- 1Institute of Ocean and Atmospheric Science, Hanyang University, Ansan, Republic of Korea
- 2Oceanic Climate & Ecology Research Division, National Institute of Fisheries Science, Busan, Republic of Korea
- 3Deparment of Marine Science and Convergent Technology, Hanyang University, Ansan, Republic of Korea
- 4Estuarine and Coastal Ecology Laboratory, Department of Marine Life Sciences, Jeju National University, Jeju, Republic of Korea
Introduction: The carbon isotope ratios (δ13C) of essential amino acids (EAAs), including valine, leucine, isoleucine, threonine, and phenylalanine, in producers are crucial for explaining food-web structures in marine ecosystems. However, few studies have tested the variability of δ13C-EAA values in marine macrophytes, such as seagrass and macroalgae, under changing environmental conditions.
Methods: In this study, we examined the responses of δ13C-EAA values in macrophytes to environmental changes and explored their usefulness in characterizing macrophyte groups and local environments. We tested seagrass and macroalgae collected at different spatial and temporal scales in the field, as well as lab-cultured Ulva algae at various temperature gradients (12°C, 20°C, and 27.5°C) with additional nitrogen sources.
Results: We found that δ13C-EAA values in macroalgae were significantly altered by seasonality and the interactive effects of temperature and nitrogen addition in comparison with mean-centered δ13C-EAA values (normalized δ13C-EAA values relative to the mean of the overall EAAs). The δ13C-EAA values detected in macroalgae within a local environment correlated with those of a co-occurring grazer, Caprella. Based on mean-centered δ13C-EAA values, macrophyte groups were distinguishable from other group (i.e., the bacteria group) even under diverse environmental conditions. Moreover, the seagrass group did not overlap with the green and the brown macroalgal group, but overlapped considerably with the red macroalgal group.
Discussion: These results suggest that the macrophyte-specific mean-centered δ13C-EAA values may be fairly consistent across broad spatial and temporal scales. Despite significant variation in δ13C-EAA values, the consistency in mean-centered δ13C-EAA values among specific macrophyte groups provides valuable insight into the characteristics of local trophic bases in regions under pressure from dramatic environmental changes.
1 Introduction
Marine macrophytes such as macroalgae (D’archino and Piazzi, 2021) and seagrass (Stockbridge et al., 2020) provide habitat for other marine organisms. They also play crucial roles in regulating nutrient cycles, particularly those involving carbon and nitrogen, within marine environments. Macrophyte populations often need to adapt to various environmental stressors, such as land-use alterations, climate change, and eutrophication. These stressors disrupt the fundamental physiological processes of native macrophytes, affecting benthic communities and facilitating the proliferation of opportunistic and fast-growing species. Such changes can induce cascading effects on benthic food webs and species interactions (Lovelock et al., 2020; Carrier-Belleau et al., 2021; Velázquez-Ochoa et al., 2022). Due to their ecological importance and susceptibility to environmental change, researchers have monitored the physiological responses of these macrophytes for understanding the dynamics of marine ecosystems (D’archino and Piazzi, 2021; Liénart et al., 2022).
Stable isotope ratios within producers are signals of biochemical and ecological responses to environmental conditions (Deniro and Epstein, 1978; Dawson et al., 2002). Carbon isotope ratios (13C/12C or δ13C) in producers vary according to factors such as photosynthesis activity, carbon source availability (CO2 and HCO3-), pH, temperature, light, and availability of inorganic N and P (Cohen and Fong, 2006; Park et al., 2016; Kang et al., 2021; Velázquez-Ochoa et al., 2022). Due to their sensitivity to spatial and temporal environmental fluctuations, the isotopic composition of bulk tissues in producers is commonly utilized as isotopic baselines that reflect local environments. However, these isotope values are also influenced by additional factors, such as taxonomy distinctions, reproductive or growth stages, tissue types, and morphological characteristics of macrophytes (Kim et al., 2014; Stephens and Hepburn, 2014; Park et al., 2016; Velázquez-Ochoa et al., 2022). Previous studies have reported significant shifts of between 3% and 5‰ in bulk isotope values of macrophytes in situ, encompassing seagrasses (e.g., Kim et al., 2014; Park et al., 2016) and macroalgae (Stephens and Hepburn, 2014; Drobnitch et al., 2018; Zuñiga-Rios et al., 2021; Liénart et al., 2022), over times and in natural field conditions. Nevertheless, bulk isotope analyses can introduce uncertainty due to the substantial influence of both environmental disturbances and the physiological status of producers (e.g., Mancinelli and Vizzini, 2015; Casella et al., 2022). Consequently, although bulk isotope ratios can provide insights into physiological changes in macrophytes within changing environments, their application to understanding impacts on ecosystems has been limited.
Carbon stable isotope values of individual compounds, such as free and protein-bound amino acids (AAs) in producers, can be powerful tools for assessing physiological, biochemical, and ecological processes. Several proteinogenic AAs, including valine (Val), leucine (Leu), isoleucine (Ile), threonine (Thr), phenylalanine (Phe), and lysine (Lys), are synthesized only by autotrophs, not by consumers. Consumers cannot survive without these AAs, which are assimilated directly from the diet and are commonly called essential amino acids (EAAs). Consumer δ13C-EAA values do not differ significantly from the dietary EAAs of producers or prey (e.g., McMahon et al., 2011; reviewed in Whiteman et al., 2019; Yun et al., 2022), indicating (in)direct trophic relationships. Large differences in δ13C-EAA values have been used to describe the biomes of basal organism groups (e.g., primary producers and microorganisms) in diverse ecosystems, including terrestrial (e.g., Larsen et al., 2009; Pollierer et al., 2019), freshwater (e.g., Liew et al., 2019), sea-ice (e.g. Vane et al., 2023b), marine, and coupled environments (e.g. Larsen et al., 2013; McMahon et al., 2016; Takizawa et al., 2020; Eglite et al., 2023).
The δ13C-EAA values of producers, such as phytoplankton (Larsen et al., 2015; Stahl et al., 2023), vary according to environmental factors, such as the δ13C values of dissolved inorganic carbon, pH, salinity, temperature, light, and specific biosynthetic pathways during the synthesis and metabolism of EAAs. Adjusting the δ13C-EAA values to the mean of multiple δ13C-EAA values (expressed as mean-centered δ13C-EAA values in this study) or bulk δ13C values can help remove the baseline effects associated with local environments. Mean-centered δ13C-EAA values can vary among phylogenetically distinctive autotroph groups that presumably have their own specific biosynthetic pathways de novo (Larsen et al., 2009; Lynch et al., 2016; Besser et al., 2022). The unique signatures of mean-centered δ13C-EAA values can be used to discriminate among the basal organisms (Scott et al., 2006; Larsen et al., 2009, 2013; Besser et al., 2022). The mean-centered δ13C-EAA values have been frequently used to classify basal organisms such as plants, aquatic algae, fungi, and bacteria. However, fewer studies have systematically tested in marine primary producers whether mean-centered δ13C-EAA values remain consistent over broad temporal and spatial scales, such as yearly, seasonal, and environmental variations, in marine producers (Larsen et al., 2015 for microalgae; Elliott Smith et al., 2022 for macroalgae).
Previous studies have shown that mean-centered δ13C-EAA values can be used to effectively differentiate among macroalgal groups, including Phaeophyta (kelp), Rhodophyta and Chlorophyta/particulate organic matter (Larsen et al., 2013; Elliott Smith et al., 2021, 2022). Mean-centered δ13C-EAA values can also distinguish macroalgae from other macrophytes such as seagrass (Larsen et al., 2013). Elliott Smith et al. (2022) further expand upon this feature by demonstrating the utility of mean-centered δ13C-EAA values across varying geographic and temporal scales, using both historical and modern samples. However, whether mean-centered δ13C-EAA values reliably classify diverse macrophyte groups under ongoing environmental changes remains unclear, as these changes can affect the variability of δ13C-EAA values. The aim of this study was to deepen our understanding of the variation of δ13C-EAA values in macrophytes across different environmental conditions. We measured the variation of δ13C-EAA and mean-centered δ13C-EAA values in diverse macrophytes, ranging from members of Phaeophyta, Rhodophyta and Chlorophyta to seagrass, collected from the field at different sites and/or times, as well as in laboratory cultures (in particular, the macroalga Ulva), under different temperature gradients and nitrogen sources. We also assessed whether macroalgal δ13C-EAA values can indicate local environmental characteristics and trophic links with co-occurring grazers. Finally, we examined if mean-centered δ13C-EAA values can be used to distinguish among macrophyte groups amid environmental changes.
2 Material and methods
2.1 Sample collection in fields
Macrophyte samples were collected from three sites in two regions (Jeju Island and Busan) in South Korea (Figure 1). In the rocky subtidal site of Munseom Island (33° 13.583′ N, 126° 33.950′ E) south of Jeju Island, samples of a representative kelp, Ecklonia cava, were collected by scuba divers in four seasons (April, August, and November of 2014, and February of 2015). Munseom Island has been managed as a marine protected area and serves as a representative site for monitoring the effects of climate change at the ecosystem scale in Korea. By applying a hole-punch marking method to the blades of Ecklonia individuals (Yokohama et al., 1987; Bearham et al., 2013), newly grown blades were used for AA analysis. In Hamdeok, northern Jeju Island (33° 32.897′ N, 126° 39.336′ E), samples of free-floating Ulva species were handpicked in December of 2020 and February and December of 2021. Ulva ohnoi was the prevalent species at this site, where a large semi-enclosed tide pool with eutrophication attributable to high nitrate levels is present year-round. Ulva was originally a subtropical genus, but it has recently been introduced to temperate regions (Kang et al., 2019). Finally, three brown algae (Sargassum horneri, S. muticum, and S. thunbergii), four red algae (Polyopes affinis, Grateloupia cornea, Gelidium amansii, and Chondria crassicaulis), and one green alga (Ulva australis) were collected from the lower intertidal zone to the upper subtidal rocky shore in Busan (35° 02.673′ N, 128° 51.025′ E) in April 2020. Along with macroalgal sampling, seagrasses (Zostera marina and Z. japonicus) were also obtained from the subtidal and intertidal zones of the soft-bottom habitat in December 2018. Co-occurring consumers in the intertidal macroalgal assemblage were also sampled, including meso-sized caprellid amphipods (Caprella sp.), which are grazers and detritus feeders. All macrophyte samples were cleaned by removing epiphytes and epibenthos following visual examination and rinsed with distilled water. The samples were then freeze-dried, ground to a fine powder, and stored at −20°C until analysis.
2.2 Ulva culture: interactive temperature and additional N source treatment
We investigated the combined effects of temperature and added nitrogen (N) on the stable isotope ratios of the Ulva tissues. Before the experiment, the specimens were cleaned and pre-cultured for 5 days in filtered seawater at 12°C and exposed to 100 μmol photons m-2 s-1 under a photoperiod of 12 h light and 12 h dark. After acclimation, 1 g (fresh weight) of healthy samples was cultivated in 500-mL conical flasks. The samples were exposed to three different temperatures (12, 20, and 27.5°C) and two different nutrient levels (control: ambient seawater only, and added N: a mix of 100 µM NO3- and 100 µM NH4+) for 2 weeks. Three replicate test flasks were used for each treatment, for a total of 18 flasks. The culture media were replaced daily during. All other conditions were the same as mentioned above for the pre-culture.
