- 1School of Life Science and Technology, Sanquan College of Xinxiang Medical University, Xinxiang, China
- 2Experimental Teaching Center of Biology and Basic Medicine Sciences, Sanquan College of Xinxiang Medical University, Xinxiang, China
- 3Shandong Key Laboratory of Eco-Environmental Science for the Yellow River Delta, Shandong University of Aeronautics, Binzhou, Shandong, China
- 4College of Life Sciences and Oceanography, Shenzhen University, Shenzhen, China
- 5School of Ecology, Resources and Environment, Dezhou University, Dezhou, China
- 6School of Environmental Science and Engineering, Southern University of Science and Technology, Shenzhen, Guangdong, China
Understanding the factors that shape the diversity, distribution, and function of denitrifying microbes is vital for managing nitrogen cycling in these ecosystems. This study explores the diversity, biogeographic distribution, assembly processes, interaction, and denitrification potential of the nirS-encoding microbial community (nirS denitrifier) in the Pearl River Estuary based on high-throughput and metagenomics sequencing dataset. The results of this study show that salinity is a crucial regulatory environmental factor that determines the spatial distribution, phylogenetic turnover, and co-occurrence patterns of nirS denitrifiers. Additionally, the dissolved organic carbon (DOC), suspended sediment concentration (SSC), and dissolved oxygen (DO) in water also significantly impact the biodiversity and abundance of nirS denitrifiers. Furthermore, our findings demonstrate that, in comparison to environmental factors, the ecological and evolutionary characteristics of nirS denitrifiers play a more prominent role in regulating their denitrification potential, suggesting that alterations in the microbial community within dynamic changes in estuarine water can profoundly affect its denitrification function. Our results indicate the significant roles of denitrification microbial structure and phylogenetic characteristics in maintaining their ecological functions. Future studies should continue to explore the interactions between microbial communities and environmental factors to further elucidate the denitrification process in estuaries and its implications for ecosystem health and water quality.
1 Introduction
With the burning of industrial fossil fuels and the application of agricultural nitrogen fertilizer, a large amount of terrestrial nitrogen is transported to the offshore area along the river (Howarth and Marino, 2006; Yu et al., 2019). This causes a series of ecological and environmental problems in offshore seawater environments, including water eutrophication, anoxia, and algae propagation (Wang et al., 2019; Zhao et al., 2021). Denitrification is the main process of water nitrogen removal. In coastal seawater, heterogeneous microbial communities can use nitrate (NO3−) as an electron acceptor to perform respiration, thus permanently removing excess nitrogen in water (Y. Li et al., 2021). The denitrification process is catalyzed by multiple kinds of metabolic enzymes, such as nitrite reductases (Nir), nitrate reductases (Nar), nitrous oxide reductases (Nos), and nitric oxide reductases (Nor) (Zumft, 1997). In particular, the rate-constraining step is catalyzed by the nitrite reductase, which is encoded by the nirS and/or nirK genes (Braker et al., 2000). Although the two genes differ in structure, both genes are similar both physiologically and functionally (Coyne et al., 1989). In comparison with nirK included in nitrifiers, nirS is more widely spread in nature, has the highest frequency, and is used as a functional biomarker of the denitrifying community (Cantera and Stein, 2007; Gao et al., 2016).
The diversity, abundance, and community structure of nirS-type denitrifiers in estuaries and waters in coastal areas are critically regulated by water environmental characteristics, such as salinity, pH, ammonia (NH4+) concentration, and oxygen concentration of seawater (Shi et al., 2019; X. Liu et al., 2020). These findings indicate that environmental selection is crucial in shaping the biogeographic patterns of nirS-type denitrifier bacteria. However, current research on microbial biogeography is shifting its focus toward the assembly processes governing microbial community biogeographic patterns (Jiao et al., 2020). Based on the theory of microbial ecology, microbial geographic distribution is affected not only by deterministic processes (environmental selection) but also by stochastic processes, such as random drift and mutation in genes, random migration in and out of microbial species, random dispersal, and random speciation and extinction (X. Hu et al., 2022). By understanding how nirS-type denitrifier bacteria diversity forms and adapts in response to environmental factors, we can gain insights into their ecological functions and contributions to biogeochemical cycles. This knowledge can also inform efforts to manipulate and engineer these bacteria for more effective biotechnological applications in environmental remediation and health (Kou et al., 2021). Currently, the assembly process of denitrifying bacteria, however, still lacks sufficient research. Microbial community assembly models can be used to distinguish the contributions of stochastic and deterministic processes in constructing microbial spatial patterns, which promotes our comprehensive understanding of microbial geographical patterns (Stegen et al., 2015). In general, the balance between the degree of genetic evolution and the ecological selection of microorganisms is the key to influencing microbial biogeographic distribution (Stegen et al., 2013). Figuring out the mechanisms that support the biogeographic patterns of microbes is a crucial topic in ecology, which raises the question of how nirS-type denitrifiers in estuaries are shaped by stochastic and deterministic processes.
Additionally, microbial species in the environment exhibit complex interactions and are significantly correlated with biogeographic characteristics (Jiao et al., 2020). Correlation-based network analysis can be used to explore their complex ecological relationships, such as interspecific competition, mutualistic symbiosis, parasitism, and predation (Faust and Raes, 2012). The interactions between denitrifier bacterial communities, such as substrate competition, may affect community assembly balance (Cai et al., 2021). However, the relationship between the assembly processes and the co-occurrence patterns within denitrifier communities remains poorly explored. Denitrification is the main release pathway of greenhouse gases (e.g., N2O) (Falkowski et al., 2008; Wang et al., 2020; Su et al., 2022). Therefore, understanding the microbial mechanism of denitrification and the secondary environmental problems caused by denitrification is essential for mitigating eutrophication and global warming in coastal estuarine waters. In contrast to the ecological characteristics of the denitrifier community, little is known about the potential denitrifying functions in coastal seawater. Metagenomics techniques can be used to reveal the overall characteristics of functional genes in microbial communities, which in part reflect the functional potential of microorganisms in a specific environment (Rasigraf et al., 2017). Denitrification in estuarine water is crucial for the biogeochemical cycling of nitrogen. However, the ecological characteristics of denitrifiers in estuarine water and their responses to estuarine environmental gradients are still poorly understood. As a typical special interface of sediment-water interactions, the bottom water in an estuary spans various physical, chemical, and biological gradients. It is also an important place for the coupling of river-estuary-marine biogeochemical cycles (Seiki et al., 2008; Jørgensen et al., 2019; Guo et al., 2023).
In this research, the functional and ecological characteristics of denitrifying microbial communities are investigated at the water-sediment interface in the Pearl River Estuary. This research aims to (1) understand the biogeographic distribution, co-occurrence pattern, and community assembly process of denitrifying microorganisms in the seawater of the Pearl River Estuary, (2) understand the denitrifying functional characteristics of estuarial seawater microorganisms and the characteristics of nitrogen metabolic pathways, and (3) construct the relationships between microbial ecological characteristics and potentially functional characteristics and to better understand the microbial mechanism of denitrification processes in estuarine water. This research may provide new inspiration for the microbial denitrification process in the water column in coastal areas and provide further indicators for the microbial mechanism of denitrification potential in coastal water.
2 Materials and methods
2.1 Research sites and fieldwork
The Pearl River Estuary (PRE) is in the northern part of the South China Sea, with a length of 80 km from north to south and an area of 2100 km2. Its dominant water source is the Pearl River, with an annual inflow of 326 billion m3 and an annual runoff of 300 billion m3 (Zeng et al., 2020). The region is characterized by a humid subtropical climate (Wong et al., 2002). The annual precipitation and temperature on average are 1690 mm and 22°C, respectively (Huang et al., 2021). The PRE is located in the core of the Guangdong-Hong Kong-Macao Greater Bay Area and has several main economic centers, including Guangzhou, Shenzhen, Zhuhai, Hong Kong, and Macao (Xia and Zhou, 2021). With the rapid development of industry and agriculture in this region, excessive anthropogenic reactive nitrogen (N) and phosphorus (P) are released into the PRE through artificial discharge, river runoff, and atmospheric deposition, which has led to water eutrophication and water quality deterioration (Niu et al., 2020a; Niu et al., 2020b).
Sampling was undertaken across the south-to-north passage, and a total of 25 sampling sites (S1-S25) were set in the study area during July 2022. Supplementary Table S1 and Figure S1 present the field sampling trips in detail. The salinity distribution was considered for dividing these study sites into 5 distinct salinity zones (zones 1–5) and 2 salinity regions, including freshwater regions (S1-S15; upstream of the estuary, mainly Pearl River water) and mixed water regions (S16-S25; downstream of the estuary, influenced by the dynamic interaction between the river and the ocean). In each sampling sites, 5 bottom water (~ 5 L, 0–0.5 m above the sediment) samples were collected using a stainless-steel water sampler from the north to the south of the estuary. After collection, some water samples were immediately transferred onboard the research cruise to analyze water pH, salinity, dissolved oxygen (DO), and turbidity. The water pH and salinity were determined by a portable multiparameter water quality meter (LMPWM-A20). Following the classic Winkler procedure, titration was applied to measure water DO. Turbidity was recorded in the light of nephelometric turbidity units (NTU), which were adopted to calculate suspended sediment concentrations (SSC, in g L−1). Dissolved organic carbon (DOC) was measured on a carbon-hydrogen-nitrogen elementary analyzer (VVarioELIII, Elementary, Germany). We adopted the ascorbic acid-molybdate blue method for assaying total phosphorus (TP) and applied a spectrophotometer to the measurements.
A sterile filter membrane (Waterman) with a pore size of 0.22 micrometers was utilized to filter water samples ranging from approximately 100 to 500 milliliters, and three filter membrane samples were collected in each sample site. The filtered membrane was kept carefully at −20°C for DNA extraction. The filtered water sample was preserved in a brown glass bottle and taken back to the laboratory to determine NOx− (including NO2− and NO3−) and NH4+ using a nutrient analyzer (SAN plus, Skalar Analytical B.V., the Netherlands), with detection limits of 0.5 μM for NH4+ and 0.1 μM for NOx− (Hou et al., 2015). The water environmental parameters were analyzed in triplicate, and the average was calculated.
