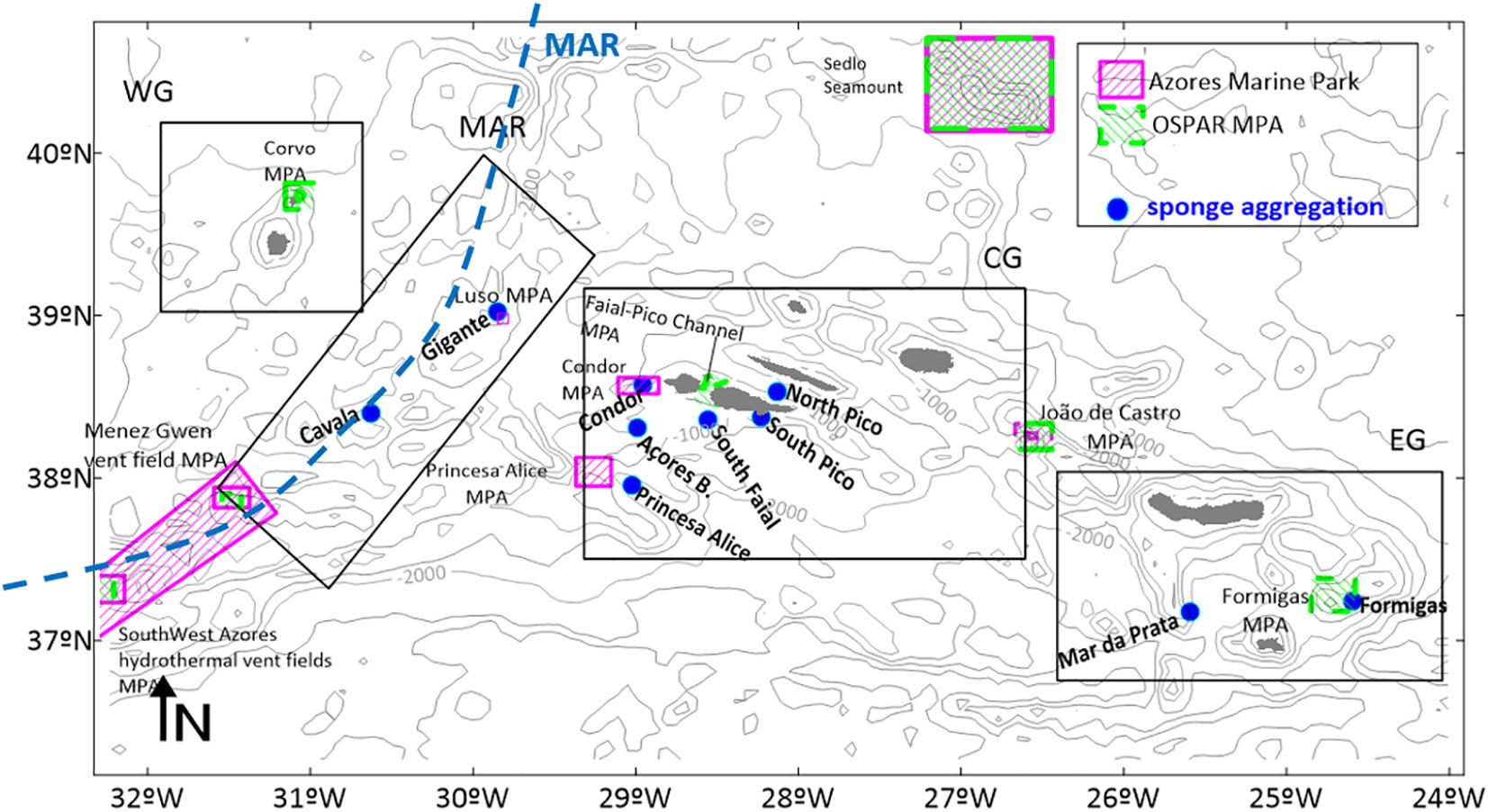
Figure 1. Bathymetry of the study area, with the representation of the Mid-Atlantic Ridge (MAR) with a blue dashed line, the 9 islands of the Azores archipelago in grey, the location of the Pheronema carpenteri sponge aggregations in the study with blue circles; black polygons depicts the groups in analysis: MAR Group (L#1 Cavala, L#2-Gigante); CG-Central Group (L#3-Princesa Alice, L#4-Açores Bank, L#5-Condor Seamount, L#6-South of Faial, L#7-South of Pico, L#8); EG- Eastern Group (L#9-Mar da Prata, and L#10-Formigas), and representation of the Azores Marine Park and OSPAR Marine Protected Areas, and the Western Group (Flores and Corvo islands), Central Group (Faial, Pico, São Jorge Terceira and Graciosa Islands), and the Eastern Group (São Miguel and Santa Maria islands).
2.1.1 Pheronema carpenteri in the Azores
The P. carpenteri sponge aggregations identified in the scope of the SPONGES Project, due to their frequent observations are the focus of this study. These P. carpenteri aggregations are located along the Azores Archipelago (Figure 1), in a bathymetric range from 630 to 1000 meters depth (Table 2). Specifically, they are found over the Mid-Atlantic Ridge (MAR) at Cavala Seamount (L#1) and Gigante Seamount (L#2); in the Central Group (CG), at the Princesa Alice (L#3), Açores Bank (L#4), in the South of Faial (L#6), South of Pico (L#7), and North of Pico (L#8); and in the Eastern Group (EG) L#9 in Mar da Prata, and L#10 in Formigas.
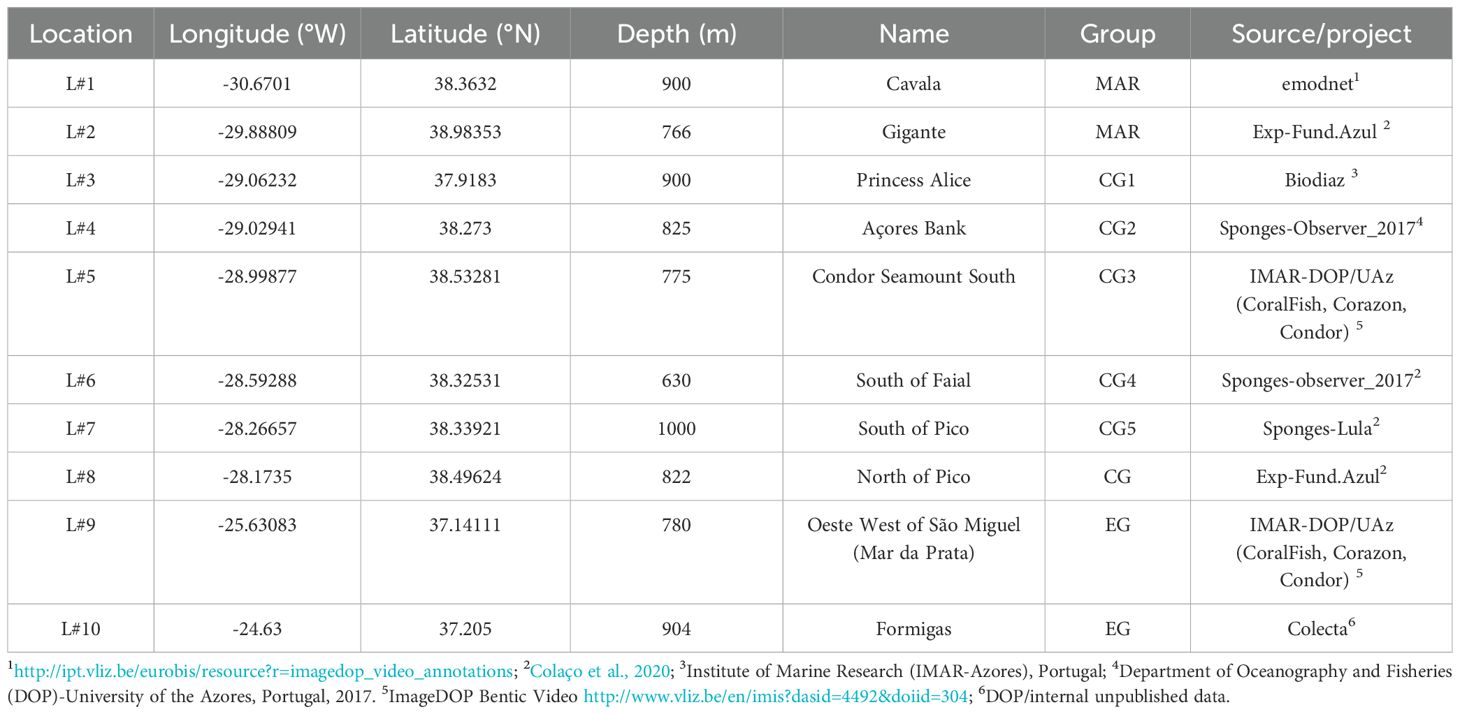
Table 2. Pheronema carpenteri sponge aggregations in the study, their location, depth, source and name/location.
For applying particle-tracking models to study larvae dispersal, it is essential to include information about their biological traits such as spawning seasonality, larval behavior, and pelagic larval duration (Busch et al., 2021). Empirical data about deep-sea sponges biology is very scarce, and specifically regarding P. carpenteri it is absent. Previous studies often assume that deep-sea sponges do not experience spawning seasonality, unlike their littoral relatives, because they are too deep to be influenced by light (Barthel et al., 1996). However, further studies pointed out that despite their deep-sea habitat, sponges experience seasonality, which influences their growth rate and perhaps reproductive period, which, in turn, may be regulated by patterns of primary production in the photic zone of the ocean and subsequent sinking of the generated production (Leys and Lauzon, 1998). A number of deep-sea species display potential spawning that is linked to organic matter input during seasonal phytoplankton blooms (Mercier et al., 2011; Sun et al., 2011; Mercier et al., 2013; Baillon et al., 2014). In particular, for deep-sea corals, some gametogenic peaks coincided with periods marked by high surface productivity, in spring and autumn (Santos et al., 2013).
The information available regarding deep-sea larval behavior is scarce. Table 1 summarizes the literature review regarding the deep-sea larvae biological traits and specifically for deep-sea sponges larvae. Different scenarios were computed to study the larval dispersal in the Azores. It was considered a yearly release since no information on spawning was available, but since some deep-sea species are believed to experience spawning seasonality, seasonal scenarios were also performed. For the seasonal scenario, a release in March was simulated to represent the spring spawning season, and a release in October to represent the autumn spawning season (Santos et al., 2013). All the scenarios ran with different pelagic larval durations (PLD) of 15 and 30 days (Table 3).
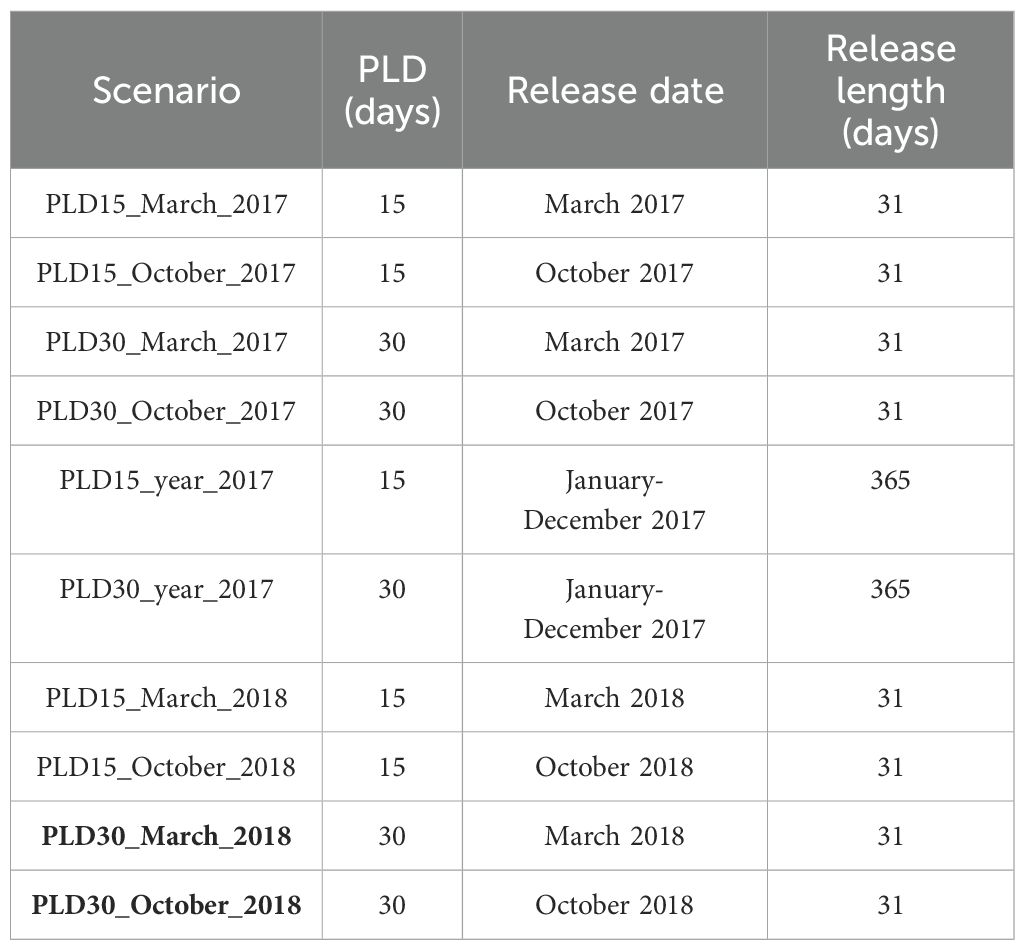
Table 3. Scenarios considered in this study, with spawning date and pelagic larval duration and the release length (in days).
