- 1School of Life and Environmental Sciences, Deakin University, Burwood, VIC, Australia
- 2Cooperative Institute for Climate, Ocean, and Ecosystem Studies, University of Washington, Seattle, WA, United States
Introduction: Human-induced environmental change is driving a global redistribution of biodiversity, resulting in shifting prey and predation landscapes. These shifting landscapes can lead to changes in behavior, health, and vital rates, with potential implications for population dynamics.
Methods: In the present study, a state-dependent life-history theory model was developed to investigate the individual- and population-level responses of Australian fur seals (Arctocephalus pusillus doriferus) to changes in prey availability and at-sea mortality risk.
Results: Rates of pregnancy, pup nursing, and abortion were unaffected by prey availability in the simulated population. Likewise, on-land and at-sea durations were largely unaffected by prey availability, with more pronounced affects for nonreproductive and pregnant females than for lactating females. There was a strong influence of prey availability on the proportion of females that were concurrently pregnant and lactating, largely due to an increase in pup abandonments under low prey availability scenarios. This effect on pup abandonments also had flow on effects for pup recruitment. Increasing at-sea mortality risk resulted in greater offspring losses due to maternal death. The combined impact of prey availability and at-sea mortality risk on the number of simulated female offspring reaching sexual maturity was substantial.
Discussion: Consequently, our results suggest high vulnerability of the Australian fur seal population to shifting prey and predation landscapes. These results indicate a need for continued monitoring of Australian fur seal pup production and population dynamics in the face of rapid environmental change.
1 Introduction
Human-induced environmental change is driving a global redistribution of biodiversity (Pecl et al., 2017). This redistribution is leading to shifting prey and predation landscapes across a range of habitats. Not only can changes in prey distribution and abundance (i.e. the prey landscape) directly influence the distribution and abundance of predators (i.e. the predation landscape), such changes can have indirect effects on the extent of intra- and inter-specific competition at higher trophic levels (Hoegh-Guldberg and Bruno, 2010). Furthermore, for central-place foraging animals, indirect effects on predation risk can also result from shifting prey landscapes due to increased exposure to predation for individuals undertaking longer foraging bouts to overcome prey limitations (Lima and Dill, 1990). These shifting landscapes can, thus, lead to changes in behavior (e.g. foraging activity) and physiology (e.g. stress responses) in wildlife populations. Such responses can aggregate, leading to changes in individual health and/or vital rates and, ultimately, population dynamics (Lima, 1998).
Effective management and conservation of wildlife populations under changing climates requires an understanding of the individual- and population-level responses to changes in their environment. This is often difficult to ascertain in wildlife populations for two main reasons. Firstly, it is difficult to monitor the long-term effects of environmental change on long-lived species, particularly those that have cryptic lifestyles. Secondly, it remains challenging to determine whether observed short-term behavioral or physiological responses to changes in the environment elicit biologically meaningful changes in population dynamics (Gill et al., 2001). Modelling approaches offer an opportunity to predict the extent to which changes in individual behavior and/or physiology aggregate to drive changes in population dynamics when direct observation is not possible (Grimm and Railsback, 2005; Schmolke et al., 2010) or when forecasting population responses to future change (Clark et al., 2001).
State-dependent life-history theory models, implemented via stochastic dynamic programming (SDP), provide a framework for investigating the population-level consequences of environmental change, including how changes in mortality risk and prey availability influence population dynamics (Houston et al., 1988; Mangel and Clark, 1988; Clark and Mangel, 2000). This framework predicts the optimal state-dependent behaviors under the assumption that individuals act to maximize some measure of Darwinian fitness, such as survival. SDP models are highly flexible making them applicable across a range of species and research questions. As a result, SDP models have been developed to study the effects of human disturbance and environmental change on a range of taxonomic groups [e.g (Bull et al., 1996; Tenhumberg et al., 2000; Satterthwaite et al., 2010; Denis et al., 2012)] including marine predators [e.g (Pirotta et al., 2018; Reimer et al., 2019; McHuron et al., 2023b)].
Here, we developed a SDP model using Australian fur seals (Arctocephalus pusillus doriferus; AUFS) as a case-study for understanding the additive effects of changes in prey availability and at-sea mortality risk on behavioral and reproductive decisions in a marine predator. Recent surveys on the species have identified declines in pup production at some natal colonies (McIntosh et al., 2022). It is hypothesized that these declines are in part due to changes in prey availability, toxicant exposure and disease, though this is largely speculative. Nonetheless, the population declines are concerning in face of the rapid oceanic warming in the south-east Australia region (Hobday and Pecl, 2014), which is expected to result in changes in the prey field in the region. Altered prey and predation landscapes can have substantial effects on the behavior, energy balance, and reproductive decisions of marine predators [e.g (Schwarz et al., 2013)]. While central-place foragers, such as AUFS, can respond behaviorally to shifting prey landscapes, they may be limited in their capacity to respond due to constraints on the fasting ability of their offspring. When behavioral changes inadequately address energy deficiencies, health and vital rates can be compromised (National Academies, 2017), leading to population level effects. Recent studies on Australian fur seals have reported behavioral and reproductive variability associated with local- and large-scale climate conditions (Speakman et al., 2020, 2021; Geeson et al., 2022), likely through climate-mediated changes in the prey landscape. However, it is unknown whether the behavioral or reproductive variability reported in these studies may lead to population-level shifts in behavior and/or vital rates, or subsequent changes in population trajectory under future climate change.
In addition to the ecological drivers for using AUFS as a model species, the large long-term dataset available for the species provides an opportunity to assess model agreement with empirical data on AUFS foraging behavior, reproductive rates and population trends. Such long-term datasets are lacking for many long-lived species. However, outcomes from this model highlight the need for additional species-specific data collection and ongoing population monitoring for AUFS. Furthermore, our results provide valuable insight on the vulnerability of marine predators to shifting prey and predation landscapes that may be expected under future climate change.
2 Methods
2.1 Study species and life cycle
Like most otariids, AUFS give birth to a single pup each year and exhibit concurrent gestation and lactation, with the energetic demands of late pregnancy and peak pup provisioning coinciding (Boness and Bowen, 1996). Pupping occurs annually between November – December in the Austral spring/summer (Gibbens and Arnould, 2009b), and mature females come into estrus 6-12 d after parturition and are mated (Atkinson, 1997). Embryo implantation is delayed for 3-4 months (Boyd, 1991), with females becoming pregnant in February – March. However, ca 43% of pregnant female AUFS experience late-term spontaneous abortions (Gibbens et al., 2010). The lactation period typically lasts 10-11 months (Arnould and Hindell, 2001) but ca 10-19% of females are observed suckling pups into a second year (Hume et al., 2001).
During lactation, females adopt a central-place foraging strategy, alternating between nursing their pup onshore (averaging 2-3 d) and foraging at sea (averaging 3-7 d) (Arnould and Hindell, 2001). As such, foraging trip durations and maternal attendance periods can have a strong influence on pup growth rates and weaning masses. Offspring weaning mass can be a strong indicator of offspring survival and recruitment (e.g. Beauplet et al., 2005). Juvenile and non-reproductive females, however, are not constrained to forage near the breeding colony and thus can remain at sea for extended periods of time. Female AUFS reach sexual maturity at 3-6 years old (Arnould and Warneke, 2002) and experience reproductive senescence at approximately 20 years (ca 1 year prior to death) (Gibbens et al., 2010). However, survivorship is estimated at less than 10% for females 15 years old or older (Gibbens et al., 2010), resulting in few observations of older females.
2.2 Model overview
Stochastic dynamic programming (SDP) is an optimization technique used to solve complex sequential decision problems under uncertainty (Bellman, 1957). SDP problems can be solved at finite and infinite timelines. When solving at a finite timeline, as in the present model, backward iteration is typically used to solve the SDP (Marescot et al., 2013). The backward iteration identifies the optimal decision for each state and time of the model, assuming an individual acts in a way to maximize some expected reward, such as survival or reproductive success (Houston et al., 1988; Clark and Mangel, 2000). This component solves dynamic programming equations to determine the decisions that result in the highest expected fitness at the terminal timepoint. These equations are solved at discrete time steps working backward in time from a terminal time point with a known (or assumed) fitness function. The optimal solution can then be coupled with a forward simulation to predict individual behavior patterns over time (here foraging and reproductive behaviors) (Clark and Mangel, 2000).
In the present study, we developed an SDP model coupled with forward simulations to predict the effects of novel changes in prey availability and at-sea mortality risk on the behavioral and reproductive choices of adult female AUFS that may be expected under future climate change. Here, AUFS were modelled to optimize lifetime reproductive success through accumulated offspring recruitment. Since perturbations were only included in the forward simulations, we are modeling a mismatch between the optimal decisions and the environment that they encounter (i.e. what they encounter is different than what they expect to encounter), potentially leading to suboptimal behavioral decisions. While climate-mediated changes in prey and predation field would have been occurring over time, the selective pressures of rapid environmental change would generally not occur within the evolutionary timeframe of long-lived species, such as AUFS. The full details of the model timeline and structure can be found in Supplementary Material-A.
2.3 Model timeline
The model ran at a daily timestep, covering the full duration of a female’s life (i.e. from age 0 to age 21). For simplicity, we have assumed a fixed date for birth and implantation, and a maximum fixed date for weaning and death. Pup birth was assumed to occur on day tB, which represents November 23rd and, thus, pups were 1 d old on model day t = 1,366, etc. Implantation was assumed to occur on day tI, corresponding to March 22nd and model day t = 120,486, etc. Offspring could be weaned at any point before the maximum weaning date of their second birthday (i.e. 730 d old).
2.4 Model decisions
In the present model, there are six reproductive states that a female can be in throughout her life: State 1 (non-reproductive), State 2 (pregnant), State 3 (nursing pup), State 4 (nursing juvenile), State 5 (nursing pup and pregnant), and State 6 (nursing juvenile and pregnant) (Figure 1). The reproductive decisions available to a female vary depending on age and current reproductive state (Figure 1). As female AUFS do not reach sexual maturity until 3-6 years old (Gibbens et al., 2010), modelled individuals under 3 years could only be non-reproductive. Additionally, a female could only be nursing a juvenile if she was old enough to have nursed a pup for one year (i.e. she must be at least 5 years old). While female AUFS are occasionally observed suckling a newborn pup and yearling concurrently, this is uncommon and was, thus, not allowed to occur in the model.
