- 1Centre for Ecology and Conservation, University of Exeter, Penryn, United Kingdom
- 2Centre for Geography and Environmental Science, University of Exeter, Penryn, United Kingdom
- 3Consiglio Nazionale delle Ricerche (CNR), Istituto di Scienze Marine (ISMAR), Rome, Italy
- 4Department of Biology, National and Kapodistrian University of Athens, Athens, Greece
The world’s oceans and seas are changing rapidly due to several natural and anthropogenic reasons. Among these, the largest and likely most threatening to marine life being the climate crisis and rising sea temperatures. Studying the dominant primary producers of most marine ecosystems, phytoplankton, and their response to these alterations is challenging, yet essential due to the critical role phytoplankton play in both the oceans and wider biosphere. Satellites are a crucial tool used to study phytoplankton but lack the timespan needed to accurately observe abundance patterns in response to climate change. Historical oceanographic data are increasingly being used to understand changes in the abundance of phytoplankton over the last century. Here, we retrace Secchi depth and Forel-Ule colour scale surveys performed during the “Pola” expeditions between 1890-1898 using contemporary satellite data, to assess changes in water colour and clarity (and by extension phytoplankton abundance) in the Red Sea and the Eastern Mediterranean Sea over the past century. The results show a significant greening of both regions investigated as well as a decrease in water clarity. The Red Sea Forel-Ule colour increased by 0.83 (± 0.08) with an average decrease in Secchi depth of 5.07 m (± 0.44). The Forel-Ule colour in the Eastern Mediterranean increased by 0.50 (± 0.07) and the historic Secchi depth readings were an average of 8.85 m (± 0.47) deeper than present day. Changes in Secchi depth between periods were greater than that which may have been caused by differences in the size of the Secchi disk used on the “Pola” expeditions, estimated using traditional Secchi depth theory. There was no clear change in seasonality of phytoplankton abundance and blooms, although winter months saw many of the largest changes in both measured variables. We discuss potential drivers for this change and the challenges and limitations of combining historical and modern datasets of water clarity and colour.
Introduction
Phytoplankton play a crucial role in both the marine environment and the wider biosphere. They contribute to approximately half of global organic net carbon uptake and oxygen production through photosynthesis and are essential for supporting marine life and fisheries (Field et al., 1998; Chassot et al., 2010). Climate change poses a significant threat to life on Earth and has wide ranging impacts in the marine environment (Doney et al., 2012). Human-induced greenhouse gases have been linked to increasing sea surface temperatures and acidity, expansion of oxygen minimum zones and increasing stratification, severely affecting marine life (IPCC, 2019). These changes are projected to continue through the 21st century, with further impacts on marine biomass and the global water cycle (Wilson et al., 2016; Bryndum-Buchholz et al., 2019). Climate change can affect phytoplankton in numerous ways. For example, through changes in the timing and magnitude of spring phytoplankton blooms, shifts in their community composition, both in terms of species and size structures, and changes in geographical and vertical distribution (Winder and Sommer, 2012; Brewin et al., 2022). Recording phytoplankton abundance is crucial for understanding these impacts and their effect on primary production and the marine ecosystem. The total chlorophyll-a concentration (Chl-a) is regularly used as an approximation of phytoplankton biomass owing to its ubiquitousness in phytoplankton and that it can be measured in both field and satellite applications (Sathyendranath et al., 2023).
Despite many studies investigating the impact of climate change on marine phytoplankton (e.g., Falkowski and Wilson, 1992; Boyce et al., 2010; Wernand and van der Woerd, 2010a; Wernand et al., 2013a; Henson et al., 2021) there is little consensus among the results. This lack of agreement is likely attributed to variations in data collection and analysis methodologies, as well as differences in spatial and temporal ranges that can result in certain biases (Brewin et al., 2023). An essential requirement for the investigation of phytoplankton biomass and productivity in response to climate change is the presence of a dataset with substantial time length. The time span required to accurately separate anthropogenic climate drivers from natural variability is estimated to be over 40 years (Henson et al., 2010). Although satellites can provide a global dataset of Chl-a measurements, derived from algorithms that relate radiometric measurements to Chl-a empirically or semi-analytically with an uncertainty in the open ocean (relative percentage difference when compared with in situ data) of around 30% (Tilstone et al., 2021), their recent employment renders them insufficient to provide the required temporal coverage (Sathyendranath et al., 2019). Thus, a combination of contemporary measurements with historical in-situ visual measurements, retrieved as a Chl-a proxy from apparatuses such as the Secchi disk, Forel-Ule colour scale or Continuous Plankton Recorder (Raitsos et al., 2013b; Wernand et al., 2013a), is needed to create a suitably long time series.
Secchi disk depth and Forel-Ule colour scale are two of the longest oceanographic datasets available, following bathymetry and sea surface temperature (Boyer et al., 2018). A Secchi disk is (typically) a 30 cm white disk which is lowered into the water and the depth at which the disk is no longer visible is proportional to the water clarity. This measurement is recorded as the Secchi disk depth (Secchi, 1865; Tyler, 1968; Wernand, 2010; Pitarch, 2020). The Forel-Ule colour scale was devised in the late 19th century by François Forel and amended by Willi Ule (Forel, 1890; Wernand and van der Woerd, 2010b). The scale consists of 21 different indexed colours, ranging from blue through green and yellow to brown. The measurement is recorded as the index of the colour in the scale that best matches that of the water. These historical techniques can provide information on many different components of marine waters and provide important biological information such as Chl-a concentrations and the depth of the euphotic zone (Lee et al., 2018; Wang et al., 2019; Ye and Sun, 2022). Specifically, Secchi depth and Forel Ule colour data are significant measurements to the oceanographic community, as they are among only a few techniques that have provided data on optical oceanography that is over a century in length. Furthermore, when the concentration of phytoplankton increases in the ocean, the water turns greener and becomes less transparent. Consequently, visual tools like the Secchi disk and Forel Ule colour scale can be used to estimate the concentration of Chl-a in the water.
A large amount of work has been performed to interrogate the effectiveness of these historical techniques and the robustness of the data obtained, for use in modern studies. Overall, studies agree that Secchi depth is a powerful predictor of Chl-a concentration, comparable to in-situ or satellite derived estimates (Boyce et al., 2012; Lee et al., 2018; Brewin et al., 2023). This is particularly true in open-ocean waters where optical variability is controlled principally by phytoplankton and its covarying material (Morel and Prieur, 1977). However, in more optically complex waters, optical variability is controlled by a variety of components that do not always covary in a predictable manner, such that the relationship between Secchi depth and Chl-a becomes more complex. The Forel-Ule colour scale has been examined spectrally and shown to have sufficient variation for capturing seasonal cycles at global scales (Wernand and van der Woerd, 2010b; Novoa et al., 2013), although the introduction of a value of zero for the clearest open oceans such as oligotrophic ocean gyres has been suggested (Pitarch et al., 2019a). This scale is also shown to be closely related to Chl-a, for all but the highest values and most complex waters (Pitarch et al., 2019a). These historical variables can be estimated using modern satellite-derived products with a high degree of confidence (uncertainty in Secchi depth of ~20% and Forel-Ule<1 for the dimensionless scale unit) providing a means to bridge historic and modern data (Lee et al., 2015; Pitarch et al., 2019a; Brewin et al., 2023). Together, the different methods provide a crucial, yet currently underutilised, tool for long term oceanographic studies.
Most studies that have used these data have focused on large spatial scales, often encompassing the entire global oceans (Boyce et al., 2010; Wernand et al., 2013a), reporting unclear trends, or differing localised trends within the global trend. This study aims to investigate changes over a smaller spatial scale, aiming to determine clearer local trends in well-sampled seas. The Eastern Mediterranean and Red Sea were two of the first marine regions to be systematically sampled during the 1890s by the Austro-Hungarian “Pola” expeditions (Luksch, 1901; Wernand, 2010). These marginal seas have warmed at a rapid rate during the last few decades (Nykjaer, 2009; Cantin et al., 2010; Raitsos et al., 2010, 2011; Sisma-Ventura et al., 2014; Chaidez et al., 2017; Mohamed et al., 2019; Pastor et al., 2020; Pisano et al., 2020), however, there is limited work performed on trends in phytoplankton abundance since the 19th century, widely used as the start of anthropogenic climate change. This work therefore provides an important opportunity to investigate potential changes to the base of these ecosystems over the last 120 years.
In this work, we compare the large historic dataset of Secchi depth and Forel-Ule measurements collected in the 1890s on the Austro-Hungarian “Pola” expeditions, with modern measurements derived from remotely sensed satellite data. To minimise differences between the two datasets and optimise data utilisation, samples are matched in space and season. This dataset is used to answer the following two questions: 1) Has the clarity and colour of the water changed in the Eastern Mediterranean and Red Sea over the past century? And 2) Are there any distinct spatial and seasonal shifts in the clarity and colour of these marginal seas?
Methods
Study region
The Red Sea is an elongated basin connected to the open ocean at its southern point, through the Strait of Bab-el-Mandeb, interacting with the Gulf of Aden where seasonal water exchange occurs (Yao et al., 2014). The Red Sea experiences strong stratification in the hot summer months and undergoes increased vertical mixing during the winter months. This change in nutrient availability is a key driver in the seasonal cycles of phytoplankton (Raitsos et al., 2013a). Despite its ecological significance and the presence of threatened habitats and species, the Red Sea remains a largely understudied region of the world’s oceans due to challenging environmental and political conditions (Berumen et al., 2013). The Eastern Mediterranean is a highly oligotrophic region, showcasing the lowest Chl-a concentrations recorded within the Mediterranean Sea (Simboura et al., 2019). Regardless of its oligotrophic nature, the area is characterized by a high level of species richness and habitat diversity, particularly in the Aegean Sea. Similar to the Red Sea, the seasonal primary productivity cycle is driven by the deepening of the mixed layer depth during winter, bringing nutrients upwards from deeper water into the sunlit layer (Simboura et al., 2019). Both these regions are facing many new and historic anthropogenic pressures, such as ship traffic, large coastal settlements and pollutants (Alahmadi et al., 2019; Simboura et al., 2019).
Measurements (both in situ and satellite) were collected from locations across the Eastern Mediterranean and Red Seas, ranging from the Ionian Sea and the coast of Salento to the southern end of the Red Sea (Figure 1).
