- 1College of Marine Science, Shanghai Ocean University, Shanghai, China
- 2South China Sea Fisheries Research Institute, Chinese Academy of Fishery Sciences, Guangdong Provincial Key Laboratory of Fishery Ecology and Environment, Scientific Observing and Experimental Station of South China Sea Fishery Resources and Environment, Ministry of Agriculture and Rural Affairs, Guangdong Engineering Technology Research Center of Marine Recreational Fishery, Key Laboratory of Marine Ranching Technology, CAFS, Guangzhou, China
- 3Southern Marine Science and Engineering Guangdong Laboratory (Zhuhai), Zhuhai, China
- 4Department of Optoelectronic Engineering, Jinan University, Guangzhou, China
- 5College of Fisheries and Life Science, Dalian Ocean University, Dalian, China
Evaluation of the ecological carrying capacity of marine ranching is a prerequisite and guarantee for ensuring the sustainable development of aquaculture activities, protecting and maintaining the stability and security of marine ecosystems. Based on high-resolution satellite remote sensing data and survey data of Wailingding marine ranching in Zhuhai from 2006 to 2021, this study constructed a marine ecological carrying capacity (MECC) index system with three levels: resources, environment, and ecology. A combination of the analytic hierarchy process (AHP) method and entropy method was applied to assign values to indexes, and then, the MECC of marine ranching was evaluated based on the state-space model and the weighted Bonferroni mean (WBM) model. Results showed that the MECC of Wailingding marine ranching increased from 0.49 (2006) before the marine ranching construction to 0.79 (2021) after the marine ranching construction. Marine ranching had elevated MECC from a loadable state to a fully loaded state. The intertidal subsystem, with a weight of 52.51%, dominates the MECC of marine ranching, in which resource and environmental factors are the major influencing factors. The increased rate of the state-space model (61.73%) was higher than that of the WBM model (44.34%), indicating that the state-space model is more feasible for the MECC assessment of Wailingding marine ranching.
1 Introduction
Marine ranching is a new type of fishery model based on the principle of marine ecosystem, taking measures such as putting artificial reefs, stocking and releasing in specific sea areas to build or repair the places needed for the growth, baiting, enemy avoidance, and reproduction of marine organisms, so as to achieve the purpose of stocking and conserving fishery resources and improving the ecological environment of the sea area, thereby realizing the sustainable protection of fishery resources (Chen et al., 2013). Compared with the traditional ones aimed at fishery production, modern marine ranching integrates modern engineering technology and management theories, developing from relying solely on ecological engineering to systematically managing biological resources, ecological environment, fishery production, and related cultural and leisure activities, forming a comprehensive system of people, fish, land, and sea. It is an effective carrier of the primary, secondary, and tertiary industries, with a greater emphasis on ecological attributes, environmental protection, ecological restoration, and resource conservation, covering seedling expansion and ecological development (Yang et al., 2019). It directly affects the carrying capacity of the marine ecosystem by changing the structure of food webs and their potential productivity (Vasconcellos and Gasalla, 2001). It is of great significance in carbon sequestration in the ocean, improving the seabed observation network, and promoting the construction of comprehensive marine pastures (Yang et al., 2018a; Chen, 2020). A responsible stock enhancement should not only increase total production and stock abundance, but also consider the potential impacts on ecosystem structure and function. Therefore, assessing the ecological carrying capacity of marine ranching, identifying potential environmental and ecological risks, and predicting factors that may influence the ecological environment are necessary prerequisites and safeguards to ensure the sustainable development of stock enhancement and to preserve the stability and security of marine ecosystems.
Marine ecological carrying capacity (MECC) is a synthesis of resource carrying capacity and environmental carrying capacity, which refers to the ability or limit of the ocean to support the coordinated development of population, environment, and economy within a certain period time, based on the principle of sustainable utilization of marine resources and the non-destruction of the marine ecosystem through the ocean’s self-regulation and self-maintenance under the material standard of living that meets the current stage of socio-cultural norms, while marine resources can be sustainably utilized and marine ecological environment is not destroyed (Di et al., 2014). It can be divided into resource supply capacity, environmental constraint capacity, ecological elasticity, and human influence. Initially, the focus of marine carrying capacity was primarily on determining sustainable thresholds for fish, shellfish, and other marine resources (Suo et al., 2023). Later, with the continuous development and utilization of marine resources and environment, studies gradually expanded from single factor to comprehensive carrying capacity (Chapman et al., 2013; Borja et al., 2022; Theodora and Spanogianni, 2022), and also included marine ecosystem service function study (Ma et al., 2019) and marine ecosystem health study (Zhao et al., 2016). At present, the state-space method, supply–demand analytical method, and system dynamics method are the major approaches to evaluate the MECC quantitatively. The state-space method evaluates the ecosystem carrying capacity by establishing an index system, such as the Pressure-State-Response (PSR) model. On this basis, the model had been extended as the Driver-Pressure-State-Impact-Response (DPSIR) model (Li et al., 2023b), the Pressure and Support-Destructiveness and Resilience-Degradation and Promotion (PS-DR-DP) model (Ying et al., 2022), and the Driver-Pressure-State-Ecosystem Service-Response (DPSIR) model (Chapman et al., 2013), among others. Since ecological carrying is often assessed based on supply–demand conditions of the resource environment, the supply–demand analytical method uses the difference relationship between them to build a model. For example, a three-dimensional ecological footprint model has been successfully applied to assess the MECC of coastal urban agglomerations (Wang et al., 2020). The system dynamics method simulates MECC through mathematical calculation models. The MERAMOD model offers the possibility to simulate and predict the impact of the environment on the carrying capacity of marine fish farms (Yucel-Gier et al., 2019). In addition, some scholars have explored the use of a multidimensional approach. Du created the DPPD evaluation model, based on driving-pressure-state-impact-response (DPSIR), Principal Component Analysis (PCA), Path Analysis (PA), and Decision Making Trial and Evaluation Laboratory (DEMATEL), being applied to the MECC evaluation of 11 coastal areas in China (Du et al., 2020). Some new technologies, like image video processing and video sequence algorithms, have also been applied to the assessment of MECC (Huamantinco Cisneros et al., 2016), offering a fresh perspective for MECC evaluation. During the evaluation process, the methods to determine the weight of index can be categorized into subjective methods, objective methods, and a combination of both (Li et al., 2023b). Among them, subjective methods include the analytic hierarchy process (AHP) (Wang et al., 2017; Du and Gao, 2020; Zhang et al., 2023) and expert judgment (Du et al., 2022), while objective methods include the entropy weight method and PCA method (Du et al., 2020). Subjective methods were influenced by the subjective factors of the evaluator in the selection of criteria and the distribution of weights. Objective analysis relies on the information of the data itself. The combination of both can improve the accuracy of the evaluation to a certain extent.
Although previous studies have laid the foundation for the evaluation of MECC of marine ranching, there are still some problems. Spatially, major focus has been on large-scale coastal areas (Huamantinco Cisneros et al., 2016; Du et al., 2020; Wang et al., 2020; Ying et al., 2022) or islands (Nam et al., 2010), with a lack of understanding of the adjacent or embedded areas of islands. In terms of time, the main focus was on the ecological effects at the beginning of marine ranching construction, neglecting long-term monitoring and early warning (Huamantinco Cisneros et al., 2016; Zhao et al., 2016; Long et al., 2019). Regarding influencing factors, the major consideration was the in situ investigation of fishery resources and ecological environments (Liao et al., 2013; Wang et al., 2018; Feng et al., 2021; Feng et al., 2023), while many key hydrodynamic factors were rarely observed or continuously monitored at the same time or in the same location as biological surveys. As the construction of marine ranching is a regional comprehensive development, the ecological carrying capacity evaluation based on a large-scale, long-term, and comprehensive survey is a prerequisite. High-resolution remote sensing has advantages of long duration, high accuracy, and full coverage, which makes it possible to continuously monitor the dynamic variations of the geographical and habitat factors of marine ranching.