2.1.2 3D Hydrodynamic model using Mohid
The 3-D hydrodynamic modelling system used was MOHID, developed at MARETEC (IST - University of Lisbon) which solves the 3D incompressible primitive equations built and developed using an object-oriented philosophy (IST, 2003; Braunschweig et al., 2004; Leitão et al., 2008). MOHID is an open-source model, available online at https://github.com/Mohid-Water-Modelling-System/Mohid. It is programmed in ANSI FORTRAN 95, following an object-oriented approach allowing the integration of different modules in implicit and explicit ways (IST, 2003). The hydrodynamic model simulates the currents and density fields, fundamental for the Lagrangian and Eulerian transports through advection and/or diffusion processes. The turbulence is solved by the General Ocean Turbulence Model (GOTM) module (Burchard et al., 1999). Model configuration and boundary conditions are described in Table 4.
The model was implemented for the Azores region from 32.4721°N to 42.91211°N, -21.40775°W to -36.2878°W, for the years 2017 and 2018. It has a horizontal resolution of 1/16° horizontal resolution (6 km grid) and a vertical resolution of 50 vertical layers covering the entire water column from the surface to the sea floor (0 – 5940 meters). The first 10 meters of the water column are divided into 7 sigma layers, which change their size based on the tide level evolution. Below these, the water column is divided into 43 Cartesian layers, which are thinner in the upper layers (less than 2 meters in length) and larger in the bottom ones.
For the atmospheric forcing, the boundary conditions are obtained from the Global Forecast System (GFS model), provided by NOAA- America National Ocean and Atmospheric Administration, available at https://www.ncdc.noaa.gov/. This model has hourly fields of surface wind, temperature, relative humidity, pressure, and solar radiation.
Model validation of the selected parameters is detailed in Viegas (2022). Comparison between model results and tide gauge data reveals the capability of the model to accurately simulate sea water level, in the Azores region. Sea surface temperature was validated against remote sensing data and predicted sea water temperature and salinity were compared with in situ data from ARGOS floats. Model validation against the ARGO float data for vertical profiles of temperature and salinity showed Pearson correlation coefficients greater than 0.97, with the majority being 0.99 (Viegas, 2022). The outputs of the hydrodynamic model (horizontal and vertical components of the velocity, sea level, temperature, salinity, and density fields) were used to feed the CMS particle tracking model to simulate the plume-dispersal processes in offline mode.
2.1.3 Particle tracking model
The CMS-Connectivity Modelling System is an open-source model, freely available online. It was created for the multi-scale tracking of biotic and abiotic particles in the ocean, based on a Lagrangian framework to study complex larval migrations (Paris et al., 2013). This model runs offline, over a 3D hydrodynamic model, applying its velocity fields (u, v, w) to each particle, using a 4th-order Runge–Kutta numerical discretization method (García-Martínez and Flores-Tovar, 1999) to differentiate particle positions through space and time. Modules distributed with the code include mortality, vertical migration, and a connectivity module designed to generate a connectivity matrix output from the source to the final destination of the particles. The model gives a probabilistic estimate of dispersion and oceanographic connectivity, transport and fate of Lagrangian phenomena. The model computes the probability of larval exchange (here in called connectivity), between source and recruitment areas, by dividing the number of larvae that reached each site by the total number of larvae release; mortality, and behavior; providing results over time including particles’ location (x, y and depth), water properties, particle status (moving, dead, out of the domain or settled) and also the particles settlement location. For the purpose of the model, there is an assumption that the larvae that reach the recruitment site will settle. However, the successful development of the settled larvae into a new organism, depending on behavioral components and the suitable conditions (e.g., hydrodynamic, environmental conditions, biological and physical processes, competency or predation) (Pineda et al., 2010), is not estimated by the model.
The model can simulate particles settlement in defined recruitment locations (in this case the sponge aggregations in the study). These recruitment locations are defined by a polygon (longitude, latitude and depth). Particle settlement starts after the defined PLD.
In this study, each aggregation is defined by a polygon of 6x6 km (the model resolution). The initial positions for the drift trajectories of each population were the centroids of each one of these areas. Each population is defined by a release location, and Lagrangian tracers represent larvae. A position (longitude, latitude and depth), the number of particles released, the release frequency, and the date characterize each release location. Since there is no information about the fecundity, frequency and seasonality of larval emissions, a hypothetical number was defined (Cowen and Sponaugle, 2009). To standardize this approach, the same number of particles for all the locations was considered, assigning the same hypothetical relevance to all the release points, with the same release size and frequency. The model simulates a release of a total of 150,000 particles per month, in a time step of 1.5 hours, during the entire release period, for all the scenarios. In this study, all the aggregations are simulated with the same number of particles, not reflecting population abundances, size or reproduction rate. Larvae were simulated as passive particles, without any active swimming behavior, being just advected by the hydrodynamics. Model configurations are described in Table 5.
The number of particles was calibrated to provide accurate larvae dispersion results, considering the computational efficiency. This calibration was performed using two different methodologies (Viegas, 2022), the Particle Density Distribution, and the evaluation of the fraction of unexplained variation (Simons et al., 2013), and by the analysis of the dispersal pathlength distances saturation (Kough et al., 2013).
The model simulates the larvae dispersal, and larvae settlement after the precompetence period (the PLD). For connectivity studies the model allows defining suitable settlement locations, defined by a polygon (longitude, latitude and depth). In this case, to study connectivity between the sponge aggregations, each sponge aggregation is also a source location and a recruitment area, defined by a polygon of 6x6km (model resolution), and depth (700 to 1000 meters depth). The larvae exchange between different locations will represent connectivity between different sponge aggregations.
Alternatively, to study potential settlement location throughout the Azores region, another methodology was applied, assuming that larvae were able to settle at any location between 700 and 1000 meters depth.
Due to the many uncertainties about deep-sea larval biology, different scenarios were considered, with different pelagic larval durations (PLD), and seasonal scenarios to encompass different potential situations. This is one advantage of using modelling tools, allowing the study of different hypotheses and scenarios (Swearer et al., 2019; Wang and Qiao, 2020).
2.1.4 Data analysis
Larvae were represented by particles, and the model results were analyzed through dispersal maps, larvae positions and larvae exchange between different source and recruitment locations. Connectivity between the sponge aggregations in the study was represented by connectivity matrices, representing the percentage of particles that reach each site, which allows for the analysis of sink and source aggregations. In this study, each particle represents one larva. When referring to settling particles, it means a particle has reached another location, representing a larva that has settled in a new location and potentially will develop successfully. Connectivity matrices also represent the percentage-recruitment levels. Connectivity between two locations was assumed when, at least, one modelled particle released from one location (source node) reach (also referred as settled in) another location (receiving node), after the PLD. When settling in the same location, it is considered self-recruitment. Source locations are represented as rows (j), and recruitment areas (receiving node) as columns (i), with self-recruitment in each location represented in the matrix diagonal. Colors represent the percentage of particles that reach each locations, calculated by dividing the total number of settled larvae from each source population that settled, at the end of the PLD, in each receiving population, by the total number of larvae released from the source population. Besides the connectivity analysis, the larvae settlement positions throughout the study area were analyzed, to identify other potential larvae settlement positions.
The particle density distribution (PDD) is represented in 2-D vertically integrated maps, where all particles are represented, and each domain grid cell (6km x 6km) correspond to the integrated number of tracers in the entire water column. In contrast, in the particle dispersal maps, particles are not integrated into the water column, and all the particles in each cell are graphed. Some particles can “mask” others, by overlapping. Travel distances were analyzed by the Shapiro-Wilk Normality Test (using Rstudio 2022.02.3), to test if they are normally distributed; and by using histograms to analyze potential travel distances from all the different release points for the different scenarios. These distances were calculated from individual particle trajectories as the sum of straight-line distances between each time step. Additionally, histograms of particle depths were used to analyze how particles are distributed along the water column.
3 Results
3.1 Passive particle drift trajectories
Larvae were simulated as passive particles, being advected by the hydrodynamic currents. The particle density distribution (PDD) illustrates the larval dispersal along the study area, (Figure 2), indicating the potential particle exchange between different locations. The results reveal a heterogeneous distribution depending on the spawning seasonality. Larvae from the MAR group can reach the Central Group (CG) only during the winter and autumn months (January, February, September, October, November and December). Larval dispersal plumes from the MAR can also reach the Western Group (WG), surrounding Flores and Corvo islands, during March, April, May and June months. Larvae exchange between CG and WG only occurs during July, November and December. However, the low PDD between these groups (Figure 2) indicates a low probability of particle exchange.
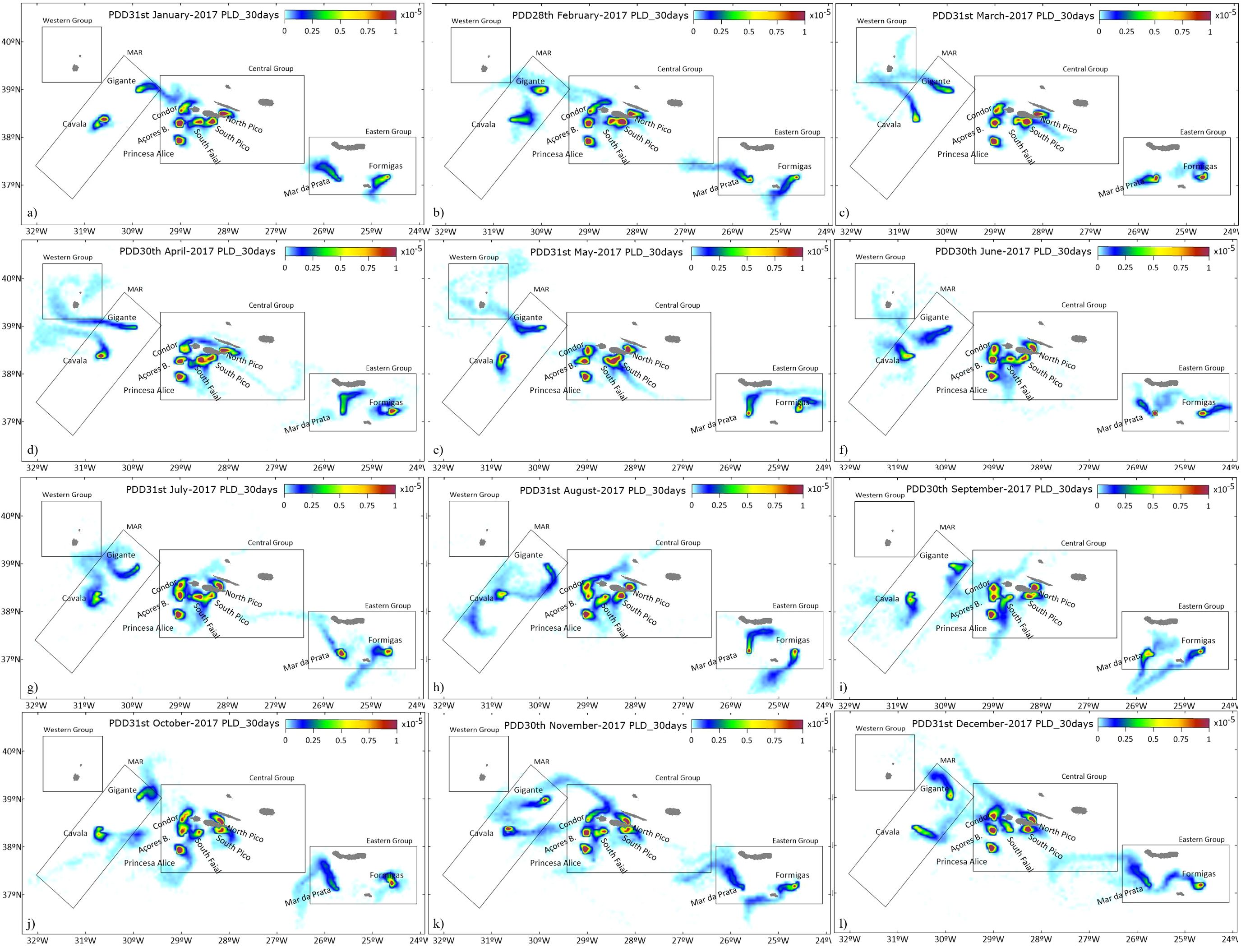
Figure 2. Particle density distribution for the annual release with a PLD of 30 days, for 31st January (A): 28th February (B); 31st March (C); 30th April (D); 31st May (E); 30th June (F); 31st July (G); 31st August (H); 30th September (I); 31st October (J); 30th November (K); 31st December (L). Black polygons represent the different location groups: MAR Group (L#1-Cavala, L#2-Gigante); Central Group (L#3-Princesa Alice, L#4-Açores Bank, L#5-Condor Seamount, L#6-South of Faial, L#7- South of Pico, L#8); Eastern Group (L#9)-Mar da Prata, and L#10-Formigas).