2.3 Sample preparation for analyzing δ13C-EAA values
Reagent-grade standard AAs, including Val, Leu, Ile, Phe (Shoko Science Co., Ltd., Yokohama, Japan), Thr, Lys, alanine, glycine, serine, aspartic acid, proline, and glutamic acid (Sigma-Aldrich, St. Louis, MO, USA) were purchased and prepared at a concentration of 50 mM in 0.1 M HCl to create a standard AA mixture. Norleucine (Shoko Science, Japan) was also added as an internal standard. Reagent-grade acetyl chloride (Thermo Fisher Scientific, Lancashire, UK), acetic anhydride, and trimethylamine (Sigma-Aldrich, St. Louis, USA) were used in this study. Analytical-grade acetone, chloroform, hexane, and ethyl acetate were purchased from Merck KGaA (Darmstadt, Germany) and 12.1 M HCl (Junsei Chemical Co. Ltd., Tokyo, Japan).
To produce AAs suitable for gas chromatography (GC) analysis, we followed a protocol described by Corr et al. (2007) with slight modifications. In brief, approximately 10 mg of macrophyte samples were hydrolyzed with 1 mL of 6 N HCl at 110°C for 20 h to obtain an AA hydrolysate. The AA layer was separated from the lipophilic fractions by adding 2 mL of hexane-DCM (6:5, v/v). The AA hydrolysate layer was transferred, dried, and stored at −20°C. Next, the AA hydrolysate was methylated with 0.63 mL of acidified methanol solution (acetyl chloride: anhydrous methanol, 1:6 by volume) at 75°C for 1 h, and the reagents were dried under a N2 flow. Subsequently, the AA methyl esters were mixed with 0.75 mL of the acetylation mixture (acetic anhydride: trimethylamine: acetone, 1:5:9 by volume) at 60°C for 10 min, and the reagents were evaporated. The AA derivatives were purified by adding ethyl acetate and a saturated NaCl solution, and the top layer was transferred to a new vial. Finally, the ethyl acetate layer was concentrated to 300 μL under a gentle N2 stream.
2.4 Gas chromatography–isotope mass spectrometry analysis
The δ13C-EAA values were measured by gas chromatography-combustion-isotope ratio mass spectrometry at the Hanyang University Isotope Ecology and Environmental Science Laboratory (Ansan, Korea). This analytical platform consists of a gas chromatograph (Hewlett Packard 7890 N series, Agilent Technologies, Santa Clara, CA) with a GC5 combustion interface (Elementar, Germany) connected to an Isoprime isotope ratio mass spectrometer (Elementar, Germany). The AA derivatives were injected in splitless mode at 250°C and separated on a VF-35 capillary column (Agilent Technologies, Santa Clara, CA USA; 30 m × 0.32 mm internal diameter, 1-µm film thickness) at a constant flow rate of 1.2 mL/min following a set temperature program: 75°C for min), 135°C at 20°C min-1, 160°C at 5°C min-1 for 3 min, and 300°C at 8°C min-1 for 10 min. The separated AAs were completely oxidized to CO2 gas and introduced into the isotope ratio mass spectrometer. The samples were run in duplicate with the standard AA mixture.
The analytical precision of the standard AA mixture was < 0.5‰ of the averaged standard deviation (SD), ranging from 0.4‰ (for Phe) to 1.1‰ (for Ile). The δ13C values of each AA for every sample were corrected using a δ13C-certified AA standard to account for the non-analyte carbons from the derivative reagent incorporated into compounds of interest during derivatization (Corr et al., 2007).
We focused on analyzing the δ13C-EAA values for Val, Ile, Leu, Thr, Phe, and Lys (Supplementary Figure 1). If the δ13C data were not reliable (e.g., > 5‰ difference within treatment of Ulva culture, Lys), the data were not used for subsequent analyses. Mean-centered δ13C-EAA values (the δ13C differences between individual EAA and the mean of EAA variables) were also recorded.
2.5 Statistical analysis
The normality was tested in Q-Q plots, and the homogeneity of all data was checked using Levene’s test. If the assumptions were met, significant differences in δ13C-EAA and mean-centered δ13C-EAA values for the Ecklonia and Ulva macroalgae collected at different sampling times were tested using one-way analysis of variance (ANOVA), and differences in the seagrass Zostera from the two habitats (intertidal and subtidal) were tested with a t-test (p-value < 0.05). When the assumptions were not met for ANOVA, Welch’s ANOVA was performed as the equivalent of normal ANOVA. The effects of the temperature increase (three levels: 12, 20, and 27.5°C) and added N (two levels: added N and a control) on δ13C-EAA and mean-centered δ13C-EAA values were examined using two-way ANOVAs. If significant interaction effects were observed in the two-way ANOVAs, we performed separate one-way ANOVAs for the control and added N treatments as an alternative to post hoc tests. These were performed after applying a sequential Bonferroni adjustment to compensate for inflated significance levels due to the multiple comparisons made during analysis (Lee and Lee, 2018). Accordingly, a more conservative p-value of 0.05/k (i.e., k = 3, where k refers to the levels of the temperature gradient in this analysis, Rice, 1989) was chosen rather than 0.05. The significant differences between δ13C-EAA and mean-centered δ13C-EAA values among macroalgae and seagrasses with the meso-grazer Caprella were tested using one-way ANOVA. Principal component analysis (PCA) was also performed to assess the applicability of the δ13C-EAA values to characterization of local environments for macrophytes and co-occurring grazers. All statistical procedures were performed using JMP Pro v17.0 and Past4.03. Linear discriminant analysis (LDA) with δ13C-EAA and mean-centered δ13C-EAA values, respectively, were used to find a best separation among macrophytes groups (red, green and brown macrolage and seagrass). The LDA model with the isotope data set of macrophytes groups was used to predict source groups for consumer Caprella individuals.
Using mean-centered δ13C-EAA values, a LDA classification model was used to calculate the probability of group membership for the samples classified based on macrophytes obtained from this study and the published data (Larsen et al., 2009, 2013; Elliott Smith et al., 2021). Standard ellipse areas were plotted for the bivariate means of the groups in the LDA plot, with each ellipse enclosing approximately 50% of the data. To estimate the overlapping degree of pairing ellipses on the LDA transformed data (Vane et al., 2023a), areas of overlapping and pairing ellipses were calculated based on their posterior distribution using R statistical software version 4.3.1 (R Core Team, 2023) and the SIBER package (Jackson et al., 2011; Jackson, 2023). Given two distributions of an A group and a B group, we calculated the Bhattacharya coefficients (BCs, Bhattacharyya, 1946) as follows:
If the BC was close to 1, the distribution of Group A and Group B were similar, while if the BC was close to 0, the distribution of two groups were dissimilar.
3 Results
3.1 Environmental variation on δ13C-EAA values
3.1.1 Field-collected macrophytes
The average δ13C-EAA values in seagrass Zostera from the intertidal zone ranged from −7.9‰ to −23.2‰, and in the subtidal zone, they ranged from −7.2‰ to −21.0‰ (Figure 2A, Table 1). Neither δ13C-EAA nor mean-centered δ13C-EAA values differed significantly between the two sites. This indicates that the site had little effect on δ13C-EAA and mean-centered δ13C-EAA values (Figure 2A). For the perennial macroalga, the average δ13C-EAA value of Ecklonia collected in spring ranged from −4.1‰ (Thr) to −32.3‰ (Leu), and a similar isotopic range was also observed for other sampling times (Figure 2B, Table 1). At the level of individual EAAs in Ecklonia, the δ13C values of Leu and Ile in Ecklonia were, on average, 3.1‰ and 8.0‰ higher in summer than in winter (Figure 2B, Table 1, p < 0.01), although there was little significant effect of seasonal variation on the δ13C values of Val, Thr, Phe and Lys. The mean-centered δ13C-EAA values of Leu, Ile, Thr, and Lys in Ecklonia did differ, by an average 1.9‰ (Leu) and 6.5‰ (Ile) among the seasons (Table 1). For the other macroalga, Ulva, an ephemeral genus, there were no significant effects of temporal variations (Figure 2C), although only Val was slightly low in February 2021 compared with other sampling times (p = 0.05). Most mean-centered δ13C-EAA values in Ulva did not differ significantly among the seasons, and only Val was isotopically low in February 2021. This difference suggests that mean-centered δ13C-EAA values for Ecklonia and Ulva allowed for a slight reduction in the magnitude of variation at temporal scales.
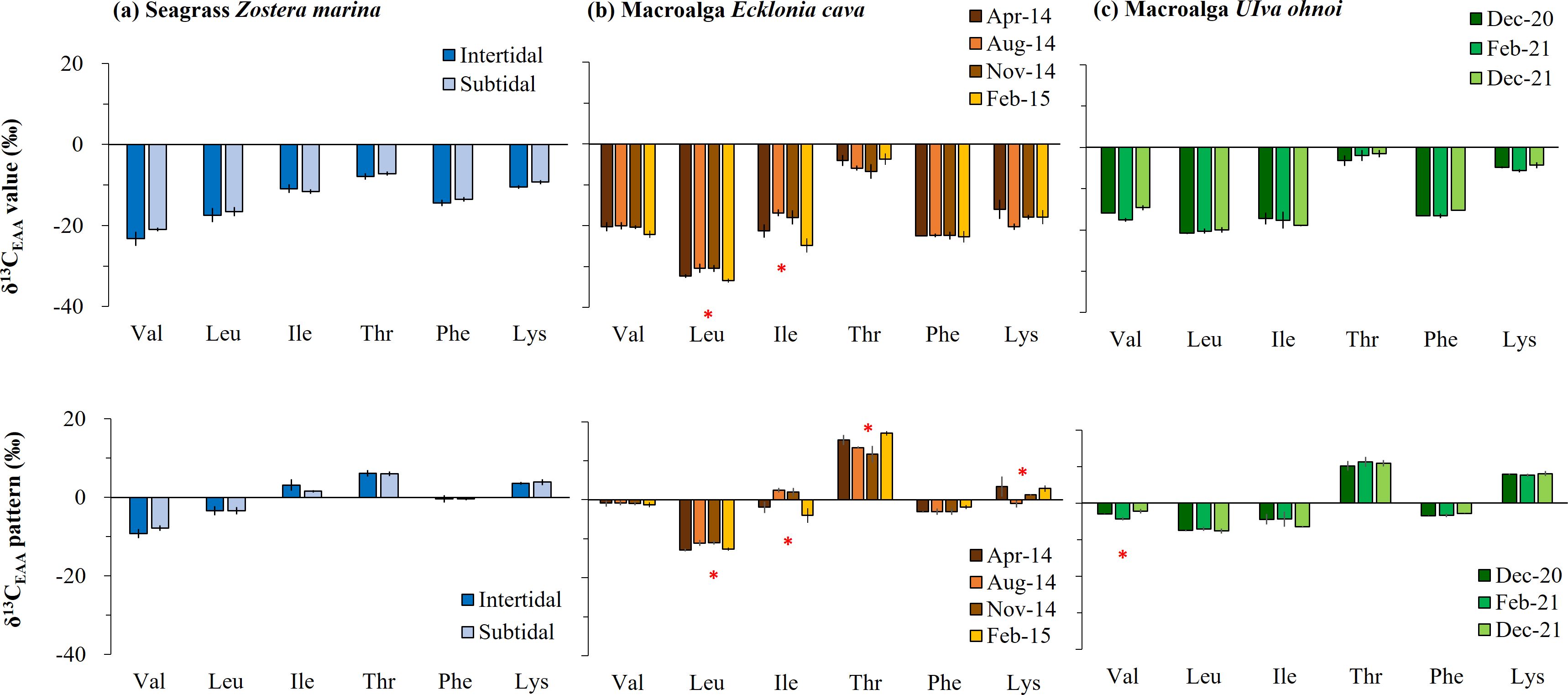
Figure 2. Temporal and spatial variation in essential amino acid (EAA) carbon stable isotope (δ13C) values for macrophytes collected in the field: (A) brown macroalga Ecklonia cava, (B) green macroalga Ulva ohnoi, and (C) seagrass Zostera marina. The δ13C-EAA values and mean-centered δ13C-EAA values (δ13C difference between individual EAA and the average of overall EAAs) are shown and represented by the mean ± SD (N = 3). Significant differences are marked by an asterisk above the bars.