2.2 DNA extraction and Illumina MiSeq sequencing
The total DNA of the filtered membrane sample was extracted by Powersoil™ DNA Isolation Kits (MO BIO, USA) based on the operation manual. Filter membranes from the same sampling sites were extracted separately for DNA, and triplicate DNA extracts were pooled for downstream molecular interpretation. A total of 25 DNA samples were collected. The pooled DNA extracts were tested with 1% agarose gel and quantified by a Nanodrop-2000 Spectrophotometer (Thermo Fisher Scientific, Waltham, the U.S.). The nirS gene was amplified from the appropriate DNA extracts by the polymerase chain reaction (PCR) primers Cd3aF and R3cd (Gao et al., 2016). PCR was conducted in a total volume of 25 μL containing 0.2 µL of Taq DNA Polymerase (5 U/μL, TaKaRa, China), 1 μL of genomic DNA (10–100 ng/μL), 0.5 μL of dNTPs (10 mM), 0.5 μL of primer (10 mM), and 2.5 μL of 10 × PCR buffer. PCRs were conducted based on our previous method (Zheng et al., 2021). Brieflly, thermal cycling for PCRs was performed at 95 °C for 30 s, followed by 40 cycles at 95 °C for 5 s, 60 °C for 30 s, and 72 °C for 10 s, and a dissolution curve program at 95 °C for 15 s, 60 °C for 15 s, and 95 °C for 15 s.
Selection Beads (Transgen, China) were used to purify the final PCR products, a Qubit3.0 DNA Kit (Life Technologies, Sweden) was used for quantification, and then the Illumina MiSeq PE250 platform was used for sequencing the products. The amplified anteroposterior sequence was spliced by the default setting of FLASH software (Edgar, 2013). First, the anteroposterior primers were removed from the spliced sequence using the fastp software (https://github.com/OpenGene/fastp). Subsequently, the resulting high-quality sequences were screened and processed using QIIME v1.8.0 software. Duplicate sequences were then removed, and the dataset was reclustered using USEARCH software (Bolyen et al., 2019; Liu et al., 2020). All read high-quality sequences were edited to the same sequence length. The sequence was analyzed according to the UPARSE-OTU algorithm using the UPARSE software package. Sequences with a similarity of 97% were designated as equivalent operational taxonomic units (OTUs) (Edgar, 2013). The representative sequence taxonomic status of every OTU was determined against the GreenGene database (RDP classifier, threshold 70%).
The water-extracted DNA was pooled (as a sample for sequencing the metagenome) from one salinity zone. Overall, 5 DNA samples were collected, consisting of 5 distinct salinity gradients. The NEBNext UltraTM DNA Library Prep Kit for Illumina (NEB, the U.S.) was used to prepare the DNA library following the manufacturer’s recommendation. Under an index code, the attribute sequence was added for the samples. AMPure XP system was used to purify the samples, and an Agilent 2100 Bioanalyzer (Agilent Technologies, CA) was used to assess the libraries. A cBot Cluster Generation System was employed for cluster generation, and then with an Illumina HiSeq2500 platform, paired-end reads (PE150) were produced to generate a metagenomic dataset of 10 Gb. Then, on the online Majorbio Cloud Platform (www.majorbio.com), the first-hand data were assessed (Ren et al., 2022). Briefly, low-quality reads, as well as adaptor and host sequences, were removed (base N > 10 bp; quality score ≤ 38; and overlap length between reads and adapter > 15 bp) in fastp software (https://github.com/OpenGene/fastp). Second, the overlapping sequences were assembled to create longer contiguous segments (contigs with lengths beyond 300 bp were kept) with Megahit (https://github.com/voutcn/megahit). Regarding assembled metagenomes, MetaGeneMark v.2.10 was used to predict the open reading frames (Zhu et al., 2010), CD-HIT v.4.5.8 was used to build a nonredundant gene catalog (unigenes), and SoapAligner v.2.21 was used for quality control (eliminating the genes with < 3 reads per sample) (Li and Godzik, 2006). DIAMOND, guided by the KEGG and NR databases (BLASTP, e-value ≤ 1e−5), was used to assign functional and taxonomic identifications (Buchfink et al., 2015). The KOs belonged to higher KEGG categories as well as KEGG pathways. The reads per kilobase of the exon model per million mapped reads (RPKM) was used to characterize the relative abundance of unigenes.
2.3 Quantitative real-time PCR
With primers cd3aF/R3cd, an ABI 7500 Sequence Detection System (Applied Biosystems, Canada) was applied to quantify nirS-encoding denitrifiers in all of the gathered samples. Supplementary Table S2 presents detailed information about the primers and amplification conditions. The Qiagen Miniprep Spin Kit was used to retrieve plasmids with the nirS gene from E. coli hosts. A Nanodrop-2000 Spectrophotometer (Thermo, U.S.) was used to quantify the plasmid DNA concentration. The plasmids were applied to construct standard curves, and the threshold cycle (CT) values were determined to quantify the target gene copy number. Briefly, the quantification standard curves were generated by plotting the CT against the logarithm of nirS gene copy numbers carried by the standard plasmid solutions, demonstrating a strong linear relationship (R2 = 0.998) and high amplification efficiency (99.7%). The standard curve spanned over 6 orders of magnitude, ranging from 1.50 × 104 to 1.50 × 109 copies per microliter of the standard plasmid’s concentrations. Furthermore, both the standards and samples exhibited a single peak at 85.4°C in their melt curves, indicating that specific DNA samples produced fluorescent signals throughout the quantitative PCR process. Triplicate qPCR assays were carried out for the whole standards and samples.
2.4 Statistical analyses
Prior to the analysis, water environment data were estimated for normal distribution with the Shapiro−Wilk test. Coefficient variations (CVs) were used to compare the dispersion of two groups of data. Log-transformation or square root transformation were applied to improve the normality of the data. In R, the “vegan” package is utilized for α-diversity analysis, employing metrics such as Sobs, Shannon and Coverage index, the “phyloseq” package is utilized to specifically calculate phylogenetic diversity (PD) for nirS-type denitrifiers at the Operational Taxonomic Unit (OTU) level. Prior to this analysis, the sequences are standardized based on the minimum sequence count of 9457 to ensure comparability across samples. The differences in denitrifying microbial abundance and diversity between sampling zones were determined using one-way analysis of variance (ANOVA) followed by Tukey’s experiment to evaluate the significance of differences. Principal Coordinates Analysis (PCoA) was used to visualize the similarities in microbial structure between different sampling sites. The Bray-Curtis dissimilarity of denitrifying microbial compositions was used for permutational multivariate analysis of variance (PERMANOVA). The difference in denitrifying microbial composition was assessed using the analysis of similarities (ANOSIM) by using the “vegan” package in the R (version 4.2.1). Pearson’s correlation was used to determine the environmental factor correlations with nirS microbial abundance and diversity. Random forest were used to identify key environmental factors. And the significance of factors was evaluated based on Mean Decrease Accuracy (MDA). Redundancy analysis (RDA) was employed to discuss the relationship between environmental factors and nirS microbial species composition. Before the RDA, detrended correspondence analysis (DCA) was applied to assess the suitability of RDA for the current sequencing analysis. RDA and DCA were conducted by CANOCO 4.5 (Microcomputer, Ithaca, NY, USA). A null model was applied to quantify the comparative significance of the deterministic and stochastic processes in the assembly processes of microbial communities. The β-nearest taxon index (βNTI) was calculated as the number of standard deviations of the observed phylogenetic turnover from the null distribution of phylogenetic turnover. βNTI values >2 or <−2 indicate heterogeneous selection (HeS, significantly more than expected phylogenetic turnover) and homogeneous selection (HS, significantly less than expected phylogenetic turnover), respectively, which represent the deterministic process. βNTI values falling within the range of −2 to 2 indicate stochastic processes that include homogenizing dispersal (HD), dispersal limitation (DL), and undominated (UN) processes. To discern these three processes, a Bray-Curtis-based Raup–Crick metric (RCbray) was calculated with RCbray > 0.95, |RCbray| < 0.95, and RCbray < −0.95 being interpreted as DL, UN, and HD processes, respectively (Stegen et al., 2013, 2015). In addition, structural equation modeling (SEM) was applied to measure the indirect and direct influences of water variables on nirS-harboring denitrifier abundance and dissimilarity (Lafuente et al., 2019).
3 Result
3.1 Variations in community structure and variance of nirS denitrifiers
A total of 1801 OTUs were identified based on a 97% sequence similarity, with a coverage ranging from 98.37% to 99.77%, indicating that the gene libraries encompass the targeted nirS-denitrifier community effectively. At the order level, the majority of nirS genes could not be classified accurately, and most OTUs were classified as Unclassified_Bacteria (UB), Unclassified_Proteobacteria (UP), and Norank_environmental samples (NES). In addition to Unclassified and Norank taxa, the predominant order identified for nirS-harboring denitrifiers was Rhodocyclales (accounting for 3.38% and 4.07% in fresh and mixed water regions, respectively), followed by Rhodobacterales (accounting for 2.61% and 2.25% in fresh and mixed water regions, respectively) (Figure 1A). Moreover, the comparative abundance of most predominant orders in nirS-harboring denitrifiers varied significantly (one-way ANOVA, P < 0.05) between the two salinity regions (Supplementary Figure S2). UP, NES, Unclassified_Betaproteobacteria (UBP), and Rhodobacterales were more abundant in fresh water, whereas mixed water regions were dominated by UB, Rhodocyclales, and Unclassified_Alphaproteobacteria (UAP). Subsequently, we calculated the local biodiversity (α-diversity) of nirS denitrifiers. In comparison, the biodiversity of nirS denitrifiers, including the Sobs and Shannon index, and the phylogenetic diversity index were markedly higher in freshwater than in the mixed water regions. However, a significantly lower community coverage index of OTU levels was observed in freshwater than in the mixed water regions (one-way ANOVA, P < 0.05) (Figure 1C). Additionally, compared to samples in mixed water regions, higher CVs of α-diversity in freshwater samples were found (Supplementary Table S3). Furthermore, the PCoA ordinations on the basis of the Bray−Curtis dissimilarity matrix showed that the nirS-type denitrifiers were clustered based on the salinity gradient (ANOSIM, P = 0.004) (Figure 1B). The PERMANOVAs revealed that the community composition of OTU levels significantly differed between freshwater and mixed-water regions (P = 0.001), which was also proven by PLS-DA analysis (Supplementary Figure S3). A total of 381 OTUs were classified as core species. In the 5 sampling zones, the number of unique OTUs was higher in zones 1 and 2, with 90 and 86 in zone 2 and zone 1, respectively. However, zone 4 had the lowest number of unique OTUs, containing only 2 unique OTUs (Figure 1D).
