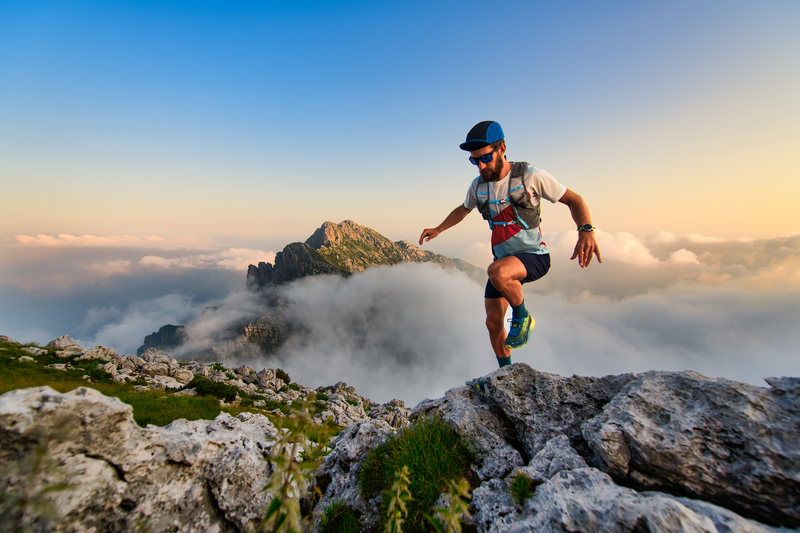
95% of researchers rate our articles as excellent or good
Learn more about the work of our research integrity team to safeguard the quality of each article we publish.
Find out more
ORIGINAL RESEARCH article
Front. Mar. Sci. , 28 October 2024
Sec. Marine Molecular Biology and Ecology
Volume 11 - 2024 | https://doi.org/10.3389/fmars.2024.1351148
Accurately investigating the composition of zooplankton species is crucial for monitoring changes in marine ecosystems and assessing biodiversity. In this study, we utilized bulk DNA and environmental DNA metabarcoding in the Ulleung Basin, known for its high zooplankton species diversity among the seas surrounding the Korean Peninsula. Genomic DNA extracted from samples collected during three summer seasons in the survey area was analyzed using high-throughput sequencing of the cytochrome c oxidase I barcode region. We identified 350 species, which were three to six times more than those identified by traditional morphological methods. Furthermore, we observed significant differences in species composition and diversity between bulk DNA and eDNA samples. Notably, eDNA metabarcoding effectively detected species with high swimming ability and those that were difficult to capture using traditional sampling methods. This study underscores the significant impact of sampling methods on research outcomes in zooplankton species diversity studies and highlights the importance of integrating different sampling techniques. Specifically, it suggests the need for the active adoption of non-invasive methods, such as eDNA metabarcoding, for the comprehensive monitoring of diverse biological groups in marine ecosystems.
Zooplankton species diversity is a key indicator of changes in marine ecosystems (Sabatés et al., 1989; Beaugrand et al., 2002; Volis et al., 2016). Zooplankton respond sensitively to environmental changes and are utilized as bioindicators to identify shifts in the marine environment (Meybeck, 2003). Specifically, monitoring the composition of zooplankton species is a valuable means of understanding the responses of marine ecosystems and identifying their diversity (Hoegh-Guldberg and Bruno, 2010; Constable et al., 2014). Furthermore, zooplankton are crucial for carbon and nitrogen cycling and facilitate energy transfer in marine ecosystems (Gismervik, 2006; Hirai et al., 2020). Accordingly, zooplankton play a pivotal role in shaping the food chain and supporting fisheries in marine ecosystems (Frederiksen et al., 2006).
In the study of zooplankton species diversity, the traditional criteria for species identification are morphological traits (Chihara and Murano, 1997; Boxshall and Halsey, 2004; Moon et al., 2022). This species classification method is time-consuming, and accurate identification requires taxonomists with extensive training (Machida et al., 2009). Moreover, accurate identification is challenging when the morphological characteristics used for species identification are similar or ambiguous across taxonomic groups (Heimeier et al., 2010).
To overcome the limitations of morphology-based species identification, DNA barcode analysis has gained increasing attention (Hebert et al., 2003; Ward et al., 2005; Valentini et al., 2009). When reporting previously unrecorded species in local areas, providing both morphological traits and DNA barcode information is becoming a standard practice. From a taxonomic perspective, although DNA barcode analysis is suitable for the accurate classification of species in individual samples, this method is not well suited for species identification in ecosystem research, which requires the rapid analysis of large-scale samples. DNA metabarcoding, which enables the analysis of mixed samples containing multiple species, is emerging as a solution to the challenges posed by single-sample DNA barcode analysis (Deiner et al., 2017). This method identifies various species by comparing a comprehensive collection of DNA barcode sequences, which serve as the criteria for species identification, with DNA barcode regions from mixed samples (Schloss et al., 2009; Bolyen et al., 2019). DNA metabarcoding facilitates the identification of invertebrate species in physically damaged samples and in the early life stages, which are difficult to identify based on morphological traits (Song et al., 2021). In particular, this method accurately identifies cryptic and sibling species with indistinct phenotypes and is effective in revealing “hidden diversity” (Lindeque et al., 2013; Bucklin et al., 2016).
Zooplankton exhibit significant differences in habitat, swimming speed, and size among taxonomic groups. These ecological characteristics vary depending on the growth stage. Their species diversity also varies depending on the method of sample collection and species identification. To overcome the challenges in species identification, DNA metabarcoding has been introduced to analyze zooplankton species diversity (Suter et al., 2021). Most samples used in DNA metabarcoding are bulk DNA samples collected from zooplankton using plankton nets. However, the composition of zooplankton species varies depending on the mesh size of the nets (Tranter and Heron, 1965; Gannon, 1980; Mack et al., 2012). Furthermore, investigating the benthic invertebrates (e.g., polychaetes) that inhabit the seafloor with plankton nets is challenging due to their habitat characteristics (Djurhuus et al., 2018). The use of plankton nets involves considerable cost and time, with various types of nets used depending on the research objectives (He et al., 2023). When benthic organisms are collected using bottom trawl nets, habitat destruction and disturbance can occur (Pusceddu et al., 2014).
Environmental DNA (eDNA) refers to the genetic material contained in organic matter (feces, saliva, urine, skin cells, etc.) released by organisms into various environments (seawater, soil, sediment, snow, etc.) and is derived from mitochondrial or nuclear DNA (Lacoursière-Roussel et al., 2016; Hervé et al., 2022). eDNA is effective for detecting species that are not collected by nets because of certain factors, such as organism size and swimming ability, without bias (Bohmann et al., 2014; Djurhuus et al., 2018; Mariani et al., 2021). Moreover, eDNA metabarcoding is a non-invasive method that is effective for assessing biodiversity in marine ecosystems (Suter et al., 2021; Spear et al., 2021; Keck et al., 2022). Therefore, eDNA metabarcoding has the potential to overcome the various disadvantages of traditional net sampling-based approaches (He et al., 2023).