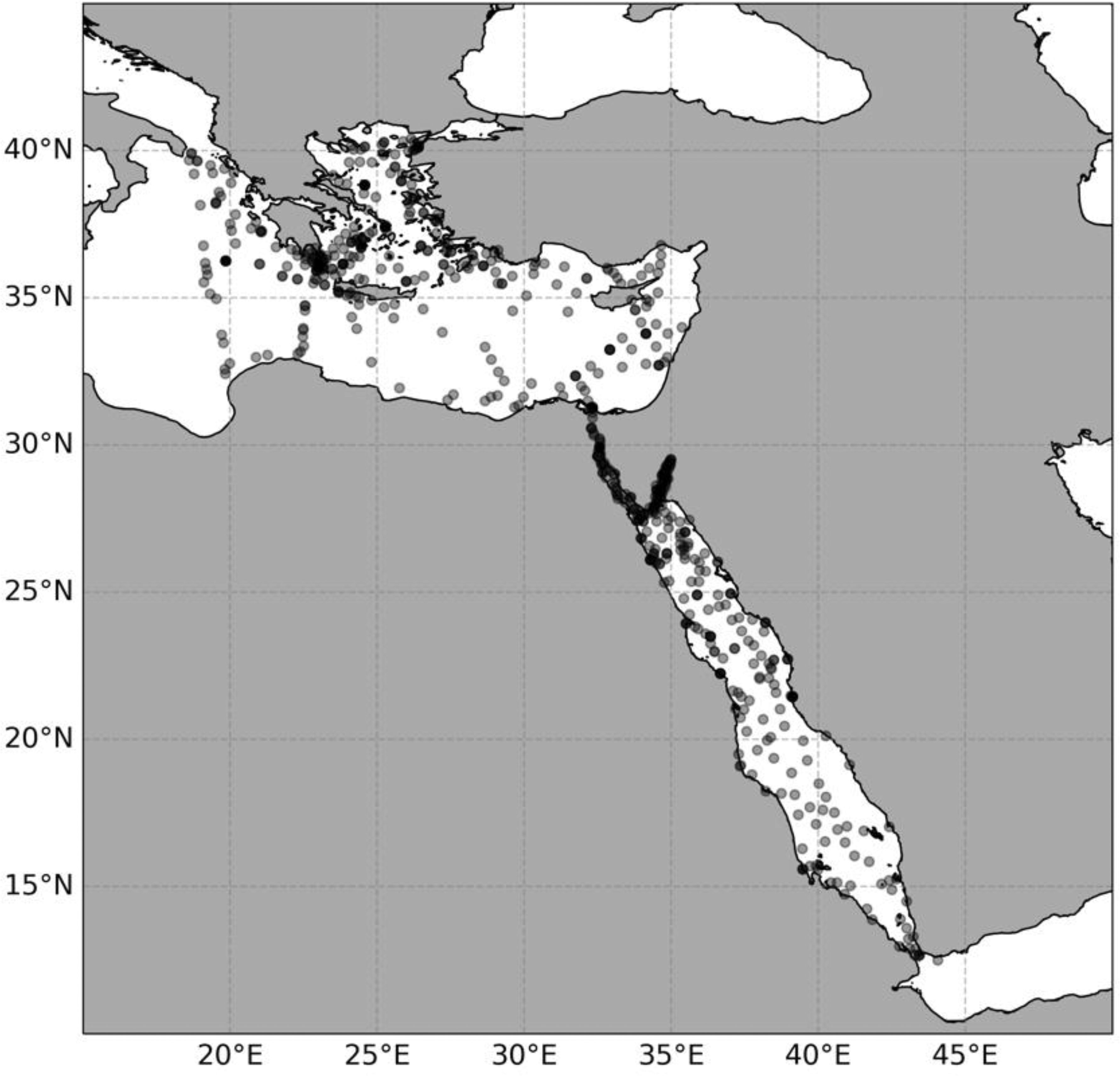
Figure 1. A map of all sample sites (black dots) included in the historic dataset, covering the Red Sea and Eastern Mediterranean.
Data sources
The historical dataset was collected aboard the navy transport vessel “Pola” during its cruises around the Eastern Mediterranean and Red Sea. This expedition was organised as an alternative to a circumnavigation and sampling from a range of waters, to instead focus on the systematic investigation of a particular region (Schefbeck, 1996). The ship was equipped with state-of-the-art survey equipment with scientists from the Viennese Academy of Sciences onboard, leading multiple oceanographic cruises in the waters of the Eastern Mediterranean between 1890 and 1894. However, following the success of these cruises, the area was expanded to include the Red Sea which was sampled during the years 1895-1898. The ship sampled a range of different oceanographic variables from depth soundings to isotherm and isohaline charts, as well as dredging samples of deep-sea life. The data used for this study were recorded by Josef Luksch, a marine physicist on board the expeditions. The methodology used for obtaining the Secchi Depth differed from the modern standardised method and instrument as standardisation didn’t occur until decades after the Pola cruises. As such, the Secchi depths were recorded using a slightly larger disk of 45 cm diameter (with occasional use of a 2 m disk) deployed from the shady side of the ship. To measure ocean colour a scale of coloured liquid vials was created, ranging from 0 to 11, and the water colour was compared to these coloured vials. The recorded vial colour measurements were later compiled and digitised, and Forel-Ule colour values were estimated from the recorded vial numbers by Marcel Wernand. The final dataset covers the period 1890-1898 and comprises the translated Forel-Ule colour scale values, Secchi depth measurements, latitude and longitude coordinates, as well as the year, month, and day for each recorded observation.
Satellite data were obtained from the dataset created by Pitarch et al. (2021), containing monthly averaged maps of Forel-Ule scale and Secchi depth from 1998 to 2018 (Pitarch et al., 2019b). These values were calculated from satellite-obtained remote sensing reflectance with a monthly frequency and projected on a 2.5 arcmin rectangular grid, corresponding to approximately a 4 km spatial resolution at the equator and decreasing towards the poles (Pitarch et al., 2021). Full technical details about the algorithms used for this retrieval are found in van der Woerd and Wernand (2015) and Lee et al. (2015). From this dataset Forel-Ule and Secchi depth values were extracted with the minimum distance to each historical observations’ coordinates and from the same month. This process aimed to ensure the closest spatial and temporal match possible, thereby minimising any effect this difference may have on the analysis. This matching was performed using a monthly scale instead of a coarser yearly or finer weekly for several reasons. Firstly, due to the lack of uniform sampling across the expedition a finer temporal scale would have resulted in fewer matched observations (as there are more gaps in satellite data due to clouds and swath coverage at weekly scales) making it more challenging to observe clear trends. Secondly, considering the large temporal difference of around 120 years between the datasets, matching them at any finer time scale than monthly is likely to have limited impact on our analysis.
The year 2008 was chosen for the retrieval of remote sensing data, in order to minimize the effect of other large scale global circumstances that are known to influence the regions, such as the El Niño-Southern Oscillation (ENSO) (Raitsos et al., 2015; Basterretxea et al., 2018). The period in which the original dataset was collected (1890-1899) was during a period of strong La Niña (Wolter and Timlin, 2011), similar to conditions experienced in 2008 (https://psl.noaa.gov/enso/mei/). The year 2008 also had very good satellite spatial coverage, with three ocean colour satellites (SeaWiFS, MERIS and MODIS-Aqua) all running (Yu et al., 2023), maximising potential coverage available in the Ocean Colour Climate Change Initiative (OC-CCI) merged ocean colour product used in Pitarch et al. (2019b). As a confirmation step, the analysis was also replicated on other years between 1998 and 2018, to investigate whether any significant trends observed in 2008 persisted across different years. Additionally, a monthly climatology of the data between 1998 and 2018 was created and analysed as an alternative option to using a single year.
Sun elevation is known to affect Secchi disk measurements in blue waters (Pitarch, 2020), and so to ensure this was not significantly affecting the results, sun angle values were calculated using the time of day and year data. The analysis was repeated by removing any values in blue waters (Forel-Ule< 2) with a sun angle greater than 70 degrees.
Statistical tests
To investigate the differences between the observed historical measurements and remote sensing estimates the following statistical tests were adopted. These tests are commonly used for comparisons between models and in-situ data (Brewin et al., 2023), and therefore the satellite data were treated as modelled data, and the historical measurements were treated as in-situ data.
The absolute Root Mean Square Difference (RMSD) was calculated according to
where, X is the variable and N is the number of samples. The superscript S denotes the satellite estimated variable and the superscript M denotes the measured historical variable.
The bias (δ) between the satellite estimation and measurement can be expressed according to
The absolute centre-pattern (or unbiased) Root Mean Square Difference (RMSDCP) was calculated according to
This describes the difference of the satellite values with respect to the measured values regardless of the average bias (i.e. the standard deviation). It can also be expressed as RMSDCP = (RMSD2 − δ2)0.5.
These statistical tests (Equations 1–3) represent the difference between the two means (δ) and the differences in variability between the two distributions (RMSDCP). Together, they provide substantial insights into the similarities between the two distributions.
The historic dataset was also checked for any irregular or unexpected results. Given the previously established inverse relationship between Secchi depth and Forel-Ule colour (Wernand, 2011; Pitarch et al., 2019a), the presence of this relationship was verified in both the satellite and historical dataset. Paired t-tests were used throughout the study as the datapoints in both the historic and satellite data were obtained from the same location and were being directly compared to one another.
To examine monthly changes in Secchi depth, a climatology was calculated for both datasets, plotting the distribution and mean value for each month. This climatology was created using the total datasets instead of the direct comparisons used for the statistical testing. This was done to avoid losing any historical data from lack of remote sensing data availability. Climatology results are presented in “box and whisker” diagrams, chosen for their simplicity in representing the properties of the datasets investigated. Statistical tests were also run for each month, calculating δ, RMSD, RMSDCP values, as well as performing paired t-tests between the historical and satellite observations. T-tests were used as some months have a low number of samples and the t-test is designed for investigating differences in means between small sample sizes. This process was then repeated for the Forel-Ule colour scale.
Spatial differences were investigated by dividing the datapoints between the Red Sea and the Mediterranean Sea. Any data point recorded at a latitude greater than 31.26° North was treated as being in the Mediterranean Sea as this is the northernmost point of the Suez Canal, and those at a latitude lower than 29.9° North were treated as being in the Red Sea as this is the southern tip of the Suez Canal. Datapoints within the narrow Suez Canal were removed from the analysis due to a lack of satellite coverage (n = 26). The distribution of values was plotted on box plots along with the mean value. The statistical values δ, RMSD, RMSDCP were then calculated for each location for both Secchi depth and Forel-Ule scale, alongside paired t-tests. To test the effectiveness of the dataset in describing spatial patterns within the study area, spatially interpolated plots of the data were created (see Supplementary Figures S1, S2).
Analysis of uncertainty
To compute uncertainties in the differences in Secchi depth and Forel-Ule colour between the two historical periods, we first require estimates of uncertainty in the individual data points. Not a trivial task, but we could make some basic decisions on this. For the in-situ data (historical), we can estimate uncertainty based on our current understanding of uncertainty when collecting data at the same location by multiple individuals.
- For in-situ Secchi depth measurements, we used an uncertainty of 10%. This is based on the average percent deviation among individuals collecting data at locations at a series of stations in the Atlantic Ocean (see Section 2.3.2 of Brewin et al., 2023). This relative error was converted to an absolute error for each in-situ measurement.
- For in-situ Forel-Ule colour measurements, we used an absolute uncertainty of 1.0 (scale unit). This was based on typical uncertainties in Forel-Ule colour reported in Burggraaff et al. (2021). Though Burggraaff et al. (2021) report uncertainties can be higher than 1.0, this value seems reasonable considering studies quantifying standard deviations in Forel-Ule colour among individuals collecting data at a set location in the ocean, typically report lower values of around 0.5 (Wernand and van der Woerd, 2010b; Brewin et al., 2023b).
For the modern satellite data, we can estimate uncertainty based on satellite validation studies.
- For satellite Secchi depth measurements, we used an uncertainty of 19.3%. This was based on a validation of the Secchi depth algorithm used by Pitarch et al. (2021) in Lee et al. (2015). This relative error was converted to an absolute error for each satellite Secchi depth measurement.
- For satellite Forel-Ule colour measurements, we used an uncertainty of 0.81. This value (0.81) was derived from the root-mean-square-deviation in a comparison of remote-sensing reflectance-based estimates of Forel-Ule colour (using the same method of van der Woerd and Wernand (2015) and Novoa et al. (2014)) with in-situ data in the Atlantic. Specifically, we computed this as the square root of the sum of the squared bias (-0.38) and unbiased- root-mean-square-deviation (0.71), reported in Figure 7A of Brewin et al. (2023).