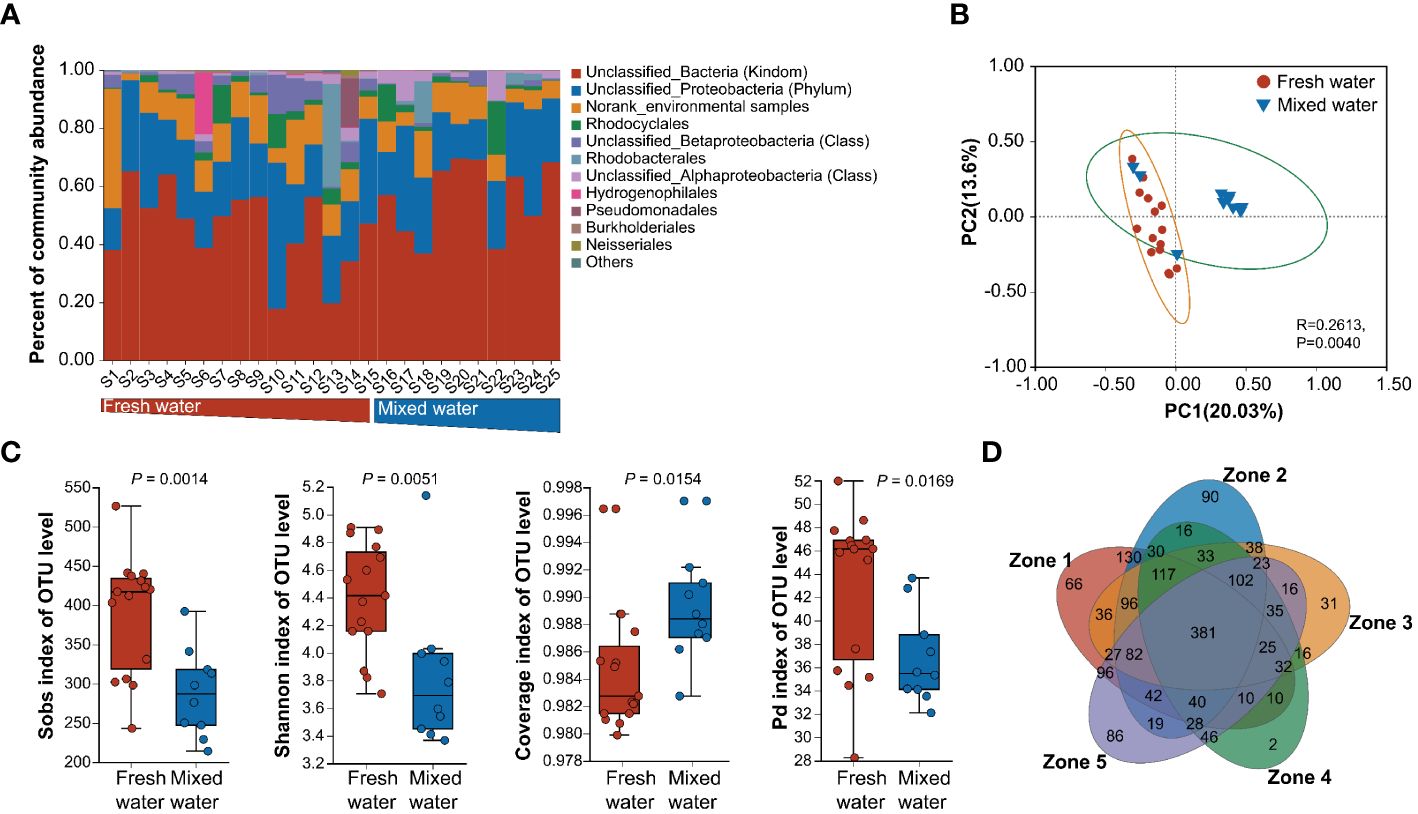
Figure 1 NirS-denitrifiers community structures, and biodiversity characteristics along the salinity gradient in Pearl River Estuary. (A) Relative abundance of the dominant order clades from low-salinity (freshwater regions) to high-salinity water regions (mixed water regions). Within the brackets denotes the lowest level with a clear taxonomic status. Other denotes relative abundances less than 0.01. (B) Principal coordinate analysis (PCoA) of the NirS-denitrifier community structure based on the Bray−Curtis distance metrics of the OTU abundances. Circles and triangles indicate water samples from the freshwater and mixed zones, respectively. (C) Alpha diversity, including the Sobs, Shannon, coverage, and phylogenetic diversity (pd) indexes of nirS-denitrifier communities between freshwater and mixed-water regions. P < 0.05 indicated a significant difference between salinity regions. (D) Venn diagram in five salinity zones based on the number of OTUs.
3.2 Effects of environmental factors on nirS-harboring denitrifier variation
In RDA, we found that the measured environmental variables, including salinity, TP, NO3–N, DOC, and SSC, appreciably impacted the variations in the composition of the nirS-denitrifier community. The SSC and DOC in waters were significantly correlated with the community composition of nirS denitrifiers in freshwater regions, and the water salinity, TP, and NO3−-N were significantly related to the nirS denitrifier community from mixed water regions (Figure 2A). However, taken together, these water properties explained only 20.20% of the total variation in community composition. This suggested that the mechanisms governing the spatial dynamics of denitrifying communities in the study area were complex. Furthermore, the random forest model results showed that water DOC, salinity, and SSC were the best predictors of spatial variation in denitrifying microorganisms (Figure 2B), with MDA values greater than 8. These results suggested that the three environmental factors (DOC, salinity, and SSC) were the major environmental factors affecting nirS denitrifiers.
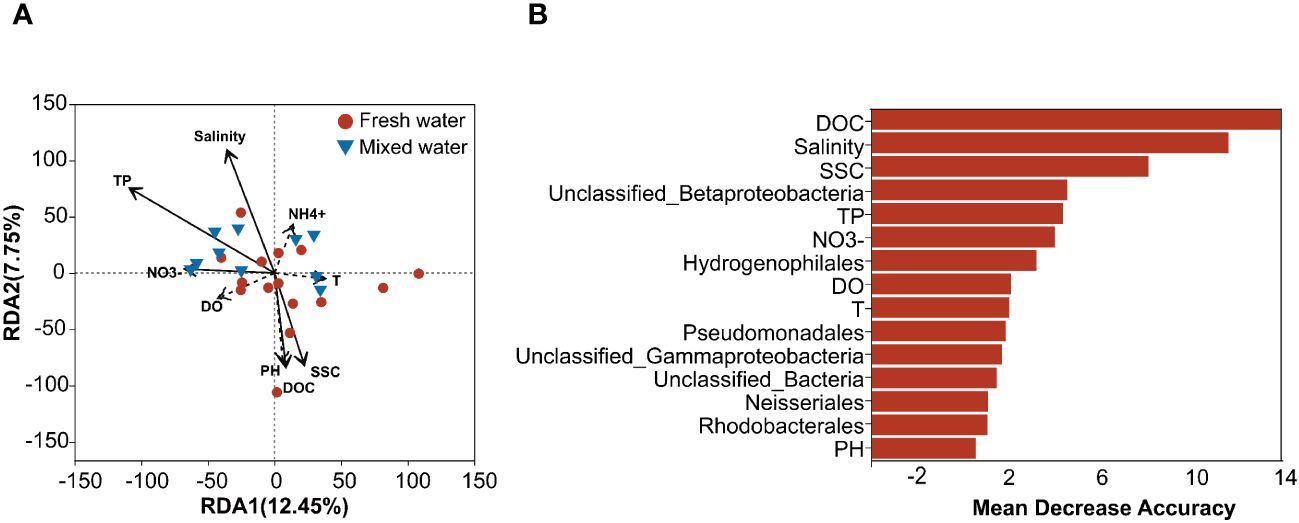
Figure 2 Influence of environmental factors, as shown by redundancy analysis (RDA) (A). The dashed arrows indicate that environmental factors did not significantly affect the dissimilarity of nirS-denitrifier communities at the OTU level. (B) Contributions of water properties to the dissimilarities of microbial communities and differences in the relative abundances of denitrifier microbial phyla based on random forest models. Environmental and taxonomic variables with an importance index (Mean Decrease Accuracy) greater than 0 were retained. NO3−, NH4+, PH and T represent NO3−-N, NH4+-N, pH and temperature, respectively. DOC, Dissolved organic carbon; SSC, Suspended sediment concentration; DO, Dissolved oxygen; TP, Total phosphorus; T, Temperature.
The results revealed that the abundance of nirS genes in denitrifying bacteria was significantly related to salinity and DOC (R = 0.496, P = 0.0012; R = 0.254, P < 0.001). In addition, DOC, SSC, NH4+-N, NO3–N, and DO were the major factors affecting the abundance of denitrifying genes in sediments (P < 0.05). The relation between dominant denitrifier orders and environmental variables was further explored, and the results showed that most predominant orders were significantly related to all measured environmental variables (Supplementary Figure S4). Judging from these connections, the nirS denitrifier orders mostly accumulated into three groups and revealed varying relationship patterns. For instance, denitrifier orders belonging to Rhodocyclales, Hydrogenophilales, and Burkholderiales (group 1) were significantly correlated with nutrient content, including water NO3−-N, TP, and NH4+-N. Additionally, denitrifiers belonging to Pseudomonadales, Unclassified_Gammaproteobacteria (UGP), Xanthomonadales, Neisseriales, Rhodospirillales, Norank_Bacteria, NES, and UBP (group 2) were positively correlated with water DOC, pH, and SSC values and negatively related to salinity and DO. In addition, denitrifier orders belonging to Unclassified_Kingdom, Rhodobacterales, UB, and UP (group 3) were weakly correlated with the water environmental factors, except for temperature, which was significantly negatively correlated with the relative abundances of Rhodobacterales (Supplementaryray Figure S4).
3.3 Community assembly processes and co-occurrence patterns
The dissimilarity of RCbray distances and pairwise βNTI among sampling sites revealed that assembly of the nirS-harboring denitrifier community was more deterministic in fresh water and moved toward stochastic in mixed water, with UN and DL processes fractions becoming more dominant (Figures 3A, B). HS process was the most important process, accounting for 57.50% of the community variation in freshwater samples, followed by DL and UN, each of which explained 29.17% and 13.33% of the total variation, respectively. While community assembly in mixed water samples was primarily structured by stochastic processes, the DL, UN, and HD processes accounted for ~97% of the total community variation. Notably, the contribution of the HD process to the community variation of denitrified microbes was 0 in the freshwater region, while there was a significant contribution (14.55%) in the mixed-water region. Furthermore, HeS processes did not appear in either the freshwater region or the mixed region, accounting for 0% of the total community variation (Figure 3C).
