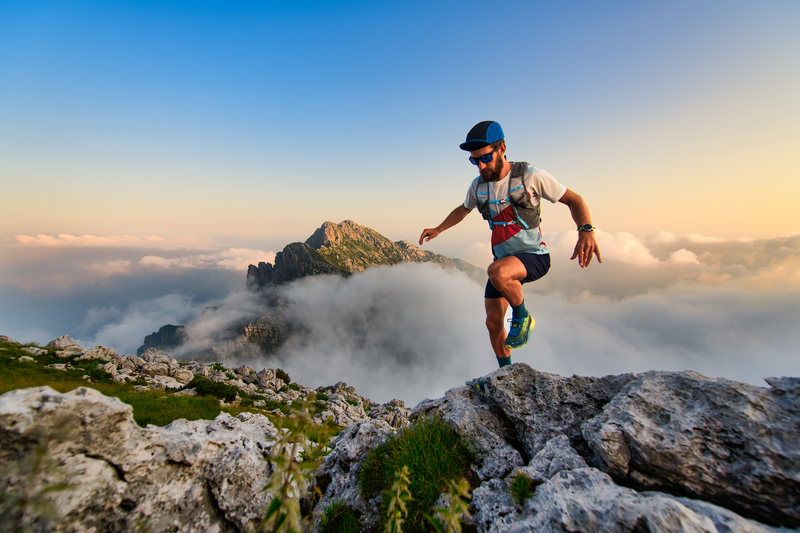
94% of researchers rate our articles as excellent or good
Learn more about the work of our research integrity team to safeguard the quality of each article we publish.
Find out more
ORIGINAL RESEARCH article
Front. Mar. Sci. , 22 November 2024
Sec. Marine Ecosystem Ecology
Volume 11 - 2024 | https://doi.org/10.3389/fmars.2024.1360541
This article is part of the Research Topic Antarctic Krill and Interactions in the East Antarctic Ecosystem View all 16 articles
During the summer of 2021, we conducted a comprehensive study on zooplankton communities along East Antarctica (55°E to 80°E) as part of the Trends in Euphausiids off Mawson, Predators, and Oceanography (TEMPO) survey program. Hierarchical agglomerative clustering identified three distinct zooplankton clusters based on environmental factors. Seven potential indicator taxa associated with specific clusters include copepods, pteropods, amphipods, and euphausiids. Mainly consisting of small copepods, chaetognaths and foraminifera, Cluster 1 (n = 34) was characterized by the highest abundance (74,386 ind./1000 m3), spanning wide latitudinal and longitudinal gradients, deeper waters (mean depth = 3,475 m ± 739 m), and higher chlorophyll-a concentrations (mean = 49.13 mg m−2 ± 24.38 mg m−2). Cluster 2 (n = 4) featured the lowest abundance (1,059 ind./1000 m3) and the fewest sampling stations along the narrowest latitudinal range. Copepods, euphausiids, and foraminifera were among the most abundant in this group. Cluster 3 (n = 10), located near the ice edge, displayed a distinct temperature range (−1.46°C to 1.18°C) and moderate zooplankton abundance (22,629 ind./1000 m3) consisting of copepods, euphausiids, and ostracods. IndVal analysis identified seven species as indicators of environmental conditions and Generalized Additive Models (GAMs) were used to model their abundance, as well as total zooplankton abundance. Across all models, significant drivers included chlorophyll-a, temperature, number of days since sea ice melt and mixed layer depth. The model for total zooplankton abundance explained 70.9% of the deviance, with number of days since ice melt and chlorophyll-a concentration emerging as the strongest predictors. These findings provide crucial insights into the ecological implications of changing climate conditions on East Antarctica zooplankton communities and their potential repercussions on the broader Southern Ocean ecosystem. This research enhances our understanding of the intricate relationship between environmental shifts and Southern Ocean ecology.
The Southern Ocean, a crucial component of global oceanic systems, is undergoing rapid transformations driven by climate change. These changes, including shifts in sea ice extent, ocean warming, and altered freshwater inputs, have widespread implications for the entire Antarctic marine food web (Constable et al., 2014; Massom et al., 2013; Poloczanska et al., 2016). Zooplankton, which serve as a critical link between primary producers and higher trophic levels, are particularly sensitive to these environmental shifts (Cavan et al., 2017; Yang et al., 2022). Fluctuations in sea ice extent, temperature regimes, and oceanographic processes can lead to changes in the abundance, distribution, and vertical structure of zooplankton communities, with significant consequences for both biogeochemical cycles and higher trophic levels (Smetacek et al., 2004).
Recent studies have identified shifts in zooplankton communities throughout the Southern Ocean, particularly in response to changing environmental conditions, such as sea ice melt and ocean warming. However, the Central Indian sector of East Antarctica, particularly the region along the Mawson coast, remains actively understudied compared to other sectors, such as the Western Antarctic Peninsula. Zooplankton communities in this region are sensitive to environmental fluctuations, particularly krill (Euphausia superba) and copepods, which serve as essential components of the Antarctic marine food web (Quetin et al., 1996; Reid et al., 2005; McCormack et al., 2020). Despite their ecological importance, gaps remain in understanding how environmental drivers such as temperature, sea ice melt, and primary productivity affect zooplankton distribution and abundance in this region.
Surveys conducted in the broader Southern Ocean, such as the BROKE-West (Nicol and Meiners, 2010) and Japanese-led krill biomass surveys (e.g., Murase et al., 2019), have provided valuable insights into the distribution and abundance of zooplankton and krill, but the Central Indian sector, including CCAMLR Division 58.4.2, has not been extensively studied. This region is vital for understanding the larger-scale implications of climate change on Antarctic ecosystems, especially given the significant role it plays in krill management and conservation efforts (Cox et al., 2022). The region’s unique oceanographic conditions, including extensive landfast ice and variable sea ice dynamics, offer a critical setting for investigating how zooplankton communities respond to shifting environmental factors (Fraser et al., 2020).
The Commission for the Conservation of Antarctic Marine Living Resources (CCAMLR) recognizes the importance of Division 58.4.2 for managing krill fisheries and ensuring the long-term sustainability of this ecologically significant region. Previous surveys (Nicol and Meiners, 2010; Swadling et al., 2010) have informed krill management strategies, but ongoing research is necessary to assess the impact of climate change on krill and zooplankton communities in East Antarctica. The objective of this study is to contribute to that understanding by examining zooplankton distribution and environmental drivers along the Mawson coast, which could have broader implications for the management of krill and other key species in the Southern Ocean.
This study aims to address critical gaps in understanding how environmental conditions influence zooplankton communities along the Mawson coast in East Antarctica during the summer of 2021. We use a combination of environmental data, cluster analysis, and generalized additive models (GAMs) to identify patterns in the structure and key drivers of zooplankton dynamics. By investigating these relationships, we contribute valuable knowledge to inform future management and conservation efforts in this ecologically significant region.