In March an eddy is formed between the Western Group and the MAR (Figure 3A), contributing to particle retention in this area. Larvae from the CG mostly remain around the islands. Nevertheless, the low current velocity, (Figure 3), is not sufficient to transport larvae from CG to the EG populations, given the PLD used in these scenarios.
Larvae from the Central Group populations, reach various sponge aggregations. Between the Central and Eastern Group, the hydrodynamic patterns go mainly in the eastward direction during March, Figure 3A, while in October there is a dominant current coming from the east and going westward (Figure 3B). In the northwest part of the study area, currents are stronger during October (Figure 3B) than during March (Figure 3A), and the major hydrodynamic fields present another pattern, where the higher velocity (>0.1m/s) matches the absence or reduced particle density, and the higher particle density occurs in locations with lower currents magnitude.
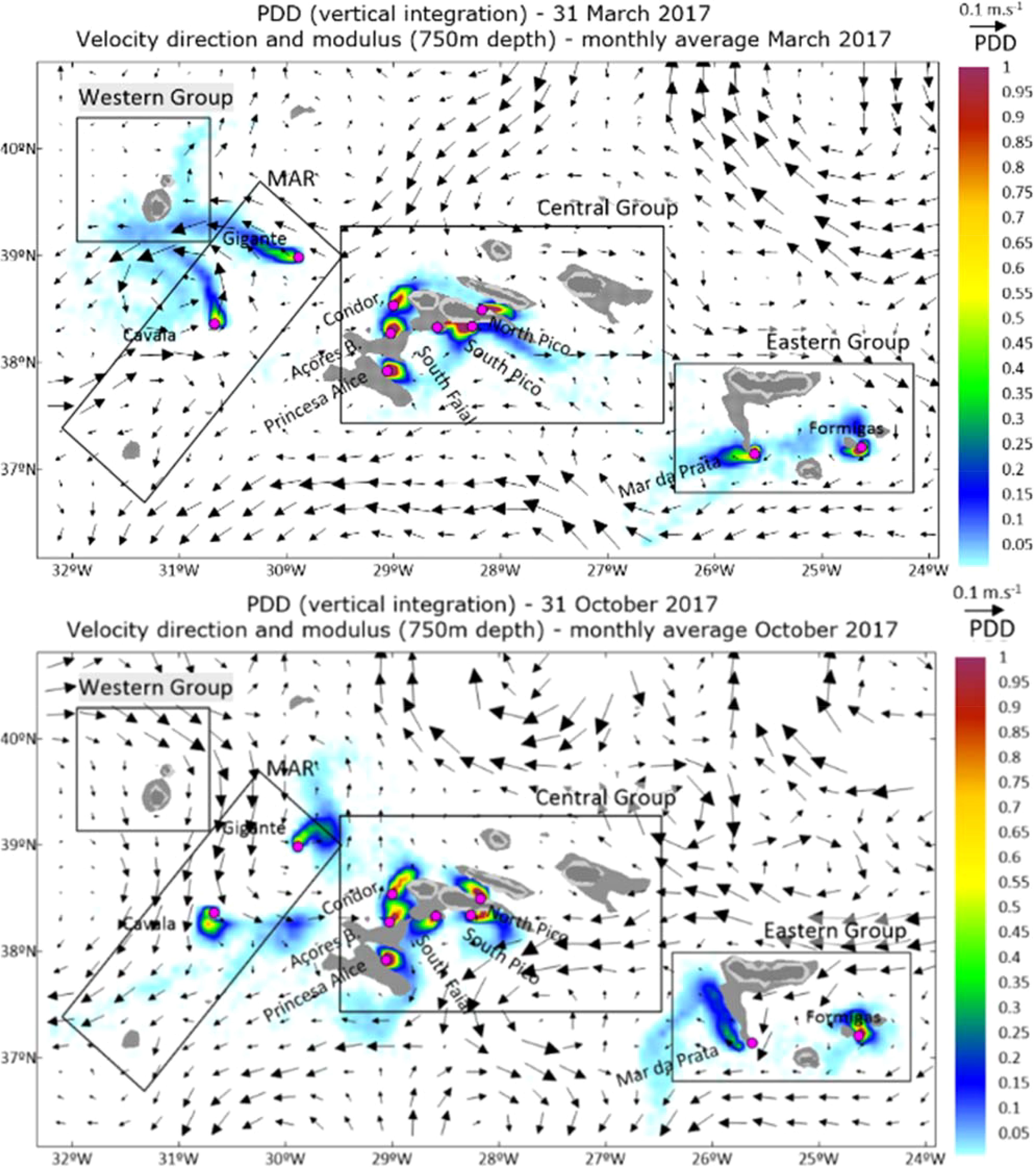
Figure 3. Particles density distribution integrated into the entire water column, like presented in Figure 2, with the representation of the velocity vectors at 750 meters depth, for 31 March 2017 (top), and 31 October 2017 (bottom). The PDD is represented with a color scale from blue to red, where 0 is white), vectors represent the monthly average of velocity modulus and direction at 750 meters depth.
3.2 Particles travel distance
Larvae can travel from a few kilometers to a maximum travelling distance of over 400km (Table 6). Travel distances are highly non-normal, confirmed by the Shapiro-Wilk Normality Test (using Rstudio 2022.02.3). Therefore, rather than considering the average values, the median and the 95th percentile are used for this analysis (Phelps, 2015). Maximum total travel distances are an order of magnitude larger than median distances. Across all March and October scenarios, the longer PLD causes higher travel distances. Median total travel distances for a PLD of 30 days are double the distances with a PLD of 15 days (Table 6). Larval travel distances vary both seasonally and spatially. During March, larvae from the MAR locations: L#1, and L#2, have higher travel distances than those from other locations (with a 95th percentile of 207 and 220 km respectively).
In the Central Group (CG, locations L#3 to L#8), the overall median particle distance in all tested scenarios is lower than in other groups, ranging from 8 to 20 km, which may contribute to higher self-recruitment levels. This lower travel distance is due to the lower current velocities in the region (Figure 3), in contrast to the MAR populations, which are situated in areas with stronger currents.
3.3 Particles depth
Simulated larvae mainly remain close to the bottom at the released bathymetry (Figures 4, 5), despite some travelling through different bathymetric ranges. Histograms of particles’ depth along their trajectory are represented in Figure 4 for the March release and in Figure 5 for the October release. In all the locations, larvae remain mostly within the bathymetric range of the release (>40%). A longer PLD contributes to a wider distribution of particles along the water column. The results show no significant difference in particle depth between the March and October releases. This demersal larval dispersal can also lead to higher levels of particle retention at their source locations.
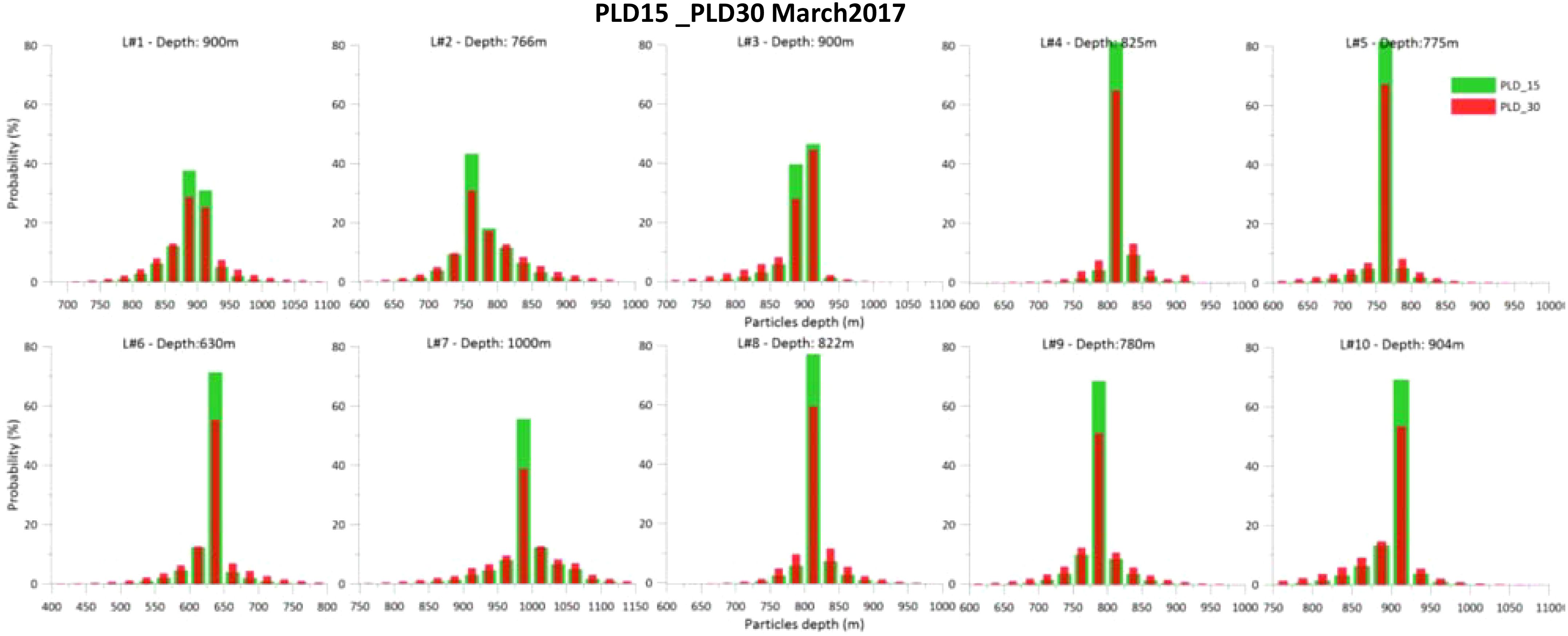
Figure 4. Histogram of particles’ depth along their trajectory, for each location, for PLD15 and PLD30 days for March 2017 release. The depth of the respective release is detailed at the top of each graph.