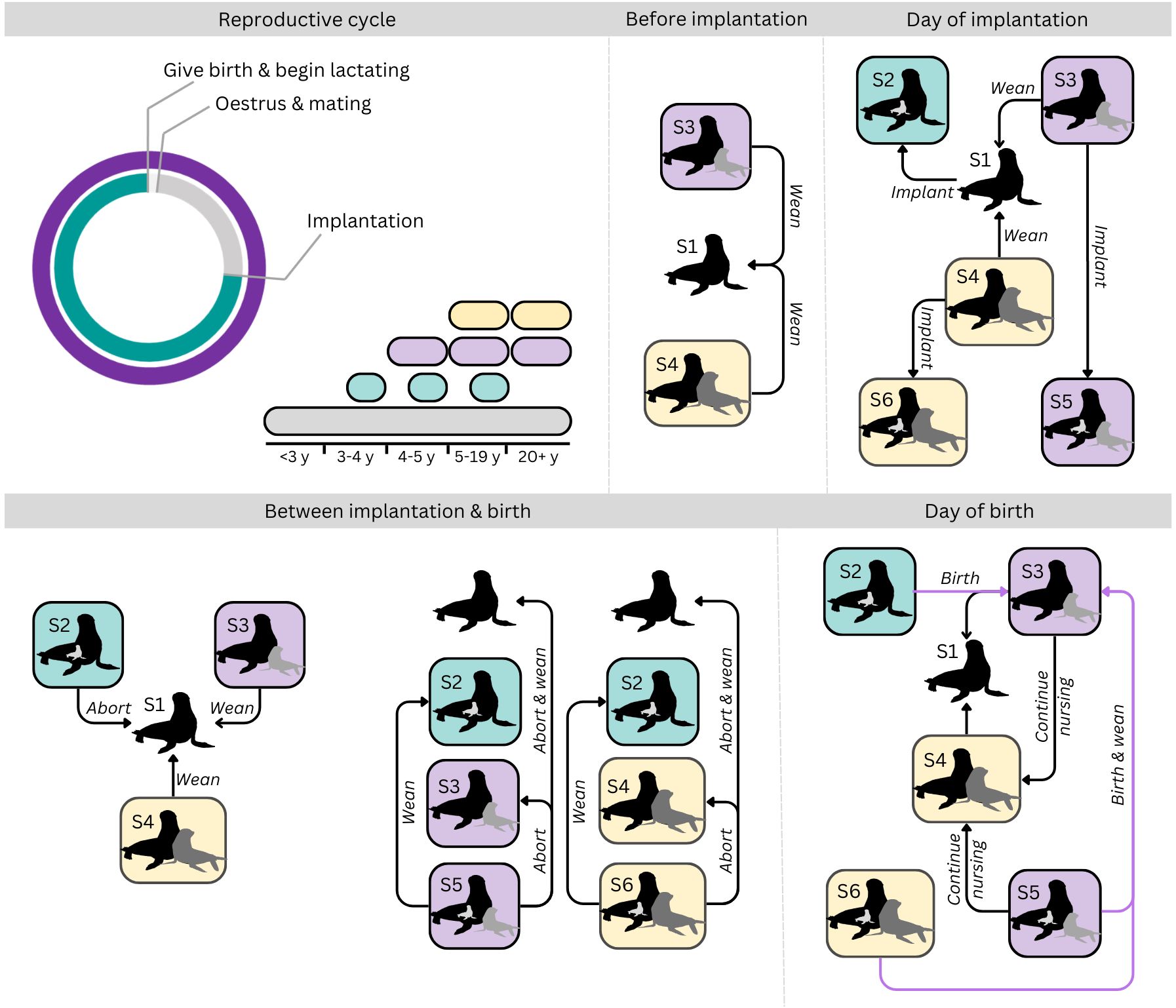
Figure 1 Visual representation of the reproductive cycle and possible reproductive decisions available to female Australian fur seals throughout the reproductive cycle. S1 – non-reproductive; S2 – pregnant; S3 – nursing a pup; S4 – nursing a juvenile; S5 – pregnant and nursing a pup; S6 – pregnant and nursing a juvenile. Arrows indicate possible state transitions for individuals in the reproductive state. For example, at the day of implantation, a female in nursing a pup (S3), may wean her pup and transition to a non-reproductive state (S1), wean her pup and implant (i.e. become pregnant; S2), continue nursing her pup without implanting (S3) or implant while continuing to nurse her pup (S5). Except for the day of birth, females may remain in their current location (land or sea) or transition to the other location. On the day of birth, females must be on land for a newborn pup to survive (S3, transitions indicated in purple), but may choose between land or sea for all other state transitions. Location based choices have been omitted from the diagram for simplicity. See Supplementary Material-D for further details.
While the model has fixed timepoints for implantation and birth, pregnant females may abort at any stage of pregnancy, returning to a non-reproductive state, and nursing females may choose to wean their offspring at any point during lactation up to the fixed maximum weaning time. Thus, non-pregnant lactating females return to a non-reproductive state and pregnant lactating females transition to a pregnant-only state (Figure 1). Pups weaned before 8 months old were considered abandoned due to their physiological immaturity (Spence-Bailey et al., 2007).
At the day of implantation, non-reproductive females and females nursing pups or juveniles may become pregnant, transitioning to State 2, 5 or 6, respectively (Figure 1) or remain in their current reproductive state.
At the time of birth, non-lactating pregnant females may give birth on land and begin nursing their pup (State 3) or may give birth at-sea, returning to a non-reproductive state (State 1). A female nursing a pup may decide to wean her pup (State 1) or continue nursing her offspring as a juvenile (State 4), while a female nursing a juvenile must wean their offspring (State 1). A pregnant female nursing a pup may wean her 1 year old pup and give birth (State 3 if on land, State 1 if at sea), wean her newborn pup and continue nursing her 1 year old as a juvenile (State 4), or give birth and wean both the newborn pup and 1 year old (State 1).
Within each reproductive state, there are two spatially implicit location-based choices that a female could make, dependent on her current physical location. At each timestep, excluding the day of birth, females on land may choose to remain on land or go to sea to forage, while females at sea may remain foraging at sea or return to land. Nursing females are, thus, deciding between improving their own condition by foraging while their offspring fasts or improving the condition of their offspring by nursing at the expense of their own condition. On the day of birth, females at sea are considered to have lost their newborn pup to drowning. As such, the model is predicting the optimal reproductive and behavioral decisions that female AUFS face throughout their lives.
2.5 Physiological dynamics
There are two physiological state variables used to determine optimal behavioral decisions, female fat mass and offspring mass (when applicable). The physiological dynamics describe how these state variables change under different behavioral and reproductive decisions. Non-reproductive and pregnant females were characterized by a single physiological state variable, fat mass (kg) at each time step, representing the energy reserves available to the female. A female’s fat mass was bounded by an age-specific minimum value and maximum value. The minimum and maximum fat mass of a female were constrained to 5% and 20% of the average age-specific body mass (kg), respectively. For example, an 80 kg female would have a minimum fat mass of 4 kg and maximum fat mass of 16 kg. If a female’s fat mass fell below the minimum value, we assumed that death by starvation had occurred. Lactating females were characterized by an additional physiological state variable, the mass (kg) of the dependent pup or juvenile at each time step. Pups and juveniles had an age-specific minimum mass, which was set to 20% below the average age-specific mass. The upper limit for pup and juvenile mass were set to 20% above the average mass at 365 d (23.4 kg) or 730 d (39.2 kg), respectively.
The physiological dynamics are comprised of two parts (energy intake and energy expenditure), which inherently differ among the different behavioral and reproductive decisions. At each timestep, each female’s fat mass was calculated considering her mass-specific field metabolic rate, growth costs, costs of gestation and lactation (if applicable), and any energy intake from foraging. Similarly, pup and juvenile masses were calculated based on their mass-specific metabolic rate, growth costs, milk intake (if nursing on land), and supplemental prey intake from foraging (if unattended). Details of the physiological dynamics can be found in Supplementary Materials-A and B.
Foraging intake for adult females was determined by their reproductive state and the probability of capturing food. Given the lack of empirical data on temporal and spatial dynamics of Australian fur seal prey and Australian fur seal prey consumption, the energy intake from foraging was calculated as a fixed multiple of a female’s gross energy requirements. For non-lactating females, this covered the costs of metabolism, growth, and, if applicable, gestation. For lactating females, this also incorporated the costs associated with nursing their pup or juvenile, assuming that the offspring was under their maximum allowable mass. The increased consumption of lactating females represents the increased requirements of females throughout lactation and is supported by the increased prey intake reported in a range of marine mammals (McHuron et al., 2023a). The metabolizable energy was then calculated from the gross energy intake accounting for fecal energy losses and urinary energy losses. The fixed multiplier was then applied to the metabolizable energy to determine the net energy intake for the female.
When offspring begin foraging, they are not as successful as adults and undergo a gradual improvement in foraging ability and physiological development (Spence-Bailey et al., 2007). As such, we assumed all offspring underwent a learning period and applied a modifier (adapted from Stephens et al., 2014) to the probability of capturing food when calculating the mass dynamics of dependent and independent offspring. Further details on the foraging intake can be found in Supplementary Material-C.
2.6 Optimal behavior developed over evolutionary time
When solving the SDP equations, we assume that individuals act in such a way to maximize some measure of Darwinian fitness. The SDP equations for this study were calculated to maximize the lifetime reproductive fitness of females through accumulated offspring recruitment (i.e. maximizing the number of offspring that survive to recruit into the population). Models were initiated with a terminal fitness (i.e. at the last model timestep) associated with offspring recruitment. Thus, the terminal fitness of nursing females is based on the offspring’s mass-specific probability of recruitment (Supplementary Material-D). Non-reproductive females have a terminal fitness of 0 since there is no possibility of increasing accumulative recruitment if non-reproductive. The model then works backward in time, solving the dynamic programming equations for all state variable combinations to identify the state- and age-dependent optimal decisions of a female. As observed empirically (Gibbens et al., 2010), very few simulated females lived beyond 16 years of age, reducing the potential effects of the terminal conditions on the model outputs. Full details on the SDP equations can be found in Supplementary Materials-D and E.
2.7 Simulating fitness consequences of novel environmental change
The optimal decisions identified in the backward iteration were used in Monte Carlo forward simulations (Mangel and Clark, 1988; Clark and Mangel, 2000) to assess the individual- and population-level consequences of environmental change for a simulated population of AUFS. Simulations were initialized with a starting population of 500 females, excluding their dependent offspring, following empirical estimates for age structure for the species (Gibbens and Arnould, 2009a). Each scenario (see below for details) was simulated for 10 years following a 1 year burn-in period with 10 replicates per scenario. Following the 1 year burn-in period, population demographics and individual behavior were stabilized and thus the effects of the initial population age and state structure on the model outputs were deemed negligible. Excluding the burn-in period resulted in non-identical starting populations for the remaining 10 years of simulation.