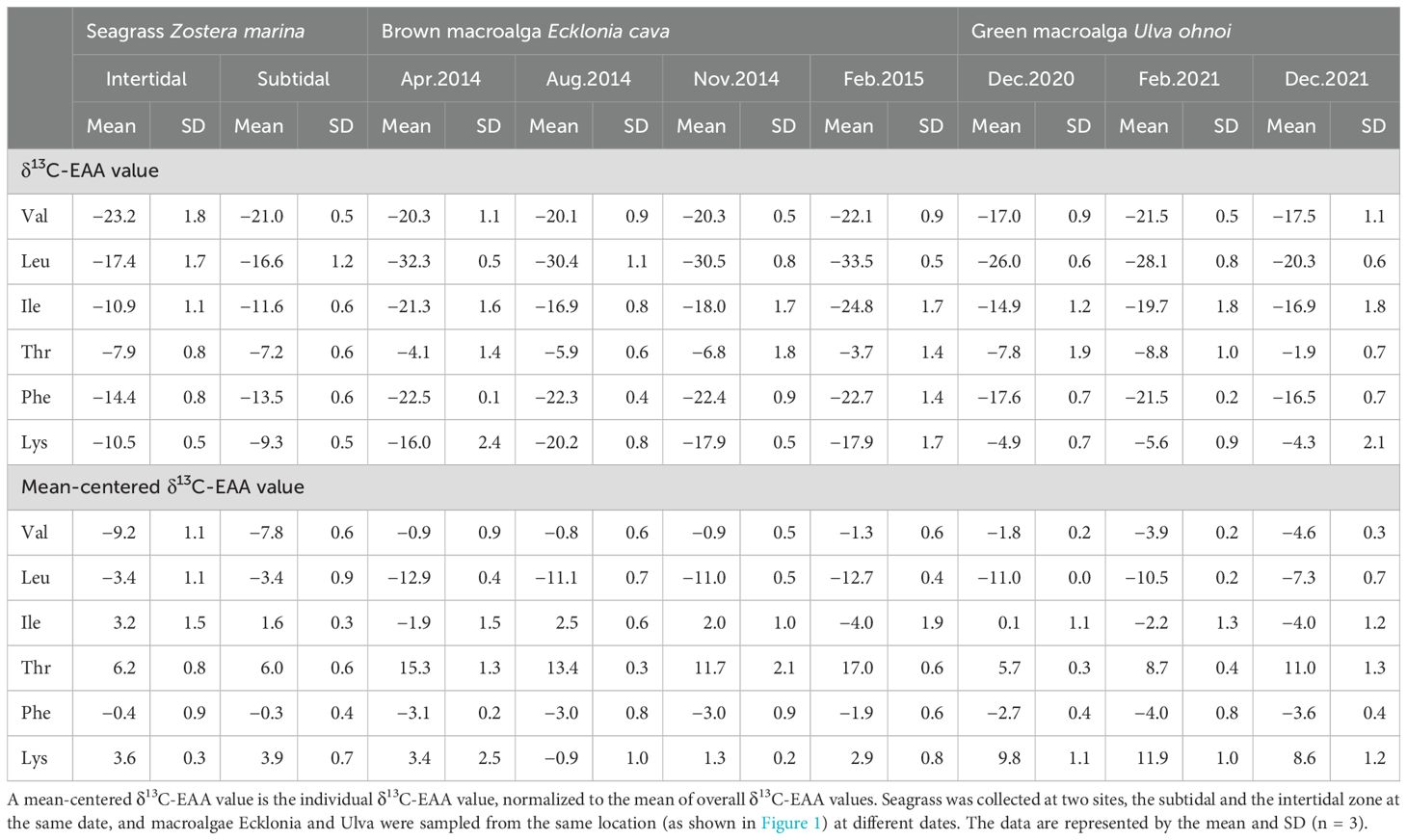
Table 1. Carbon stable isotope values of essential amino acids (δ13C-EAA values) such as valine (Val), leucine (Leu), isoleucine (Ile), threonine (Thr), phenylalanine (Phe), and lysine (Lys) from macrophytes collected in the field in Korea.
3.1.2 Lab-cultured Ulva
There were significant interaction effects of temperature gradients and added N on most AAs, particularly for Val, Ile, Thr, and Phe, and on mean-centered δ13C-EAA values of Leu in Ulva (Supplementary Table 1, Figure 3). In fact, most AAs in Ulva incubated in seawater (controls) did not differ significantly among the temperature gradients, but the δ13C values of Leu and Ile at 12°C were lower by approximately > 2.9‰ than the one at 27.5°C (Figure 3A). The temperature-induced effect was stronger in Ulva under added N. That is, the EAAs of Ulva under added N had a low δ13C value at 12°C and a high δ13C value at 27.5°C (pcorrected < 0.017, Figure 3B), and the variability was approximately 7.7‰ throughout the overall AAs. However, no temperature-induced effect was observed in mean-centered δ13C-EAA values; Val, Thr, and Phe were not significantly affected by temperature increases, although Leu and Ile was higher at 12°C compared with 27.5°C (Figure 3B).
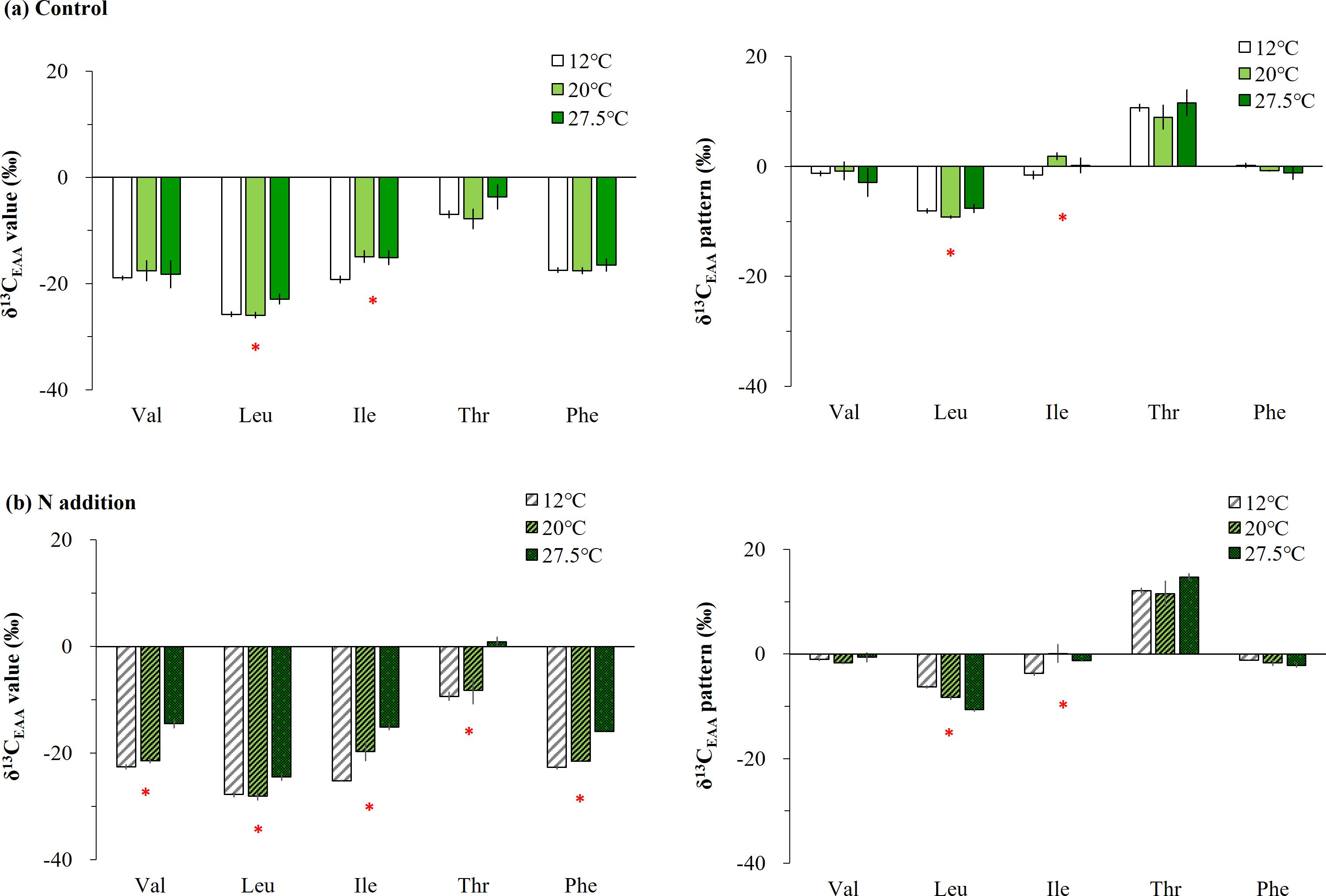
Figure 3. Comparing essential amino acid (EAA) δ13C variability under diverse temperature gradients of 12, 20, and 27.5°C in Ulva cultured in (A) ambient seawater only and (B) those with additional sources of N (nitrate and ammonia). The δ13C-EAA values and the mean-centered δ13C-EAA values are shown and represented by the mean ± SD (N = 3). Significant differences among the temperature gradients are marked by an asterisk above the bars.