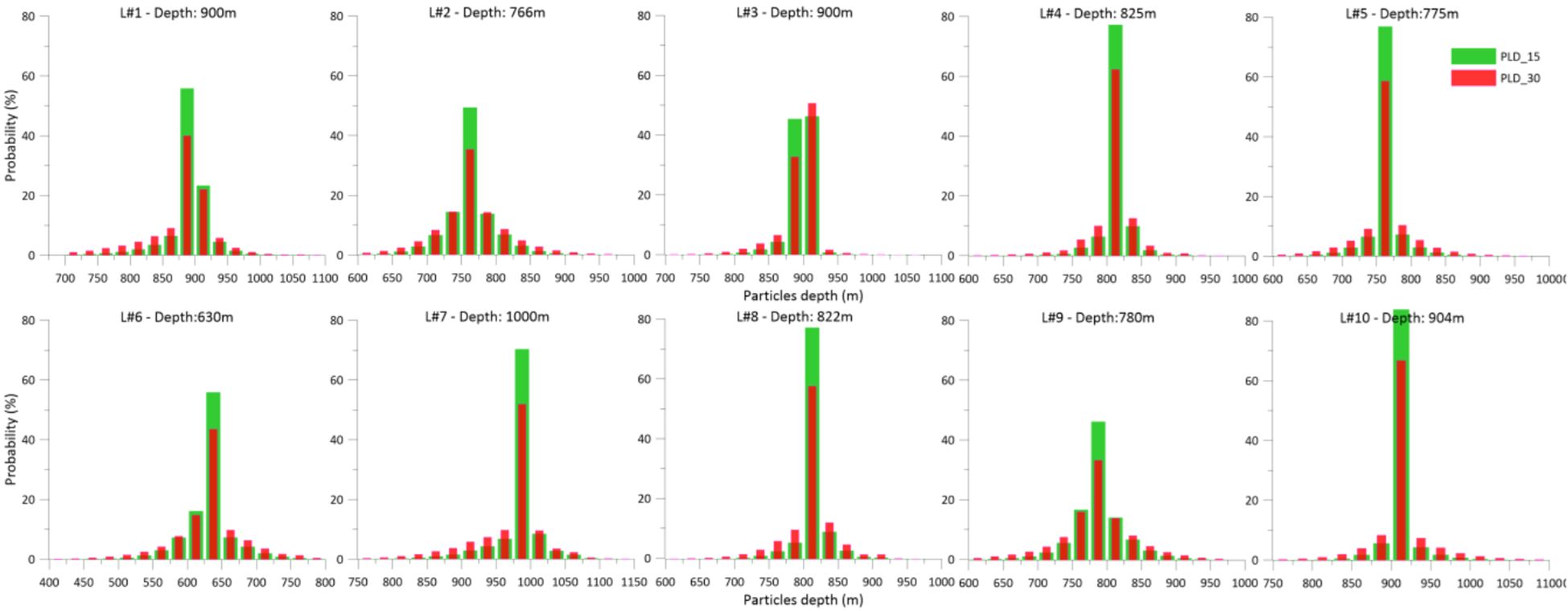
Figure 5. Histogram of the particles’ depth along their trajectory, for each location, for PLD15 days and a PLD30 for the October 2017 release. The depth of each respective release is detailed at the top of each graph.
3.4 Connectivity between populations
Results show variation in the level of population connectivity depending on the PLD of 15 or 30 days. Connectivity between the different populations in the study is illustrated by connectivity matrices, and also by connectivity arrows represented on the maps (Figure 6). The matrices depict the percentage of larvae exchanged between different populations, indicating connectivity, or in the case of self-recruitment, the percentage of larvae that settled in the source population after the PLD. A PLD of 30 days enables more connectivity between the different sponge aggregations than a PLD of 15 days (Figure 6). Conversely, a shorter PLD, results in higher self-recruitment levels. There is no connectivity between the EG and the CG or WG, in both PLD scenarios. In the WG, connectivity between Formigas (L#10) and Mar da Prata Seamount (L#9) is unidirectional, with a PLD of 15 days, and bidirectional with a PLD of 30 days. Between the CG and the EG, the larvae exchange is minimal, with no significant larvae exchange among the different populations, as is represented in the connectivity matrices.
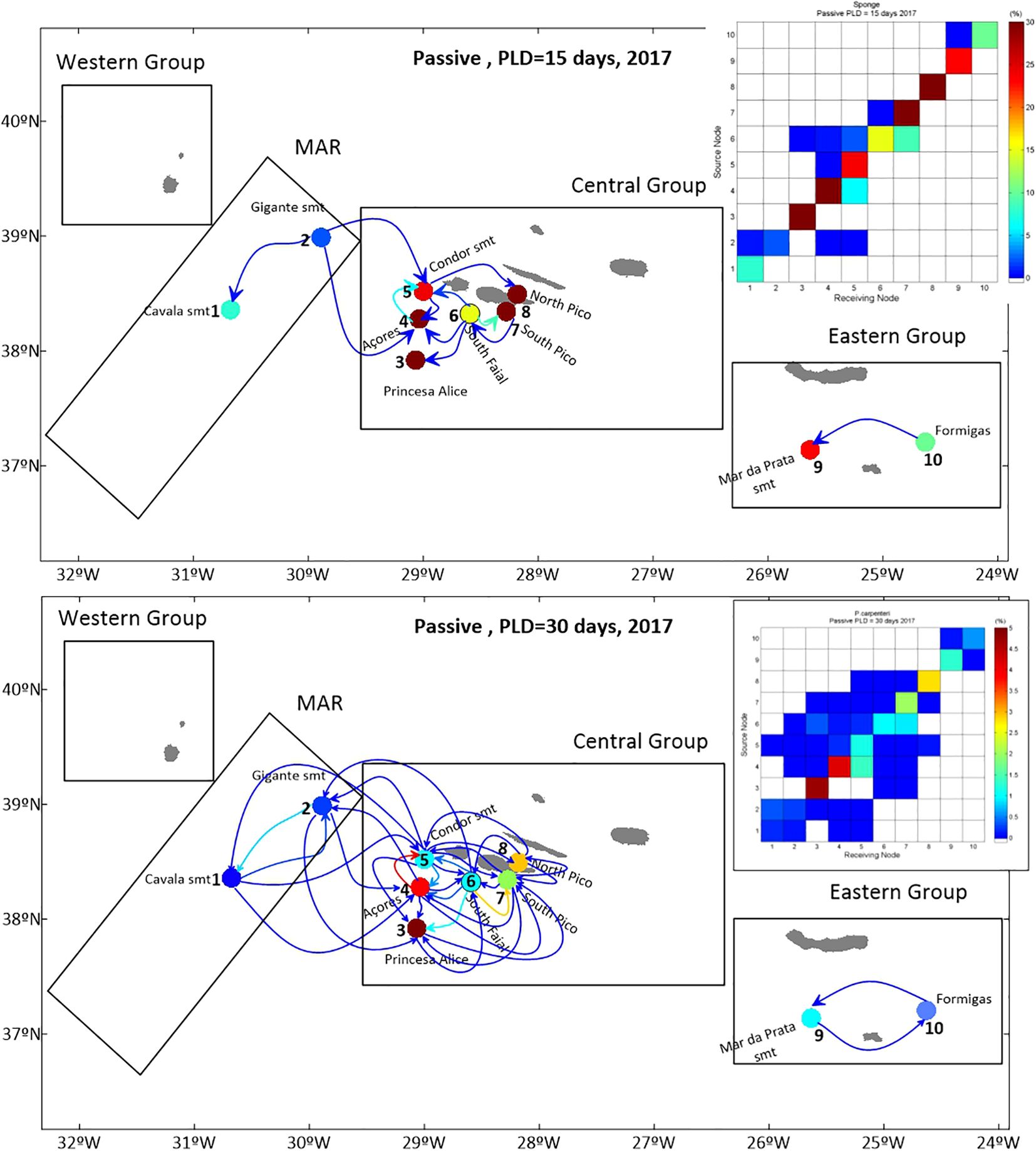
Figure 6. Map of connectivity relations, and respective connectivity matrix, for a yearly release (2017) with a PLD of 15 days (top), and PLD of 30 days (bottom), connectivity arrows and auto-recruitment circle colours represent the percentage of settled particles.
This yearly release allows for the analysis of different spawning and hydrodynamic scenarios. The hypothesis of a seasonal spawning release was studied, considering releases of one month in March and one month in October to cover spring and fall scenarios, respectively. The results indicate different connectivity patterns in terms of seasonality. The October release (Figure 7), generates lower larvae exchange between the different sponge aggregations. Population connectivity between MAR and CG only occurs in the March_PLD_30_2018 scenario, namely between the Azores Bank (L#4) and the Cavala (L#1) and Gigante (L#2) Seamounts. In the other scenarios, there is no connectivity between the different groups. In the CG, the connectivity between different sponge aggregations reveals seasonality, with a higher number of connectivity relations in the March scenarios. The percentage of self-recruitment (Supplementary Tables A3–A6 in the Supplementary Material) is different from March to October, with the releases from CG revealing the highest self-recruitment percentage in October. In different situations, there is neither self-recruitment, nor connectivity between Gigante Seamount (L#2) and any other populations. The same occurs in the Mar da Prata Seamount (L#9), which shows no self-recruitment in October_PLD30_2017.
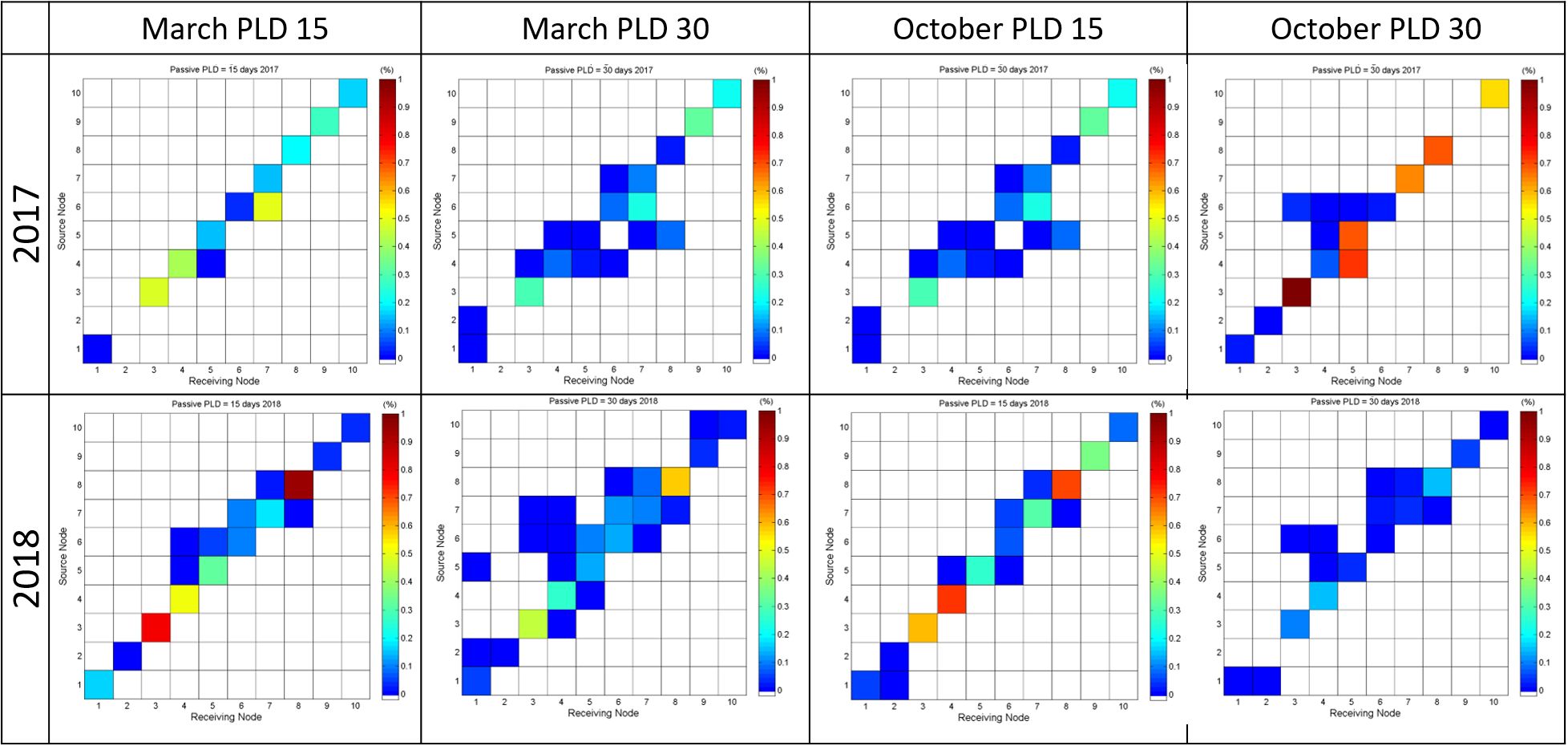
Figure 7. Connectivity matrices for the March_PLD15, March_PLD30, October_PLD15 and October_PLD30 for 2017 on top and 2018 on the bottom, connectivity and auto-recruitment colours represent the percentage of settled particles.
