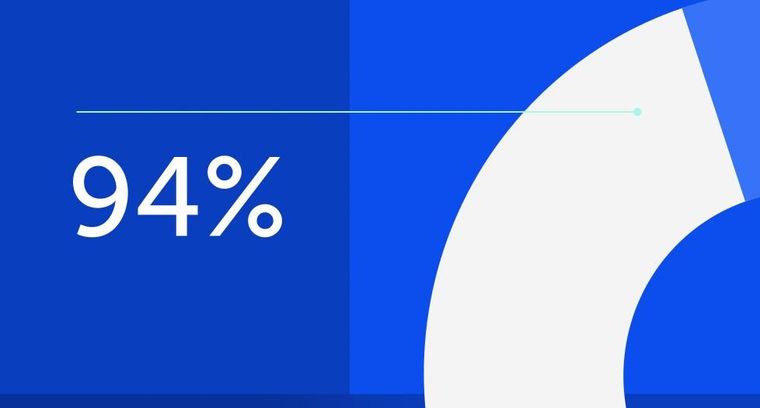
94% of researchers rate our articles as excellent or good
Learn more about the work of our research integrity team to safeguard the quality of each article we publish.
Find out more
ORIGINAL RESEARCH article
Front. Mar. Sci., 21 June 2024
Sec. Marine Fisheries, Aquaculture and Living Resources
Volume 11 - 2024 | https://doi.org/10.3389/fmars.2024.1383063
The Mediterranean Sea represents the lower latitudinal limit of the European sprat range, where it is considered a sentinel species favoring temperate–cold temperatures. Sprattus sprattus is a plankton feeder that plays an important ecological role in contributing to the transfer of energy from lower to higher trophic levels, but climate-driven increases in sea temperatures may reduce the suitability of the pelagic habitat and threaten the tropho-dynamic role of sprat in areas such as the Adriatic Sea. The latter is an enclosed basin characterized by shallow waters and high annual temperature variations. Here, to investigate present and future habitat suitability areas for sprat, we applied four species distribution models (SDMs) using fishery-independent data collected from 2004 to 2021, along with remotely sensed and modeled environmental variables. A set of nine environmental predictors was tested, and the resulting best model was averaged in an ensemble model approach. The best ensemble models revealed good to high accuracy (sensitivity and specificity ≥ 0.8). The sea surface temperature and chlorophyll concentration emerged as the main explanatory variables in predicting the potential habitat of sprats, followed by bathymetry. The resulting probability of occurrence maps revealed that the species is bounded in the northern Adriatic Sea, where a longitudinal shift of high-suitability habitats from inshore to deeper and colder waters was detected between early and late summer. Future projections under IPCC representative concentration pathway (RCP) scenarios 4.5 (intermediate emission) and 8.5 (high emission-warm) underline small changes along with a gain of new areas in late summer in the short-term period up to 2050. Conversely, the temperature increase projected for the end of the century is predicted to cause a loss of suitable habitat area for sprats of up to 88% under a high emission-warm scenario relative to current habitat occupancy throughout the basin.
Anthropogenic activities are the most important drivers of ocean biome change. The high level of greenhouse gas emissions is leading to global climate change, which affects marine organisms (Yu et al., 2018; Kanamori et al., 2019). The shift in climate regime is attributed to changes in sea temperature, salinity, and circulation, which are controlled by regional atmospheric variations and large-scale teleconnections (Cheung et al., 2009; Shaltout and Omstedt, 2014). Such changes result in a northward shift of fish populations, with unpredictable consequences for regional communities and food-web structure (Brander, 2010; Cheung et al., 2013; Chust et al., 2013). Enclosed basins are more susceptible to climate change, including the Mediterranean Sea, which is regarded as a climate change hotspot particularly vulnerable to the rise in sea temperatures (Shaltout and Omstedt, 2014).
In this context, studies on the distribution of fish species in relation to environmental conditions are of particular interest for ecosystem-based management strategies (Effrosynidis et al., 2020; Schickele et al., 2021). Several species play a key role in mediating severe community changes due to environmental variations (Azzurro et al., 2022). Cold-favoring (boreal) species such as sprats (Sprattus sprattus) can be used as sentinels as they are highly sensitive to increases in sea temperatures (De Felice et al., 2021).
Sprat, S. sprattus (Linnaeus 1758) is one of the main clupeids species found in the Mediterranean Sea, along with Sardina pilchardus and Sardinella aurita (Froese and Pauly, 2023). Since sprat is a boreal species, it is mainly distributed in the northern part of the Mediterranean basin in northern Spain (GFCM Geographical Sub-Area (GSA) 6), in the Gulf of Lion (GSA 7), and in the northern Adriatic Sea (GSA 17), where it is found in shallow waters up to a depth of 50 m, see Figure 1 (Froese and Pauly, 2023). As a cold-favoring species, it may be threatened by rising sea temperatures, leading to an overall alteration of the pelagic ecosystem (Schickele et al., 2021). In addition to temperature, an ensemble of abiotic and biotic factors have been shown to affect the life cycle and distribution of sprat, such as wind, dissolved oxygen concentration, ocean circulation, salinity, and nutrient concentrations (Voss et al., 2008; Ojaveer and Kalejs, 2010; Tsikliras et al., 2019). The distribution of sprat in the Adriatic Sea depends on migration between the more productive feeding area close to the Po River Delta (44.950°N–12.417°E) and the spawning grounds in the eastern Adriatic (Tičina et al., 2000; Tičina, 2003; Piccinetti et al., 2012). In fact, sprat is a multiple (batch) spawning species that reaches the peak of its spawning activity in the winter months in the Adriatic Sea (Tičina et al., 2000).
Figure 1 GFCM Geographical Sub-Area (GSA) division of the Western and Central Mediterranean Sea on top. Below is the map of the Adriatic Sea with the acoustic transects annually carried out in the western and eastern parts of the basin in the framework of the Mediterranean International Acoustic Survey (MEDIAS) project.
Sprat is the third species in terms of landings by weight in the General Fisheries Commission of the Mediterranean (GFCM) area (Mediterranean Sea and Black Sea) (FAO, 2023). It is a target species of purse seine and pelagic trawl in the Black Sea, while in the western Mediterranean Sea and Adriatic Sea, it is a by-catch species with low commercial value, which contributes with a very small percentage (less than 1%) to pelagic trawl landings being more frequently discarded (Tičina, 2003; FAO, 2023). Nevertheless, in the pelagic ecosystem, sprat plays an important tropho-dynamic role both by exerting top-down controls on zooplankton and by being an abundant prey resource for piscivorous species (Peck et al., 2012; Fanelli et al., 2023). The first stock assessment models carried out for sprat in the Mediterranean Sea have shown a pessimistic situation for the stock (Angelini et al., 2021) confirming the decline in biomass registered by acoustic surveys in the Adriatic Sea (Leonori et al., 2021).