In this study, the MECC of the Zhuhai Wailingding National Marine Ranching Demonstration Zone was evaluated based on high-resolution satellite remote sensing. Furthermore, major factors affecting the MECC were analyzed. Results will contribute to a better understanding of the ecological variations of marine ranching and provide theoretical support for the scientific planning and sustainable development of modernized marine ranching.
2 Materials and methods
2.1 Research area
Wailingding Island is located in the Pearl River estuary, where seawater and freshwater intermingle. This island spans from 114°1′20.26″E to 114°3′5.91″E and 22°5′17.87″N to 22°6′46.24″N (Figure 1). It is part of the Wanshan Archipelago with an area of approximately 4.23 km2. The National Marine Ranching Demonstration Zone of Wailingding Island was established in the eastern sea area. Artificial reefs were first deployed in the Wailingding National Marine Ranching Demonstration Zones between February 2007 and February 2009, followed by a second placement in 2021. The area currently hosts 2,937 artificial reefs. According to the distance to the artificial reef area, 12 survey stations were set (indicated by S1, S2, S3, S4, S5, S6, S7, S8, S9, C1, C2, and C3 in Figure 1). Field investigation was conducted during the pre-artificial reefs deployment period in August 2006 and the post-artificial reefs deployment period in August 2009, September 2016, April 2020, September 2020, and September 2021, respectively. Among them, water quality, sediment, environmental factors, and fishery resources surveys were conducted at stations S1–S6, S8, and S10–S12, water quality surveys were conducted at stations S7 and S9, while the intertidal benthos investigation were undertaken at stations C1–C3.
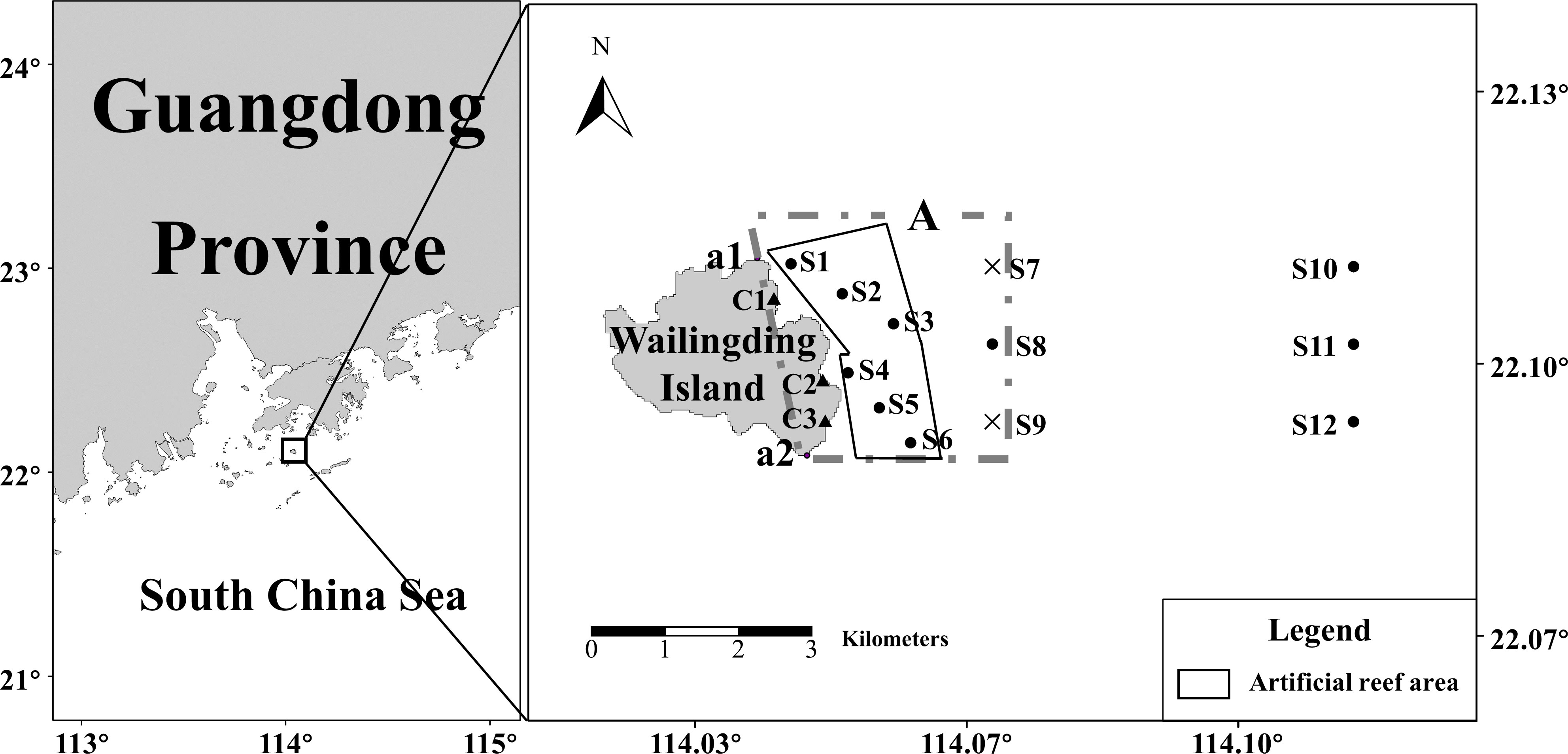
Figure 1 Research area and survey stations (box A is the area of satellite remote sensing data, and the land part between A1 and A2 is the area of remote sensing data of intertidal area).
2.2 Data process
All investigations, analysis,and identification were carried out following the Specification for Marine Monitoring (GB17378-2007) and the Specification for Oceanographic Survey-Marine Biological Survey (GB12763.6-2007). The survey data included seawater quality [temperature, depth, and salinity (‰)], dissolved inorganic nitrogen (DIN), reactive phosphate (PO43-P), marine organism (intertidal organisms, phytoplankton, zooplankton, benthic organisms, swimming organisms, fish larvae, and so on), and chlorophyll a concentration (Chl-a). Satellite remote sensing data included sea surface Chl-a, current, and intertidal data. In this study, as the high-resolution satellite was launched in 2018, Chl-a in 2006, 2009, and 2016 were survey data, and Chl-a in 2020 and 2021 were satellite remote sensing data. Among them, the Chl-a were obtained from the China HY-1 C satellite (https://osdds.nsoas.org.cn/), with a temporal resolution of 1 day and a spatial resolution of 50 m. The current data were from Global Ocean Physical Reanalysis Product of the Copernicus Marine Environment Management Service (CMEMS, http://marine.copernicus.eu/). Five remote sensing images were used to extract the intertidal area (Table 1).
MATLAB software were used to extract Chl-a information, removing the invalid value and calculating the monthly average Chl-a. Coastline length was extracted by high-resolution remote sensing data (Table 1). ENVI software was applied to preprocess the coastline length (the land area between A1 and A2 of box A in Figure 1), including reading, radiometric calibration, atmospheric correction, and image fusion. The FVCOM tidal model was used to compute the elevation when the satellite passed, and then it was inserted into the tidal level calculation formula (Wang and Chu, 2005) to get the extent of the intertidal zone. Finally, the length of the coastline and the area of the island and beach were calculated in ArcGIS 10.3 (Esri, Redlands, CA, USA).