In this study, we investigated zooplankton species diversity through DNA metabarcoding analysis of bulk DNA and eDNA samples collected from the Ulleung Basin, located in the southeastern waters of the Korean Peninsula. This region is known for its high zooplankton species diversity among the seas surrounding the Korean Peninsula and is influenced by the Tsushima Warm Current and the North Korea Cold Current (Lee et al., 2017; Shin et al., 2017). Previous studies have collected zooplankton using nets and morphology-based species identification methods (Park and Choi, 1997; Park et al., 1998; Kang et al., 2002; Rho et al., 2010). However, this sampling method can introduce bias toward certain species and is likely to underestimate species diversity. Therefore, in this study, in addition to bulk DNA from zooplankton net samples, we attempted a more accurate and comprehensive classification of zooplankton at the species level by utilizing the DNA metabarcoding of eDNA collected by filtering seawater.
The samples for this study were of two types, bulk DNA and eDNA, collected from the Ulleung Basin, located in the southeastern waters of the Korean Peninsula, during summer. Sampling was performed three times on board the Onnuri R/V from August 7 to 9, 2019, the Isabu R/V from June 26 to 28, 2020, and the Eardo R/V from July 20 to 21, 2021. Bulk DNA samples were obtained by vertical towing using a zooplankton net (with a mouth diameter of 60 cm and a mesh size of 200 μm). The towing depths of the zooplankton nets ranged from a minimum of 140 m to a maximum of 600 m, based on the depth of the sampling stations (Supplementary Table S1). The collected samples were preserved in the field using 95% ethanol. The sample volume comprised less than 10% of the total sample bottle. In total, 17 bulk DNA samples were collected. During the sampling period, six samples were collected in August 2019, six in June 2020, and five in July 2021 (Figure 1 and Supplementary Table S1). eDNA samples were collected at the same time and station as those of bulk DNA samples. A total of 71 eDNA samples were collected: 12 in August 2019, 33 in June 2020, and 26 in July 2021. eDNA specimens were collected from two to six depth strata at each station using a rosette sampler (Sea-Bird 911, Sea-Bird Scientific, Bellevue, WA, USA), considering the station depth (Supplementary Table S1). To prevent contamination between samples, the bottles were washed with a 4% commercial bleach solution before sampling. For each stratum, 4 L of seawater was sampled, transferred to a 4-L bottle, and then filtered through 47 mm diameter mixed cellulose ester filters (pore size of 0.45 μm). The filters were stored at −80°C, and genomic DNA (gDNA) was extracted immediately after transport to the laboratory. Temperature and salinity at the sampling sites were measured using a CTD (Sea-Bird 911, Sea-Bird Scientific, Bellevue, WA, USA).
Figure 1. Sampling stations in the Ulleung Basin of the Southeastern Korean Peninsula during the summer. Sampling periods were as follows: August 2019 - E1, E4, E5, E6, S1, and S6; June 2020 - E1, E3, E5, S1, S5, and S6; July 2021 - E1, E3, E5, S1, and S6.
A TIANamp Marine Animals DNA Kit (Tiangen Biotech, China) was used to extract DNA from both the bulk DNA and eDNA samples. To enhance the detection efficiency of various taxa, DNA was extracted from each bulk DNA sample four times with separate pre-treatments. The pre-treatment involved centrifuging approximately 10 ml of the sample in a 50 ml tube at 2000 × g for 10 min to remove the supernatant. The remaining sample was then mixed with 20 ml of phosphate-buffered saline, vortexed for 30 s, and centrifuged again at 2000 × g for 10 min to eliminate residual ethanol. The samples were subsequently homogenized using stainless steel beads with diameters of 5.0 mm, and homogenization was performed for 3 minutes at a frequency of 30 (Frequency 1/s) using the TissueLyser II (Qiagen, Germany). Following the protocol of the manufacturer, 400 μl of gDNA (100 μl per extraction) was eluted with distilled water and stored at −20°C.
For the extraction of eDNA, half of the filter was used, while the other half was preserved at −80°C. The sample, subjected to homogenization similar to that for the bulk-DNA samples, was incubated at 56°C for 3 h in GA buffer (600 μl) and proteinase K (60 μl). After incubation, the samples were centrifuged at 2000 × g for 10 min to separate the solution from the filter. The supernatant was then divided into two 1.5 ml tubes for DNA extraction. Finally, 100 μl of gDNA (50 μl per extraction) was eluted from each eDNA sample using distilled water and stored at −20°C.
The 313 bp DNA barcode region of mitochondria cytochrome c oxidase I (COX1) was amplified using the metazoan universal primers mlCOIintF (5′-GGWACWGGWTGAACWGTWTAYCCYCC-3′) and jgHCO2198 (5′-TAIACYTCIGGRTGICCRAARAAYCA-3′), as described by Leray et al. (2013). For each sample, the first polymerase chain reaction (PCR) mixture included 2 μL of gDNA, 10 μL of 2X DNA Free-Multiplex Master Mix (Cellsafe, Korea), 0.4 μL of each 10 μM forward and reverse primer, and 7.2 μL of distilled water. This PCR was repeated three times for each sample. The PCR temperature conditions consisted of an initial denaturation cycle at 95°C for 5 min, followed by 35 cycles of denaturation at 95°C for 30 s, annealing at 46°C for 30 s, and extension at 72°C for 1 min. A final extension was performed at 72°C for 10 min, and after PCR completion, the samples were stored at 4°C. Negative controls were used to monitor for potential contamination. The PCR products were validated by electrophoresis on a 2% tris-acetate-ethylenediaminetetraacetic acid agarose gel and were subsequently purified using the QIAquick PCR Purification Kit (Qiagen, USA). Subsequently, a second round of PCR was performed to attach the multiplex identifier tags. Finally, the amplicons were sequenced using the Illumina MiSeq platform at Macrogen Inc. (Seoul, Republic of Korea), following the instructions of the manufacturer.
COX1 reference sequences for zooplankton species identification were obtained from the “All Marine Fauna + Flora Combo (Mode-C)” database in MetaZooGene (https://www.st.nmfs.noaa.gov/copepod/collaboration/metazoogene/atlas/html-src/data:MZGdbALL:o00.html) (Bucklin et al., 2021). In total, 602,592 COX1 sequences were extracted, of which 561,518 were attributed to the Animal Kingdom. The distribution of sequences for other taxa included Protozoa (692), Chromista (19,990), Plantae (19,434), and bacteria (954). A COX1 reference library was established for utilization in the Qiime2 pipeline with the Animal Kingdom COX1 sequences in the following manner: 1) Abyssogena phaseoliformis (AP014557) was selected as the seed COX1 sequence from the 561,518 Animal Kingdom COX1 sequences in the “Mode-C” database. 2) The forward primer mlCOIintF and reverse primer jgHCO2198 were annotated to this sequence. The length of the seed COX1 sequence with the attached primers was 365 bp. 3) All Animal Kingdom sequences in Mode-C were aligned to seed COX1 using the Geneious 11.1.5 mapper (option: highest sensitivity/slow). At this stage, the 3′-5′ COX1 sequences were converted to the 5′-3′ direction. The number of aligned contigs was 536,470, which was 82.3% of the initial sequences. The regions outside the mlCOIintF and jgHCO2198 primers were trimmed from the aligned contig sequences using Geneious 11.1.5. The lengths of the trimmed contig sequences ranged from 10 to 678 bp, with an average of 317.1 ± 54.1 bp. Among these, the number of unique sequences with lengths ≥250 bp was 225,759.