Despite its importance, sprat habitat suitability has never been assessed at a regional scale in the Mediterranean Sea (De Felice et al., 2021; Schickele et al., 2021), unlike sardine and anchovy, which have been extensively studied through the application of species distribution models (SDMs) (Giannoulaki et al., 2011; Tugores et al., 2011; Giannoulaki et al., 2013). Habitat loss, along with exploitation, is considered one of the main causes of target and nontarget fish species’ local extinctions (Dulvy et al., 2003). SDMs are powerful tools for understanding species distribution patterns in response to environmental factors and predicting habitat loss under climate change scenarios (Guisan and Zimmermann, 2000; Guisan and Thuiller, 2005). They allow spatially explicit predictions of habitat suitability areas to be derived from the statistical relationships between environmental drivers and occurrence data for a given species (Guisan and Zimmermann, 2000). A large number of statistical approaches have been developed for SDMs including regression-based methods such as Generalized Linear Models (GLM) and Generalized Additive Models (GAM) and machine learning methods such as Random Forest (RF) and Generalized Boosting Models (GBM). RF uses an ensemble of decision trees, created by randomly resampling data from the original dataset, which are combined to classify suitable habitats. At the end of the run, the grid cell is categorized based on probability into favorable and nonfavorable habitats. GBM on the other hand, combines the use of regression trees with boosting, which is another ensemble method based on the generation of sequential trees, that attempts to explain the variation that cannot be explained by existing trees so that each new tree minimizes a loss function (Marmion et al., 2009; Quinci et al., 2022). Therefore, they are more suitable when only presence data are available or when pseudo-absence data are included in the computations (Gomez and Cassini, 2015; Effrosynidis et al., 2020). Conversely, when enough real absence and presence data are available, regression models are usually preferred (Lauria et al., 2015; Bonanno et al., 2016; Ebango et al., 2020). Binomial GLM is a generalization of the linear regression model making the function of the variance of each measurement dependent on its predicted value. However, GAM can account for nonlinear relationships between environmental variables and species occurrence or abundance being a flexible and powerful method that provides more realistic interactions and nonlinearities being more similar to machine learning methods (Marra and Wood, 2011; Albo-Puigserver et al., 2016). The use of different models to estimate the habitat of a species can result in a large variability of predictions, leading to possible biases linked to the choice of the model. For this reason, results from different SDMs are usually combined in an ensemble model, averaging their assessments to enhance the robustness of the final forecast (Robinson et al., 2017; Araùjo et al., 2019).
In this study, we examined the long-term potential range shift of the species to evaluate any possible implications for the ecosystem and small pelagic fish resources management in the Adriatic Sea. We employed 18 years of data collected during national and international acoustic surveys in the Adriatic Sea along with a combination of present and future modeled environmental variables with the aim of investigating the environmental processes that lead to sprat’s habitat suitability. Four different SDMs in an ensemble model approach were applied to predict present and past habitat suitability maps of sprat and project its future distribution in the Adriatic Sea under two IPCC climate change Representative concentration pathway (RCP) scenarios, 4.5 (intermediate emission) and 8.5 (high emission-warm), derived from the POLCOM-ERSEM model (Kay, 2020) in short-, mid-, and long-term periods.
Presence/absence data were obtained from over 1,200 sampling hauls conducted during several acoustic surveys in the Adriatic Sea. Specifically, we scrutinized data collected on board R/V G Dallaporta and R/V BIOS DVA performed in early summer and late summer between 2004 and 2021, in the framework of national (ECHOADRI and PELMON) and Mediterranean International Acoustic Survey (MEDIAS) projects (Leonori et al., 2021), as shown in Table 1. The survey design consists of systematic parallel line transects perpendicular to the coastline or adapted to the geomorphology of the survey area, conducted during the daytime in bathymetry between 10 m and 200 m, as shown in Figure 1 (MEDIAS, 2021).
Table 1 List of acoustic surveys and the corresponding number of pelagic trawl hauls scrutinized in the present study.
In parallel to acoustic sampling, routine biological sampling was carried out to enable identification of echo traces of fish schools and to obtain a biological sample. Therefore, pelagic trawl hauls were made on-demand at a vessel speed of ~ 4 knots for 30 min at different locations within the survey area, adapting the sampling intensity to the occurrence of echo traces and fish schools in each area. Biological sampling was performed by the two research vessels equipped with the same type of four-seam pelagic trawl net ~ 15 m horizontal and ~ 11 m vertical opening with an 18-mm stretched mesh size cod-end suitable for catching the small pelagic fish schools of juveniles and adults that are the survey’s target species. On the R/V G. Dallaporta, however, the net behavior is currently monitored in real time owing to the SIMRAD FX80 trawl sonar system, whereas on the R/V BIOS DVA, the net positions are monitored using the SIMRAD ITI system. Once on board, the total catch is weighted, and in the event of very abundant catches, a subsample is collected for further analysis.
In this study, any haul holding at least 10 sprat specimens was designated as a sprat presence location (1); otherwise, it was deemed absent (0). Several thresholds were attempted during preprocessing scrutinization. A threshold of 10 specimens was selected based on the expertise of the authors of the present work and the available dataset dealing with the minimum sample size needed for the analysis (Hernandez et al., 2006), yielding a data matrix encompassing 215 presence and 1,035 absence points (see map in Supplementary Figure S1).
The Adriatic Sea is an enclosed basin characterized by an extensive continental shelf that, with the exception of Pomo/Jabuka Pit, does not exceed the 200-m isobath in the north-mid-part as shown in Figure 1 (Russo and Artegiani, 1996). The shallow waters, together with the characteristic cyclonic (anticlockwise) circulation, cause high-seasonal and annual temperature variations, while the nutrient load is strongly influenced by the Po runoff in the northern part of the basin, which could lead to strong variations affecting the primary production. The freshwater inflow determines more pronounced eutrophic conditions for the western Adriatic Sea compared to the eastern Adriatic (Giani et al., 2012).
Despite the fact that the small pelagic fish biomass and distribution in the Mediterranean Sea can be affected by the Atlantic Multidecadal Oscillation (AMO) and North Atlantic Oscillation (NAO) (Tsikliras et al., 2019), the most important environmental drivers for the sprat life cycle in semi-enclosed basins, like the Adriatic and Baltic seas, are temperature, primary production, depth, salinity, and oxygen concentration (Ojaveer and Kalejs, 2010; Tsikliras et al., 2019; Schickele et al., 2021). The feeding success of sprat larvae can be affected even by wind and cloud coverage, but temperature emerged as the main driver for ontogenesis and sprat growth (Petereit et al., 2008; Voss et al., 2008; Frisk et al., 2015). For these reasons, in this work, we focused on the factors that most commonly affect the distribution of small pelagic fish that may be relevant to the study area and the target species (Fernández-Corredor et al., 2021). Specifically, satellite data derived chlorophyll-a concentration (CHL in mg m−3), sea surface water potential temperature (SST in °C), bottom seawater potential temperature (BST in °C), dissolved oxygen (O2 in mmol m−3), net primary production (NPP in mol m−3), phytoplankton concentration (PHYC in mol m−3), sea surface salinity (SSS in PSU), and sea-level anomaly (SLA in m) were downloaded from the Copernicus Marine Services (Escudier et al., 2020; Clementi et al., 2021; Cossarini et al., 2021), in the period 2004–2021, while depth in meters was downloaded from the MARSPEC database (Sbrocco and Barber, 2013). The POLCOM-ERSEM model (Kay, 2020) of total chlorophyll-a concentration (CHLT in mg m−3) and sea surface water potential temperature (SSTF in °C) under IPCC RCP 4.5 and 8.5 scenarios were downloaded from the Climate Data Storage for the contemporary period (2006–2021), short-term projections (2029–2049), mid-term projections (2054–2074), and long-term projections (2079–2099). All environmental variables were downloaded at the maximum resolution, and only the layer at 5 m depth was retained for computations except for BST and SLA (see Supplementary Table S1 for more details).
When available, daily values encompassing years and months in which the surveys were conducted, (June, July, and September from 2004 to 2021) were processed in the Q-GIS environment using the SAGA plugin to obtain seasonally averaged regular grids (Conrad et al., 2015). Early summer raster files were derived from daily values for June and July, while late summer values were obtained by averaging September values. Subsequently, satellite environmental maps were interpolated through a cubic-spline interpolation and aligned to obtain a finer grid of 0.031° E (approximately one nautical mile) for each explanatory variable.
The environmental variables and the response variable were treated as late summer (data from the western Adriatic Sea from 2004 to 2014 and the eastern Adriatic Sea from 2009 to 2021) and early summer (data from the western Adriatic Sea from 2015 to 2021), merging data from different years and areas. Each dataset was randomly split into training (70% of the total hauls) and validation datasets (30% of the total samples), ensuring a balance was maintained to avoid any bias in model performance (Fielding and Bell, 1997). Since CHL and PHYC had shown a non-normal distribution, they were logarithmically transformed to achieve a normal distribution of the data. Depth was similarly transformed using a cubic root. Before applying the models, we assessed the correlation between independent environmental variables by means of the Pearson coefficient. Any variables exhibiting a significant correlation, characterized by a Pearson correlation r greater than 0.7 and less than −0.7, were independently selected (Bosch et al., 2018). Accordingly, from any possible combination of uncorrelated variables, four and three predictor sets were defined for early summer and late summer, respectively (see Table 2).