3.2 Application of δ13C-EAA values in food web analysis
The δ13C-EAA values of seven common macrophytes collected in Busan are shown in Supplementary Table 2. The δ13C-EAA values ranged from −30.8‰ to −1.2‰ for red macroalgae, from −27.3‰ to −3.2‰ for brown macroalgae, and from −23.8‰ to −4.7‰ for green one, which were overlapped from −22.4‰ to 2.5‰ for seagrass (Figure 4A). The δ13C values for Val, Leu, Ile, Thr, Phe and Lys were low in red macroalgae (e.g., Gelidium), but high in green macroalga and seagrass. However, such differences among macrophytes were less detected in mean-centered δ13C-EAA values (Figure 4B).
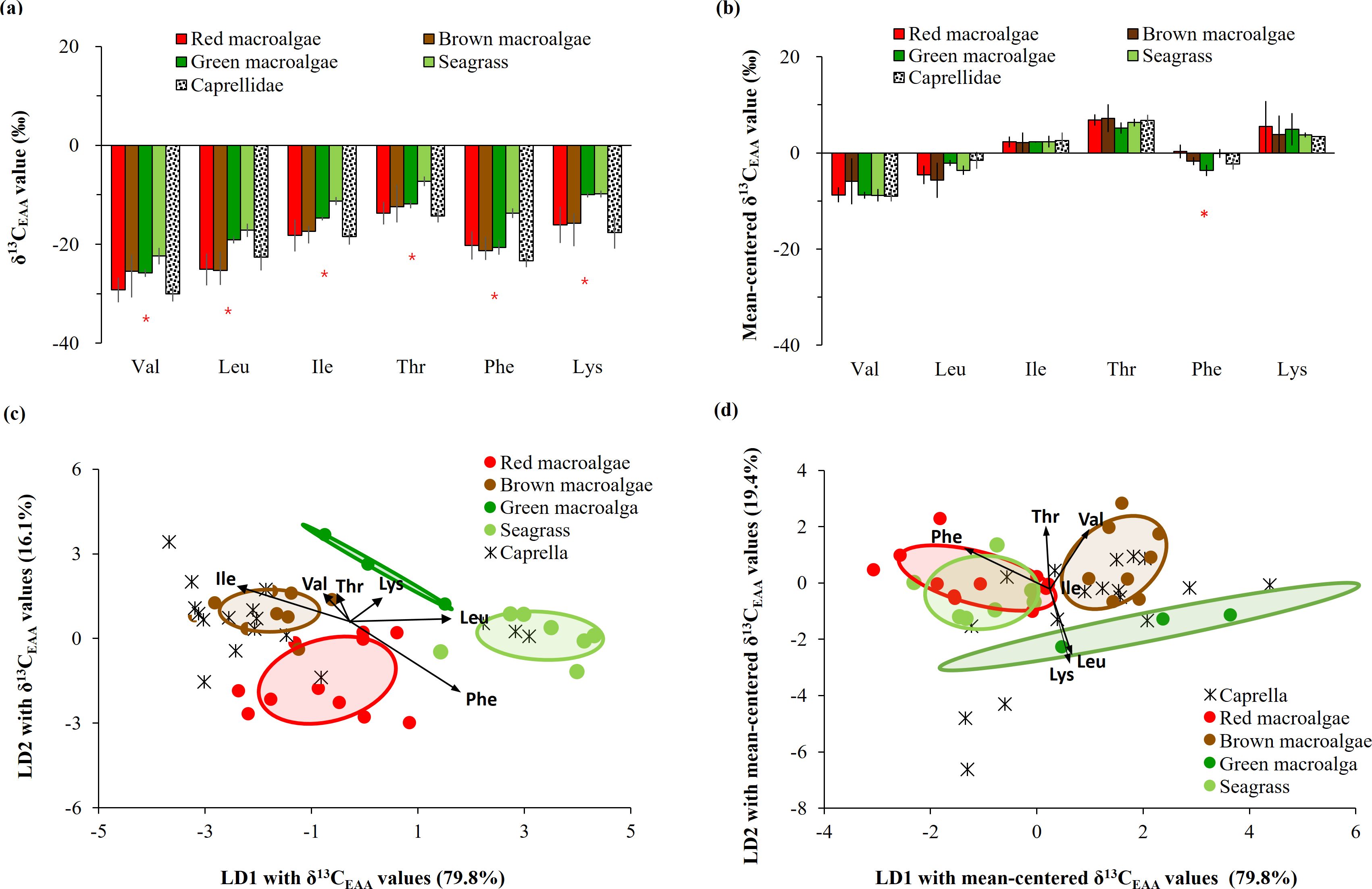
Figure 4. Comparison of (A) δ13C-EAA values and (B) mean-centered δ13C-EAA values from meso-grazer Caprella with macrophytes collected from Busan. An * is added if there is a significant difference (p < 0.05) in the AA isotopic values between any macrophyte producers and the meso-grazer, as determined by one-way ANOVA. Multivariate separation of Caprella and four macrophyte groups as source end-members, based on linear discriminant analysis based on (C) δ13C-EAA values and (D) mean-centered δ13C-EAA values. Ellipses present 50% of the data.
The δ13C-EAA values of the consumer Caprella differed significantly from those of the seagrass groups (with an average difference of > 5.45‰, Figure 4A), and they were relatively similar to those of the macroalgal groups. The difference in δ13C-EAA values between Caprella and seagrass was observed for Phe only in the context of mean-centered δ13C-EAA values (Figure 4B).
A LDA plot with δ13C-EAA values of four macrophyte groups from Busan showed that red, brown, and green macroalgal groups and seagrass group were classified with 90.0% probability within their own groups (Wilkis’ λ = 0.05, F = 6.27, p < 0.001, Figure 4C). This supports that δ13C-EAA values can be used to distinguish among macrophytes. Moreover, the LDA model among four macrophyte groups predicted that overall Caprella individuals were closely located to macroalgal groups (mostly brown macroalgae), but not to seagrass groups (Figure 4C, Supplementary Table 3). A PCA with the δ13C-EAA values also showed that Caprella was clustered with the co-sampled macroalgae from a local environment (Busan) and separated from the other environments, such as the seagrass site (Figure 5). This distinctiveness implies that the δ13C-EAA values in a consumer can reveal direct trophic links with a co-occurring macrophyte in a local environment.
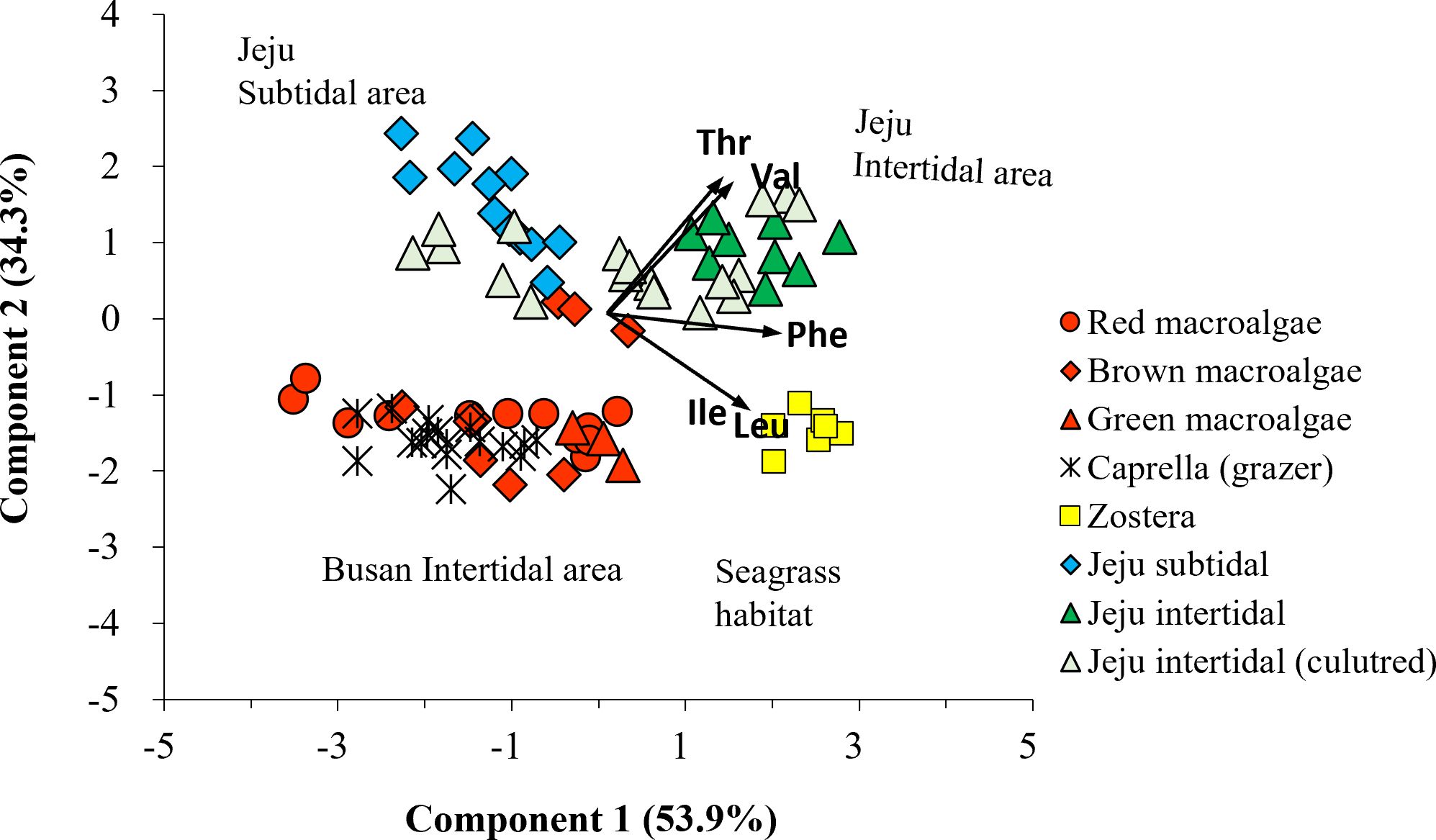
Figure 5. Principal component analysis of δ13C-EAA values (including Val, Leu, Ile, Thr, and Phe) in macrophytes (circle: red algae; diamond: brown algae; triangle: green algae; square: seagrass) collected at multiple sites (red: Busan intertidal area; yellow: seagrass area; blue: Jeju subtidal area; green: Jeju intertidal area; pale green: Ulva culture). The δ13C-EAA values from the meso-grazer group Caprella overlapped with those of the macroalgal groups, which were collected on the Busan intertidal shore.
3.3 Assessing the consistency of mean-centered δ13C-EAA values across field and comparative studies
When LDA analysis was performed with mean-centered δ13C-EAA values for macroalgal groups collected from Busan, red, brown and green macroalgal groups were classified with 95.6% probability within their own groups (Wilkis’ λ = 0.18, F = 3.35, p = 0.004). Upon adding mean-centered δ13C-EAA values for the seagrass group, LDA analysis showed that the seagrass group was classified to its own group with 71.4% posterior probability, but 28.6% of the seagrass group overlapped with red macroalgal groups (Figure 4D). The green macroalgal group did not overlap with either the red macroalgal or seagrass group. The LDA model based on mean-centered δ13C-EAA values of the four macrophyte groups showed that overall Caprella individuals were widely distributed from macroalgal groups (green and brown macroalgae) to seagrass group (Figure 4D), with lower prediction probabilities than the LDA model based on δ13C-EAA values (Supplementary Table 3). The separation among the four macrophytes in Busan remained even after including macroalgal samples collected from Jeju and lab-cultured Ulva in the analysis (Figure 6).