Mesozooplankton net sampling was conducted aboard the RV Investigator as part of the multidisciplinary research voyage named Trends in Euphausiids off Mawson, Predators, and Oceanography (TEMPO). On 29 January 2021, the research vessel departed Hobart, Australia and was bound for the eastern half of the CCAMLR Division 58.4.2, spanning 62°S to 68°S and 55°E to 80°E (Figure 1). Active sampling along six north-south transects occurred within the seasonal ice zone between 13 February and 12 March 2021, starting at the southwest corner of the sampling region (‘R01’ in Figure 1). The vessel returned to Hobart on 24 March 2021.
Figure 1. Locations of RMT1 + 8 plankton net deployments along six north-south transects. Deployment types were either routine or target, denoted in sampling site names by ‘R’ and ‘T’, respectively, which refers to planned and/or targeted trawls with reference to Antarctic krill sampling for biomass estimates (Cox et al., 2022). The background bathymetry grid is sourced from the GEBCO_2014 Grid, version 20150318, www.gebco.net.
A rectangular midwater trawl net (RMT1 + 8), with an 8 m2 mouth opening and mesh size of 4.5 mm, was used to target larger plankton and nekton groups (e.g., Antarctic krill), and tapered to a mouth opening of 1.5 m2 in the last 1.8 m of net (Hosie et al., 2000). Smaller plankton groups were sampled through a 1 m2 mouth area with a 300 µm mesh size. Net trawls were either ‘routine’ where sampling occurred at pre-designated locations to match previous surveys (see Nicol and Meiners, 2010), or ‘target’ in which sampling was conducted based on observations using the vessel’s echo sounders that suggested the presence of Antarctic krill swarms (see Kelly et al., 2021 for full survey details). All net trawling was conducted through a water speed of 2 knots, and routine trawls were deployed obliquely from surface to 200 m for up to 18 minutes. Nets during target trawls were kept open between 7 to 9 minutes. A flow meter was positioned at the mouth of the RMT8 net to measure the volume of seawater filtered. Antarctic krill from the RMT8 nets were sampled specifically for a distribution and biomass study by Cox et al. (2022). The samples from the RMT1 net were reserved for this study and preserved in 5% buffered formaldehyde and seawater solution prior to transportation to the Institute for Marine and Antarctic Studies in Hobart, Australia, for further analyses.
While in the laboratory, zooplankton samples were rinsed in seawater and split to a subsample of approximately n = 600 individuals using a Motoda box splitter (Motoda, 1985). Individuals at each station were identified to the lowest taxonomic level possible (henceforth taxon). Whilst this was usually to species or genus, some individuals were grouped at higher taxonomic level due to difficulties in identification (see Supplementary Table S1 for full list of taxonomic units). Individuals in each taxon were counted and, wherever possible, assigned life stage using a Leica M165 C stereoscopic microscope. Counts were divided by the splitting ratio then divided by the calibration value determined from the flowmeter. Abundances were calculated per taxon per sampling location and are reported as the number of individuals per 1000 m3.
A Seabird SBE911 Plus CTD-mounted rosette sampler was deployed to perform full-depth profile water sampling at pre-determined depths throughout the water column and prior to each plankton net deployment. Profiles were created for temperature, salinity and fluorescence.
To investigate the relationships between environmental variables and zooplankton abundance, we used a suite of variables measured during the voyage, in the laboratory and additional satellite-derived datasets (Table 1). These variables were selected to provide a comprehensive understanding of the physical and biological factors influencing zooplankton abundance in our study area.
Table 1. Environmental and spatial variables tested as zooplankton community predictors for generalized additive modeling (GAMs).
Chlorophyll-a concentration (mg m-2), an essential indicator of phytoplankton biomass and, indirectly, primary productivity, was integrated over depths from 10 m to 200 m, aligning with the zooplankton sampling depth. This estimate was derived from in situ florescence measurements collected onboard from the CTD-mounted rosette sampler. For more details on how these values were derived, see Heidemann et al. (2024) in this issue.
Temperature and salinity were also collected using the CTD rosette sampler. Temperature (CT_200) was represented as absolute temperature (°C) averaged between the surface and 200 m, while salinity (SA_200) was represented as absolute salinity (g kg-1) averaged over the same depth range. For temperature and salinity profiles, see Foppert et al. (2024) in this issue. The mixed layer depth (MLD_N2max) was determined as the depth where the maximum buoyancy frequency (N2) occurred, i.e., the pycnocline. This method was chosen because pycnocline significantly influences the vertical distribution of zooplankton and their prey through the supply of nutrients (Priddle et al., 2003). The maximum buoyancy frequency was derived from CTD profiles of temperature and salinity (Foppert et al., 2024, this issue).
Bathymetric depth (m) at each sampling station was directly measured to understand the spatial distribution of zooplankton in relation to ocean depth. Ice concentration (%) and number of days since sea ice melted were obtained from satellite observations, specifically daily passive microwave estimated percent sea ice concentration from the National Snow and Ice Data Centre (NSIDC). Ice concentration represents the percentage of an area covered in sea ice at the time of sampling, while the days since ice melt provide temporal information on the recency of ice melt. Additionally, the distance from each sampling site to the edge of the ice was calculated using satellite-derived ice concentration data.
As a preprocessing step, a fourth root transformation was applied to the abundance values to normalize the data, which is appropriate for minimizing the influence of a few highly abundant species (Quinn and Keough, 2002). Zooplankton community patterns were analyzed using Q-mode cluster analysis, which classified sampling sites based on Bray-Curtis dissimilarity. Hierarchical agglomerative clustering (UPGMA) was used to generate a cluster dendrogram, illustrating the similarity between sampling sites.
Non-metric multidimensional scaling (NMDS) was performed to complement the cluster analysis, providing a visual representation of similarity patterns among sites, also based on Bray-Curtis dissimilarities. The NMDS analysis was initialized with random starting configurations and iterated 1000 times to ensure the stability of the solution (Oksanen et al., 2022). Environmental vectors were fit onto the ordination to quantify how well each variable correlated with the site configuration in NMDS space (Table 1). The environmental variables included chlorophyll-a concentration, temperature, salinity, days since ice melt, mixed layer depth, latitude, longitude, sea ice concentration, distance to ice edge, and depth.
To assess the significance of community composition differences among clusters, we conducted a Permutational Multivariate Analysis of Variance (PERMANOVA), based on a Bray-Curtis dissimilarity matrix derived from the abundance data. The initial clustering identified 5 distinct clusters. Furthermore, a similarity profile (SIMPROF) analysis was conducted to validate the non-randomness of the similarity profiles within the cluster structure. We used this to test the number of significant clusters based on the Czekanowski distance measure. This combined approach highlights both the clustering structure and the multidimensional relationships within the data.