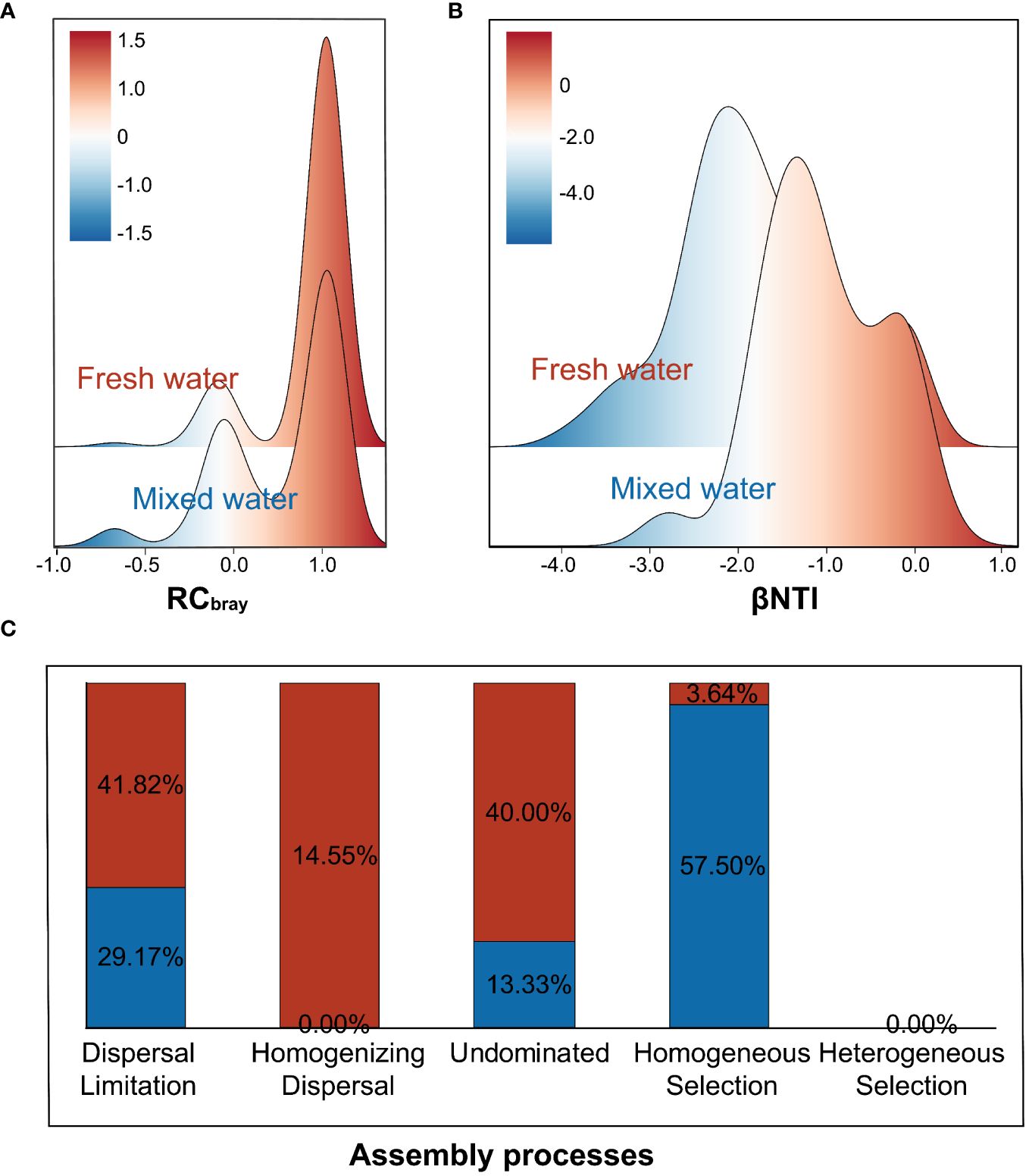
Figure 3 Distribution of standardized taxonomic turnover (RCbray; A), phylogenetic turnover (βNTI; B), and the relative contribution of different ecological processes (C) of the nirS-denitrifier communities in fresh water and mixed water, the columns colors by red and blue represent the freshwater and mixed-water regions, respectively.
The network analysis revealed that the co-occurrence relationships of nirS-denitrifier communities in freshwater regions were composed of 644 OTUs and 14903 edges (OTUs were closely associated). There were only 355 OTUs and 9616 edges in the co-occurrence network of nirS-denitrifier communities in the mixed area. Relationships between nodes showed higher positive correlations in both freshwater and mixed zones, suggesting that symbiosis was higher than exclusion between nirS-denitrifier communities. Additionally, an obviously higher number of positive correlations were identified in freshwater sample networks (85.18% of total edges) than in mixed-water networks (69.90% of total edges). Compared with the co-occurrence pattern of denitrifying communities in freshwater areas, nirS denitrifiers in mixed areas showed weaker network complexity and connectivity (Figure 4, Table 1). The network topology indexes of nirS-denitrifier communities in freshwater regions, including degree, network diameter (ND), average path length (APL), graph density (GD), and clustering coefficient (CC), were significantly larger than those in the mixed regions, while the modularity coefficient was significantly smaller, indicating that the denitrifying microbiome in the mixed region showed higher OTU modularity.
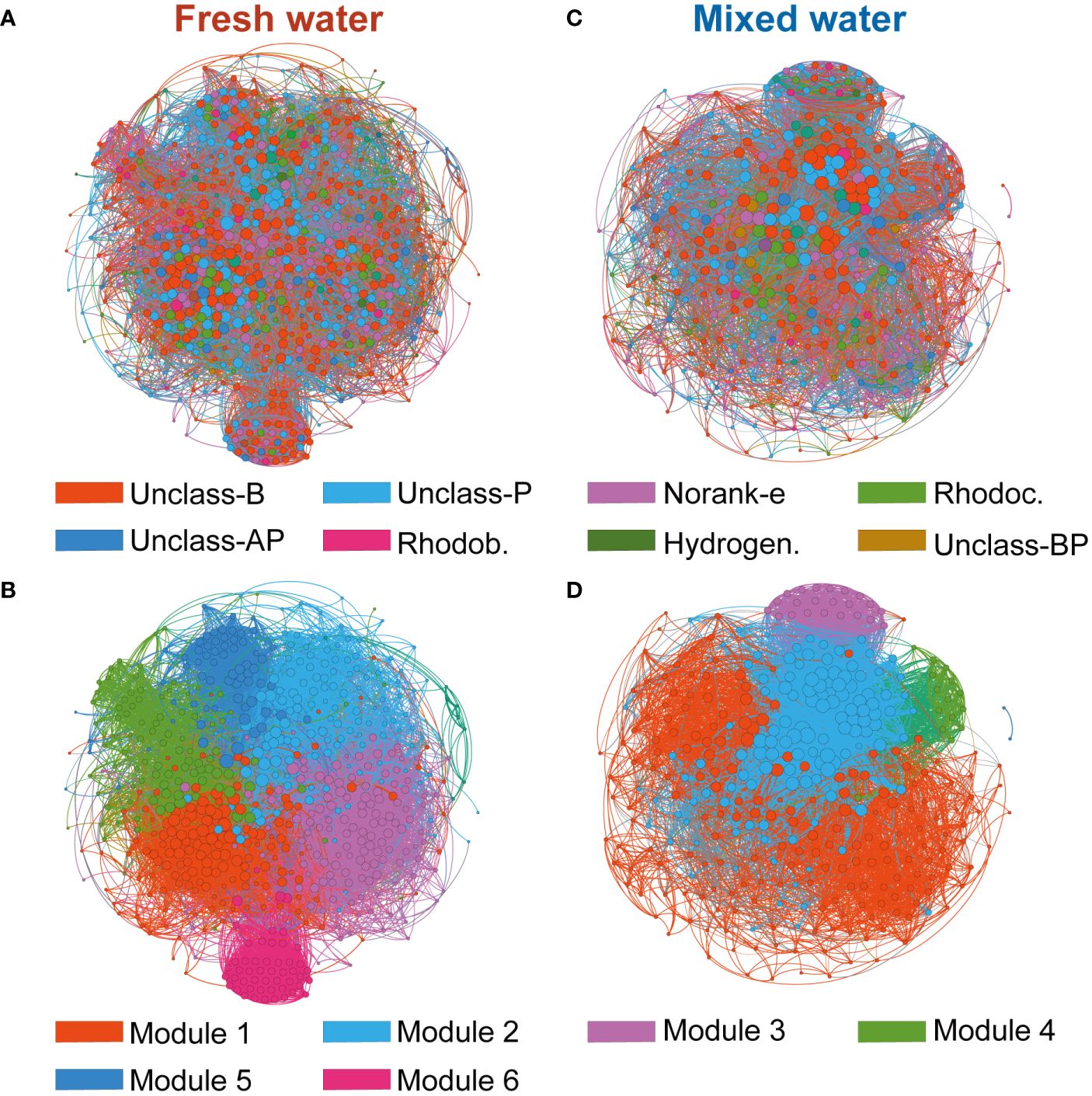
Figure 4 Co-occurrence networks of the nirS-denitrifier microbial community based on pairwise Spearman’s correlations between OTUs. Each shown connection has a correlation coefficient >|0.67| and a P value < 0.05. The size of each node is proportional to the number of connections. Left panel shows the network of freshwater samples with OTUs coloured by taxonomy (A) and modularity (B), and the right panel shows the network of mixed water samples with OTUs coloured by taxonomy (C) and modularity (D). Unclass-B: Unclassified_Bacteria; Unclass-P: Unclassified_Proteobacteria; Norank-e: Norank_environmental samples; Rhodoc, Rhodocyclales; Unclass-AP, Unclassified_Alphaproteobacteria; Rhodob, Rhodobacterales; Hydrogen, Hydrogenophilales; Unclass-BP, Betaproteobacteria.

Table 1 The network-level properties of the nirS-denitrifier microbial community cooccurrence patterns.
3.4 Potential functional characteristics of denitrification
Metagenome prediction generated large gene numbers in each dataset, ranging from 239,712,289 to 483,683,208 copies. A total of 432,409,0 nonredundant genes were detected in the metagenomes, and 8429 KOs were annotated. These KOs fell into higher KEGG categories (Supplementaryray Figure S5) and showed a high frequency of sequences enriched in fundamental features: global and overview maps (512,037,6 reads), amino acid metabolism (461,610,4 reads), and carbohydrate metabolism (458,562,0 reads).
Only genes involved in N metabolism and the denitrification process are discussed for simplicity. For N metabolism, 46 KOs were annotated to N metabolism, which covered most of the entire N metabolic pathway (Supplementaryray Figure S6). Annotation in the metagenome dataset showed the gene dominance included in the coupling between glutamate metabolism, with a high occurrence of glutamine synthetase (glnA), glutamate synthase large chain (gltB), small chain (gltD), glutamate dehydrogenase (GDH2) and carbonic anhydrase (cynT) (ranking first to fifth). In addition, the high occurrence of nitrate reductase (narG), glutamate dehydrogenase (gdhA) (6th and 7th), and transporters (NRT2, 8th) was also discovered, which showed large genomic potential for nitrite/nitrate transformation. N transformation was also associated with genes annotated to N dissimilatory/assimilatory nitrate reduction to ammonium (DNRA and ANRA), denitrification, fixation, anammox, and nitrification. Here, we found a high frequency of sequences linked to the N-removal process involved in denitrification, followed by dissimilatory nitrate reduction to ammonium (DNRA). The relative abundance of functional genes included in denitrification also differed between sampling zones, with the highest salinity region of zone 3 harboring more denitrification genes than other sampling zones (Figure 5B).