Using presence/absence data as response variables and the predictor sets as explanatory variables, four modeling techniques were fitted in the “biomod2” package in R (Thuiller et al., 2023), including two regression models, GAM and GLM, and two machine learning models, RF and GBM. This package has the advantage of being able also to compute an ensemble model consisting of the average of the fitted individual models. As is customary for presence/absence data, the two regression models were fitted to the training dataset assuming a binomial error distribution and a logit link function selecting the best model on the basis of Akaike’s information criteria (AIC), and deviance explained (Marra and Wood, 2011). GAM models were computed using the “GAM_mgcv” algorithm assuming a binomial error distribution and a logit link function, while the degree of smoothing, expressed as the number of knots k, was automatically selected (Wood, 2017). The two machine learning methods were set using the default settings of tuning and fitting parameters given in the biomod2. Finally, an ensemble model was computed through the weighted mean technique (EMwmean), where the probabilities derived from the chosen models are assigned weights based on their respective evaluation true skill statistic (TSS) scores.
The combination of two datasets, predictor sets, four modeling techniques, and ensemble models led to 70 fitted models. Firstly, we evaluated the variable importance through the method used in the biomod2 package. It adopts a machine-learning approach after the models have been trained. It randomly perturbs one of the variables in each permutation and calculates a correlation score between the standard prediction and the new prediction. This score is regarded as an estimate of the variable’s significance within the model. The higher the score, the greater the importance of the predictor variable in the model. It is important to note that this method does not consider interactions between variables that have been previously assessed through a correlation matrix (Thuiller et al., 2023). Only environmental variables that reached a variable importance score of 0.3 in at least one of the four models were retained for the following steps. The obtained refined predictor sets were fitted for a second run of the individual models. Next, an ensemble model was performed for each refined predictor set. The predictive performance of each model was validated by validation with the independent test dataset and quantified based on the TSS, the area under the curve (AUC), and accuracy (sensitivity and specificity) values. In particular, the TSS value was used to choose the model with the best performance, characterized by the lowest level of potential prediction error for sprat-habitat suitability projection. The model cannot accurately discriminate if the AUC is less than 0.5; an AUC of 0.7 indicates satisfactory discrimination; an AUC of 0.8 indicates good discrimination; and an AUC of 0.9 or more indicates very good discrimination (Hosmer et al., 2013). TSS is a measure of agreement between the predicted and observed values. Values between 0.0 and 0.4 indicate slight to fair model performance; values between 0.4 and 0.6 indicate moderate model performance; values between 0.6 and 0.8 indicate substantial model performance; and values between 0.8 and 1.0 indicate almost perfect model performance (Somodi et al., 2017). Accuracy is determined by sensitivity and specificity, which measure the model’s ability to properly identify the values correctly assigned as presence (positive) and absence (negative), respectively. We calculated the aforementioned metrics by comparing the predicted values to the observed values using a threshold criterion based on equal sensitivity and specificity with the exception of AUC, which is a threshold-independent measure.
Based on the validation process, the best ensemble models were used to predict the habitat suitability map of sprat in early summer and late summer for the entire period (from 2004 to 2021) in the Adriatic Sea. Specifically, we plotted the probability of occurrence of the species over a grid mesh size of 0.031°. Rare, occasional, preferential, and persistency habitat allocation maps were created by applying a threshold to the mean probability values: areas with a probability of occurrence< 0.25 were set as rare; areas with a probability of occurrence between 0.25 and 0.5 were set as occasional; areas with a probability of occurrence > 0.5 and< 0.75 were designated as preferential; areas with a probability of occurrence > 0.75 were set as persistency (Giannoulaki et al., 2017). The average seasonally mean environmental values of each selected variable were used to predict the annual habitat suitability map over the time series and seasons, providing information on the distribution of the species during seasons and areas where acoustic surveys had not been conducted.
Since there is often a noticeable shift in the scale of the IPCC-style model variables when compared to observed satellite oceanographic conditions, we compared the temperature and chlorophyll-a concentration between the hindcast (Copernicus) and model (POLCOM-ERSEM) in the overlapping period (2006–2021). Hence, we calculated the differences between the two datasets and adjusted the model-derived variable following the delta model bias correction approach described in Hare et al. (2012). The difference between hindcast T° (SST) and model T° of the overlapping period (SPTP) (ΔTijk) was computed for each year (i), season (j), and RCP (k). Next, we computed the mean delta of the overlapping period (jk) where n is the number of years.
Accordingly, the difference between satellite CHL and modeled CHLTP (ΔCHLijk) was computed through the ln difference.
Successively, the mean values for the future SST() and CHL() were computed across three distinct time spans to encompass short-term periods (2029–2049), mid-term periods (2054–2074), and long-term periods (2079–2099). Finally, the two variables were corrected for each period (l) as follows:
After the treatment of the future environmental dataset, the best-fit ensemble models identified through the validation process for predicting the sprat-habitat suitability (hindcast) were employed for forecasting the sprat-habitat suitability under the new environmental conditions in (i) early and late summer, (ii) RCP 4.5 and 8.5, and (iii) short-, mid-, and long-term periods. Finally, we quantified the difference in the extension of the habitat suitability map of the species comparing current end future projections in terms of percentage gain and loss of area through the “BIOMODRangesize” function.
The environmental factors describing the primary production of CHL, PHYC, and NPP were significantly correlated with each other (r > 0.9; p-value< 0.0001) in both seasons. Moreover, NPP has a strong negative correlation with SSS and a strong positive correlation with BT (r > 0.75; p-value< 0.0001). Therefore, only CHL was retained as a proxy for primary production during the computations of the single and ensemble models GLM, GAM, RF, GBM, and EMwmean (the correlation matrix can be found in Supplementary Figures S2, S3). Four sets of predictor variables for early summer and three for late summer were used, as listed in Table 2. Since SLA and O2 consistently had a score< 0.3, these variables were discarded, and a second run for each single and ensemble model was performed using only the selected variables from Table 2.
The best ensemble model performance was obtained with predictor set 1 (P1) in both seasons, as shown in Figure 2. Therefore, the variables used in the final EMwmean for early and late summer present, past, and future projections are SST, CHL, and depth. Individual model evaluation metrics shown in Table 3 indicated that the model performs better for early summer predictions than for the late summer model. Nevertheless, in both seasons, the sensitivity scores ≥ 0.90 indicate a very low probability of obtaining false absence values, and the specificity ≥ 0.80 proves a high accuracy in detecting presence values. Moreover, the machine learning methods RF and GBM gave more accurate results than regression models GAM and GLM. Overall, SST and CHL resulted as the main explanatory variables for sprat, followed by depth in both seasons, as shown in Figure 3.
Figure 2 True skill statistic (TSS) score values for each combination of predictor set and modeling technique. The environmental variables in each predictor set (P1–P4) and modeling technique (GLM, GAM, RF, GBM, and EM) are described in Table 3. EM depicts the weighted mean ensemble model.
Table 3 Model evaluation of four modeling techniques and the derived ensemble model predicting the distribution of sprat-habitat suitability.
Figure 3 Variable importance boxplot results from the modeling techniques GLM, GAM, RF, GBM, and EMwmean, which depict the weighted mean ensemble model. The medians (horizontal lines), percentiles (box borders), and 5%–95% percentiles (vertical lines) are shown. Early summer on top; late summer below.
According to response curves shown in Figure 4, areas characterized by shallow waters and elevated primary production, as measured by the chlorophyll-a concentration (mg m−3) in early summer, had a greater likelihood of harboring sprat. CHL is the main driver in late summer too, when there is a shift in the preferential depth of the species to deeper waters. It is interesting to note the discrete thermal niche of sprat in early summer, when the species in the Adriatic Sea is mainly found between temperatures of 23°C and 24°C, as opposed to late summer, when the probability of occurrence increases at temperatures of approximately 21°C (Figure 4).