Making these assumptions, we computed the absolute uncertainties for each data point. We then estimated the absolute uncertainty in the difference () between two corresponding data points at a given location, one historical [in-situ, denoted ] and one modern [satellite, denoted ]), by square rooting the sum of the squared estimates, such that
Given the uncertainties in the differences ( for individual data point matches, and following the laws of error propagation, we then propagated these uncertainties to the average uncertainty () among a dataset of length n, according to
Equations 4 and 5 were used to estimate the uncertainty () in the mean differences between the two historical periods, for the two variables (Secchi depth and Forel-Ule colour). It is important to note that this approach assumes that the uncertainties associated with each data point are independent and normally distributed.
Results
Suitability of data
The δ, RMSD and RMSDCP values for Forel-Ule and Secchi depth data were calculated for each year (Table 1) to test whether results from the year 2008 differed from other years (1998-2018). Any value outside the range of 1st quartile - 1.5IQR to the 3rd quartile + 1.5IQR was treated as an outlier. For both Forel-Ule and Secchi depth the result for the year 2008 fall within this range (Secchi depth: 1st quartile = -7.495, median = -7.090, 3rd quartile = -6.611, IQR = 0.884; Forel-Ule: 1st quartile = 0.489, median = 0.564, 3rd quartile = 0.661, IQR = 0.171). Overall, the δ results are tightly distributed suggesting that the results from the comparison were not sensitive to the selection of reference year (2008) from other years during the recent period (1998-2018). This analysis also shows that the climatology does not differ from the year selected for analysis. Filtered data (removing any values with a sun angle greater than 70 degrees and Forel-Ule< 2) also showed similar trends (Table 1).
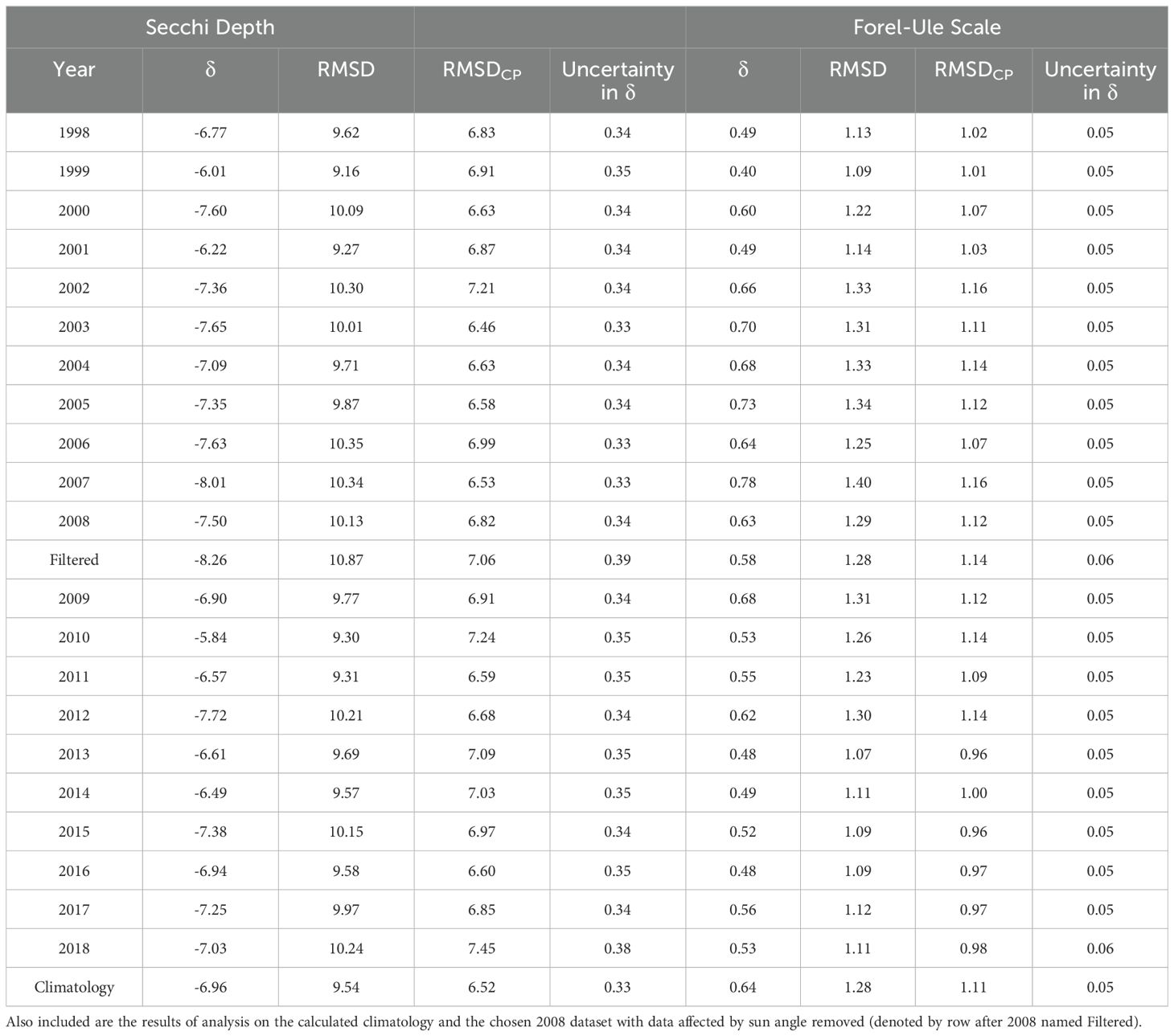
Table 1. The results of analysis on each year of satellite data, showing the δ, RMSD and RMSDCP for both Secchi depth and the Forel-Ule colour scale.
Spatially interpolated plots of the data (see Supplementary Figures S1, S2) revealed the presence of known oceanographic features (oligotrophic and mesotrophic biomes) that were consistent with the 2008 climatology. Some unusual finer scale differences were present in the interpolated products, suggesting the spatial coverage of the data was not well suited to make conclusions at sub-basin scales.
Total dataset
After removing datapoints from the Suez Canal, a total of 385 historical Secchi Disk measurements and 723 Forel-Ule colour recordings were present, and a total of 643 satellite estimations for each variable. This enabled a total of 343 comparisons for the Secchi Disk data and 643 for the colour scale. Paired t-tests performed for the whole dataset revealed a significant decrease in Secchi depth and an increase in the Forel-Ule between the historic and modern data (Secchi depth: t=-20.38, d.f. = 342, p< 0.01, Forel-Ule: t=15.00, df=642, p< 0.01). The total bias for Secchi depth was found to be -7.50 m (uncertainty of ±0.34 m) and the bias for the Forel-Ule scale 0.64 (uncertainty of ±0.05), suggesting a decrease in Secchi depth of approximately 0.06 m y-1 and an increase in the Forel-Ule scale of 0.005 y-1 over the ~123-year period.
Relationships between Secchi depth and Forel-Ule were investigated separately for the historical data and for the satellite data used in this study, and a log-linear model was found to fit the data closest (R2 = 0.767 for the historical data, R2 = 0.940 for the satellite dataset, see Figure 2). The model fits were broadly consistent (similar parameters) between the two datasets, and consistent with those reported in previous works (Figure 2).
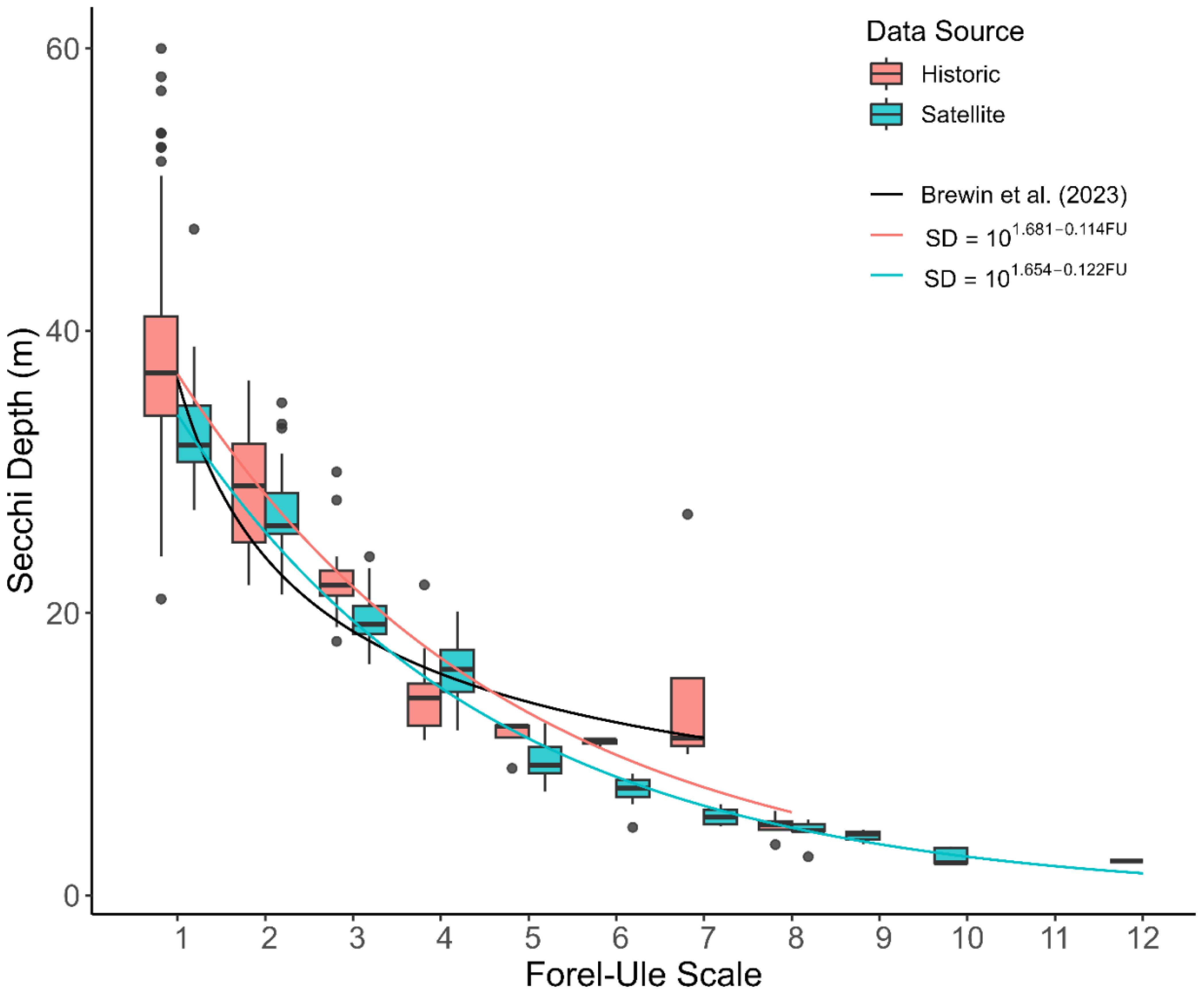
Figure 2. The relationship between observed Secchi disk and Forel-Ule scale. Boxes in red represent the historic data, and blue represents the satellite derived data. The relationship calculated by Brewin et al., 2023 is plotted in black (SD = 36.56FU-0.61 where SD represents Secchi depth and FU represents the Forel-Ule scale score). This relationship was calculated for Forel-Ule values up to 7 and is plotted for this range. Relationships were calculated for both the historical and satellite data used in this study and are plotted in red and blue respectively.