2.3 Methods
2.3.1 Marine ecological carrying capacity index system
According to the concept of MECC, it should include resource supply capacity, environmental constraint capacity, ecological elasticity, and human influence (Fu et al., 2009; Di et al., 2014). Compared to land studies, as a small and medium-sized sea area, MECC of marine ranching is relatively simple. Therefore, the carrying capacity of the ocean on the actual population was not considered in the present study. Indicators of resource supply capacity were mainly considered by the supply level of various types of marine resources. Indicators of environmental constraint capacity were selected from the quality of the marine environment and the capacity of the marine environment, respectively. Ecological elasticity indicators were selected from biodiversity and taxa structure. The marine ranching ecosystem integrates characteristics of wetlands and marine ecosystems (Song, 2006). In order to construct a comprehensive and unbiased evaluation model, the select indexes were based on the characteristics of different subsystems, and the marine ranching ecosystem was divided into the intertidal subsystem and the nearshore subsystem (Zhao et al., 2016; Du et al., 2020; Borja et al., 2022; Bui and Tran, 2022). The evaluation system was constructed by factors from three levels: resource, environment, and ecology, respectively (Carneiro, 1960) (Figure 2). The index system was divided into four layers, namely, the target layer (A), system layer (B), factor layer (C), and index layer (D). The target layer (A) was the MECC of the marine ranching layer, while the system layer (B) was the intertidal subsystem layer and the nearshore subsystem layer. The factor layer (C) included the resource layer, environment layer, and ecology layer. The index layer consisted of 24 indexes. Indexes were divided into a positive index, a negative index, and a moderate index. The positive index means the effect is promoting, and the larger the index value, the better. The negative index means the effect is inhibitory, and the smaller the index value, the better. A moderate index means there is a reasonable range of the ideal value, which should not be too high or too low.
The positive index included natural shoreline (D1, shoreline between A1 and A2 in box A in Figure 1), beach retention rate (D2), island area (D3), vegetation coverage (D4), biomass (D5), biodiversity index (D6), artificial reef area (D8), species of fish eggs and larvae (D15), benthic biomass (D16), benthic biodiversity index (D17), zooplankton biomass (D18), zooplankton biodiversity index (D19), phytoplankton density (D20), phytoplankton biodiversity index (D21), nekton density (D22), and nekton diversity index (D23). The negative index included open aquaculture area (D7), dissolved inorganic nitrogen concentration (D9), and reactive phosphate concentration (D10). The moderate index included temperature (D11), salinity (D12), depth (D13), current velocity (D14), and Chl-a (D24).
2.3.2 Calculation of index
(1) Beach retention rate
The beach retention rate () is used to indicate the variation of the intertidal zone (Su et al., 2018), which is calculated as follows:
where Si is the intertidal area in i year; is the intertidal area in 1990.
(2) Vegetation coverage
Vegetation coverage (VC) is one of the important indexes that indicate the change of beach environment, and the Normalized Different Vegetation Index (NDVI) is used to extract the vegetation area from remote sensing data (Chen et al., 2001). Wailingding Island is located in the subtropical monsoon climate area, where VC varies little from season to season (Xie et al., 2022; Wu et al., 2022b).
where is the area covered by vegetation; SI is the area of island.
(3) Diversity index
The Shannon–Weaner index () (Wilhm, 1968) is used to indicate species diversity of organisms, which is calculated as follows:
where S is the total number of species captured at each station and is the ratio of the number of individuals of the ith species to the total number of individuals of all species.
(4) Data standardization
Owing to the different nature, units, and quantity level of index, standardization is required to eliminate the effect of the scale between the raw data (Wang, 1999). In order to avoid the occurrence of value 0 after normalization, an adjustment index k was added. The moderate index was standardized by introducing ideal values, which were obtained from the opinions of local experts, scholars, government decision-makers, or existing international and domestic standards. The reduction of calculation errors can be achieved by employing diverse methods tailored to specific index types (Yue et al., 2020; Yu and Du, 2023).
where is the standardized value of each index, xi is the original value of each index, is the ideal value of each index, and xmin are the maximum and minimum values of each index, respectively, and k is the adjustment coefficient of 0.01.
2.3.3 Index weighted
In order to avoid the bias caused by subjective factors and data itself, the weights calculated by AHP (Saaty and Kearns, 1985) and entropy method (Zou et al., 2006) were integrated, which is calculated as follows:
where is the combined weight, is the weight calculated by AHP, and is the weight calculated by the entropy method.
2.3.4 Evaluation model
(1) Three-dimensional state-space model
The three-dimensional state-space model is an evaluation method that uses vector modes (e.g., OD) composed of the origin point and system state points in a coordinate system to represent the ecological carrying capacity value (Mao and Yu, 2001). In the three-dimensional state space, each point represents the spatial combination of the resource environment at a certain moment, and the position of the point in space is used to determine the carrying capacity of the ecosystem (Figure 3). There is a surface ABC corresponding to resource, environmental, and ecological allocation at any point on the surface reach a balance. When the point is lower than the ABC surface, it indicates that MECC is loadable; when the point is higher than the ABC surface, it indicates that MECC is overloaded.
where n is the number resource indexes, m is the number of environmental indexes, p is the number of ecological indexes, Ri is the standard value of each resource index, Ii is the standard value of each environmental index, and is the standard value of each ecological index.
The range of the standard value is 0–1, then the ideal value of MECC is:
The vector mode of MECC when overloaded is larger than that of the ideal state; in contrast, the vector mode of MECC when loadable is smaller than that of the ideal state. Based on the ecosystem complexity and fluctuation of its development, the tolerance of criterion was set to 0.3 (Wang, 2007). The evaluation criteria were as follows:
When MECC ≥ 1.2, overloaded;
When 0.9 ≤ MECC< 1.2, fully loaded;
When MECC< 0.9, loadable.
(2) Weighted Bonferroni mean
The weighted Bonferroni mean (WBM) was applied to evaluate MECC through the iterative algorithm (Xia et al., 2012). It uses standardized results of each index as attribute variables and the combined weights after the multiplication of subjective and objective weight as the weight vector of variables, and calculates MECC through an iterative algorithm.
Where Si is the value of MECC, m is the number of index, and p = q = 1.
3 Results
3.1 Beach retention rate
ENVI and ArcGIS software were applied to process the high-resolution remote sensing images of Wailingding Island (Figure 4), and the length of natural shoreline, the area of the island, the beach retention rate, and the vegetation coverage were calculated (Equations (1) and (2), Figure 5). Results showed a small change in the area of the island during 2006–2021, with a mean value of 4.58 km2 and a gap of 0.28 km2 between the maximum and minimum values (Figure 5A). The average length of the natural shoreline was 4.28 km, with a maximum value of 4.81 km in 2020 and similar values in other years (Figure 5A). The beach retention rate of Wailingding Island remained at a consistently high level, with an average value of 86.8%. Notably, beach retention was greatest in 2021 at 92%, with a slight decline in 2009 and 2016 (Figure 5B). Vegetation coverage rate reached its maximum in 2009 at 83% and the minimum in 2016 and 2020 at 78%. It fluctuated approximately 80% between 2006 and 2021 (Figure 5B).