3.5 Larvae settlement locations
Besides the sponge aggregations in the study, larvae can also settle in other regions of the domain. This study was performed within the defined bathymetric range of 600 to 1000 meters depth. Seasonal dynamics can induce different settlement positions. The yearly release considers all the monthly scenarios, and consequently more settled larvae along the Azores region (Figure 8). Considering the bathymetric range from 600m to 1000m, larvae can settle around all the islands except Terceira island. Larvae settle along the MAR and on seamounts situated on its western and eastern flanks but also at different locations of the CG region’. The Central Group is an important recruitment area. This result highlights the potential connectivity between the populations in the Central Group. Major results show that during the yearly release, larvae from Cavala and Gigante seamounts, settle along the MAR, around Flores and Corvo islands, and along the west slope of the Central Group, but they hardly reach the East part of the Central Group. Gigante Seamount can receive larvae from different locations (Cavala (L#1), Gigante(L#2), Açores Bank (L#4), Condor (L#5), and South of Faial (L#6)), contrasting with the seasonal releases where larvae exchange occurs only during the October scenario, between Gigante (L#2) and Cavala (L#1) seamounts. The larvae from South of Faial (L#6) are the ones with a wider range of settling locations; they can settle along MAR, in the WG, in the CG, in João de Castro bank, and in the EG, in the North of São Miguel (Figure 9F).
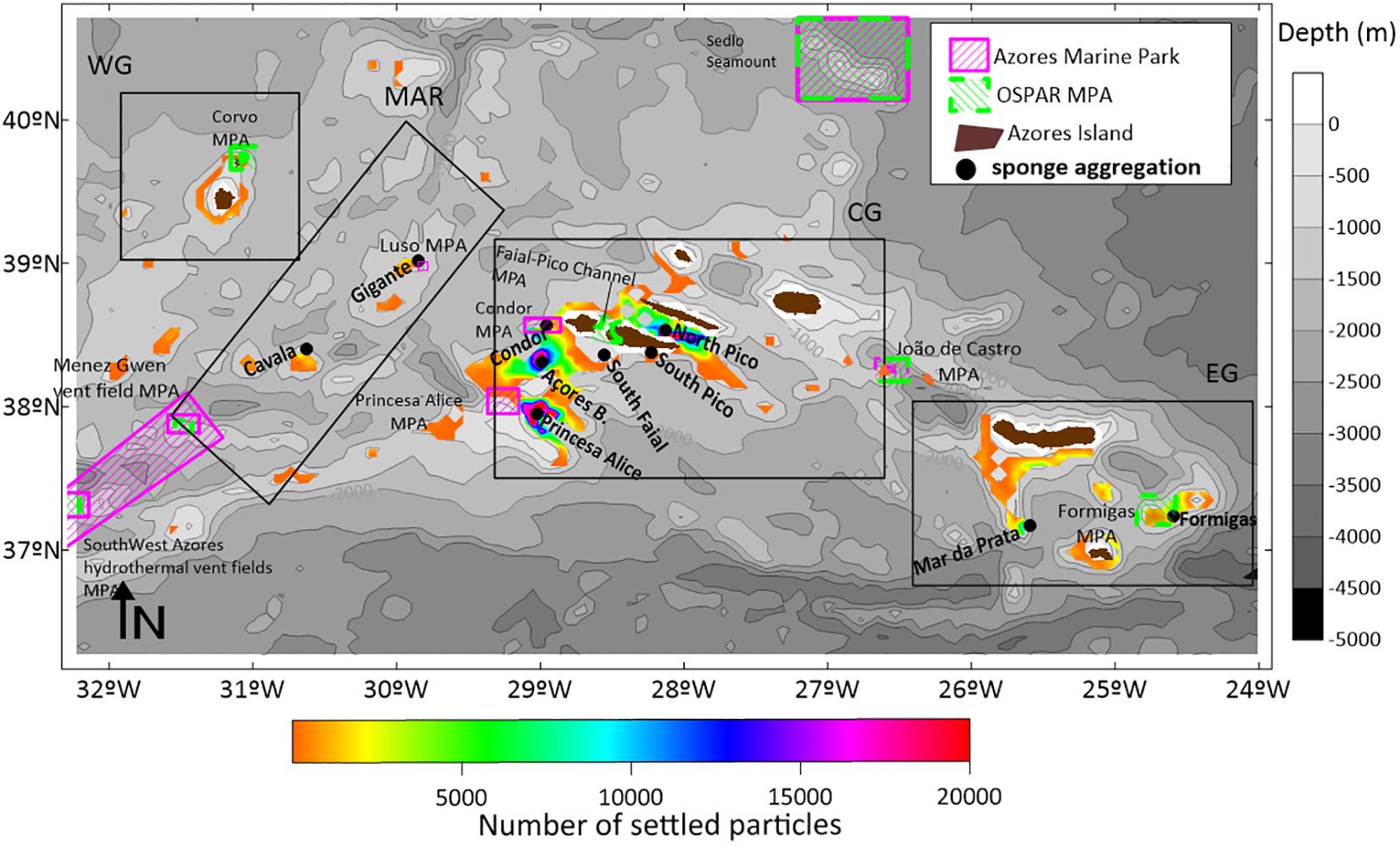
Figure 8. Location of settled particles along the domain, in the bathymetric range from 600 to 1000m depth, for the yearly release. Different colours represent number of settled larvae. Bathymetry is represented with a grey colour scale. The Azores islands are represented in dark brown, the Azores Marine Park in pink, and the OSPAR MPAs in green.
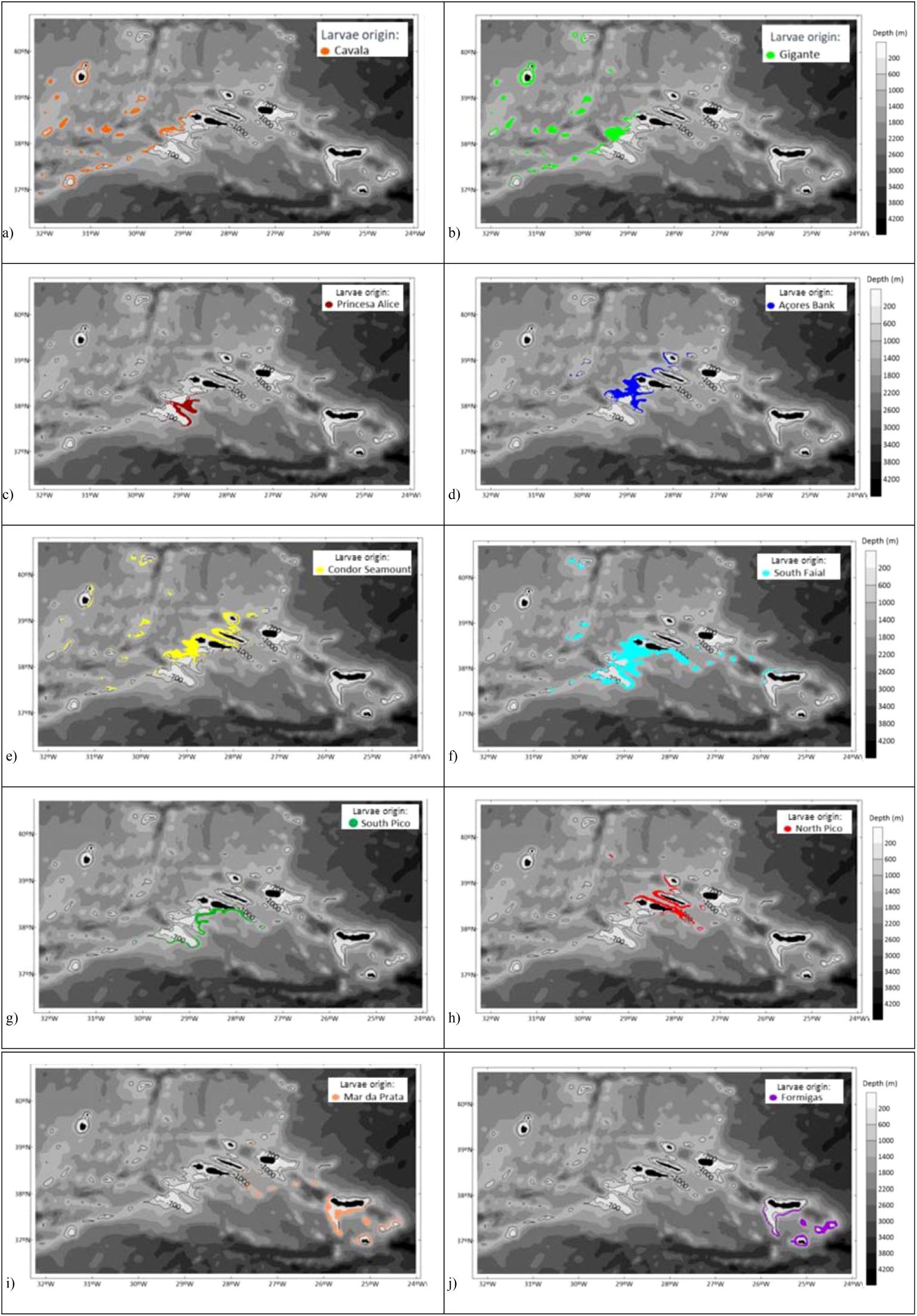
Figure 9. Location of settled particles, in the entire domain, in the bathymetric range from 600 to 1000m depth, for the yearly release of 2017 with a PLD of 30 days, for each origin. Different colours represent different releases: (A) Cavala; (B) Gigante; (C) Princesa Alice; (D) Açores Bank; (E) Condor Seamount; (F) South Faial; (G) South Pico; (H) North Pico, (I) Mar da Prata, (J) Formigas. Bathymetry is represented in a grey colour scale.
4 Discussion
This study aims to use specific sponge aggregations to study the dispersal of their larvae, and the potential connectivity among these different sponge grounds, considering spring and autumn spawning scenarios, and a yearly release, as a first approach to studying the dispersal of deep-sea larvae in the Azores. Given the many knowledge gaps in the reproductive and larval biology of deep-sea sponges, the use of models offers an advantage by allowing the study of different scenarios. P. carpenteri larvae were modelled as passive tracers, similar to other deep-sea sponge larval dispersal modelling studies (Kenchington et al., 2019; Swearer et al., 2019). The few studies of connectivity of sponge grounds in the deep sea in the Northeast Atlantic do not cover the Azores area, but they showed genetically a strong panmixia, and that the observed patterns were very well correlated with the physical drivers and the prevailing currents and topography (Taboada et al., 2023). In the Cantabrian sea, the same pattern emerged from the deep sea fan shaped sponges, where genetically a panmixia was present. However, when applying a virtual particle tracking model to assess oceanographic connectivity it showed a strong retention of larvae in the study area and a variable inter-annual connectivity highly correlated with the current regime (Busch et al., 2021).
Model results show that the hydrodynamic patterns have a strong influence on larval dispersal, causing different distribution patterns depending on the season. Larvae can be advected from a few kilometers to hundreds of kilometers, depending on the PLD and the hydrodynamic currents. The water-mass circulation in the Azores region is highly dynamic, and the influence of the different currents has seasonal effects on larval dispersal. The strong presence of hydrodynamic eddies (Caldeira and Reis, 2017) can contribute to particle retention in different areas, as is the case in March when an eddy forms between the Western Group and the MAR. These results show the importance of studying different spawning season scenarios for larval dispersal. Consequently, due to knowledge gaps in sponge reproductive traits, different seasonal scenarios and PLD were tested. Multiple connectivity relationships exist between the sponge aggregations in the CG, showing a redundancy in connectivity networks is a positive feature as it helps to mitigate recruitment failures by providing alternative connectivity pathways. These multiple connectivity relationships may result from the proximity between these sponge aggregations, but also from the local hydrodynamic patterns characterized by lower velocities. This is in contrast to the strong hydrodynamic patterns that occur between the WG and the MAR in March, and between CG and EG in October.
A significant limitation of this and other deep-sea dispersal models is the lack of information on deep-sea species biological traits (Arellano et al., 2014; Hilário et al., 2015; Graves et al., 2023).
4.1 Connectivity between sponge aggregations and self-recruitment
There are no connectivity studies of benthic organisms in the Azores region. A retention particle study between 0 and 150 meters showed the potential of the different island groups of the Azores to retain particles, and their dependence on the current regime and topography (Sala et al., 2013). Close to the Azores a study of the physical connectivity between the NE Atlantic Seamounts showed self-recruitment abilities and great retention of particles on the seamounts (Lima et al., 2020).
This study shows larval dispersal between different sponge aggregations in the Azores, (Figure 9A–J) namely between the different sponge aggregations located in the same group (MAR, CG and EG), as well as between aggregations located in the MAR and the CG. However, there is no particle exchange between the CG and the EG. Moreover, these Eastern Group sponge aggregations do not exchange larvae with the other populations, and are the most isolated. Therefore, these populations may be spatially fragmented, similar to what was identified in other larval dispersal model studies for deep-sea populations (Hilário et al., 2015).