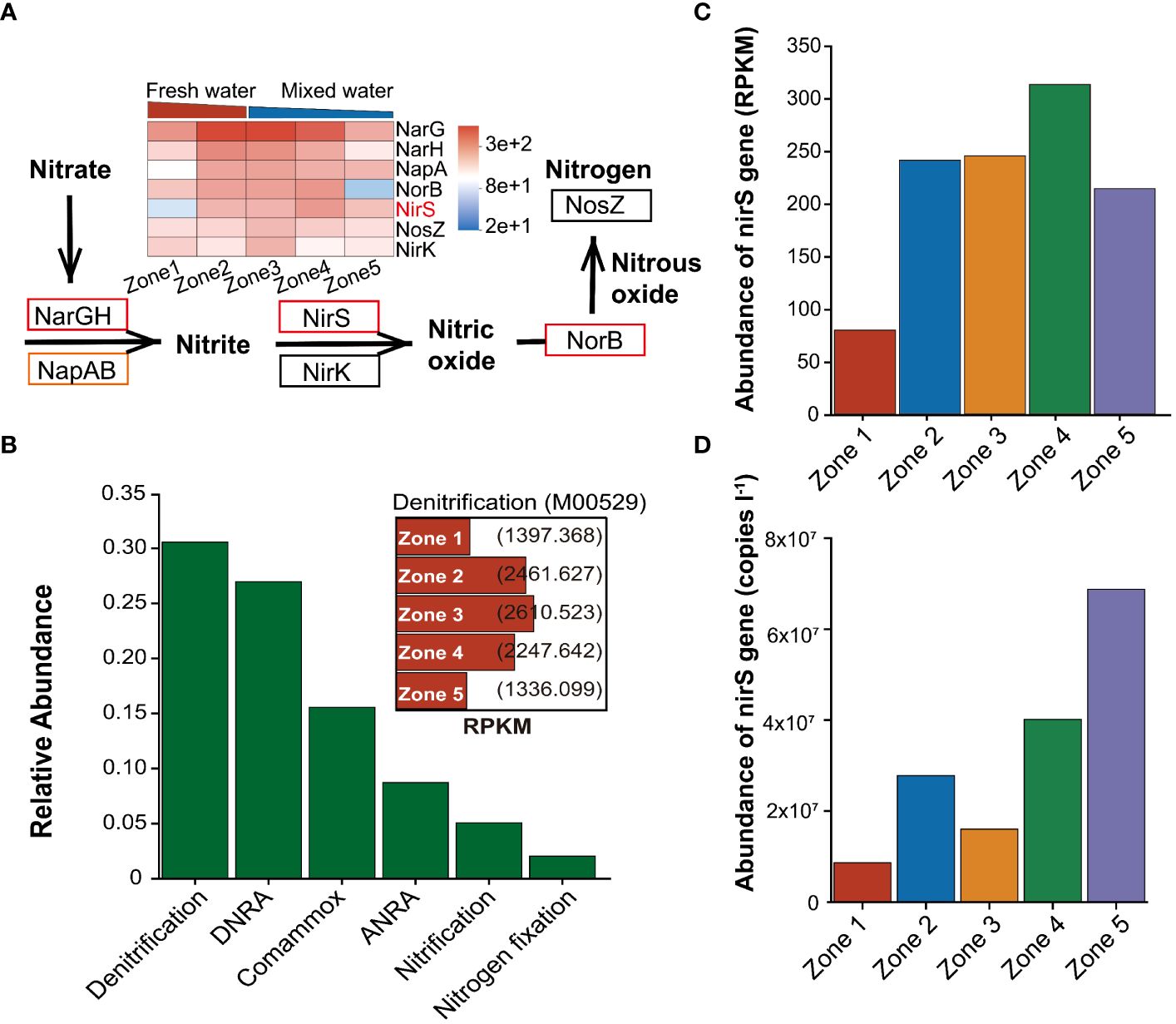
Figure 5 Metagenomic sequencing and qPCR were used to characterise the denitrification function. (A) Relative abundance of key functional genes and metabolic pathways involved in denitrification. The genes significant more abundant in the freshwater region are marked in red, and those more abundant in the mixed-water region are marked in orange. (B) N metabolism and denitrification function (shown in the box) in Pearl River Estuary waters across all samples. (C) Relative abundance and (D) gene copy number of nirS genes from Zone 1 to Zone 5, based on shotgun sequencing and qPCR results, respectively. RPKM: reads per kilobase of exon model per million mapped reads. DNRA, Dissimilatory nitrate reduction to ammonium; ANRA, Assimilatory nitrate reduction to ammonium; RPKM, Reads Per Kilobase per Million mapped reads.
For functional genes in denitrification, the relative abundance of the major functional genes that mediate denitrification, including narG/H, napA/B, nirS, and norB, showed significant variations across salinity regions. The relative abundance of narG/H, nirS, and norB genes was significantly higher in the freshwater region (ANOVA, P < 0.01), while the relative abundance of napA/B genes was significantly higher in the mixed-water regions (ANOVA, P < 0.01). However, the relative abundances of the nirK and nosZ genes were not obviously different between within five different salinity gradients (Figure 5A). Furthermore, whether based on metagenomic gene sets or qPCR results, the results demonstrated that the nirS gene abundance in mixed-water regions was significantly higher than that in low-salinity regions (Figures 5C, D). This further implied a significant regulation of nirS gene abundance by water salinity.
3.5 Links between denitrifying microbial biodiversity and abundances and environmental variables
As illustrated by the concrete arrows in Figure 6. The nirS gene abundance was directly positively related to salinity and negatively related to SSC and ACE. The nirS-denitrifier dissimilarities were weakly directly related to salinity and more indirectly regulated by environmental variables and microbial α-diversity (Figures 6A, B). To better exhibit and illustrate the impact of microbial α-diversity on denitrification function, as well as the abundance and dissimilarity in nirS-type denitrifiers, models with only environmental variables or microbial α-diversity were compared. The SSC and phylogenetic diversity index significantly influenced nirS-denitrifier dissimilarities; however, the correlation with salinity was not significant. Similar to the model without the microbial α-diversity index, salinity was a key regulatory factor affecting nirS denitrifier abundances. The denitrification function was negatively correlated with nirS-denitrifier dissimilarities and gene abundance. Additionally, salinity can indirectly influence denitrification function via reductions in the SSC and ACE index. In addition, the SEM including microbial community diversity enhanced the predictability of nirS gene spatial dissimilarity and the potential function in denitrification. When replacing environmental factors (Figure 6A) with the microbial α-diversity index (Figure 6B), the total variance of nirS gene dissimilarity explained by the fixed factors improved from 0.470 to 0.527. Additionally, the total variance of the denitrification potential improved by ~ 2 times (R2 from 0.314 to 0.615). The differences between these two models implied the potential contribution of microbial community characteristics to denitrification processes in the PRE ecosystem.
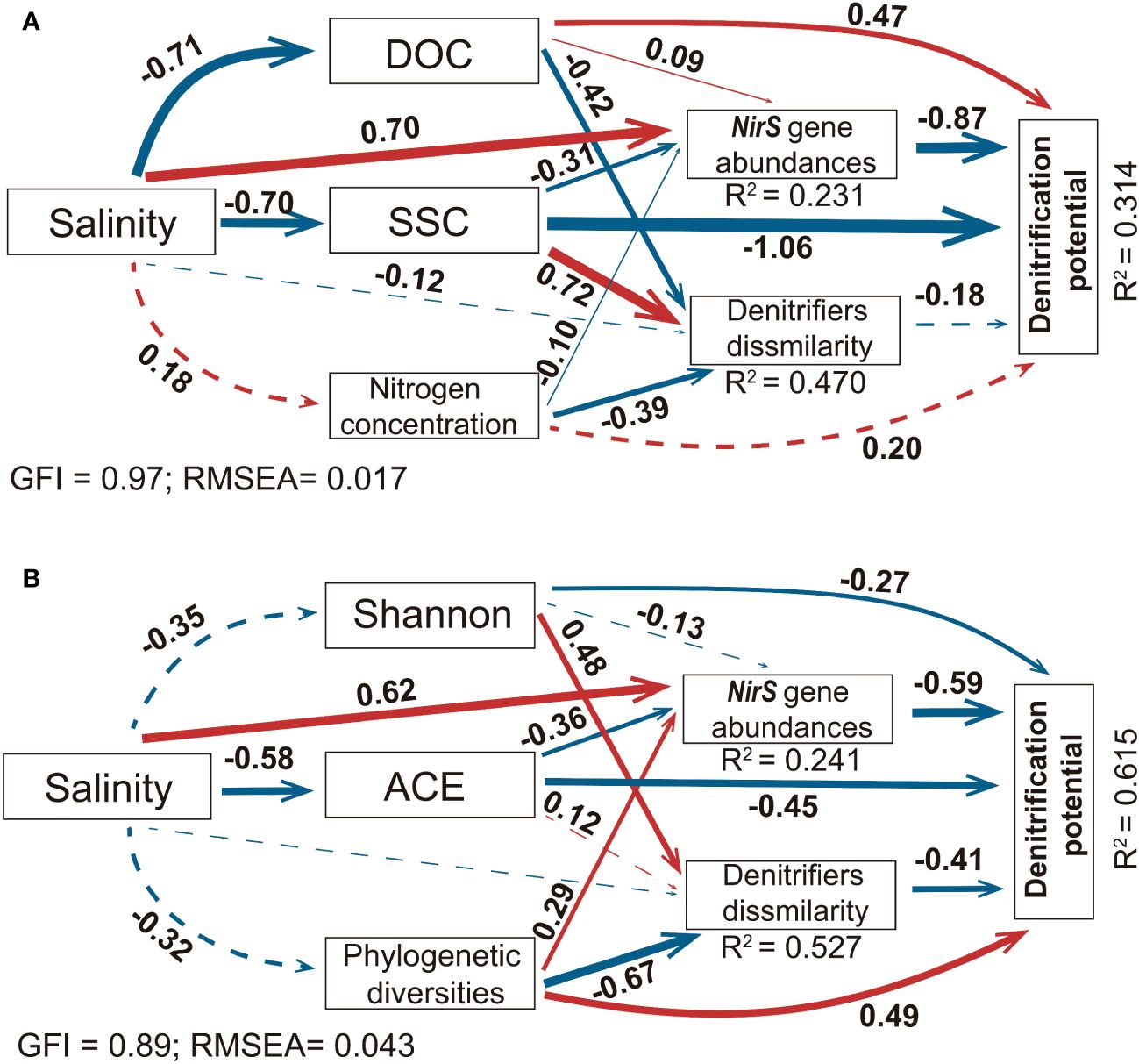
Figure 6 Structural equation model describing the effects of salinity, dissolved organic carbon (DOM), suspended sediment concentration (SSC), dissolved oxygen (DO), N concentration (NH4+ and NO3−), and microbial characteristics (Shannon, ACD, and phylogenetic diversity index) on the abundance of the nirS gene, denitrifier β-diversity and denitrification potential. (A) Conceptual model without microbial characteristics (Shannon, ACD, and phylogenetic diversity index). (B) Conceptual model without environmental characteristics (SSCM, DOC, and N concentration). Red lines indicate positive relations, while blue lines indicate negative relations. Solid lines indicate significant and stable correlations (P < 0.05), and dashed lines indicate nonsignificant correlations (P ≥ 0.05). Numbers next to the arrow lines show standardized path coefficients. Arrow widths are proportional to the strength of the relationship.