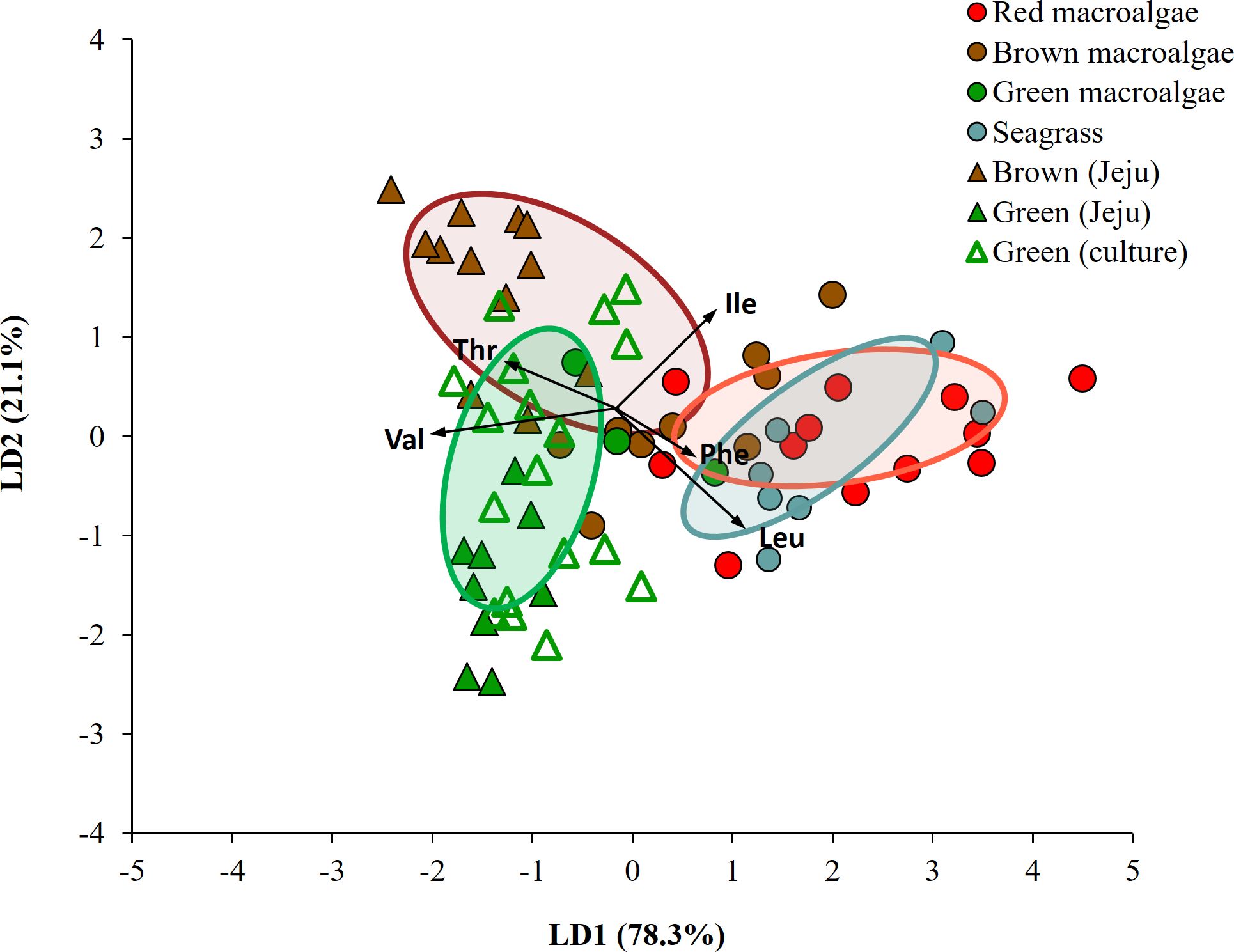
Figure 6. Linear discriminant analysis with mean-centered δ13C-EAA values (5 EAAs: Val, Leu, Ile, Thr, Phe) of macrophytes collected in the field (Busan and Jeju) and lab-cultured sample. Ellipses present 50% of the data.
On applying diverse taxonomic groups, our LDA model correctly classified 76 of 100 samples (76%) within their own groups of bacteria, microalgae, macroalgae, and seagrass, using previously published dataset (Larsen et al., 2009, 2013; Elliott Smith et al., 2021). LDA confidence ellipses for bacteria, microalgae, macroalgae, and seagrass did not fully overlap, and the bacteria and seagrass groups in particular were distinct from each other (Supplementary Figure 2A). Both macroalgae and seagrass showed comparably low dissimilarity (i.e., a low BC value) to bacteria (Supplementary Table 4). This consistency was observed with a predictability of 75% using seagrass and macroalgal groups from the field as end members (Supplementary Figure 2B). The model successfully classified all bacteria, 96% of microalgae, all seagrasses, and 47% of macroalgae. A low similarity (a low BC value) was seen between macroalgae and bacteria, but high similarity (a high BC value) with seagrass (Supplementary Table 4). With the addition of lab-cultured macroalgal samples to the macroalgal group, the model successfully classified all bacteria, the majority of macroalgae (64%), most microalgae (96%), and all seagrass (Figure 7). This suggests that phylogeny grouping plays a more significant role in separation than the physiological responses of macroalgae to environmental changes.
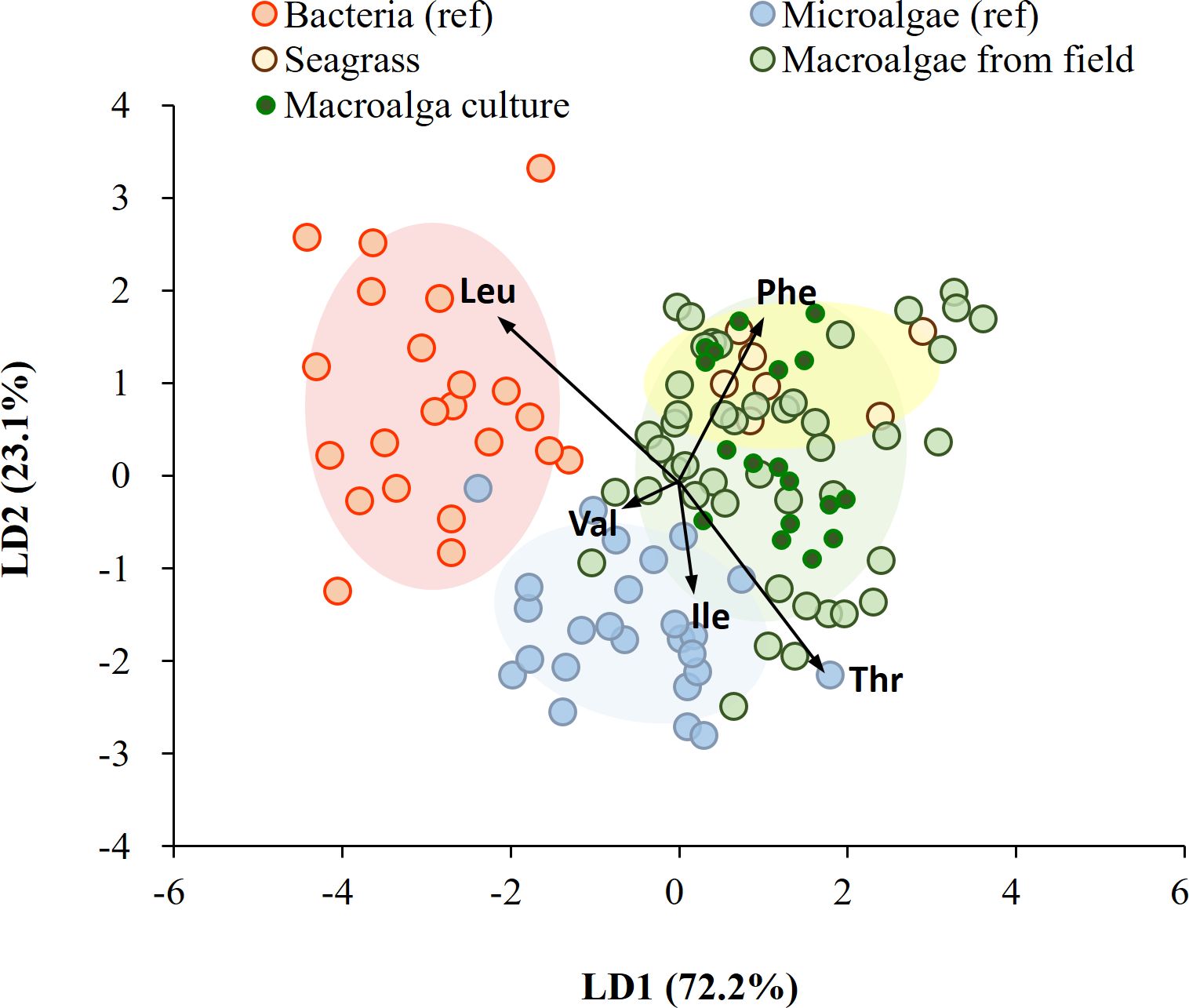
Figure 7. Linear discriminant analysis of mean-centered δ13C-EAA values (five EAAs considered: Val, Leu, Ile, Thr, and Phe) from microalgae, bacteria, seagrass, and macroalgae. Data for microalgae and bacteria were obtained from Larsen et al. (2013), and macroalgae and seagrass were field-collected and lab-cultured for this study. Ellipses represent 70% of the data.
4 Discussion
Our findings show that mean-centered δ13C-EAA values in macrophytes remain consistent under environmental changes. Similar to microalgal cultures over diverse abiotic conditions (Larsen et al., 2015; Stahl et al., 2023), our study re-confirms that field sampling over temporal variations and cultured setups with added N and temperature gradients can influence the δ13C-EAA values of macrophytes. We found that interactions between higher temperatures and added N increased δ13C-EAA values. Carbon isotope fractionation in EAAs in response to temperature and nutrient availability can be mediated by increasing biomolecule quantities, such as those for AAs and sugars (Gao et al., 2018; He et al., 2018; Chen et al., 2023). However, distinct mean-centered δ13C-EAA values among different macroalgal groups were observed across spatio-temporal scales, which is consistent with previous studies (Elliott Smith et al., 2022). Both δ13C-EAA and mean-centered δ13C-EAA values in macrophytes can therefore be used to assess ecological changes, including eutrophication and climate change, in a local environment.