Females were assigned a reproductive state based on their age following empirical estimates on the prevalence of pregnant and lactating females in the AUFS population (Gibbens et al., 2010; SM-F). Overall, all females ≤3 years old were assigned non-reproductive, while 15% of sexually mature females (≥4 years old) were non-reproductive, 80% were nursing a pup and 5% were nursing juveniles. Females started the simulation at their maximum fat mass and offspring masses were drawn from normal distribution using an age-based mean and standard deviation. All new pups were randomly assigned a sex with equal probability of male or female. Only pups assigned as females were able to recruit into the simulated population, with recruitment following a mass-specific curve (Supplementary Material-D). Recruited male offspring were included in the offspring recruitment metrics for adult females but did not become simulated individuals in the population.
When a female pup or juvenile was weaned, a random number (0-1) was generated and compared with the mass-specific recruitment probability to determine if the offspring recruited successfully. If the random number was lower than the recruitment probability, the offspring is assumed to survive and recruit. At each timestep, we determined if a female had died from background mortality (mortality from non-starvation related causes) using the same approach. If the randomly generated number was lower than the age-specific mortality risk for AUFS (Supplementary Material-C), the female died. If the female had a dependent offspring over 8 months old, the offspring could recruit into the population as described above, otherwise they were removed from the simulation. Offspring that were weaned by the mother before 8 months old were considered abandoned and unable to survive independently. This threshold age was used as AUFS pups under 9 months old are not yet physiologically capable of extracting adequate resources from their environment (Spence-Bailey et al., 2007).
2.8 Scenarios
To predict the individual- and population-level responses in AUFS to changes in prey availability and/or distribution, we modified the probability of capturing food and the number of productive areas per day from ‘baseline’ conditions (the foraging conditions used in the backward iteration to determine optimal decisions and in the initial forward simulation). While AUFS are benthic foragers that dive continuously for prey at the seafloor, there is limited data on the prey field within Bass Strait. As such, simplified proxies for prey availability have been used in this model. Here, the probability of capturing food represents the abundance of prey within productive areas (i.e. areas where prey are available). In contrast, the number of productive areas encountered is a proxy for prey distribution and overall abundance, with fewer productive areas representing more patchily distributed prey while more evenly distributed prey are represented by more productive areas. Under baseline conditions, females encountered four productive areas per day with a cumulative probability of 85% for capturing prey. When individuals were unsuccessful at capturing prey at a productive area, the potential intake from the area was deducted from the total daily energy intake assuming that all foraging attempts were equivalent. If females were successful at all productive areas, they gained the full amount of energy intake for the day. Since scenarios were implemented in the forward simulation while maintaining the ‘baseline’ level in the backward iteration, we are modeling a mismatch between the environment used to determine optimal behaviors and the encountered environment. That is, the output of forward simulations under these different scenarios is the sequence of behaviors that emerge when seals make decisions in a new environment using optimal behaviors from another environment. This is a natural first step in exploring responses to climate change, as we assume that initially animals experiencing novel environmental conditions will not necessarily have good knowledge of the prey landscape.
The probability of capturing food was modified by ±5% and ±10% from the baseline level (85%). Additionally, the number of productive areas encountered was set to 2, 4, 6 or 8 per foraging day. While AUFS are benthic foragers that continuously dive in search of prey during foraging bouts, there was limited change in model outputs when exceeding 8 productive areas. These scenarios were selected to simulate potential environmental changes arising from climate change. For example, existing prey species may shift their distribution outside of the current AUFS foraging range, thus reducing prey availability. Alternatively, there may be an increased abundance of adaptable species or movement of new prey species into the foraging region. As such, we have modelled prey simulations that both worsen and improve foraging conditions for AUFS. The extent of change in the number of productive areas and the foraging success used in the present study may over-estimate the effects of climate-mediated changes in prey landscape. However, due to the poor understanding of the prey field in the foraging range of AUFS, and an even poorer understanding of how climate change may influence this prey field, we have chosen to explore the likely possible extent of change that may be observed in the region. Further data collection on the prey field throughout Bass Strait, Victoria is needed to be able to incorporate more realistic prey landscapes into the model.
The potential additive effects of novel mortality pressures (e.g. predation risk, fisheries-related mortality, disease incidence) and changes in prey availability were investigated by modifying the daily at-sea mortality risk in the forwards iteration in combination with the prey change scenarios above. The age-specific at-sea mortality risk was increased by 5% and 15% above the baseline level and, thus, represented an at-sea mortality risk 10% and 20% higher than the on-land risk. Changes in prey intake that alter the time spent at sea foraging will, plausibly, result in different exposure levels to mortality pressures, which may have an additive effect on the population dynamics of AUFS.
A total of 36 scenarios were investigated using a combination of each of the scenario components (probability of capturing prey, number of productive areas, and at-sea mortality risk) (Table 1). This number includes the baseline scenario with an 85% probability of capturing prey, four productive areas encountered per foraging day, and an at-sea mortality risk that was 5% above the on-land mortality risk. For each scenario, we calculated summary statistics (mean ± SD) per replicate, including trip and shore durations, pup growth rates, lactation duration, pup wean mass, age at first reproduction, lifetime reproductive output, reproductive rates (pregnancy, abortion, nursing, concurrent lactation and gestation, abandonment, recruitment), and survival rates. The resulting values were averaged across all replicates for each scenario.
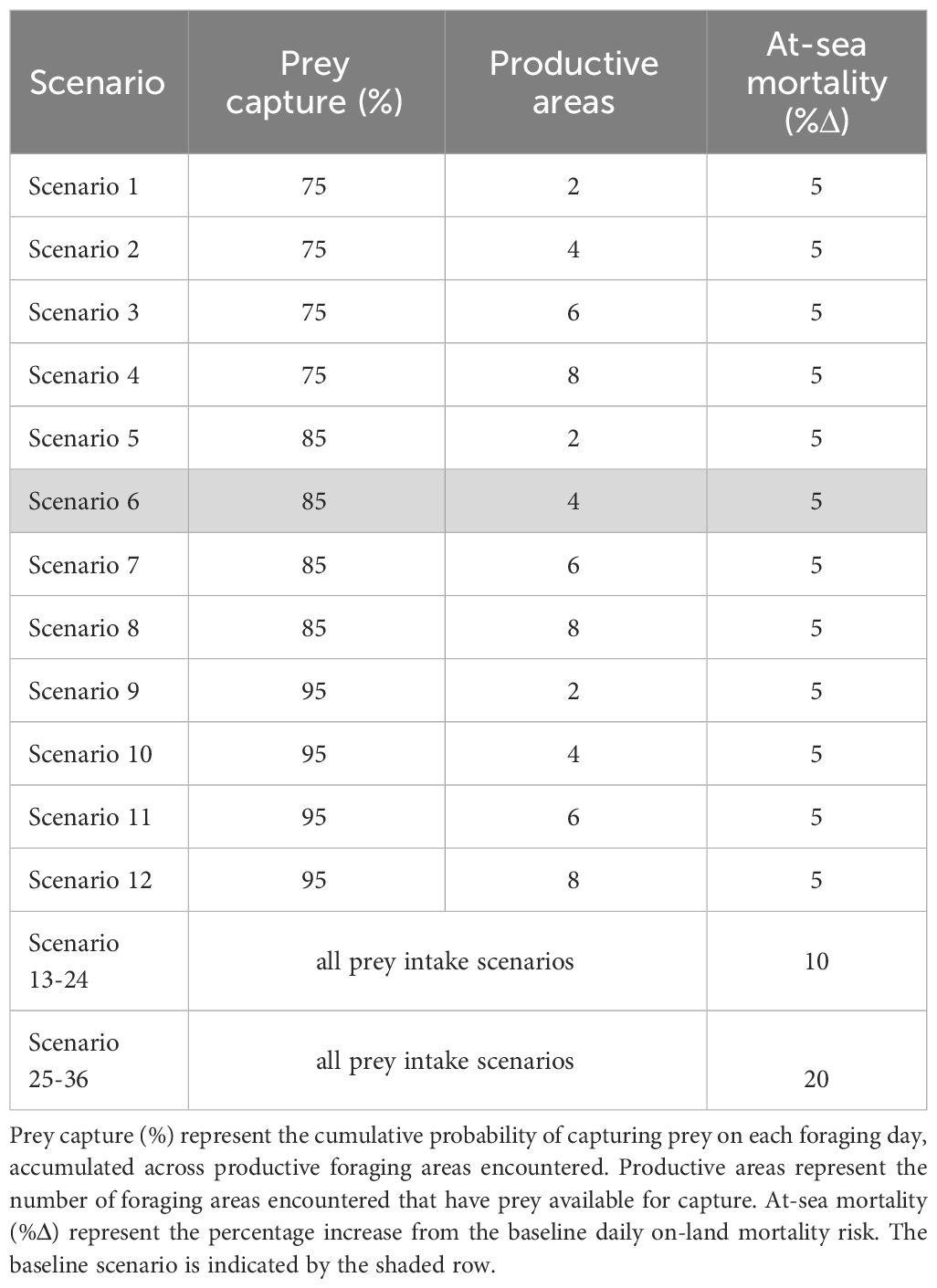
Table 1 Forward simulation scenarios used to investigate the effects of changes in at-sea mortality risk and prey availability on the behavioural and reproductive decisions of female Australian fur seals.
2.9 Sensitivity analyses
We used Cohen’s d statistic to determine the effect of uncertain parameters on the behavioral and reproductive decisions and, thus, the population dynamics, of female AUFS. Cohen’s d calculates the difference between means in the simulation outputs between the baseline and modified parameterizations scaled by their pooled standard deviation (Cohen, 2013). Parameters that were assessed included the pup, juvenile and female metabolic rates, and the shape of the recruitment function. Metabolic rates were modified by ±10%, ± 20%, and ±40%. Except for +40%, which was just outside of the reported range for marine mammals, this resulted in adult metabolic rates within the range documented for marine mammals (Noren and Rosen, 2023). These values were used due to the lack of certainty in the baseline metabolic rates used in the model since these were derived from related species from a different environment (Williams et al., 2007; McDonald et al., 2012; McHuron, 2016). For recruitment, the shape of the recruitment function curve was modified to be either shallower or steeper than the curve used for model simulations. The shallower curve meant that there was a greater range of offspring masses that could theoretically recruit, while the steeper curve narrowed the range of potential weaning masses. All parameter changes were implemented in both the backward iteration and forward simulation. Details on the sensitivity analyses can be found in Supplementary Material-G. We computed mean values by averaging the mean abortion, pup abandonment, pup recruitment, and female survival rates across simulation replicates. Values of Cohen’s d of 0.2, 0.5 and 0.8 were taken to indicate small, moderate, and large effect sizes, respectively (Cohen, 2013).
