- 1School of Ocean Technology, Fisheries and Marine Institute - Memorial University, St. John’s, NL, Canada
- 2Department of Geography, Memorial University, St. John’s, NL, Canada
- 3Department of Earth Sciences, Memorial University of Newfoundland, St. John’s, NL, Canada
- 4Northwest Atlantic Fisheries Centre, Fisheries and Oceans Canada, St. John’s, NL, Canada
Benthic species assemblages are groups of species that co-occur on the seafloor. Linking assemblages to physical environmental features allows for understanding and predicting their spatial distribution. Species identity and abundance are commonly quantified using a taxonomic approach to assess benthic diversity, yet functional traits that describe the behavior, life history, and morphology of a species may be equally or more important. Here, we investigate the biodiversity of five benthic species assemblages in relation to their habitat and environmental conditions in an Ecologically and Biologically Significant Area (EBSA) along Canada’s east coast, using both a taxonomic approach and biological traits analysis. Random Forest regression was applied to map spatial patterns of functional and taxonomic diversity metrics, including richness, Shannon index, and Rao’s quadratic entropy. We evaluate discrepancies between related taxonomic and trait measures, and the community-weighted mean of trait data was calculated to characterize each assemblage. Taxonomic and functional richness – representing the number of species and the species community volume in the trait space, respectively – showed similar spatial patterns. However, when considering diversity, which also accounts for the relative abundance and differences among species or traits, these patterns diverged. Taxonomically different assemblages exhibited similar trait compositions for two assemblages, indicating potential trait equivalencies, while one assemblage exhibited traits potentially indicating sensitivity to human activity. The taxonomic and functional metrics of richness and diversity were low close to the coast, which could be indicative of disturbance. Consideration of functional metrics can support spatial planning and prioritization for management and conservation efforts by assessing the sensitivity of traits to different stressors.
1 Introduction
Coastal ecosystems are highly productive, supporting diverse habitats that provide a wide range of important functions and services (Feist and Levin, 2016; Halpern et al., 2007; Snelgrove et al., 2014). Coastal benthic fauna provide services including food and habitat structure, nutrient cycling, biodiversity maintenance, climate regulation, and carbon sequestration, and also support cultural and commercial industries such as fisheries and tourism (Galparsoro et al., 2014; Snelgrove et al., 2014). These species may also be highly sensitive to disturbances resulting from increased human activities and environmental change (Feist and Levin, 2016; Miatta et al., 2021; Snelgrove et al., 2014). Although stressors such as pollution, fishing, and climate change may alter benthic community composition, they may not necessarily result in a loss of important ecosystem functions (Pinsky et al., 2020; Snelgrove et al., 2014). Ecosystem processes may be more resistant to climate change if species that are undergoing range expansions replace those with similar functional traits (Beche and Resh, 2007; Bonada et al., 2007; Muller et al., 2021; Pinsky et al., 2020; Weigel et al., 2016).
There is growing consensus that managing coastal habitats and their associated functions should reflect an ecosystem-based management (EBM) approach that requires managers to identify sensitive habitats, species, and regions of high biodiversity value as priority areas for conservation (Cogan et al., 2009; Frid et al., 2008; Myers, 1988). Effective EBM requires tools that clearly communicate knowledge of ecosystems to decision-makers and stakeholders (Frid et al., 2008; Murillo et al., 2020a, b). Marine habitat maps, which depict the spatial distribution of communities and assemblages of co-occurring species and seabed types, are one common approach used to disseminate ecological information. These maps can be used to assess changes to the spatial extent and composition of different seabed habitats, facilitating broader EBM efforts (Brown et al., 2011; Buhl-Mortensen et al., 2015; Cogan et al., 2009). Despite increasing efforts to map the seafloor globally, recent evidence suggests that species composition and diversity alone may be insufficient to support EBM, and that functional characteristics of organisms should also be considered (Törnroos et al., 2015).
Biological traits analysis (BTA) is a method for classifying organisms based on their behavioral, life history, and morphological traits, enabling characterization of the functional composition of assemblages (Bremner et al., 2006a; Frid et al., 2008; de Juan et al., 2022). Traits analyses are important for assessments of biodiversity as they can inform how species respond to their environment (response traits) and the impact they have on ecosystem functioning (effect traits; Lavorel and Garnier, 2002; Sterk et al., 2013). This approach can be applied at any taxonomic level and be assessed across broad geographic regions of varying species composition, where traditional species-based assessments may not be applicable (Beauchard et al., 2017; Bremner et al., 2006a, b; Frid et al., 2008; Gagic et al., 2015; Miatta et al., 2021; de Juan et al., 2022). Additionally, analysis of functional trait diversity may provide insight into the provisioning of ecosystem functions and services supported by the abundance of given traits in a region (Cadotte et al., 2011; Miatta et al., 2021; Törnroos et al., 2015). Trait-based diversity approaches can further complement taxonomy-based patterns by identifying alternate biodiversity hotspots, traditionally defined as regions hosting high numbers of endemic or endangered species (Beauchard et al., 2017; Myers, 1988; Roberts et al., 2002; Stuart-Smith et al., 2013). For example, Stuart-Smith et al. (2013) observed that reef fish functional diversity differed between temperate and tropical regions globally, highlighting additional diversity hotspots at higher temperate latitudes outside the tropics. This was due to higher observed species evenness in temperate regions, where a greater proportion of moderately abundant species supported unique trait configurations that could more strongly influence ecological processes (Stuart-Smith et al., 2013).
In light of shifting species’ distributions and compositions due to global changes, area-based conservation measures relying solely on taxonomic measures may be less reliable long term than efforts that incorporate traits-based measures. The latter may be more informative of the ecosystem processes and functions that may be sustained in a given region. This makes BTA particularly relevant for risk assessment frameworks by determining which species exhibiting a combination of traits are vulnerable to specific stressors (Hewitt et al., 2018). Despite the recognition that BTA can provide enhanced understanding of benthic biodiversity, this approach is seldom used for predictive mapping, especially at smaller, local scales (de la Torriente et al., 2020; Murillo et al., 2020b; de Juan et al., 2022). In this study we use BTA to compare the taxonomic and trait-based diversity and composition of coastal benthic assemblages which were classified and mapped by Nemani et al. (2022). Specifically, we 1) investigate the composition of biological traits among species assemblages across two coastal sites in Placentia Bay, Newfoundland, Canada, 2) develop maps depicting the variation in spatial patterns of taxonomic and functional diversity, and 3) discuss how this information may be used to target areas for management and planning.
2 Materials and methods
2.1 Study area
Placentia Bay is an ‘Ecologically and Biologically Significant Area’ (EBSA) that supports a diverse marine environment. The bay contains habitats for several sensitive taxa (e.g., Zostera marina eelgrass; corals and sponges; Dermochelys coriacea leatherback turtle), and commercial species (e.g., Homarus americanus lobster; Mallotus villosus capelin; Clupea harengus herring; Gadus morhua Atlantic cod), and large seabird colonies (Wells et al., 2019). Despite recognized ecological significance, human activities are widespread in the area, including industries such as oil and gas, fishing, aquaculture, and shipping. These industries and their supporting activities (e.g., vessel traffic) render this EBSA as one of the highest oil spill risk areas in Canada (DFO, 2007; DFO Maritimes Region OPP Atlantic Hub, 2020; LGL Limited, 2018).
Given the risks to this EBSA, understanding the distribution of its habitats is critical to monitoring and mitigating environmental risks from increasing anthropogenic stressors, and to improve the response to potential environmental accidents. Two coastal areas located within proximity to the largest population centers and harbors in Placentia Bay were selected as focal sites for this study. These include 1) Mortier Bay, located near Marystown, NL, on the west coast of Placentia Bay, and 2) Long and Ship Harbor near the town of Placentia, NL, on the east coast (Figure 1). Coastal waters at both sites are at risk of human impact due to relatively high population densities, shipping traffic – particularly near Long and Ship Harbor – and a budding aquaculture industry near Mortier Bay (DFO, 2007; LGL Limited, 2018). Both sites were previously mapped by the Canadian Hydrographic Service (CHS) and the Geological Survey of Canada (GSC) during multiple joint surveys spanning 1989 to 2006, providing bathymetry and backscatter data for this analysis (Shaw et al., 2011).
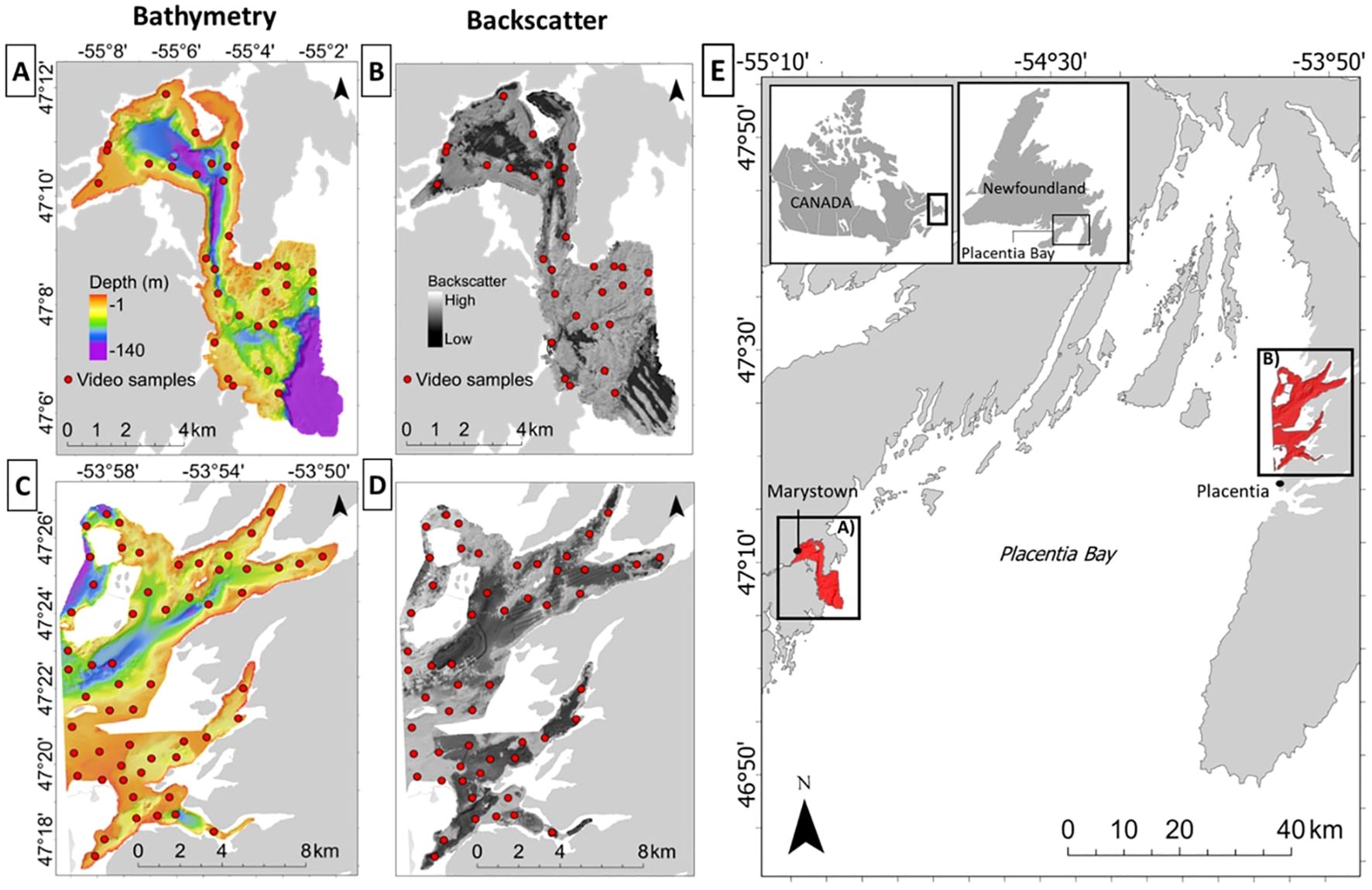
Figure 1. Mortier Bay (A, B), and Long and Ship Harbor (C, D) in Placentia Bay (E), Newfoundland and Labrador, Canada. Red dots represent underwater video sample distribution.