Changes by month
A total of 343 matchups of Secchi Depth were obtained, containing both historical and satellite measurements. Comparisons were possible for every month except May and June due to the lack of historical Secchi depth measurements. The distribution of measurements was uneven throughout the year, with some months having more samples than others (Table 2). The historic Secchi depth measurements were greater for every month measured (Figure 3). The greatest value was recorded in September, and the highest mean was in August. Overall, the trend across the year is similar between the modern and historical measurements, with lower values observed in the early and late months, and greater values in the late summer and early autumn.
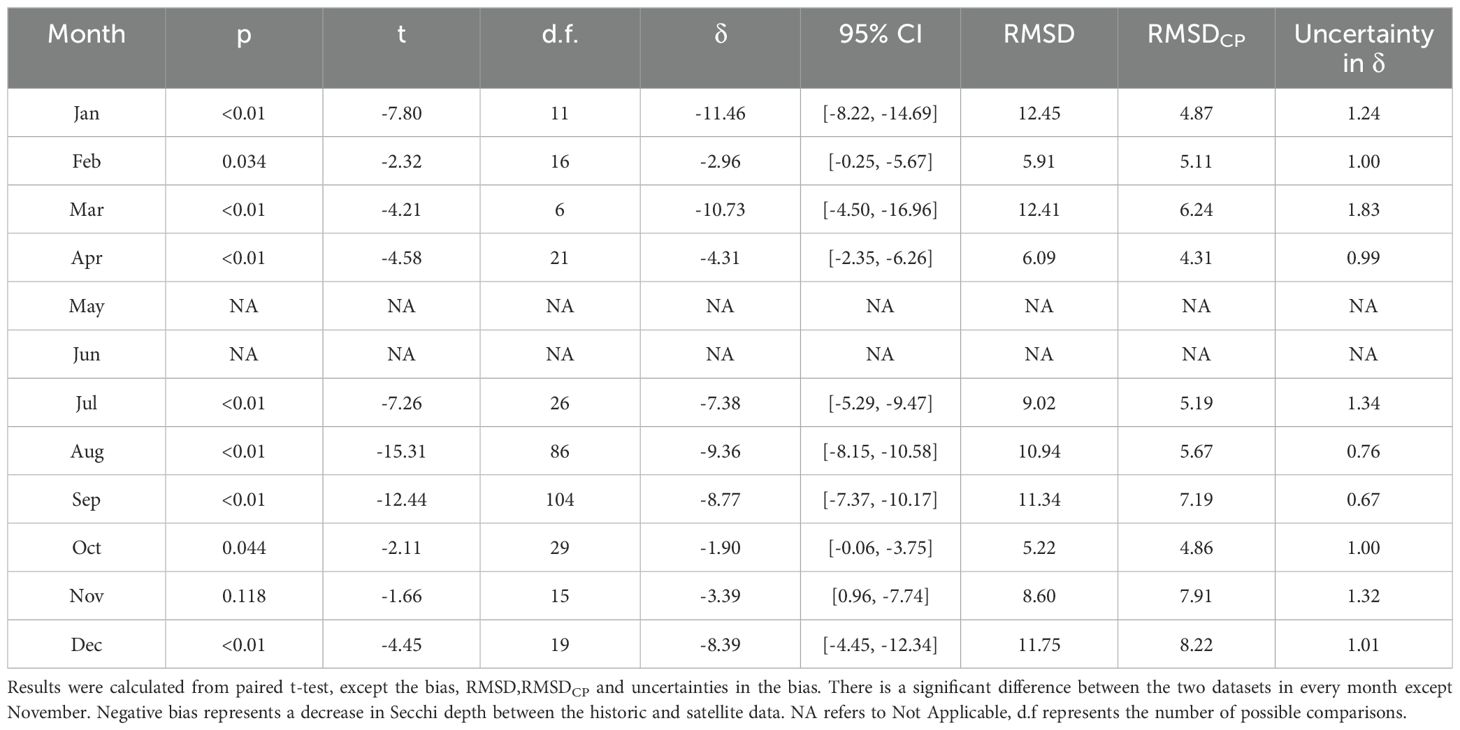
Table 2. p – values, t – score, degrees of freedom, bias, lower and upper 95% confidence interval of the bias, RMSD, RMSDCP and uncertainties in the bias calculated for Secchi depths grouped by month.
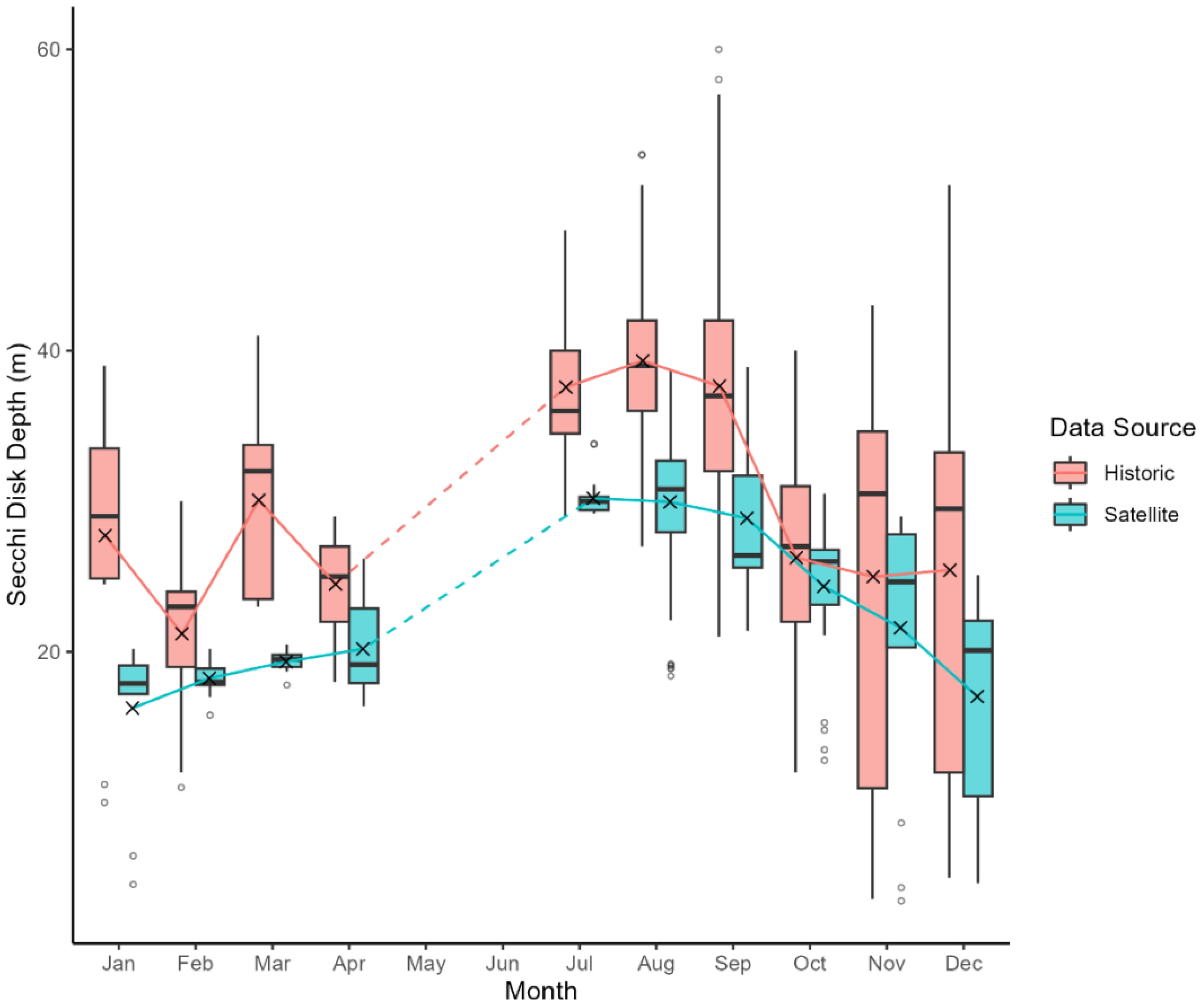
Figure 3. Boxplot comparing Secchi depth seasonal distribution between modern satellite data and in-situ historical data. Mean values are plotted as crosses on each box plot, and variations between the mean values are represented by coloured lines matching the box fill. Outliers are represented by empty circles above the limits represented by the lines outside the boxes. Dashed trendlines are drawn for months with no data. The mean and trendline are consistently higher in the historic data than in the satellite estimations.
Paired t-tests were performed between the datasets for each month, as well as δ, RMSD and RMSDCP calculations (Table 2). There was a significant negative bias in the Secchi depth between the historic and satellite measurements in every month except November, although a decrease was still present. The greatest bias was observed in January, with historic measurements over 10 m deeper on average than the satellite calculated observations. The greatest RMSDCP values were during December, meaning that these observations had the highest range (widest distribution).
Of the 749 historical measurements of Forel Ule, 650 satellite retrieved estimates were obtained for the modern era. The monthly distribution of these results across a year are plotted in Figure 4. The distribution of Forel-Ule data was more even across the year than with the Secchi depth data. Over 25 comparisons were feasible for all months except May and June. A similar trend is observed over the year for the two datasets, with the variation plotted revealing the same peaks and troughs over the seasons. The months of May and June were poorly sampled due to a low number of historical measurements (n = 4) and no matched satellite sampling (n = 0).
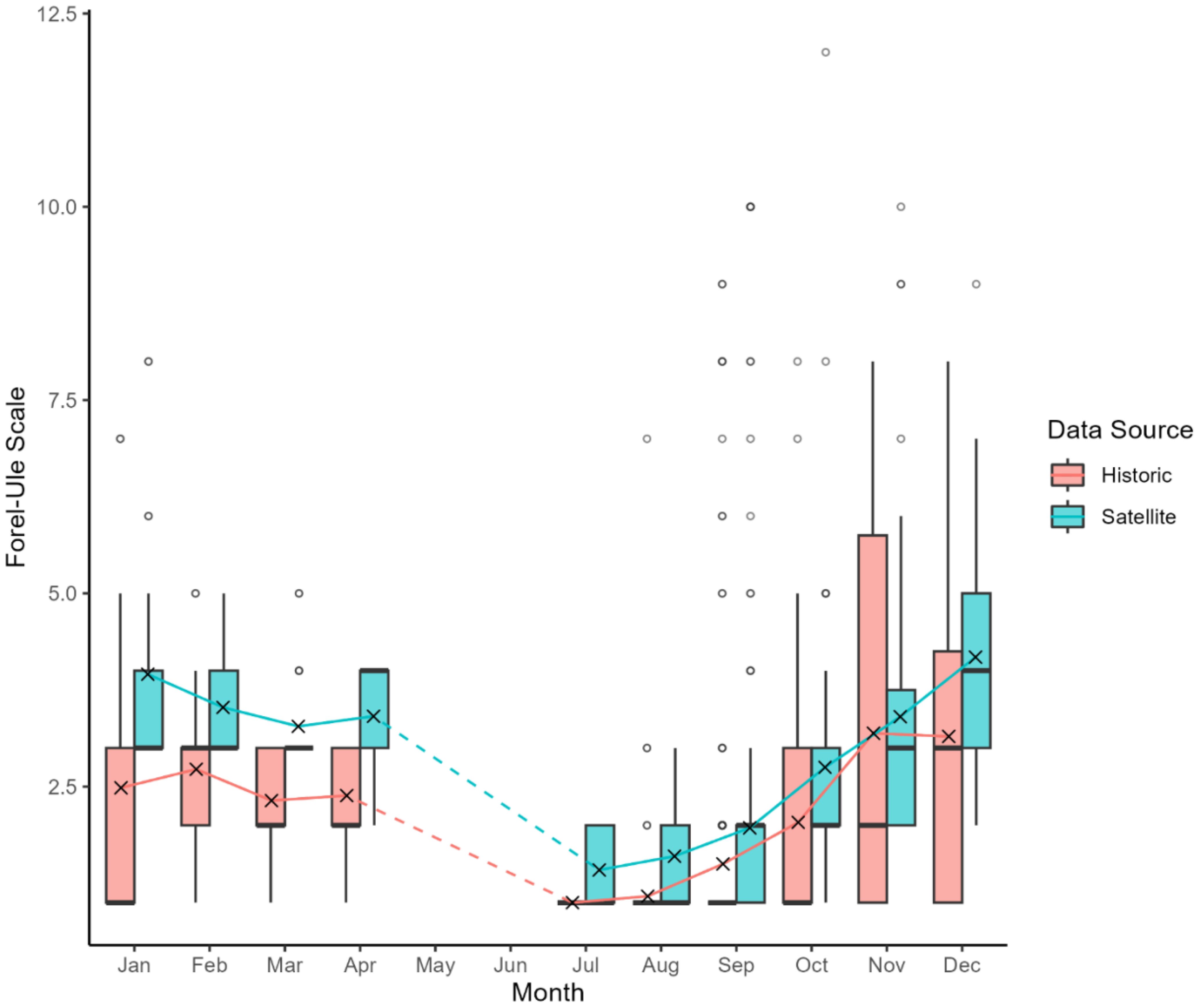
Figure 4. The distribution of Forel-Ule colour measurements grouped by month for both datasets. The means for each box are represented by a black cross, variations between the mean values are represented by coloured lines matching the box fill. The means for the satellite data are higher for every month and the highest mean results are recorded in the winter months, peaking in December. The lowest Forel-Ule values were observed during July and August for both datasets.