Figure 4 Response curve showing the variables that account for more than 30% of determining the distribution of sprat in early summer on top and late summer below. Logarithmically transformed chlorophyll-a concentration (CHL in mg m−3), sea surface water potential temperature (SST in °C), and cubic root transformed bathymetry. The color lines denote the four individual modeling techniques, GLM, GAM, RF, and GBM, and the weighted mean ensemble model, EMwmean. The shadow areas depict the confidence intervals at p = 0.05. The density of points for different variable values is indicated by the rug present beneath the plots displaying single variable effects.
The habitat suitability maps depicted in Figure 5 show a persistency area located in front of the Po Delta in the eastern part of the basin during early summer, followed by a reduction in late summer. The overall suitable areas are concentrated in the northeastern coastal waters during early summer, with an occasional presence up to the mid-Adriatic Sea. In late summer, these areas split into two main preferential spots, one on the western side of the basin and the other around the early summer persistency area.
Figure 5 Overall habitat suitability map of sprat in the Adriatic Sea on top and habitat allocation map below. The color scales depicted the probability of occurrence of the species in each square of the grid. Persistency areas are in yellow, preferential areas are in green, occasional areas are in light blue, and rare areas are in blue.
Along the time series, past predictions of the sprat-habitat suitability map reveal a fluctuating extent of suitability area. The area associated with a high probability of sprat presence extended further south and offshore in both seasons in 2007, 2010, and 2014 (Supplementary Figures S4, S5). Early summer is characterized by a general increase in the suitability region across the time series, with the exception of 2017 and 2018, when the suitability range was limited to the north Adriatic Sea (Supplementary Figure S4). An opposite trend was observed in late summer, when the last 6 years of the studied time series (2015–2021) were characterized by a shrinkage of suitable sea grounds for sprat, which is particularly evident in 2018, along with a reduction in the western Adriatic Sea in 2011 (Supplementary Figure S5).
The goodness-of-fit results of the ensemble models under the long-term RCP 8.5 scenario presented in Table 3 underline a good to high accuracy and a moderate to good performance of the final ensemble model in forecasting the habitat suitability of sprat between 2079 and 2099. The robustness of the models under other scenarios is presented in Supplementary Table S2. Our projections indicate in early summer a loss of habitat of up to 94% for the warming scenario RCP 8.5 and up to 51% for the stabilization scenario 4.5 in the long-term period (2079–2099), as shown in Table 4 and Figure 6. Conversely, in the short-term period (2029–2049), we observed a spread of sprat-suitability habitat toward the Croatian waters, which are characterized by lower SST (Figures 7, 6). The same shift was detected also in the mid-term period (2054–2074), when, however, the habitat loss in the northeastern Adriatic Sea overtook largely the gain (Figure 6; Table 4). Late summer is generally characterized by a predicted migration of the species from persistency areas to deep and offshore waters. In this season, we registered a severe drop in habitat suitability wideness in the long-term period, albeit smaller compared to early summer, with a peak for RCP 8.5 of −43% (Table 4).
Table 4 Results of habitat suitability extent changes in comparison with contemporary environmental suitability areas under two IPCC representative concentration pathway (RCP) scenarios 4.5 and 8.5 in short- (2029–2049), mid- (2054–2074), and long-term (2079–2099) periods.
Figure 6 Habitat suitability extent changes in comparison with contemporary environmental suitability areas in early summer (left panel) and late summer (right panel) under IPCC representative concentration pathway (RCP) scenarios 4.5 and 8.5 in short- (2029–2049), mid- (2054–2074), and long-term (2079–2099) periods.
Figure 7 Future prediction map of the probability of occurrence (color scale) of sprat in early summer (left panel) and late summer (right panel) under two IPCC representative concentration pathway (RCP) scenarios 4.5 and 8.5 in short- (2029–2049), mid- (2054–2074), and long-term (2079–2099) periods.
Habitat suitability modeling is an effective technique for predicting species distribution for Ecosystem-Based Management (EBM) purposes, as it enables the prediction of species distribution by examining the nonlinear interactions between species and environmental variables (Araùjo et al., 2019). The present study used an ensemble model to present a comprehensive analysis of the past, present, and future habitat suitability maps of a sentinel species, sprat, in the Adriatic Sea in line with the good practice in SDMs (Araùjo et al., 2019), unlike previous studies conducted on sprat at a regional scale (Voss et al., 2008; De Felice et al., 2021). One of the main advantages of this approach is the characterization of model uncertainty. Along with the modeling technique, the main strength of this study comprises the amount and quality of presence/absence data employed to train and test the model. For this purpose, we used two decades of data collected during acoustic surveys in the Adriatic Sea (Leonori et al., 2021). These comprise a higher number of presence values at the local scale compared to the publicly available dataset used by Schickele et al. (2021) to forecast sprat distribution in the Mediterranean and the Atlantic seas. The use of a fishery-independent dataset may reduce the uncertainty due to the fishery behavior and make available a real absence dataset instead of pseudo-absence data which are usually applied in basin scale studies and may affect model results (Pennino et al., 2016; Schickele et al., 2021). We obtained good to high performance and accuracy of the single models and the ensemble under current and future scenarios (see Table 3), which validates the robustness of the data. As demonstrated by other authors, machine learning methods resulted in being the most accurate ones (Quinci et al., 2022). Conversely, we obtained a similar evaluation score between regression models and machine learning methods, which means that both approaches are able to provide good predictions of sprat-habitat suitability (Čengić et al., 2020).
Nevertheless, some bias may persist due to the consistency between present and future environmental data and variables selection. The delta model bias correction approach is considered one of the best methods for bias correction of coastal data, dealing with any possible shift in scale between climate models and current environmental variables (Hare et al., 2012). However, the choice of bias correction method could influence the spatial and temporal variability of the future projection (Pozo Buil et al., 2023). The nine environmental parameters considered in the present study may not adequately explain the whole variability in sprat-habitat suitability since other authors demonstrated the influence of other parameters such as currents, distance from the coast, wind, and cloud coverage (Petereit et al., 2008; Voss et al., 2008; Frisk et al., 2015; Tsikliras et al., 2019). However, we ended up with seven sets of parameters composed of up to four environmental variables and final ensemble models with three main explanatory variables, which explained over 40% of the model’s deviance according to GAM and GLM.
CHL was assessed as the main driver of sprat distribution, as suggested for other clupeid species (Fernández-Corredor et al., 2021). Primary production has seldom been linked to sprat presence. Nevertheless, De Felice et al. (2021) demonstrated a significant positive correlation in the Gulf of Lion as well as for SLA in the Adriatic Sea, in contrast to this work, where SLA did not show any significant influence on sprat distribution. This strong positive correlation may be mostly attributable to the diet of sprat, which consists primarily of copepods that are particularly abundant when CHL concentration levels are high (Tičina et al., 2000; Chust et al., 2014). Therefore, a clear link through food web between primary producers (CHL as a proxy of phytoplankton abundance) and secondary producers (zooplankton) as sprat’s prey and sprats is an important factor in sprat’s habitat suitability. SST, as expected, is the second key factor in determining sprat population dynamics since it is a cold-favoring species (Petereit et al., 2008; Peck et al., 2012). SST is an important factor affecting the main pelagic fish species worldwide (Lanz et al., 2009; Giannoulaki et al., 2013; Ebango et al., 2020). It affects the sprat adult growth rate and fecundity, as well as the rate of larval development and survival (Peck et al., 2012; Frisk et al., 2015). Our findings indicate an optimal thermal niche between 23°C and 24°C in early summer, which decreases to less than 22°C in late summer. Depth is the third factor that affects sprat-habitat suitability. Our results indicate that sprat are more likely to be found at depths between 35m and 65 m but not deeper than 125 m, as already demonstrated by other studies (Dänhardt et al., 2007; Voss et al., 2008). The importance of depth in determining the sprat’s habitat suitability might be related to the cooler and more dense water mass present in deeper areas, below the thermocline, and eventually to the species’ feeding behavior, which involves feeding on zooplankton and small crustaceans found in the mid-water layers (Tičina et al., 2000). In fact, the most northern part of the Adriatic Sea is an area where North Adriatic Dense Water (NAddW), the densest Mediterranean water, is generated by extreme surface layer cooling by winter cold winds, eventually driving the thermohaline circulation, ventilating the deep layers, and changing the biogeochemical properties of the Adriatic Sea (Pranić et al., 2024).