4.1 Effect of environmental variations on macrophytes
We examined the δ13C-EAA values in Zostera seagrass from two habitats (subtidal and intertidal areas) collected simultaneously. Zostera is widely distributed in both intertidal and subtidal zones due to its physiological flexibility to cope with diverse abiotic conditions, such as high irradiance and desiccation-induced stress in intertidal zones relative to lower irradiance and submergence in the subtidal zone (Park et al., 2016). We expected to find distinct δ13C-EAA values in Zostera at spatial scales. However, there was no significant difference in individual δ13C-EAA values between intertidal and the subtidal specimens (Figure 2), although the overall mean of the δ13C-EAA values in the intertidal algae (−14.1‰) was slightly lower than that of the subtidal algae (−13.2‰). Marine macrophytes are known to utilize HCO3- (which has a relatively high δ13C value) as well as CO2 (which has a relatively low δ13C value) for photosynthesis (Fry and Sherr, 1989; Raven et al., 2002a). Bulk δ13C values ranging from −10‰ to −30‰ indicate that macrophytes use both CO2 and HCO3- with variable gradients as inorganic carbon sources (Raven et al., 2002a, 2002). Within this isotopic range, HCO3- users tend to be higher, while CO2 users tend to be lower due to the active use of carbon-concentrating mechanisms during photosynthesis (Raven et al., 2002a, 2002). The slightly low carbon isotope value in intertidal Zostera relative to subtidal algae indicates that the major carbon sources for photosynthesis involve a higher proportion of atmospheric and/or dissolved CO2 compared with HCO3-. Consistent with our isotopic results, the δ13C value of bulk leaf tissue is considerably lower in intertidal algae compared with subtidal counterparts, particularly those collected in fall and winter in Korea (Park et al., 2016). The negligible difference in δ13C-EAA values between the two sites in our results indicates that seagrasses may exhibit a similar physiological response during the winter, possibly because of the less-dynamic conditions relative to other seasons and considering abiotic factors such as carbon sources, temperature, irradiance, and desiccation in intertidal and subtidal areas.
The perennial brown macroalga Ecklonia exhibited temporally variable δ13C-EAA values at diverse temporal scales (across seasons, as shown in Table 1 and Figure 2). For Ecklonia, the lowest δ13C-EAA values (Leu and Ile) were detected in the winter season (February 2015). Coinciding with the time period of the Ecklonia sampling for isotope measurement, both the blade length and biomass of the mature plant significantly increased in the field (Munseom Island) between winter and spring (Kim et al., 2016). Furthermore, active growth of Ecklonia in the lab was observed, particularly at low temperatures simulating ambient conditions during winter, in addition to nitrogen sources (Choi et al., 2020). Several studies consistently reported a decrease in the 13C of bulk macroalgal tissues during winter, based on the brown macroalgae Macrocystis (Drobnitch et al., 2018) and Laminaria (Shim et al., 2017). This phenomenon occurs during the coldest winter months in seawater, an active growth period, as indicated by the increase in both length and weight. This temperature-dependent response was also observed in opportunistic species of Ulva. In particular, field-collected Ulva exhibited a significant decrease in δ13C-Val values in February 2021 (the coldest month for seawater around Jeju Island) compared with the other sampling months (Figure 2). The lower δ13C-EAA values in macroalgae may therefore be a physiological response to colder temperatures, inducing active growth in the field.
Similar to the Ulva specimens collected from the field (Figure 2C), Ulva cultured with added nutrients (100 µM NO3- and 100 µM NH4+) exhibited isotopic changes influenced by temperature gradients. Specifically, all δ13C-EAA values changed gradually across the temperature gradients, with lower values observed at 12°C and higher values at 27.5°C (Figure 3B). To a lesser extent, Leu and Ile δ13C values from cultured Ulva in ambient seawater were also influenced by temperature, showing lower values at 12°C compared with 20°C or 27.5°C (Figure 3A). Previous studies on members of the Ulva genus report that the interactive effects of high N nutrients (nitrate and ammonium) and temperature differences increase the amount of AAs (Gao et al., 2018; He et al., 2018; Chen et al., 2023). In terms of AA metabolism, U. ohnoi under high-temperature conditions (35°C) has a much higher quantity of diversity of AAs (alanine, glycine, serine, glutamic acid, Val, Leu, Ile, Phe, and Tyr), while Ulva under low-temperature conditions (15°C) has increased quantities of a few AAs (Leu, Ile, Tyr, and proline) (He et al., 2018). Moreover, Gao et al. (2018) reported that the protein content and AA quantities in U. rigida increased after 12 days of culturing with added N (e.g., 6 µM to 150 µM nitrate) and temperature conditions (14°C and 18°C). Active EAA accumulation in response to the combination of added N and temperature would therefore be expected in our Ulva sample.
A recently introduced species from subtropical regions, U. ohnoi, demonstrates strong adaptability to elevated temperatures and inorganic nitrogen sources. It can grow continuously even in warm seawater in temperate regions under diverse N sources (Kang et al., 2021). Our Ulva samples, when subjected to extremely high nitrogen supplies and a high temperature of 27.5°C, showed no dramatic decrease or degradation in the thalli. Instead, steady growth was observed over a two-week period (personal observation by SR Park). Previous studies have reported that an increase in temperature and nutrient additions may induce the accumulation AAs in Ulva thalli (Gao et al., 2018; He et al., 2018; Chen et al., 2023), as well as fructose, galactose, and mannose (involved in galactose metabolism), and fructose, sucrose, maltose, and glucose (involved in starch/sucrose metabolism) (He et al., 2018). Given the relationship between products and precursors, the δ13C values of products are expected to be lower than those of the precursors. This is because lighter isotopes such as 12C are used in larger quantities during biochemical processes, leaving behind a higher proportion of heavier isotopes such as 13C, in a process known as isotopic discrimination. The principle of isotopic discrimination suggests a general decrease in δ13C-EAA values, particularly if a single precursor is incorporated to produce a large amount of biomolecules or products (AAs). However, a broad spectrum of isotope values in diverse precursors would affect the δ13C-EAA values in the resulting products. The δ13C-EAA values in Ulva were unexpectedly high in our study (Figure 3B). This supports the possibility that the production of AAs and the carbon skeletons supplying them, under increased temperatures and added N, may be derived from different intermediates of the carbon backbones and the interconnection of diverse biosynthetic routes. The isotopic fractionation of δ13C-EAA values in Ulva may vary depending on environmental conditions, but further study is needed to determine whether a macroalgal AA–based indicator can be effective over a wide range of N concentrations, including the field gradient (Jones et al., 1996). However, our findings suggest that δ13C-EAA values can serve as a critical indicator for adaptations of macroalgae to changing environmental conditions in coastal oceans.
4.2 Ecological insights of δ13C-EAA and mean-centered δ13C-EAA values
In a PCA plot of δ13C-EAA values (Figure 5), the distinct effect of the sampling location is clear, with most intertidal macroalgae from Busan separated from seagrass (Busan), Ecklonia (Jeju subtidal area), and Ulva (Jeju intertidal area). A brown macroalga from Busan (Sargassum muticum only) was located close to other macroalgal species from Jeju Island. Ulva specimens (sampled in Jeju and grown in lab conditions for two weeks) exhibit a wide distribution relative to Ulva collected from the field (sampled in Busan) in the PCA plot. Focusing on the Busan area, LDA plot with δ13C-EAA values also showed that macroalgal groups (red, green and brown macroalgae, respectively) were differentiated from seagrass group (Figure 4C). These results suggest that δ13C-EAA values of macrophytes can serve as indicators of basal organisms, local growth conditions, which vary across time and space at different geographic scales (Vokhshoori et al., 2014; Larsen et al., 2015; Elliott Smith et al., 2022; Stahl et al., 2023).
Specimens of the consumer Caprella were overlapped with co-sampled intertidal macroalgal samples in the Busan area (Figure 4). The LDA model with δ13C-EAA values of four macrophyte groups predicted overall Caprella individuals as macroalgal groups, mostly brown macroalgae (Figure 4C, Supplementary Table 3). Although three Caprella samples appeared to be close to the seagrass group (Figure 4C), due to LD3 component in LDA analysis (Supplementary Figure 3), they were actually predicted to belong to the green macroalgal group (Supplementary Table 3). Comparably, the LDA model with mean-centered δ13C-EAA values also showed that most Caprella samples were likely predicted as macroalgal groups rather than seagrass group (Figure 4D). Overall results indicate that Caprella samples seem to have assimilated EAAs from mainly brown macroalgae, but likely also other macroalgae or basal organism that they have not been tested. It is consistent with the feeding behavior of Caprella, which is both a grazer and a detritus feeder (Kang et al., 2008) that is unlikely to feed on bacteria or assimilate bacteria-produced EAAs.
At the level of individual EAAs, the δ13C values of Val, Leu, Ile, Thr, and Phe in the consumer Caprella were isotopically similar to one of macroalgal groups (red, green and brown macroalgal groups), but were significantly different from δ13C-EAA values in seagrass (Figure 4A). δ13C-EAA values, originating from trophic bases, are generally passed on through trophic transfer to consumers without significant changes in isotopic values (reviewed in Whiteman et al., 2019; Yun et al., 2022). As macrophytes groups exhibit distinguishable δ13C-EAA values (e.g., Figure 4), the small consumers co-occurring with seagrass beds should show isotopically different values from consumers co-occurring with macroalgal beds. When considering the “isotopic baseline” with δ13C-EAA values of the consumer Caprella, a difference in baseline δ13C-EAA values is expected, based on the assumption that consumer δ13C-EAA values reflect those of their primary food source. Although several studies have reported the potential of the δ13C-EAA values of consumers to serve as “isotopic baselines” adapted for specific geographic ecosystems and variations (e.g., Vokhshoori et al., 2014; McMahon et al., 2016; Vane et al., 2023b), the δ13C-EAA values of a mixed basal resource user (e.g., Caprella) might not be utilized as “isotopic baselines” to indicate specific local basal organisms in coastal areas. If one wants to trace the contribution of EAAs synthesized by specific basal organisms to a marine benthic consumer, it is essential to measure each basal organism directly, as is done in this study by analyzing individual macrophytes.
To minimize the influence of environmental characteristics on the physiological traits specific to macrophytes, we used mean-centered δ13C-EAA values (Larsen et al., 2013, 2015). Our hypothesis is consistent with prior research that found that mean-centered δ13C-EAA values remain robust across different times and lab-cultured conditions, unlike the variable δ13C-EAA values. Indeed, both the temporal variation (Figure 2) and lab-cultured conditions (Figure 3) had a less significant impact on mean-centered δ13C-EAA values for macrophytes. The variability range for mean-centered δ13C-EAA values of cultured Ulva (approximately 1.0‰ to 4.4‰) was narrower than the δ13C-EAA values of 3.6‰ to 10.3‰. Similarly, field-collected Ulva exhibited a limited variability range of 1.2‰ to 5.3‰ for mean-centered δ13C-EAA values in contrast to 4.5‰ to 7.8‰ for δ13C-EAA values (Figure 2C). Our results support the notion that mean-centered δ13C-EAA values in hard- and soft-bottom portions of subtidal and intertidal zones remain consistent. Previous studies also report consistent mean-centered δ13C-EAA values in both laboratory experiments and collections from natural environments (Larsen et al., 2015; Elliott Smith et al., 2022). This approach can therefore be used to reliably characterize large taxonomic groups.