2.2 Data
Underwater videos were collected to assess the composition and abundance of benthic fauna in the study area. Mortier Bay was surveyed from November 18-20th, 2019 using a custom-built drop-camera system comprising a Sony FDRX 3000 camera in an underwater housing, an LED light at 3500 lumens for illumination, and two green-light lasers spaced 10-cm apart for scaling. Videos were recorded in HD at a resolution of 1920 x 1080 and 60 FPS. On August 5th, 2020, Long and Ship Harbor was sampled using the DTPod drop camera system manufactured by Deeptrekker inc. DTPod includes an LED light (1000 Lumens) and two red-light scaling lasers spaced 2.5 cm apart. Videos were also recorded at this location in high definition, at a resolution of 1920 x 1080, and 30 FPS. Sample stations were distributed at each site using a spatially balanced random sampling scheme (Kermorvant et al., 2019) stratified by depth and acoustic backscatter from sonar data collected prior to this study. At each station, the camera was lowered to approximately two meters off the seabed and allowed to drift for a two-minute duration. To account for boat drift during sample collection, we transformed raw species counts to densities (number of organisms per m2) using a frame-by-frame analysis of average image width based on the distance between lasers, and length of transect using data from the GPS track to standardized distances. Thirty-one videos were recorded at Mortier Bay and 57 at Long and Ship Harbor ( total).
All organisms greater than 2 cm were identified to the highest possible taxonomic resolution (Harvey-Clark, 1997; Martinez and Martinez, 2011; Salvo et al., 2018) or assigned to morphotaxa when species level identification could not be achieved and tallied into a site-by-taxa matrix of observed taxa densities (organisms per m2). Sample size and video resolution may have affected the interpretation of “rarer” occurrences and the resulting morpho-taxonomic classification. Accordingly, for all video samples, species occurring less than 5 times across all stations (mainly species which could not be assigned to a phylum), and stations with low species abundances (<5 organisms) were excluded. This was consistent with the methods presented in Nemani et al. (2022), which described five species assemblages (Supplementary Table S1) based on hierarchical clustering of Hellinger-transformed data (Breine et al., 2018). These same 60 sample sites and resulting five assemblages are analyzed further here for trait composition.
Nine biological traits representing the life history, morphology, and behavior of the 27 taxa observed in video were chosen for this analysis (Table 1). The traits were further sub-divided into 39 modalities of trait expression. For example, the trait ‘movement’ was divided into four modalities: “sessile”, “burrower”, “crawler”, and “swimmer”. Since there is currently no standardized methodology for the selection of specific traits (Miatta et al., 2021), this process was guided by the limited data available for benthic taxa identified from the videos (Bremner, 2008). Information on modalities for taxa were acquired from the MarLIN open-access trait database (http://www.marlin.ac.uk/biotic/biotic.php; MarLIN, 2006) and the Arctic Traits Database (https://www.univie.ac.at/arctictraits/taxonpage), since the Newfoundland shelf marine ecoregions contains species with Arctic affinities (Degen et al., 2018). Additionally, the literature was reviewed to fill data gaps for biota of lower taxonomic resolution (Bremner et al., 2006a). The phylum Porifera was the most poorly resolved, so traits were broadly assigned for those morphotaxa. When taxa-specific trait information was unavailable, modalities were inferred using closely related taxa (Henseler et al., 2019; Tornroos et al., 2013). Since some taxa exhibit several modalities of a trait, a fuzzy coding method was applied, where a value of 0 represents no affinity, and 3 represents full affinity to the trait category (Chevenet et al., 1994). For example, a species that deposit feeds or suspension feeds with equal probability was coded 2 for both modalities (Degen and Faulwetter, 2019; Munari, 2013; Sutton et al., 2020; Van der Linden et al., 2012). All fuzzy-coded modality scores within a trait were then standardized and weighted to 1 for each taxon and trait (Tornroos et al., 2013). Compilation of data for BTA requires three matrices: 1) taxa abundance for each site, 2) biological traits for each taxon, and 3) biological traits for each site – the latter resulting from abundance-weighted combination of the first two matrices (Bremner et al., 2006b; Munari, 2013; Van der Linden et al., 2012).
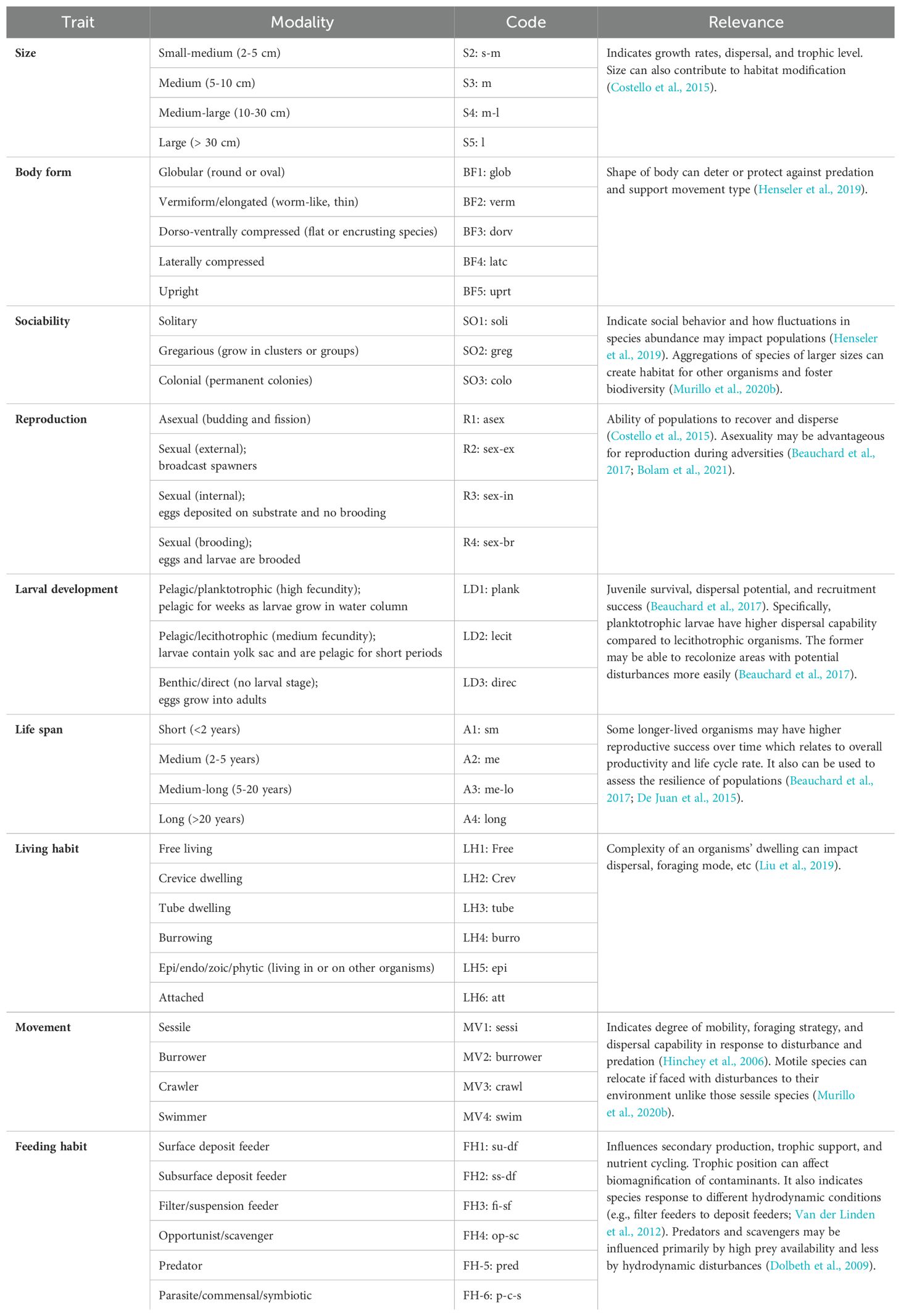
Table 1. List of biological traits and modalities (sub-categories) examined for each taxon observed from underwater videos.
Processed sonar data from previous surveys of the bay were used to characterize seabed morphology and texture. Bathymetry was obtained using multibeam echosounder (MBES), and acoustic backscatter was collected via side-scan sonar (SSS). Backscatter is the intensity of sound returned to the sonar and is commonly used as a proxy for substrate type (e.g., softer sediments return less energy than hard sediments) (Brown et al., 2011). The acoustic data were gridded at 10 m horizontal resolution and projected to metric map units (UTM Zone 21N).
The bathymetry and backscatter surfaces were used to derive secondary features to describe seabed terrain and texture. These features represent abiotic surrogates that are typically used to infer species-environment relationships for predictive mapping, as it can be challenging to measure more direct environmental drivers (e.g., temperature) at sufficient spatial resolutions (McArthur et al., 2010). Terrain features included slope to measure the steepness gradient and aspect derived as northness and eastness to assess seabed orientation (Wilson et al., 2007). Relative difference to the mean value (RDMV) was derived to assess topographic position and the bathymetric standard deviation (SD) was calculated to quantify seabed roughness (Walbridge et al., 2018; Lecours et al., 2015). Additional measures of curvature (curvature, profile, and planar curvature) were used to highlight contours along the seabed such as ridges, valleys, and mounds (Walbridge et al., 2018; Wilson et al., 2007). Local topographic variation (highs and lows) was determined using the bathymetric position index (BPI) and measured at two scales prior to the multi-scale analysis (Misiuk et al., 2018; Walbridge et al., 2018; Wilson et al., 2007): BPI fine (inner and outer radius of 1 and 10 cells) and BPI broad (inner and outer radius of 10 and 30 cells). Seabed morphology and substrate composition can influence the predominance of traits, such as burrowing and crevice dwelling movement types, and these abiotic conditions can be inferred from bathymetric and backscatter derivatives. Further, textural information was derived from the backscatter using grey-level co-occurrence matrices (GLCMs) to quantify local backscatter variation and similarities in seabed texture (Che Hasan et al., 2014).
Euclidean distance from the coast was calculated in ArcGIS Pro V.2.7 to assess community structure patterns in smaller inlets along the coast compared to the more exposed sections of the study sites. For example, Weigel et al. (2016) compared functional diversity and composition in sheltered and exposed sites and found differences in functional identity with respect to particular feeding traits (more sub-surface feeders in exposed sites due to increased sediment reworking). Distance to coast has also been applied as a proxy for sediment transport away from the coast (Misiuk et al., 2018; Stephens and Diesing, 2015; Wilson et al., 2018), which may influence habitat suitability for different taxa.
Multi-scale approaches have been recommended to capture both fine and broad scale terrain characteristics that may influence species-environment relationships (Lecours et al., 2015). Environmental features were calculated at ten spatial scales using increasing focal window sizes from 3x3 to 21x21 cells (Dolan, 2012; Misiuk et al., 2021). This produced 120 features at “analysis distances” (Misiuk et al., 2021) between 10 and 210 m that describe the seabed terrain and its texture across multiple spatial scales (Table 2).
2.3 Data analysis
Trait composition for each assemblage identified by Nemani et al. (2022) was assessed using the community-level weighted mean (CWM), which was calculated by averaging the mean trait values of taxa present in each sample weighted by their relative abundance using the ‘FD’ package in the R programming language (Duarte et al., 2018; Pecuchet et al., 2018; Wen et al., 2019). The CWM is useful for representing shifts in trait composition among communities and for identifying dominant traits that may affect ecosystem processes (Dolbeth et al., 2013; Lepš et al., 2011; Ricotta and Moretti, 2011).
