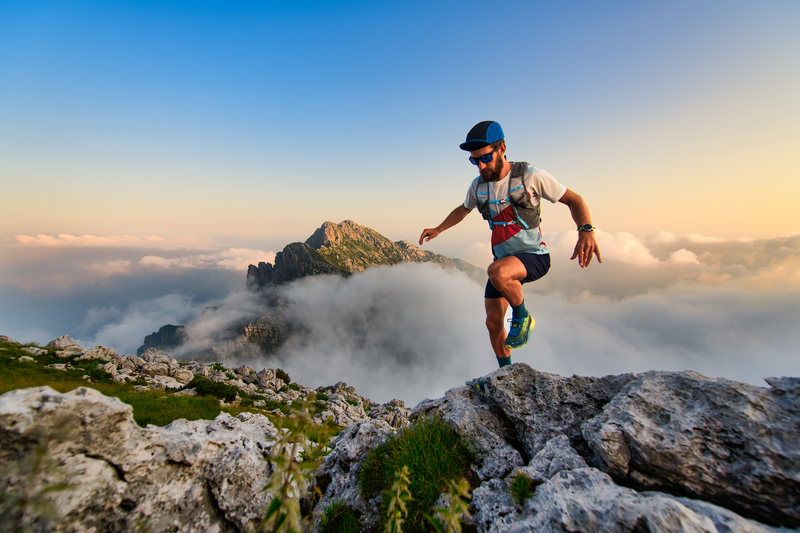
95% of researchers rate our articles as excellent or good
Learn more about the work of our research integrity team to safeguard the quality of each article we publish.
Find out more
EDITORIAL article
Front. Mar. Sci. , 05 October 2023
Sec. Ocean Observation
Volume 10 - 2023 | https://doi.org/10.3389/fmars.2023.1296334
This article is part of the Research Topic Spatiotemporal Modeling and Analysis in Marine Science View all 13 articles
Editorial on the Research Topic
Spatiotemporal modeling and analysis in marine science
Recent advancements in monitoring technologies have ushered in an era characterized by an explosion of spatiotemporal data across diverse fields, notably within global oceans. This surge of data, sourced from satellites, unmanned aerial vehicles, buoys, and unmanned underwater vehicles, poses challenges in extracting meaningful insights and bridging the gap between raw data and scientific understanding (Frankel and Reid, 2008; Wu et al., 2020). Data-driven spatiotemporal modeling and analysis present potential solutions, offering avenues to uncover inherent features within the data, delineate characteristics of natural phenomena, refine general knowledge or theories, and bolster the management and conservation of oceans. Consequently, based on these spatiotemporal, spatial or temporal data, specific methodologies are tailored and employed for analysis. For instance, prevalent machine learning and deep learning techniques excel in modeling the nonlinear and non-stationary aspects of spatiotemporal, spatial, or temporal data (Ham et al., 2019; Reichstein et al., 2019; Runge et al., 2019; Callaghan et al., 2021). Geostatistical methods adeptly capture spatial or spatiotemporal correlations within such data (He and Kolovos, 2018; He and Christakos, 2021; Wu et al., 2021). Additionally, time-frequency methods decompose temporal data into distinct series for nuanced variation detection (Cazelles et al., 2008; Xiao et al., 2019). Cutting-edge frameworks, such as those integrating geostatistical and machine learning methods, have been proposed to more accurately reflect the natural phenomena (Du et al., 2021). The primary objective of this Research Topic is to furnish a platform for scholars to disseminate novel methodologies or insights within the spatiotemporal context of marine or coastal regions.