To determine the optimal number of clusters, we employed silhouette analysis, which measures how similar each sample is to its own cluster compared to others. The two-cluster solution yielded the highest silhouette width (asw = 0.24), indicating the strongest separation; however ecological systems are often complex, and selecting too few clusters may overlook important ecological variability. Therefore, we selected three clusters (asw = 0.18), balancing interpretability and clustering quality. This decision was made to capture more nuanced community distinctions that might be lost in a simpler two-cluster solution. The resulting cluster assignments were stored as a factor variable.
For each cluster, we first identified taxa contributing to the top 50% of the total abundance. This step focuses on determining the dominant taxa within each cluster, which allows us to characterize the general composition of the community. However, high abundance alone does not necessarily equate to a species being a good indicator of specific environmental conditions. To address this, we employed a separate Indicator Value (IndVal) analysis (De Cáceres et al., 2012) to statistically identify indicator species, which are taxa that are not only representative of a cluster but are also associated with specific environmental conditions or community compositions. The IndVal analysis provides a more refined approach by considering the fidelity and specificity of each taxon to the clusters, independent of their overall abundance. This allows us to identify taxa that may be less abundant but still serve as strong indicators of particular ecological settings. Thus, the identification of indicator species is based on a statistical representation of environmental conditions rather than abundance alone. A taxon is deemed a significant indicator if its associated p-value was less than 0.05, ensuring robustness in differentiating between clusters.
To explore the relationship between environmental predictors and zooplankton abundance, we applied generalized additive models (GAMs) across all zooplankton data, regardless of cluster assignments. The GAMs focused on species-specific abundance (e.g., Euphausia superba, Clausocalanus laticeps, Paraeuchaeta sp.) as well as total zooplankton abundance. This approach allowed us to investigate how environmental factors, such as chlorophyll-a concentration, temperature, and mixed layer depth (MLD), influenced the abundance of key taxa across the entire dataset rather than within specific clusters.
Before fitting the GAMs, we tested for collinearity between environmental variables using Pearson’s Correlation analysis, retaining variables with correlation coefficients below the threshold of 0.7 to avoid multicollinearity. Each GAM included smooth terms for predictor variables, such as chlorophyll-a concentration, sea surface temperature, and the number of days since sea ice melt, to capture potential non-linear relationships between environmental drivers and zooplankton abundance. The models were fitted using a Tweedie distribution with a log link function, which is appropriate for modeling non-negative, continuous data with skewness.
Model selection was based on the Akaike Information Criterion (AIC), where lower AIC values indicated better-fitting models with an optimal balance between goodness-of-fit and model complexity. A series of candidate models were constructed using various combinations of predictor variables, including chlorophyll-a concentration, sea surface temperature, salinity, and mixed layer depth. Each model included smooth terms to capture potential non-linear relationships between zooplankton abundance and the environmental predictors. The degree of smoothing (k) was selected to avoid overfitting while ensuring adequate flexibility to model non-linear patterns. All models were fitted using restricted maximum likelihood (REML) to provide more accurate parameter estimates, especially when comparing models with different levels of complexity.
All analyses were performed using R version 4.3.0 (R Core Team, 2023) with the following packages ‘vegan’ (Oksanen et al., 2022), ‘cluster’ (Maechler et al., 2023), ‘indicspecies’ (De Cáceres and Legendre, 2009), and ‘mcgv’ (Wood, 2017). All figures were made using SOmap (Maschette et al., 2019), ‘GGally’ (Schloerke et al., 2012) and ggplot (Wickham, 2016). Throughout this study, we considered results to be statistically significant at a 95% confidence level (p < 0.05).
The southern Antarctic Circumpolar Current front (SACCF) enters the westernmost transect at 63.5°E in the northwest of the survey region. It extends poleward until the third transect (65°E) before heading northward to 63.38°E at the fourth transect (70°E). The SACCF then shifts poleward again across the fifth and sixth transects (75°E and 80°E). The Southern Boundary (SB) of the Antarctic Circumpolar Current enters the western transect at 64.45°E and progresses to its highest latitude of 65.53°E by the second transect (60°E) and its lowest latitude of 63.85°E at the 70°E transect. Closest to the Antarctic continent, the Antarctic Slope Front (ASF) enters the westernmost transect at 65.3°E and progressively heads as far south as 66.5°E at the fifth transect (75°E) before heading northwards to 65.05°E at the final transect (80°E), however there is uncertainty surrounding the exact location as there was likely an eddy located here, as well. There is also uncertainty surrounding the exact locations of the ASF at the third and fourth transects given the depths ranged across the sampling region, with a maximum depth of 4,992 m located at the north-westernmost station of the survey region (at trawl site R06; 62°S and 55°E), and a minimum depth of 451 m located at trawl site R30 (67°S and 74.6°E) towards Prydz Bay and north of the Amery Ice Shelf. The ASF is estimated near 65°S, where isotherms drop sharply.
The mixed layer depth (MLD) ranged from 20 m (trawl sites R18 and T06) (Figure 1) to 73 m at site R15, approximately 92 nm south and the closest sampling site to Mawson Coast. The pycnocline depth, i.e., the depth at which the maximum of buoyancy frequency (N2 maximum) was found at the MLD, ranged across survey regions, where the shallowest pycnocline was 25 m, located at trawl sites R29 and T10 (towards the Amery Ice Shelf), and the deepest pycnocline depth at 88 m, located at T04 along the Mawson coast. Sea surface temperatures for the upper 200 m ranged from -1.73°C, at the south-westernmost station (R01), to 1.18°C, which was located at the northeast area of the survey region (site R38). Salinity values from the upper 200 m of the water column ranged from 34.57 (R02) to 34.10 (R22), which is the northernmost sampling site along the 65°E transect line where the freshest seawater was recorded. Integrated chlorophyll-a ranged widely throughout the survey region, where the smallest value recorded was 18.4 mg m-2 at trawl site R37, at the northern end of the easternmost transect (80°E), and 102.4 mg m-2 at trawl sites R32, T11 and T12, which are all located within the Cooperation Sea.
Seventy-nine zooplankton taxa were identified from all 48 sampling sites. Total zooplankton abundances ranged from 115 to 59,970 ind./1000 m3 with a mean abundance of 13,083 ind/1000 m3 (± 15,670 ind./1000 m3) that represent 17 broader taxonomic groups, of which 88% were copepods, 4% pteropods, 3% chaetognaths, 2% krill, 1% ostracods and 1% foraminifera (Supplementary Table S1 for a complete list of taxa identified). On 24 February 2021, the lowest abundance was recorded at site R18, located along the central transect of the survey region, on the 65°S line, with increasing abundance towards the east along the ASF (Figure 2). The maximum abundance was sampled on 27 February 2021 at trawl site R23 (62°S and 70°E), which was located north of the SACCF. Among the taxonomic groups, copepods dominated with the small sized species being the most dominant, including Oithona similis showing the highest estimated abundance (29,902 ind./1000 m3), followed by Microcalanus pygmaeus (14,478 ind./1000 m3). Large copepod species, Calanoides acutus and Rhincalanus gigas, were also dominant throughout the study region (21,181 ind./1000 m3 and 9,300 ind./1000 m3, respectively).