Univariate taxonomic metrics of richness and Shannon diversity, excluding rare species, were calculated for all sites used in Nemani et al. (2022), using the ‘vegan’ package in R (Oksanen et al., 2018). Comparable functional metrics were derived to assess trait richness using the ‘FD’ package, and trait diversity using the ‘SYNCSA’ package (Laliberté and Legendre, 2010; Petchey and Gaston, 2006). Taxonomic richness refers to the number of unique species recorded in each assemblage, while functional richness represents the amount of functional space occupied by traits in the assemblage (Colwell, 2009; Karadimou et al., 2016; Laliberté and Legendre, 2010; Pakeman, 2011; Petchey and Gaston, 2006). Taxonomic diversity was measured using Shannon diversity (H), which accounts for both abundance and evenness of the species (He et al., 2015; Liu et al., 2021; Spellerberg and Fedor, 2003; Xu et al., 2018). Functional trait diversity was assessed using Rao’s quadratic entropy (FDQ), which is used to assess community dispersion of species weighted by their relative abundance within functional trait space (Leps et al., 2006; Rao, 1982; Stuart-Smith et al., 2013). For calculation of these functional indices, the initial fuzzy-coded trait categories were weighted by the total number of categories for a given trait to establish equal importance for each category (Henseler et al., 2019).
Random Forest (RF) was used to model diversity indices using the environmental data to create full-coverage maps and explore the terrain features influencing spatial patterns of diversity. RF is an ensemble modelling approach that uses bootstrap aggregation (i.e., “bagging”) to combine results from a large number of decision trees (Breiman, 2001; Luan et al., 2020). For regression, RF predicts new values using the mean prediction of all decision trees in the “forest” (Breiman, 2001; Guisan et al., 2019). Here, RF was performed using default regression parameters as implemented in the ‘randomForest’ package in R, which included a forest of 500 trees, random selection of 3 out of the 11 predictors to test for each split, and a minimum terminal node size of 5 (Liaw and Wiener, 2002). There was no limit set to the maximum number of nodes for each tree; non-linear interaction between predictors was thus allowed to proceed until the minimum node size was reached at each branch of each tree. The Boruta wrapper algorithm was used to identify subsets of features defined for each scale, as described in Nemani et al. (2022). A leave-one-out cross-validation (LOOCV) was used to derive R2 values to assess the variance explained (Hoeppner et al., 2020; Kuhn, 2008). Features with the greatest explanatory power were ranked using the VarImp function in the ‘Caret’ package (Kuhn, 2012). Finally, relationships between the environmental features and predicted richness and diversity metrics were depicted by univariate partial dependence plots using the ‘pdp’ package (Franklin, 2009; Greenwell, 2017).
3 Results
3.1 Functional trait composition
Functional trait composition (Figure 2) varied among the five assemblages identified by Nemani et al. (2022) and described in Supplementary Table S1 (spatial predictions of assemblages presented in Supplementary Figure S1). Assemblage 1, which was dominated by sand-dollars, occurred in shallow sandy sediments, and exhibited traits including planktotrophic larval development, gregarious sociability, crawling movement, burrowing living habit, and medium body sizes (Figure 2A). Similar traits were observed for the assemblage characterized by urchins and northern sea stars (Assemblage 3; Figure 2C), illustrating that taxonomically different assemblages can exhibit similar functional trait compositions. The main differences among these two assemblages related to living habit (free-living) and globular body forms associated with the dense aggregations of urchins. The affinity for softer sediment demonstrated by Assemblage 1 accommodated burrowing traits, while the urchin aggregations occurred on hard substrate, such as encrusting coralline algae.
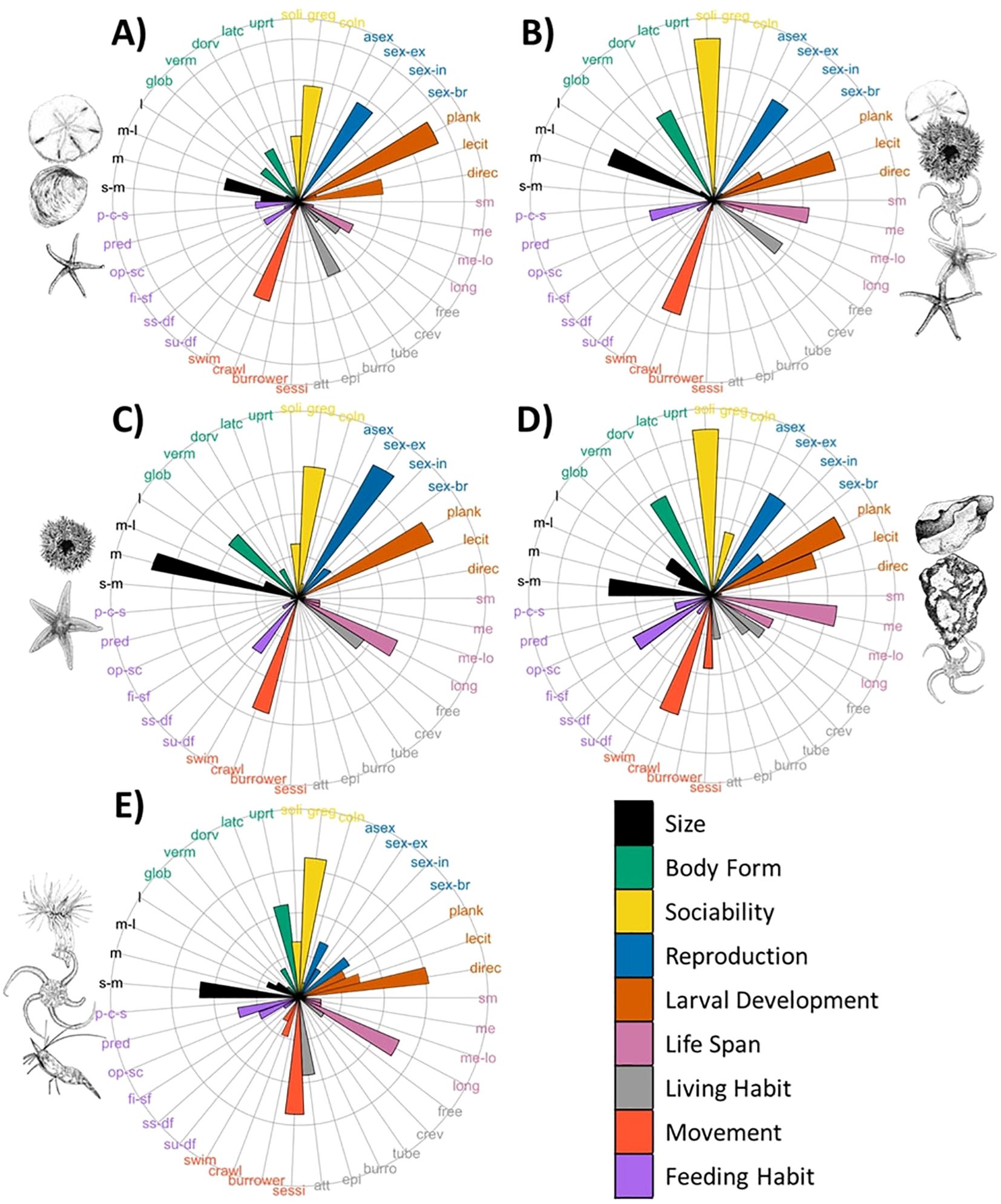
Figure 2. Community weighted mean of trait composition for species assemblages. Labels represent codes for trait modalities from Table 1. Assemblages include Assemblage 1: sand-dollar dominated (A); Assemblage 2: echinoderm mix (B); Assemblage 3: urchin and northern sea stars (C); Assemblage 4: encrusting sponge and brittle star (D); and Assemblage 5: anemone-shrimp-brittle star (E). Taxa illustrations by Julia Mackin-McLaughlin.
Assemblage 5, which contained anemones, shrimps, and brittle stars as dominant taxa, was characterized by small size, sessile, upright body forms, and attached living habit traits (Figure 2E). This functional composition was driven by traits of the Hormathia sp.1 anemone, the dominant taxa in this assemblage. Assemblage 4, characterized by encrusting sponges and brittle star beds, also contained sessile and attached traits, but to a lesser extent (Figure 2D). The high density of brittle stars in Assemblage 4 contributed to more solitary sociability, planktotrophic larval development, medium life spans, crawling movement, and flatter body form (dorso-ventrally composed) traits. Flat body forms were also evident in Assemblage 2, with a mix of commonly occurring echinoderms with higher mobility (free-living, solitary crawlers) and lecithotrophic larval development (Figure 2B).
3.2 Spatial patterns of diversity
Distribution maps were predicted using RF models (model performance metrics and feature importance in Table 3) to visualize local patterns of taxonomic and functional diversity among both coastal sites (Figures 3A, B). Assemblages 1 and 2 exhibited the lowest richness across all metrics. Taxonomic and functional richness, and Shannon diversity (H), were highest at depths exceeding 50 m (Figures 4A–C) where Assemblages 4 and 5 were found to occur.
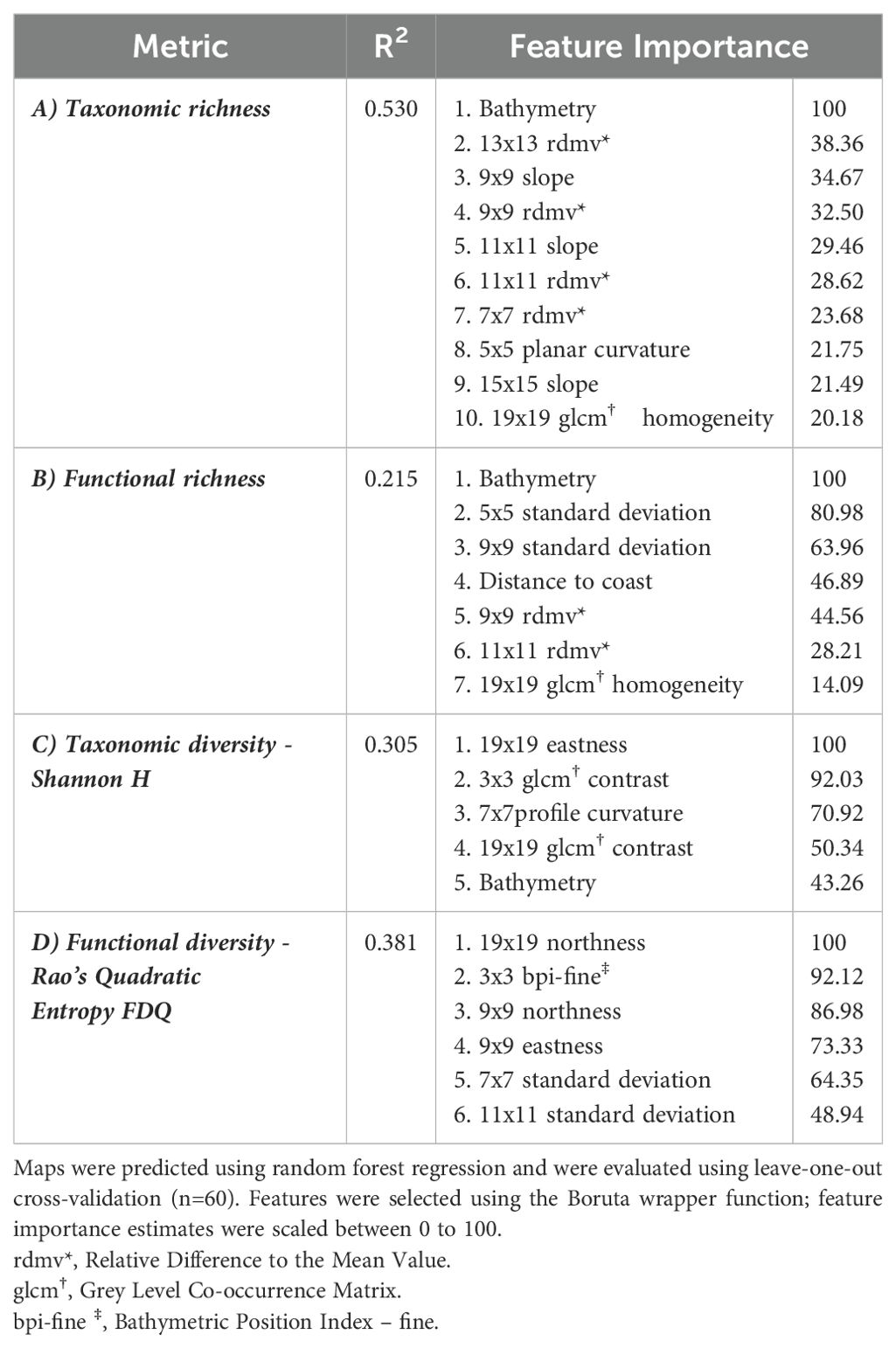
Table 3. Model evaluation metrics for predictions of A) taxonomic richness, B) functional richness, C) Shannon diversity, and D) functional diversity.