Chen et al. posited that integrating artificial intelligence (AI) technology with extensive datasets from ocean observations can significantly address challenges in advancing marine science or theory, especially in the intermediate ocean depths ranging from approximately 100 to 1,000 meters. In their review titled “Deep Blue AI for Knowledge Discovery of Intermediate Ocean,” they synthesized findings from satellite remote sensing at around 100 meters deep and in situ observations at approximately 1,000 meters. Additionally, they discussed three distinct AI methodologies: associative statistical, physically informed, and mathematically driven neural networks. The application of these methodologies was reviewed in context, covering areas such as the 3-D identification and trajectory prediction of oceanic eddies, the vertical reconstruction of Ekman drift, internal wave forecasting, and subsurface chlorophyll maxima prediction.
Wang et al. centered their research on predicting typhoon activity to bolster decision-making aimed at mitigating associated risks. A pivotal challenge in modeling arose when selecting the optimal number of historical satellite images for future predictions. Using too few images might not offer adequate information on typhoon trajectories, while an excessive number could diminish the size of the training dataset. To address this, the team devised a feature enhancement module paired with a channel attention module. This combination was designed to amplify the intrinsic characteristics of typhoons and determine the appropriate number of images for subsequent modeling. The spatiotemporal attributes of typhoons were then modeled using a symmetrical encode-decode module comprising convolutional long short-term memory networks (ConvLSTM). Furthermore, a multi-scale strategy was implemented to curtail information loss during the ConvLSTM process. In their quest to design the optimal structure for the Enhanced Multi-Scale Deep Neural Network (EMSN), the team explored multi-scale components, channel attention modules, and spatiotemporal capture units. By adjusting various parameters within these modules, they assessed the accuracy of typhoon predictions. Notably, the EMSN outperformed both the MSCIP satellite image predictor and the conventional U-net.
While hyperspectral remote sensing data are limited and costly, Hu et al. investigated the potential of reconstructing hyperspectral images using economical RGB images, a development that could enhance marine observations. By replacing the standard convolution kernel with atrous convolution and incorporating a multi-scale atrous convolution residual block, the image’s multi-scale spatial features were more effectively extracted by integrating images across different scales into a multi-scale feature layer without increasing computational costs. The introduced Multi-Scale Atrous Convolution Residual Network (MACRN) comprises three segments: low-level feature extraction, high-level feature extraction, and feature transformation. MACRN’s efficacy was evaluated using clean and real-world datasets and benchmarked against exsiting algorithms like HSCNN-R, HSCNN-D, HRNet, AWAN, and MST++.
Li devoted significant effort to applying the greedy strategy for mode identification with significantly crossed frequencies and overlapped component separation. Consequently, the Spatio-Temporal Nonconvex Penalty Adaptive Chirp Mode Decomposition (STNP-ACMD) algorithm was proposed. It addresses the limitations of traditional algorithms, such as ACMD and Variational Mode Decomposition (VMD), which primarily prioritize channel-wise processing without accommodating the coupled nature and spatio-temporal characteristics. The STNP-ACMD employs a recursive mode extraction approach to segregate overlapped components or crossed intrinsic functions and accentuates the spatio-temporal relationship within the coupled nature by refining the spatial and temporal matrices. Comparative analysis, using both numerical and real-world case studies, demonstrated that STNP-ACMD surpassed the Ensemble Empirical Mode Decomposition and VMD.
Chiu et al. leveraged the spatial correlation traits found within observed data to estimate blue carbon (BC) stocks in marsh soils. A Bayesian linear mixed-effects model was devised to assimilate auxiliary information from variables such as marsh type, soil category, soil depth, and marsh site. This model assumed that site effects adhered to the intrinsic conditional autoregressive (ICAR) spatial dependence. By incorporating the ICAR marsh site effects, an inherent spatial clustering of sites became discernible, a pattern not reflected in the primary auxiliary predictors. Notably, the ICAR model produced a narrower confidence interval for the marsh site effects’ coefficient compared to its non-ICAR counterpart, highlighting the spatial correlation of BC stock and underscoring the importance of including these characteristics in BC assessment models.
JH: Funding acquisition, Writing – original draft, Writing – review & editing. ZD: Writing – review & editing. XX: Writing – review & editing.