Because of the discrete nature of the Forel-Ule scale, and the extreme positive skewness of the values recorded (2.12 and 2.01 for the historic and satellite results respectively), the bias was less distinct over the year than the Secchi disk results. However, there is still a significantly positive difference present for every month compared except March and November (Table 3). The greatest bias was observed in January, with the latter along with April and December displaying a bias exceeding 1, meaning that the satellite retrieved data was on average at least 1 higher in the historical Forel-Ule scale data.

Table 3. p – values, t – score, degrees of freedom, bias, lower and upper 95% confidence interval of the bias, RMSD, RMSDCP and uncertainties in the bias calculated for the Forel-Ule scale grouped by month.
Overall, similar trends are seen across the year for both the Secchi depth and Forel-Ule scale across the two datasets, with the highest and lowest values seen in the same months for both satellite and historical data. The trends between the two variables are also similar, with the deepest Secchi depths corresponding to lower values in the Forel-Ule scale, observed in the late summer/early autumn.
Changes by location
The comparison between historical and satellite data revealed a significant decrease in Secchi depth recorded in both the Mediterranean and the Red Sea between the historic and satellite data (Med: t=-20.59, df=219, p< 0.01, paired t-test; Red Sea: t=-8.08, df=122, p<0.01, paired t-test) (Figure 5). The mean absolute bias value was greater in the Mediterranean (|δ| = 8.85 m, uncertainty 0.47 m), indicating that, on average, the decrease in Secchi depth was more pronounced in this region than in the Red Sea (|δ| = 5.07 m, uncertainty 0.44 m), though both regions indicate a significant reduction in Secchi depth, and by proxy, phytoplankton Chl-a concentration. A wide range of bias scores were recorded for both locations, shown in Figure 5, suggesting increases in Secchi depth varied within the two regions (see also Supplementary Figures S1, S2).
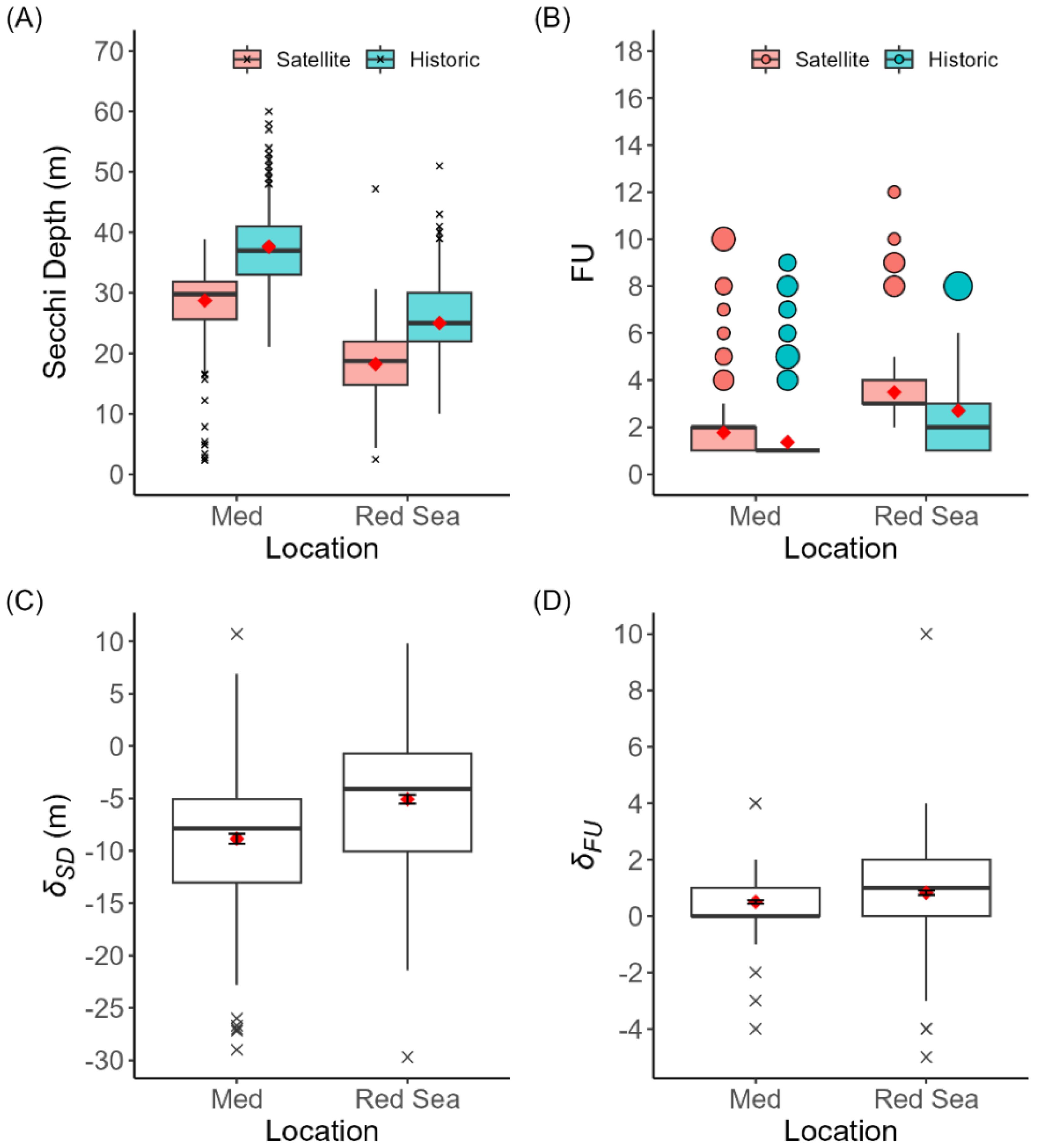
Figure 5. Boxplots of the distribution of measurements and biases for the two variables. Plots (A, B) display the distribution of measurements of Secchi depth and Forel-Ule scale respectively, with the boxplots grouped by location and subdivided by the data source. Plots (C, D) represent the distribution of bias scores for Secchi depth and Forel-Ule respectively. Subscript SD indicates the values displayed are calculated for Secchi depth, and subscript FU represents values calculated for the Forel-Ule scale. Across the plots the red diamond represents the mean of values plotted, and crosses plotted outside the boxes represent outliers excluding plot (B). For plot (B) points were used to represent outliers, with the size of the point equivalent to the number of points. This was to avoid not representing any data due to the discrete nature of the Forel-Ule scale. Error bars on plots (C, D) represent the uncertainty around the bias calculations.
There was a significant increase in the mean Forel-Ule recorded between the two sets of data for both the locations (Med: t=11.23, df=382, p< 0.01, paired t-test; Red Sea: t=9.74, df=266, p<0.01, paired t-test). The mean Forel-Ule values for the Mediterranean were 1.39 and 1.89 for the historical and satellite data respectively. In the Red Sea the mean Forel-Ule values were 2.67 and 3.50 for the historic and satellite data. The calculated bias value was greater for the Red Sea (δ = 0.83, uncertainty ±0.08) compared to the Mediterranean (δ = 0.50, uncertainty ±0.07). The greatest Forel-Ule increase recorded was 10, in the Red Sea, and the greatest decrease in the Forel-Ule scale was -7, in the Mediterranean.
Discussion
Overall, and considering our estimates of uncertainty, our results suggest a greening of the Mediterranean and Red Sea, in support of previous results (Wernand et al., 2013a), and a decrease in water clarity. This greening, although more pronounced in the Red Sea, was present in both regions. The seasonality in both the colour and clarity of the water was relatively consistent between the historical and modern datasets, as was the relationship between Secchi depth and Forel-Ule (Figure 2). The presence of similar differences between the historical and modern datasets, irrespective of the satellite reference years tested, provides confidence in the veracity of the trends reported.
Changes in colour (Forel-Ule)
The increase in the mean Forel-Ule calculated for the Mediterranean in this study is lower than reported previously in Wernand et al. (2013a). However, this may be due to the strict spatial data filtering process used in their study (samples within 100 km of the coast were excluded) which also included a low amount of data from intermediate years.
Although the absolute increases for the Forel-Ule scale in both locations are small, they can indicate a large change in Chl-a. For open, oligotrophic waters with lower Forel-Ule values there is an exponential relationship between the colour scale and Chl-a (Wernand et al., 2013b; Pitarch et al., 2019a). Using the equation from Brewin et al. (2023), to convert Forel-Ule to Chl-a, the mean Chl-a concentration may have increased on average from 0.24 mg m-3 to 0.34 mg m-3. The lack of high Forel-Ule scale values, typically observed in coastal or estuarine regions and shelf seas (Garaba et al., 2015; Li et al., 2021), indicate that phytoplankton are likely the dominate factor driving the change in ocean colour and clarity in our dataset. However, further verification is required to ascertain if such a large increase in Chl-a over this period is real.
Changes in clarity (Secchi depth)
The Secchi depth data broadly supports the trends revealed in the colour scale data. The decrease in water clarity observed in the modern dataset provides further evidence for an increase in optically active components in the water, and, when considered along with the Forel-Ule results, implies an increase in phytoplankton abundance and Chl-a. Using a blend of Secchi depth measurements and in-situ Chl-a, Boyce et al. (2010) reported a decrease in Chl-a in the easternmost Mediterranean Levantine Sea and Northern Red Sea, in contrast to our results, and an increase in the Southern Red Sea and the Aegean Sea. Differences between studies may possibility be caused by differences in the spatial grouping of data, with the Boyce et al. (2010) study including the Gulf of Aden not sampled in our study, as well as differences in the datasets used (their study did not utilise the historical dataset used here). Boyce et al. (2010) also applied one blanket relationship between Secchi depth and Chl-a for all the world’s seas, when this relationship differs between regions. Later work by Boyce et al. (2012) included Forel-Ule colour scale values, and a larger sample of Secchi depth and Chl-a measurements, however, excluded Forel-Ule values below 2 due to the saturation of the scale at this point. This led to the exclusion of a substantial portion of historical data for regions such as the Eastern Mediterranean and Red Sea and so trends were not reported in either region.