3 Results
3.1 Maternal attendance and foraging trip durations
Under baseline conditions, simulated non-reproductive females spent on average 3.4 ± 11.0 d on land and 24.4 ± 90.5 d at sea foraging and pregnant females spent 2.2 ± 5.2 d on land and 3.1 ± 5.8 d at sea foraging. Thus, non-reproductive and pregnant females spent an average of 87.8% and 58.5% of their time at sea, respectively. It is important to note that non-reproductive females include non-pregnant females that have recently weaned their offspring and are, thus, regaining condition. Females nursing pups spent less time on land (1.3 ± 0.6 d) and at sea (2.9 ± 1.8 d), with an average of 69.0% of their maternal cycle spent at sea. Likewise, pregnant females nursing pups spent 1.0 ± 0.3 d on land and 2.5 ± 1.9 d at sea (i.e. 71.4% of their maternal cycle was spent at sea). Simulated maternal attendance periods and foraging trip durations for lactating females fell within the reported ranges for the species (0.1-8.4 d and 0.3-21.8 d, respectively) (Arnould and Hindell, 2001). Estimates for other reproductive states are not currently available. There were no instances of juvenile nursing beyond the initialization year.
There was minimal effect of each prey scenario on the maternal attendance periods or foraging trip durations for females nursing pups or pregnant females nursing pups (Figure 2). The exception for non-pregnant females nursing pups being under the worst foraging conditions, which resulted in foraging trips averaging 4.1 ± 5.0 d. However, this resulted in only a 2.9% increase in the proportion of time spent at sea from baseline conditions. For pregnant females, both on land resting periods and foraging trip durations increased in duration as foraging conditions improved (i.e. with increasing probability of capturing prey and more productive areas). On-land and at-sea periods ranged from 1.3 ± 1.8 d and 2.7 ± 3.7 d, under the worst foraging conditions, to 3.9 ± 8.2 d and 4.4 ± 8.0 d, under the best foraging conditions, respectively. This changed the proportion of time spent at sea by -5.5% for the best foraging conditions and by 9.0% for the worst foraging conditions. Non-reproductive females, including independent juveniles, also had longer on land and at sea periods under better foraging conditions than under poorer foraging conditions. For example, under the best and worst foraging conditions, non-reproductive females spent 6.6 ± 17.2 d vs 1.5 ± 3.6 d on land and 50.3 ± 131.0 d vs 5.9 ± 33.6 d at sea. This represented a change in the proportion of time spent at sea of 0.6% and -8.0% under the best and worst foraging conditions, respectively.
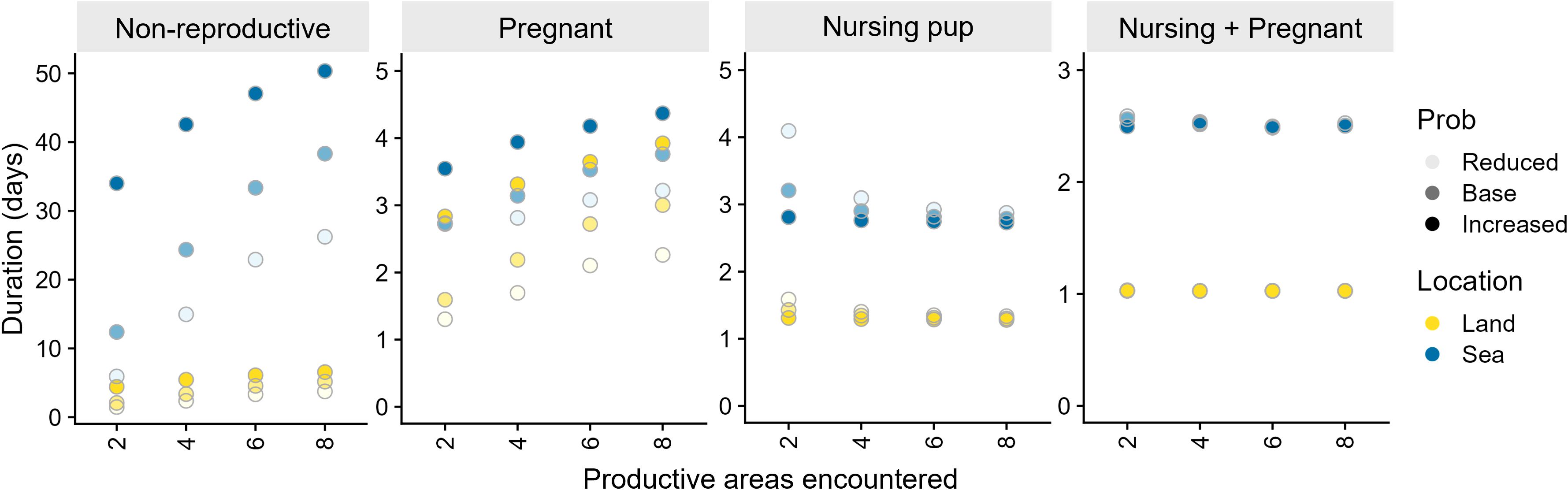
Figure 2 Influence of prey availability scenario on the duration spent on land or at sea for simulated Australian fur seal females in each of the reproductive states. No simulated females nursed juveniles. Productive areas encountered indicates the number of productive foraging areas encountered per foraging day and Prob (shading) indicates the probability of prey capture.
3.2 Growth rates
Pups gained an average of 13.6 ± 8.3 kg during the lactation period with pup growth rates averaging 48.9 ± 22.9 g·d-1 under baseline conditions. These pup growth rates were slightly below those reported for the species [53-62 g·d-1; (Arnould and Hindell, 2002)]. Females had an average fat mass loss of 2.7 ± 2.6 kg during the lactation period under baseline conditions, representing a loss of 7.4 ± 7.3 g·d-1 from body mass stores. Foraging conditions had a strong influence on pup and female mass changes. Female mass losses were lower and pup mass gains were higher under better foraging conditions. Reducing the number of productive areas encountered to 2 per foraging day had the greatest effect on pup and female mass changes. Females lost up to 2.8 kg or 7.6 g·d-1 more when reducing from 4 to 2 productive areas per day, except when the probability of capturing food within the productive areas was increased to 95% where losses were lower (2.3 kg or 6.2 g·d-1). Likewise, pup mass gains were up to 9.0 kg or 23.0 g·d-1 lower when reducing from 4 to 2 productive areas encountered per day, except for under increased probability of prey capture (1.1 kg or 1.9 g·d-1).
3.3 Lactation and weaning
Under baseline conditions, excluding females that abandoned their pups before 8 months (243 d) old, females nursed their pups until 316.1 ± 12.6 d old and 26.6 ± 0.7 kg. As mentioned previously, no juveniles were nursed beyond the initialization year. Pups were abandoned at 107.3 ± 75.5 d old and 14.2 ± 5.7 kg on average. When combining pups that were weaned and abandoned, the average weaning age was 189.5 ± 118.0 d old and 19.1 ± 7.5 kg.
For pups that were weaned after 9 months old, there was little variability in the mean age (315.4-320.0 d) or mass (26.5-26.6 kg) at weaning between all prey and mortality scenarios. Pups were abandoned earlier and at lower masses, on average, under poorer foraging conditions (67.9 ± 65.3 d old and 11.0 ± 5.1 kg for the worst-case scenario) than under better foraging conditions (123.5 ± 76.8 d old and 15.7 ± 5.5 kg for the best-case scenario). When accounting for all pups that were weaned, abandoned, or whose mother died before reaching 9 months old, average weaning age and mass increased with improved foraging conditions. Under the worst-case scenario, pups stopped being nursed at 174.4 ± 134.0 d old and 17.6 ± 8.6 kg. In contrast, pups under the best-case scenario stopped being nursed at 200.3 ± 111.6 d old and 20.1 ± 6.8 kg.
3.4 Reproductive decisions
Under baseline conditions, an average of 95.5 ± 1.0% of adult females were pregnant, all adult females nursed pups for at least some period, and 78.1 ± 8.6% of adult females (over 4 years old) were concurrently pregnant and lactating. Mid-gestation pregnancy rates and lactation rates in AUFS have been estimated at 83.8% and 84.9% of adult females, respectively, at the Kanowna Island breeding colony (Gibbens et al., 2010). No pregnancies were aborted, compared with the ca 43% reported for AUFS, however 8.8 ± 1.7% of pregnancies were lost due to death of the pregnant female. Pup abandonment rates averaged 17.8 ± 8.6%, while 8.5 ± 1.7% of females nursing pups died before their offspring was old enough to survive independently. This resulted in an average pup loss of 26.3% under baseline conditions. An average of 58.3 ± 6.7% of offspring were successfully recruited into the population at 1 year old, with 18.2 ± 2.8% of pups surviving to at least 3 years old. This represents an average addition of 32.2 sexually mature females to the simulated population per year. No simulated females in the baseline scenario nursed juveniles, compared with the ca 10-57% of females reported nursing juveniles at the natal colonies (Hume et al., 2001).
There was minimal effect of foraging conditions on the number of females that were pregnant or nursing a pup, and on the number of pregnancies aborted. However, foraging conditions had a notable effect on the proportion of adult females that were concurrently pregnant and lactating (Figure 3), ranging from 27.4 ± 14.1% under the worst foraging conditions to 92.3 ± 2.7% under the best foraging conditions. This was largely due to the influence of foraging conditions on pup abandonment rates (Figure 3). Pup abandonment rates increased from 3.3 ± 2.4% to 69.9 ± 14.6% with worsening foraging conditions. Consequently, pup recruitment rates reduced from 69.1 ± 3.2% to 20.4 ± 10.7% with worsened foraging conditions (Figure 3), though survival to three years showed a less marked effect (22.5 ± 2.2% to 5.3 ± 2.6%).