The results show that the local ocean currents shape the larval dispersal and connectivity, creating sub-regions not connected, given the studied scenarios. These kind of hydrodynamic barriers were also identified in other modelling approaches for deep-sea larval sponge dispersal on the east coast of North America (Wang et al., 2021). Moreover, this modelling approach also shows that the local eddies can supply and induce local retention of larvae, promoting connectivity and self-recruitment (Wang et al., 2021), as occurs in this case study in the Azores, namely between the WG and the MAR populations. A retention particle study in the Azores at 150 meters also showed the Eastern group isolation and the large capacity of the central area in the Azores to retain particles (Sala et al., 2016), which is coherent with the observed pattern in this study.
4.2 Larval dispersal patterns and connectivity for P. carpenteri in the Azores Marine Park
Connectivity among different benthic populations promotes the increase of their genetic diversity (Busch et al., 2021; Wang et al., 2021) and the resilience of the species (Bracco et al., 2019). It is an essential aspect in the development of management and conservation plans for marine ecosystems (Combes et al., 2021). Detailed knowledge of the hydrodynamic patterns and biological interactions that drive the transport of planktonic phases is crucial for improving the effectiveness of the MPAs (Stratoudakis et al., 2019). The model results of larval dispersal and larvae settlement positions for this target species in the Azores were analyzed considering the MPAs from the Azores Marine Park. When MPAs are isolated from each other, they are more vulnerable to local extinction as they cannot be replenished by organisms or larvae from other locations (Stratoudakis et al., 2019). This is especially true for sessile organisms such as deep-sea sponges, which rely on larval dispersal to colonize new habitats (Metaxas and Saunders, 2009), and to support remote populations (Gary et al., 2020). Networks of marine protected areas arranged considering the larval dispersal patterns are considered ideal for the protection of marine species (Steneck et al., 2009). Therefore, studying larval dispersal patterns and identifying the main larval source and sink locations can contribute to the better implementation and management of conservation plans (Combes et al., 2021).
The study of larval dispersal and connectivity of these P. carpenteri aggregations serves as a pilot study for the connectivity of deep-sea benthic organisms in the Azores. The current regime and topography have been shown in other studies to be the main drivers of physical connectivity, explaining the genetic connectivity patterns (Busch et al., 2021; Taboada et al., 2023). Using P. Carpenteri as a proxy, we can discuss how effective the network of MPA from the Azores Marine Park is from the physical connectivity point of view. Among the 10 deep-sea sponge aggregations in the study, two are located in MPAs: Condor and Formigas populations. The Princesa Alice aggregation is located on the southern slope of the Princesa Alice Seamount, outside but adjacent to the MPA delimitation. Larval dispersal and connectivity results, indicate that Condor is an important source population, exchanging larvae with 8 of the 10 aggregations in the study (Figure 6). In addition, its larvae can achieve different locations in the Central Group, in the Western Group (around the islands of Flores and Corvo), and along the MAR: in the north, at 40.3°N (seamount south of the Kurchatov Fracture Zone); at 39°N in the Gigante Seamount; and in the Voador Seamount at 37.5°N (Figure 9E). The MPAs of Princesa Alice and Condor may act as sink locations, once that larvae from different aggregations in the study achieve these locations. These results demonstrate the potential connectivity that sponge populations from Condor may have with different populations in the Azores region.
The Princesa Alice sponge aggregation in the study, adjacent to the limits of the MPA, demonstrates the importance of extending the limits of this MPA as it has the potential to retain larvae from multiple locations in the study: Cavala (Figure 9A), Gigante (Figure 9B) and Condor (Figure 9E) seamounts, and South of Faial (Figure 9F), aggregations.
The main results show a lack of larvae exchange between the EG and the other aggregations in the study (Figures 9I, J), mainly due to its distance from the remaining sponge grounds, but also as a consequence of the hydrodynamic patterns between the CG and the EG, impeding the larvae advection in the westward direction. No larvae exchange was identified between EG and the remaining sponge grounds. Therefore, the protection of an area in Mar da Prata will favor the maintenance of the sponge aggregations on the plateau south of São Miguel island, and both Mar da Prata and Formigas populations, which can be isolated from the remaining archipelago, due to their distance, but also restricted by the hydrodynamic patterns. The vulnerability of Formigas sponge grounds, which face several scenarios with no larvae exchange and the absence of self-recruitment, also reinforces the need to maintain the MPA of Formigas.
The João de Castro Bank MPA can receive larvae from the CG and from the Mar da Prata (in the EG) being an steppingstone location between the Central and the Eastern parts of the archipelago. Therefore, this site is very important for the longer-term protection of these aggregations, by promoting the establishment of a linkage between the CG and the EG. The Gigante Seamount, in the MAR, is a recruitment area for deep-sea sponges, retaining larvae from different sources from the CG (Condor, Açores Bank and South of Faial), as well as from Cavala and Gigante Seamount. The results of larval dispersal also show that larvae from the Gigante seamount can achieve the MAR region, up to seamounts located South of the Kurchatov Fracture Zone (north of the study area), and in seamounts west of the MAR like the Buchanon Seamount. On the other hand, larvae from aggregations of Voador Seamount will favor resilience along the MAR, and South of the Azores EEZ.
This study does not take into account the density of these sponge aggregations. Research by Graves et al. (2023) on the drivers of P. carpenteri density suggests that the available environmental data, combined with the scarcity of long-term time series for deep-sea environmental data, do not capture the key factors influencing this species’ density. This limitation restricts the ability to model and identify areas where aggregations are likely to occur, particularly those with dense aggregations that could be considered VMEs. Increasing to a multi-year analysis would provide the opportunity to examine variability over the years and test other hypotheses, such as the average temperature variability, which has been identified as an important driver of P. carpenteri distribution (Graves et al., 2023).
The application of biological larvae traits and their parameterization in biophysical models presents a challenge (Hilário et al., 2015) even in well studied shallow-water systems (Metaxas and Saunders, 2009). When regarding these biophysical models for deep-sea dispersal, the application of biological traits is even more challenging due to the scarcity of data, and difficulty data collection, observation and model validation (Adams et al., 2012; Ross et al., 2016). So, these mode results can be used as a first approach to study the potential larval dispersal in the Azores, but always considering the associated uncertainties. However, models are always a simplification of the reality and despite all the uncertainty regarding the biological parameters (Simons et al., 2013), the validation of the physical circulation model used in this case study (Viegas, 2022) provides us with the guarantee of the correct physical model component, providing a baseline to study different scenarios, and also to be used to study climate change scenarios hypothesis.
Furthermore, this modelling approach could be used in combination with the species distribution models (Beazley et al., 2021) to construct explicit spatial hypotheses for deep-sea populations connectivity following a seascape framework approach (Kenchington et al., 2006; Kavanaugh et al., 2014; Zeng et al., 2020; Swanborn et al., 2022), in this case for the Azores region. In the future, the possibility of collecting physical samples and performing a population genetic study, will contribute to a deeper knowledge of these populations connectivity (Kool et al., 2011; Selkoe and Toonen, 2011; Antonio Baeza et al., 2019; Wang et al., 2021), and will help to validate our hypothesis.
5 Conclusions and final remarks
The physical connectivity among different deep-sea P. carpenteri sponge aggregations in the Azores region was studied using a biophysical particle-tracking model. P. carpenteri larvae were modeled as passive tracers (Kenchington et al., 2019; Swearer et al., 2019) under pelagic larval duration (PLD) scenarios of 15 and 30 days and seasonal spawning, based on other deep-sea sponge studies (Kenchington et al., 2019; Ross et al., 2020; Wang et al., 2020, 2021). Model results indicate that PLD and spawning seasonality are crucial for larval dispersal and population connectivity in the Azores. Seasonal effects are mainly driven by the region’s dynamic oceanographic conditions. Local hydrodynamic vortices can lead to larval retention, while stronger currents (exceeding 0.1 m/s) between the Western and Central Groups in spring, and between the Central and Western Groups in autumn, hinder larval exchange. Therefore, more information on the species’ biological traits, including spawning seasonality, is crucial for understanding effective connectivity across the archipelago.
A PLD of 30 days would enhance population persistence and recovery after disturbances (Cowen and Sponaugle, 2009), whereas a shorter PLD of 15 days could increase population fragmentation. Connectivity and larval dispersal results were analyzed in relation to the current design of the Azores MPAs, demonstrating their effectiveness in promoting connectivity across the archipelago by protecting key sink and source locations. Stepping-stone sites, such as the João de Castro Bank, were identified as critical links between isolated populations of the Eastern Group and the central archipelago. Other locations along the Mid-Atlantic Ridge (MAR), including Gigante, Cavala, Ferradura, and Voador seamounts, also facilitate connectivity, serving as potential sink locations. These locations should be the target of greater protection measures in the design and implementation of protection plans.
This study also identifies potential areas for future exploration, such as João de Castro, Voador, Ferradura, and Buchanan banks, where larvae are predicted to settle. These locations are promising for studying not only P. carpenteri but also other deep-sea species cohabitating in these habitats.
This type of investigation highlights the utility of larval dispersal models in designing Marine Protected Areas (MPAs) (Lima et al., 2020), specifically their role in identifying source and sink locations. It allows the identification of major larval dispersal and connectivity patterns among sponge aggregations in the Azores, providing a framework that can be extended to other deep-sea sponge and coral habitats.
Future studies should include more detailed biological and larval behavior data for deep-sea sponges. Integrating genetic connectivity studies will offer valuable insights into the behavioral dynamics and resilience mechanisms of these organisms within the Azores. Additionally, considering aggregation density and population size in models will provide a more precise quantification of recruitment efficiency. Novel insights into the biology, size, and density of sponge aggregations will be invaluable for understanding the dynamics of this species within the Azorean ecosystem.
Regardless of the lack of information about P. carpenteri biological traits, this dispersal modelling approach can provide an overall understanding of deep-sea larval transport in the Azores region, considering the studied PLD scenarios. Moreover, this modelling methodology can therefore be replicated for other species considering these or any other biological traits scenarios, or to study other hypothesis. These results can therefore be used to long-term conservation plans for deep-sea species, and support protection actions for local populations in the Azores.
Data availability statement
The raw data supporting the conclusions of this article will be made available by the authors, without undue reservation.
Author contributions
CV: Conceptualization, Data curation, Formal analysis, Investigation, Methodology, Validation, Writing – original draft, Writing – review & editing. MJ: Data curation, Methodology, Software, Supervision, Writing – original draft, Writing – review & editing. AC: Conceptualization, Funding acquisition, Investigation, Project administration, Resources, Writing – original draft, Writing – review & editing.
Funding
The authors declare financial support was received for the research, authorship, and/or publication of this article. This research is part of the DEEP REST project that was funded through the 2020- 2021 Biodiversa and Water JPI joint call for research projects, under the BiodivRestore ERA-NET Cofund (GA N°101003777), with the EU and the following funding organizations: Agence Nationale de la Recherche (ANR-21-BIRE-0003), France, Ministry of Agriculture, Nature and Food Quality (LNV), Netherlands, Research Foundation -Flanders (FWO), Belgium, German Federal Ministry of Research (BMBF) through VDI/VDE-IT, Germany, Environmental Protection Agency (EPA), Ireland, Fundacão para a Ciência e a Tecnologia (FCT), Portugal, Fundo Regional para a Ciência e Tecnologia (FRCT), Portugal-Azores and State Research Agency (AEI), Spain. CV work was supported by FCT (PhD scholarship) and DEEPREST (M2.2/DEEPREST/004/2022). AC work was supported by FCT/MCTES through national funds in the scope of the CEEC contract CEECIND/00101/2021 (https://doi.org/10.54499/2021.00101.CEECIND/CP1669/CT0001). This work received national funds through the FCT – Foundation for Science and Technology, I.P., under the project UIDB/05634/2020 and UIDP/05634/2020 and through the Regional Government of the Azores through the initiative to support the Research Centers of the University of the Azores and through the project M1.1.A/REEQ.CIENTÍFICO UI&D/2021/010.
Acknowledgments
The authors would like to acknowledge the contributions of many scientists and young researchers that were actively involved in the SPONGES project in the Azores.
Conflict of interest
The authors declare that the research was conducted in the absence of any commercial or financial relationships that could be construed as a potential conflict of interest.
Publisher’s note
All claims expressed in this article are solely those of the authors and do not necessarily represent those of their affiliated organizations, or those of the publisher, the editors and the reviewers. Any product that may be evaluated in this article, or claim that may be made by its manufacturer, is not guaranteed or endorsed by the publisher.