Seasonality
We observed no clear evidence for a change in phytoplankton phenology, with both the historical and satellite data following a similar seasonal trend. Both the Red Sea and Eastern Mediterranean experience the highest phytoplankton and Chl-a concentrations between December and April (Gittings et al., 2018; Salgado-Hernanz et al., 2019) consistent with the high Forel-Ule values and lower Secchi depths observed in those months. However, the greatest differences between historical and modern datasets were observed in these months, suggesting a possible increase in the magnitude of blooms, disproportionate to the increase seen across the dataset. It is worth considering that studies that have reported a change in timing in phytoplankton blooms report changes in the scale of weeks rather than months (Gittings et al., 2018) and so any change may potentially be masked by the coarser temporal scale used in this study. Furthermore, our uncertainty analysis does not consider uncertainty in seasonal changes in atmospheric properties that could be influencing atmospheric correction and consequently, satellite estimates of Secchi depth and Forel-Ule colour.
Spatial differences
Whilst both the Red Sea and Eastern Mediterranean are known to be oligotrophic, the southern Red Sea is characterised by a higher productivity than the northern area, due to the intrusion of nutrient-rich water masses from the Indian Ocean (Raitsos et al., 2015). Nonetheless, the Secchi depth data from the Mediterranean did include some lower values, suggesting that whilst predominantly unproductive, there are hotspots of productivity. The northern Aegean Sea and southern coastline of the Levantine Basin at the mouth of the Nile Delta are two areas of the Mediterranean sampled that typically have a high Chl-a concentration (Tsiaras et al., 2012; Kotta and Kitsou, 2019). The changes between the historic and satellite data for both variables show broadly the same trend, however, the decrease in Secchi depth was found to be greater in the Mediterranean than in the Red Sea, whilst the Forel-Ule score increased slightly more in the Red Sea than the Mediterranean (Figure 5). Finer spatial scale analysis revealed some spatial differences within the two regions (see Supplementary Figures S1, S2) but was limited by lower numbers of observations.
Potential drivers
Both the Red Sea and Eastern Mediterranean Sea are largely oligotrophic systems, highly stratified with plenty of light, meaning phytoplankton abundance at the surface is limited primarily by the availability of nutrients (Krom et al., 1991; Raitsos et al., 2013a), though light limitation can occur during some periods (Bellacicco et al., 2016). As a result of these conditions, an increase in phytoplankton is often associated with an increase in nutrient availability (Marañón et al., 2018). This increase in nutrients can come from several sources, including an increased degree of mixing of the water column by physical forcing, enabling access to nutrient-rich deep water, or horizontal advection of coastal nutrients from eddies (Raitsos et al., 2013a; 2017), or other external inputs on nutrients (Churchill et al., 2014), for example, from aeolian deposition or increased nitrogen fixation. In the Red Sea, a decrease in wind and wave heights have been reported (Langodan et al., 2017) as well as increases in temperature (Raitsos et al., 2011), which are likely to drive further stratification. Thus, increasing vertical mixing is unlikely to be the key driver behind the Forel-Ule increased values in the Red Sea. However, Raitsos et al. (2015) revealed that ENSO positive phases (and its extreme i.e., El Niño) lead to an increase in wind-driven advection of fertile waters from the Indian Ocean into the Red Sea, ultimately showing an increase in phytoplankton biomass during positive ENSO years (usually warmer years). In addition, Cai et al. (2014) reported that greenhouse warming has led to more frequent and abrupt occurrence of extreme El Niño events. Thus, changes in this climate index could potentially provide an explanation for the increase in phytoplankton abundance observed in this study in the Red Sea. Nonetheless, such links with El Niño are speculative and require further substantiation, since the data used in this study is not of sufficient temporal resolution to quantify El Niño related effects.
Another potential mechanism for the increase in nutrient availability in the Eastern Mediterranean is runoff from human activity and coastal settlements and subsequent nutrient enrichment. Additionally, aquaculture in the northern and southern Red Sea has been linked to nutrient enrichment in this area (Loya et al., 2004; Gokul et al., 2020), as have untreated effluent discharges due to inefficient or above capacity treatment plants (Jessen et al., 2013). In nutrient limited systems, this discharge may result in an increase in phytoplankton abundance, and in extreme cases eutrophication. Drivers of this observed change are likely to be similar in the Eastern Mediterranean due to similar oceanographic and nutrient conditions (Siokou-Frangou et al., 2010). In some areas of the sampled Mediterranean, eutrophication has been reported, primarily in coastal areas close to large urban centres (Pavlidou et al., 2015).
Limitations of historical methods
There are limitations in the usage of historic Forel-Ule data. The first is the saturation of the scale at a value of one, which makes it difficult to observe small variations in colour in oligotrophic systems. Recent analysis has recommended the creation and addition of a zero value to the scale (Pitarch et al., 2019a), enabling better identification of trends even in the bluest waters such as the oligotrophic gyres. This is not possible using historical data as we are limited to what was sampled originally. The second factor is the presence of differences when recording the Forel-Ule colour over a Secchi disk (as is often used in historic or in-situ measurements), or against the water column alone, as retrieved from satellites (Pitarch, 2017). This is not an issue in this study as the original cruise reports describe comparing the coloured solutions directly to the water column, rather than over a Secchi disk. The historical data used here also contains more observations of the Forel-Ule scale than Secchi depth, confirming that the colour scale is likely to have been observed over the water column directly. Recent optical modelling applied to data from the Atlantic Ocean has suggested that most historical Forel-Ule data were likely collected over the water column alone, and not over a Secchi disk (Brewin et al., 2023).
There may also be systematic differences in the historical methods used in these studies. The Secchi depth collected by Josef Luksch was collected primarily using a disk of 45 cm, as opposed to the standard 30 cm disk used in modern studies (Wernand, 2010). The original cruise performed by Secchi examined differences in visibility of disks measuring 43 cm and 237 cm and revealed that larger disks are visible at deeper depths, particularly in clear waters such as in this study (Secchi, 1865; Pitarch, 2020). Traditional Secchi depth theory (Preisendorfer, 1986) includes the influence of disk size on Secchi depth. Using a standard set of parameters as reported by Preisendorfer (1986), and for a Secchi depth of 24 m using a 30 cm disk (average Secchi depth of satellite data in this study for the modern period) and assuming a solar zenith angle of 10 degrees and wind speed of 10 ms-1, we estimate the Secchi depth to be around 4.2 m deeper using a 45 cm disk (size used on the “Pola” expeditions). Though significant, and certainly accounting for some of the decreasing Secchi depth trend between period, it does not account for the large differences observed (average of 7.7m +/- 0.34). Furthermore, more recent work has suggested the influence of disk size on Secchi depth can be much smaller than traditional theory suggests (Hou et al., 2007). Further work evaluating the difference in visibility of Secchi disks of variable sizes is recommended.
Implications of this study
This study is unique in analysing direct pairs of observations from historic and satellite estimations, by ‘resampling’ the original locations sampled by Luksch in the 19th century. Whilst this means that less data were used than in studies using spatially grouped means (Boyce et al., 2010; Wernand et al., 2013b) it allowed a more direct comparison by minimising spatial and temporal differences in sampling between historical and modern data. Overall, our analysis provides some evidence for an increase in phytoplankton abundance over the past century in the Red and Eastern Mediterranean Seas.
The observed decrease in Secchi depth has further ecological implications beyond an increase in phytoplankton. This decrease in Secchi depth would mean that light is more concentrated in the upper layer, which may have caused warming and an increase of stratification. Lower water transparency has direct implications for benthic habitats through changes in light availability for photosynthesis. The bodies of water examined contain coral reef systems (Berumen et al., 2013) and seagrass beds which may have been impacted (Simboura et al., 2019). Decreasing light availability, in conjunction with other stressors, such as rising temperatures or anthropogenic disturbances, may inhibit the growth and detrimentally affect the health of these benthic ecosystems (Grech et al., 2012).
Our results suggest changes may have happened to the baseline of the ecosystems of the Red Sea and Eastern Mediterranean over the past century through an increase in productivity. Increased sampling is required to continue monitoring these baselines and allow for effective management decisions to limit negative ecosystem impacts. Satellite sensing is believed to be the future for monitoring large bodies of water, yet studies such as this prove the need for further utilisation of the wealth of historical oceanographic data buried in archives. As such, the discovery, digitisation, and analysis of these historical datasets, alongside the evaluation of their power to estimate crucial variables such as Chl-a, including rigorous estimates of measurement uncertainty, may help understand long-term anthropogenic impacts on our seas.
Data availability statement
The original contributions presented in the study are included in the article/Supplementary Material. Further inquiries can be directed to the corresponding author.
Author contributions
JH: Formal analysis, Investigation, Methodology, Software, Validation, Visualization, Writing – original draft, Writing – review & editing. RB: Conceptualization, Formal analysis, Funding acquisition, Investigation, Methodology, Project administration, Resources, Supervision, Writing – review & editing. JP: Conceptualization, Data curation, Funding acquisition, Investigation, Methodology, Resources, Supervision, Writing – review & editing. DR: Funding acquisition, Supervision, Writing – review & editing.
Funding
The author(s) declare financial support was received for the research, authorship, and/or publication of this article. RB is supported by a UKRI Future Leader Fellowship (MR/V022792/1) and by the the Gordon and Betty Moore Foundation (GBMF11171). JP acknowledges funding by the European Union through the NextGenerationEU Program, Project IR0000032 – ITINERIS - Italian Integrated Environmental Research Infrastructures System - CUP B53C22002150006. DR acknowledges funding by the European Union HORIZON EUROPE program ACTNOW: Advancing understanding of Cumulative Impacts on European marine biodiversity, ecosystem functions and services for human wellbeing (Grant no. 101060072).
Acknowledgments
We acknowledge all those involved in the collection of data used in this study, particularly those involved in the “Pola” expeditions in the late 19th century. We are strongly indebted to early work by Marcel Wernand who pioneered the mining of these historical data allowing us to perform this analysis. This work was supported by a MSci project in Marine Biology at the University of Exeter, Penryn, Cornwall, and we acknowledge all the staff and students on that programme who helped make this work possible. We thank Dionysia Rigatou for reading an early version of our manuscript and providing useful textual edits. We thank the reviewers for insightful comments that helped us improve our paper.
Conflict of interest
The authors declare that the research was conducted in the absence of any commercial or financial relationships that could be construed as a potential conflict of interest.
Publisher’s note
All claims expressed in this article are solely those of the authors and do not necessarily represent those of their affiliated organizations, or those of the publisher, the editors and the reviewers. Any product that may be evaluated in this article, or claim that may be made by its manufacturer, is not guaranteed or endorsed by the publisher.