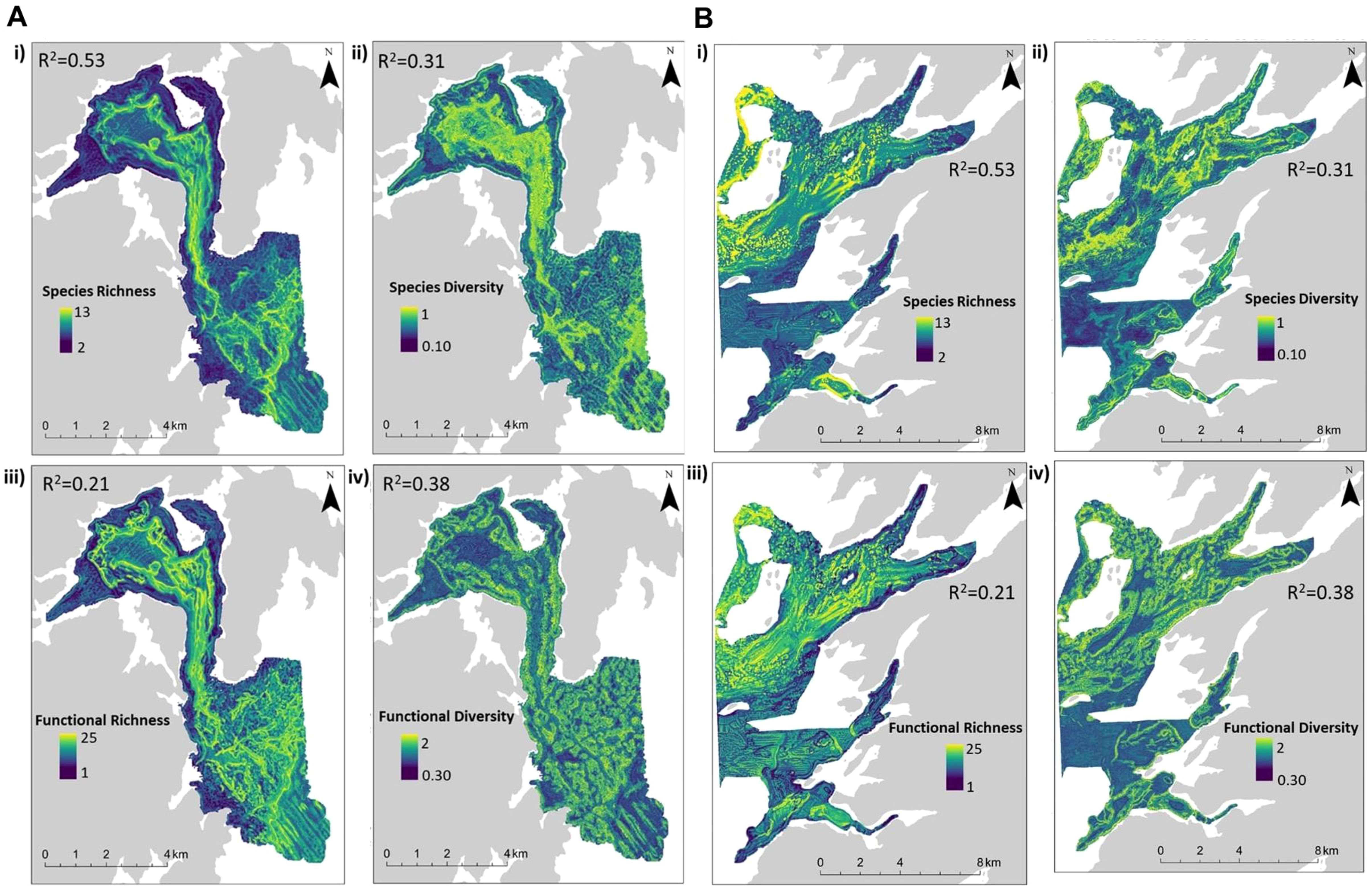
Figure 3. (A) Predicted taxonomic and functional richness and diversity distributions at 10 m resolution for Mortier Bay, including taxonomic richness (i), Shannon diversity (ii), functional richness (iii), and Rao’s quadratic entropy (iv). (B) Predicted taxonomic and functional richness and diversity distributions at 10 m resolution for Long and Ship Harbor, including taxonomic richness (i), Shannon diversity (ii), functional richness (iii), and Rao’s quadratic entropy (iv).
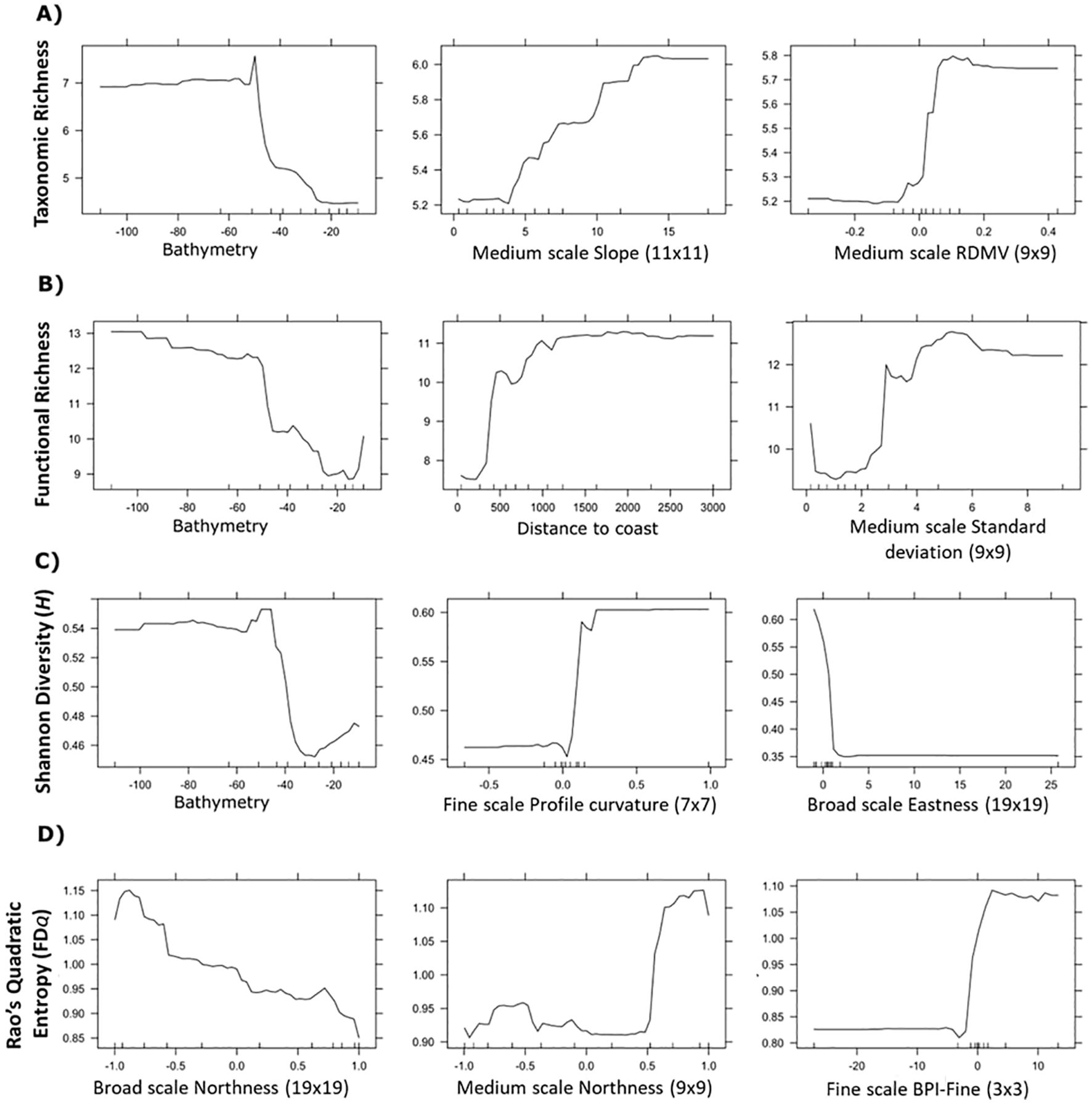
Figure 4. Select partial dependence plots of environmental features depicting the influence of bathymetry and seabed position which were used to model taxonomic richness (A), functional richness (B), taxonomic diversity - Shannon H (C), and functional diversity - Rao’s Quadratic Entropy FDQ (D). Plots for all features are provided in Supplementary Figures S3A–D.
The importance of calculating environmental features across multiple scales was evident. Functional diversity was highest on south facing slopes as indicated by northness at the broadest scale (190 m); however, at a finer scale (90 m), functional diversity was highest on north facing slopes and more rugged fine-scale topographic highs (Figure 4D; Supplementary Figure S4).
Spatially, higher functional richness was predicted more broadly and included regions that were not taxonomically rich – for example, areas dominated by Assemblage 3, which were largely barren because of high urchin abundance (Supplementary Figure S1). Taxonomic richness was high among steep slopes – particularly within sloped valleys near the centers of both coastal sites. Regions characterized by high taxonomic diversity were not necessarily functionally diverse (Figures 3A, B ii vs. iv). Taxonomic diversity was highest in the center of Mortier Bay and towards the mouth of Placentia Bay. However, functional diversity was higher along high slopes and near the deepest areas where Assemblage 5 was predicted to occur, particularly in Mortier Bay. These sloped areas supported a heterogeneous seabed environment, largely composed of softer sediment with underlying rock substrate. Overall, areas with the highest taxonomic and functional diversity and richness were associated with Assemblage 4. These areas were of mixed substrate, with large boulders, smaller cobbles, sediment veneers, and patches of soft sediment with underlying rock, which supported a broad range of taxa and traits.
4 Discussion
Understanding the distribution and diversity of seabed habitats is crucial in the face of increasing environmental impacts from human activities. Traits-based analyses are beneficial in this regard since they are not species-specific – changing species compositions may not necessarily impact broader functions within a region. This study leverages traits-based analyses to assess the functional composition of five benthic assemblages, highlighting the value of this approach in understanding ecological functions independent of taxonomic diversity. The use of life history, behavior, and morphology traits allows us to identify functional similarities among taxonomically different assemblages, suggesting that functional diversity may be maintained despite changes in species composition. Moreover, our spatial patterns of diversity reveal additional regions of ecological importance that might be overlooked by traditional taxonomic assessments.