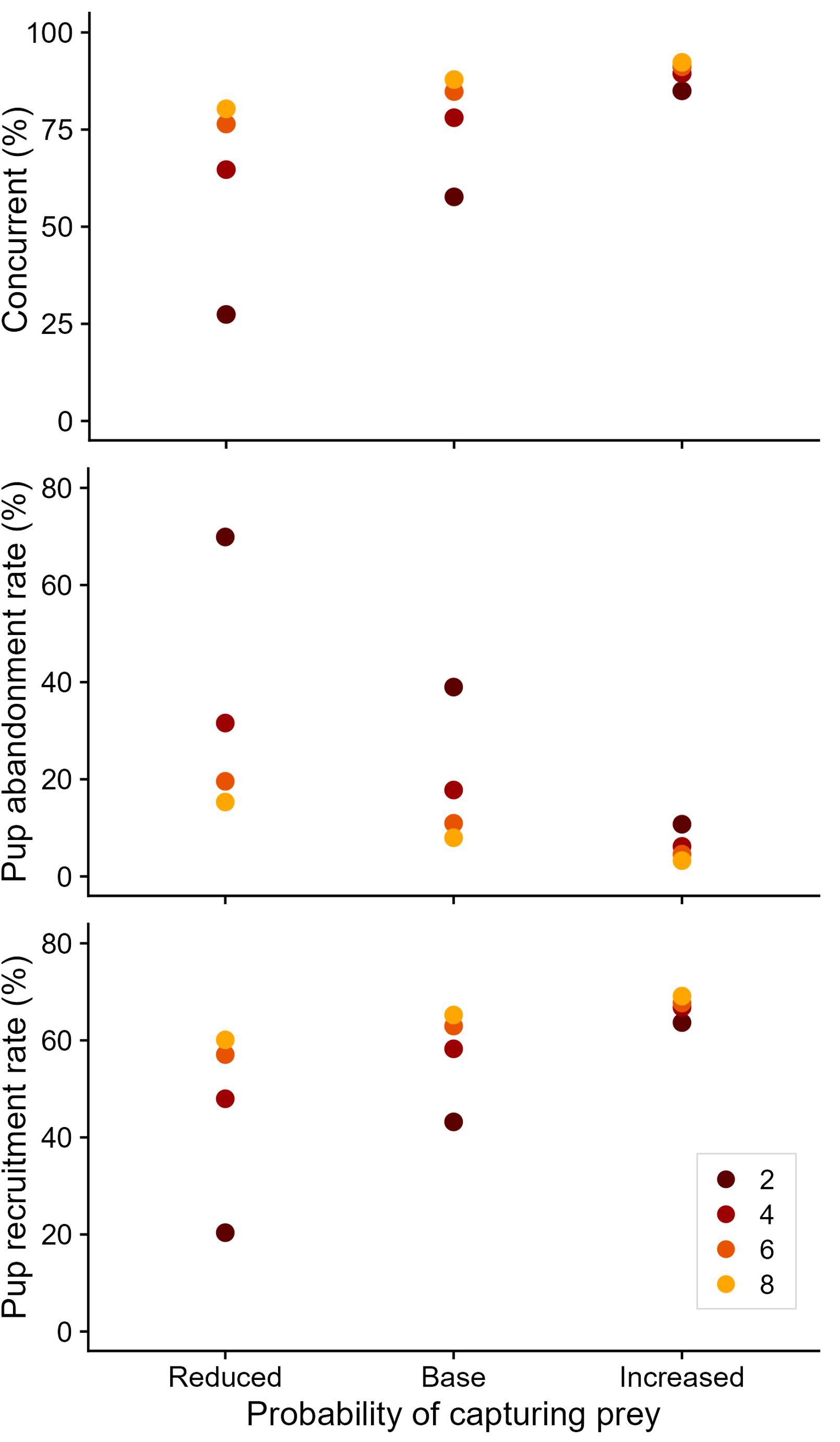
Figure 3 Influence of prey availability scenario on reproductive rates for simulated Australian fur seal females. Concurrent (%) refers to the proportion of sexually mature females that are concurrently pregnant and nursing a pup. Colors represent the number of productive foraging areas encountered per foraging day. Other reproductive rates were unaffected by simulated prey availability.
Increased at-sea mortality risk resulted in higher pregnancy and pup losses via maternal death (Figure 4), increasing by 5.9% and 5.8% from baseline levels, respectively. These losses had flow on effects for overall pup recruitment due to fewer pups being born and subsequently recruiting. The combined effect of poorer foraging conditions and higher at-sea mortality risk had substantial impact on offspring survival to three years of age (Figure 5). Under the best-case foraging conditions, three-year recruitment rates were reduced by 6.4% between the baseline and highest at-sea mortality risk. This constituted a reduction in sexually mature females added to the population from 44.0 to 20.4 per year (Figure 5). The combined influence of high at-sea mortality and low prey availability resulted in just 3.0 adult females recruiting into the simulated population.
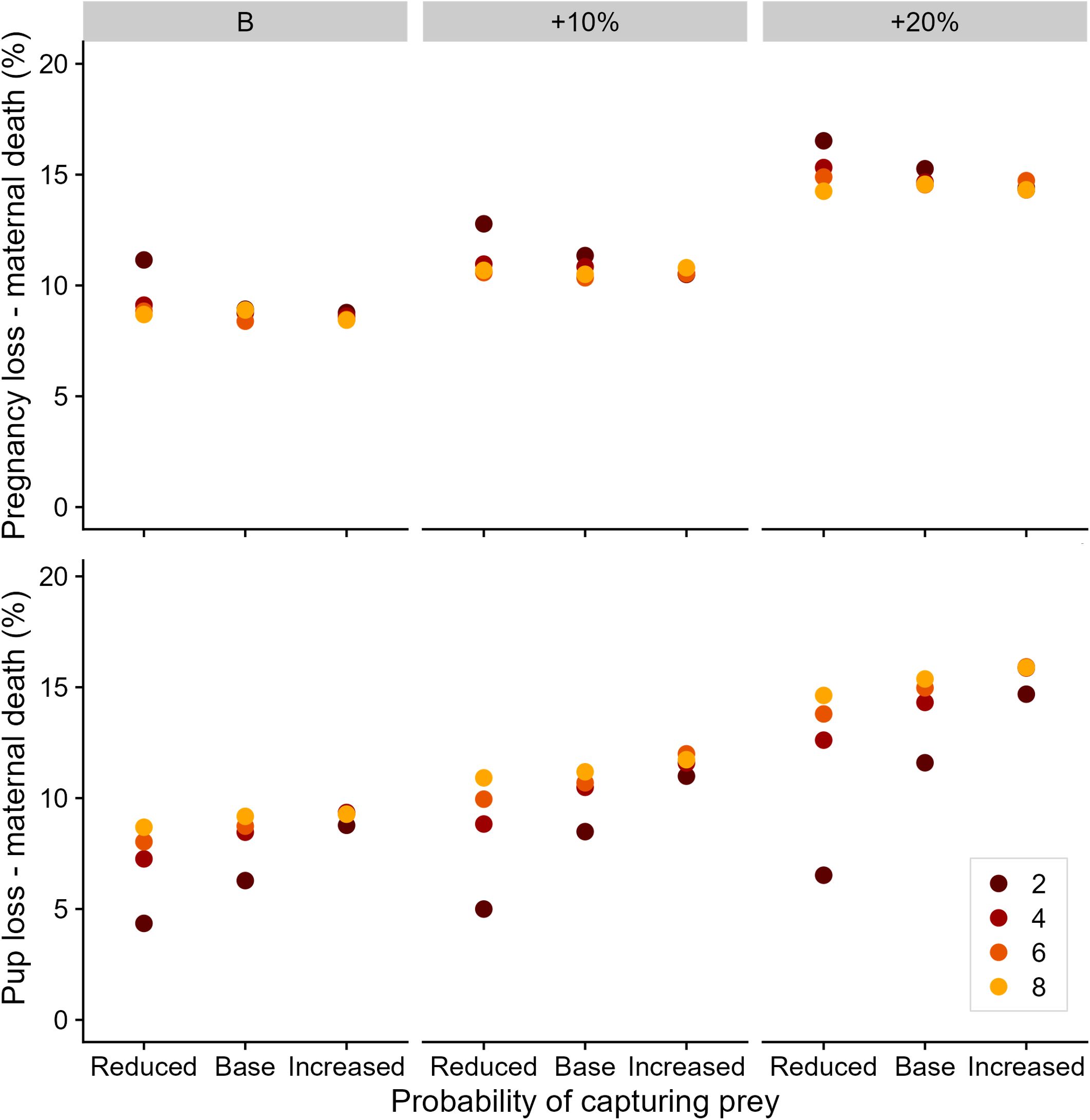
Figure 4 Influence of prey availability and mortality scenario on the proportion of offspring losses due to maternal death for simulated Australian fur seals. Colors represent the number of productive foraging areas encountered per foraging day. The paneling represents the at-sea mortality risk scenario.
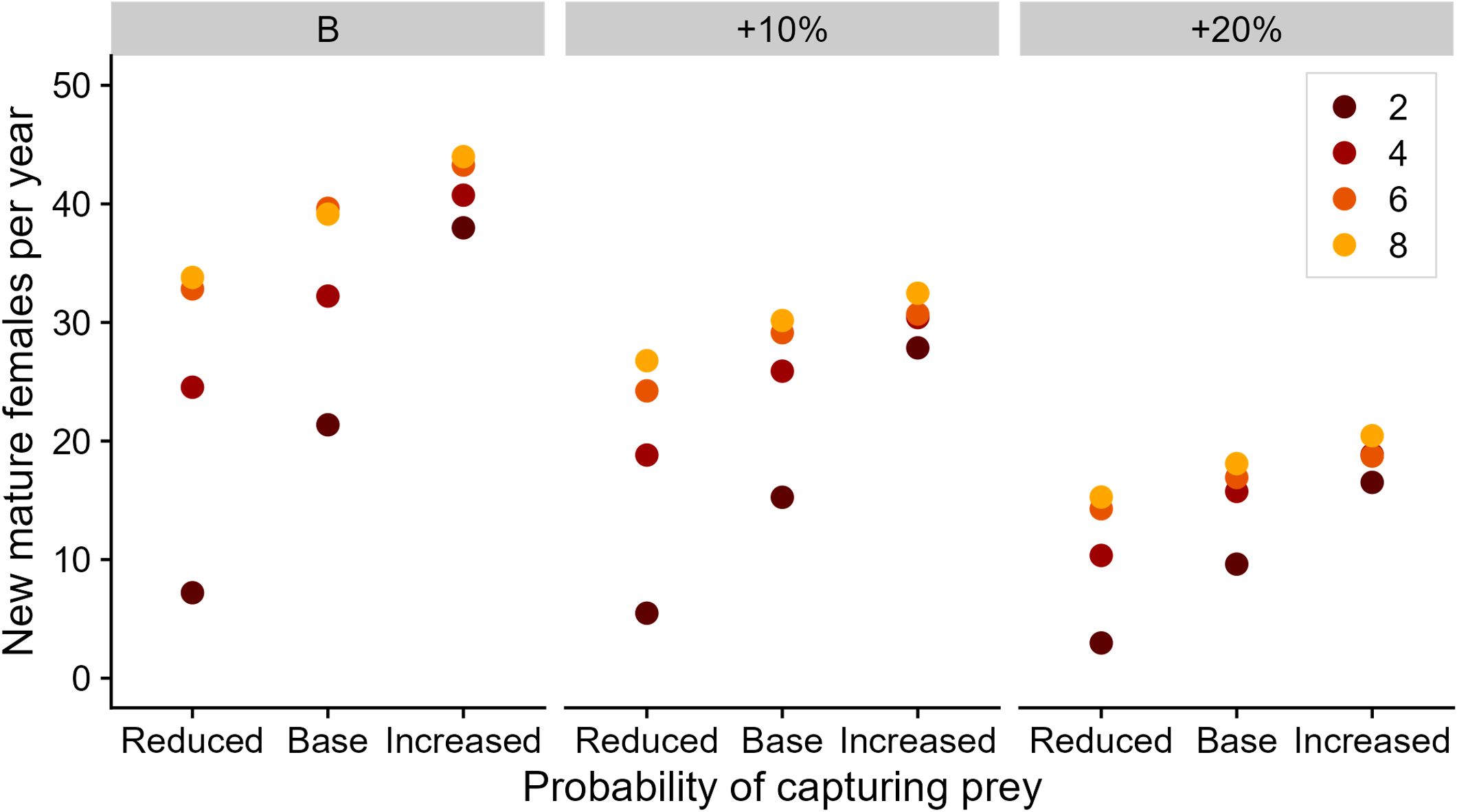
Figure 5 Influence of prey availability and mortality scenario on the average number of female offspring recruited into the simulated Australian fur seal adult (3+ years old) population per year. Colors represent the number of productive foraging areas encountered per foraging day. The paneling represents the at-sea mortality risk scenario.