Figure 2. Total zooplankton abundance (ind./1000 m3) at each sampling site. Solid border with darker regions indicates eastern sector of CCAMLR Division 58.4.2 from 55°E to 80°E. Dashed lines indicate other CCAMLR Division borders. Background bathymetry grid is from the GEBCO_2014 Grid, version 20150318, www.gebco.net. Solid, green lines represent oceanic frontal zones Southern Antarctic Circumpolar Current Front (SACCF), and the Antarctic Slope Front (ASF) determined through Orsi et al. (1995) and point estimates from Foppert et al. (2024).
Q-mode cluster analysis identified three groups at approximately 45% similarity (Figure 3). Our study employed NMDS to visualize the underlying patterns in environmental data across various sampling sites (Figure 4). NMDS showed clear separation among three clusters (stress = 0.18). Ecological zones were differentiated according to chlorophyll-a, depth, temperature, salinity, MLD, sea ice concentration, and proximity to the ice edge. Our PERMANOVA analysis, based on a Bray-Curtis dissimilarity matrix calculated from the zooplankton abundance data, revealed a significant difference in community composition among the sampling sites (PERMANOVA, p < 0.05), and that the observed dissimilarity between groups was significantly higher than expected by change (F = 1). The SIMPROF analysis confirmed that 13 clusters were significantly distinct (p < 0.05 for each), suggesting that the observed groupings were not due to random chance.
Figure 3. Dendrogram representing hierarchical clustering of sampling stations based on Bray-Curtis dissimilarity of zooplankton community compositions. Stations are labelled R or T and listed at the ends of the branches. Colored boxes around branches surround each of the three clusters. The length of the branches depicts percent similarity, illustrating the hierarchical structure of station similarities.
Figure 4. Non-metric Multidimensional Scaling (NMDS) plot displaying similarity patterns among sampling sites based on sampling sites, color-coded according to cluster assignment. Arrows indicate direction and strength of the relationship between environmental variables and the ordination space, with the length of each arrow proportional to the influence of the variable.
Cluster 1 included 34 sampling sites and was primarily associated with SACCF and SB frontal zones that exhibited high chlorophyll-a concentrations (49.13 ± 24.38 mg m-2) and temperatures (0.02 ± 0.85°C) (Figure 5; Table 2). This cluster was dominated by Calanoides acutus and Rhincalanus gigas that accounted for 78.6% (21,181 ± 681 ind./1000 m3) and 20.7% (9,300 ± 938 ind./1000 m3) of total abundance (Table 3).
Figure 5. Station cluster groupings as determined by hierarchical agglomerative clustering analysis and NMDS. Solid, green lines represent oceanic frontal zones Southern Antarctic Circumpolar Current Front (SACCF) and the Antarctic Slope Front (ASF) determined through Orsi et al. (1995) and point estimates from Foppert et al. (2024).
Table 2. Mean ± standard deviation and range (minimum—maximum) values for environmental variables per cluster, as determined through hierarchical clustering.
Table 3. Taxa, abundance (ind./1000 m3) and proportion (%) contributing to the top 50% of the total abundance for each cluster.
This cluster also had the highest total abundance of zooplankton (74,386 ind./1000 m-3), with copepods contributing 58,524 ind./1000 m³ (Table 3). Other significant taxa included chaetognaths (9,716 ind./1000 m³), foraminifera (1,782 ind./1000 m³), and ostracods (1,327 ind./1000 m³). Overall, copepods dominated Cluster 1 with 78.6% of the community composition (Figure 6). Additionally, this cluster showed high species diversity, with 34 taxa, including Clausocalanus breviceps (4.50%), Aetideops antarctica (3.98%), and Candacia sp. (3.94%) (Figure 7; Table 4).
Figure 6. Abundance (A) and percent composition (B) of the taxonomic group Copepoda (teal color) and all other taxa (coral color) divided by clusters determined from Q-mode clustering analysis.
Figure 7. Abundance (A) and percent composition (B) of all taxa excluding Copepoda and divided by clusters determined from Q-mode clustering analysis.
Table 4. Average abundances ± standard deviations (ind./1000 m3) for all zooplankton taxa of each of the three clusters determined through agglomerative hierarchical clustering and NMDS analysis.
Environmental analysis revealed that Cluster 1 was characterized by deeper waters (3,475 ± 739 m) compared to Clusters 2 and 3, and its sites were primarily distributed across all northernmost regions (Table 2). This cluster appeared to have the greatest spatial distribution, spanning all transects from 55°E to 85°E.
Cluster 2 included four sampling sites, which were located along transects 2 (60°E) and 3 (65°E) (Figure 5). This cluster was associated with mid-latitude regions and exhibited lower chlorophyll-a concentrations (30.04 ± 5.14 mg m-2) and intermediate temperatures (-0.75 ± 0.86°C) compared to the other clusters (Table 2).
In terms of community composition, Cluster 2 had a more diverse array of species, with copepods contributing 72.5% of the total abundance (767 ind./1000 m³) (Figures 6, 7; Table 3). The dominant species in this cluster included Calanus simillimus (30.12%), Rhincalanus gigas (25.31%), Calanoides acutus (23.39%), and Calanus propinquus (21.17%). This cluster exhibited the lowest overall zooplankton abundance (1,059 ind./1000 m³).
The IndVal analysis identified several indicator species for Cluster 2, including the shelled pteropod Clio pyramidata f. sulcata (IndVal = 0.649, p = 0.024) and the pelagic worm Rhynchonerella brongraini (IndVal = 0.617, p = 0.026). Clausocalanus laticeps was also found to be significant across Clusters 1 and 2 (IndVal = 0.845, p = 0.018).
Cluster 3 included ten sampling sites and was located in the southernmost regions of the survey, primarily along the Mawson coastline and within the Cooperation Sea (Figure 5). This cluster was most distinct from the others, separating at approximately 44% similarity. It was associated with lower chlorophyll-a concentrations (27.80 ± 7.79 mg m-2) and colder temperatures (-1.43 ± 0.34°C) than Clusters 1 and 2 (Table 2).
The total zooplankton abundance for Cluster 3 was 22,629 ind./1000 m³, with copepods contributing 19,743 ind./1000 m³ and making up 87% of the community composition (Figure 6; Table 3). The dominant copepod species in this cluster were Calanus simillimus (13.89%), Calanus propinquus (12.66%), and Oncaea antarctica (10.70%). In addition, this cluster had notable proportions of euphausiids (1,044 ind./1000 m³) and ostracods (Figure 7; Table 4).
The IndVal analysis identified Paraeuchaeta sp. as a statistically significant indicator species for Cluster 3, suggesting its strong association with the environmental conditions in this region.