The reference sequences were separated at the degenerate base codes (R, Y, M, K, S, W, V, H, B, D, and N). 221,194 COX1 sequences were extracted, each with more than 250 base pairs (approximately 80% of the 313 bp length) and without any degenerate bases. Subsequently, unique sequences with lengths ≥250 bp without degenerate bases, totaling 221,194, were isolated. The sequence lengths varied from a minimum of 250 bp to a maximum of 678 bp, with an average of 329.5 ± 26.2 bp. The same taxonomic hierarchy file corresponding to these sequences was obtained from “Mode-C” in mothur format. The COX1 sequences and their corresponding classification information were formatted for the Qiime2 pipeline (Supplementary Table 1).
Raw data from Illumina Miseq were analyzed using the Qiime 2 pipeline (Supplementary Table 2) (Bolyen et al., 2019). The amplicon sequence variants (ASVs), with a 99% sequence match to the MZG database were assigned to the corresponding taxon names in the MZG database. ASVs that did not meet this criterion were excluded from the analysis. The excluded ASVs were primarily identified as bacteria, fungi, and other microorganisms. In order to reduce the impact of cross contamination, ASV with reads below 10 were removed from the ASV table (Suter et al., 2021; Zhang et al., 2024). The scientific names of the taxa based on the ASVs were in accordance with the World Register of Marine Species (WoRMS, http://www.marinespecies.org). The read copy number per species was subjected to an ln (value+1) transformation. The ln-transformed data were analyzed using ggplot2 (Wickham, 2016), vegan (Oksanen et al., 2019), and fossil (Vavrek, 2011), etc., in R v4.1.3 (R Core Team, 2018).
To assess the species diversity indices of bulk DNA and eDNA, we used the Shannon diversity index, which calculates diversity by considering both species richness and evenness (Shannon, 1948), and the ACE index, which provides an estimate of total species richness, including rare species (Chao and Lee, 1992). Subsequently, the Wilcoxon rank-sum test was used to identify the differences between the two samples. This statistical test is used to determine whether there is a statistically significant difference in the data obtained from two independent samples (Wilcoxon, 1992). The presence of common and independent species in the two sample types was evaluated using Venn diagrams (https://bioinfogp.cnb.csic.es/tools/venny/). The similarity in zooplankton species composition between the two samples was assessed through NMDS and ANOSIM. NMDS, a nonmetric form of multidimensional scaling that places data in a lower-dimensional space based on the similarity between species, was conducted using 123 iterative analyses based on the Bray–Curtis dissimilarity distance matrix. ANOSIM, conducting 999 permutation tests using the same distance matrix, compared the similarity in species composition analyzed in the two sample types, bulk DNA and eDNA, using the global R-value and P-value (Clarke, 1993). ANOSIM assesses the degree of similarity based on the average rank differences between groups. These methods are extensively used in ecological research to assess differences in species diversity and composition, with each method selected according to specific conditions and requirements.
In total, 23,108,264 sequence reads were obtained from high-throughput sequencing analysis of two types of samples, bulk DNA (17 samples) and eDNA (71 samples), collected from the Ulleung Basin during summer over three years. eDNA and bulk DNA accounted for 71.3% and 28.7% of the reads, respectively (Table 1). Sequences that passed quality filtering (QF) in Qiime2 amounted to 4,492,087 reads, representing 19.4% of the initial total. By sample type, 3,595,412 reads (54.2% of initial data) from bulk DNA samples and 896,675 reads (5.4% of initial data) from eDNA samples passed the Qiime2 QF. Read depth per sample ranged from 30,516 to 692,052 reads in bulk DNA samples, averaging 211,495 ± 228,569 reads, and from 1,488 to 293,389 reads in eDNA samples, averaging 52,746 ± 79,429 reads. The pass rate of sequence reads for the Qiime2 QF was approximately tenfold higher for bulk DNA samples than for eDNA samples.
Of the 1,761 Metazoan ASVs constructed with Qiime2 QF-passing reads, 350 species belonging to 12 phyla, 27 classes, 74 orders, 177 families, and 261 genera were detected (Supplementary Table S2). Using the sample types used for species identification, the number of ASVs was 1,049 for bulk DNA and 901 for eDNA. The 350 identified species were distributed across 12 phyla, with the highest number of species in the phylum Arthropoda (152 species, 43.4%), followed by Chordata (77 species, 22%) and Mollusca (46 species, 13.1%) (Table 2).
The phylum Arthropoda included Branchiopoda, Copepoda, Malacostraca, Ostracoda, and Thecostraca. The class with the highest number of species was Copepoda at 96 species (63.2%) (Table 3). Malacostraca had 24 species (15.8%), and Thecostraca had 17 species (11.2%). In the class Copepoda, species such as Centropages abdominalis, Clausocalanus pergens, Ctenocalanus vanus, Paracalanus parvus, Ditrichocorycaeus affinis, and Oithona similis were detected at all stations (Supplementary Table S3). In addition, 26 species with detection frequencies of over 50% included Calanus sinicus and Oithona atlantica. In the class Malacostraca, Euphausia pacifica exhibited the highest detection frequency (100%). Other species, such as Themisto japonica, Belzebub intermedius, and Oregonia gracilis, showed frequencies of over 50%. In the class Branchiopoda, Pseudevadne tergestina was present at all survey stations, and Penilia avirostris was detected 10 times. The remaining four species (Evadne spinifera, Daphnia sp., Evadne nordmanni, and Pleopis polyphemoides) showed detection frequencies of 50% or less. In the class Ostracoda, Discoconchoecia pseudodiscophora and Euconchoecia sp. showed frequencies of over 50%, whereas the other 7 species showed frequencies below 50%. In the class Thecostraca, only Balanus trigonus showed a detection frequency of over 50%, with the other 16 species showing detection frequencies of 50% or less.
Table 3. Taxonomic composition and detection frequency of the three major groups: Arthropoda, chordata, and mollusca.
The phylum Chordata comprised the classes Actinopterygii, Appendicularia, Ascidiacea, and Thaliacea. Actinopterygii represented 92.2% of the total and comprised 71 species (Table 3). The number of species in the other three classes ranged between one and three each. In Actinopterygii, Perciformes exhibited the highest species count at 40, followed by Scorpaeniformes and Pleuronectiformes, each comprising 8 species. The number of species in the other eight orders ranged from one to five. Despite being less common, Engraulis japonicus from Clupeiformes and Maurolicus japonicus from Stomiiformes demonstrated high detection frequencies, with 15 (88%) and 13 (76%) occurrences, respectively (Supplementary Table S3). Notably, there were nine occurrences of Arctoscopus japonicus in Perciformes.