3.5 Sensitivity analysis
When assessing the sensitivity of the model output to uncertain parameters, the most notable effects related to changes in female and pup metabolic rates (Figure 6). Abortion rates were largely unaffected by changes in female, juvenile and pup metabolic rates. However, there was a moderate to large non-linear effect on abortion rates when female metabolic rates were increased or decreased by 40%, when pup metabolic rates increased by 20%, and when using the shallow recruitment curve (i.e. when the probability of recruitment varied over a larger range of weaning masses) (Figure 6). When female metabolic rates were reduced, simulated females were more likely to be concurrently pregnant and lactating, leading to trade-offs between current (pup) and future (fetus) offspring when in poorer condition. In contrast, high female metabolic rates resulted in poorer female condition throughout gestation and, thus, increased abortion rates. Pup abandonment rates were strongly influenced by female and pup metabolic rates, with lower abandonment rates when metabolic rates were reduced and higher abandonment rates when metabolic rates were increased (Figure 6). Pup weaning mass was similarly impacted by female and pup metabolic rates (Figure 6). Pup recruitment rates were moderately affected by increasing pup and female metabolic rates by 10% and 40%, respectively (Figure 6). Further increases in pup metabolic rates had a large negative effect on pup recruitment rates. Finally, juvenile nursing rates were moderately affected by the shape of the recruitment curve, exhibiting higher frequencies of juvenile nursing when using the shallow recruitment curve (Figure 6).
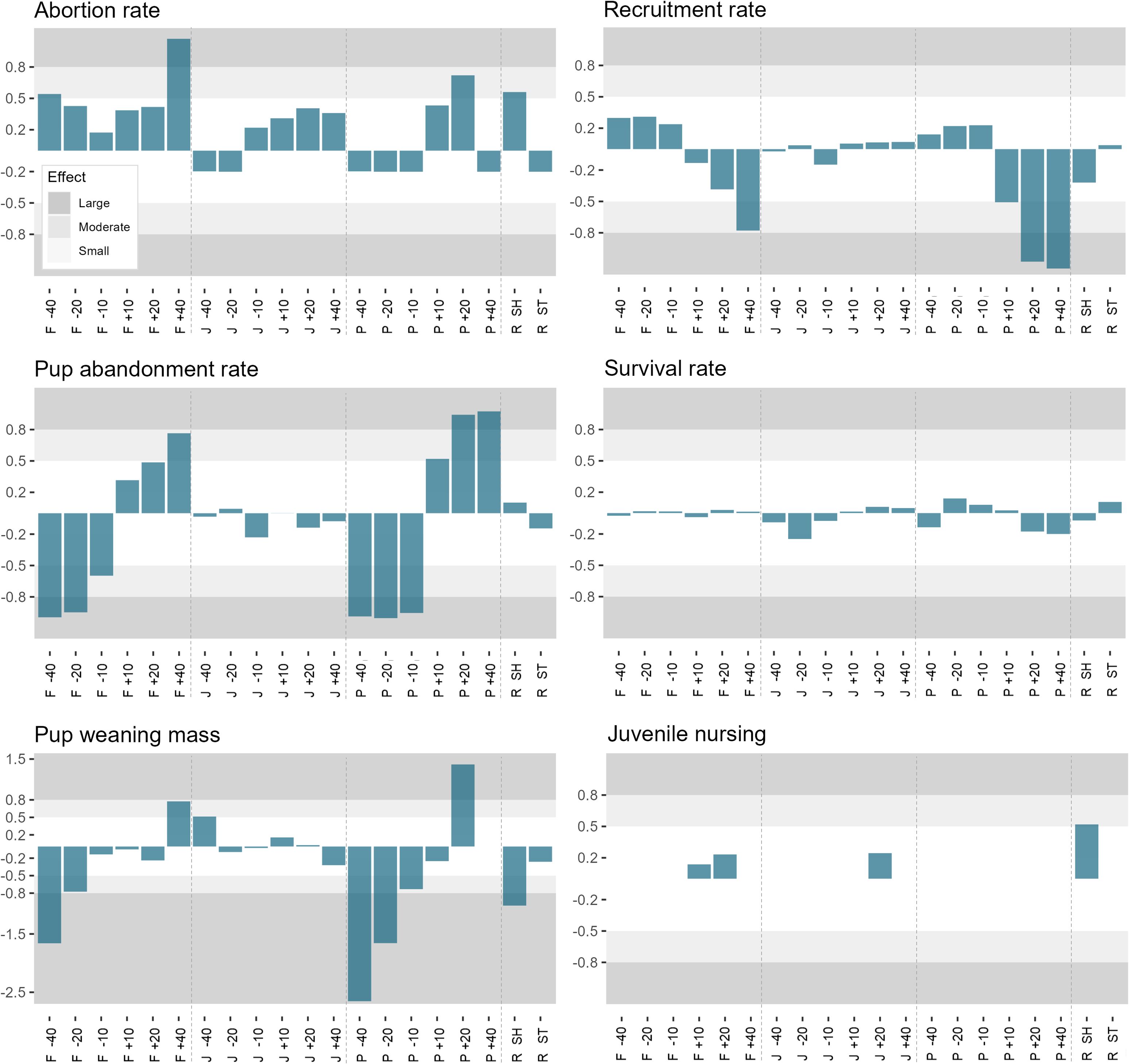
Figure 6 Outputs from the sensitivity analysis assessing the effect of metabolic rates and the shape of the recruitment curve on key model outputs. The modification for the ‘F’, ‘J’ and ‘P’ refer to changes in adult female, juvenile, and pup metabolic rates, respectively. ‘-40’, ‘-20’ and ‘-10’ represent a reduction by 40%, 20% and 10%, respectively. ‘+10’, ‘+20’ and ‘+40’ represent an increase by 10%, 20% and 40%, respectively. ‘R SH’ is the shallow recruitment curve and ‘R ST’ is the steep recruitment curve.
Sensitivity analyses also identified small to moderate effects of female and pup metabolic rates on the foraging trip and maternal attendance periods (Supplementary-G Figure 4; Supplementary-G Table 2). Maternal attendance periods were shorter when pup or female metabolic rates were reduced, and longer when pup or female metabolic rates were increased. Likewise, foraging trip durations followed a similar pattern, except when pup metabolic rates were reduced, where foraging trips were longer than under baseline parameterization.
4 Discussion
A dynamic model was developed to investigate the individual- and population-level consequences of changes in prey availability and mortality risk on a marine predator, the AUFS. Under anticipated environmental change, many marine species are expected to experience range shifts that may alter the availability and quality of prey species resulting in increased competition for resources. Range shifts may also influence the abundance or distribution of predators, with potential impacts on mortality from predation. As such, marine central place foraging predators, like the AUFS, may be vulnerable to combined ecosystem-level effects of climate change. The state-dependent model developed here provides a foundation for understanding the effects of environmental perturbations in a region being rapidly impacted by climate change. The interactive effects of prey intake and mortality risk observed in the simulated AUFS population suggest that the species may be vulnerable to future climate impacts.
Foraging trip and on-land durations of non-lactating females, as well as the proportion of their time spent at sea, were more strongly impacted by changes in prey availability than for lactating females, with minimal effect of prey availability on the foraging and maternal attendance periods of simulated lactating females. This was counterintuitive to what was expected, as evidence from lactating otariids indicate that females often extend foraging trip durations under poor environmental conditions, which reduces pup provisioning and growth rates (e.g. Beauplet et al., 2004). The lack of a strong behavioral response to poor foraging conditions in our model may have in part been due to the specific values used to parameterize the model. For example, interactions between starvation limits and pup metabolic rates may have constrained trip durations to a narrow range, such that females had little flexibility to alter foraging trip or shore durations and still remain lactating. Indeed, the sensitivity analyses indicated that changes in pup metabolic rates did have small to moderate effects on trip and shore durations. These findings deserve further exploration to fully tease apart the contributing factors, such as implementing the prey scenarios in both the backward iteration and forward simulation to examine the optimal behavioral and reproductive responses to altered environments. The proportion of time spent at sea varied greatly for pregnant females, ranging from 53% to 68% from best to worst foraging conditions, suggesting that behavioral responses were necessary to cope with altered foraging conditions during a time when energy requirements are increasing. While pregnant females were able to adjust to simulated changes in foraging conditions, there is an upper limit to this flexibility, suggesting that pregnant females may be vulnerable to adverse effects associated with climate change.
Baseline pup growth rates were consistent with empirical estimates, with simulated pup growth rates slightly below those reported for AUFS pups (Arnould and Hindell, 2002). Simulated growth rates were closer to empirical estimates when prey availability was increased, indicating that pup growth data may have been collected during a period of high prey availability. The average weaning mass (26.6 kg) and age (316.1 d) for simulated pups were consistent with those calculated using pup birth masses, growth rates, and weaning ages reported for AUFS [ca 23.8-30.6 kg; (Arnould and Hindell, 2002)]. However, pup weaning mass was highly sensitive to changes in pup and female metabolic rates and, to a lesser extent, the shape of the recruitment curve. The influence of metabolic rates on the weaning mass may be associated with the effect of metabolic rates on pup abandonments (i.e. due to fewer/more pups being abandoned, which may skew the average weaning mass higher or lower).
While the model simulations overestimated the proportion of adult females that were pregnant or nursing pups, abortion rates were considerably lower than those reported for the species. The discrepancy in the simulated and reported abortion rates suggest that abortion is likely driven by other factors than energy balance (e.g. vitamin and/or mineral deficiencies or disease). For example, abortion has been attributed to disease in other marine mammals, including bottlenose dolphins Tursiops truncatus (Miller et al., 1999) and California sea lions Zalophus californianus (Goldstein et al., 2009). Disease is also suspected to drive the high rate of late-term abortions in AUFS (Lynch et al., 2011; Gardner et al., 2022). We may have observed lower simulated pregnancy and, thus, nursing rates if implantation was able to fail (i.e. gestation did not begin). That is, we have assumed that all females of reproductive age could become pregnant if they chose to, whereas implantation failure would likely occur in wild animals. Future models could incorporate some chance of implantation failure, though the rate of failed implantation in AUFS is unknown.