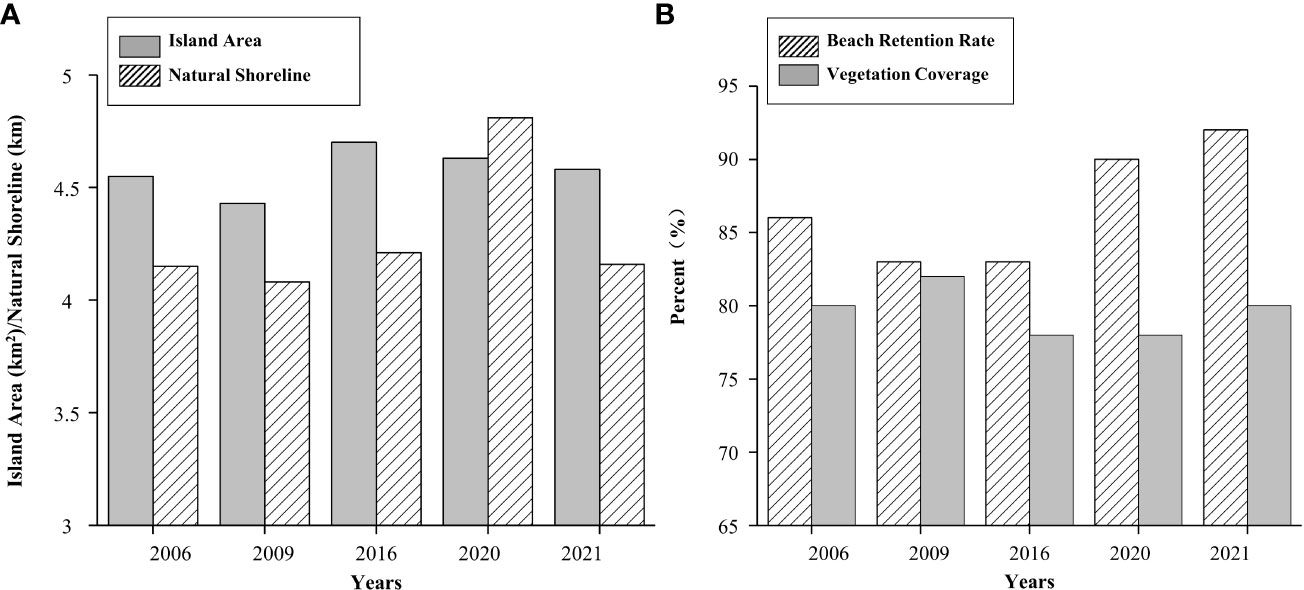
Figure 5 (A) Island area and natural shoreline of Wailingding Island, 2006–2021. (B) Beach retention rate and vegetation coverage of Wailingding Island, 2006–2021.
3.2 Evaluation system and combined weight
Biodiversity index is calculated according to Equation 3, the raw data of the positive index, negative index, and moderate index in the MECC system were dimensionless, according to Equations (4–6) (Table 2).
The weight of each index was calculated using AHP and the entropy weight method, respectively. Then, the results were integrated according to Equation (7). Combined weights of each index are as follows (Table 3). The intertidal subsystem dominated the MECC of marine ranching with a weight of 52.51%, followed by the nearshore subsystem with a weight of 47.49%. In the factor level, resource factor is the major influencing factor with a weight of 37.54%, followed by environmental factor (35.13%) and ecological factor (27.33%). In the index level, vegetation coverage (D4) held the highest weighting (19.18%), followed by beach retention rate (D2, 10.66%), open aquaculture area (D7, 10.13%) and biomass (D5, 9.62%). The index with the smallest weighting was Chl-a (D24) at 0.85%.
3.3 MECC of marine ranching
The state-space model and WBM were used to calculate MECC score of Wailingding marine ranching ecosystem Equations (8–10), as well as the intertidal subsystem and the nearshore subsystem, respectively. Results from the WBM and the state-space model were also compared (Figure 6).
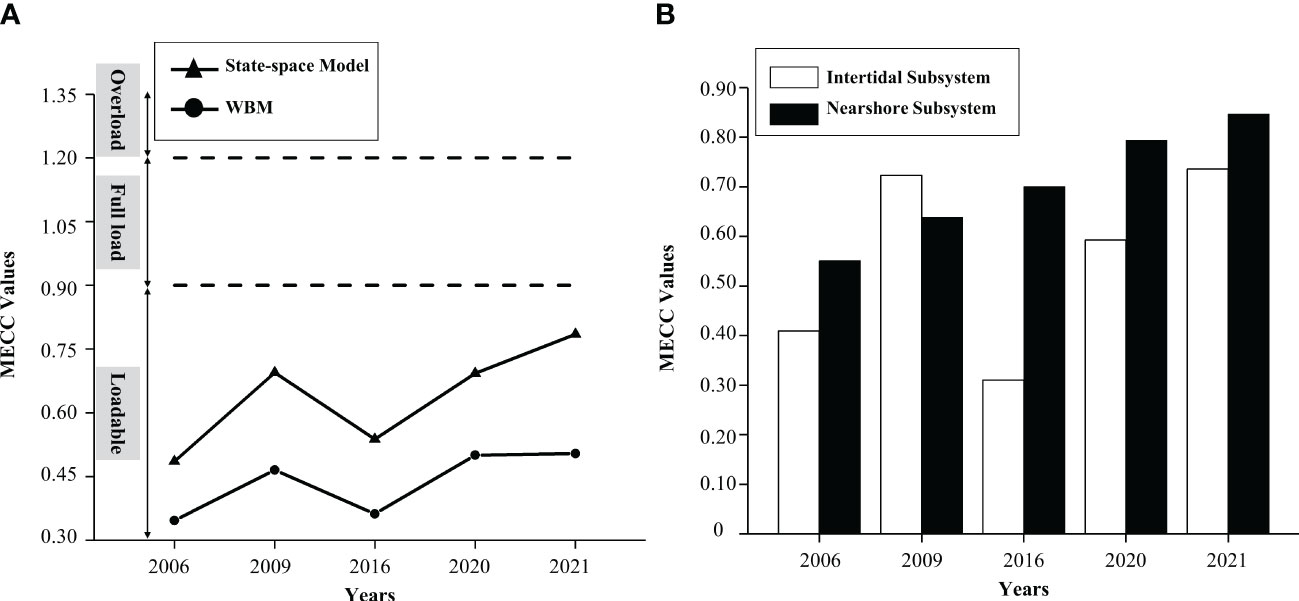
Figure 6 MECC values of the Wailingding marine ranching from 2006 to 2021. (A) Marine ranching ecosystem. (B) Marine ranching subsystem.
The MECC of the Wailingding marine ranching ecosystem remained within the safe carrying range for the period 2006–2021 (Figure 6A). It showed a steady upward trend in every year except 2016. Evaluation of the state-space model indicated that the maximum was 0.79 in 2021, indicating that MECC was close to being fully loaded. From 2006 to 2021, the MECC increased by 61.73%. Evaluation from WBM showed that the maximum was 0.50 in 2021, and the minimum was 0.35 in 2006. The increase of MECC between 2006 and 2021 was 44.34%. These showed that results calculated by WBM were lower than those obtained from the state-space model.
Based on the state-space model, the MECC of the nearshore subsystem fluctuated at a higher level and increased from 0.55 in 2006 to 0.85 in 2021. This indicated that MECC gradually moved from being loadable to full, but remained within the safe loadable range (Figure 6B). The minimum and maximum of MECC for the intertidal subsystem were 0.31 (2016) and 0.74 (2021), respectively.