The phylum Mollusca included Bivalvia, Cephalopoda, and Gastropoda. Gastropoda exhibited the most diverse species composition at 31 species (67.4%) (Table 3), followed by Bivalvia and Cephalopoda. In Gastropoda, species with occurrence frequencies of over 50% included Atlanta californiensis, Hermissenda emurai (10 occurrences each), Creseis virgula, and Clione sp. (9 occurrences each) (Supplementary Table S3). The Bivalvia species exhibited very low detection frequencies, with only Atrina pectinata and Magallana gigas appearing more than five times. In Cephalopoda, relatively high occurrence frequencies were observed for Watasenia scintillans and Todarodes pacificus.
In total, 279 species were detected in bulk DNA (Table 4). A total of 99% (276 species) of the detected species were identified at the species or genus level (Supplementary Table S4). Among these, Copepoda exhibited the highest species count at 88 (31.5%), followed by Actinopterygii (41 species, 14.7%), Gastropoda (30 species, 10.8%), and Malacostraca (21 species, 7.5%). In the bulk DNA, 29 species with detection frequencies of 10 or more were identified, belonging to groups such as Copepoda, Malacostraca, and Actinopterygii. Notably, in Copepoda, Clausocalanus pergens, Ctenocalanus vanus, Oithona similis, and Paracalanus parvus were observed across all stations. In Malacostraca, Euphausia pacifica (16 occurrences), Themisto japonica (14 occurrences), and Belzebub intermedius (10 occurrences) were frequently detected. In Actinopterygii, high detection frequencies were recorded for Engraulis japonicus (14 occurrences) and Maurolicus japonicus (10 occurrences), while in Gastropoda, Atlanta californiensis and Hermissenda emurai (10 occurrences each) were notable (Supplementary Table S5).
In total, 190 species were detected in the eDNA (Table 4). A total of 98% (187 species) of the detected species were identified at the species or genus level (Supplementary Table S4). Among these, Copepoda exhibited the highest number of species at 54 (28.4%), followed by Actinopterygii (53 species, 27.9%), Hydrozoa (10 species, 5.8%), Malacostraca, Polychaeta, and Echinoidea (8 species, 4.2% each), among others (Table 4). In the eDNA, 15 species belonging to Copepoda, Malacostraca, Branchiopoda, Ascidiacea, and Actinopterygii were identified with detection frequencies of 10 or more. In Copepoda, Centropages abdominalis and Paracalanus parvus appeared across all stations, while Clausocalanus pergens, Oithona similis, and Triconia borealis had 14 or more occurrences. In Malacostraca, Euphausia pacifica (11 occurrences) was observed at all stations, whereas in Actinopterygii, Engraulis japonicus and Maurolicus japonicus both had 10 occurrences (Supplementary Table S5).
A total of 119 species (34%) (189 ASVs, 10.8%) were commonly detected in both the bulk DNA and eDNA, with Copepoda, Actinopterygii, and Hydrozoa being the major taxonomic groups (Figure 2 and Supplementary Table S6). Among these, Copepoda accounted for the majority at 46 species (38.7%), and Paracalanus parvus, Clausocalanus pergens, Centropages abdominalis, and Oithona similis exhibited high occurrence frequencies (Figure 2 and Supplementary Table S7). For Actinopterygii (23 species, 19.3%), Engraulis japonicus, Maurolicus japonicus, and Sillago japonica were prominent in frequency. For Hydrozoa (8 species, 6.7%), Agalma elegans, Apolemia sp., and Muggiaea atlantica were frequently observed. A total of 160 species (860 ASVs, 48.8%) were detected exclusively in the bulk DNA, while 71 species (712 ASVs, 40.4%) were unique to the eDNA (Figure 2). Among the taxonomic groups found only in bulk DNA, Copepoda comprised 42 species (26.3%), followed by Gastropoda with 25 species (15.6%), Actinopterygii with 18 species (11.3%), and Malacostraca with 16 species (10%) (Figure 2 and Supplementary Table S7). At the class level in Copepoda, Paraeuchaeta sp., Acartia negligens, Clausocalanus furcatus, and Gaetanus minutus had the highest frequencies of occurrence, with each appearing six times (Supplementary Table S7). In Gastropoda, Hermissenda emurai (ten times) and Clione sp. (nine times) were frequently detected. Although Actinopterygii included numerous species, the highest frequency observed was only three times for Trachurus japonicus. In Malacostraca, Themisto japonica was the most frequent, with 14 occurrences. Conversely, among the taxonomic groups exclusively detected in the eDNA, Actinopterygii had the highest number of species with 30 (42.3%), followed by Copepoda (8 species, 11.3%), Polychaeta (5 species, 7%), and Thecostraca and Eurotatoria (4 species each, 5.6%) as the primary groups (Figure 2 and Supplementary Table S7). In Actinopterygii, Arctoscopus japonicus (nine times), Plecoglossus altivelis, and Sardinops sagax (five times each) were frequently detected. Although Eurotatoria included a significant number of species, its highest frequency was only three times for Synchaeta hutchingsi. Tharyx sp. (four times) in Polychaeta and Amphibalanus improvisus (four times) in Thecostraca showed high occurrence frequencies.
Figure 2. Comparison of species detected by each method. (A) Venn diagram representing the number of shared and unique species detected by the bulk DNA and eDNA methods. (B) Stacked bar plot representing the composition of shared and unique species for the bulk DNA and eDNA methods. The categories are as follows: ‘Common’: species observed in both methods; ‘Bulk DNA (Ex)’: species observed exclusively with the bulk DNA method; ‘eDNA (Ex)’: species observed exclusively with the eDNA method.
We analyzed the differences in species composition between the two sample types of bulk DNA and eDNA. The taxonomic groups that exhibited significantly different occurrence frequencies between the sample types were Copepoda, Gastropoda, Actinopterygii, Echinoidea, Ostracoda, Eurotatoria, Hydrozoa, Bivalvia, and Malacostraca (Wilcoxon rank-sum test, P-value <0.05; Supplementary Table S8). Differences in the species diversity indices between bulk DNA and eDNA were also observed (Figure 3 and Supplementary Table S9). The monthly average Shannon–Wiener index was highest in July (4.34) for bulk DNA, followed by August (3.94) and June (3.51). Similarly, eDNA recorded its highest value in July (4.32), followed by June (3.31) and August (2.62). For the ACE index, the monthly averages peaked in July (89.99) for bulk DNA, followed by August (57.07) and June (38.91). For eDNA, the highest value was recorded in July (81.3), followed by June (32.5) and August (15.62). Statistically significant differences in these diversity indices were observed in August (Wilcoxon rank-sum test, P-value < 0.05). NMDS analysis, based on Bray–Curtis dissimilarity, was conducted to compare the similarity in species composition between bulk DNA and eDNA (Figure 4). The analysis confirmed statistically significant differences in species composition between the bulk DNA and eDNA samples (stress value = 0.14; ANOSIM, global R= 0.4692; P-value <0.01).
Figure 3. Comparison of alpha-diversity indices between bulk DNA and eDNA. Statistical differences in biodiversity indices according to the methods used were calculated using the Wilcoxon rank-sum test. (A) Shannon diversity index, (B) ACE index. (Wilcoxon rank-sum test, P-value < 0.01 (**), P-value < 0.05 (*)).