Supplementary material
The Supplementary Material for this article can be found online at: https://www.frontiersin.org/articles/10.3389/fmars.2024.1358899/full#supplementary-material
References
Alahmadi S., Al-Ahmadi K., Almeshari M. (2019). Spatial variation in the association between NO2 concentrations and shipping emissions in the Red Sea. Sci. Total Environment 676, 131–143. doi: 10.1016/j.scitotenv.2019.04.161
Basterretxea G., Font-Muñoz J. S., Salgado-Hernanz P. M., Arrieta J., Hernández-Carrasco I. (2018). Patterns of chlorophyll interannual variability in Mediterranean biogeographical regions. Remote Sens. Environment 215, 7–17. doi: 10.1016/j.rse.2018.05.027
Bellacicco M., Volpe G., Colella S., Pitarch J., Santoleri R. (2016). Influence of photoacclimation on the phytoplankton seasonal cycle in the Mediterranean Sea as seen by satellite. Remote Sens. Environ. 184, 595–604. doi: 10.1016/j.rse.2016.08.004
Berumen M. L., Hoey A. S., Bass W. H., Bouwmeester J., Catania D., Cochram J. E. M., et al. (2013). The status of coral reef ecology research in the Red Sea. Coral Reefs 32, 737–748. doi: 10.1007/s00338-013-1055-8
Boyce D. G., Lewis M. R., Worm B. (2010). Global phytoplankton decline over the past century. Nature 466, 591–596. doi: 10.1038/nature09268
Boyce D. G., Lewis M., Worm B. (2012). Integrating global chlorophyll data from 1890 to 2010. Limnol. Oceanogr.: Methods 10, 840–852. doi: 10.4319/lom.2012.10.840
Boyer T. P., Baranova O. K., Coleman C., Garcia H. E., Locarnini R. A., Mishonov A. V., et al. (2018). World Ocean Database 2018. A.V. Mishonov, Technical Ed. NOAA Atlas NESDIS 87. Available online at: https://www.ncei.noaa.gov/sites/default/files/2020-04/wod_intro_0.pdf.
Brewin R. J. W., Dall’Olmo G., Gittings J., Sun X., Lange P. K., Raitsos D. E., et al. (2022). A conceptual approach to partitioning a vertical profile of phytoplankton biomass into contributions from two communities. J. Geophysical Res.: Oceans 127, e2021JC018195. doi: 10.1029/2021JC018195
Brewin R. J. W., Pitarch J., Dall’Olmo G., van der Woerd H. J., Lin J., Sun X., et al. (2023). Evaluating historic and modern optical techniques for monitoring phytoplankton biomass in the Atlantic Ocean. Front. Mar. Sci. 10. doi: 10.3389/fmars.2023.1111416
Brewin R. J. W., Pitarch J., Dall’Olmo G., van der Woerd H. J., Lin J., Sun X., et al. (2023b). Modern and traditional optical measurements, and environmental data, collected on four Atlantic Meridional Transect cruises between 2013 and 2018 (NERC EDS British Oceanographic Data Centre NOC). doi: 10.5285/f3198e10-faf3-1525-e053-6c86abc0d2f6
Bryndum-Buchholz A., Tittensor D. P., Blanchard J. L., Cheung W. W. L., Coll M., Galbraith E. D., et al. (2019). Twenty-first-century climate change impacts on marine animal biomass and ecosystem structure across ocean basins. Glob. Change Biol. 25, 459–472. doi: 10.1111/gcb.14512
Burggraaff O., Panchagnula S., Snik F. (2021). Citizen science with colour blindness: A case study on the Forel-Ule scale. PloS One 16, e0249755. doi: 10.1371/journal.pone.0249755
Cai W., Borlace S., Lengaigne M., van Rensch P., Collins M., Vecchi G., et al. (2014). Increasing frequency of extreme El Niño events due to greenhouse warming. Nat. Clim Change 4, 111–116. doi: 10.1038/nclimate2100
Cantin N. E., Cohen A. L., Karnauskas K. B., Tarrant A. M., McCorkle D. C. (2010). Ocean warming slows coral growth in the central Red Sea. Science 329, 322–325. doi: 10.1126/science.1190182
Chaidez V., Dreano D., Agusti S., Duarte C. M., Hoteit I. (2017). Decadal trends in Red Sea maximum surface temperature. Sci. Rep. 7, 8144. doi: 10.1038/s41598-017-08146-z
Chassot E., Bonhommeau S., Dulvy N. K., Mé lin F., Watson R., Gascuel D., et al. (2010). Global marine primary production constrains fisheries catches. Ecol. Lett. 13, 495–505. doi: 10.1111/j.1461-0248.2010.01443.x
Churchill J. H., Bower A. S., McCorkle D. C., Abualnaja Y. (2014). The transport of nutrient-rich Indian Ocean water through the Red Sea and into coastal reef systems. J. Mar. Res. 72, 165–181. doi: 10.1357/002224014814901994
Doney S. C., Ruckelhaus M., Duffy J. E., Barry J. P., Chan F., English C. A., et al. (2012). Climate change impacts on marine ecosystems. Annu. Rev. Mar. Sci. 4, 11–37. doi: 10.1146/annurev-marine-041911-111611
Falkowski P., Wilson C. (1992). Phytoplankton productivity in the North Pacific Ocean since 1900 and implications for absorption of anthropogenic CO2. Nature 358, 741–743. doi: 10.1038/358741a0
Field C. B., Behrenfeld M. J., Randerson J. T., Falkowski P. G. (1998). Primary production of the biosphere: integrating terrestrial and oceanic components. Science 281, 237–240. doi: 10.1126/science.281.5374.237
Forel F. A. (1890). “Une nouvelle forme de la gamme de couleur pour l’étude de l’eau des lacs,” in Archives des Sciences Physiques et Naturelles/Société de Physique et d’Histoire Naturelle de Genève, vol. 6. , 25.
Garaba S. P., Voβ D., Zielinski O. (2015). Physical, bio-optical state and correlations in North–Western European shelf seas. Remote Sensing 6, 5042–5066. doi: 10.3390/rs6065042
Gittings J. A., Raitsos D. E., Krokos G., Hoteit I. (2018). Impacts of warming on phytoplankton abundance and phenology in a typical tropical marine ecosystem. Sci. Rep. 8, 2240. doi: 10.1038/s41598-018-20560-5
Gokul E. A., Raitsos D. E., Gittings J. A., Hoteit I. (2020). Developing an atlas of harmful algal blooms in the Red Sea: Linkages to local aquaculture. Remote Sensing 12, 3695. doi: 10.3390/rs12223695
Grech A., Chartrand-Miller K., Erftmeijer P., Fonseca M., McKenzie L., Rasheed M., et al. (2012). A comparison of threats, vulnerabilities and management approaches in global seagrass bioregions. Environ. Res. Letters 7, 24006. doi: 10.1088/1748-9326/7/2/024006
Henson S. A., Cael B. B., Allen S. R., Dutkiewicz S. (2021). Future phytoplankton diversity in a changing climate. Nat. Commun. 12, 1–8. doi: 10.1038/s41467-021-25699-w
Henson S. A., Sarmiento J. L., Dunne J. P., Bopp L., Lima I., Doney S. C., et al. (2010). Detection of anthropogenic climate change in satellite records of ocean chlorophyll and productivity. Biogeosciences 7, 621–640. doi: 10.5194/bg-7-621-2010
Hou W., Lee Z., Weidemann A. D. (2007). Why does the Secchi disk disappear? An imaging perspective. Optics Express 15, 2791–2802. doi: 10.1364/OE.15.002791
IPCC (2019). IPCC Special Report on the Ocean and Cryosphere in a Changing Climate. eds. Pörtner H.-O., Roberts D. C., Masson-Delmotte V., Zhai P., Tignor M., Poloczanska E., et al. (Cambridge, UK and New York, NY, USA: Cambridge University Press), 755. doi: 10.1017/9781009157964
Jessen C., Roder C., Villa Lizcano J. F., Voolstra C. R., Wild C. (2013). In-situ effects of simulated overfishing and eutrophication on benthic coral reef algae growth, succession, and composition in the central Red Sea. PloS One 8, e66992. doi: 10.1371/journal.pone.0066992
Kotta D., Kitsou D. (2019). Chlorophyll in the Eastern Mediterranean sea: correlations with environmental factors and trends. Environments 6, 98. doi: 10.3390/environments6080098
Krom M. D., Kress N., Brenner S., Gordon L. I. (1991). Phosphorus limitation of primary productivity in the eastern Mediterranean Sea. Limnol. Oceanogr. 36, 424–432. doi: 10.4319/lo.1991.36.3.0424
Langodan S., Cavaleri L., Vishwanadhapalli Y., Pomaro A., Bertotti L., Hoteit I. (2017). The climatology of the Red Sea – part 1: the wind. Int. J. Climatol. 37, 4509–4517. doi: 10.1002/joc.5103
Lee Z., Shang S., Du K., Wei J. (2018). Resolving the long-standing puzzles about the observed Secchi depth relationships. Limnol. Oceanogr. 63, 2321–2336. doi: 10.1002/lno.10940
Lee Z., Shang S., Hu C., Du K., Weidemann A., Hou W., et al. (2015). Secchi disk depth: A new theory and mechanistic model for underwater visibility. Remote Sens. Environment 169, 139–149. doi: 10.1016/j.rse.2015.08.002
Li M., Sun Y., Li X., Cui M., Huang C. (2021). An improved eutrophication assessment algorithm of estuaries and coastal waters in Liaodong bay. Remote Sensing 13, 3867. doi: 10.3390/rs13193867
Loya Y., Lubinevsky H., Rosenfield M., Kramarsky-Winter E. (2004). Nutrient enrichment caused by in situ fish farms at Eilat, Red Sea is detrimental to coral reproduction. Mar. Pollut. Bulletin 49, 344–353. doi: 10.1016/j.marpolbul.2004.06.011
Luksch J. (1901). Expeditionen SM Schiff “Pola im Mittelländischen, Ägäischen und Rothen Meere in den Jahren 1890–1898. Wissenschaftliche Ergebnisse XIX. Untersuchungen über die Transparenz und Farbe de Seewassers. Berichte der Commission für Oceanographische Foeschungen, Collectiv-Ausgabe aus dem LXIX Bande der Denkschriften Kaiserlichen Akademie der Wissenschafte, A. Forschungen im Rothen Meere, B. Forschungen im Östlichen Mittelmeere, 400–485.