4 Discussion
4.1 Marine ranching improves MECC
After the construction of marine ranching, fishery, benthic biomass, and species diversity have increased, and the MECC increased from 0.49 (pre-artificial reefs) in 2006 to 0.79 (post-artificial reefs) in 2021 (Figure 6A), indicating a slow fluctuating growth toward ideal carrying pattern. Studies had shown that after the deployment of artificial reefs, the community structure remained stable, with an even interspecific ratio (Feng et al., 2021), and the biomass and diversity indexes of phytoplankton, zooplankton, and zoobenthos more than doubled before reefing (Table 2). Indexes such as the biodiversity were at a medium-high level, and there was an increase in intertidal biomass and species diversity as well (CN-SC, 2015). This was consistent with the studies on marine ranching in Daya Bay (Liao et al., 2013) and Zhelin Bay (Ma et al., 2019). On the one hand, upwelling is generated near the artificial reefs, which carries nutrient-rich bottom water to the well-lit euphotic zone through the process of resuspension and diffusion, thereby accelerating nutrient circulation (Lin and Zhang, 2006). On the other hand, the flow field effect, feeding effect, and shelter effect produced by artificial reefs not only provided habitat, spawning, and shelter for organisms (Chen et al., 2002), but also offered rich bait for fish, shrimp, and shellfish, resulting in fish-gathering effect and enhancing the biodiversity of marine ranching ecosystem along with the complexity of food web. Moreover, Chl-a increased year by year after reefing, reaching a maximum in 2021 (Table 2). Primary producers define the extent of ecosystems, due to the presence of bottom-up energy regulation (Del Monte-Luna et al., 2004). Chl-a serves as an indicator of the current phytoplankton stock (Culver and Smith, 1989). As the initial (Yu et al., 2015) in the marine food chain, phytoplankton further affects the stock density of fishery through influencing the feeding, habitation, and reproduction of fish, shrimp, and shellfish in the sea (Yu et al., 2015). Fishing methods such as trawling are limited by the deployment of artificial reefs, thereby reducing the fishing pressure in the reef areas (Zeng et al., 2021). This increase in biomass and biodiversity enhances the stability and carrying capacity of the ecosystem in the marine ranching, as well as its ability to resist external changes and restore after damage (Broman and Robert, 2017). Seaweed farms have the potential to expand the environmental capacity of grazing areas (Han et al., 2021). Major seaweed in Wailingding marine ranching were Sargassum (Sargassum henslowianum, Sargassum hemiphyllum, and Hizikia fusiforme) and Caulerpa lentillifera. These macroalgae are able to improve the local ecological environment by absorbing inorganic salts, heavy metal, etc (Zhang et al., 2019). They can also enhance the primary productivity by converting CO2 to organic carbon in the seawater (Jiang et al., 2013; Yu et al., 2021). In addition, there are Reishia clavigera, Saccostrea echinata, Nodilittorina (N.) trochoides, and Septifer bilocularis in the Wailingding marine ranching. Shellfish has a well-developed filter-feeding system, which enhances seawater exchange and control of phytoplankton biomass, and promotes a balanced distribution of nutrients to alleviate eutrophication and improve water quality (Wang et al., 2018). Environmental capacity is the constraint of MECC, and increased resource and ecological improvement are the material foundation and support conditions (Fu et al., 2009). The improvement of marine ranching environment provides suitable habitats for organisms and reduces the impact of pollutants (Fu et al., 2009), which, in turn, improves the MECC.
It was found that MECC values of Wailingding marine ranching decreased in 2016 (Figure 6A) to 0.54, which slightly exceeded the pre-construction level value. This decline may be due to the development of tourism projects on Wailingding Island in 2009 occupied wetland resources (Li, 2017). With the increase of human flow on the island, the discharge of domestic sewage had also increased. The trumpet-shaped geographical form of Lingdingyang Estuary weakened the dilution of pollutants (Zeng et al., 2020), leading to high nutrient concentration in the waters. Increased DIN and PO43-P, reduced intertidal area, decreased the beach retention to 83%, decreased the vegetation coverage from 82% to 78% (Figure 5B), and slightly decreased the intertidal biomass and diversity index. Studies showed that 64% of the 11 marine ranching in the Bohai and Yellow Seas had very low ecosystem security status (Du and Gao, 2020). Increased discharges of pollutants due to human activities may lead to higher nutrient concentrations in the sea area and a reduction in the local MECC. In addition, artificial reefs have a certain service life (Lin and Zhang, 2006), and the physical stability of reef can affect its ecological effects. It has been more than 10 years since the first reef was deployed in 2007 in Wailingding marine ranching, and the long duration of reefing is also one of the reasons affecting the decrease in the MECC in 2016. Marine ranching ecosystems have the ability of self-regulation (Wang et al., 2010). Although the deployment of artificial reefs breaks the original ecological environment of the Wailingding water, a new ecosystem balance will be established after the buffer of ecosystem’s self-regulation ability. This is why after the second reefing in 2020, MECC of Wailingding marine ranching improved from 2016, rising to 0.79 in 2021, close to ideal full capacity.
4.2 Resource and environment factors affecting MECC of marine ranching
Intertidal ecosystem and nearshore ecosystem have close material and energy exchanges, and they interact with each other. Reclamation projects or nearshore pollutants can bring substantial changes in hydrodynamics, estuarine circulation, and solute transport, which can have considerable environmental impacts on estuarine ecosystems (Yang et al., 2018b; Hu et al., 2021). Studies on the MECC of marine ranching ecosystems cannot be limited to nearshore ecosystems and need to incorporate the impacts of intertidal subsystems. Studies have shown that intertidal subsystems play an important role in the MECC of marine ranching, in which resource and environmental factors are the major influencing factors. The MECC of the nearshore subsystem has been far higher than that of the intertidal subsystem, except in 2009 (Figure 6B). However, in the MECC evaluation index system, the weight of the intertidal subsystem accounted for 52.51% (Table 3), which had the greatest impact on the MECC of the Wailingding marine ranching. The intertidal subsystem is in the sensitive transitional region of ocean–land atmosphere interaction, rich in biomass and biodiversity (Li et al., 2023a). It provides habitat and food resources for marine organisms and also plays an important role in the stabilization and regulation of the marine environment. The complexity of the geographic location makes the ecology of the region very sensitive and fragile (Wang et al., 2015).
The construction marine ranching has an effect on increase of benthic biomass (Wang et al., 2018) and conservation of intertidal resources. Intertidal biomass reached its maximum in 2021 (Table 2). Studies have shown that the geographic location of island and their habitats affect the species composition of intertidal communities, and salinity and hydrodynamic changes affect the distribution of communities, of which changes in flushing and siltation directly affect the intertidal benthic biodiversity and distribution characteristics (Zhu and Lu, 2003). Factors affecting the hydrodynamic processes of the intertidal area include tidal currents and waves, and the deployment of reefs changes regional tidal wave and tidal patterns, bringing about erosion and resuspension of the sedimentary substrate (Lin and Zhang, 2006). In this study, the intertidal subsystems with higher weights were vegetation coverage (D4) with a weight of 19.18%, and beach retention (D2) with a weight of 10.66% (Table 3), indicating that resource and environmental factors are the major factors limiting MECC of the intertidal subsystems. Resources and environmental conditions play a decisive role in the stability of ecosystems and the diversity and distribution of fish and other biological groups in the region (Lv et al., 2014), affecting the regional ecological carrying capacity (Del Monte-Luna et al., 2004). They provide habitats and foods, purify water, and maintain ecosystem stability.
4.3 Selection of MECC methods for marine ranching
Considering the uniqueness of marine ranching ecosystems, it is a challenge to assess the MECC. Although previous studies have provided a variety of referable methods (Chapman et al., 2013; Di et al., 2014; Wang et al., 2017; Du and Gao, 2020; Du et al., 2020; Wang et al., 2020; Borja et al., 2022; Bui and Tran, 2022; Ying et al., 2022; Suo et al., 2023), the rationality and practicality of their applications in marine ranching still need to be further explored and improved.
The most popular conceptual model for quantitative evaluation of MECC is the PSR model, from which the evaluation system based on the concept of MECC is evolved (Wu et al., 2022a). However, the causal relationship between indexes in this model is not accurate enough. Compared with this, the establishment of the evaluation index system from the concept can provide a clearer idea for the classification of the assessment indexes. Studies have shown that the assessment of carrying capacity of fishery is based on the theory of the food chain/food web and energy flow (Suo et al., 2023). As a small-scale energy system, marine ranching does not have standardized and comprehensive access to energy flow data, as is the case for global or national systems, and there are uncertainty of sources, which reduces the credibility of the calculations (Du et al., 2022). The study of ocean carrying capacity should not be limited to a single carrying capacity, but should also include the coastal zone and surrounding waters, seabed and bottom, etc. Its application to relatively closed marine ecosystems requires a clearer understanding of the ability of the water to allow pollutants to enter without causing long-term deterioration or pollution. The MECC of small-scale areas is the carrying capacity under dominant function determination and needs to take into account physical processes (such as dilution, dispersion, sedimentation, and evaporation) as well as all chemical and biological processes. Therefore, in this study, temperature, salinity, depth, current velocity, and other hydrodynamic factors were added to construct the model to make the evaluation system more complete. AHP and the entropy method are the combination of expert experience and objective data, eliminating subjectivity and ambiguity in the evaluation process (Wu et al., 2022a). Combing through the multiplicative integration (Equation 7) makes the results of weight allocation more objective, and the influence of each index can be revealed as well.