Using mean-centered δ13C-EAA values for macroalgae in Busan, an LDA plot (Figure 4D) shows distinct separation among brown (Phaeophyta), red (Rhodophyta), and/or green (Chlorophyta) algae, which is consistent with findings from previous studies (Larsen et al., 2013; Elliott Smith et al., 2021, 2022). This separation may be related to significant phylogenetic distances among macroalgal groups (e.g., Kloareg et al., 2021) and emphasizes the reliability of mean-centered δ13C-EAA values in distinguishing among macrophyte groups. This reliability is primarily influenced by taxonomic, morphological, and biochemical differences among macroalgal groups, while environmental variations have less of an impact. However, upon inclusion of seagrass group, a considerable overlap was observed between seagrass and red macroalgae, and this trend remained even after lab-cultured Ulva samples (green macroalga) were included in the LDA analysis (Figure 6). Although phylogenic distances are relatively long between seagrass and red macroalgae (Kloareg et al., 2021), there was an overlap in the LDA plot. This overlap is likely due to the large variability among the macrophytes and the extreme variation in site-specific environmental conditions reported in this study, and therefore could add variances to the macroalgal groups in our LDA analysis. Furthermore, as training data from larger phylogenetic groups such as bacteria and microalgae were included, macroalgae overlapped with seagrass (Figure 7). However, the LDA results based on mean-centered δ13C-EAA values (Figure 7 and Supplementary Figure 2) were robust, correctly classifying seagrass, bacteria, and microalgae (> 88%). Including more EAAs in an LDA analysis may improve its ability to distinguish macrophytes for food web studies. These results indicate that mean-centered δ13C-EAA values of macrophytes can be used effectively to characterize benthic food webs, particularly in environments where macroalgae and seagrass do not abundantly co-occur, such as rocky shores (macroalgae-dominant environments) and sandy/muddy bottoms (e.g., seagrass meadows).
5 Conclusions
We investigated the variation of δ13C-EAA values in macrophytes, ranging from seagrass to red, brown and green macroalgae, across diverse environmental conditions. We confirmed that diverse macrophytes can be classified by their mean-centered δ13C-EAA values, although the distinction between red macroalgae and seagrass was not clear. However, we were able to use δ13C-EAA values to differentiate among seagrass and macroalgal groups, particularly those from the Busan region. This indicates that δ13C-EAA values can serve as “isotopic baselines” to characterize local environments. Variation in δ13C-EAA and mean-centered δ13C-EAA values can therefore provide diverse insights into the characteristics of local trophic bases at sites undergoing environmental changes. To monitor the biochemical and ecological impacts of environmental problems, future studies should explore the effects of acidification, CO2 levels, and eutrophication on the stable isotope values of AAs in a key macrophyte species and their associated consumers across geographic scales.
Data availability statement
The data presented in the study are deposited in Figshare, available here: https://doi.org/10.6084/m9.figshare.26519647.v3.
Ethics statement
The manuscript presents research on animals that do not require ethical approval for their study.
Author contributions
HY: Conceptualization, Data curation, Formal analysis, Funding acquisition, Investigation, Methodology, Project administration, Resources, Supervision, Validation, Visualization, Writing – original draft, Writing – review & editing. SK: Formal analysis, Methodology, Resources, Writing – review & editing. HC: Formal analysis, Methodology, Writing – review & editing. JK: Formal analysis, Methodology, Writing – review & editing. SP: Methodology, Resources, Writing – review & editing. KS: Funding acquisition, Writing – review & editing.
Funding
The author(s) declare financial support was received for the research, authorship, and/or publication of this article. This work was supported by the Basic Science Research Program through the National Research Foundation (NRF−2021R1l1A1A01061284), and funded by the Korea Institute of Marine Science & Technology Promotion (KIMST) - “Shift of marine ecosystem in response to climate change in the Tsushima Warm Current system of Korea waters” (KIMST-20220559) and “Development of living shoreline technology based on blue carbon science toward climate change adaptation” (KIMST-20220526).
Acknowledgments
Special thanks to the students for lab-culture at Jeju National University and for field sampling from Busan National University. We deeply appreciate the reviewers who contributed to the improvement of this manuscript.
Conflict of interest
The authors declare that the research was conducted in the absence of any commercial or financial relationships that could be construed as a potential conflict of interest.
The reviewer TL declared a past co-authorship with the authors.
Publisher’s note
All claims expressed in this article are solely those of the authors and do not necessarily represent those of their affiliated organizations, or those of the publisher, the editors and the reviewers. Any product that may be evaluated in this article, or claim that may be made by its manufacturer, is not guaranteed or endorsed by the publisher.
Supplementary material
The Supplementary Material for this article can be found online at: https://www.frontiersin.org/articles/10.3389/fmars.2024.1368801/full#supplementary-material
References
Bearham D., Vanderklift M. A., Gunson J. R. (2013). Temperature and light explain spatial variation in growth and productivity of the kelp Ecklonia radiata. Mar. Ecol. Prog. Ser. 476, 59–70. doi: 10.3354/meps10148
Besser A. C., Elliott Smith E. A., Newsome S. D. (2022). Assessing the potential of amino acid δ13C and δ15N analysis in terrestrial and freshwater ecosystems. J. Ecol. 110, 935–950. doi: 10.1111/1365-2745.13853
Bhattacharyya A. (1946). On a measure of divergence between two multinomial populations. Sankhyā: Indian J. Stat (1933-1960) 7, 401–406.
Carrier-Belleau C., Drolet D., Mckindsey C. W., Archambault P. (2021). Environmental stressors, complex interactions and marine benthic communities’ responses. Sci. Rep. 11, 4194. doi: 10.1038/s41598-021-83533-1
Casella N., Careddu G., Calizza E., Sporta Caputi S., Rossi L., Belluscio A., et al. (2022). Increasing nutrient inputs over the last 500 years in an Italian low-impacted seagrass meadow. Mar. pollut. Bull. 174, 113298. doi: 10.1016/j.marpolbul.2021.113298
Chen Y., Zheng M., Jiang J., Hu W., Xu N., Li Y. (2023). Enhancement of growth in Ulva prolifera by diurnal temperature difference combined with nitrogen enrichment. Mar. Environ. Res. 186, 105905. doi: 10.1016/j.marenvres.2023.105905
Choi S. K., Kang Y. H., Park S. R. (2020). Growth responses of kelp species Ecklonia cava to different temperatures and nitrogen sources. Korean J. Environ. Biol. 38, 404–415. doi: 10.11626/KJEB.
Cohen R. A., Fong P. (2006). Using opportunitic green macroalgae as indicators of nitrogen supply and sources to estuaries. Ecol. Appl. 16, 1405–1420. doi: 10.1890/1051-0761(2006)016[1405:UOGMAI]2.0.CO;2
Corr L. T., Berstan R., Evershed R. P. (2007). Development of N-acetyl methyl ester derivatives for the determination of δ13C values of amino acids using gas chromatography-combustion- isotope ratio mass spectrometry. Anal. Chem. 79, 9082–9090. doi: 10.1021/ac071223b
D’archino R., Piazzi L. (2021). Macroalgal assemblages as indicators of the ecological status of marine coastal systems: A review. Ecol. Indic. 129, 107835. doi: 10.1016/j.ecolind.2021.107835
Dawson T. E., Mambelli S., Plamboeck A. H., Templer P. H., Tu K. P. (2002). Stable isotopes in plant ecology. Annu. Rev. Ecol. Evol. Syst. 33, 507–559. doi: 10.1146/annurev.ecolsys.33.020602.095451
Deniro M. J., Epstein S. (1978). Influence of diet on the distribution of carbon isotopes in animals. Geochim. Cosmochim. Acta 42, 495–506. doi: 10.1016/0016-7037(78)90199-0
Drobnitch S. T., Pochron T., Miranda C. (2018). Patterns and drivers of δ13C variation in the giant kelp, Macrocystis pyrifera. Limnol. Oceanogr. 63, 871–885. doi: 10.1002/lno.10675
Eglite E., Mohm C., Dierking J. (2023). Stable isotope analysis in food web research: Systematic review and a vision for the future for the Baltic Sea macro-region. Ambio 52, 319–338. doi: 10.1007/s13280-022-01785-1
Elliott Smith E. A., Fox M. D., Fogel M. L., Newsome S. D. (2022). Amino acid δ13C fingerprints of nearshore marine autotrophs are consistent across broad spatiotemporal scales: An intercontinental isotopic dataset and likely biochemical drivers. Funct. Ecol. 36, 1191–1203. doi: 10.1111/1365-2435.14017
Elliott Smith E. A., Harrod C., Docmac F., Newsome S. D. (2021). Intraspecific variation and energy channel coupling within a Chilean kelp forest. Ecology 102, e03198. doi: 10.1002/ecy.3198
Fry B., Sherr E. B. (1989). “δ13C measurements as indicators of carbon flow in marine and freshwater ecosystems,” in Stable Isotopes in Ecological Research, vol. vol 68 . Eds. Rundel P. W., Ehleringer J. R., Nagy K. A. (Springer, New York, NY). doi: 10.1007/978-1-4612-3498-2_12
Gao G., Clare A. S., Chatzidimitriou E., Rose C., Caldwell G. (2018). Effects of ocean warming and acidification, combined with nutrient enrichment, on chemical composition and functional properties of Ulva rigida. Food Chem. 258, 71–78. doi: 10.1016/j.foodchem.2018.03.040
He Y., Hu C., Wang Y., Cui D., Sun X., Li Y., et al. (2018). The metabolic survival strategy of marine macroalga Ulva prolifera under temperature stress. J. Appl. Phycol. 30, 3611–3621. doi: 10.1007/s10811-018-1493-3
Jackson A. L. (2023). Stable Isotope Bayesian Ellipses in R (R package version SIBER 2.1.9). Available online at: https://cran.r-project.org/web/packages/SIBER/index.html.