4.1 Spatial patterns of diversity
Features calculated across multiple scales of analysis were influential for predicting spatial patterns of richness and diversity. Though our results do not capture the traits of rare species that may be functionally equivalent to other taxa in the data, or which may host unique traits, our results align with research recommendations to incorporate multiple components of biodiversity and scale (Petchey and Gaston, 2006; White et al., 2023). Bathymetry was the most important driver of both taxonomic and functional richness, indicating that deep regions of both study areas supported a greater range of species and traits. While bathymetry was not correlated with functional diversity, multiple scales of geomorphic features were important predictors of both taxonomic and functional diversity. For taxonomic diversity, seabed terrain attributes and texture indices were more influential than depth. Traits such as globulose body forms and gregarious traits found in mixed environments with high contrast, meanwhile high slope environments supported attached, colonial organisms (e.g., sponges and tunicates). This aligns with previous observations, wherein higher terrain heterogeneity (e.g., canyons walls, seamounts) is linked to increased biodiversity, possibly due to supporting more niche space that supports a broader range of taxa (de la Torriente et al., 2019; Hewitt et al., 2008; Robert et al., 2014; Thrush et al., 2011; Zeppilli et al., 2016). Taxonomic diversity was also highest along areas with more concave depressions among west facing slopes than elevated mounds or crests, which may experience increased disturbance. Functional diversity was predicted strongly by the northness of the slope direction at both moderate (90 m) and broad (190 m) scales, although these appeared to have contrasting effects (Figure 4D). Finer scale bottom currents could be occurring in these regions, contributing to local heterogeneity by influencing food availability, but such data were not available at sufficient resolutions to include in our analysis.
All map predictions suggested that taxonomic and functional richness and diversity were lowest near the coast. Shallow regions may be at greater risk of anthropogenic stress resulting from increased human activity (Feist and Levin, 2016; Kenny et al., 2017). Additionally, these areas are exposed to increased natural disturbance such as wind and wave action, high tidal activity, and storms (Llanos et al., 2019). The cumulative effects of these stressors may have marked impact on biodiversity compared to areas further from the coasts.
It is important to note that the functional diversity patterns we observed in our maps are inherently tied to the specific set of response traits we used - these traits may not have been the most responsive trait combination to reflect environmental pressures. The functional description of the assemblages displayed in Figure 2 was based on CWM. However, recent studies (Peres-Neto et al., 2017; Ter Braak, 2017; Miller et al., 2018) showed that CWM can be subject to inflated type I errors when searching for relationships between environment (i.e., samples) and traits (i.e., species). These studies recommend using the RLQ-Fourth-corner approach (Dray and Legendre, 2008; Dray et al., 2014) which, unlike CWM, also considers biological variations within assemblages. In line with this, we performed further analyses using the RLQ approach to examine trait responsiveness to environmental variables (Supplementary Figure S2). The observed pattern was not found significant, (details in Supplementary Table S2), possibly due our small sample size that can lower statistical power (Dray and Legendre, 2008), even though some of our traits (which are typical of life history strategies) are often found responsive to environmental forces. There might be other trait combinations verifying the environment-traits relationships, and from which calculations of functional diversity indices might yield spatial patterns that may be more or less different in the resulting maps. These limitations notwithstanding, our findings provide valuable insights for future research, particularly in understanding the potential divergence of functional and taxonomic diversity across the Placentia Bay seascape.
4.2 Trait-specific vulnerabilities of assemblages
Human disturbances such as commercial fishing (e.g., trawling), aggregate dredging, effluent discharge, and other industrial activities, can strongly influence functional indices and composition of benthic invertebrate assemblages (Bolam et al., 2017; Zhulay et al., 2021). Gladstone-Gallagher et al. (2019) observed how increasing spatial extent and frequency of a disturbance can influence a community’s resilience across multiple scales of ecological organization. For example, Assemblage 5, which was characterized by anemones, shrimp, and brittle stars, was distributed broadly at both sites (Supplementary Figure S1), yet its functional composition, which included sessile movement traits, attached living habit, and upright body forms, could make it vulnerable to disturbances such as fishing, trawling, or dredging. Using habitat maps to better understand the distribution of these assemblages has the potential to inform zoning and multi-use management efforts. The distribution of functional traits vary among different seabed habitats and assemblages, and this habitat heterogeneity contributes to the range of ecosystem functions evident in a region (Hewitt et al., 2008; Thrush et al., 2011). Thus, including functional diversity metrics into these maps can provide managers and decision-makers with additional tools to monitor and preserve key ecological processes and functions, despite inevitable taxonomic changes resulting from climate change (Morley et al., 2018; Thrush et al., 2017).
The Placentia Bay region is at risk of oil spills and the release of other pollutants due to high vessel traffic (DFO, 2007), driving a need to assess vulnerabilities of local habitats to these stressors. The release of pollutants could have marked impacts on the benthic communities in this area, including changes to habitats induced by the introduction of toxins (e.g., petroleum hydrocarbons) and potential loss of habitat forming species that support higher diversity (Kotta et al., 2008; Lee and Lin, 2013; Nunnally et al., 2020). Nunnally et al. (2020) observed how sites surrounding the Macondo wellhead, Gulf of Mexico, became increasingly homogenous, with fewer functional traits following the Deepwater Horizon oil spill. Higher presence of mobile predators and scavengers were also observed at impacted sites, while sessile and filter-feeding megafauna had diminished (Nunnally et al., 2020). Conversely, Kotta et al. (2008) noted that specific feeding modes (e.g., deposit feeders) in the northeastern Baltic Sea have benefitted from organic enrichment resulting from an oil spill in 2006. Positive effects on suspension feeders were noted adjacent to sites with organic enrichment due to an increase in phytoplankton productivity (Kotta et al., 2008). The results of our study suggest that Assemblage 5 may be at particular risk of oil spill impacts. The attached and sessile traits exhibited by the Hormathia anemones may increase its sensitivity, whereas mobile scavenging shrimp species could be more abundant in response to increases in organic matter (but see Rotkin-Ellman et al., 2012 for examples of exposure to other long-term stressors). In the event of an oil spill, the efficiency of management efforts could potentially be increased by focusing on areas hosting more vulnerable traits (e.g., Assemblage 5).
On the west coast of Placentia Bay, Mortier Bay hosts a new industrial park at the site of a now defunct shipyard where a large-scale aquaculture hatchery and nursery facility is currently in operation (LGL Limited, 2018). Fish farming can alter benthic ecosystem functioning through release of dissolved nutrients, increased sedimentation of feed, and fecal matter from farmed fish, which can ultimately lead to reduced seafloor diversity (Hargrave, 2010). These disturbances contribute to changes in benthic community composition (Oug et al., 2012), but also impact ecosystem functioning. For example, Sweetman et al. (2014) noted significantly lower bacterial biomass, sediment oxygen consumption, and carbon sequestration near aquaculture sites. Higher sedimentation rates could have detrimental impacts on suspension feeding assemblages, such as Assemblage 4 (Bouvais, 2016). Dredge disposal is also evident in both coastal sites (Shaw et al., 2011), and various studies have observed lower species richness and density, and more variable trait composition and function among dredged sites (Bolam et al., 2016). Bolam et al. (2016) observed that organisms with chitinous or calcareous exoskeletons, some soft-bodied annelid worms, and deeper-dwelling burrowing species were more common among dredge disposal sites. In Placentia Bay, long-term developmental pressure for any new infrastructure will likely result in increased dredging and disposal in both bays and alter the underlying trait composition in these regions.
4.3 Management
Considerations of trait composition can support planning initiatives that are guided within an EBM framework, and further supported by spatial management tools such as habitat and diversity maps. For EBM, prioritizing the maintenance of functional traits rather than focusing efforts on preserving individual species could be more valuable ecologically, especially given anticipated climate-related shifts in species distributions. As current climate change projections predict continued global shifts in species compositions through species loss, invasions, and turnover, management approaches based on taxonomy alone may become increasingly challenging and potentially ineffective over time (Morley et al., 2018; Kaplan, 2019; Pinsky et al., 2020; Miatta et al., 2021). Against this backdrop of global change, functional trait measures for biodiversity monitoring can be particularly beneficial as they are not species-specific and can facilitate broader regional comparisons (Vandewalle et al., 2010). Species with overlapping ecological roles can exhibit similar trait compositions, which may be important for conservation priorities since communities may be more stable with respect to traits than individual taxa (Bonada et al., 2007; Vandewalle et al., 2010; Powney et al., 2014; Miatta et al., 2021; Muller et al., 2021). For example, MPAs, which are commonly designated to conserve specific species and communities, may be considered in poor health with changing community compositions, even though broader ecosystem functions may still be preserved (Pinsky et al., 2020; Miatta et al., 2021; de Juan et al., 2022).
Taxonomic approaches commonly identify habitat and reef forming species such as corals and certain sponge gardens to be ecologically valuable due to their role in forming three-dimensional structures that provide habitat for other species (Aguilar et al., 2017), yet additional taxa, characterized by different sets of traits, can also provide structural habitat. For example, the urchin dominated assemblage may provide three-dimensional biogenic substrate for species such as hydroids and barnacles (Lauzon-Guay and Scheibling, 2007). High densities of taxa with burrowing traits (e.g., Assemblage 1 sand-dollars), and duality of suspension and deposit feeding (e.g., Assemblage 4 brittle star aggregations), can support broader biogeochemical cycling by accelerating energy transfer from the water column to the benthos, including important processes such as nitrogen fixation and carbon sequestration (Bertics et al., 2010; Bauer et al., 2013; Folkers and Rombouts, 2019). Thus, these examples demonstrate how explicit consideration of functional traits can broaden our understanding of ecosystem functioning, laying the groundwork for more effective EBM. Such a framework may be particularly relevant for risk assessment through assessing the sensitivity and vulnerability of benthos to ongoing and emerging stressors based on their underlying trait composition (Hewitt et al., 2018).
Future work could benefit from incorporating aspects of ecosystem functioning to complement the response traits examined in this study. As highlighted by Beauchard (2023), relying exclusively on response traits may not fully capture the complexities of functional diversity. Specifically, areas of high functional diversity values on our maps may not always indicate areas critical from a management perspective as communities with high functional diversity may be composed of both vulnerable and resilient species. Contrastingly, assemblages 3 and 5 exhibiting more vulnerable species (i.e., slow growth, long lifespan) can be found in regions of both high and low species richness. This suggests that high values of functional diversity, based solely on response traits (which reflect species’ abilities to cope with environmental pressures), might not fully represent ecosystem functions, which are primarily indicated by effect traits (Lavorel and Garnier, 2002). For effective management, it is crucial to consider both types of traits to accurately identify communities that perform essential functions and are vulnerable to disturbances (Beauchard, 2023).
5 Conclusion
Functional diversity can complement measures of taxonomic diversity and provide data on ecosystem function that can improve our definitions of ecologically important marine regions. Our results indicated differences between taxonomic and functional diversity at local scales, highlighting potential areas of consideration for EBM that would not have been identified by taxonomic considerations alone. Assessment of functional composition provides insight into the vulnerabilities of specific assemblages to stressors that are present in the bay. We recommend that managers should incorporate biological traits and functional diversity measures to support a comprehensive understanding of the ecology and range of ecosystem functions.
Data availability statement
The original contributions presented in the study are included in the article/Supplementary Material. Further inquiries can be directed to the corresponding author.
Author contributions
SN: Conceptualization, Visualization, Validation, Formal analysis, Writing – review & editing, Data curation. BM: Writing – review & editing, Data curation. DC: Conceptualization, Visualization, Validation, Formal analysis, Writing – review & editing. EE: Conceptualization, Visualization, Validation, Formal analysis, Writing – review & editing. JM-M: Writing – review & editing, Data curation. AT: Writing – review & editing, Data curation. KR: Conceptualization, Visualization, Validation, Formal analysis, Writing – review & editing.