The state-space model solved the difficulties in determining the ideal index value of MECC, based on the evaluation criteria of the conservation effect of marine ranching. Survey data are processed according to national standards. Remote sensing data extraction information had been used in many ecological studies (Su et al., 2018; Pan et al., 2020; Zhu et al., 2022) and is consistent with survey data. These data conformed to the spatial scale and time frame required for our research. Therefore, the data were reliable, and methods can reduce the systematic error in the evaluation process (Yu and Du, 2023). The evaluation system in this study consists of 24 indexes in four levels. WBM has excellent performance in processing multi-criteria decision-making, where the optimal solution is selected from multiple schemes, or synthesizing the performance of each scheme under multi-criteria to obtain its ranking (Xia et al., 2012). Therefore, introducing WBM in the evaluation of MECC can deal with the situation where there is a link between the criteria in multi-criteria decision making. In this study, the two methods show a similar trend of change, with an overall upward trend in MECC scoring value from 2006 to 2021, and a significant decline in 2016 (Figure 6A). It can be considered that both methods are feasible. The difference is that the calculation results of WBM are significantly lower than those of the state-space model, and the variation of the state-space model is 61.73%, while the change in the WBM is 44.34%, which is relatively gentle compared to the state-space model. That is, results of WBM showed that MECC of Wailingding marine ranching was at a low level, and there was little change in the MECC after and before the deployment of artificial reefs. This was partly associated with the iterative operation of WBM that weakened the correlation between the indexes (Yang et al., 2020). The MECC of the marine ranching ecosystem is a relatively single in terms of the carrying objects, which leads to the possibility of strong correlations between various indexes. Furthermore, MECC is mainly influenced by resources and environmental factors (Woodworth-Jefcoats et al., 2017), and biomass is considered a dynamic component of MECC (Del Monte-Luna et al., 2004). The key resource elements in this study, such as beach retention rate and artificial reef area, have increased significantly compared to the pre-reefing period (Table 2). Environmental factors such as temperature, salinity, and current velocity remain relatively constant over the same period of time. As biomass and Chl-a increased, it is reasonable to predict that the MECC should be at a higher level, and there is a significant change from the initial state. Results of the state-space model were relatively high, which was a significant improvement and was closer to the actual situation than that of the WBM. Therefore, the state-space model is more optimal and suitable for Wailingding marine ranching.
5 Conclusion
An evaluation index system from three levels was constructed, based on high-resolution satellite remote sensing and survey data. Two methods of the state-space model and WBM were used to comprehensively evaluate the MECC of Wailingding marine ranching. Results provide new ideas for the construction of modern marine ranching. Firstly, attention needs to be paid to the protection and restoration of the intertidal areas near the marine ranching. Measures such as designation of protected areas and restrictions on fishing can be taken to increase investment in resource protection. The health and integrity of intertidal resources can be protected and the MECC of the intertidal system can be enhanced through planting mangroves, Tamarix chinensis Lour, Suaeda glauca (Bunge) Bunge, etc. Secondly, the management of pollutant discharge should be strengthened and the scale of coastal reclamation should be controlled. A healthy ecosystem is a prerequisite for enhancing MECC. Thirdly, a digital platform that can monitor the ecological environment and fishery variations of marine ranching should be built. Early warning of ecological safety and environmental security, and big data processing and analysis for resources and environments should be considered as well. An effective assessment of marine ranching MECC provides data references for determining appropriate scale, establishing reasonable fishery quotas and protective measures, and implementing risk management in the construction of modern marine ranching (Wang and Du, 2023). The research has expanded the application of high-resolution satellite remote sensing in nearshore waters, and enriched marine ranching ecological carrying capacity assessment.
Data availability statement
The original contributions presented in the study are included in the article/supplementary material. Further inquiries can be directed to the corresponding author.
Author contributions
ZW: Writing – original draft, Writing – review & editing. LY: Writing – review & editing. JY: Writing – review & editing. PC: Writing – review & editing. ZL: Writing – review & editing. WY: Writing – review & editing.
Funding
The author(s) declare financial support was received for the research, authorship, and/or publication of this article. This study was supported by the following funds: (1) Southern Marine Science and Engineering Guangdong Laboratory (Zhuhai) (SML2023SP237); (2) R & D Projects in Key Areas of Guangdong Province, China (2020B1111030002); (3) Central Public-Interest Scientific Institution Basal Research Fund, CAFS, China (2018HY-ZD0104, 2023TD06, 2021SD02).
Conflict of interest
The authors declare that the research was conducted in the absence of any commercial or financial relationships that could be construed as a potential conflict of interest.
Publisher’s note
All claims expressed in this article are solely those of the authors and do not necessarily represent those of their affiliated organizations, or those of the publisher, the editors and the reviewers. Any product that may be evaluated in this article, or claim that may be made by its manufacturer, is not guaranteed or endorsed by the publisher.
References
Borja A., Pouso S., Galparsoro I., Manca E., Vasquez M., Lu W., et al. (2022). Applying the China’s marine resource-environment carrying capacity and spatial development suitability approach to the Bay of Biscay (North-East Atlantic). Front. Mar. Sci. 9. doi: 10.3389/fmars.2022.972448
Broman G. I., Robert K. H. (2017). A framework for strategic sustainable development. J. Cleaner Production 140, 17–31. doi: 10.1016/j.jclepro.2015.10.121
Bui L. T., Tran D. L. T. (2022). Assessing marine environmental carrying capacity in semi-enclosed coastal areas - Models and related databases. Sci. Total Environ. 838, 156043. doi: 10.1016/j.scitotenv.2022.156043
Carneiro R. L. (1960). “Slash-and-burn Agriculture: A Closer Look at its Implications for Settlement Patterns,” in Men and Cultures. (Philadelphia: University of Pennsylvania Press), 229–234.
Chapman M. G., Kelble C. R., Loomis D. K., Lovelace S., Nuttle W. K., Ortner P. B., et al. (2013). The EBM-DPSER conceptual model: integrating ecosystem services into the DPSIR framework. PloS One 8. doi: 10.1371/journal.pone.0070766
Chen Y. (2020). Research and construction of modern marine ranching in China: a review. J. OF DALIAN OCEAN Univ. 35, 147–154. doi: 10.16535/j.cnki.dlhyxb.2019-260
Chen J., Chen Y.-H., He C.-Y., Shi P.-J. (2001). Sub-pixel model for vegetation fraction estimation based on land cover classification. J. OF Remote SENSING-BEIJING- 5, 416–422. doi: 10.3321/j.issn:1007-4619.2001.06.003
Chen X., Gao F., Guan W., Lei L., Wang J. (2013). Review of fishery forecasting technology and its models. J. Fisheries China 37, 1270–1280. doi: 10.3724/SP.J.1231.2013.38313
Chen Y., Yu C., Zhang G., Zhang S. (2002). The environmental function and fish gather effect of artificial reefs. J. Dalian Fisheries College/Dalian Shuichan Xueyuan Xuebao. Dalian 17, 64–69. doi: 10.1007/s11769-002-0026-8
CN-SC (2015). Technical specification for evaluation of the effects of artificial fish reef (Beijing, China: The ministry of agriculture of the People's Republic of China).