Figure 4. Nonmetric multidimensional scaling (NMDS) plots for zooplankton communities identified using the bulk DNA and eDNA methods. The NMDS analysis for zooplankton communities was based on Bray–Curtis dissimilarities according to each method. Statistical differences were calculated using the analysis of similarity (ANOSIM) (stress value = 0.17; ANOSIM, global R = 0.6402, P-value < 0.01).
A total of 350 taxa were detected in bulk DNA derived from zooplankton collected with nets and from eDNA released into their habitat in the Ulleung Basin, known for its high species diversity among marine areas surrounding the Korean Peninsula. This was achieved through DNA metabarcoding of the COX1 barcode region (Supplementary Table S2). The number of species detected in this study was three to six times greater than the 111 species reported by Park and Choi (1997), 101 species reported by Park et al. (1998), 59 species reported by Kang et al. (2002), and 116 species reported by Rho et al. (2010) in the same and adjacent waters surveyed. Furthermore, of the 350 taxa detected in this study, 299 were identified to the species level and 47 to the genus level, representing approximately 99% of the total identification (Supplementary Table S4). This significant increase in species identification is attributed to the use of DNA barcodes for species identification and the simultaneous analysis of eDNA.
DNA barcoding plays a crucial role in enhancing the accuracy of species identification, particularly in cases where genetic variation is low or specimens are atypical (Hebert et al., 2003; Hajibabaei et al., 2007). This makes DNA barcoding a reliable tool for biodiversity studies, especially in marine ecosystems, where it significantly contributes to the identification of new or rarely observed species (Valentini et al., 2016). In this study, the ASV was assigned to a taxonomy in the MZG database when its sequence showed a 99% match with sequences in the MZG database (Bucklin et al., 2021). The reliability of DNA metabarcoding is inherently dependent on the scope and quality of the reference database that links genetic sequences to taxonomic names (Vuataz et al., 2024). Therefore, continuous updates to the reference library are essential to enhance the accuracy and objectivity of research outcomes (Bucklin et al., 2016; Ransome et al., 2017). These updates not only reduce the number of unidentified sequences in metabarcoding analyses but also aid in uncovering undescribed species within poorly studied groups (Pappalardo et al., 2021).
DNA metabarcoding identified 99% of the ASVs at the species or genus level (Supplementary Table S4), which was higher than the identification rate achieved through morphology-based methods. Certain groups, such as those identified at the order level for Amphipoda, genus level for Euphausiids, family level for Salps, developmental stages of Calyptopsis, and larvae of Copepoda, were particularly challenging to identify at the species or genus level (Park and Choi, 1997; Park et al., 1998; Kang et al., 2002; Rho et al., 2010). Additionally, cryptic species within groups such as Bivalvia, Hydrozoa, Polychaeta, and Scyphozoa present challenges for species identification (Hirai et al., 2015). The difficulties in morphology-based species identification arise from the limited availability of species classification information, discrepancies between records of the same species, and the significant impact of developmental stage and specimen damage (Chihara and Murano, 1997; Richards, 2005; Okiyama, 2014). In contrast, DNA barcode information is updated and accessible in real-time through resources such as MZG, GenBank, BOLD, and SILVA. DNA metabarcoding, utilizing DNA barcodes, is not affected by morphological trait changes across developmental stages, such as those of larvae or eggs with indistinct characteristics, or by physical damage to specimens (Hofmann et al., 2017; Suter et al., 2021; Breitbart et al., 2023).
Another factor contributing to the large number of species detected in this study was the simultaneous analysis of eDNA present in traces released by organisms into their habitats and bulk DNA obtained from zooplankton net samples. By utilizing both sampling methods, a total of 119 species (189 ASVs, 10.8%) were commonly detected, with the major taxonomic groups being Copepoda, Actinopterygii, and Hydrozoa (Figure 2 and Supplementary Table S6). Excluding these common taxa, the number of species detected exclusively by each collection method was 160 species (860 ASVs, 48.8%) for bulk DNA and 71 species (712 ASVs, 40.4%) for eDNA (Figure 2 and Supplementary Table S6). Notably, among the species detected exclusively in bulk DNA, Copepoda had the highest number, whereas Actinopterygii was the most abundant among the species detected only in eDNA. The species composition of zooplankton collected with nets is limited to mesoplankton (200 μm–2 mm) due to the mesh size (typically 200–300 μm) and towing speed (approximately 1 m/s) of zooplankton nets. Therefore, zooplankton species diversity is influenced by the type and size of nets used for collection (Berry et al., 2019). In particular, the species composition of small zooplankton with conventional mesh sizes (<200 μm) may be underestimated when using conventional zooplankton nets compared to when using ultra-fine nets (Gallienne and Robins, 2001; Turner, 2004). Taxonomic groups with excellent swimming abilities, such as fish, are difficult to collect with zooplankton nets because of their avoidance abilities; however, taxonomic groups with gelatinous components that have difficulty maintaining morphological traits, such as jellyfish, can be damaged during collection (Djurhuus et al., 2018; van Bleijswijk et al., 2020). In this study, the simultaneous analysis of bulk DNA based on zooplankton nets and metabarcoding of eDNA contained in seawater was considered an effective solution for these problems. In particular, as eDNA analyzes DNA fragments from organisms in the ocean, a wide range of taxonomic groups were detectable, ranging from ultra-nanoplankton (<2 μm) to mega-plankton (>2 cm), as well as fast-swimming Actinopterygii and Cephalopoda.
In our three zooplankton diversity surveys using bulk DNA from zooplankton nets and eDNA from filtered seawater, the species composition and diversity detected in the two sample types were different. The taxonomic groups with distinct differences were Copepoda, Actinopterygii, Gastropoda, Hydrozoa, Echinoidea, Ostracoda, Eurotatoria, Bivalvia, and Malacostraca (Supplementary Table S8). Among the twenty classes, including Copepoda, Gastropoda, Malacostraca, Hydrozoa, Echinoidea, Ostracoda, and Bivalvia, more species were detected in the bulk DNA than in the eDNA (Table 4 and Supplementary Table S6). These results have been similarly observed in other studies, such as Djurhuus et al. (2018); Koziol et al. (2019), and Leduc et al. (2019). This may be because holoplanktonic species and meroplanktonic larvae can be collected more efficiently with bulk DNA sampling methods using zooplankton nets than with eDNA sampling methods (Djurhuus et al., 2018).
In contrast to the above taxonomic groups, seven classes, including Actinopterygii, Cephalopoda, Eurotatoria, and Scyphozoa, had more species detected in the eDNA than in the bulk DNA. Actinopterygii and Cephalopoda classes, due to their fast swimming speeds, make collection nearly impossible with small zooplankton nets. However, various types of organic matter containing DNA fragments released into their habitat, that is, eDNA, can be easily obtained by filtering seawater, allowing species-level identification through fragmented DNA barcode analysis. Moreover, the Scyphozoa (jellyfish) class, which has poorer swimming abilities, exhibited a greater number of detected species in eDNA analysis compared to bulk DNA analysis. Species within this class, such as Nemopilema nomurai, Cyanea nozakii, Sanderia malayensis, and Cyanea purpurea, are challenging to collect using zooplankton nets due to their delicate bodies (Di Capua et al., 2021). However, eDNA metabarcoding is able to effectively detect these species by analyzing the diverse DNA traces they release into the environment.