Funding
The author(s) declare financial support was received for the research, authorship, and/or publication of this article. This study was funded by the Fisheries and Oceans Canadas’ Coastal Environmental Baseline Program. Author SN received baseline funding from Memorial University’s School of Graduate Studies as part of the Master of Science program. Additional funds were received by Principal Investigator K. Robert’s Canada Research Chair in Ocean Mapping.
Acknowledgments
Data collection of underwater videos was also supported by Kirk Regular and Eugene Antle onboard the RV D. Cartwright provided by Memorial University’s Fisheries and Marine Institute (Holyrood campus), Newfoundland and Labrador, Canada. Special thank you to Dr. Benjamin Weigel who provided code to develop the radial plots describing the functional composition of species assemblages in Figure 2. Further, all taxa illustrations were designed by co-author J. Mackin-McLaughlin.
Conflict of interest
The authors declare that the research was conducted in the absence of any commercial or financial relationships that could be construed as a potential conflict of interest.
Publisher’s note
All claims expressed in this article are solely those of the authors and do not necessarily represent those of their affiliated organizations, or those of the publisher, the editors and the reviewers. Any product that may be evaluated in this article, or claim that may be made by its manufacturer, is not guaranteed or endorsed by the publisher.
Author disclaimer
All authors on the manuscript have seen and approved the submitted version of the manuscript and have substantially contributed to the work. This manuscript has been submitted solely to Frontiers in Marine Science and has not been published elsewhere, either in part or whole, nor is it in press or under consideration for publication in another journal.
Supplementary material
The Supplementary Material for this article can be found online at: https://www.frontiersin.org/articles/10.3389/fmars.2024.1141737/full#supplementary-material
References
Aguilar R., Perry A. L., López J. (2017). Conservation and management of vulnerable marine benthic ecosystems. Mar. Anim. Forests, 1165–1207. doi: 10.1007/978-3-319-21012-4_34
Bauer J. E., Cai W.-J., Raymond P. A., Bianchi T. S., Hopkinson C. S., Regnier P. A. G. (2013). The changing carbon cycle of the coastal ocean. Nature 504, 61–70. doi: 10.1038/nature12857
Beauchard O. (2023). The importance of trait selection on the meaning of functional diversity in benthic studies. Front. Mar. Sci. 10. doi: 10.3389/fmars.2023.1195595
Beauchard O., Veríssimo H., Queirós A. M., Herman P. M. J. (2017). The use of multiple biological traits in marine community ecology and its potential in ecological indicator development. Ecol. Indic. 76, 81–96. doi: 10.1016/j.ecolind.2017.01.011
Beche L. A., Resh V. H. (2007). Biological traits of benthic macroinvertebrates in California mediterranean-climate streams: long-term annual variability and trait diversity patterns. Fundam. Appl. Limnol. 161, 1–23. doi: 10.1127/1863-9135/2007/0169-0001
Bertics V. J., Sohm J. A., Treude T., Chow C. E. T., Capone D. G., Fuhrman J. A., et al. (2010). Burrowing deeper into benthic nitrogen cycling: The impact of bioturbation on nitrogen fixation coupled to sulfate reduction. Mar. Ecol. Prog. Ser. 409, 1–15. doi: 10.3354/meps08639
Bolam S. G., Garcia C., Eggleton J., Kenny A. J., Buhl-Mortensen L., Gonzalez-Mirelis G., et al. (2017). Differences in biological traits composition of benthic assemblages between unimpacted habitats. Mar. Environ. Res. 126, 1–13. doi: 10.1016/j.marenvres.2017.01.004
Bolam S. G., McIlwaine P. S. O., Garcia C. (2016). Application of biological traits to further our understanding of the impacts of dredged material disposal on benthic assemblages. Mar. pollut. Bull. 105, 180–192. doi: 10.1016/j.marpolbul.2016.02.031
Bolam S. G., McIlwaine P., Garcia C. (2021). Marine macrofaunal traits responses to dredged material disposal. Mar. pollut. Bull. 168, 112412. doi: 10.1016/j.marpolbul.2021.112412
Bonada N., Dolédec S., Statzner B. (2007). Taxonomic and biological trait differences of stream macroinvertebrate communities between Mediterranean and temperate regions: implications for future climatic scenarios. Global Change Biol. 13, 1658–1671. doi: 10.1111/j.1365-2486.2007.01375.x
Bouvais P. (2016). Influence of increased sediment exposure on suspension-feeder assemblages in a temperate seagrass meadow. Available online at: https://ro.ecu.edu.au/theses/1814. (Access date: 9/20/2024).
Breine N. T., De Backer A., Van Colen C., Moens T., Hostens K., Van Hoey G. (2018). Structural and functional diversity of soft-bottom macrobenthic communities in the Southern North Sea. Estuarine Coast. Shelf Sci. 214, 173–184. doi: 10.1016/j.ecss.2018.09.012
Bremner J. (2008). Species’ traits and ecological functioning in marine conservation and management. J. Exp. Mar. Biol. Ecol. 366, 37–47. doi: 10.1016/j.jembe.2008.07.007
Bremner J., Rogers S. I., Frid C. L. J. (2006a). Methods for describing ecological functioning of marine benthic assemblages using biological traits analysis (BTA). Ecol. Indic. 6, 609–622. doi: 10.1016/j.ecolind.2005.08.026
Bremner J., Rogers S. I., Frid C. L. J. (2006b). Matching biological traits to environmental conditions in marine benthic ecosystems. J. Mar. Syst. 60, 302–316. doi: 10.1016/j.jmarsys.2006.02.004
Brown C. J., Smith S. J., Lawton P., Anderson J. T. (2011). Benthic habitat mapping: A review of progress towards improved understanding of the spatial ecology of the seafloor using acoustic techniques. Estuarine Coast. Shelf Sci. 92, 502–520. doi: 10.1016/j.ecss.2011.02.007
Buhl-Mortensen L., Buhl-Mortensen P., Dolan M. J. F., Gonzalez-Mirelis G. (2015). Habitat mapping as a tool for conservation and sustainable use of marine resources: Some perspectives from the MAREANO Programme, Norway. J. Sea Res. 100, 46–61. doi: 10.1016/j.seares.2014.10.014
Cadotte M. W., Carscadden K., Mirotchnick N. (2011). Beyond species: Functional diversity and the maintenance of ecological processes and services. J. Appl. Ecol. 48, 1079–1087. doi: 10.1111/j.1365-2664.2011.02048.x
Che Hasan R., Ierodiaconou D., Laurenson L., Schimel A. (2014). Integrating multibeam backscatter angular response, mosaic, and bathymetry data for benthic habitat mapping. PloS One 9, 1–14. doi: 10.1371/journal.pone.0097339
Chevenet F., Doléadec S., Chessel D. (1994). A fuzzy coding approach for the analysis of long-term ecological data. Freshw. Biol. 31, 295–309. doi: 10.1111/j.1365-2427.1994.tb01742.x
Cogan C. B., Todd B. J., Lawton P., Noji T. T. (2009). The role of marine habitat mapping in ecosystem-based management. ICES J. Mar. Sci. 66, 2033–2042. doi: 10.1093/icesjms/fsp214
Colwell R. K. (2009). “Biodiversity: Concepts, Patterns and Measurement,” in The Princeton Guide to Ecology. Ed. Levin S. A. (Princeton University Press, Princeton), 257–263.
Costello M. J., Claus S., Dekeyzer S., Vandepitte L., Tuama É., Lear D., et al. (2015). Biological and ecological traits of marine species. PeerJ 2015, 1–29. doi: 10.7717/peerj.1201
Degen R., Aune M., Bluhm B. A., Cassidy C., Kędra M., Kraan C., et al. (2018). Trait-based approaches in rapidly changing ecosystems: A roadmap to the future polar oceans. Ecol. Indic. 91, 722–736. doi: 10.1016/j.ecolind.2018.04.050
Degen R., Faulwetter S. (2019). The Arctic traits database – a repository of Arctic benthic invertebrate traits. Earth System Sci. Data 11, 301–322. doi: 10.5194/essd-11-301-2019
de Juan S., Bremner J., Hewitt J., Törnroos A., Mangano M. C., Thrush S., et al. (2022). Biological traits approaches in benthic marine ecology: Dead ends and New Paths. Ecol. Evol. 12 (6), e9001. doi: 10.1002/ece3.9001
de Juan S., Gelcich S., Ospina-Alvarez A., Perez-Matus A., Fernandez M. (2015). Applying an ecosystem service approach to unravel links between ecosystems and society in the coast of central Chile. Sci. Total Environ. 533, 122–132. doi: 10.1016/j.scitotenv.2015.06.094
de la Torriente A., Aguilar R., González-Irusta J. M., Blanco M., Serrano A. (2020). Habitat forming species explain taxonomic and functional diversities in a mediterranean seamount. Ecol. Indic. 118, 106747. doi: 10.1016/j.ecolind.2020.106747
de la Torriente A., González-Irusta J. M., Aguilar R., Fernández-Salas L. M., Punzón A., Serrano A. (2019). Benthic habitat modelling and mapping as a conservation tool for marine protected areas: A seamount in the western Mediterranean. Aquat. Conservation: Mar. Freshw. Ecosyst. 29, 732–750. doi: 10.1002/aqc.3075
DFO. (2007). Placentia Bay Integrated Management Plan (St. John’s, NL, A1C 5X1: Department of Fisheries and Ocean #2007/1292, Canadian Science Advisory Secretariat).
DFO Maritimes Region OPP Atlantic Hub. (2020). Canada’s Ocean Protection Plan - Pan-Atlantic Report (DFO Maritimes Region OPP Atlantic Hub: Fisheries and Oceans Canada).
Dolan M. F. (2012). Calculation of slope angle from bathymetry data using GIS-effects of computation algorithm, data resolution and analysis scale.
Dolbeth M., Cardoso P., Grilo T., Raffaelli D., Pardal M. A. (2013). Drivers of estuarine benthic species distribution patterns following a restoration of a seagrass bed: A functional trait analyses. Mar. Pollut. Bull. 72, 47–54. doi: 10.1016/j.marpolbul.2013.05.001
Dolbeth M., Teixeira H., Marques J. C., Pardal M.Â. (2009). Feeding guild composition of a macrobenthic subtidal community along a depth gradient. Scientia Marina 73, 225–237. doi: 10.3989/scimar.2009.73n2225
Dray S., Choler P., Dolédec S., Peres-Neto P. R., Thuiller W., Pavoine S., et al. (2014). Combining the fourth-corner and the RLQ methods for assessing trait responses to environmental variation. Ecology 95, 14–21. doi: 10.1890/13-0196.1
Dray S., Legendre P. (2008). Testing the species traits–environment relationships: The fourth-corner problem revisited. Ecology 89, 3400–3412. doi: 10.1890/08-0349.1
Duarte L. D. S., Debastiani V. J., Carlucci M. B., Diniz-Filho J. A. F. (2018). Analyzing community-weighted trait means across environmental gradients: should phylogeny stay or should it go? Ecology 99, 385–398. doi: 10.1002/ecy.2018.99.issue-2
Feist B. E., Levin P. S. (2016). Novel indicators of anthropogenic influence on marine and coastal ecosystems. Front. Mar. Sci. 3. doi: 10.3389/fmars.2016.00113
Folkers M., Rombouts T. (2019). Sponges revealed: A synthesis of their overlooked ecological functions within aquatic ecosystems. YOUMARES 9 - The Oceans: Our Research, Our Future. (Bremerhaven, Germany: Alfred Wegener Institute, Helmholtz Centre for Polar and Marine Research) 181–193.
Franklin J. (2009). Mapping Species Distributions - Spatial Inference and Prediction (Cambridge, UK: Cambridge University Press).