Jackson A. L., Inger R., Parnell A. C., Bearhop S. (2011). Comparing isotopic niche widths among and within communities: SIBER – Stable Isotope Bayesian Ellipses in R. J. Anim. Ecol. 80, 595–602. doi: 10.1111/jane.2011.80.issue-3
Jones A. B., Dennison W. C., Stewart G. R. (1996). Macroalgal responses to nitrogen source and availability: Amino acid metabolic profiling as a bioindicator using Gracilaria edulis (Rhodophyta). J. Phycol. 32, 757–766. doi: 10.1111/j.0022-3646.1996.00757.x
Kang C.-K., Choy E. J., Son Y., Lee J.-Y., Kim J. K., Kim Y., et al. (2008). Food web structure of a restored macroalgal bed in the eastern Korean peninsula determined by C and N stable isotope analyses. Mar. Biol. 153, 1181–1198. doi: 10.1007/s00227-007-0890-y
Kang E. J., Han A. R., Kim J.-H., Kim I.-N., Lee S., Min J.-O., et al. (2021). Evaluating bloom potential of the green-tide forming alga Ulva ohnoi under ocean acidification and warming. Sci. Total Environ. 769, 144443. doi: 10.1016/j.scitotenv.2020.144443
Kang J. H., Jang J. E., Kim J. H., Byeon S. Y., Kim S., Choi S. K., et al. (2019). Species composition, diversity, and distribution of the genus Ulva along the coast of Jeju Island, Korea based on molecular phylogenetic analysis. Plos One 14, e0219958. doi: 10.1371/journal.pone.0219958
Kim S., Kang Y. H., Kim T. H., Park S. R. (2016). Recovery pattern and seasonal dynamics of Kelp Species Ecklonia cava population formed following the large-scale disturbance. Sea 21, 103–111. doi: 10.7850/jkso.2016.21.3.103
Kim M.-S., Lee S.-M., Kim H.-J., Lee S.-Y., Yoon S.-H., Shin K.-H. (2014). Carbon stable isotope ratios of new leaves of Zostera marina in the mid-latitude region: Implications of seasonal variation in productivity. J. Exp. Mar. Biol. Ecol. 461, 286–296. doi: 10.1016/j.jembe.2014.08.015
Kloareg B., Badis Y., Cock J. M., Michel G. (2021). Role and evolution of the extracellular matrix in the acquisition of complex multicellularity in eukaryotes: a macroalgal perspective. Genes 12, 1059. doi: 10.3390/genes12071059
Larsen T., Bach L. T., Salvatteci R., Wang Y. V., Andersen N., Ventura M., et al. (2015). Assessing the potential of amino acid 13C patterns as a carbon source tracer in marine sediments: effects of algal growth conditions and sedimentary diagenesis. Biogeosciences 12, 4979–4992. doi: 10.5194/bg-12-4979-2015
Larsen T., Taylor D. L., Leigh M. B., O’brien D. M. (2009). Stable isotope fingerprinting: a novel method for identifying plant, fungal, or bacterial origins of amino acids. Ecology 90, 3526–3535. doi: 10.1890/08-1695.1
Larsen T., Ventura M., Andersen N., O’brien D. M., Piatkowski U., Mccarthy M. D. (2013). Tracing carbon sources through aquatic and terrestrial food webs using amino acid stable isotope fingerprinting. PloS One 8, e73441. doi: 10.1371/journal.pone.0073441
Lee S., Lee D. K. (2018). What is the proper way to apply the multiple comparison test? Korean J. Anesthesiol. 71, 353–360. doi: 10.4097/kja.d.18.00242
Liénart C., Garbaras A., Qvarfordt S., Walve J., Karlson A. M. L. (2022). Spatio-temporal variation in stable isotope and elemental composition of key-species reflect environmental changes in the Baltic Sea. Biogeochemistry 157, 149–170. doi: 10.1007/s10533-021-00865-w
Liew J. H., Chua K. W. J., Arsenault E. R., Thorp J. H., Suvarnaraksha A., Amirrudin A., et al. (2019). Quantifying terrestrial carbon in freshwater food webs using amino acid isotope analysis: Case study with an endemic cavefish. Methods Ecol. Evol. 10, 1594–1605. doi: 10.1111/2041-210X.13230
Lovelock C. E., Reef R., Raven J. A., Pandolfi J. M. (2020). Regional variation in δ13C of coral reef macroalgae. Limnol. Oceanogr. 65, 2291–2302. doi: 10.1002/lno.11453
Lynch A. H., Kruger N. J., Hedges R. E. M., Mccullagh J. S. O. (2016). Variability in the carbon isotope composition of individual amino acids in plant proteins from different sources: 1 Leaves. Phytochemistry 125, 27–34. doi: 10.1016/j.phytochem.2016.01.011
Mancinelli G., Vizzini S. (2015). Assessing anthropogenic pressures on coastal marine ecosystems using stable CNS isotopes: State of the art, knowledge gaps, and community-scale perspectives. Estuar. Coast. Shelf Sci. 156, 195–204. doi: 10.1016/j.ecss.2014.11.030
McMahon K. W., Berumen M. L., Mateo I., Elsdon T. S., Thorrold S. R. (2011). Carbon isotopes in otolith amino acids identify residency of juvenile snapper (Family: Lutjanidae) in coastal nurseries. Coral Reefs 30, 1135–1145. doi: 10.1007/s00338-011-0816-5
McMahon K. W., Thorrold S. R., Houghton L. A., Berumen M. L. (2016). Tracing carbon flow through coral reef food webs using a compound-specific stable isotope approach. Oecologia 180, 809–821. doi: 10.1007/s00442-015-3475-3
Park S. R., Kim S., Kim Y. K., Kang C.-K., Lee K.-S. (2016). Photoacclimatory responses of Zostera marina in the intertidal and subtidal zones. PloS One 11, e0156214. doi: 10.1371/journal.pone.0156214
Pollierer M. M., Larsen T., Potapov A., Brückner A., Heethoff M., Dyckmans J., et al. (2019). Compound-specific isotope analysis of amino acids as a new tool to uncover trophic chains in soil food webs. Ecol. Monogr. 89, e01384. doi: 10.1002/ecm.1384
Raven J. A., Johnston A. M., Kübler J. E., Korb R., Mcinroy S. G., Handley L. L., et al. (2002a). Mechanistic interpretation of carbon isotope discrimination by marine macroalgae and seagrasses. Funct. Plant Biol. 29, 355–378. doi: 10.1071/PP01201
Raven J. A., Johnston A. M., Kuebler J. E., Korb R., Mcinroy S. G., Handley L. L., et al. (2002b). Seaweeds in cold seas: evolution and carbon acquisition. Ann. Bot. 90, 525–536. doi: 10.1093/aob/mcf171
R Core Team (2023). R: A language and environment for statistical computing (Version 4.3.1) (R Foundation for Statistical Computing, Vienna, Austria). Available at: https://www.R-project.org/.
Rice W. R. (1989). Analyzing tables of statistical tests. Evolution 43, 223–225. doi: 10.2307/2409177
Scott J. H., O’Brien D. M., Emerson D., Sun H., Mcdonald G. D., Salgado A., et al. (2006). An examination of the carbon isotope effects associated with amino acid biosynthesis. Astrobiology 6, 867–880. doi: 10.1089/ast.2006.6.867
Shim J., Kim J. B., Hwang D.-W., Choi H.-G., Lee Y. (2017). Variations in carbon and nitrogen stable isotopes and in heavy metal contents of mariculture kelp Undaria pinnatifida in Gijang, southeastern Korea. Algae 32, 349–357. doi: 10.4490/algae.2017.32.12.3
Stahl A. R., Rynearson T. A., Mcmahon K. W. (2023). Amino acid carbon isotope fingerprints are unique among eukaryotic microalgal taxonomic groups. Limnol. Oceanogr. 68, 1331–1345. doi: 10.1002/lno.12350
Stephens T. A., Hepburn C. D. (2014). Mass-transfer gradients across kelp beds influence Macrocystis pyrifera growth over small spatial scales. Mar. Ecol. Prog. Ser. 515, 97–109. doi: 10.3354/meps10974
Stockbridge J., Jones A. R., Gillanders B. M. (2020). A meta-analysis of multiple stressors on seagrasses in the context of marine spatial cumulative impacts assessment. Sci. Rep. 10, 11934. doi: 10.1038/s41598-020-68801-w
Takizawa Y., Takano Y., Choi B., Dharampal P. S., Steffan S. A., Ogawa N. O., et al. (2020). A new insight into isotopic fractionation associated with decarboxylation in organisms: implications for amino acid isotope approaches in biogeoscience. Prog. Earth Planet. Sci. 7, 50. doi: 10.1186/s40645-020-00364-w
Vane K., Cobain M. R. D., Larsen T. (2023a). The power and pitfalls of amino acid carbon stable isotopes for tracing origin and fate of basal resources in food webs (EcoEvoRxiv). Available at: https://ecoevorxiv.org/repository/view/5403/. doi: 10.32942/X2VG6G
Vane K., Cobain M. R. D., Trueman C. N., Vonnahme T. R., Rokitta S., Polunin N. V. C., et al. (2023b). Tracing basal resource use across sea-ice, pelagic, and benthic habitats in the early Arctic spring food web with essential amino acid carbon isotopes. Limnol. Oceanogr. 68, 862–877. doi: 10.1002/lno.12315
Velázquez-Ochoa R., Ochoa-Izaguirre M. J., Soto-Jiménez M. F. (2022). An analysis of the variability in δ13C in macroalgae from the Gulf of California: indicative of carbon concentration mechanisms and isotope discrimination during carbon assimilation. Biogeosciences 19, 1–27. doi: 10.5194/bg-19-1-2022
Vokhshoori N. L., Larsen T., Mccarthy M. D. (2014). Reconstructing δ13C isoscapes of phytoplankton production in a coastal upwelling system with amino acid isotope values of littoral mussels. Mar. Ecol. Prog. Ser. 504, 59–72. doi: 10.3354/meps10746
Whiteman J. P., Elliott Smith E. A., Besser A. C., Newsome S. D. (2019). A guide to using compound-specific stable isotope analysis to study the fates of molecules in organisms and ecosystems. Diversity 11, 8. doi: 10.3390/d11010008
Yokohama Y., Tanaka J., Chihara M. (1987). Productivity of the Ecklonia cava community in a bay of Izu Peninsula on the Pacific Coast of Japan. Botanical Magazine Tokyo 100, 129–141. doi: 10.1007/BF02488318
Yun H. Y., Larsen T., Choi B., Won E.-J., Shin K.-H. (2022). Amino acid nitrogen and carbon isotope data: Potential and implications for ecological studies. Ecol. Evol. 12, e8929. doi: 10.1002/ece3.8929
Keywords: compound-specific isotope analysis, physiological ecology, biomarker, trophic base, seaweed, producer, climate change
Citation: Yun HY, Kim S, Choi H, Kim J-E, Park SR and Shin K-H (2024) Essential amino acid carbon isotope ratios as indicators of marine macrophyte response to environmental variation. Front. Mar. Sci. 11:1368801. doi: 10.3389/fmars.2024.1368801
Received: 11 January 2024; Accepted: 29 July 2024;
Published: 23 August 2024.
Edited by:
Yunchao Wu, Chinese Academy of Sciences (CAS), ChinaReviewed by:
Kim Vane, Alfred Wegener Institute Helmholtz Centre for Polar and Marine Research (AWI), GermanyThomas Larsen, Max Planck Institute for Geoanthropology, Germany
Copyright © 2024 Yun, Kim, Choi, Kim, Park and Shin. This is an open-access article distributed under the terms of the Creative Commons Attribution License (CC BY). The use, distribution or reproduction in other forums is permitted, provided the original author(s) and the copyright owner(s) are credited and that the original publication in this journal is cited, in accordance with accepted academic practice. No use, distribution or reproduction is permitted which does not comply with these terms.
*Correspondence: Kyung-Hoon Shin, c2hpbmtoQGhhbnlhbmcuYWMua3I=