Culver M. E., Smith W. O. (1989). Effects of environmental variation on sinking rates of marine phytoplankton1. J. Phycology. 25 (2), 262–270. doi: 10.1111/j.1529-8817.1989.tb00122.x
Del Monte-Luna P., Brook B. W., Zetina-Rejón M. J., Cruz-Escalona V. H. (2004). The carrying capacity of ecosystems. Global Ecol. Biogeography 13, 485–495. doi: 10.1111/j.1466-822X.2004.00131.x
Di Q., Zhang J., Wu J. (2014). Assessment of marine ecological carrying capacity in Liaoning province based on the ecosystem health. J. Nat. Resour 29, 256–264. doi: 10.11849/zrzyxb.2014.02.007
Du Y.-W., Gao K. (2020). Ecological security evaluation of marine ranching with AHP-entropy-based TOPSIS: A case study of Yantai, China. Mar. Policy 122. doi: 10.1016/j.marpol.2020.104223
Du Y.-W., Li B.-Y., Quan X.-J. (2020). Construction and application of DPPD model for evaluating marine resources and environment carrying capacity in China. J. Cleaner Production 252. doi: 10.1016/j.jclepro.2019.119655
Du Y.-W., Wang Y.-C., Li W.-S. (2022). Emergy ecological footprint method considering uncertainty and its application in evaluating marine ranching resources and environmental carrying capacity. J. Cleaner Production 336. doi: 10.1016/j.jclepro.2022.130363
Feng X., Dai X., Fan J., San X. (2023). Seasonal variation of fishery resources in Wailingding marine ranching and adjacent waters. South China Fisheries Sci. 19, 32–38. doi: 10.12131/20220308
Feng X., Fan J., San X. (2021). The stock enhancement effect evaluation of artificial reef in Wailingding, Zhuhai. J. South. Agric. 52, 3228–3236. doi: 10.3969/j.issn.2095-1191.2021.12.005
Fu H., Liu X., Sun Y. (2009). Assessment on wetland ecosystem health in Daguhe Estuary. Mar. Environ. Sci. 28 (3), 329–332. doi: 10.3969/j.issn.1007-6336.2009.03.024
Han T., Shi R., Qi Z., Huang H., Gong X. (2021). Impacts of large-scale aquaculture activities on the seawater carbonate system and air-sea CO2 flux in a subtropical mariculture bay, southern China. Aquaculture Environ. Interact. 13, 199–210. doi: 10.3354/aei00400
Hu R., Yao L., Yu J., Chen P., Wang D. (2021). Remote sensing of the coastline variation of the guangdong–hongkong–macao greater bay area in the past four decades. J. Mar. Sci. Eng. 9. doi: 10.3390/jmse9121318
Huamantinco Cisneros M. A., Revollo Sarmiento N. V., Delrieux C. A., Piccolo M. C., Perillo G. M. E. (2016). Beach carrying capacity assessment through image processing tools for coastal management. Ocean Coast. Manage. 130, 138–147. doi: 10.1016/j.ocecoaman.2016.06.010
Jiang Z., Fang J., Mao Y., Han T., Wang G. (2013). Influence of seaweed aquaculture on marine inorganic carbon dynamics and sea-air CO2 flux. J. World Aquaculture Soc. 44, 133–140. doi: 10.1111/jwas.12000
Li J. (2017). Study on sustainable development of Zhuhai Wailingding island tourism. XI AN: Southwest Jiaotong University.
Li B. X., Li A. X., Dong J. Y., Lv S. L., Wang X. F. (2023a). Intertidal macrozoobenthic community structure and its disturbed state in Zhanjiang Bay. South China Fisheries Sci. 19, 12–20. doi: 10.12131/20220199
Li Y., Sun L., Wu Z., Liu H. (2023b). Evaluation and obstacle factors of marine resources and environment carrying capacity in Beibu Gulf Urban Agglomeration. Front. Mar. Sci. 10. doi: 10.3389/fmars.2023.1196196
Liao X., Chen P., Ma S., Chen H. (2013). Community structure of phytoplankton and its relationship with environmental factors before and after construction of artificial reefs in Yangmeikeng, Daya Bay. South China Fisheries Sci. 9, 109–119. doi: 10.3969/j.issn.2095-0780.2013.05.017
Lin J., Zhang S. (2006). Research advances on physical stability and ecological effects of artificial reef. Mar. fisheries/Haiyang Yuye 28, 257–262. doi: 10.3969/j.issn.1004-2490.2006.03.015
Long X., Chen P., Yuan H., Feng X., Yu J., Shu L., et al. (2019). Evaluation on carrying capacity of fishery resources in coastal waters of Dapeng Peninsula, Shenzhen. South China Fisheries Sci. 15, 56–64. doi: 10.12131/20190024
Lv W., Ma C. A., Huang Y., Yang Y., Yu J., Zhang M., et al. (2014). Macrobenthic diversity in protected, disturbed, and newly formed intertidal wetlands of a subtropical estuary in China. Mar. pollut. Bull. 89, 259–266. doi: 10.1016/j.marpolbul.2014.09.051
Ma H., Qin C., Chen P., Lin H., Duan D. (2019). Valuation of ecosystem service of marine ranching in zhelin bay. South China Fisheries Sci. 15, 10–19. doi: 10.12131/20180041
Mao H., Yu D. (2001). A study on the quantitative research of regional carrying capacity. Adv. Earth Sci. 16, 549–555. doi: 10.11867/j.issn.1001-8166.2001.04.0549
Nam J., Chang W., Kang D. (2010). Carrying capacity of an uninhabited island off the southwestern coast of Korea. Ecol. Model. 221, 2102–2107. doi: 10.1016/j.ecolmodel.2010.05.016
Pan J.-h., Wei W., Yang X.-r., Yang L.-j. (2020). Spatio-temporal evolution and influencing factors of water resource carrying capacity in Shiyang River Basin: based on the geographical detector method. Water Supply 20, 1409–1424. doi: 10.2166/ws.2020.057
Saaty T. L., Kearns K. P. (1985). The analytic hierarchy process. Analytical Planning 19–62. doi: 10.1016/B978-0-08-032599-6.50008-8
Song Y. (2006). Health assessment methods and application of island ecosystem (Ocean University of China: Doctor's thesis).
Su X., Suo A., Song D. (2018). Ecological carrying capacity assessment of tidal flat in the Yangtze River Economic Belt by remote sensing. Mar. Environ. Sci. 37, 528–536. doi: 10.13634/j.cnki.mes.2018.04.009
Suo A., Li H., Zhou W., Jiao M., Zhang L., Yue W. (2023). Estimation of ecological carrying capacity of small-scale fish in marine ranch of the Pearl River Estuary, China. Regional Stud. Mar. Sci. 61. doi: 10.1016/j.rsma.2023.102901
Theodora Y., Spanogianni E. (2022). Assessing coastal urban sprawl in the Athens' southern waterfront for reaching sustainability and resilience objectives. Ocean Coast. Manage. 222, 106090. doi: 10.1016/j.ocecoaman.2022.106090
Vasconcellos M., Gasalla M. A. (2001). Fisheries catches and the carrying capacity of marine ecosystems in southern Brazil. Fisheries Res. 50, 279–295. doi: 10.1016/S0165-7836(00)00217-4
Wang Z. G. (1999). Quantitative analysis on bearing capacity of ecological environment. J. Changjiang Institute Technol. 04, 9–12. doi: CNKI:SUN:CJZG.0.1999-04-003
Wang K. Y. (2007). System and application of ecological carrying capacity composite model (BeiJing: Science Press).