The habitat suitability maps presented here indicated that the sprat distribution area is concentrated in the northern Adriatic Sea, while the suitability decreased toward the southern regions. Moreover, our investigation reveals a west-east migration from the shallow feeding area near the Po delta in June and July to deeper waters in September for the reproductive season, where a further drop in temperature during the winter months will determine the start of the spawning season for the species in the Adriatic Sea (Dulčić, 1998). The temperature shift could be responsible for the migration from coastal to offshore and deeper and colder waters (see Supplementary Figures S7, S9) that we recorded in Figure 5. The specific cyclonic circulation of the Adriatic Sea, influenced by river inflow and strong seasonal winds, could lead to a breakdown of the thermocline and mixing of the water layers in the late summer (Raicevich et al., 2015). The unique characteristics of the Adriatic Sea and the studied period could also justify the nonsignificant influence of salinity and water circulation dynamics considered in this study through SSS and SLA, unlike other studies conducted in the Mediterranean Sea and the Baltic Sea (Voss et al., 2008; Tsikliras et al., 2019).
The Mediterranean Sea is one of the most threatened basins in the world due to multiple anthropogenic pressures and its specific characteristics (Azzurro et al., 2022). It is characterized by high biodiversity and highly variable oceanographic conditions, but it is also the fastest-warming marine region in the world (Pastor et al., 2020). It is well known that abiotic changes in marine ecosystems, driven by climate changes, affect the spatial distribution of marine fauna, as demonstrated by Andrews et al. (2019) in the Barents Sea and by Ouled-Cheikh et al. (2022) in the Mediterranean Sea. Since the Adriatic Sea is a semi-enclosed basin located in the most northern part of the Mediterranean Sea, the boreal pelagic species, which are migratory and capable of covering vast distances, are unable to escape northward toward suboptimal conditions due to the semi-enclosed nature of the basin, driven by global warming. This leads to a complex orography characterized by a widely extended continental shelf with a predominant depth of 50 m in the northern region (Russo and Artegiani, 1996). These conditions expose, even more, the Adriatic Sea to the negative effects of climate change (Denamiel et al., 2020), which might be a climatic “dead end” for the Mediterranean boreal marine species if global warming continues (Ben Rais Lasram et al., 2010). Along with overfishing, this threatens the Mediterranean Sea’s most productive region (FAO, 2023).
The application of SDMs provides the possibility to assess population dynamics along the time series and under future scenarios, which can be particularly valuable as part of EBM’s purpose (O’Higgins et al., 2020). We found a noticeable contraction in the extent of the sprat-habitat suitability map under high SST and low CHL, such as in 2018, which was the warmest month of the time series, especially in late summer. Conversely, we detected an expansion of sprat-suitable areas during colder years such as 2007, 2010, and 2013 (see Supplementary Figures S6–S9). These findings are in accordance with the sprat’s biology and ecology, considering that the sprat is a boreal species favoring cooler habitats.
We argue that the sprat population in the Adriatic Sea is likely to be negatively impacted by the temperature increase due to global warming. An increase in seawater temperature could negatively affect the spawning success of boreal species (Peck et al., 2012; Frisk et al., 2015). The habitat suitability projections presented in the results highlight a severe decline in suitable areas for sprats under increasing carbon dioxide emission scenario 8.5. We detected a continuous decline during early summer from short- to long-term projections, which led to an almost extinction of suitable areas between 2079 and 2099, while during the late summer, we foresaw a reduction of almost 50%. The projections under the “stabilization” scenario 4.5 in late summer reveal a general shift from coastal warm-temperature waters to deeper and colder waters, underlined by a gain of new habitat in the period between 2029 and 2074. Nevertheless, albeit minor compared to RCP 8.5 projections, we observed a strong shrinkage of sprat-habitat suitability in both seasons for a long-term period, as shown in Figure 7. Climate change is affecting fish stocks around the world, and the recently observed poleward shift in cold-favoring species is leading to a change in the pelagic communities’ composition in favor of warmer water species (Cheung et al., 2013; Kanamori et al., 2019). The northward spread of sprat has already been foreseen in the Baltic and North-Atlantic Oceans due to an increase in SST, unlike in the Adriatic and the Mediterranean Sea, where the physical boundaries will prevent the northward spreading of the species (Schickele et al., 2021). The reduction in the sprat’s suitable habitat area found in our simulations is likely to have negative effects on future recruitments of the sprat population and significant ecological and economic consequences in the Adriatic Sea, since the sprat is an important forage species in the pelagic ecosystem for many predators, including seabirds and commercially important pelagic fish such as swordfish, tuna, and mackerel (Fasola et al., 1989; Knudsen et al., 2009; Navarro et al., 2017). The gradual decline in sprat biomass is therefore likely to have cascading effects throughout the ecosystem, both in terms of bottom-up control of predators and top-down control of zooplankton abundance. Consequently, other small pelagic species, such as anchovy in the Mediterranean Sea, may be more suited to forthcoming local conditions, competing with S. sprattus for the same ecological niche, being less affected by climate change (Bourg et al., 2015; Schickele et al., 2021). The trophodynamic role of sprat in the Adriatic Sea may be gradually replaced by the warm-temperate Clupeid species round sardinella, Sardinella aurita, which is already spreading its area of distribution northward, from the south-central Mediterranean Sea to the north Adriatic Sea, where its presence is currently considered uncommon (Schickele et al., 2021).
The results of this study provide valuable insights into the potential impacts of climate change on the distribution of sprat in the Adriatic Sea. The study revealed that currently, sprat is mostly found in the shallow northern area of the basin, where its distribution and movements are primarily related to chlorophyll-a concentration and sea surface temperature. Our analysis revealed that the suitability of the sprat’s habitat in the Adriatic Sea is very vulnerable to climate change, suggesting that if global warming continues, the species may disappear from the Adriatic region by 2099, particularly under the IPCC RCP scenario 8.5. This result is of great concern, as the sprat is an important forage species within the Adriatic Sea’s food web, and its disappearance could have far-reaching implications for the marine ecosystem in the region. While SDMs have been extensively used to map small pelagic fish habitats under current environmental conditions (Bellido et al., 2008; Giannoulaki et al., 2013; Milisenda et al., 2018), only recently the potential of SDMs to predict species distribution under future scenarios has been explored (Schickele et al., 2021). We should encourage further exploration of SDMs for predicting future habitat suitability maps of pelagic fish species in the Mediterranean Sea. This should be supported by additional studies using ecosystem models, helping to foresee the impacts of shifts in the distribution of small pelagic fish species on the entire ecosystem. Future research on changes in the Adriatic ecosystem caused by climate change, with an emphasis on studies of changes in pelagic fish assemblages, is likely to be crucial for future fishery resource management in this area.
The raw data supporting the conclusions of this article will be made available by the authors, without undue reservation.
The requirement of ethical approval was waived by EU DG MARE Maritime Affairs and Fisheries for the studies involving animals, due to fish specimens’ collection being authorized by the MEDIAS project as part of annual research surveys under the EU Fisheries Data Collection Framework (EC 665/2008), all involving lethal sampling. The studies were conducted in accordance with the local legislation and institutional requirements.
AP: Conceptualization, Formal analysis, Investigation, Methodology, Visualization, Writing – original draft, Writing – review & editing. AF: Conceptualization, Data curation, Supervision, Validation, Writing – review & editing. GC: Investigation, Visualization, Writing – review & editing. IB: Data curation, Investigation, Visualization, Writing – review & editing. IC: Data curation, Investigation, Visualization, Writing – review & editing. MC: Data curation, Investigation, Visualization, Writing – review & editing. SM: Data curation, Investigation, Methodology, Visualization, Writing – review & editing. DG: Data curation, Investigation, Visualization, Writing – review & editing. VT: Data curation, Investigation, Project administration, Supervision, Writing – review & editing. IL: Conceptualization, Data curation, Project administration, Supervision, Validation, Writing – review & editing.