Supplementary material
The Supplementary Material for this article can be found online at: https://www.frontiersin.org/articles/10.3389/fmars.2024.1393385/full#supplementary-material
Supplementary Table 1 | Particle travel distances for the different scenarios. Maximum distance (max); median distance (med) and percentile 95th.
Supplementary Table 2 | Connectivity tables detailing the percentage of particles released particles from each location (rows) that settled in each recruitment area (columns). For the scenario: Annual PLD15.
Supplementary Table 3 | Connectivity tables detailing the percentage of particles released particles from each location (rows) that settled in each recruitment area (columns). For the scenario: Annual PLD30.
Supplementary Table 4 | Connectivity tables detailing the percentage of particles released particles from each location (rows) that settled in each recruitment area (columns). For the scenario: March PLD15 2017.
Supplementary Table 5 | Connectivity tables detailing the percentage of particles released particles from each location (rows) that settled in each recruitment area (columns). For the scenario: March PLD30 2017.
Supplementary Table 6 | Connectivity tables detailing the percentage of particles released in each location (rows) that settled in each recruitment area (columns). For the scenario October PLD15 2017.
References
Adams D. K., Arellano S. M., Govenar B. (2012). Larval dispersal, vent life in the water column. Oceanography 25, 255–268. doi: 10.5670/oceanog.2012.24
Antonio Baeza J., Holstein D., Umaña-Castro R., Mejía-Ortíz L. M. (2019). Population genetics and biophysical modeling inform metapopulation connectivity of the Caribbean king crab Maguimithrax spinosissimus. Mar. Ecol. Prog. Ser. 610, 83–97. doi: 10.3354/meps12842
Arellano S. M., Van Gaest A. L., Johnson S. B., Vrijenhoek R. C., Youn C. M. (2014). Larvae from deep-sea methane seeps disperse in surface waters. Proc. R. Soc B Biol. Sci. 281, 1–8. doi: 10.1098/rspb.2013.3276
Baillon S., Hamel J. F., Wareham V. E., Mercier A. (2014). Seasonality in reproduction of the deep-water pennatulacean coral Anthoptilum grandiflorum. Mar. Biol. 161, 29–43. doi: 10.1007/s00227-013-2311-8
Barthel D., Tendal O. S., Thiel H. (1996). A Wandering Population of the HexactinelIid Sponge Pheronema carpenteri on the Continental Slope off Morocco, Northwest Africa. Mar. Ecol. 17, 603–616. doi: 10.1111/j.1439-0485.1996.tb00420.x
Beazley L., Kenchington E., Murillo F. J., Brickman D., Wang Z., Davies A. J., et al. (2021). Climate change winner in the deep sea? predicting the impacts of climate change on the distribution of the glass sponge vazella pourtalesii. Mar. Ecol. Prog. Ser. 657, 1–23. doi: 10.3354/meps13566
Bett B. J., Rice A. L. (1992). The influence of hexactinellid sponge (Pheronema carpenteri) spicules on the patchy distribution of macrobenthos in the porcupine seabight (Bathyal ne atlantic). Ophelia 36, 217–226. doi: 10.1080/00785326.1992.10430372
Black K. S., Peppe O. C., Gust G. (2003). Erodibility of pelagic carbonate ooze in the northeast Atlantic. J. Exp. Mar. Bio. Ecol. 285–286, 143–163. doi: 10.1016/S0022-0981(02)00524-5
Boury-Esnault N., Efremova S., BÉzac C., Vacelet J. (1999). Reproduction of a hexactinellid sponge: First description of gastrulation by cellular delamination in the porifera. Invertebr. Reprod. Dev. 35, 187–201. doi: 10.1080/07924259.1999.9652385
Bracco A., Liu G., Galaska M. P., Quattrini A. M., Herrera S. (2019). Integrating physical circulation models and genetic approaches to investigate population connectivity in deep-sea corals. J. Mar. Syst. 198, 103189. doi: 10.1016/j.jmarsys.2019.103189
Braunschweig F., Leitao P. C., Fernandes L., Pina P., Neves R. J. J. (2004). The object-oriented design of the integrated water modelling system MOHID. Dev. Water Sci. 55, 1079–1090. doi: 10.1016/S0167-5648(04)80126-6
Burchard H., Bolding K., Villarreal M. (1999). GOTM – a general ocean turbulence model. Theory, applications and test cases.
Busch K., Taboada S., Riesgo A., Koutsouveli V., Ríos P., Cristobo J., et al. (2021). Population connectivity of fan-shaped sponge holobionts in the deep Cantabrian Sea. Deep. Res. Part I Oceanogr. Res. Pap. 167, 103427. doi: 10.1016/j.dsr.2020.103427
Caldeira R. M. A., Reis J. C. (2017). The Azores confluence zone. Front. Mar. Sci. 4. doi: 10.3389/fmars.2017.00037
Colaço A., Creemers M., Mienis F., Beazley L., Hanz U., Bouchard-Marmen M., et al. (2020). Deliverable D4.5 (SponGES) - Deep-sea Sponge Grounds Ecosystems of the North Atlantic: an integrated approach towards their preservation and sustainable exploitation.
Combes M., Vaz S., Grehan A., Morato T., Arnaud-Haond S., Dominguez-Carrió C., et al. (2021). Systematic conservation planning at an ocean basin scale: identifying a viable network of deep-sea protected areas in the north Atlantic and the Mediterranean. Front. Mar. Sci. 8. doi: 10.3389/fmars.2021.611358
Cowen R. K., Sponaugle S. (2009). Larval dispersal and marine population connectivity. Ann. Rev. Mar. Sci. 1, 443–466. doi: 10.1146/annurev.marine.010908.163757
Creemers M. F., Pham C., Defise A., Goulard J., Smith V., Silva A. P., et al. (2019). Sponges on the rocks: exploring A new deep-sea sponge ground of the Azores (Portugal). Deep. Life 12, 7–8.
EMODnet Consortium. (2018). EMODnet digital bathymetry (DTM 2018). doi: 10.12770/18ff0d48-b203-4a65-94a9-5fd8b0ec35f6
FAO. (2008). International guidelines: Management of deep-sea fisheries in the high seas (Rome: Food and Agriculture Organization of the United Nations (FAO)).
García-Martínez R., Flores-Tovar H. (1999). Computer modeling of oil spill trajectories with a high accuracy method. Spill Sci. Technol. Bull. 5, 323–330. doi: 10.1016/S1353-2561(99)00077-8
Gary S. F., Fox A. D., Biastoch A., Roberts J. M., Cunningham S. A. (2020). Larval behaviour, dispersal and population connectivity in the deep sea. Sci. Rep. 10, 1–12. doi: 10.1038/s41598-020-67503-7
Gomes-Pereira J. N., Carmo V., Catarino D., Jakobsen J., Alvarez H., Aguilar R., et al. (2017). Cold-water corals and large hydrozoans provide essential fish habitat for Lappanella fasciata and Benthocometes robustus. Deep. Res. Part II Top. Stud. Oceanogr. 145, 33–48. doi: 10.1016/j.dsr2.2017.09.015
Graves K. P., Bridges A. E. H., Dabrowski T., Furey T., Lyons K., Howell K. L. (2023). Oceanographic variability drives the distribution but not the density of the aggregation forming deep-sea sponge pheronema carpenteri. Deep. Res. Part I Oceanogr. Res. Pap. 191, 103917. doi: 10.1016/j.dsr.2022.103917
Guillas K. C., Kahn A. S., Grant N., Archer S. K., Dunham A., Leys S. P. (2019). Settlement of juvenile glass sponges and other invertebrate cryptofauna on the Hecate Strait glass sponge reefs. Invertebr. Biol. 138, 1–11. doi: 10.1111/ivb.12266
Henry L. A., Roberts J. M. (2014). Applying the OSPAR habitat definition of deep-sea sponge aggregations to verify suspected records of the habitat in UK waters. (Peterborough: Joint Nature Conservation Committee Report No 508).
Hilário A., Metaxas A., Gaudron S. M., Howell K. L., Mercier A., Mestre N. C., et al. (2015). Estimating dispersal distance in the deep sea: Challenges and applications to marine reserves. Front. Mar. Sci. 2. doi: 10.3389/fmars.2015.00006
Howell K. L., Piechaud N., Downie A. L., Kenny A. (2016). The distribution of deep-sea sponge aggregations in the North Atlantic and implications for their effective spatial management. Deep. Res. Part I Oceanogr. Res. Pap. 115, 309–320. doi: 10.1016/j.dsr.2016.07.005
Kavanaugh M. T., Hales B., Saraceno B., Spitz Y. H., White A. E., Letelier R. M. (2014). Hierarchical and dynamic seascapes: A quantitative framework for scaling pelagic biogeochemistry and ecology. Prog. Oceanogr. 120, 291–304. doi: 10.1016/j.pocean.2013.10.013
Kenchington E. L., Patwary M. U., Zouros E. J., Bird C. (2006). Genetic differentiation in relation to marine landscape in a broadcast-spawning bivalve mollusc (Placopecten magellanicus). Mol. Ecol., 1781–1796. Available at: https://doi.org/10.1111/j.1365-294X.2006.02915.x
Kenchington E., Wang Z., Lirette C., Murillo F. J., Guijarro J., Yashayaev I., et al. (2019). Connectivity modelling of areas closed to protect vulnerable marine ecosystems in the northwest Atlantic. Deep. Res. Part I Oceanogr. Res. Pap. 143, 85–103. doi: 10.1016/j.dsr.2018.11.007
Kool J. T., Paris C. B., Barber P. H., Cowen R. K. (2011). Connectivity and the development of population genetic structure in Indo-West Pacific coral reef communities. Glob. Ecol. Biogeogr. 20, 695–706. doi: 10.1111/j.1466-8238.2010.00637.x
Kough A. S., Paris C. B., Butler M. J. IV (2013). Larval connectivity and the international management of fisheries. PloS One 8, 1–11. doi: 10.1371/journal.pone.0064970
Koutsouveli V., Cárdenas P., Conejero M., Rapp H. T., Riesgo A. (2020). Reproductive biology of geodia species (Porifera, tetractinellida) from Boreo-Arctic North-Atlantic deep-sea sponge grounds. Front. Mar. Sci. 7. doi: 10.3389/fmars.2020.595267
Leis J. M. (2020). Perspectives on larval behaviour in biophysical modelling of larval dispersal in marine, demersal fishes. Oceans 2, 1–25. doi: 10.3390/oceans2010001
Leitão P. C., Mateus M., Braunschweig F., Fernandes L., Neves R. (2008). Modelling coastal systems: the MOHID water numerical lab. Perspect. Integr. Coast. Zo. Manage. South Am. 77–88. doi: 10.13140/2.1.1482.4008
Lellouche J. M., Greiner E., Le Galloudec O., Garric G., Regnier C., Drevillon M., et al. (2018). Recent updates to the copernicus marine service global ocean monitoring and forecasting real-time 1g 12° high-resolution system. Ocean Sci. 14, 1093–1126. doi: 10.5194/os-14-1093-2018
Leys S. P., Lauzon N. R. J. (1998). Hexactinellid sponge ecology: Growth rates and seasonality in deep water sponges. J. Exp. Mar. Bio. Ecol. 230, 111–129. doi: 10.1016/S0022-0981(98)00088-4
Leys S. P., Ereskovsky A. V. (2006). Embryogenesis and larval differentiation in sponges. Can. J. Zool. 84, 262–287. doi: 10.1139/z05-170
Lima M. J., Sala I., Caldeira R. M. A. (2020). Physical connectivity between the NE Atlantic seamounts. Front. Mar. Sci. 7. doi: 10.3389/fmars.2020.00238
Lopes D. (2005). Redescription of two Hexactinosida (Porifera, Hexactinellida) from the southwestern Atlantic, collected by Programme REVIZEE. Zootaxa, 43–56.