Frid C. L. J., Paramor O. A. L., Brockington S., Bremner J. (2008). Incorporating ecological functioning into the designation and management of marine protected areas. Hydrobiologia 606, 69–79. doi: 10.1007/s10750-008-9343-y
Gagic V., Bartomeus I., Jonsson T., Taylor A., Winqvist C., Fischer C., et al. (2015). Functional identity and diversity of animals predict ecosystem functioning better than species-based indices. Proc. R. Soc. B: Biol. Sci. 282 (1801), 20142620. doi: 10.1098/rspb.2014.2620
Galparsoro I., Borja A., Uyarra M. C. (2014). Mapping ecosystem services provided by benthic habitats in the European North Atlantic Ocean. Front. Mar. Sci. 1. doi: 10.3389/fmars.2014.00023
Gladstone-Gallagher R. V., Pilditch C. A., Stephenson F., Thrush S. F. (2019). Linking traits across ecological scales determines functional resilience. Trends Ecol. Evol. 34, 1080–1091. doi: 10.1016/j.tree.2019.07.010
Greenwell B. M. (2017). Pdp: an R package for constructing partial dependence plots. R J. 9, 421–436. doi: 10.32614/RJ-2017-016
Guisan A., Mod H. K., Scherrer D., Münkemüller T., Pottier J., Alexander J. M., et al. (2019). Scaling the linkage between environmental niches and functional traits for improved spatial predictions of biological communities. Global Ecol. Biogeogr. 28, 1384–1392. doi: 10.1111/geb.v28.10
Halpern B. S., Selkoe K. A., Micheli F., Kappel C. V. (2007). Evaluating and ranking the vulnerability of global marine ecosystems to anthropogenic threats. Conserv. Biol. 21, 1301–1315. doi: 10.1111/j.1523-1739.2007.00752.x
Hargrave B. T. (2010). Empirical relationships describing benthic impacts of salmon aquaculture. Aquacult. Environ. Interact. 1, 33–46. doi: 10.3354/aei00005
Harvey-Clark C. (1997). Eastern Tidepool and Reef: North Atlantic Marine Life (Surrey, BC: Hancock House Pub Ltd).
He F., Jiang W., Tang T., Cai Q. (2015). Assessing impact of acid mine drainage on benthic macroinvertebrates: Can functional diversity metrics be used as indicators? J. Freshw. Ecol. 30, 513–524. doi: 10.1080/02705060.2014.998730
Henseler C., Nordström M. C., Törnroos A., Snickars M., Pecuchet L., Lindegren M., et al. (2019). Coastal habitats and their importance for the diversity of benthic communities: A species- and trait-based approach. Estuarine Coast. Shelf Sci. 226, 106272. doi: 10.1016/j.ecss.2019.106272
Hewitt J. E., Lundquist C. J., Ellis J. (2018). Assessing sensitivities of marine areas to stressors based on biological traits. Conserv. Biol. 33, 142–151. doi: 10.1111/cobi.2019.33.issue-1
Hewitt J. E., Thrush S. F., Dayton P. D. (2008). Habitat variation, species diversity and ecological functioning in a marine system. J. Exp. Mar. Biol. Ecol. 366, 116–122. doi: 10.1016/j.jembe.2008.07.016
Hinchey E. K., Schaffner L. C., Hoar C. C., Vogt B. W., Batte L. P. (2006). Responses of estuarine benthic invertebrates to sediment burial: the importance of mobility and adaptation. Hydrobiologia 556, 85–98. doi: 10.1007/s10750-005-1029-0
Hoeppner J. M., Skidmore A. K., Darvishzadeh R., Heurich M., Chang H.-C., Gara T. W. (2020). Mapping canopy chlorophyll content in a temperate forest using airborne hyperspectral data. Remote Sens. 12, 3573. doi: 10.3390/rs12213573
Kaplan S. (2019). The world’s ecosystems are being fundamentally transformed in the human era. The Washington Post. Retrieved November 27, 2021. Available online at: https://www.washingtonpost.com/science/2019/10/17/worlds-ecosystems-are-being-fundamentally-transfigured-human-era/. (Access date: 9/20/2024).
Karadimou E. K., Kallimanis A. S., Tsiripidis I., Dimopoulos P. (2016). Functional diversity exhibits a diverse relationship with area, even a decreasing one. Sci. Rep. 6, 35420. doi: 10.1038/srep35420
Kenny A. J., Jenkins C., Wood D., Bolam S. G., Mitchell P., Scougal C., et al. (2017). Assessing cumulative human activities, pressures, and impacts on North Sea benthic habitats using a biological traits approach. ICES J. Mar. Sci. 75, 1080–1092. doi: 10.1093/icesjms/fsx205
Kermorvant C., D’Amico F., Bru N., Caill-Milly N., Robertson B., Amico F. D. (2019). Spatially balanced sampling designs for environmental surveys. Environ. Monit. Assess. 191, 524. doi: 10.1007/s10661-019-7666-y
Kotta J., Aps R., Herkül. K. (2008). Predicting ecological resilience of marine benthic communities facing a high risk of oil spills. Environ. Problems Coast. Regions 7, 101–110. doi: 10.2495/CENV080091
Kuhn M. (2008). Building predictive models in R using the caret package. J. Stat. Software 28, 1–26. doi: 10.18637/jss.v028.i05
Kuhn M. (2012). Variable selection using the caret package. Available online at: http://cran.r-project.org/web/packages/caret/vignettes/caretSelection.pdf. (Access date: 9/20/2024).
Laliberté E., Legendre P. (2010). A distance-based framework for measuring functional diversity from multiple traits. Ecology 91, 299–305. doi: 10.1890/08-2244.1
Lauzon-Guay J. S., Scheibling R. E. (2007). Seasonal variation in movement, aggregation, and destructive grazing of the green sea urchin (Strongylocentrotus droebachiensis) in relation to wave action and sea temperature. Mar. Biol. 151, 2109–2118. doi: 10.1007/s00227-007-0668-2
Lavorel S., Garnier E. (2002). Predicting changes in community composition and ecosystem functioning from plant traits: Revisiting the Holy Grail. Funct. Ecol. 16, 545–556. doi: 10.1046/j.1365-2435.2002.00664.x
Lecours V., Devillers R., Schneider D. C., Lucieer V. L., Brown C. J., Edinger E. N. (2015). Spatial scale and geographic context in benthic habitat mapping: Review and future directions. Mar. Ecol. Prog. Ser. 535, 259–284. doi: 10.3354/meps11378
Lee L.-H., Lin H.-J. (2013). Effects of an oil spill on benthic community production and respiration on subtropical intertidal sandflats. Mar. pollut. Bull. 73, 291–299. doi: 10.1016/j.marpolbul.2013.05.006
Leps J., Bello D. F., Lavorel S., Berman S. (2006). Quantifying and interpreting functional diversity of natural communities: practical considerations matter. Preslia 78, 481–501.
Lepš J., de Bello F., Šmilauer P., Doležal J. (2011). Community trait response to environment: disentangling species turnover vs intraspecific trait variability effects. Ecography 34 (5), 856–863. http://www.jstor.org/stable/41315806.
LGL Limited. (2018). Environmental impact statement of the placentia Bay Atlantic Salmon aquaculture project (Grieg NL, Marystown, N: FA014 by LGL Limited), 528. Available at: https://www.mae.gov.nl.ca/env_assessment/projects/Y2016/1834/1834_eis_docs/1834_main_text_eis.pdf.
Liu Z., Li Z., Castro D. M., Tan X., Jiang X., Meng X., et al. (2021). Effects of different types of land-use on taxonomic and functional diversity of benthic macroinvertebrates in a subtropical river network. Environ. Sci. pollut. Res. 28, 44339–44353. doi: 10.1007/s11356-021-13867-w
Liu K., Lin H., He X., Huang Y., Li Z., Lin J., et al. (2019). Functional trait composition and diversity patterns of marine macrobenthos across the Arctic Bering Sea. Ecol. Indic. 102, 673–685. doi: 10.1016/j.ecolind.2019.03.029
Llanos E. N., Becherucci M. E., Garaffo G. V., Vallarino E. A. (2019). A shift of ecosystem engineers during the succession of an intertidal benthic community associated with natural and anthropogenic disturbances. Regional Stud. Mar. Sci. 191(8), 524. doi: 10.1016/j.rsma.2019.100754
Luan J., Zhang C., Xu B., Xue Y., Ren Y. (2020). The predictive performances of random forest models with limited sample size and different species traits. Fisheries Res. 227, 105534. doi: 10.1016/j.fishres.2020.105534
MarLIN, Marine Life Information Network (2006). BIOTIC – biological traits information catalogue (Plymouth, United Kingdom: The Marine Biological Association). Available at: www.marlin.ac.uk/biotic.
Martinez A. J., Martinez C. S. (2011). Marine Life of the North Atlantic: Canada to Cape May (Locust Valley, NY: Aqua Quest Publications).
McArthur M. A., Brooke B. P., Przeslawski R., Ryan D. A., Lucieer V. L., Nichol S., et al. (2010). On the use of abiotic surrogates to describe marine benthic biodiversity. Estuarine Coast. Shelf Sci. 88, 21–32. doi: 10.1016/j.ecss.2010.03.003
Miatta M., Bates A. E., Snelgrove P. V. R. (2021). Incorporating biological traits into conservation strategies. Annu. Rev. Mar. Sci. 13, 421–443. doi: 10.1146/annurev-marine-032320-094121
Miller J. E., Damschen E. I., Ives A. R. (2018). Functional traits and community composition: A comparison among community-weighted means, weighted correlations, and multilevel models. Methods Ecol. Evol. 10, 415–425. doi: 10.1111/2041-210X.13119
Misiuk B., Lecours V., Bell T. (2018). A multiscale approach to mapping seabed sediments. PloS One 13, 1–24. doi: 10.1371/journal.pone.0193647
Misiuk B., Lecours V., Dolan M. F. J., Robert K. (2021). Evaluating the suitability of multi-scale terrain attribute calculation approaches for seabed mapping applications. Marine Geodesy 44 (4), 327–385.
Morley J. W., Selden R. L., Latour R. J., Frölicher T. L., Seagraves R. J., Pinsky M. L. (2018). Projecting shifts in thermal habitat for 686 species on the North American Continental Shelf. PloS One 13 (5), e0196127. doi: 10.1371/journal.pone.0196127
Muller A., Poitrimol C., Nunes F. L., Boyé A., Curd A., Desroy N., et al. (2021). Musical chairs on temperate reefs: Species turnover and replacement within functional groups explain regional diversity variation in assemblages associated with Honeycomb worms. Front. Mar. Sci. 8. doi: 10.3389/fmars.2021.654141
Munari C. (2013). Benthic community and biological trait composition in respect to artificial coastal defense structures: A study case in the northern Adriatic sea. Mar. Environ. Res. 90, 47–54. doi: 10.1016/j.marenvres.2013.05.011
Murillo F. J., Kenchington E., Koen-Alonso M., Guijarro J., Kenchington T. J., Sacau M., et al. (2020a). Mapping benthic ecological diversity and interactions with bottom-contact fishing on the Flemish Cap (northwest Atlantic). Ecol. Indic. 112, 106135. doi: 10.1016/j.ecolind.2020.106135
Murillo F. J., Weigel B., Bouchard Marmen M., Kenchington E. (2020b). Marine epibenthic functional diversity on Flemish Cap (north-west Atlantic)—Identifying trait responses to the environment and mapping ecosystem functions. Diversity Distributions 26, 460–478. doi: 10.1111/ddi.13026
Myers N. (1988). Threatened Biotas: “Hot spots” in tropical forests. Environmentalist 8, 187–208. doi: 10.1007/BF02240252
Nemani S., Cote D., Misiuk B., Edinger E., Mackin-McLaughlin J., Templeton A., et al. (2022). A multi-scale feature selection approach for predicting benthic assemblages. Estuarine Coast. Shelf Sci. 277, 108053. doi: 10.1016/j.ecss.2022.108053
Nunnally C. C., Benfield M. C., McClain C. R. (2020). Trait-based diversity of deep-sea benthic megafauna communities near the Deepwater Horizon Oil Spill Site. Mar. Ecol. 41 (5), e12611. doi: 10.1111/maec.12611
Oksanen J., Blanchet F. G., Friendly M., Kindt R., Legendre P., McGlinn D., et al. (2018). Vegan: Community Ecology Package (R Package Version 2.), 4–6. https://cran.r-project.org/web/packages/vegan/vegan.pdf.