While there are currently no empirical estimates for pup abandonment rates for AUFS, it is presumably lower than those simulated here (ca 18%). This assumption is due to the high late-term abortion rate, which, in combination with abandonment rates of this magnitude, would lead to population growth rates far below those previously estimated for the species [ca 5%; (Kirkwood et al., 2005)]. The baseline simulations instead produced population growth rates of approximately 3%. However, recent studies have reported declining pup production at some AUFS colonies with potential reductions in population growth (McIntosh et al., 2022). Simulated three-year recruitment rates (i.e. recruitment into the adult population) under most baseline mortality scenarios were consistent with previously modelled estimates (ca 20%) for AUFS (Arnould et al., 2003). Sensitivity analyses revealed moderate to large effects of female and pup metabolic rates on abandonment and recruitment rates. Specifically, abandonment rates increased with increasing metabolic costs, and, to a lesser degree, recruitment rates decreased with increasing metabolic costs. The lack of a strong behavioral response to poor foraging conditions could have exacerbated these abandonment rates. Being able to compensate and reduce pup growth rates allows females to hang on to their pups for longer, potentially getting them closer to the age at which they might be able to successfully recruit, albeit likely at a lower probability given they would have reduced body mass.
Juvenile nursing rates were moderately affected by shifts in the recruitment curve to a shallower curve, which resulted in an increase in juvenile nursing from 0% to 6% of females nursing pups. The increased prevalence of juvenile nursing occurred exclusively in females 4-8 years of age, with up to 40% of 4-year-old females continuing to nurse their pups as juveniles. This age effect makes sense energetically, since young females are more likely to struggle with the energetic demands of lactation due to their smaller mass and lower experience levels. Extended juvenile nursing is suggested to provide fitness benefits to the mother following newborn pup mortality (Hume et al., 2001; Trillmich and Wolf, 2008). The structuring of the present model did not account for newborn mortality, except for instances of maternal death, and thus could not represent juvenile nursing following newborn loss. Still, there is a potential that extended maternal investment may buffer the AUFS population to future environmental change. Indeed, juvenile nursing was reportedly considerably higher at the Judgement Rocks breeding colony (51-57%) than the Kanowna Island breeding colony (10-19%), potentially due to high pup mortality from exposure to storm-wave activity (Hume et al., 2001). However, we still do not fully understand the dynamics of mother-juvenile nursing interactions, particularly regarding the offspring side of the relationship. Some juveniles may be more aggressive or disinterested in weaning and some females may be less assertive with weaning, resulting in juveniles that continue to nurse beyond when their mother attempted weaning (Trillmich and Wolf, 2008). Further exploration should examine how optimal behaviors change under different prey scenarios (i.e. by implementing prey scenarios in the backward iteration) to see if we might expect juvenile nursing to become more prevalent under long-term shifts in foraging conditions.
The sensitivity of key model outputs to changes in female and pup metabolic rates highlights a need to collect species-specific field metabolic rates for AUFS. This is a common issue for marine mammal bioenergetics models and such models vary in their sensitivity to metabolic demands. For example, simulated harbor porpoises, Phocoena phocoena, were mildly sensitive to changes in metabolic rates (Gallagher et al., 2018) while simulated blue whales, Balaenoptera musculus, were highly sensitive to such changes (Pirotta et al., 2018). As is often done for marine mammal bioenergetics models, the metabolic rates used for this model are based on closely related species. However, relatedness does not always indicate that the species is an appropriate proxy for the parameter in question. Differences in foraging mode, growth rates, habitat, and climate, among other factors, can all influence metabolic rates. Likewise, methods used to measure metabolic rates can vary in reliability and in what energetic costs (e.g. thermoregulation, digestion, etc.) are encapsulated in the estimate (Noren and Rosen, 2023). Nonetheless, the model was most sensitive to large changes in the metabolic rates. While the majority of metabolic rates assessed were within the range reported for marine mammals (Noren and Rosen, 2023), the extremes (± 40%) are unlikely to be representative of the metabolic costs for AUFS. The model was also moderately sensitive to the shape of the recruitment curve used and, as such, data linking pup weaning mass, weaning age, and recruitment success should be obtained for the species.
Since changes in prey and predation fields were only simulated in the forward component of the model, the results are most applicable to unexpected changes in the environment (e.g. heat waves) since animals would presumably learn from their experience of the environment. Simulations that included modifications in both the backward and forward component of the model would provide more information about how behavioral patterns might be expected to change once animals have learned about their environment. Many of the effects of climate change are likely to produce novel environments but the timescales over which individuals learn about their environment are unknown, making forecasting difficult. The model also only looks at the indirect effects of predation, since the at-sea mortality risk is not modified in the backward component and thus does not influence behavioral decisions. Here, prey landscape changes the amount of time individuals spend at sea, which changes their exposure to predators. Thus, our model predicts the combined effects of shifting prey and predation landscapes on AUFS in a novel environment.
In summary, a state-dependent life-history theory model was developed to investigate how novel changes in prey availability and at-sea mortality risk impact a central place foraging marine predator. The model highlights the trade-offs individuals face between their condition, reproductive needs, and the environment they experience. The emergent patterns from the model simulations are largely consistent with those observed in wild AUFS, providing a robust means to predict the effects of environmental change on the AUFS population. The independent and cumulative influences of prey and predation landscapes on the individual behavior and vital rates of simulated females suggest that the AUFS population may be vulnerable to future environmental change. The outcomes of this study suggest that abortion rates should be much lower than is reported for AUFS, highlighting a need for continued monitoring of potential diseases and contaminants that may be driving this reproductive failure. Further monitoring of population trends is also vital given the recent declines in pup production, and thus population growth, at some colonies (McIntosh et al., 2022). Future modelling efforts should allow individuals to learn and, thus, adjust to poor foraging conditions, by including prey availability changes in both the backward iteration and forward simulation, as well as extend the simulation time frame to enable forecasting of population trends under anticipated climate conditions.
Data availability statement
Publicly available datasets were analyzed in this study. This data can be found here: https://github.com/CassieSpeakman/AUFS_SDP_model_prey-and-predation/tree/main.
Author contributions
CS: Conceptualization, Formal Analysis, Investigation, Methodology, Validation, Visualization, Writing – original draft. EM: Methodology, Validation, Writing – review & editing. JA: Conceptualization, Supervision, Writing – review & editing.
Funding
The author(s) declare that no financial support was received for the research, authorship, and/or publication of this article.
Conflict of interest
The authors declare that the research was conducted in the absence of any commercial or financial relationships that could be construed as a potential conflict of interest.
Publisher’s note
All claims expressed in this article are solely those of the authors and do not necessarily represent those of their affiliated organizations, or those of the publisher, the editors and the reviewers. Any product that may be evaluated in this article, or claim that may be made by its manufacturer, is not guaranteed or endorsed by the publisher.
Supplementary material
The Supplementary Material for this article can be found online at: https://www.frontiersin.org/articles/10.3389/fmars.2024.1367655/full#supplementary-material
References
Arnould J. P. Y., Boyd I. L., Warneke R. M. (2003). Historical dynamics of a fur seal population: Evidence of regulation by man? Can. J. Zool. 81, 1428–1436. doi: 10.1139/z03-134
Arnould J. P. Y., Hindell M. A. (2001). Dive behaviour, foraging locations, and maternal-attendance patterns of Australian fur seals (Arctocephalus pusillus doriferus). Can. J. Zool. 79, 35–48. doi: 10.1139/z00-178
Arnould J. P. Y., Hindell M. A. (2002). Milk consumption, body composition and pre-weaning growth rates of Australian fur seal (Arctocephalus pusillus doriferus) pups. J. Zool. 256, 351–359. doi: 10.1017/S0952836902000389
Arnould J. P. Y., Warneke R. M. (2002). Growth and condition in Australian fur seals (Arctocephalus pusillus doriferus) (carnivora: Pinnipedia). Aust. J. Zool. 50, 53–66. doi: 10.1071/ZO01077
Atkinson S. (1997). Reproductive biology of seals. Rev. Reprod. 2, 175–194. doi: 10.1530/ror.0.0020175
Beauplet G., Barbraud C., Chambellant M., Guinet C. (2005). Interannual variation in the post-weaning and juvenile survival of subantarctic fur seals: Influence of pup sex, growth rate and oceanographic conditions. J. Anim. Ecol. 74, 1160–1172. doi: 10.1111/j.1365-2656.2005.01016.x
Beauplet G., Dubroca L., Guinet C., Cherel Y., Dabin W., Gagne C., et al. (2004). Foraging ecology of Subantarctic fur seals Arctocephalus tropicalis breeding on Amsterdam Island: seasonal changes in relation to maternal characteristics and pup growth. Mar. Ecol. Prog. Ser. 273, 211–225. doi: 10.3354/meps273211
Boness D. J., Bowen W. D. (1996). The evolution of maternal care in pinnipeds. Bioscience 46, 645–654. doi: 10.2307/1312894