Through DNA metabarcoding of bulk DNA and eDNA from marine areas surrounding the Korean Peninsula, this study successfully detected 350 species of various marine animals, including significantly more zooplankton than in previous studies. These methods demonstrated a high species identification accuracy of over 99%, confirming their potential to enhance taxonomic resolution compared to that of traditional morphological methods. Moreover, the differences in species composition and diversity detected between the two types of specimens indicate that sampling methods can have significant effects on the assessment of species diversity. eDNA analysis is particularly useful for detecting species with high swimming ability or those that are difficult to sample. Therefore, it is necessary to integrate various sampling and analytical methods to obtain more accurate and comprehensive data on species diversity.
The raw sequences datasets presented in this study can be found in the Sequence Read Archive (SRA) repository of the NCBI (August 2019: Bulk DNA: PRJNA1048109, eDNA: PRJNA1048132; June 2020: Bulk DNA: PRJNA1048135, eDNA: PRJNA1048650; July 2021: Bulk DNA: PRJNA1048549, eDNA: PRJNA1048645).
JC: Data curation, Formal analysis, Methodology, Resources, Writing – original draft, Writing – review & editing, Conceptualization, Funding acquisition, Investigation, Software, Validation, Visualization. SK: Writing – review & editing, Data curation, Methodology, Resources, Software, Supervision. CK: Writing – review & editing, Conceptualization, Funding acquisition, Methodology, Project administration, Resources, Supervision.
The author(s) declare financial support was received for the research, authorship, and/or publication of this article. This research was funded by the Korea Institute of Ocean Science and Technology (grant number: PEA0201 and PE99964).
We express our gratitude to the KIOST researchers and all crew members of the R/V Onnuri, Isabu, and Eardo for their invaluable assistance with the sampling process.
The authors declare that the research was conducted in the absence of any commercial or financial relationships that could be construed as a potential conflict of interest.
All claims expressed in this article are solely those of the authors and do not necessarily represent those of their affiliated organizations, or those of the publisher, the editors and the reviewers. Any product that may be evaluated in this article, or claim that may be made by its manufacturer, is not guaranteed or endorsed by the publisher.
The Supplementary Material for this article can be found online at: https://www.frontiersin.org/articles/10.3389/fmars.2024.1351148/full#supplementary-material
Beaugrand G., Reid P. C., Ibañez F., Lindley J. A., Edwards M. (2002). Reorganization of North Atlantic marine copepod biodiversity and climate. Science 296, 1692–1694. doi: 10.1126/science.1071329
Berry T. E., Saunders B. J., Coghlan M. L., Stat M., Jarman S., Richardson A. J., et al. (2019). Marine environmental DNA biomonitoring reveals seasonal patterns in biodiversity and identifies ecosystem responses to anomalous climatic events. PloS Genet. 15, e1007943. doi: 10.1371/journal.pgen.1007943
Bohmann K., Evans A., Gilbert M. T. P., Carvalho G. R., Creer S., Knapp M., et al. (2014). Environmental DNA for wildlife biology and biodiversity monitoring. Trends Ecol. Evol. 29, 358–367. doi: 10.1016/j.tree.2014.04.003
Bolyen E., Rideout J. R., Dillon M. R., Bokulich N. A., Abnet C. C., Al-Ghalith G. A., et al. (2019). Reproducible, interactive, scalable and extensible microbiome data science using QIIME 2. Nat. Biotechnol. 37, 852–857. doi: 10.1038/s41587-019-0209-9
Boxshall G. A., Halsey S. H. (2004). An Introduction to Copepod Diversity (London, UK: The Ray Society).
Breitbart M., Kerr M., Schram M. J., Williams I., Koziol G., Peebles E., et al. (2023). Evaluation of DNA metabarcoding for identifying fish eggs: A case study on the West Florida Shelf. PeerJ 11, e15016. doi: 10.7717/peerj.15016
Bucklin A., Lindeque P. K., Rodriguez-Ezpeleta N., Albaina A., Lehtiniemi M. (2016). Metabarcoding of marine zooplankton: prospects, progress, and pitfalls. J. Plankton Res. 38, 393–400. doi: 10.1093/plankt/fbw023
Bucklin A., Peijnenburg K. T. C. A., Kosobokova K. N., Ortman B. D., Jennings R. M., Hoarau G. (2021). Toward a global reference database of COI barcodes for marine zooplankton. Mar. Biol. 168 (78). doi: 10.1007/s00227-021-03887-y
Chao A., Lee S. M. (1992). Estimating the number of classes via sample coverage. J. Am. Stat. Assoc. 87, 210–217. doi: 10.1080/01621459.1992.10475194
Chihara M., Murano M. (1997). An Illustrated Guide to Marine Plankton in Japan (Tokyo, Japan: Tokai University Press).
Clarke K. R. (1993). Non-parametric multivariate analyses of changes in community structure. Aust. J. Ecol. 18, 117–143. doi: 10.1111/j.1442-9993.1993.tb00438.x
Constable A. J., Melbourne-Thomas J., Corney S. P., Arrigo K. R., Barbraud C., Barnes D. K., et al. (2014). Climate change and Southern Ocean ecosystems I: How changes in physical habitats directly affect marine biota. Glob. Change Biol. 20, 3004–3025. doi: 10.1111/gcb.12623
Deiner K., Bik H. M., Mächler E., Seymour M., Lacoursière-Roussel A., Altermatt F., et al. (2017). Environmental DNA metabarcoding: transforming how we survey animal and plant communities. Mol. Ecol. 26, 5872–5895. doi: 10.1111/mec.14350
Di Capua I., Piredda R., Mazzocchi M. G., Zingone A. (2021). Metazoan diversity and seasonality through eDNA metabarcoding at a Mediterranean long-term ecological research site. ICES J. Mar. Sci. 78, 3303–3316. doi: 10.1093/icesjms/fsab059
Djurhuus A., Pitz K., Sawaya N. A., Rojas-Márquez J., Michaud B., Montes E., et al. (2018). Evaluation of marine zooplankton community structure through environmental DNA metabarcoding. Limnol. Oceanogr. Methods 16, 209–221. doi: 10.1002/lom3.10237
Frederiksen M., Edwards M., Richardson A. J., Halliday N. C., Wanless S. (2006). From plankton to top predators: bottom-up control of a marine food web across four trophic levels. J. Anim. Ecol. 75, 1259–1268. doi: 10.1111/j.1365-2656.2006.01148.x
Gallienne C. P., Robins D. B. (2001). Is Oithona the most important copepod in the world’s oceans? J. Plankton Res. 23, 1421–1432. doi: 10.1093/plankt/23.12.1421
Gannon J. E. (1980). Towards improving the use of zooplankton in water quality surveillance of the St. Lawrence Great Lakes. Can. Tech. Rep. Fish. Aquat. Sci. 976, 87–109.