The author(s) declare financial support was received for the research, authorship, and/or publication of this article. Data used in this study were collected in the framework of the acoustic surveys financed by Croatian and Italian National Fisheries directorates—Ministries of Agriculture and supported by the EU-DCF program, as well as supported by the Croatian Ministries of Science and Education through the institutional funding program (Project RIBAR). The work has been founded by the Croatian Ministry of Agriculture and Ministry of Science and Education (Grant No. 001-0013077-0532) and by the MEDIAS research project in the framework of the EC - MIPAAF Italian National Fisheries Data Collection Programs.
The authors are also grateful to all the participants involved in the acoustic surveys and to the captains and crews of the research vessels.
The authors declare that the research was conducted in the absence of any commercial or financial relationships that could be construed as a potential conflict of interest.
All claims expressed in this article are solely those of the authors and do not necessarily represent those of their affiliated organizations, or those of the publisher, the editors and the reviewers. Any product that may be evaluated in this article, or claim that may be made by its manufacturer, is not guaranteed or endorsed by the publisher.
The Supplementary Material for this article can be found online at: https://www.frontiersin.org/articles/10.3389/fmars.2024.1383063/full#supplementary-material
Albo-Puigserver M., Navarro J., Coll M., Layman C. A., Palomera I. (2016). Trophic structure of pelagic species in the northwestern Mediterranean Sea. J. Sea. Res. 117, 27–35. doi: 10.1016/j.seares.2016.09.003
Andrews A. J., Christiansen J. S., Bhat S., Lynghammar A., Westgaard J. Y., Christophe Pampoulie C., et al. (2019). Boreal marine fauna from the Barents Sea disperse to Arctic Northeast Greenland. Sci. Rep. 9, 5799. doi: 10.1038/s41598-019-42097-x
Angelini S., Armelloni E. N., Costantini I., De Felice A., Isajlović I., Leonori I., et al. (2021). Understanding the dynamics of ancillary pelagic species in the Adriatic sea. Front. Mar. Sci. 8. doi: 10.3389/fmars.2021.728948
Araùjo M. B., Anderson R. P., Màrcia Barbosa A., Beale C. M., Dormann C. F., Early R., et al. (2019). Standards for distribution models in biodiversity assess-ments. Sci. Adv. 5, eaat4858. doi: 10.1126/sciadv.aat4858
Azzurro E., Ballerini T., Antoniadou C., Aversa G. D., Souissi J. B., Blašković A., et al. (2022). ClimateFish: A collaborative database to track the abundance of selected coastal fish species as candidate indicators of climate change in the Mediterranean sea. Front. Mar. Sci. 9. doi: 10.3389/fmars.2022.910887
Bellido J. M., Brown M. B., Valavanis V. D., Giraldez A., Pierce G. J., Iglesias M., et al. (2008). Identifying essential fish habitat for small pelagic species in Spanish Mediterranean waters. Hydrobiologia 612, 171–184. doi: 10.1007/s10750-008-9481-2
Ben Rais Lasram F., Guilhaumon F., Albouy C., Somot S., Thuiller W., Mouillot D. (2010). The Mediterranean Sea as a ‘cul-de-sac’ for endemic fishes facing climate change. Glob. Change Biol. 16, 3233–3245. doi: 10.1111/j.1365-2486.2010.02224.x
Bonanno A., Barra M., Basilone G., Genovese S., Rumolo P., Goncharov S., et al. (2016). Environmental processes driving anchovy and sardine distribution in a highly variable environment: the role of the coastal structure and riverine input. Fish. Oceanogr. 25, 471–490. doi: 10.1111/fog.12166
Bosch S., Tyberghein L., Deneudt K., Hernandez F., De Clerck O. (2018). In search of relevant predictors for marine species distribution modelling using the MarineSPEED benchmark dataset. Divers. Distrib. 24, 144–157. doi: 10.1111/ddi.12668
Bourg B., Le B. D., Saraux C., Nowaczyk A., Luherne E., Le Jadaud A., et al. (2015). Trophic niche overlap of sprat and commercial small pelagic teleosts in the Gulf of Lions ( NWMediterranean Sea ) Trophic niche overlap of sprat and commercial small pelagic teleosts in the Gulf of Lions ( NW Mediterranean Sea ). J. Sea. Res. 103, 138–146. doi: 10.1016/j.seares.2015.06.011
Brander K. (2010). Impacts of climate change on fisheries. J. Mar. Syst. 79, 389–402. doi: 10.1016/j.jmarsys.2008.12.015
Čengić M., Rost J., Remenska D., Janse J. H., Huijbregts M. A. J., Schipper A. M. (2020). On the importance of predictor choice, modelling technique, and number of pseudo-absences for bioclimatic envelope model performance. Ecol. Evol. 10, 12307–12317. doi: 10.1002/ece3.6859
Cheung W. W. L., Lam V. W. Y., Sarmiento J. L., Kearney K., Watson R., Pauly D. (2009). Projecting global marine biodiversity impacts under climate change scenarios. Fish. Fish. 10, 235–251. doi: 10.1111/j.1467-2979.2008.00315.x
Cheung W. W. L., Watson R., Pauly D. (2013). Signature of ocean warming in global fisheries catch. Nature 497, 365–368. doi: 10.1038/nature12156
Chust G., Albaina A., Aranburu A., Borja Á., Diekmann O. E., Estonba A., et al. (2013). Connectivity, neutral theories and the assessment of species vulnerability to global change in temperate estuaries. Estuar. Coast. Shelf. Sci. 131, 52–63. doi: 10.1016/j.ecss.2013.08.005
Chust G., Allen J. I., Bopp L., Schrum C., Holt J., Tsiaras K., et al. (2014). Biomass changes and trophic amplification of plankton in a warmer ocean. Glob. Change Biol. 20, 2124–2139. doi: 10.1111/gcb.12562
Clementi E., Aydogdu A., Goglio A. C., Pistoia J., Escudier R., Drudi M., et al. (2021) Mediterranean Sea Physical Analysis and Forecast (CMEMS MED-Currents, EAS6 system) (Copernicus Monitoring Environment Marine Service (CMEMS) (Accessed 08/2023).
Conrad O., Bechtel B., Bock M., Dietrich H., Fischer E., Gerlitz L., et al. (2015). System for automated geoscientific analyses (SAGA) v. 2.1.4. Geosci. Model. Dev. 8, 1991–2007. doi: 10.5194/gmd-8-1991-2015
Cossarini G., Feudale L., Teruzzi A., Bolzon G., Coidessa G., Solidoro C., et al. (2021). High-resolution reanalysis of the Mediterranean Sea biogeochemistry, (1999-2019). Front. Mar. Sci. Front. Mar. Sci. 8. doi: 10.3389/fmars.2021.741486
Dänhardt A., Peck M. A., Clemmesen C., Temming A. (2007). Depth-dependent nutritional condition of sprat Sprattus sprattus larvae in the central Bornholm Basin, Baltic Sea. Mar. Ecol. Prog. Ser. 341, 217–228. doi: 10.3354/meps341217
De Felice A., Iglesias M., Saraux C., Bonanno A., Ticina V., Leonori I., et al. (2021). Environmental drivers influencing the abundance of round sardinella (Sardinella aurita) and European sprat (Sprattus sprattus) in different areas of the Mediterranean Sea. Mediterr. Mar. Sci. 22, 812–826. doi: 10.12681/mms.25933
Denamiel C., Pranić P., Quentin F., Mihanović H., Vilibić I. (2020). Pseudo-global warming projections of extreme wave storms in complex coastal regions: the case of the Adriatic Sea. Clim. Dyn. 55, 2483–2509. doi: 10.1007/s00382-020-05397-x
Dulčić J. (1998). Larval growth of sprat, Sprattus sprattus phalericus, larvae in the Northern Adriatic. Fish. Res. 36, 117–126. doi: 10.1016/S0165-7836(98)00108-8
Dulvy N. K., Sadovy Y., Reynolds J. D. (2003). Extinction vulnerability in marine populations. Fish. Fish 4, 25–64. doi: 10.1046/j.1467-2979.2003.00105.x
Ebango N. N., Song L., Cui H., Xu S. (2020). Relationship between the spatiotemporal distribution of dominant small pelagic fishes and environmental factors in Mauritanian waters. J. Ocean. Univ. China 19, 393–408. doi: 10.1007/s11802-020-4120-2
Effrosynidis D., Tsikliras A., Arampatzis A., Sylaios G. (2020). Species distribution modelling via feature engineering and machine learning for pelagic fishes in the mediterranean sea. Appl. Sci. 10, 1–23. doi: 10.3390/app10248900
Escudier R., Clementi E., Omar M., Cipollone A., Pistoia J., Aydogdu A., et al. (2020) Mediterranean Sea Physical Reanalysis (CMEMS MED-Currents) (Version 1) (Copernicus Monitoring Environment Marine Service (CMEMS) (Accessed 08/2023).