Lyard F. H., Allain D. J., Cancet M., Carrère L., Picot N. (2021). FES2014 global ocean tides atlas: design and performances florent. Ocean Sci. 17, 615–649. doi: 10.5194/os-17-615-2021
Maldonado M. (2006). The ecology of the sponge larva. Can. J. Zool. 84, 175–194. doi: 10.1139/z05-177
Maldonado M., Aguilar R., Bannister R. J., James J., Conway K. W., Dayton P. K., et al. (2016). Sponge Grounds as Key Marine Habitats : A Synthetic Review of Types, Structure, Functional Roles, and Conservation Concerns Sponge Grounds as Key Marine Habitats : A Synthetic Review of Types, Structure, Functional Roles, and Conservation Concerns. (Switzerland: Springer International Publishing). doi: 10.1007/978-3-319-17001-5
Maldonado M., Carmona M. C., Velásquez Z., Puig A., Cruzado A., López A., et al. (2005). Siliceous sponges as a silicon sink: An overlooked aspect of benthopelagic coupling in the marine silicon cycle. Limnol. Oceanogr. 50, 799–809. doi: 10.4319/lo.2005.50.3.0799
Maldonado M., Young C. M. (1999). Effects of the duration of larval life on postlarval stages of the demosponge Sigmadocia caerulea. J. Exp. Mar. Bio. Ecol. 232, 9–21. doi: 10.1016/S0022-0981(98)00076-8
Mercier A., Sewell M. A., Hamel J. F. (2013). Pelagic propagule duration and developmental mode: Reassessment of a fading link. Glob. Ecol. Biogeogr. 22, 517–530. doi: 10.1111/geb.12018
Mercier A., Sun Z., Hamel J. F. (2011). Reproductive periodicity, spawning and development of the deep-sea scleractinian coral Flabellum angulare. Mar. Biol. 158, 371–380. doi: 10.1007/s00227-010-1565-7
Metaxas A., Saunders M. (2009). Quantifying the “Bio-” Components in biophysical models of larval transport in marine benthic invertebrates: advances and pitfalls. Biol. Bull. 216, 257–272. doi: 10.1086/bblv216n3p257
Morato T., Dominguez-Carrió C., Mohn C., Ocaña Vicente O., Ramos M., Rodrigues L., et al. (2021). Dense cold-water coral garden of Paragorgia johnsoni suggests the importance of the Mid-Atlantic Ridge for deep-sea biodiversity. Ecol. Evol. 11, 16426–16433. doi: 10.1002/%0Aece3.8319
National Centers for Environmental Prediction, National Weather Service, NOAA, U.S. Department of Commerce. (2015). NCEP GFS 0.25 degree global forecast grids historical archive. Available online at: https://rda.ucar.edu/datasets/dsd084001/.
North E. W., Gallego A., Petitgas P., Ådlandsvik B., Bartsch J., Brickman D., et al. (2009). Manual of recommended practices for modelling physical-biological interactions during fish early life.
Paris C. B., Helgers J., van Sebille E., Srinivasan A. (2013). Connectivity Modeling System: A probabilistic modeling tool for the multi-scale tracking of biotic and abiotic variability in the ocean. Environ. Model. Software 42, 47–54. doi: 10.1016/j.envsoft.2012.12.006
Pham C. K., Vandeperre F., Menezes G., Porteiro F., Isidro E., Morato T. (2015). The importance of deep-sea vulnerable marine ecosystems for demersal fish in the Azores. Deep. Res. Part I Oceanogr. Res. Pap. 96, 80–88. doi: 10.1016/j.dsr.2014.11.004
Phelps J. J. C. (2015). Modelling Hydrodynamic Transport and Larval Dispersal in North-East Atlantic Shelf Seas. [PhD Thesis]. University of Liverpool.
Pineda J., Porri F., Starczak V., Blythe J. (2010). Causes of decoupling between larval supply and settlement and consequences for understanding recruitment and population connectivity. J. Exp. Mar. Bio. Ecol. 392, 9–21. doi: 10.1016/j.jembe.2010.04.008
Rice A. L., Thurston M. H., New A. L. (1990). Dense aggregations of a hexactinellid sponge, Pheronema carpenteri, in the Porcupine Seabight (northeast Atlantic Ocean), and possible causes. Prog. Oceanogr. 24, 179–196. doi: 10.1016/0079-6611(90)90029-2
Rix L., De Goeij J. M., Van Oevelen D., Struck U., Al-Horani F. A., Wild C., et al. (2018). Reef sponges facilitate the transfer of coral-derived organic matter to their associated fauna via the sponge loop. Mar. Ecol. Prog. Ser. 589, 85–96. doi: 10.3354/meps12443
Ross R. E., Nimmo-Smith W. A. M., Howell K. L. (2016). Increasing the depth of current understanding: Sensitivity testing of deep-sea larval dispersal models for ecologists. PloS One 11, 1–25. doi: 10.1371/journal.pone.0161220
Ross R. E., Nimmo-Smith W. A. M., Torres R., Howell K. L. (2020). Comparing deep-sea larval dispersal models: A cautionary tale for ecology and conservation. Front. Mar. Sci. 7. doi: 10.3389/fmars.2020.00431
Ross R. E., Wort E. J. G., Howell K. L. (2019). Combining distribution and dispersal models to identify a particularly vulnerable marine ecosystem. Front. Mar. Sci. 6. doi: 10.3389/fmars.2019.00574
Sala I., Caldeira R. M. A., Estrada-allis S. N., Froufe E., Couvelard X. (2013). Lagrangian transport pathways in the northeast Atlantic and their environmental impact. Limnol. Oceanogr. Fluids Environ. 3, 40–60. doi: 10.1215/21573689-2152611
Sala I., Harrison C. S., Caldeira R. M. A. (2016). The role of the azores archipelago in capturing and retaining incoming particles. J. Mar. Syst. 154, 146–156. doi: 10.1016/j.jmarsys.2015.10.001
Samuelsen A., Schrum C., Yumruktepe V. Ç., Daewel U., Roberts E. M. (2022). Environmental change at deep-sea sponge habitats over the last half century: A model hindcast study for the age of anthropogenic climate change. Front. Mar. Sci. 9. doi: 10.3389/fmars.2022.737164
Santos M., Moita M. T., Bashmachnikov I., Menezes G. M., Carmo V., Loureiro C. M., et al. (2013). Phytoplankton variability and oceanographic conditions at Condor seamount, Azores (NE Atlantic). Deep. Res. Part II Top. Stud. Oceanogr. 98, 52–62. doi: 10.1016/j.dsr2.2013.05.037
Selkoe K. A., Toonen R. J. (2011). Marine connectivity: A new look at pelagic larval duration and genetic metrics of dispersal. Mar. Ecol. Prog. Ser. 436, 291–305. doi: 10.3354/meps09238
Simons R. D., Siegel D. A., Brown K. S. (2013). Model sensitivity and robustness in the estimation of larval transport: A study of particle tracking parameters. J. Mar. Syst. 119–120, 19–29. doi: 10.1016/j.jmarsys.2013.03.004
Somoza L., Medialdea T., González F. J., Machancoses S., Candón J. A., Cid C., et al. (2021). High-resolution multibeam bathymetry of the northern Mid-Atlantic Ridge at 45–46° N: the Moytirra hydrothermal field. J. Maps 17, 184–196. doi: 10.1080/17445647.2021.1898485
Spetland F., Rapp H. T., Hoffmann F., Tendal O. S. (2007). Sexual reproduction of Geodia barretti Bowerbank 1858 (Porifera, Astrophorida) in two Scandinavian fjords. Mus. Nac. Ser. Livros 1858, 613–620.
Steneck R. S., Paris C. B., Arnold S. N., Ablan-Lagman M. C., Alcala A. C., Butler M. J., et al. (2009). Thinking and managing outside the box: Coalescing connectivity networks to build region-wide resilience in coral reef ecosystems. Coral Reefs 28, 367–378. doi: 10.1007/s00338-009-0470-3
Stratoudakis Y., Hilario A., Ribeiro C., Abecasis D., Gonçalves E. J., Andrade F., et al. (2019). Environmental representativity in marine protected area networks over large and partly unexplored seascapes. Glob. Ecol. Conserv. 17. doi: 10.1016/j.gecco.2019.e00545
Sun Z., Hamel J. F., Mercier A. (2011). Planulation, larval biology, and early growth of the deep-sea soft corals Gersemia fruticosa and Duva florida (Octocorallia: Alcyonacea). Invertebr. Biol. 130, 91–99. doi: 10.1111/j.1744-7410.2011.00229.x
Swanborn D. J. B., Huvenne V. A. I., Pittman S. J., Woodall L. C. (2022). Bringing seascape ecology to the deep seabed: A review and framework for its application. Limnol. Oceanogr. 67, 66–88. doi: 10.1002/lno.11976
Swearer S. E., Treml E. A., Shima J. S. (2019). A review of biophysical models of marine larval dispersal. Oceanogr. Mar. Biol. 57, 325–356. doi: 10.1201/9780429026379-7
Taboada S., Whiting C., Wang S., Ríos P., Davies A. J., Mienis F., et al. (2023). Long distance dispersal and oceanographic fronts shape the connectivity of the keystone sponge Phakellia ventilabrum in the deep northeast Atlantic. Front. Mar. Sci. 10. doi: 10.3389/fmars.2023.1177106
Taranto G. H. (2022). Mapping deep-sea biodiversity and good environmental status in the Azores: assisting with the implementation of EU Marine Strategy Framework Directive.
Teixidó N., Gili J. M., Uriz M. J., Gutt J., Arntz W. E. (2006). Observations of asexual reproductive strategies in antarctic hexactinellid sponges from ROV video records. Deep. Res. Part II Top. Stud. Oceanogr. 53, 972–984. doi: 10.1016/j.dsr2.2006.02.008
Thomas T., Moitinho-Silva L., Lurgi M., Björk J. R., Easson C., Astudillo-García C., et al. (2016). Diversity, structure and convergent evolution of the global sponge microbiome. Nat. Commun. 7, 1–12. doi: 10.1038/ncomms11870
Vic C., Gula J., Roullet G., Pradillon F. (2018). Dispersion of deep-sea hydrothermal vent effluents and larvae by submesoscale and tidal currents. Deep. Res. Part I Oceanogr. Res. Pap. 133, 1–18. doi: 10.1016/j.dsr.2018.01.001
Viegas C. (2022). 3-D Biogeochemical and hydrodynamic models in the Azores – A tool to understand marine ecosystems. [PhD Thesis]. University of the Azores.
Vieira R. P., Bett B. J., Jones D. O. B., Durden J. M., Morris K. J., Cunha M. R., et al. (2020). Deep-sea sponge aggregations (Pheronema carpenteri) in the Porcupine Seabight (NE Atlantic) potentially degraded by demersal fishing. Prog. Oceanogr. 183, 102189. doi: 10.1016/j.pocean.2019.102189
Wang B., Fennel K., Yu L., Gordon C. (2020). Assessing the value of biogeochemical Argo profiles versus ocean color observations for biogeochemical model optimization in the Gulf of Mexico. Biogeosciences 17, 4059–4074. doi: 10.5194/bg-17-4059-2020
Wang S., Kenchington E., Wang Z., Davies A. J. (2021). Life in the fast lane: modeling the fate of glass sponge larvae in the gulf stream. Front. Mar. Sci. 8, 1–14. doi: 10.3389/fmars.2021.701218
Wang G., Qiao F. (2020). Mirror patterns of physical variables in the ocean. Clim. Dyn. 54, 3109–3120. doi: 10.1007/s00382-020-05161-1
White M. (2003). Comparison of near seabed currents at two locations in the Porcupine Sea Bight - Implications for benthic fauna. J. Mar. Biol. Assoc. United Kingdom 83, 683–686. doi: 10.1017/S0025315403007641h
Xavier J. R., Tojeira I., Van Soest R. W. M. (2015). On a hexactinellid sponge aggregation at the Great Meteor seamount (North-east Atlantic). J. Mar. Biol. Assoc. United Kingdom 95, 1389–1394. doi: 10.1017/S0025315415000685
Keywords: deep-sea sponge, connectivity, larval dispersal, modelling, hydrodynamics, Pheronema carpenteri
Citation: Viegas C, Juliano M and Colaço A (2024) Larval dispersal and physical connectivity of Pheronema carpenteri populations in the Azores. Front. Mar. Sci. 11:1393385. doi: 10.3389/fmars.2024.1393385
Received: 29 February 2024; Accepted: 16 August 2024;
Published: 22 October 2024.
Edited by:
Stefán Áki Ragnarsson, Marine and Freshwater Research Institute, IcelandReviewed by:
Fanny Girard, University of Hawaii at Manoa, United StatesDidier Alain Jollivet, Centre National de la Recherche Scientifique (CNRS), France
Copyright © 2024 Viegas, Juliano and Colaço. This is an open-access article distributed under the terms of the Creative Commons Attribution License (CC BY). The use, distribution or reproduction in other forums is permitted, provided the original author(s) and the copyright owner(s) are credited and that the original publication in this journal is cited, in accordance with accepted academic practice. No use, distribution or reproduction is permitted which does not comply with these terms.
*Correspondence: Cláudia Viegas, cnviegas@gmail.com