Gismervik I. (2006). Top-down impact by copepods on ciliate numbers and persistence depends on copepod and ciliate species composition. J. Plankton Res. 28, 499–507. doi: 10.1093/plankt/fbi135
Hajibabaei M., Singer G. A. C., Hebert P. D. N., Hickey D. A. (2007). DNA barcoding: How it complements taxonomy, molecular phylogenetics and population genetics. Trends Genet. 23, 167–172. doi: 10.1016/j.tig.2007.02.001
He W., Wang L., Ou D., Li W., Huang H., Ou R., et al. (2023). Fish diversity monitoring using environmental DNA techniques in the clarion–Clipperton zone of the Pacific Ocean. Water 15, 2123. doi: 10.3390/w15112123
Hebert P. D. N., Cywinska A., Ball S. L., deWaard J. R. (2003). Biological identifications through DNA barcodes. Proc. R. Soc. B.: Biol. Sci. 270, 313–321. doi: 10.1098/rspb.2002.2218
Heimeier D., Lavery S., Sewell M. A. (2010). Using DNA barcoding and phylogenetics to identify Antarctic invertebrate larvae: lessons from a large scale study. Mar. Genomics 3, 165–177. doi: 10.1016/j.margen.2010.09.004
Hervé A., Domaizon I., Baudoin J. M., Dejean T., Gibert P., Jean P., et al. (2022). Spatio-temporal variability of eDNA signal and its implication for fish monitoring in lakes. PloS One 17, e0272660. doi: 10.1371/journal.pone.0272660
Hirai J., Tachibana A., Tsuda A. (2020). Large-scale metabarcoding analysis of epipelagic and mesopelagic copepods in the Pacific. PloS One 15, e0233189. doi: 10.1371/journal.pone.0233189
Hirai J., Yasuike M., Fujiwara A., Nakamura Y., Hamaoka S., Katakura S., et al. (2015). Effects of plankton net characteristics on metagenetic community analysis of metazoan zooplankton in a coastal marine ecosystem. J. Exp. Mar. Biol. Ecol. 469, 36–43. doi: 10.1016/j.jembe.2015.04.011
Hoegh-Guldberg O., Bruno J. F. (2010). The impact of climate change on the world’s marine ecosystems. Science 328, 1523–1528. doi: 10.1126/science.1189930
Hofmann T., Knebelsberger T., Kloppmann M., Ulleweit J., Raupach M. J. (2017). Egg identification of three economically important fish species using DNA bar coding in comparison to a morphological determination. J. Appl. Ichthyol. 33, 925–932. doi: 10.1111/jai.13389
Kang J. H., Kim W. S., Shim J. H. (2002). Species composition and abundance of zooplankton community in spring and autumn around Dokdo. Ocean Polar. Res. 24, 407–417. doi: 10.4217/OPR.2002.24.4.407
Keck F., Blackman R. C., Bossart R., Brantschen J., Couton M., Hürlemann S., et al. (2022). Meta-analysis shows both congruence and complementarity of DNA and eDNA metabarcoding to traditional methods for biological community assessment. Mol. Ecol. 31, 1820–1835. doi: 10.1111/mec.16364
Koziol A., Stat M., Simpson T., Jarman S., DiBattista J. D., Harvey E. S., et al. (2019). Environmental DNA metabarcoding studies are critically affected by substrate selection. Mol. Ecol. Resour. 19, 366–376. doi: 10.1111/1755-0998.12971
Lacoursière-Roussel A., Rosabal M., Bernatchez L. (2016). Estimating fish abundance and biomass from eDNA concentrations: variability among capture methods and environmental conditions. Mol. Ecol. Resour. 16, 1401–1414. doi: 10.1111/1755-0998.12522
Leduc N., Lacoursière-Roussel A., Howland K. L., Archambault P., Sevellec M., Normandeau E., et al. (2019). Comparing eDNA metabarcoding and species collection for documenting Arctic metazoan biodiversity. Environ. DNA. 1, 342–358. doi: 10.1002/edn3.35
Lee S. H., Kang C. K., Lee C. I., Kwak J. H. (2017). Current status of the East Sea ecosystem in a changing world. Deep Sea Res. II. 146, 101–103. doi: 10.1016/j.dsr2.2017.12.004
Leray M., Yang J. Y., Meyer C. P., Mills S. C., Agudelo N., Ranwez V., et al. (2013). A new versatile primer set targeting a short fragment of the mitochondrial COI region for metabarcoding metazoan diversity: application for characterizing coral reef fish gut contents. Front. Zool. 10, 34. doi: 10.1186/1742-9994-10-34
Lindeque P. K., Parry H. E., Harmer R. A., Somerfield P. J., Atkinson A. (2013). Next generation sequencing reveals the hidden diversity of zooplankton assemblages. PloS One 8, e81327. doi: 10.1371/journal.pone.0081327
Machida R. J., Hashiguchi Y., Nishida M., Nishida S. (2009). Zooplankton diversity analysis through single-gene sequencing of a community sample. BMC Genomics 10, 438. doi: 10.1186/1471-2164-10-438
Mack H. R., Conroy J. D., Blocksom K. A., Stein R. A., Ludsin S. A. (2012). A comparative analysis of zooplankton field collection and sample enumeration methods. Limnol. Oceanogr. Methods 10, 41–53. doi: 10.4319/lom.2012.10.41
Mariani S., Fernandez C., Baillie C., Magalon H., Jaquemet S. (2021). Shark and ray diversity, abundance and temporal variation around an Indian Ocean Island, inferred by eDNA metabarcoding. Conserv. Sci. Pract. 3, e407. doi: 10.1111/csp2.407
Meybeck M. (2003). Global analysis of river systems: from Earth system controls to Anthropocene syndromes. Philos. Trans. R. Soc Lond. B. Biol. Sci. 358, 1935–1955. doi: 10.1098/rstb.2003.1379
Moon S. Y., Lee M. H., Jung K. M., Kim H., Jung J. H. (2022). Spatial and temporal distribution and characteristics of zooplankton communities in the southern coast of Korea from spring to summer period. Korean J. Fish. Aquat. Sci. 55, 154–170. doi: 10.5657/KFAS.2022.0154
Okiyama M. (2014). An Atlas of the Early Stage Fishes in Japan (Tokyo, Japan: Tokai University Press).
Oksanen J., Simpson G. L., Blanchet F. G., Kindt R., Legendre P., Minchin P. R., et al. (2019). vegan: Community Ecology Package.
Pappalardo P., Collins A. G., Pagenkopp Lohan K. M., Hanson K. M., Truskey S. B., Jaeckle W., et al. (2021). The role of taxonomic expertise in interpretation of metabarcoding studies. ICES J. Mar. Sci. 78, 3397–3410. doi: 10.1093/icesjms/fsab082
Park C., Choi J. K. (1997). Zooplankton community in the front zone of the East sea of Korea (the Sea of Japan): 1. Species list, distribution of dominant taxa, and species association. Korean J. Fish. Aquat. Sci. 30, 225–238.
Park C., Lee C. R., Kim J. C. (1998). Zooplankton community in the front zone of the East Sea (the Sea of Japan), Korea: 2. Relationship between abundance distribution and seawater temperature. Korean J. Fish. Aquat. Sci. 31, 749–759.