Fanelli E., Da Ros Z., Menicucci S., Malavolti S., Biagiotti I., Canduci G., et al. (2023). The pelagic food web of the Western Adriatic Sea: a focus on the role of small pelagics. Sci. Rep. 13, 14554. doi: 10.1038/s41598-023-40665-w
FAO (2023). The State of Mediterranean and Black Sea Fisheries 2023-Special Edition (Rome: General Fisheries Commission for the Mediterranean). doi: 10.4060/cc3370en
Fasola M., Bogliani G., Saino N., Canova L. (1989). Foraging, feeding and time-activity niches of eight species of breeding seabirds in the coastal wetlands of the Adriatic Sea. Bolletino di Zool. 56, 61–72. doi: 10.1080/11250008909355623
Fernández-Corredor E., Albo-Puigserver M., Pennino M. G., Bellido J. M., Coll M. (2021). Influence of environmental factors on different life stages of European anchovy (Engraulis encrasicolus) and European sardine (Sardina pilchardus) from the Mediterranean Sea: A literature review. Reg. Stud. Mar. Sci. 41, 101606. doi: 10.1016/j.rsma.2020.101606
Fielding A. H., Bell J. F. (1997). A review of methods for the assessment of prediction errors in conservation presence/absence models. Environ. Conserv. 24, 38–49. doi: 10.1017/S0376892997000088
Frisk C., Andersen K. H., Temming A., Herrmann J. P., Madsen K. S., Kraus G. (2015). Environmental effects on sprat (Sprattus sprattus) physiology and growth at the distribution frontier: A bioenergetic modelling approach. Ecol. Modell. 299, 130–139. doi: 10.1016/j.ecolmodel.2014.11.026
Giani M., Djakovac T., Degobbis D., Cozzi S., Solidoro C., Umani S. F. (2012). Recent changes in the marine ecosystems of the northern Adriatic Sea. Estuar. Coast. Shelf. Sci. 115, 1–13. doi: 10.1016/j.ecss.2012.08.023
Giannoulaki M., Iglesias M., Tugores M. P., Bonanno A., Patti B., De Felice A., et al. (2013). Characterizing the potential habitat of European anchovy Engraulis encrasicolus in the Mediterranean Sea, at different life stages. Fish. Oceanogr. 22, 69–89. doi: 10.1111/fog.12005
Giannoulaki M., Pyrounaki M. M., Bourdeix J.-H., Ben Abdallah L., Bonanno A., Basilone G. (2017). Habitat suitability modeling to identify the potential nursery grounds of the Atlantic mackerel and its relation to oceanographic conditions in the Mediterranean sea. Front. Mar. Sci. 4. doi: 10.3389/fmars.2017.00230
Giannoulaki M., Pyrounaki M., Liorzou B., Leonori I., Valavanis V., Tsagarakis K., et al. (2011). Habitat suitability modelling for sardine juveniles (Sardina pilchardus) in the Mediterranean Sea. Fish. Oceanogr. 20, 367–382. doi: 10.1111/fog.2011.20.issue-5
Gomez J. J., Cassini M. H. (2015). Environmental predictors of habitat suitability and biogeographical range of Franciscana dolphins (Pontoporia blainvillei). Glob. Ecol. Conserv. 3, 90–99. doi: 10.1016/j.gecco.2014.11.007
Guisan A., Thuiller W. (2005). Predicting species distribution: Offering more than simple habitat models. Ecol. Lett. 8, 993–1009. doi: 10.1111/j.1461-0248.2005.00792.x
Guisan A., Zimmermann N. E. (2000). Predictive habitat distribution models in ecology. Ecol. Modell. 135, 147–186. doi: 10.1016/S0304-3800(00)00354-9
Hare J. A., Wuenschel M. J., Kimball M. E. (2012). Projecting Range Limits with Coupled Thermal Tolerance - Climate Change Models: An Example Based on Gray Snapper (Lutjanus griseus) along the U.S. East Coast. PloS One 7, e52294. doi: 10.1371/journal.pone.0052294
Hernandez P. A., Graham C. H., Master L. L., Albert D. L. (2006). The effect of sample size and species characteristics on performance of different species distribution modeling methods. Ecography 29, 773–785. doi: 10.1111/j.0906-7590.2006.04700.x
Hosmer D. W., Lemeshow S., Sturdivant R. X. (2013). Applied Logistic Regression. 3rd ed (NJ, USA: John Wiley & Sons: Hoboken). doi: 10.1002/SERIES1345
Kanamori Y., Takasuka A., Nishijima S., Okamura H. (2019). Climate change shifts the spawning ground northward and extends the spawning period of chub mackerel in the western North Pacific. Mar. Ecol. Prog. Ser. 624, 155–166. doi: 10.3354/meps13037
Kay S. (2020) Marine biogeochemistry data for the Northwest European Shelf and Mediterranean Sea from 2006 up to 2100 derived from climate projections (Copernicus Climate Change Service (C3S) Climate Data Store (CDS) (Accessed 08-2023).
Knudsen F. R., Hawkins A., McAllen R., Sand O. (2009). Diel interactions between sprat and mackerel in a marine lough and their effects upon acoustic measurements of fish abundance. Fish. Res. 100, 140–147. doi: 10.1016/j.fishres.2009.06.015
Lanz E., López-Martínez J., Nevárez-Martínez M., Dworak J. A. (2009). Small pelagic fish catches in the Gulf of California associated with sea surface temperature and chlorophyll. Calif Coop Ocean Fish Investig. Rep. 50, 134–146.
Lauria V., Gristina M., Attrill M. J., Fiorentino F., Garofalo G. (2015). Predictive habitat suitability models to aid conservation of elasmobranch diversity in the central Mediterranean Sea. Sci. Rep. 5, 1–16. doi: 10.1038/srep13245
Leonori I., Tičina V., Giannoulaki M., Hattab T., Iglesias M., Bonanno A., et al. (2021). History of hydroacoustic surveys of small pelagic fish species in the European Mediterranean Sea. Mediterr. Mar. Sci. 22, 751–768. doi: 10.12681/mms.26001
Marmion M., Parviainen M., Luoto M., Heikkinen R. K., Thuiller W. (2009). Evaluation of consensus methods in predictive species distribution modelling. Div. Dist. 12, 59–69. doi: 10.1111/j.1472-4642.2008.00491.x
Marra G., Wood S. N. (2011). Practical variable selection for generalized additive models. Comput. Stat. Data. Anal. 55, 2372–2387. doi: 10.1016/j.csda.2011.02.004
MEDIAS (2021) MEDIAS HANDBOOK Common protocol for the MEDiterranean International Acoustic Survey (MEDIAS). Available at: http://www.medias-project.eu/medias/website/.