4 Discussion
The study of the distribution pattern, community assembly, and bio-interaction of its functional microbial community structure is extremely important for revealing and predicting the N cycling process and response mechanism of the estuarine water ecological environment. Here, we explored the diversity, abundance, community assembly processes, and co-occurrence pattern of nirS-denitrifying bacteria and the denitrification potential in typical estuarine waters. Additionally, we analyzed the key regulatory role of the water environmental gradient on their community ecological characteristics and community abundance, providing new insights into the microbial driving mechanism of the denitrification process in estuaries and marine waters.
The results revealed that the diversity index and richness index of nirS denitrifiers in freshwater environments significantly exceeded those in mixed-water areas, suggesting that salinity in this study area plays a crucial role in regulating denitrification bacteria. High salinity reduces bacterial diversity (Bernhard et al., 2005; Ruhl et al., 2018). It has been reported that nirS-harboring bacterial diversity is higher in lower salinity environments than in higher salinity environments (Zheng et al., 2015), which is consistent with our results. A high degree of salinity can impede bacterial development by limiting the activities of cell membranes and enzymes. Due to high salinity, low salt-tolerant bacteria must allocate more energy to combat high osmotic pressure, resulting in insufficient fecundity. In addition, if salt tolerance is lacking, microorganisms may perish due to cell vitality loss (Uygur, 2006; Chen et al., 2019). Studies have shown that Rhodanobacter and Pseudomonas are the major nirS denitrifiers in artificial environments (Wu et al., 2017). However, the results of this study showed that most denitrifying bacteria cannot be identified at the genus and phylum levels. At the phylum classification level, unclassified bacterial and nonranked environmental samples accounted for more than half of the nirS sequences in total, which in is agreement with the results of previous studies (Zhou et al., 2016; Jiang et al., 2020). Although the molecular method based on PCR amplification is the best method to study the nir community, noticeably, the coverage of the frequently applied nirS primers to the entire gene pool is low. A report by Ma et al. (2019) showed that the primer group cd3aF/R3cd could only account for 77.70% of nirS clade I. Therefore, in areas with less natural environment or human interference, it is still necessary to use metagenomics or culture-dependent methods to isolate and identify major denitrifying bacteria.
DOC and salinity in water are the key driving factors affecting the spatial variation in β diversity and abundance of the nirS denitrifier microbial community. As a carbon source, DOC can be directly utilized by microbial communities and provides essential energy and material for synthesizing extracellular and transport enzymes, significantly regulating the ecological and physiological characteristics of microbial communities (A. Hu et al., 2022). Furthermore, when denitrifying microbial communities are not subject to resource constraints or other environmental pressures, the abundance and diversity of denitrifying microorganisms may be limited by salinity. Salinity can influence the denitrifying microbial community’s species composition through various pathways, such as soluble salts, which typically increase the water’s osmotic potential and draw water from cells, which may decrease the activity of cells. Finally, certain microorganisms are killed through solute decomposition (Yang et al., 2016). Research results have shown that SSC in water also significantly impacts the abundance and spatial variation in denitrifying microorganisms, which may also be a crucial factor affecting the structure of the denitrifying community (Zheng et al., 2021). The elevated SSC would lead to flocculation and cause higher oxygen depletion next to the suspended sediment interface, thus supplying denitrifying bacteria with more microsites. Hence, SSC was crucial for shaping the abundance and dynamics of denitrifiers in the PRE-water system. In this research, although the results and previous studies demonstrate that the complex water environment significantly manipulates the diversity and abundance characteristics of denitrifying bacteria, environmental variables cannot explain a large portion of the community differences in nirS microbial community structures. The unexplained changes may result from unmeasured spatial and environmental elements in this research or by the random assembly process of microbial communities, such as dispersal limitations caused by sampling point distance and random drift within microbial communities (including genetic and ecological drift). These factors have been shown to significantly impact the composition of nirS denitrifier microorganisms in aquatic ecosystems (Jones and Hallin, 2010; Li et al., 2022). We showed that the samples from mixed-water regions, the stochastic process was overwhelming. In the present study, deep-sea sediments with relatively uniform environments (Jacob et al., 2013) allow stochastic processes to dominate community assembly. In systems with fewer environmental differences, stochastic processes may exceed deterministic processes (Wang et al., 2013; Jiao et al., 2020). In contrast, physical disturbances occur frequently at low salinity sites, resulting in a weak response to stochastic factors. It was found that the assembly of denitrifying microorganisms was mainly controlled by stochastic processes in freshwater environments. However, our findings demonstrated that the construction process of the denitrifying community in the freshwater area in the upper reaches of the Pearl River is mainly affected by the process of homogeneous selection, which may be because freshwater is more obviously affected by runoff, which contributes to the diffusion process of denitrifying bacteria (J. Liu et al., 2020; Zhang et al., 2023). Moreover, the source of denitrifying bacteria in the upper reaches of the Pearl River inshore estuary is more complex, and the water environment has been affected by the discharge of urban domestic and industrial sewage for a long time, which causes the environmental filtration of this area to be more obvious (M. Li et al., 2021).
The primary focus of microbial ecologists lies in the broader classification of microbial communities, encompassing fungi, bacteria, and archaea (Dassonville Fabrice, 2002; Newman, 2006; Veiga et al., 2010; Ma et al., 2016; Jiao et al., 2020; Lu et al., 2023), while their awareness regarding the functional communities of microorganisms remains relatively limited. In this study, the denitrifying bacterial communities in the freshwater region exhibited a higher number of positive correlations compared to those in the mixed-water region, indicating that the denitrifying bacteria in the freshwater region displayed stronger synergistic and symbiotic relationships. This, to some extent, might contribute to the complexity and stability of the denitrifying bacteria co-occurrence association (Liu et al., 2020). The complexity of the denitrifying bacterial network in freshwater regions exceeds that in mixed-water regions, suggesting that the interaction between denitrifying bacteria is enhanced by a higher selection process or lower diffusion restriction process in freshwater areas (Hernandez et al., 2021). Furthermore, the higher dispersal limitations of denitrifying microbial communities in mixed-water regions promoted a more clustered co-occurrence pattern of denitrifying communities (that is, with higher network module characteristics), while the enhancement of salinity or diffusion limitations reduced the network complexity of microbial communities, which was consistent with the distribution characteristics of low abundance, diversity, and phylogenetic diversity of denitrifying microorganisms in mixed-water regions. Since denitrifying bacteria are the key functional groups driving the N cycle process in water, it is essential to understand their potential functional characteristics and conduct a correlation analysis between their functional characteristics and diversity characteristics. However, the ability to directly understand the functional characteristics of microorganisms based on microbial ecological information is limited. At present, with the emergence of modern high-throughput technologies such as metagenomics, the acquisition of microbial ecology information is more rapid and accurate, making it easier to complete the above work. Furthermore, macro-genomics can expose the genetic foundation of microbial communities engaged in biogeochemical procedures and identify the impact of microbial communities on the ecological processes of estuarine ecosystems (Lu et al., 2014; Zhang et al., 2023). Similar to previous studies (Zheng et al., 2015; Zhang et al., 2020), there was a significant salinity gradient distribution in the functional profiles of microbial denitrification in the PRE. The results revealed that microbial communities had higher denitrification potential in mixed-water regions. SSC, DOC, salinity, nirS denitrifier abundances, and community similarity significantly impacted denitrification function (Figure 6A). Furthermore, by comparing the results of two SEMs with and without microbial community ecological and evolutionary data, we demonstrated the importance of microbial characteristics in driving denitrification function (Figure 6B), suggesting the potential relationship between the spatial heterogeneity of environmental factors or microbial ecological characteristics and the functional potential of denitrification microbial communities. Suspended sediments in water are the substrate of most bacterial activities (Muylaert and Sabbe, 1999). Suspended particles provide the necessary carbon source to increase the denitrification process of denitrifying bacteria by aerobic degradation of organic matter and oxygen diffusion intervention and create anoxic microniches for anaerobic community growth (Stoodley et al., 2002; Zhang et al., 2020). The tributaries of the Pearl River basin and the cities along the Pearl River may change the N input and water environmental factors. However, it was difficult to incorporate and analyze the changes in the water environment quickly and accurately in SEM, which was a possible explanation for the low regression factor in the models. Due to the corresponding lag of microbial communities to the environment, the biological characteristics of functional microbial groups in water (such as nirS denitrifier groups) may be the key indicators in predicting the functional characteristics of denitrification.
Our study provides new insights and detailed data for evaluating carbon and N fluxes and ecological health in this region in the future. Which have important implications and provide new information for predicting microbial responses to anthropogenic activities and global climate change. Additionally, it should still be noted that coastal areas are often subject to periodic and occasional environmental disturbances due to typhoons and other large storms (Ward et al., 2017), which critically alter environmental factors and increase nutrient inputs from coastal areas to the open ocean. Therefore, it is still necessary to conduct periodic and long-term spatiotemporal surveys to clarify the major driving factors of microbial distribution and the biogeochemical cycle in the estuary.
Data availability statement
The datasets presented in this study can be found in online repositories. The names of the repository/repositories and accession number(s) can be found below: https://www.ncbi.nlm.nih.gov/bioproject/PRJNA1006209/.