Pusceddu A., Bianchelli S., Martín J., Puig P., Palanques A., Masqué P., et al. (2014). Chronic and intensive bottom trawling impairs deep-sea biodiversity and ecosystem functioning. Proc. Natl. Acad. Sci. U. S. A. 111, 8861–8866. doi: 10.1073/pnas.1405454111
R Core Team (2018). R: A Language and Environment for Statistical Computing (Vienna, Austria: R Foundation for Statistical Computing).
Ransome E., Geller J. B., Timmers M., Leray M., Mahardini A., Sembiring A., et al. (2017). The importance of standardization for biodiversity comparisons: A case study using autonomous reef monitoring structures (ARMS) and metabarcoding to measure cryptic diversity on Mo’orea coral reefs, French Polynesia. PloS One 12, e0175066. doi: 10.1371/journal.pone.0175066
Rho T. K., Kim Y. B., Park J. I., Lee Y. W., Im D. H., Kang D. J., et al. (2010). Plankton community response to physico-chemical forcing in the Ulleung Basin, East Sea during summer 2008. Ocean Polar. Res. 32, 269–289. doi: 10.4217/OPR.2010.32.3.269
Richards W. J. (2005). Early Stages of Atlantic Fishes: an Identification Guide for the Western Central North Atlantic (Boca Raton, FL: CRC Press).
Sabatés A., Gili J. M., Pagès F. (1989). Relationship between zooplankton distribution, geographic characteristics and hydrographic patterns off the Catalan coast (western Mediterranean). Mar. Biol. 103, 153–159. doi: 10.1007/BF00543342
Schloss P. D., Westcott S. L., Ryabin T., Hall J. R., Hartmann M., Hollister E. B., et al. (2009). Introducing Mothur: open-source, platform-independent, community-supported software for describing and comparing microbial communities. Appl. Environ. Microbiol. 75, 7537–7541. doi: 10.1128/AEM.01541-09
Shannon C. E. (1948). A mathematical theory of communication. Bell Syst. Tech. J. 27, 379–423. doi: 10.1002/j.1538-7305.1948.tb01338.x
Shin J. W., Park J., Choi J. G., Jo Y. H., Kang J. J., Joo H., et al. (2017). Variability of phytoplankton size structure in response to changes in coastal upwelling intensity in the southwestern East Sea. JGR Oceans. 122, 10262–10274. doi: 10.1002/2017JC013467
Song C. U., Choi H., Jeon M. S., Kim E. J., Jeong H. G., Kim S., et al. (2021). Zooplankton diversity monitoring strategy for the urban coastal region using metabarcoding analysis. Sci. Rep. 11, 24339. doi: 10.1038/s41598-021-03656-3
Spear M. J., Embke H. S., Krysan P. J., Vander Zanden M. J. (2021). Application of eDNA as a tool for assessing fish population abundance. Environ. DNA. 3, 83–91. doi: 10.1002/edn3.94
Suter L., Polanowski A. M., Clarke L. J., Kitchener J. A., Deagle B. E. (2021). Capturing open ocean biodiversity: comparing environmental DNA metabarcoding to the continuous plankton recorder. Mol. Ecol. 30, 3140–3157. doi: 10.1111/mec.15587
Tranter D. J., Heron A. C. (1965). Filtration characteristics of Clarke-Bumpus samplers. Mar. Freshw. Res. 16, 281–292. doi: 10.1071/MF9650281
Turner J. T. (2004). The importance of small planktonic copepods and their roles in pelagic marine food webs. Zool. Stud. 43, 255–266.
Valentini A., Pompanon F., Taberlet P. (2009). DNA barcoding for ecologists. Trends Ecol. Evol. 24, 110–117. doi: 10.1016/j.tree.2008.09.011
Valentini A., Taberlet P., Miaud C., Civade R., Herder J., Thomsen P. F., et al. (2016). Next-generation monitoring of aquatic biodiversity using environmental DNA metabarcoding. Mol. Ecol. 25, 929–942. doi: 10.1111/mec.13428
van Bleijswijk J. D. L., Engelmann J. C., Klunder L., Witte H. J., Witte J. I., van der Veer H. W. (2020). Analysis of a coastal North Sea fish community: comparison of aquatic environmental DNA concentrations to fish catches. Environ. DNA. 2, 429–445. doi: 10.1002/edn3.67
Vavrek M. J. (2011). Fossil: palaeoecological and palaeogeographical analysis tools. Palaeontol. Electron. 14, 16.
Volis S., Ormanbekova D., Shulgina I. (2016). Role of selection and gene flow in population differentiation at the edge vs. interior of the species range differing in climatic conditions. Mol. Ecol. 25, 1449–1464. doi: 10.1111/mec.13565
Vuataz L., Reding J. P., Reding A., Roesti C., Stoffel C., Vinçon G., et al. (2024). A comprehensive DNA barcoding reference database for Plecoptera of Switzerland. Sci. Rep. 14, 6322. doi: 10.1038/s41598-024-56930-5
Ward R. D., Zemlak T. S., Innes B. H., Last P. R., Hebert P. D. (2005). DNA barcoding Australia’s fish species. Philos. Trans. R. Soc Lond. B. Biol. Sci. 360, 1847–1857. doi: 10.1098/rstb.2005.1716
Wickham H. (2016). Data Analysis. In ggplot2: Elegant Graphics for Data Analysis (Cham, Switzerland: Springer International Publishing), 189–201. doi: 10.1007/978-3-319-24277-4
Wilcoxon F. (1992). “Individual comparisons by ranking methods,” in Breakthroughs in Statistics: Methodology and Distribution (Springer, New York), 196–202. doi: 10.1007/978-1-4612-4380-9_16
Keywords: zooplankton, species diversity, environmental DNA (eDNA), bulk DNA, DNA metabarcoding
Citation: Choi JH, Kim S and Kim C-g (2024) Evaluating zooplankton species diversity using environmental DNA and bulk-DNA metabarcoding in the Ulleung Basin of the Southeastern Korean Peninsula in the summer. Front. Mar. Sci. 11:1351148. doi: 10.3389/fmars.2024.1351148
Received: 06 December 2023; Accepted: 09 October 2024;
Published: 28 October 2024.
Edited by:
Leocadio Blanco-Bercial, Bermuda Institute of Ocean Sciences, BermudaReviewed by:
Ashrenee Govender, South African Association for Marine Biological Research, South AfricaCopyright © 2024 Choi, Kim and Kim. This is an open-access article distributed under the terms of the Creative Commons Attribution License (CC BY). The use, distribution or reproduction in other forums is permitted, provided the original author(s) and the copyright owner(s) are credited and that the original publication in this journal is cited, in accordance with accepted academic practice. No use, distribution or reproduction is permitted which does not comply with these terms.
*Correspondence: Choong-gon Kim, a2ltY2dAa2lvc3QuYWMua3I=; Sung Kim, c2tpbUBraW9zdC5hYy5rcg==
Disclaimer: All claims expressed in this article are solely those of the authors and do not necessarily represent those of their affiliated organizations, or those of the publisher, the editors and the reviewers. Any product that may be evaluated in this article or claim that may be made by its manufacturer is not guaranteed or endorsed by the publisher.
Research integrity at Frontiers
Learn more about the work of our research integrity team to safeguard the quality of each article we publish.