Boyd I. L. (1991). Environmental and physiological factors controlling the reproductive cycles of pinnipeds. Can. J. Zool. 69, 1135–1148. doi: 10.1139/z91-162
Bull C. D., Metcalfe N. B., Mangel M. (1996). Seasonal matching of foraging to anticipated energy requirements in anorexic juvenile salmon. Proc. R. Soc. B: Biol. Sci. 263, 13–18. doi: 10.1098/rspb.1996.0003
Clark C. W., Mangel M. (2000). Dynamic state variable models in ecology: Methods and applications (New York, USA: Oxford University Press on Demand). doi: 10.1093/oso/9780195122664.001.0001
Clark J. S., Carpenter S. R., Barber M., Collins S., Dobson A., Foley J. A., et al. (2001). Ecological forecasts: An emerging imperative. Science 293, 657–660. doi: 10.1126/science.293.5530.657
Cohen J. (2013). Statistical power analysis for the behavioral sciences (New York, USA: Academic Press). doi: 10.4324/9780203771587
Denis D., Pierre J.-S., Van Baaren J., Van Alphen J. J. M. (2012). Physiological adaptations to climate change in pro-ovigenic parasitoids. J. Theor. Biol. 309, 67–77. doi: 10.1016/j.jtbi.2012.06.005
Gallagher C. A., Stern S. J., Hines E. (2018). The metabolic cost of swimming and reproduction in harbor porpoises (Phocoena phocoena) as predicted by a bioenergetic model. Mar. Mammal. Sci. 34, 875–900. doi: 10.1111/mms.12487
Gardner B. R., Stenos J., Hufschmid J., Arnould J. P. Y., Mcintosh R. R., Tadepalli M., et al. (2022). An old pathogen in a new environment–implications of Coxiella burnetii in Australian fur seals (Arctocephalus pusillus doriferus) 9, 809075. doi: 10.3389/fmars.2022.809075
Geeson J. J., Hobday A. J., Speakman C. N., Arnould J. P. Y. (2022). Environmental influences on breeding biology and pup production in Australian fur seals. R. Soc. Open Sci. 9, 211399. doi: 10.1098/rsos.211399
Gibbens J., Arnould J. P. Y. (2009a). Age-specific growth, survival, and population dynamics of female Australian fur seals. Can. J. Zool. 87, 902–911. doi: 10.1139/Z09-080
Gibbens J., Arnould J. P. Y. (2009b). Interannual variation in pup production and the timing of breeding in benthic foraging Australian fur seals. Mar. Mammal. Sci. 25, 573–587. doi: 10.1111/j.1748-7692.2008.00270.x
Gibbens J., Parry L. J., Arnould J. P. Y. (2010). Influences on fecundity in Australian fur seals (Arctocephalus pusillus doriferus). J. Mammal. 91, 510–518. doi: 10.1644/08-MAMM-A-377.1
Gill J. A., Norris K., Sutherland W. J. (2001). Why behavioural responses may not reflect the population consequences of human disturbance. Biol. Conserv. 97, 265–268. doi: 10.1016/S0006-3207(00)00002-1
Goldstein T., Zabka T. S., Delong R. L., Wheeler E. A., Ylitalo G., Bargu S., et al. (2009). The role of domoic acid in abortion and premature parturition of California sea lions (Zalophus californianus) on San Miguel Island, California. J. Wildlife Dis. 45, 91–108. doi: 10.7589/0090-3558-45.1.91
Grimm V., Railsback S. F. (2005). Individual-based modeling and ecology (Princeton, New Jersey, USA: Princeton University Press). doi: 10.1515/9781400850624
Hobday A. J., Pecl G. T. (2014). Identification of global marine hotspots: Sentinels for change and vanguards for adaptation action. Rev. Fish Biol. Fisheries 24, 415–425. doi: 10.1007/s11160-013-9326-6
Hoegh-Guldberg O., Bruno J. F. (2010). The impact of climate change on the world’s marine ecosystems. Science 328, 1523–1528. doi: 10.1126/science.1189930
Houston A. I., Clark C. W., Mcnamara J. M., Mangel M. (1988). Dynamic models in behavioural and evolutionary ecology. Nature 332, 29–34. doi: 10.1038/332029a0
Hume F., Arnould J. P. Y., Kirkwood R., Davis P. (2001). Extended maternal dependence by juvenile Australian fur seals (Arctocephalus pusillus doriferus). Aust. Mammal. 23, 67–70. doi: 10.1071/AM01067
Kirkwood R., Gales R., Terauds A., Arnould J. P., Pemberton D., Shaughnessy P. D., et al. (2005). Pup production and population trends of the Australian fur seal (Arctocephalus pusillus doriferus). Mar. Mammal. Sci. 21, 260–282. doi: 10.1111/j.1748-7692.2005.tb01227.x
Lima S. L. (1998). Nonlethal effects in the ecology of predator-prey interactions: What are the ecological effects of anti-predator decision-making? Bioscience 48, 25–34. doi: 10.2307/1313225
Lima S. L., Dill L. (1990). Behavioral decisions made under the risk of predation: A review and prospectus. Can. J. Zool. 68, 619–640. doi: 10.1139/z90-092
Lynch M., Taylor T. K., Duignan P. J., Swingler J., Marenda M., Arnould J. P. Y., et al. (2011). Mycoplasmas in Australian fur seals (Arctocephalus pusillus doriferus): Identification and association with abortion. J. Veterinary Diagn. Invest. 23, 1123–1130. doi: 10.1177/1040638711425699
Mangel M., Clark C. W. (1988). Dynamic modeling in behavioral ecology (Princeton, New Jersey, USA: Princeton University Press). doi: 10.1515/9780691206967
Marescot L., Chapron G., Chadès I., Fackler P. L., Duchamp C., Marboutin E., et al. (2013). Complex decisions made simple: a primer on stochastic dynamic programming. Methods Ecol. Evol. 4, 872–884. doi: 10.1111/2041-210X.12082
McDonald B. I., Goebel M. E., Crocker D. E., Costa D. P. (2012). Biological and environmental drivers of energy allocation in a dependent mammal, the antarctic fur seal pup. Physiol. Biochem. Zool. 85, 134–147. doi: 10.1086/664948
McHuron E. A. (2016). An individual-based approach to the foraging behavior and energetics of a generalist marine predator (Santa Cruz: University of California).
McHuron E. A., Adamczak S., Costa D. P., Booth C. (2023a). Estimating reproductive costs in marine mammal bioenergetic models: A review of current knowledge and data availability. Conserv. Physiol. 11, coac080. doi: 10.1093/conphys/coac080
McHuron E. A., Sterling J. T., Mangel M. (2023b). The influence of prey availability on behavioral decisions and reproductive success of a central-place forager during lactation. J. Theor. Biol. 560, 111392. doi: 10.1016/j.jtbi.2022.111392
McIntosh R. R., Sorrell K. J., Thalmann S., Mitchell A., Gray R., SChinagl H., et al. (2022). Sustained reduction in numbers of Australian fur seal pups: Implications for future population monitoring. PloS One 17, e0265610. doi: 10.1371/journal.pone.0265610
Miller W. G., Adams L. G., Ficht T. A., Cheville N. F., Payeur J. P., Harley D. R., et al. (1999). Brucella-induced abortions and infection in bottlenose dolphins (Tursiops truncatus). J. Zoo Wildlife Med. 30 (1), 100–110.
National Academies of Sciences, Engineering, and Medicine (2017). Approaches to understanding the cumulative effects of stressors on marine mammals (Washington, DC: The National Academies Press).
Noren S. R., Rosen D. A. S. (2023). What are the metabolic rates of marine mammals and what factors impact this value: A review. Conserv. Physiol. 11, coad077. doi: 10.1093/conphys/coad077
Pecl G. T., Araújo M. B., Bell J. D., Blanchard J., Bonebrake T. C., Chen I.-C., et al. (2017). Biodiversity redistribution under climate change: Impacts on ecosystems and human well-being. Science 355, eaai9214. doi: 10.1126/science.aai9214
Pirotta E., Mangel M., Costa D. P., Mate B., Goldbogen J. A., Palacios D. M., et al. (2018). A dynamic state model of migratory behavior and physiology to assess the consequences of environmental variation and anthropogenic disturbance on marine vertebrates. Am. Nat. 191, E40–E56. doi: 10.1086/695135
Reimer J. R., Mangel M., Derocher A. E., Lewis M. A. (2019). Modeling optimal responses and fitness consequences in a changing arctic. Global Change Biol. 25, 3450–3461. doi: 10.1111/gcb.14681
Satterthwaite W. H., Beakes M. P., Collins E. M., Swank D. R., Merz J. E., Titus R. G., et al. (2010). State-dependent life history models in a changing (and regulated) environment: Steelhead in the California central valley. Evolutionary Appl. 3, 221–243. doi: 10.1111/j.1752-4571.2009.00103.x
Schmolke A., Thorbek P., Deangelis D. L., Grimm V. (2010). Ecological models supporting environmental decision making: A strategy for the future. Trends Ecol. Evol. 25, 479–486. doi: 10.1016/j.tree.2010.05.001
Schwarz L. K., Goebel M. E., Costa D. P., Kilpatrick A. M. (2013). Top-down and bottom-up influences on demographic rates of Antarctic fur seals Arctocephalus gazella. J. Anim. Ecol. 82, 903–911. doi: 10.1111/1365-2656.12059
Speakman C. N., Hoskins A. J., Hindell M. A., Costa D., Hartog J. R., Hobday A. J., et al. (2020). Environmental influences on foraging effort, success and efficiency in female Australian fur seals. Sci. Rep 10, 17710. doi: 10.1038/s41598-020-73579-y
Speakman C. N., Hoskins A. J., Hindell M., Costa D. P., Hobday A. J., Hartog J. R., et al. (2021). Influence of environmental variation on spatial distribution and habitat-use in a benthic foraging marine predator. R. Soc. Open Sci. 8, 211052. doi: 10.1098/rsos.211052
Spence-Bailey L. M., Verrier D., Arnould J. P. (2007). The physiological and behavioural development of diving in Australian fur seal (Arctocephalus pusillus doriferus) pups. J. Comp. Physiol. B 177, 483–494. doi: 10.1007/s00360-007-0146-7
Stephens P. A., Houston A. I., Harding K. C., Boyd I. L., Mcnamara J. M. (2014). Capital and income breeding: The role of food supply. Ecology 95, 882–896. doi: 10.1890/13-1434.1
Tenhumberg B., Tyre A. J., Roitberg B. (2000). Stochastic variation in food availability influences weight and age at maturity. J. Theor. Biol. 202, 257–272. doi: 10.1006/jtbi.1999.1049
Trillmich F., Wolf J. B. W. (2008). Parent–offspring and sibling conflict in galápagos fur seals and sea lions. Behav. Ecol. Sociobiol. 62, 363–375. doi: 10.1007/s00265-007-0423-1
Keywords: benthic predator, bioenergetic modelling, dynamic state variable modelling, income breeding, pinniped, population dynamics, state-dependent life-history theory, stochastic dynamic programming
Citation: Speakman CN, McHuron EA and Arnould JPY (2024) Vulnerability to fluctuations in prey and predation landscape in a central place foraging marine predator. Front. Mar. Sci. 11:1367655. doi: 10.3389/fmars.2024.1367655
Received: 09 January 2024; Accepted: 18 March 2024;
Published: 27 March 2024.
Edited by:
Leslie New, Ursinus College, United StatesReviewed by:
Brigitte Tenhumberg, University of Nebraska-Lincoln, United StatesVincent Hin, Wageningen Marine Research IJmuiden, Netherlands
Copyright © 2024 Speakman, McHuron and Arnould. This is an open-access article distributed under the terms of the Creative Commons Attribution License (CC BY). The use, distribution or reproduction in other forums is permitted, provided the original author(s) and the copyright owner(s) are credited and that the original publication in this journal is cited, in accordance with accepted academic practice. No use, distribution or reproduction is permitted which does not comply with these terms.
*Correspondence: Cassie N. Speakman, Y2Fzc2llLnNwZWFrbWFuQG91dGxvb2suY29t