Author contributions
HoZ: Writing – original draft. HaoZ: Data curation, Investigation, Software, Writing – original draft. XH: Formal Analysis, Methodology, Writing – review & editing. CL: Investigation, Software, Writing – review & editing. F: Formal Analysis, Visualization, Writing – review & editing. QC: Writing – review & editing. HY: Writing – review & editing. CZ: Formal Analysis, Investigation, Writing – review & editing. HanZ: Writing – review & editing. HH: Writing – review & editing. ZZ: Writing – review & editing, Writing – original draft.
Funding
The author(s) declare that financial support was received for the research, authorship, and/or publication of this article. This work was jointly supported by the National Natural Science Foundation of China (82072802), the Shandong Provincial Natural Science Foundation (ZR2023QD103 and ZR2020QC052), the Youth Innovation Support Program of Shandong Universities (2021KJ081). It was also funded by the Introduction and Cultivation Plan of Youth Innovative Talent in Shandong Province Colleges, the Research and Innovation Team of Coastal Wetland Ecological Protection and Restoration in the Yellow River Delta, the Key Scientific and Technological Projects in Henan province (212102310630), the Backbone Teachers Program of Sanquan College of Xinxiang Medical University (SQ2023GGJS03), and Academic Technology Leader Program of Sanquan College of Xinxiang Medical College (SQ2023XSJSDTR01).
Conflict of interest
The authors declare that the research was conducted in the absence of any commercial or financial relationships that could be construed as a potential conflict of interest.
Publisher’s note
All claims expressed in this article are solely those of the authors and do not necessarily represent those of their affiliated organizations, or those of the publisher, the editors and the reviewers. Any product that may be evaluated in this article, or claim that may be made by its manufacturer, is not guaranteed or endorsed by the publisher.
Supplementary material
The Supplementary Material for this article can be found online at: https://www.frontiersin.org/articles/10.3389/fmars.2024.1328684/full#supplementary-material
References
Bernhard A. E., Donn T., Giblin A. E., Stahl D. A. (2005). Loss of diversity of ammonia-oxidizing bacteria correlates with increasing salinity in an estuary system. Environ. Microbiol. 7, 1289–1297. doi: 10.1111/j.1462-2920.2005.00808.x
Bolyen E., Rideout J. R., Dillon M. R., Caporaso J. G. (2019). Reproducible, interactive, scalable and extensible microbiome data science using QIIME 2. Nat. Biotechnol. 37, 852–857. doi: 10.1038/s41587-019-0209-9
Braker G., Zhou J., Wu L., Devol A. H., Tiedje J. M. (2000). Nitrite reductase genes (nirK and nirS) as functional markers to investigate diversity of denitrifying bacteria in pacific northwest marine sediment communities. Appl. Environ. Microbiol. 66, 2096–2104. doi: 10.1128/AEM.66.5.2096-2104.2000
Buchfink B., Xie C., Huson D. (2015). Fast and sensitive protein alignment using DIAMOND. Nat. Methods 12, 59–60. doi: 10.1038/nmeth.3176
Cai M. H., Luo G., Li J., Li W. T., Li Y., Li A. M. (2021). Substrate competition and microbial function in sulfate-reducing internal circulation anaerobic reactor in the presence of nitrate. Chemosphere 280, 130937. doi: 10.1016/j.chemosphere.2021.130937
Cantera J. J. L., Stein L. Y. (2007). Molecular diversity of nitrite reductase genes (nirK) in nitrifying bacteria. Environ. Microbiol. 9, 765–776. doi: 10.1111/j.1462-2920.2006.01198.x
Chen D., Wang Z., Zhang M., Wang X., Lu S. (2019). Effect of increasing salinity and low C/N ratio on the performance and microbial community of a sequencing batch reactor. Environ. Technol. (United Kingdom) 42, 1213–1224. doi: 10.1080/09593330.2019.1660417
Coyne M. S., Arunakumari A., Averill B. A., Tiedje J. M. (1989). Immunological identification and distribution of dissimilatory heme cd1 and nonheme copper nitrite reductases in denitrifying bacteria. Appl. Environ. Microbiol. 55, 2924–2931. doi: 10.1128/aem.55.11.2924-2931.1989
Dassonville Fabrice P. R. (2002). Interactions between microbial processes and geochemical transformations under anaerobic conditions: a review. Agronomie 22, 51–68. doi: 10.1051/agro:2001001
Edgar R. C. (2013). UPARSE: Highly accurate OTU sequences from microbial amplicon reads. Nat. Methods 10, 996–998. doi: 10.1038/nmeth.2604
Falkowski P. G., Fenchel T., Delong E. F. (2008). The microbial engines that drive earth’s biogeochemical cycles. Science 80-.). 320, 1034–1039. doi: 10.1126/science.1153213
Faust K., Raes J. (2012). Microbial interactions : from networks to models. Nat. Publ. Gr. 10, 538–550. doi: 10.1038/nrmicro2832
Gao J., Hou L., Zheng Y., Liu M., Yin G., Li X., et al. (2016). nirS-Encoding denitrifier community composition, distribution, and abundance along the coastal wetlands of China. Appl. Microbiol. Biotechnol. 100, 8573–8582. doi: 10.1007/s00253-016-7659-5
Guo X., Su J., Guo L., Liu Z., Yang W., Li Y., et al. (2023). Coupling of carbon and oxygen in the pearl river plume in summer: upwelling, hypoxia, reoxygenation and enhanced acidification. JGR Oceans. 128, e2022JC019326. doi: 10.1029/2022JC019326
Hernandez D. J., David A. S., Menges E. S., Searcy C. A., Afkhami M. E. (2021). Environmental stress destabilizes microbial networks. ISME J. 15, 1722–1734. doi: 10.1038/s41396-020-00882-x
Hou L., Zheng Y., Liu M., Li X., Lin X., Yin G., et al. (2015). Anaerobic ammonium oxidation and its contribution to nitrogen removal in China’s coastal wetlands. Sci. Rep. 5, 1–11. doi: 10.1038/srep15621
Howarth R. W., Marino R. (2006). Nitrogen as the limiting nutrient for eutrophication in coastal marine ecosystems: Evolving views over three decades. Limnol. Oceanogr. 51, 364–376. doi: 10.4319/lo.2006.51.1_part_2.0364
Hu A., Choi M., Tanentzap A. J., Liu J., Jang K. S., Lennon J. T., et al. (2022). Ecological networks of dissolved organic matter and microorganisms under global change. Nat. Commun. 13, 1–15. doi: 10.1038/s41467-022-31251-1
Hu X., Gu H., Liu J., Liu Z., Li L., Du S., et al. (2022). Biogeographic distribution patterns and assembly processes of nirS-type and nirK-type denitrifiers across the black soil zone in Northeast China. Soil Sci. Soc Am. J. 86, 1383–1396. doi: 10.1002/saj2.20345
Huang F., Lin X., Hu W., Zeng F., He L., Yin K. (2021). Catena Nitrogen cycling processes in sediments of the Pearl River Estuary : Spatial variations, controlling factors, and environmental implications. Catena 206, 105545. doi: 10.1016/j.catena.2021.105545
Jacob M., Soltwedel T., Boetius A., Ramette A. (2013). Biogeography of Deep-sea benthic bacteria at regional scale (LTER HAUSGARTEN, Fram Strait, Arctic). PloS One 8, e72779. doi: 10.1371/journal.pone.0072779
Jiang X., Liu W., Yao L., Liu G., Yang Y. (2020). The roles of environmental variation and spatial distance in explaining diversity and biogeography of soil denitrifying communities in remote Tibetan wetlands. FEMS Microbiol. Ecol. 96, 1–11. doi: 10.1093/FEMSEC/FIAA063
Jiao S., Yang Y., Xu Y., Zhang J., Lu Y. (2020). Balance between community assembly processes mediates species coexistence in agricultural soil microbiomes across eastern China. ISME J. 14, 202–216. doi: 10.1038/s41396-019-0522-9
Jones C. M., Hallin S. (2010). Ecological and evolutionary factors underlying global and local assembly of denitrifier communities. ISME J. 4, 633–641. doi: 10.1038/ismej.2009.152
Jørgensen B. B., Findlay A. J., Pellerin A. (2019). The biogeochemical sulfur cycle of marine sediments. Front. Microbiol. 10. doi: 10.3389/fmicb.2019.00849
Kou Y., Liu Y., Li J., Li C., Tu B., Yao M., et al. (2021). Patterns and Drivers of nirK -Type and nirS -Type Denitrifier Community Assembly along an Elevation Gradient. mSystems 6, e00667–e00621. doi: 10.1128/mSystems.00667-21
Lafuente A., Bowker M. A., Delgado-Baquerizo M., Durán J., Singh B. K., Maestre F. T. (2019). Global drivers of methane oxidation and denitrifying gene distribution in drylands. Glob. Ecol. Biogeogr. 28, 1230–1243. doi: 10.1111/geb.12928
Li Y., Jin H., Chen J., Wang D., Yang Z., Wang B., et al. (2021). Nitrogen removal through sediment denitrification in the Yangtze Estuary and its adjacent East China Sea: A nitrate limited process during summertime. Sci. Total Environ. 795, 148616. doi: 10.1016/j.scitotenv.2021.148616
Li M., Mi T., He H., Chen Y., Zhen Y., Yu Z. (2021). Active bacterial and archaeal communities in coastal sediments: Biogeography pattern, assembly process and co-occurrence relationship. Sci. Total Environ. 750, 142252. doi: 10.1016/j.scitotenv.2020.142252
Li G., Yan D., Xia P., Cao H., Lin T., Yi Y. (2022). Community structure and assembly of denitrifying bacteria in epiphytic biofilms in a freshwater lake ecosystem. J. Oceanol. Limnol. 40, 1039–1050. doi: 10.1007/s00343-021-1084-z
Li W., Godzik A. (2006). Cd-hit: a fast program for clustering and comparing large sets of protein or nucleotide sequences. Bioinformatics 22, 1658–1659. doi: 10.1093/bioinformatics/btl158
Liu X., Wu J., Hong Y., Jiao L., Li Y., Wang L., et al. (2020). Nitrogen loss by nirS-type denitrifying bacterial communities in eutrophic coastal sediments. Int. Biodeterior. Biodegrad. 150, 104955. doi: 10.1016/j.ibiod.2020.104955
Liu J., Zhu S., Liu X., Yao P., Ge T., Zhang X. H. (2020). Spatiotemporal dynamics of the archaeal community in coastal sediments: assembly process and co-occurrence relationship. ISME J. 14, 1463–1478. doi: 10.1038/s41396-020-0621-7