Wang X. L., Chu J. L. (2005). Extraction of remotely sensed information of island intertidal zone and wetland——Taking the Dongsha island as an example. Adv. Mar. Sci. 23, 477–481. doi: 10.3969/j.issn.1671-6647.2005.04.013
Wang Y. C., Du Y. W. (2023). Evaluation of resources and environmental carrying capacity of marine ranching in China: An integrated life cycle assessment-emergy analysis. Sci. Total Environ. 856, 159102. doi: 10.1016/j.scitotenv.2022.159102
Wang J., Huang Y., Huang X. (2015). Ecological risk assessment of heavy metals in surface sediments in Yancheng coastal mudflat. Mar. Sci. Bull. 34, 353–360. doi: 10.11840/j.issn.1001-6392.2015.03.016
Wang W.-D., Liang J., Zhang S.-Y. (2010). Influence of artificial reef construction on nutrition and water quality in off-shore area of Shengsi, Zhejiang. Acta Hydrobiologica Sin. 34, 78–87. doi: 10.3724/SP.J.1035.2010.00078
Wang S.-H., Wang Y.-C., Song M.-L. (2017). Construction and analogue simulation of TERE model for measuring marine bearing capacity in Qingdao. J. Cleaner Production 167, 1303–1313. doi: 10.1016/j.jclepro.2017.05.185
Wang Y., Yu J., Chen P. (2018). Remote sensing assessment of ecological effects of marine ranching in the eastern Guangdong waters, China. J. Geosci. Environ. Prot. 06, 101–113. doi: 10.4236/gep.2018.612007
Wang Y.-N., Zhou Q., Wang H.-W. (2020). Assessing ecological carrying capacity in the Guangdong-Hong Kong-Macao greater bay area based on a three-dimensional ecological footprint model. Sustainability 12. doi: 10.3390/su12229705
Wilhm J. L. (1968). Use of biomass units in Shannon's formula. Ecology 49, 153–156. doi: 10.2307/1933573
Woodworth-Jefcoats P. A., Polovina J. J., Drazen J. C. (2017). Climate change is projected to reduce carrying capacity and redistribute species richness in North Pacific pelagic marine ecosystems. Glob Chang Biol. 23, 1000–1008. doi: 10.1111/gcb.13471
Wu K., Chen K., Gao Y., Jiang S., Huang H. (2022a). Applying a set of potential methods for the integrated assessment of the marine eco-environmental carrying capacity in coastal areas. Sustainability 14. doi: 10.3390/su14084416
Wu P., Liu Y., Xiao Y., Xie Y., Tang G., Lin L., et al. (2022b). Evaluation of fisheries ecological environment in adjacent sea areas of Wanshan Archipelago in Pearl River Estuary in spring. South China Fisheries Sci. 18, 1–8. doi: 10.12131/20210332
Xia M., Xu Z., Zhu B. (2012). Generalized intuitionistic fuzzy Bonferroni means. Int. J. Intelligent Syst. 27, 23–47. doi: 10.1002/int.20515
Xie X., Chen P., Tong F., Yuan H., Feng X., Yu J., et al. (2022). Site selection of marine ranching in Wailingding Island sea area of Zhuhai. South China Fisheries Sci. 18, 18–29. doi: 10.12131/20210241
Yang Y., Chui T. F. M., Shen P. P., Yang Y., Gu J. D. (2018b). Modeling the temporal dynamics of intertidal benthic infauna biomass with environmental factors: Impact assessment of land reclamation. Sci. Total Environ. 618, 439–450. doi: 10.1016/j.scitotenv.2017.10.325
Yang Z., Li X., Garg H., Qi M. (2020). Decision Support Algorithm for Selecting an Antivirus Mask over COVID-19 Pandemic under Spherical Normal Fuzzy Environment. Int. J. Environ. Res. Public Health 17. doi: 10.3390/ijerph17103407
Yang H., Yang X., Lin C., Zhang L., Qiang X. U. (2018a). Strive to realize modernization of concept, equipment, technology, and management of modern marince ranching development. Bull. Chin. Acad. Sci. 33 (7), 732–738. doi: 10.16418/j.issn.1000-3045.2018.07.010
Yang H., Zhang S., Zhang X., Chen P., Tian T., Zhang T. (2019). Strategic thinking on the construction of modern marine ranching in China. J. Fisheries China 43, 1255–1262. doi: 10.11964/jfc.20190211670
Ying C., Yang Y., Liu Y. (2022). Analysis of the marine resource environmental carrying capacity in Zhejiang Province based on the Pressure-Support and Destructiveness-Resilience and Degradation-Promotion (PS-DR-DP) model. Front. Mar. Sci. 9. doi: 10.3389/fmars.2022.1023769
Yu J., Chen P., Tang D., Qin C. (2015). Ecological effects of artificial reefs in Daya Bay of China observed from satellite and in situ measurements. Adv. Space Res. 55, 2315–2324. doi: 10.1016/j.asr.2015.02.001
Yu L., Du W. (2023). Research on the systematic error generation mechanism of TOPSIS method in academic evaluation. Inf. Studies:Theroy Appl. 46 (12), 1–13. doi: 10.16353/j.cnki.1000-7490.2023.12.006
Yu J., Mao J., Wang D. (2021). Remote sensing assessment of marine primary productivity and fishery resources in the Daya bay, China. Pakistan J. Zoology 53. doi: 10.17582/journal.pjz/20200325060334
Yucel-Gier G., Eronat C., Sayin E. (2019). The impact of marine aquaculture on the environment; the importance of site selection and carrying capacity. Agric. Sci. 10, 259–266. doi: 10.4236/as.2019.103022
Yue L., Xu K., Gunglei S. (2020). Property analysis and method selection of the indexes being dimensionless. Stat Inf. Forum 35, 3–9. doi: 10.3969/j.issn.1007-3116.2020.06.001
Zeng D., Niu L., Tao W., Fu L., Yang Q. (2020). Nutrient dynamics in Pearl River Estuary and their eutrophication evaluation. J. Guangdong Ocean Univers 40, 73–82. doi: 10.3969/j.issn.1673-9159.2020.03.010
Zeng L., Tang Z., Chen P., Yu J., Chen G. (2021). Optimization of fishery resources assessment methods and ecological effects evaluation of artificial reefs. Mar. Biol. Res. 17, 72–85. doi: 10.1080/17451000.2021.1887497
Zhang P., Li Z., Song H., Cai H., Xia F. (2023). AHP-based evaluation index system on site selection for offshore cage culture. South China Fisheries Sci. 19, 1–9. doi: 10.12131/20220253
Zhang S., Liu S., Zhou X., Wang Z., Wang K. (2019). Ecological function of seaweed-formed habitat and discussion of its application to sea ranching. J. Fisheries China 43, 2004–2014. doi: 10.11964/jfc.20190711873
Zhao M., Yu J., Chen P., Feng X. (2016). Ecosystem health assessment of the Egong Bay fisheries area in Shenzhen. J. South. Agric. 47, 1025–1031. doi: 10.3969/j:issn.2095-1191.2016.06.1025
Zhu X.-j., Lu J.-j. (2003). Functional groups of zoobenthos in the intertidal zone of Jiuduansha, the Yangtze River Estuary. Zoological Res. 24, 355–361.doi: 10.3321/j.issn:0254-5853.2003.05.006
Zhu Z., Mei Z., Li S., Ren G., Feng Y. (2022). Evaluation of ecological carrying capacity and identification of its influencing factors based on remote sensing and geographic information system: A case study of the yellow river basin in Shaanxi. Land 11. doi: 10.3390/land11071080
Keywords: marine ecological carrying capacity, marine ranching, high-resolution remote sensing, Wailingding, three-dimensional state-space model, weighted Bonferroni mean
Citation: Wang Z, Yao L, Yu J, Chen P, Li Z and Yang W (2024) Evaluation of the ecological carrying capacity of Wailingding marine ranching in Zhuhai, China by high-resolution remote sensing. Front. Mar. Sci. 11:1354407. doi: 10.3389/fmars.2024.1354407
Received: 12 December 2023; Accepted: 25 January 2024;
Published: 20 February 2024.
Edited by:
Lina Cai, Zhejiang Ocean University, ChinaReviewed by:
Xuefeng Wang, Guangdong Ocean University, ChinaFei Wang, Chinese Academy of Fishery Sciences, China
Copyright © 2024 Wang, Yao, Yu, Chen, Li and Yang. This is an open-access article distributed under the terms of the Creative Commons Attribution License (CC BY). The use, distribution or reproduction in other forums is permitted, provided the original author(s) and the copyright owner(s) are credited and that the original publication in this journal is cited, in accordance with accepted academic practice. No use, distribution or reproduction is permitted which does not comply with these terms.
*Correspondence: Jing Yu, eXVqaW5nQHNjc2ZyaS5hYy5jbg==