Pearson’s Correlation analysis was run to assess collinearity between and among all environmental variables selected (Supplementary Figure S1 in Supplementary Materials for matrix). Since none of the correlations exceeded the 0.7 threshold, all predictor variables were retained for subsequent analysis. Due to insufficient abundance, three of the seven species identified previously as indicator species were excluded from further analysis: the pteropod Clio pyramidata f. sulcata, the pelagic worm Rhynchonerella brongraini, and the amphipod Hyperiella sp.
Generalized additive models (GAMs) were applied to examine how key environmental factors, such as chlorophyll-a, temperature, and MLD, influenced zooplankton abundance across the entire dataset. This analysis provided insights into how these environmental drivers impacted the abundance and distribution of key species, including copepods Haloptilus sp. (Supplementary Figure S2), Clausocalanus laticeps (Supplementary Figure S3), and Paraeuchaeta sp. (Supplementary Figure S4) and krill Euphausia superba (Supplementary Figure S5), as well as total zooplankton abundance (Supplementary Figure S6). Summary statistics for each model, including adjusted R2, deviance explained, and significant smooth terms are provided in Supplementary Table S2 of Supplementary Materials. See Supplementary Figures (S2-S6) for the linear and response scale visualizations of these relationships for each of the species and total zooplankton abundances. The resultant models for each species and total zooplankton abundance are presented in Supplementary Table S3, which includes predictor variables and their AIC values, indicating model fit. Across all models, significant environmental drivers included chlorophyll-a concentration, temperature (integrated to 200 m), days since ice melt, and mixed layer depth (MLD).
For total zooplankton abundance, the model explained 70.9% of the deviance (adjusted R2 = 0.642), suggesting that a substantial portion of zooplankton variability was driven by these factors (Supplementary Table S2), which included chlorophyll-a concentration, temperature, number of days since ice melt, and MLD. The smooth terms for days since ice melt was the most significant (F-value = 23.283; p = 2.07x10-5), indicating a strong influence on total zooplankton abundance. Chlorophyll-a concentration was also highly significant (F-value = 15.478; p = 3.10x10-3), reflecting its importance as a proxy for primary productivity. Temperature (F-value = 7.672, p = 5.84 × 10-5) and MLD (F-value = 5.282, p = 2.68 × 10-2) also showed significant effects. Similarly, Clausocalanus laticeps abundance was significantly influenced by temperature (F-value = 7.262, p = 1.03 × 10-2) and MLD (F-value = 14.126, p = 5.60 × 10-4).
For taxon-specific results, the GAM for the copepod Clausocalanus laticeps showed that environmental variables explained 81.6% of the variation in abundance (adjusted R² = 0.885; Supplementary Table S2), making it one of the best-fitting models in the analysis. The strongest predictors of C. laticeps abundance were chlorophyll-a concentration (F-value = 10.056; p = 2.77 × 10-4) and mixed MLD (F-value = 14.126; p = 5.60 × 10-4), suggesting that primary productivity and physical oceanographic conditions strongly influence this species. Days since ice melt also significantly affected abundance (F-value = 5.777; p = 4.08 × 10-3), indicating that seasonal variability in sea ice may play a role.
For Haloptilus sp., the environmental variables explained 37.6% of the variation in abundance (adjusted R² = 0.409; Supplementary Table S2), with latitude being the most significant predictor (F-value = 14.636; p = 4.08 × 10-4), highlighting the spatial distribution of this species. Salinity (integrated to 200 m) was also significant (F-value = 4.421; p = 4.12 × 10-2), while days since ice melt had a marginal effect (F-value = 3.862; p = 6.24 × 10-2).
For the krill E. superba, environmental variables explained 42.3% of the variation in abundance (adjusted R² = 0.151; Supplementary Table S2). Latitude was a significant predictor (F-value = 6.684; p = 1.34 × 10-2), indicating spatial patterns in krill distribution. Days since ice melt also significantly influenced krill abundance (F-value = 4.173; p = 4.75 × 10-2), while other variables, such as chlorophyll-a and temperature, did not significantly impact abundance.
Lastly, for Paraeuchaeta sp., the model explained 99.9% of the variation in abundance (adjusted R² = 0.999; Supplementary Table S2), though none of the predictors were statistically significant. Mixed layer depth was marginally non-significant (F-value = 1.975; p = 6.62 × 10-2), suggesting that deeper oceanographic processes may influence this species, but further research is needed to confirm these effects the observed dominance of certain species, such as C. acutus in warmer waters and E. superba in regions with earlier sea ice melt, highlights the nuanced interactions between zooplankton and their environmental. These species-specific patterns underscore the critical role of environmental gradients in structuring the overall community.
Our study provides new insights into the distribution and abundance of mesozooplankton communities along the Mawson coast, East Antarctica, highlighting the roles of environmental variables such as temperature, primary productivity, and sea ice dynamics. These factors are critical in shaping the community structure and influencing the dominance of specific zooplankton species across environmental gradients.
Mesozooplankton abundance across the survey ranged from 115 to 59,970 ind./1000 m³, generally lower than in other Southern Ocean regions. This pattern likely reflects regional variations in environmental conditions, particularly temperature and chlorophyll-a concentrations. Areas with elevated chlorophyll-a and warmer waters, typically associated with oceanographic fronts such as the SACCF and ASF, supported higher zooplankton abundances. These results align with previous studies in the Southern Ocean, including the BROKE-West survey, which also identified similar patterns of enhanced zooplankton abundance in areas with increased primary productivity and nutrient availability (Nicol et al., 2000).
The zooplankton surveys from the BROKE-West study (Swadling et al., 2010) showed that abundances tended to be higher towards the northeast sector of the region, and our study aligns with these findings. Notably, the highest average chlorophyll-a concentration linked with the highest average zooplankton abundance in the BROKE-West survey. Our average estimates of chlorophyll-a concentration and zooplankton abundance were lower by an order of magnitude compared with the BROKE-West survey. This pattern suggests a complex dynamic between phytoplankton availability (i.e., food for zooplankton grazers), chlorophyll-a concentrations, and zooplankton grazing pressure. Further investigation into primary production rates and turnover is warranted to better understand these interactions in this region.
As reported by Swadling et al. (2010) across the same location, copepods emerge as the dominant group in our study, particularly the smaller species, such as Oithona similis, Microcalanus pygmaeus and Ctenocalanus sp. The dominance of large copepod species, like Calanoides acutus and Rhincalanus gigas observed here, has also been observed in previous studies across the same survey region and other regions of the Southern Ocean (Johnston et al., 2022). The large abundances of copepods in this region underscore their crucial role in the transfer of energy within the Antarctic food web, connecting primary productivity to higher trophic levels, as well as their conduit remineralization role (Swadling et al., 2010). Interestingly, Schaafsma et al. (2024) found that C. propinquus and O. similis were also dominant in surface waters, but with varying densities depending on the location and time of sampling. This suggests that surface conditions can significantly influence copepod distributions.