Marañón E., Lorenzo M. P., Cermeño P., Mouriño-Carballido B. (2018). Nutrient limitation suppresses the temperature dependence of phytoplankton metabolic rates. ISME J. 12, 1836–1845. doi: 10.1038/s41396-018-0105-1
Mohamed B., Abdallah A. M., Alam El-Din K., Nagy H., Shaltout M. (2019). Inter-annual variability and trends of sea level and sea surface temperature in the Mediterranean Sea over the last 25 years. Pure Appl. Geophysics 176, 3787–3810. doi: 10.1007/s00024-019-02156-w
Morel A., Prieur L. (1977). Analysis of variations in ocean color. Limnol. Oceanogr. 22, 709–722. doi: 10.4319/lo.1977.22.4.0709
Novoa S., Wernand M. R., van der Woerd H. J. (2013). The Forel-Ule scale revisited spectrally: preparation protocol, transmission measurements and chromaticity. J. Of Eur. Optical Soc. - Rapid Publications 8, 13057. doi: 10.2971/jeos.2013.13057
Novoa S., Wernand M. R., van der Woerd H. J. (2014). The modern Forel-Ule scale: a ‘do-it-yourself’ colour comparator for water monitoring. J. Of Eur. Optical Soc. - Rapid Publications 9, 1–10. doi: 10.2971/jeos.2014.14025
Nykjaer L. (2009). Mediterranean Sea surface warming 1985–2006. Climate Res. 39, 11–17. doi: 10.3354/cr00794
Pastor F., Valiente J. A., Khodayar S. (2020). A warming Mediterranean: 38 years of increasing sea surface temperature. Remote Sens. 12, 2687. doi: 10.3390/rs12172687
Pavlidou A., Simboura N., Rousselaki E., Tsapakis M., Pagou K., Drakopoulou P., et al. (2015). Methods of eutrophication assessment in the context of the water framework directive: Examples from the Eastern Mediterranean coastal areas. Continental Shelf Res. 108, 156–168. doi: 10.1016/j.csr.2015.05.013
Pisano A., Marullo S., Artale V., Falcini F., Yang C., Leonelli F. E., et al. (2020). New evidence of Mediterranean climate change and variability from sea surface temperature observations. Remote Sens. 12, 132. doi: 10.3390/rs12010132
Pitarch J. (2017). Biases in ocean color over a Secchi disk. Optics Express 25, 1124–1131. doi: 10.1364/OE.25.0A1124
Pitarch J. (2020). A review of Secchi’s contribution to marine optics and the foundation of Secchi disk science. Oceanography 33, 26–37. doi: 10.5670/oceanog.2020.301
Pitarch J., Bellacicco M., Marullo S., van der Woerd H. J. (2021). Global maps of Forel–Ule index, hue angle and Secchi disk depth derived from 21 years of monthly ESA Ocean Colour Climate Change Initiative data. Earth Syst. Sci. Data 13, 481–490. doi: 10.5194/essd-13-481-2021
Pitarch J., van der Woerd H. J., Brewin R. J. W., Zielinski O. (2019a). Optical properties of Forel-Ule water types deduced from 15 years of global satellite ocean color observations. Remote Sens. Environment 231, 111249. doi: 10.1016/j.rse.2019.111249
Pitarch J., van der Woerd H. J., Brewin R. J. W., Zielinski O. (2019b). Twenty years of monthly global maps of Hue angle, Forel-Ule and Secchi disk depth, based on ESA-OC-CCI data. PANGAEA. doi: 10.1594/PANGAEA.904266
Preisendorfer R. W. (1986). Secchi disk science: Visual optics of natural waters. Limnol. Oceanogr. 31, 909–926. doi: 10.4319/lo.1986.31.5.0909
Raitsos D. E., Beaugrand G., Georgopoulos D., Zenetos A., Pancucci-Papadopoulou M. A., Theocharis A., et al. (2010). Global climate change amplifies the entry of tropical species into the eastern Mediterranean Sea. Limnol. Oceanogr. 55, 1478–1484. doi: 10.4319/lo.2010.55.4.1478
Raitsos D. E., Brewin R. J. W., Zhan P., Dreano D., Pradhan Y., Nanninga G. B., et al. (2017). Sensing coral reef connectivity pathways from space. Sci. Rep. 7, 9338. doi: 10.1038/s41598-017-08729-w
Raitsos D. E., Hoteit I., Prihartato P. K., Chronis T., Triantafyllou G., Abualnaja Y. (2011). Abrupt warming of the red sea. Geophys. Res. Lett. 38, L14601. doi: 10.1029/2011GL047984
Raitsos D. E., Pradhan Y., Brewin R. J. W., Stenchikov G., Hoteit I. (2013a). Remote sensing the phytoplankton seasonal succession. PloS One 8, e64909. doi: 10.1371/journal.pone.0064909
Raitsos D. E., Walne A., Lavender S. J., Licandro P., Reid P. C., Edwards M. (2013b). A 60-year ocean colour data set from the continuous plankton recorder. J. Plankton Res. 35, 158–164. doi: 10.1093/plankt/fbs079
Raitsos D. E., Yi X., Platt T., Racault M.-F., Brewin R. J. W., Pradhan Y., et al. (2015). Monsoon oscillations regulate fertility of the Red Sea. Geophysical Res. Letters 42, 855–862. doi: 10.1002/2014GL062882
Salgado-Hernanz P. M., Racault M.-F., Font-Munoz J. S., Basterretxea G. (2019). Trends in phytoplankton phenology in the Mediterranean Sea based on ocean-colour remote sensing. Remote Sens. Environment 221, 50–64. doi: 10.1016/j.rse.2018.10.036
Sathyendranath S., Brewin R. J. W., Brockmann C., Brotas V., Calton B., Chuprin A., et al. (2019). An ocean-colour time series for use in climate studies: the experience of the ocean-colour climate change initiative (OC-CCI). Sensors 19, 4285. doi: 10.3390/s19194285
Sathyendranath S., Brewin R. J. W., Jonsson B. F., Ciavatta S., Jackson T., Kulk G., et al. (2023). Ocean biology from space. Survey Geophysics 44, 1287–1308. doi: 10.1007/s10712-023-09805-9
Schefbeck G. (1996). “On the pathways of the "Pola" expeditions. The Austro-Hungarian Deep-Sea Expeditions: in Deep Sea and Extreme Shallow-water Habitats: Affinities and Adaptations. – Biosystematics and Ecology – 11, 1–27. Available online at: https://www.zobodat.at/pdf/BioEco_11_0001-0027.pdf.
Secchi P. A. (1865). Relazione delle esperienze fatte a bordo della pontificia pirocorvetta l’Immacolata concezione per determinare la trasparenza del mare; memoria del P. A. Secchi. Il Nuovo Cimento, (1855-1868). 20, 205–238. doi: 10.1007/BF02726911
Simboura N., Maragou P., Paximadis G., Kapiris K., Papadopoulos V. P., Sakellariou D., et al. (2019). “Greece,” in World Seas: an Environmental Evaluation, 2nd ed., vol. I . Ed. Sheppard C. (Academic Press, Europe, The Americas and West Africa), 227–260.
Siokou-Frangou I., Christake U., Mazzochi M. G., Montresor M., d’Alcala M. R., Vaque D., et al. (2010). Plankton in the open Mediterranean Sea: a review. Biogeosciences 7, 1543–1586. doi: 10.5194/bg-7-1543-2010
Sisma-Ventura G., Yam R., Shemesh A. (2014). Recent unprecedented warming and oligotrophy of the eastern Mediterranean Sea within the last millennium. Geophysical Res. Lett. 41, 5158–5166. doi: 10.1002/2014GL060393
Tilstone G. H., Pardo S., Dall’Olmo G., Brewin R. J., Nencioli F., Dessailly D., et al. (2021). Performance of Ocean Colour Chlorophyll a algorithms for Sentinel-3 OLCI, MODIS-Aqua and Suomi-VIIRS in open-ocean waters of the Atlantic. Remote Sens. Environ. 260, 112444. doi: 10.1016/j.rse.2021.112444
Tsiaras K. P., Kourafalou V. H., Raitsos D. E., Triantafyllou G., Petihakis G., Korres G. (2012). Inter-annual productivity variability in the North Aegean Sea: influence of thermohaline circulation during the Eastern Mediterranean Transient. J. Mar. Syst. 96-97, 72–78. doi: 10.1016/j.jmarsys.2012.02.003
van der Woerd H. J., Wernand M. (2015). True colour classification of natural waters with medium-spectral resolution satellites: Seawifs, MODIS, MERIS and OLCI. Sensors 15, 25663–25680. doi: 10.3390/s151025663
Wang S., Lee Z., Shang S., Li J., Zhang B., Lin G. (2019). Deriving inherent optical properties from classical water color measurements: Forel-Ule index and Secchi disk depth. Optics Express 27, 7642–7655. doi: 10.1364/OE.27.007642
Wernand M. R. (2010). On the history of the Secchi disc. J. Eur. Optical Soc - Rapid Publications 5, 10013s. doi: 10.2971/jeos.2010.10013s
Wernand M. (2011). Poseidon’s paintbox: historical archives of ocean colour in global-change perspective. (PhD Thesis). Utrecht University, Netherlands.
Wernand M. R., Hommersom A., van der Woerd H. J. (2013b). MERIS-based ocean colour classification with the discrete Forel–Ule scale. Ocean Sci. 9, 477–487. doi: 10.5194/os-9-477-2013
Wernand M. R., van der Woerd H. J. (2010a). Ocean colour changes in the North Pacific since 1930. J. Eur. Optical Soc - Rapid Publications 5, 10015s. doi: 10.2971/jeos.2010.10015s
Wernand M. R., van der Woerd H. J. (2010b). Spectral analysis of the Forel-Ule ocean colour comparator scale. J. Eur. Optical Soc - Rapid Publications 5, 10014s. doi: 10.2971/jeos.2010.10014s
Wernand M. R., van der Woerd H. J., Gieskes W. W. C. (2013a). Trends in ocean colour and chlorophyll concentration from 1889 to 2000, worldwide. PloS One 8, e63766. doi: 10.1371/journal.pone.0063766
Wilson L. J., Fulton C. J., Hogg A. M., Joyce K. E., Radford B. T. M., Fraser C. I. (2016). Climate-driven changes to ocean circulation and their inferred impacts on marine dispersal patterns. Global Ecol. Biogeogr. 25, 923–939. doi: 10.1111/geb.12456
Winder M., Sommer U. (2012). Phytoplankton response to a changing climate. Hydrobiologia 698, 5–16. doi: 10.1007/s10750-012-1149-2
Wolter K., Timlin M. S. (2011). El Niño/Southern Oscillation behaviour since 1871 as diagnosed in an extended multivariate ENSO index (MEI.ext). Int. J. Climatol. 31, 1074–1087. doi: 10.1002/joc.2336
Yao F., Hoteit I., Pratt L. J., Bower A. S., Zhai P., Köhl A., et al. (2014). Seasonal overturning circulation in the Red Sea: 1. Model validation and summer circulation. J. Geophys. Res. Oceans 119, 2238–2262. doi: 10.1002/2013JC009004
Ye M., Sun Y. (2022). Review of the Forel–Ule Index based on in situ and remote sensing methods and application in water quality assessment. Environ. Sci. pollut. Res. 29, 13024–13041. doi: 10.1007/s11356-021-18083-0
Keywords: phytoplankton, Forel-Ule colour scale, Secchi disk, ocean colour, climate change
Citation: Heath JR, Brewin RJW, Pitarch J and Raitsos DE (2024) Detecting centennial changes in the clarity and colour of the Red and Eastern Mediterranean Seas by retracing the “Pola” expeditions. Front. Mar. Sci. 11:1358899. doi: 10.3389/fmars.2024.1358899
Received: 20 December 2023; Accepted: 29 July 2024;
Published: 22 August 2024.
Edited by:
Astrid Bracher, Alfred Wegener Institute Helmholtz Centre for Polar and Marine Research (AWI), GermanyReviewed by:
Ana B. Ruescas, University of Valencia, SpainAndrew Banks, Hellenic Centre for Marine Research (HCMR), Greece
Copyright © 2024 Heath, Brewin, Pitarch and Raitsos. This is an open-access article distributed under the terms of the Creative Commons Attribution License (CC BY). The use, distribution or reproduction in other forums is permitted, provided the original author(s) and the copyright owner(s) are credited and that the original publication in this journal is cited, in accordance with accepted academic practice. No use, distribution or reproduction is permitted which does not comply with these terms.
*Correspondence: Robert J. W. Brewin, ci5icmV3aW5AZXhldGVyLmFjLnVr
†Present address: Jonathan R. Heath, School of Ocean Sciences, Bangor University, Menai Bridge, United Kingdom