Lu H., Chandran K., Stensel D. (2014). Microbial ecology of denitrification in biological wastewater treatment. Water Res. 64, 237–254. doi: 10.1016/j.watres.2014.06.042
Lu T., Xu N., Lei C., Zhang Q., Zhang Z., Sun L., et al. (2023). Bacterial biogeography in China and its association to land use and soil organic carbon. Soil Ecol. Lett. 5, 230172. doi: 10.1007/s42832-023-0172-8
Ma B., Wang H., Dsouza M., Lou J., He Y., Dai Z., et al. (2016). Geographic patterns of co-occurrence network topological features for soil microbiota at continental scale in eastern China. ISME J. 10, 1891–1901. doi: 10.1038/ismej.2015.261
Ma Y., Zilles J. L., Kent A. D. (2019). An evaluation of primers for detecting denitrifiers via their functional genes. Environ. Microbiol. 21, 1196–1210. doi: 10.1111/1462-2920.14555
Muylaert K., Sabbe K. (1999). Spring phytoplankton assemblages in and around the maximum turbidity zone of the estuaries of the Elbe (Germany), the Schelde (Belgium/The Netherlands) and the Gironde (France). J. Mar. Syst. 22, 133–149. doi: 10.1016/S0924-7963(99)00037-8
Newman M. E. J. (2006). Modularity and community structure in networks. Proc. Natl. Acad. Sci. U. S. A. 103, 8577–8582. doi: 10.1073/pnas.0601602103
Niu L., Luo X., Hu S., Liu F., Cai H., Ren L., et al. (2020a). Impact of anthropogenic forcing on the environmental controls of phytoplankton dynamics between 1974 and 2017 in the Pearl River estuary, China. Ecol. Indic. 116, 106484. doi: 10.1016/j.ecolind.2020.106484
Niu L., van Gelder P., Luo X., Cai H., Zhang T., Yang Q. (2020b). Implications of nutrient enrichment and related environmental impacts in the pearl river estuary, China: Characterizing the seasonal influence of riverine input. Water (Switzerland) 12. doi: 10.3390/w12113245
Rasigraf O., Schmitt J., Jetten M. S. M., Lüke C. (2017). Metagenomic potential for and diversity of N-cycle driving microorganisms in the Bothnian Sea sediment. Microbiologyopen 6, 1–13. doi: 10.1002/mbo3.475
Ren Y., Yu G., Shi C., Liu L., Guo Q. (2022). Majorbio Cloud : A one - stop, comprehensive bioinformatic platform for multiomics analyses. iMeta 1, 1–7. doi: 10.1002/imt2.12
Ruhl I. A., Grasby S. E., Haupt E. S., Dunfield P. F. (2018). Analysis of microbial communities in natural halite springs reveals a domain-dependent relationship of species diversity to osmotic stress. Environ. Microbiol. Rep. 10, 695–703. doi: 10.1111/1758-2229.12695
Seiki T., Date E., Okada M. (2008). Denitrification and nitrogen fixation rates at tidal flats and coastal bottom areas. Nippon Suisan Gakkaishi (Japanese Ed. 74, 678–687. doi: 10.2331/suisan.74.678
Shi R., Huang H., Qi Z., Han T. (2019). Distribution Patterns of nirS-Encoding and nirK-Encoding Denitrifiers in the Surface Sediment of the Pearl River Estuary. Russ. J. Mar. Biol. 45, 453–463. doi: 10.1134/S1063074019060099
Stegen J. C., Lin X., Fredrickson J. K., Chen X., Kennedy D. W., Murray C. J., et al. (2013). Quantifying community assembly processes and identifying features that impose them. ISME J. 7, 2069–2079. doi: 10.1038/ismej.2013.93
Stegen J. C., Lin X., Fredrickson J. K., Konopka A. E. (2015). Estimating and mapping ecological processes influencing microbial community assembly. Front. Microbiol. 6. doi: 10.3389/fmicb.2015.00370
Stoodley P., Sauer K., Davies D. G., Costerton J. W. (2002). Biofilms as complex differentiated communities. Annu. Rev. Microbiol. 56, 187–209. doi: 10.1146/annurev.micro.56.012302.160705
Su X., Cui L., Tang Y., Wen T., Yang K., Wang Y., et al. (2022). Denitrification and N2O emission in estuarine sediments in response to ocean acidification: from process to mechanism. Environ. Sci. Technol. 56, 14828–14839. doi: 10.1021/acs.est.2c03550
Uygur A. (2006). Specific nutrient removal rates in saline wastewater treatment using sequencing batch reactor. Process Biochem. 41, 61–66. doi: 10.1016/j.procbio.2005.03.068
Veiga D. F. T., Dutta B., Balázsi G. (2010). Network inference and network response identification: Moving genome-scale data to the next level of biological discovery. Mol. Biosyst. 6, 469–480. doi: 10.1039/b916989j
Wang F., Chen N., Yan J., Lin J., Guo W., Cheng P., et al. (2019). Major processes shaping mangroves as inorganic nitrogen sources or sinks: insights from a multidisciplinary study. J. Geophys. Res. Biogeosciences 124, 1194–1208. doi: 10.1029/2018JG004875
Wang H., Li J., Wang B., Chen G. (2020). Deciphering pollutants removal mechanisms and genetic responses to ampicillin stress in simultaneous heterotrophic nitrification and aerobic denitrification (SHNAD) process treating seawater-based wastewater. Bioresour. Technol. 315, 123827. doi: 10.1016/j.biortech.2020.123827
Wang J., Shen J., Wu Y., Tu C., Soininen J., Stegen J. C., et al. (2013). Phylogenetic beta diversity in bacterial assemblages across ecosystems: Deterministic versus stochastic processes. ISME J. 7, 1310–1321. doi: 10.1038/ismej.2013.30
Ward C. S., Yung C. M., Davis K. M., Blinebry S. K., Williams T. C., Johnson Z. I., et al. (2017). Annual community patterns are driven by seasonal switching between closely related marine bacteria. ISME J. 11, 1412–1422. doi: 10.1038/ismej.2017.4
Wong S. C., Li X. D., Zhang G., Qi S. H., Min Y. S. (2002). Heavy metals in agricultural soils of the Pearl River Delta, South China. Environ. pollut. 119, 33–44. doi: 10.1016/S0269-7491(01)00325-6
Wu H., Wang X., He X., Zhang S., Liang R., Shen J. (2017). Effects of root exudates on denitrifier gene abundance, community structure and activity in a micro-polluted constructed wetland. Sci. Total Environ. 598, 697–703. doi: 10.1016/j.scitotenv.2017.04.150
Xia W., Zhou Z. (2021). Analysis of meteorological and hydrological elements in the sea around the pearl river estuary based on observed data in summer. Trans. Oceanol. Limnol. 43, 60–65. doi: 10.13984/j.cnki.cn37-1141.2021.05.008
Yang J., Ma L., Jiang H., Wu G., Dong H. (2016). Salinity shapes microbial diversity and community structure in surface sediments of the Qinghai-Tibetan Lakes. Sci. Rep. 6, 6–11. doi: 10.1038/srep25078
Yu C. Q., Huang X., Chen H., Godfray H. C. J., Wright J. S., Hall J. W., et al. (2019). Managing nitrogen to restore water quality in China. Nature 567, 516–520. doi: 10.1038/s41586-019-1001-1
Zeng D., Niu L., Tao W., Fu L., Yang Q. (2020). Nutrient dynamics in pearl river estuary and their eutrophication evaluation. J. Guangdong Ocean Univ. 40, 73–82. doi: 10.3969/j.issn.1673-9159.2020.03.010
Zhang Z., Guo Y., Guo L., Hu F., Zhao Y., Jin C., et al. (2020). Elucidating salinity adaptation and shock loading on denitrification performance: Focusing on microbial community shift and carbon source evaluation. Bioresour. Technol. 305, 123030. doi: 10.1016/j.biortech.2020.123030
Zhang Z., Han P., Zheng Y., Jiao S., Dong H., Liang X., et al. (2023). Spatiotemporal dynamics of bacterial taxonomic and functional profiles in estuarine intertidal soils of China coastal zone. Microb. Ecol. 85, 383–399. doi: 10.1007/s00248-022-01996-9
Zhao C., Zhou Y., Pang Y., Zhang Y., Huang W., Wang Y., et al. (2021). The optical and molecular signatures of DOM under the eutrophication status in a shallow, semi-enclosed coastal bay in southeast China. Sci. China Earth Sci. 64, 1090–1104. doi: 10.1007/s11430-020-9728-4
Zheng Y., Hou L., Liu M., Gao J., Yin G., Li X., et al. (2015). Diversity, abundance, and distribution of nirS-harboring denitrifiers in intertidal sediments of the yangtze estuary. Microb. Ecol. 70, 30–40. doi: 10.1007/s00248-015-0567-x
Zheng Y., Hou L., Zhang Z., Ge J., Li M., Yin G., et al. (2021). Overlooked contribution of water column to nitrogen removal in estuarine turbidity maximum zone (TMZ). Sci. Total Environ. 788, 147736. doi: 10.1016/j.scitotenv.2021.147736
Zhou S., Huang T., Zhang C., Fang K., Xia C., Bai S., et al. (2016). Illumina MiSeq sequencing reveals the community composition of NirS-Type and NirK-Type denitrifiers in Zhoucun reservoir-a large shallow eutrophic reservoir in northern China. RSC Adv. 6, 91517–91528. doi: 10.1039/c6ra18017e
Zhu W., Lomsadze A., Borodovsky M. (2010). Ab initio gene identification in metagenomic sequences. Nucleic. Acids Res. 38, e132. doi: 10.1093/nar/gkq275
Keywords: estuary environments, denitrification, nirS gene, denitrifier diversity, geographical separation
Citation: Zhou H, Zhang H, He X, Liu C, Fozia, Cui Q, Yang H, Zhao C, Zhang H, Hu H and Zhang Z (2024) Taxonomic and functional dynamics of nirS denitrifiers along a salinity gradient in the Pearl River Estuary. Front. Mar. Sci. 11:1328684. doi: 10.3389/fmars.2024.1328684
Received: 27 October 2023; Accepted: 12 March 2024;
Published: 22 March 2024.
Edited by:
Siyuan Ye, Qingdao Institute of Marine Geology (QIMG), ChinaReviewed by:
Yiran Hou, Chinese Academy of Fishery Sciences, ChinaMingcong Li, Shandong Agricultural University, China
Copyright © 2024 Zhou, Zhang, He, Liu, Fozia, Cui, Yang, Zhao, Zhang, Hu and Zhang. This is an open-access article distributed under the terms of the Creative Commons Attribution License (CC BY). The use, distribution or reproduction in other forums is permitted, provided the original author(s) and the copyright owner(s) are credited and that the original publication in this journal is cited, in accordance with accepted academic practice. No use, distribution or reproduction is permitted which does not comply with these terms.
*Correspondence: Zongxiao Zhang, zhangzx@sustech.edu.cn
†These authors have contributed equally to this work