The cluster analysis identified three distinct zooplankton communities, each characterized by dominant species and environmental conditions. Cluster 1, situated in warmer, northern waters, was dominated by Calanoides acutus (21,181 ± 681 ind./1000 m³), a species known to thrive in regions with higher primary productivity. In contrast, Euphausia superba was more abundant in Cluster 3, associated with earlier sea ice melt and extended open-water periods conducive to phytoplankton growth. The significant variation in species abundance across clusters underscores the importance of environmental gradients in shaping zooplankton communities, with species responding differently to changes in temperature, sea ice, and nutrient availability.
The dominance of Calanoides acutus in Cluster 1, characterized by higher chlorophyll-a concentrations, mirrors findings from the BROKE-West survey, where similar species were found to dominate in regions of high primary productivity (Swadling et al., 2010). However, the lower abundances observed in our study compared to BROKE-West likely reflect regional differences in oceanographic conditions and temporal variability in phytoplankton blooms. The presence of Euphausia superba in Cluster 3, which is closely linked to areas of early sea ice melt, also aligns with observations from BROKE-West, where krill abundance was higher in regions with extended open-water periods following ice retreat.
Our findings also reveal the role of smaller copepod species, such as Clausocalanus laticeps, in driving community composition in Cluster 2, where environmental conditions were more moderate. These species-specific responses to environmental variability highlight the complexity of zooplankton community dynamics in this region, with key species occupying ecological niches that align with their physiological tolerances and feeding strategies.
Generalized Additive Models (GAMs) provided species-specific insights into how environmental drivers shape zooplankton abundance, independent of the clustering analysis. Chlorophyll-a concentration and temperature emerged as significant predictors of abundance for Clausocalanus laticeps, while latitude and salinity were important for Haloptilus sp. The BROKE-West survey similarly highlighted the importance of these environmental drivers in structuring zooplankton communities, particularly the role of primary productivity in driving the distribution of key species across the region (Swadling et al., 2010; Nicol et al., 2000).
Oceanographic features such as the Antarctic Circumpolar Current Front (SACCF), Southern Boundary (SB) and the Antarctic Slope Front (ASF) play a critical role in shaping the distribution of mesozooplankton communities in the Southern Ocean. These fronts and currents create distinct ecological boundaries, where enhanced mixing and nutrient availability influence the movement and abundance of zooplankton (Nicol and Meiners, 2010). Species such as Calanoides acutus and Rhincalanus gigas were more abundant near these frontal systems, which provide favorable feeding conditions. These patterns are consistent with other Southern Ocean studies, where zooplankton abundance and community composition have been linked to oceanographic fronts (Atkinson et al., 2008; Takahashi et al., 2002). The dynamic interaction of water masses in these areas leads to localized conditions that support different zooplankton assemblages, similar to patterns observed in other regions of the Southern Ocean (Atkinson et al., 2008; Pakhomov et al., 2000; Tarling et al., 2012). These features underscore the importance of oceanographic processes in structuring zooplankton communities and shaping broader ecosystem dynamics.
While our study provides valuable insights into zooplankton distribution and abundance along the Mawson coast, several caveats must be acknowledged. First, the temporal scope of our sampling was limited to the austral summer, which may not capture the full extent of seasonal variability in zooplankton communities. As zooplankton populations are known to exhibit significant changes across seasons due to shifts in sea ice cover and primary productivity (Swadling et al., 2023; Pinkerton et al., 2020), future studies should aim to include year-round sampling to better understand the temporal dynamics of these communities.
Second, our analysis primarily focused on surface environmental drivers, such as temperature and chlorophyll-a concentrations. However, deeper oceanographic processes, such as subsurface currents and nutrient upwelling, may also play a critical role in shaping zooplankton communities, particularly for species that undergo diel vertical migrations (Schaafsma et al., 2024). Future research should consider the influence of these deeper processes to provide a more comprehensive understanding of zooplankton ecology in the Southern Ocean.
Additionally, our study relied on Generalized Additive Models (GAMs) to explore species-environment relationships. While GAMs are powerful tools for identifying key environmental drivers, their application assumes a level of independence between variables that may not fully capture the complexity of ecosystem interactions (Dormann et al., 2013). Future studies may benefit from employing more complex ecological models, such as those that account for species interactions and multicollinearity between environmental predictors (Wisz et al., 2013).
Finally, our findings highlight the importance of understanding zooplankton responses to changing environmental conditions, particularly in light of climate change. Long-term monitoring programs will be essential for detecting shifts in zooplankton communities over time and assessing the resilience of Southern Ocean ecosystems. Further research into the functional roles of key zooplankton species, such as Euphausia superba, and their interactions with other trophic levels will be crucial for predicting future ecosystem responses.
As the Southern Ocean continues to experience climate-driven changes in sea ice extent, temperature, and primary productivity, zooplankton communities will likely undergo significant shifts. Species like Euphausia superba may face disadvantages in regions with reduced sea ice cover, as earlier ice melt could disrupt the timing of phytoplankton blooms and krill recruitment (Atkinson et al., 2019; Flores et al., 2012). Conversely, species such as Calanoides acutus, which thrive in warmer waters, may benefit from these changing conditions. These shifts could have cascading effects on higher trophic levels, particularly krill-dependent predators like seabirds, fish, and marine mammals.
Our findings highlight the need for continued monitoring of zooplankton communities to better understand their responses to climate change. The significant role of environmental drivers in shaping taxon-specific abundance and community composition suggests that future changes in these drivers will alter the structure of Southern Ocean ecosystems. By identifying key environmental factors that influence zooplankton dynamics, this study contributes to the growing body of knowledge needed to predict the ecological impacts of climate change in polar regions.
The original contributions presented in the study are included in the article/Supplementary Materials, further inquiries can be directed to the corresponding author.
The manuscript presents research on animals that do not require ethical approval for their study.
CW: Conceptualization, Data curation, Formal Analysis, Investigation, Methodology, Project administration, Validation, Visualization, Writing – original draft, Writing – review & editing. MB: Methodology, Writing – review & editing. AB: Methodology, Writing – review & editing. OJ: Methodology, Writing – review & editing. DM: Data curation, Formal Analysis, Investigation, Methodology, Writing – review & editing.
The author(s) declare financial support was received for the research, authorship, and/or publication of this article. This research was supported by the Australian Antarctic Program Partnership - a partnership of Australia's leading Antarctic research institutions supported by the Australian Government Antarctic Science Collaborative Initiative. This project was also supported and funded by the Australian Antarctic Science Project 4512 and 4636, and received financial support from Pew Charitable Trust and the Antarctic Science Foundation.
We thank Dr. So Kawaguchi, Rob King, Jessica Melvin, Associate Professor Kerrie Swadling, and Dr. Karen Westwood for cruise and zooplankton sampling organization. Thanks to all crew and scientific team aboard the RV Investigator. We also thank Dr. Mike Sumner and Dr. Ben Raymond for providing distance to ice and ice concentration measurements, Dr. Annie Foppert for mixed layer depth estimates, and Dr. Karen Westwood for integrated chlorophyll-a values. Finally, we would also like to thank the reviewers whose input greatly improved the quality of this research output.