Oug E., Fleddum A., Rygg B., Olsgard F. (2012). Biological traits analyses in the study of pollution gradients and ecological functioning of marine soft bottom species assemblages in a fjord ecosystem. J. Exp. Mar. Biol. Ecol. 432–433, 94–105. doi: 10.1016/j.jembe.2012.07.019
Pakeman R. J. (2011). Functional diversity indices reveal the impacts of land use intensification on Plant Community Assembly. J. Ecol. 99, 1143–1151. doi: 10.1111/j.1365-2745.2011.01853.x
Pecuchet L., Reygondeau G., Cheung W. W. L., Licandro P., van Denderen P. D., Payne M. R., et al. (2018). Spatial distribution of life-history traits and their response to environmental gradients across multiple marine taxa. Ecosphere 9 (10), e02460. doi: 10.1002/ecs2.2018.9.issue-10
Peres-Neto P. R., Dray S., ter Braak C. J. (2017). Linking trait variation to the environment: Critical issues with community-weighted mean correlation resolved by the fourth-corner approach. Ecography 40, 806–816. doi: 10.1111/ecog.02302
Petchey O. L., Gaston K. J. (2006). Functional diversity: Back to basics and looking forward. Ecol. Lett. 9, 741–758. doi: 10.1111/j.1461-0248.2006.00924.x
Pinsky M. L., Selden R. L., Kitchel Z. J. (2020). Climate-driven shifts in marine species ranges: Scaling from organisms to communities. Annu. Rev. Mar. Sci. 12, 153–179. doi: 10.1146/annurev-marine-010419-010916
Powney G. D., Preston C. D., Purvis A., Van Landuyt W., Roy D. B. (2014). Can trait-based analyses of changes in species distribution be transferred to new geographic areas? Global Ecol. Biogeogr. 23, 1009–1018. doi: 10.1111/geb.2014.23.issue-9
Rao C. R. (1982). Diversity and dissimilarity coeffiicients: a unified approach. Theoretic Population Biol. 21, 24–43. doi: 10.1016/0040-5809(82)90004-1
Ricotta C., Moretti M. (2011). CWM and Rao's quadratic diversity: a unified framework for functional ecology. Oecologia 167 (1), 181–188. doi: 10.1007/s00442-011-1965-5
Robert K., Jones D. O. B., Huvenne V. A. I. (2014). Megafaunal distribution and biodiversity in a heterogeneous landscape: The iceberg-scoured Rockall Bank, NE Atlantic. Mar. Ecol. Prog. Ser. 501, 67–88. doi: 10.3354/meps10677
Roberts C. M., McClean C. J., Veron J. E., Hawkins J. P., Allen G. R., McAllister D. E., et al. (2002). Marine Biodiversity Hotspots and conservation priorities for tropical reefs. Science 295, 1280–1284. doi: 10.1126/science.1067728
Rotkin-Ellman M., Wong K. K., Solomon G. M. (2012). Seafood contamination after the BP Gulf Oil Spill and risks to vulnerable populations: A critique of the FDA risk assessment. Environ. Health Perspect. 120, 157–161. doi: 10.1289/ehp.1103695
Salvo F., Oldford V., Bungay T., Boone C. D., Hamoutenem D. (2018). Guide for video monitoring of hard bottom benthic communities of the south coast of Newfoundland for aquaculture impact assessments. Fisheries and Oceans Canada. (St. John’s, NL: Canadian Data Report of Fisheries and Aquatic Sciences). Report 1284.
Shaw J., Potter D. P., Kostylev V. E. (2011). Seascapes, Placentia Bay, Newfoundland and Labrador. (Geological Survey of Canada – Atlantic Region: Geological Survey of Canada) Open File 6683.
Snelgrove P. V. R., Thrush S. F., Wall D. H., Norkko A. (2014). Real world biodiversity-ecosystem functioning: A seafloor perspective. Trends Ecol. Evol. 29, 398–405. doi: 10.1016/j.tree.2014.05.002
Spellerberg I. F., Fedor P. J. (2003). A tribute to Claude Shannon, (1916–2001) and a plea for more rigorous use of species richness, species diversity and the ‘Shannon–Wiener’Index. Global Ecol. Biogeogr. 12.3, 177–179. doi: 10.1046/j.1466-822X.2003.00015.x
Stephens D., Diesing M. (2015). Towards quantitative spatial models of seabed sediment composition. PloS One 10, 1–23. doi: 10.1371/journal.pone.0142502
Sterk M., Gort G., Klimkowska A., van Ruijven J., van Teeffelen A. J. A., Wamelink G. W. W. (2013). Assess ecosystem resilience: Linking response and effect traits to environmental variability. Ecol. Indic. 30, 21–27. doi: 10.1016/j.ecolind.2013.02.001
Stuart-Smith R. D., Bates A. E., Lefcheck J. S., Duffy J. E., Baker S. C., Thomson R. J., et al. (2013). Integrating abundance and functional traits reveals new global hotspots of fish diversity. Nature 501, 539–542. doi: 10.1038/nature12529
Sweetman A. K., Norling K., Gunderstad C., Haugland B. T., Dale T. (2014). Benthic ecosystem functioning beneath fish farms in different hydrodynamic environments. Limnol. Oceanogr. 59, 1139–1151. doi: 10.4319/lo.2014.59.4.1139
ter Braak C. J. F. (2017). Fourth-corner correlation is a score test statistic in a log-linear trait–environment model that is useful in permutation testing. Environ. Ecol. Stat 24, 219–242. doi: 10.1007/s10651-017-0377-9
Thrush S. F., Chiantore M., Asnagi V., Hewitt J., Fiorentino D., Cattaneo-Vietti R. (2011). Habitat-diversity relationships in rocky shore algal turf infaunal communities. Mar. Ecol. Prog. Ser. 424, 119–132. doi: 10.3354/meps08960
Thrush S. F., Hewitt J. E., Kraan C., Lohrer A. M., Pilditch C. A., Douglas E. (2017). Changes in the location of biodiversity-ecosystem function hot spots across the seafloor landscape with increasing sediment nutrient loading. Proc. R. Soc. B 284, 20162861. doi: 10.1098/rspb.2016.2861
Tornroos A., Bonsdorff E., Bremner J., Blomqvist M., Josefson A. B., Garcia C., et al. (2015). Marine benthic ecological functioning over decreasing taxonomic richness. J. Sea Res. 98, 49–56. doi: 10.1016/j.seares.2014.04.010
Tornroos A., Nordström M. C., Bonsdorff E. (2013). Coastal habitats as surrogates for taxonomic, functional and trophic structures of benthic faunal communities. PloS One 8, 1–14. doi: 10.1371/journal.pone.0078910
Van Der Linden P., Patrício J., Marchini A., Cid N., Neto J. M., Marques J. C. (2012). A biological trait approach to assess the functional composition of subtidal benthic communities in an estuarine ecosystem. Ecol. Indic. 20, 121–133. doi: 10.1016/j.ecolind.2012.02.004
Vandewalle M., de Bello F., Berg M. P., Bolger T., Dolédec S., Dubs F., et al. (2010). Functional traits as indicators of biodiversity response to land use changes across ecosystems and organisms. Biodiversity Conserv. 19, 2921–2947. doi: 10.1007/s10531-010-9798-9
Walbridge S., Slocum N., Pobuda M., Wright D. (2018). Unified geomorphological analysis workflows with benthic terrain modeler. Geosciences 8, 94. doi: 10.3390/geosciences8030094
Weigel B., Blenckner T., Bonsdorff E. (2016). Maintained functional diversity in benthic communities in spite of diverging functional identities. Oikos 125, 1421–1433. doi: 10.1111/oik.2016.v125.i10
Wells N., Tucker K., Allard K., Warren M., Olson S., Gullage L., et al. (2019). Re-evaluation of the Placentia Bay-Grand Banks Area of the Newfoundland and Labrador Shelves Bioregion to Identify and Describe Ecologically and Biologically Significant Areas. (St. John’s, NL: DFO Canadian Science Advisory Secretariat). Research Document: 2019/049), viii + 151.
Wen Z., Zheng H., Smith J. R., Zhao H., Liu L., Ouyang Z. (2019). Functional diversity overrides community-weighted mean traits in linking land-use intensity to hydrological ecosystem services. Sci. total Environ. 682, 583–590. doi: 10.1016/j.scitotenv.2019.05.160
White H. J., McKeon C. M., Pakeman R. J., Buckley Y. M. (2023). The contribution of geographically common and rare species to the spatial distribution of biodiversity. Global Ecol. Biogeogr. 32, 1730–1747. doi: 10.1111/geb.v32.10
Wilson M. F. J., O’Connell B., Brown C., Guinan J. C., Grehan A. J. (2007). Multiscale terrain analysis of multibeam bathymetry data for habitat mapping on the continental slope. Mar. Geodesy 30 (1–2), 3–35. doi: 10.1080/01490410701295962
Wilson R. J., Speirs D. C., Sabatino A., Heath M. R. (2018). A synthetic map of the north-West European shelf sedimentary environment for applications in Marine Science. Earth System Sci. Data 10, 109–130. doi: 10.5194/essd-10-109-2018
Xu Y., Stoeck T., Forster D., Ma Z., Zhang L., Fan X. (2018). Environmental status assessment using biological traits analyses and functional diversity indices of benthic ciliate communities. Mar. pollut. Bull. 131, 646–654. doi: 10.1016/j.marpolbul.2018.04.064
Zeppilli D., Pusceddu A., Trincardi F., Danovaro R. (2016). Seafloor heterogeneity influences the biodiversity–ecosystem functioning relationships in the Deep Sea. Sci. Rep. 6 (1), 26352. doi: 10.1038/srep26352
Keywords: biological traits analysis, coastal habitats, functional diversity, geomorphometry, predictive modelling, species assemblages, benthic habitat mapping
Citation: Nemani S, Misiuk B, Cote D, Edinger E, Mackin-McLaughlin J, Templeton A and Robert K (2024) Incorporating functional traits with habitat maps: patterns of diversity in coastal benthic assemblages. Front. Mar. Sci. 11:1141737. doi: 10.3389/fmars.2024.1141737
Received: 10 January 2023; Accepted: 11 September 2024;
Published: 03 October 2024.
Edited by:
Ibon Galparsoro, Technological Center Expert in Marine and Food Innovation (AZTI), SpainReviewed by:
Olivier Beauchard, Royal Netherlands Institute for Sea Research (NIOZ), NetherlandsJudi Hewitt, The University of Auckland, New Zealand
Copyright © 2024 Nemani, Misiuk, Cote, Edinger, Mackin-McLaughlin, Templeton and Robert. This is an open-access article distributed under the terms of the Creative Commons Attribution License (CC BY). The use, distribution or reproduction in other forums is permitted, provided the original author(s) and the copyright owner(s) are credited and that the original publication in this journal is cited, in accordance with accepted academic practice. No use, distribution or reproduction is permitted which does not comply with these terms.
*Correspondence: Shreya Nemani, c2hyZXlhLm5lbWFuaTBAZ21haWwuY29t