Milisenda G., Garofalo G., Fezzani S., Rjeibi O., Jarboui O., Chemman B., et al. (2018). Biomass HotSpot distribution model and spatial interaction of two exploited species of horse mackerel in the south- central Mediterranean Sea. Hydrobiologia 821, 135–150. doi: 10.1007/s10750-017-3378-x
Navarro J., Sáez-Liante R., Albo-Puigserver M., Coll M., Palomera I. (2017). Feeding strategies and ecological roles of three predatory pelagic fish in the western Mediterranean Sea. Deep Res. Part. II Top. Stud. Oceanogr. 140, 9–17. doi: 10.1016/j.dsr2.2016.06.009
O’Higgins T. G., Lago M., DeWitt T. H. (2020). Ecosystem-Based Management, Ecosystem Services and Aquatic Biodiversity Theory, Tools and Applications (Oregon, USA: US Environmental Protection Agency Newport). doi: 10.1007/978-3-030-45843-0
Ojaveer E., Kalejs M. (2010). Ecology and long-term forecasting of sprat (Sprattus sprattus balticus) stock in the Baltic Sea: A review. Rev. Fish. Biol. Fish. 20, 203–217. doi: 10.1007/s11160-009-9130-5
Ouled-Cheikh J., Coll M., Cardona L., Steenbeek J., Ramírez F. (2022). Fisheries-enhanced pressure on Mediterranean regions and pelagic species already impacted by climate change. Elementa: Sci. Anthropocene 10, 00028. doi: 10.1525/elementa.2022.00028
Pastor F., Valiente J. A., Khodayar S. (2020). A warming Mediterranean: 38 years of increasing sea surface temperature. Remote Sens 12, 1–16. doi: 10.3390/rs12172687
Peck M. A., Baumann H., Bernreuther M., Clemmesen C., Herrmann J. P., Haslob H., et al. (2012). Reprint of: The ecophysiology of Sprattus sprattus in the Baltic and North Seas. Prog. Oceanogr. 107, 31–46. doi: 10.1016/j.pocean.2012.10.009
Pennino M. G., Conesa D., Lo´pez-Quı´lez A., Mun˜oz F., Ferna´ndez A., Bellido J. M. (2016). Fishery-dependent and -independent data lead to consistent estimations of essential habitats. ICES J. Mar. Sci. 73, 2302–2310. doi: 10.1093/icesjms/fsw062
Petereit C., Haslob H., Kraus G., Clemmesen C. (2008). The influence of temperature on the development of Baltic Sea sprat (Sprattus sprattus) eggs and yolk sac larvae. Mar. Biol. 154, 295–306. doi: 10.1007/s00227-008-0923-1
Piccinetti C., Vrgoč N., Marčeta B., Manfredi C. (2012). Recent state of demersal resource in the Adriatic Sea (Croatia: Institute of Oceanography and Fisheries Split).
Pozo Buil M., Fiechter J., Jacox M. G., Bograd S. J., Alexander M. A. (2023). Evaluation of different bias correction methods for dynamical downscaled future projections of the California current upwelling system. Earth Space Sci. 10, e2023EA003121. doi: 10.1029/2023EA003121
Pranić P., Denamiel C., Vilibić I. (2024). Kilometre-scale assessment of the adriatic dense water multi-decadal dynamics. ESS Open Archive. doi: 10.22541/essoar.171284509.97697750/v1
Quinci E. M., Torri M., Cuttitta A., Patti B. (2022). Predicting potential spawning habitat by ensemble species distribution models: the case study of European anchovy (Engraulis encrasicolus) in the strait of Sicily. Water (Switzerland), 14, 1400. doi: 10.3390/w14091400
Raicevich S., Bullo M., Sabatini L. (2015). Un futuro per la pesca in Alto Adriatico. Risultati e proposte del percorso partecipativo GAP2 tra ricercatori e pescatori di Chioggia. Ed. Giovanardi O. (Roma: ISPRA, Quaderni - Ricerca Marine), 112.
Robinson N. M., Nelson W. A., Costello M. J., Sutherland J. E., Lundquist C. J. (2017). A systematic review of marine-based species distribution models (SDMs) with recommendations for best practice. Front. Mar. Sci. 4. doi: 10.3389/fmars.2017.00421
Sbrocco E. J., Barber P. H. (2013). MARSPEC: Ocean climate layers for marine spatial ecology. Ecology 94, 979. doi: 10.1890/12-1358.1
Schickele A., Goberville E., Leroy B., Beaugrand G., Hattab T., Francour P., et al. (2021). European small pelagic fish distribution under global change scenarios. Fish. Fish. 22, 212–225. doi: 10.1111/faf.12515
Shaltout M., Omstedt A. (2014). Recent sea surface temperature trends and future scenarios for the Mediterranean Sea. Oceanologia 56, 411–443. doi: 10.5697/oc.56-3.411
Somodi I., Lepesi N., Botta-Dukát Z. (2017). Prevalence dependence in model goodness measures with special emphasis on true skill statistics. Ecol. Evol. 7, 863–872. doi: 10.1002/ece3.2654
Thuiller W., Georges D., Gueguen M., Engler R., Breiner F., Lafourcade B., et al. (2023). biomod2: Ensemble Platform for Species Distribution Modeling. R package version 4.2-5. doi: 10.32614/CRAN.package.biomod2
Tičina V., Vidjak O., Kačič I. (2000). Feeding of adult sprat, Sprattus sprattus, during spawning season in the adriatic sea. Ital. J. Zool. 67, 307–311. doi: 10.1080/11250000009356329
Tsikliras A. C., Licandro P., Pardalou A., McQuinn I. H., Gröger J. P., Alheit J. (2019). Synchronization of Mediterranean pelagic fish populations with the North Atlantic climate variability. Deep Res. Part II Top Stud. Oceanogr 159, 143–151. doi: 10.1016/j.dsr2.2018.07.005
Tugores P., Giannoulaki M., Iglesias M., Bonanno A., Tičina V., Leonori I., et al. (2011). Habitat suitability modelling for sardine Sardina pilchardus in a highly diverse ecosystem: The Mediterranean Sea. Mar. Ecol. Prog. Ser. 443, 181–205. doi: 10.3354/meps09366
Voss R., Dickmann M., Hinrichsen H. H., Floeter J. (2008). Environmental factors influencing larval sprat Sprattus sprattus feeding during spawning time in the Baltic Sea. Fish Oceanogr. 17, 219–230. doi: 10.1111/j.1365-2419.2008.00474.x
Wood S. N. (2017). Generalized Additive Models: An Introduction with R. 2nd ed. (New York: Chapman and Hall/CRC). doi: 10.1201/9781315370279
Keywords: climate change, European sprat, habitat suitability, species distribution modeling, Mediterranean Sea
Citation: Palermino A, De Felice A, Canduci G, Biagiotti I, Costantini I, Centurelli M, Menicucci S, Gašparević D, Tičina V and Leonori I (2024) Modeling of the habitat suitability of European sprat (Sprattus sprattus, L.) in the Adriatic Sea under several climate change scenarios. Front. Mar. Sci. 11:1383063. doi: 10.3389/fmars.2024.1383063
Received: 06 February 2024; Accepted: 03 June 2024;
Published: 21 June 2024.
Edited by:
Torstein Pedersen, UiT The Arctic University of Norway, NorwayReviewed by:
Rui Wang, State Oceanic Administration, ChinaCopyright © 2024 Palermino, De Felice, Canduci, Biagiotti, Costantini, Centurelli, Menicucci, Gašparević, Tičina and Leonori. This is an open-access article distributed under the terms of the Creative Commons Attribution License (CC BY). The use, distribution or reproduction in other forums is permitted, provided the original author(s) and the copyright owner(s) are credited and that the original publication in this journal is cited, in accordance with accepted academic practice. No use, distribution or reproduction is permitted which does not comply with these terms.
*Correspondence: Iole Leonori, aW9sZS5sZW9ub3JpQGNuci5pdA==
Disclaimer: All claims expressed in this article are solely those of the authors and do not necessarily represent those of their affiliated organizations, or those of the publisher, the editors and the reviewers. Any product that may be evaluated in this article or claim that may be made by its manufacturer is not guaranteed or endorsed by the publisher.
Research integrity at Frontiers
Learn more about the work of our research integrity team to safeguard the quality of each article we publish.