The authors declare that the research was conducted in the absence of any commercial or financial relationships that could be construed as a potential conflict of interest.
All claims expressed in this article are solely those of the authors and do not necessarily represent those of their affiliated organizations, or those of the publisher, the editors and the reviewers. Any product that may be evaluated in this article, or claim that may be made by its manufacturer, is not guaranteed or endorsed by the publisher.
The Supplementary Material for this article can be found online at: https://www.frontiersin.org/articles/10.3389/fmars.2024.1360541/full#supplementary-material
Atkinson A., Hill S. L., Pakhomov E. A., Siegel V., Reiss C. S., Loeb V. J., et al. (2019). Krill (Euphausia superba) distribution contracts southward during rapid regional warming. Nat. Climate Change 9, 142–147. doi: 10.1038/s41558-018-0370-z
Atkinson A., Siegel V., Pakhomov E. A., Rothery P., Loeb V., Ross R. M., et al. (2008). Oceanic circumpolar habitats of Antarctic krill. Mar. Ecol. Prog. Ser. 362, 1–23. doi: 10.3354/meps07498
Cavan E. L., Henson S. A., Belcher A., Sanders R. (2017). Role of zooplankton in determining the efficiency of the biological carbon pump. Biogeosciences. 14, 177–186. doi: 10.5194/bg-14-177-2017
Constable A. J., Melbourne-Thomas J., Corney S. P., Arrigo K. R., Barbraud C., Barnes D. K. A. A., et al. (2014). Climate change and Southern Ocean ecosystems I: how changes in physical habitats directly affect marine biota. Glob. Change Biol. 20, 3004–3025. doi: 10.1111/gcb.12623
Core Team R. (2023). R: A Language and Environment for Statistical Computing (Vienna, Austria: R Foundation for Statistical Computing). Available at: https://www.R-project.org/.
Cox M. J., Macaulay G., Brasier M. J., Burns A., Johnson O. J., King R., et al. (2022). Two scales of distribution and biomass of Antarctic krill (Euphausia superba) in the eastern sector of the CCAMLR Division 58.4.2 (55°E to 80°E). PloS One 17, 1–19. doi: 10.1371/journal.pone.0271078
De Cáceres M., Legendre P. (2009). Associations between species and groups of sites: Indices and statistical inference. Ecology 90, 3566–3574. doi: 10.1890/08-1823.1
De Cáceres M., Legendre P., Wiser S. K., Brotons L. (2012). Using species combinations in indicator value analyses. Methods Ecol. Evol. 3, 973–982. doi: 10.1111/j.2041-210X.2012.00246.x
Dormann C. F., Elith J., Bacher S., Buchmann C., Carl G., Carré G., et al. (2013). Collinearity: a review of methods to deal with it and a simulation study evaluating their performance. Ecography 36, 27–46. doi: 10.1111/j.1600-0587.2012.07348.x
Flores H., Atkinson A., Kawaguchi S., Krafft B., Milinevsky G., Nicol S., et al. (2012). Impact of climate change on Antarctic krill. Mar. Ecol. Prog. Ser. 458, 1–19. doi: 10.3354/meps09831
Foppert A., Bestley S., Shadwick E. H., Klocker A., Vives C. R., Liniger G., et al. (2024). Observed water-mass characteristics and circulation off Prydz Bay, Antarctica. Front. Mar. Sci. 11. doi: 10.3389/fmars.2024.1456207
Fraser A. D., Massom R. A., Ohshima K. I., Willmes S., Kappes P. J., Cartwright J., et al. (2020). High-resolution mapping of circum-Antarctic landfast sea ice distribution 2000–2018. Earth Syst. Sci. Data 12, 2987–2999. doi: 10.5194/essd-12-2987-2020
Heidemann A. C., Westwood K. J., Foppert A., Wright S. W., Klocker A., Vives C. R., et al. (2024). Drivers of phytoplankton distribution, abundance and community composition off East Antarctica, from 55-80°E (CCAMLR division 58.4.2 east). Front. Mar. Sci. 11. doi: 10.3389/fmars.2024.1454421
Hosie G. W., Schultz M. B., Kitchener J. A., Cochran T. G., Richards K. (2000). Macrozooplankton community structure off East Antarctica (80-150°E) during the Austral summer of 1995/1996. Deep Sea Res. Part II 47, 2437–2463. doi: 10.1016/S0967-0645(00)00031-X
Johnston N. M., Murphy E. J., Atkinson A. A., Constable A. J., Cotté S., Cox M., et al. (2022). Status, change and futures of zooplankton in the Southern Ocean. Front. Ecol. Evol. 9. doi: 10.3389/fevo.2021.624692
Kelly N., Bestley S., Burns A., Clarke L., Collins K., Cox M., et al. (2021). An overview of the ecosystem survey to quantify krill abundance for krill monitoring and management in Eastern Sector of CCAMLR Division 58.4.2: Trends in Euphausiids off Mawson, Predators, and Oceanography “TEMPO” (Working Group on Ecosystem Monitoring and Management (WG-EMM-2021/07).
Maechler M., Rousseeuw P., Struyf A., Hubert M., Hornik K. (2023). cluster: Cluster Analysis Basics and Extensions. R package version 2.1.4. Available at: https://CRAN.R-project.org/package=cluster.
Maschette D., Raymond B., Hosie G. (2019). SOmap: Southern Ocean Mapping Tools. R package version 0.1.5. https://CRAN.R-project.org/package=SOmap.
Massom R., Reid P., Stammerjohn S., Raymond B., Fraser A., Ushio S. (2013). Change and variability in east antarctic sea ice seasonality 1979/80-2009/10. PloS One 8, e64756. doi: 10.1371/journal.pone.0064756
McCormack S. A., Griffith G., Hill S. L., Hoover C., Johnston N. M., Marina T. I., et al. (2020). Southern Ocean food webs: progress, prognoses, and future priorities for research and policy makers. Front. Ecol. Evol. 9, 624763. doi: 10.3389/fevo.2021.624763
Murase H., Abe K., Matsukura R., Sasaki H., Driscoll R., Schaafsma F., et al. (2019). Cruise report of multidisciplinary ecosystem survey in the eastern Indian sector of the Antarctic (CCAMLR Division 58.4.1) with a focus on Antarctic krill during 2018/19 season by the Japanese survey vessel Kaiyo-maru. CCAMLR Rep.
Nicol S., Meiners K. (2010). [amp]]ldquo;BROKE-West” a biological/oceanographic survey off the coast of east Antarctica (30-80oE) carried out in January-March 2006. Deep-Sea Res. 57, 693–992. doi: 10.1016/j.dsr2.2009.11.002
Nicol S., Pauly T., Bindoff N. L., Wright S., Thiele D., Hosle G. W., et al. (2000). Ocean circulation off east Antarctica affects ecosystem structure and sea-ice extent. Nature 406, 504–507. doi: 10.1038/35020053
Oksanen J., Simpson G., Blanchet F., Kindt R., Legendre P., Minchin P. R., et al. (2022). vegan: Community Ecology Package (R package version 2.6-4). Available at: https://CRAN.R-project.org/package=vegan.
Orsi A. H., Whitworth T. III, Nowlin W. D. Jr (1995). On the meridional extent and fronts of the Antarctic Circumpolar Current. Deep-Sea Res. I 42, 641–673. doi: 10.1016/0967-0637(95)00021-W
Pakhomov E. A., Perissinotto R., McQuaid C. D., Froneman P. W. (2000). Zooplankton structure and grazing in the Atlantic sector of the Southern Ocean in late austral summer 1993: Part 1. Ecological zonation. Deep-Sea Res. I 47, 1663–1686. doi: 10.1016/S0967-0637(99)00122-3
Pinkerton M. H., Décima M., Kitchener J. A., Takahashi K. T., Robinson K. V., Stewart R., et al. (2020). Zooplankton in the Southern Ocean from the continuous plankton recorder: Distributions and long-term change. Deep-Sea Res. I 162, 103303. doi: 10.1016/j.dsr.2020.103303
Poloczanska E. S., Burrows M. T., Brown C. J., Molinos J. G., Halpern B. S., Hoegh-Guldberg O., et al. (2016). Responses of marine organisms to climate change across oceans. Front. Mar. Sci. 3. doi: 10.3389/fmars.2016.00062
Priddle J., Whitehouse M. J., Ward P., Shreeve R. S., Brierley A. S., Atkinson A., et al. (2003). Biogeochemistry of a Southern Ocean plankton ecosystem: Using natural variability in community composition to study the role of metazooplankton in carbon and nitrogen cycles. J. Geo Res. 103, 8082. doi: 10.1029/2000JC000425
Quetin L. B., Ross R. M., Frazer T. K., Haberman K. L. (1996). Factors affecting distribution and abundance of zooplankton, with an emphasis on Antarctic krill, Euphausia superba. Antarct Res. Ser. 70, 357–371. doi: 10.1029/ar070p0357
Quinn G. P., Keough M. J. (2002). Experimental Design and Data Analysis for Biologists (Cambridge, UK: Cambridge University Press).
Reid K., Croxall J. P., Briggs D. R., Murphy E. J. (2005). Antarctic ecosystem monitoring: Quantifying the response of ecosystem indicators to variability in Antarctic krill. ICES J. Mar. Sci. 62, 366–373. doi: 10.1016/j.icesjms.2004.11.003
Schaafsma F. L., Matsuno K., Driscoll R., Sasaki H., van Regteren M., Driscoll S., et al. (2024). Zooplankton communities at the sea surface of the eastern Indian sector of the Southern Ocean during the austral summer of 2018/2019. Prog. Ocean 226, 103303. doi: 10.1016/j.pocean.2024.103303
Schloerke B., Crowley J., Cook D., Hofmann H., Wickham H. (2012). GGAlly: Extension to ggplot2 (R package version 2.2.1).
Smetacek V., Assmy P., Henjes J. (2004). The role of grazing in structuring Southern Ocean pelagic ecosystems and biogeochemical cycles. Antarctic Sci. 16, 541–558. doi: 10.1017/S0954102004002317
Swadling K. M., Constable A. J., Fraser A. D., Massom R. A., Borup M. D., Ghigliotti L., et al. (2023). Biological responses to change in Antarctic sea ice habitats. Front. Ecol. Evol. 10. doi: 10.3389/fevo.2022.1073823
Swadling K. M., Kawaguchi S., Hosie G. W. (2010). Antarctic mesozooplankton community structure during BROKE-West (30°E-80°E), January-February 2006. Deep Res. Part II Top. Stud. Oceanogr 57, 887–904. doi: 10.1016/j.dsr2.2008.10.041
Takahashi K. T., Kawaguchi S., Kobayashi M., Hosie G. W., Fukuchi M., Toda T (2002). Zooplankton distribution patterns in relation to the Antarctic Polar Front Zones recorded by Continuous Plankton Recorder (CPR) during 1999/2000 Kaiyo Maru cruise. Polar Bioscience 15, 97–107. doi: 10.15094/00006187
Tarling G. A., Stowasser G., Ward P., Poulton A. J., Zhou M., Venables H. J., et al. (2012). Seasonal trophic structure of the Scotia Sea pelagic ecosystem considered through biomass spectra and stable isotope analysis. Deep Sea Research Part II: Topical Studies in Oceanography 59, 222–236. doi: 10.1016/j.dsr2.2011.10.001
Wickham H.. (2016). ggplot2: Elegant Graphics for Data Analysis. New York: Springer-Verlag. https://ggplot2.tidyverse.org
Wisz M. S., Pottier J., Kissling W. D., Pellissier L., Lenoir J., Damgaard C. F., et al. (2013). The role of biotic interactions in shaping distributions and realised assemblages of species: implications for species distribution modelling. Biol. Rev. 88, 15–30. doi: 10.1111/j.1469-185X.2012.00235.x
Wood S. N. (2017). Generalized Additive Models: An Introduction with R (2nd edition) (Boca Raton, FL: Chapman and Hall/CRC).
Keywords: zooplankton, community structure, East Antarctica, distribution, abundance, multivariate analysis, Generalized Additive Models
Citation: Weldrick CK, Brasier MJ, Burns A, Johnson OJ and Maschette D (2024) Zooplankton abundance and distribution along the Mawson coast, East Antarctica. Front. Mar. Sci. 11:1360541. doi: 10.3389/fmars.2024.1360541
Received: 23 December 2023; Accepted: 23 October 2024;
Published: 22 November 2024.
Edited by:
Yuqiu Wei, Chinese Academy of Fishery Sciences (CAFS), ChinaReviewed by:
Kusum Komal Karati, Centre for Marine Living Resources and Ecology (CMLRE), IndiaCopyright © 2024 Weldrick, Brasier, Burns, Johnson and Maschette. This is an open-access article distributed under the terms of the Creative Commons Attribution License (CC BY). The use, distribution or reproduction in other forums is permitted, provided the original author(s) and the copyright owner(s) are credited and that the original publication in this journal is cited, in accordance with accepted academic practice. No use, distribution or reproduction is permitted which does not comply with these terms.
*Correspondence: Christine K. Weldrick, Y2hyaXN0aW5lLndlbGRyaWNrQHV0YXMuZWR1LmF1
Disclaimer: All claims expressed in this article are solely those of the authors and do not necessarily represent those of their affiliated organizations, or those of the publisher, the editors and the reviewers. Any product that may be evaluated in this article or claim that may be made by its manufacturer is not guaranteed or endorsed by the publisher.
Research integrity at Frontiers
Learn more about the work of our research integrity team to safeguard the quality of each article we publish.