This work is supported by National Natural Science Foundation of China (Nos. 42301374 and 42171398), the Major Program of Natural Science Foundation of Zhejiang for Creative Research Group (Grant No. LDT23D06024D06) and Science Foundation of Donghai Laboratory (Grant No. 2022KF01012).
We thank Dr. Dongxiao Zhang for his help on handling one manuscript submitted to this Research Topic. We also thank all authors of the articles published in this Research Topic for their significant contributions and the reviewers for their valuable comments. We appreciate the kindly help and supports from Rui Fernandes, Ronan Conlon, Alice Lickley, Joshua Chew, Minea Kaartinen, Francesco Rossetti, and Emily Keynton.
The authors declare that the research was conducted in the absence of any commercial or financial relationships that could be construed as a potential conflict of interest.
The author(s) declared that they were an editorial board member of Frontiers, at the time of submission. This had no impact on the peer review process and the final decision.
All claims expressed in this article are solely those of the authors and do not necessarily represent those of their affiliated organizations, or those of the publisher, the editors and the reviewers. Any product that may be evaluated in this article, or claim that may be made by its manufacturer, is not guaranteed or endorsed by the publisher.
Callaghan M., Schleussner C.-F., Nath S., Lejeune Q., Knutson T. R., Reichstein M., et al. (2021). Machine-learning-based evidence and attribution mapping of 100,000 climate impact studies. Nat. Clim. Change 11, 966–972. doi: 10.1038/s41558-021-01168-6
Cazelles B., Chavez M., Berteaux D., Ménard F., Vik J. O., Jenouvrier S., et al. (2008). Wavelet analysis of ecological time series. Oecologia 156, 287–304. doi: 10.1007/s00442-008-0993-2
Du Z., Qi J., Wu S., Zhang F., Liu R. (2021). A spatially weighted neural network based water quality assessment method for large-scale coastal areas. Environ. Sci. Technol. 55, 2553–2563. doi: 10.1021/acs.est.0c05928
Frankel F., Reid R. (2008). Big data: Distilling meaning from data. Nature 455, 30–30. doi: 10.1038/455030a
Ham Y.-G., Kim J.-H., Luo J.-J. (2019). Deep learning for multi-year ENSO forecasts. Nature 573, 568–572. doi: 10.1038/s41586-019-1559-7
He J., Christakos G. (2021). “Bayesian maximum entropy,” in Encyclopedia of mathematical geosciences, encyclopedia of earth sciences series. Eds. Daya Sagar B. S., Cheng Q., McKinley J., Agterberg F. (Cham: Springer International Publishing), 1–9. doi: 10.1007/978-3-030-26050-7_50-1
He J., Kolovos A. (2018). Bayesian maximum entropy approach and its applications: a review. Stoch Environ. Res. Risk Assess. 32, 859–877. doi: 10.1007/s00477-017-1419-7
Reichstein M., Camps-Valls G., Stevens B., Jung M., Denzler J., Carvalhais N., et al. (2019). Deep learning and process understanding for data-driven Earth system science. Nature 566, 195–204. doi: 10.1038/s41586-019-0912-1
Runge J., Bathiany S., Bollt E., Camps-Valls G., Coumou D., Deyle E., et al. (2019). Inferring causation from time series in Earth system sciences. Nat. Commun. 10, 2553. doi: 10.1038/s41467-019-10105-3
Wu L., Chen Z., Lin X., Liu Y. (2020). Building the integrated observational network of “Transparent Ocean.” CSB 65 (25), 2654–2661. doi: 10.1360/TB-2020-0558
Wu J., He J., Christakos G. (2021). Quantitative analysis and modeling of earth and environmental data (Amsterdam, Netherlands: Elsevier).
Keywords: spatiotemporal, modeling, marine science, machine learning, time-frequency
Citation: He J, Du Z and Xiao X (2023) Editorial: Spatiotemporal modeling and analysis in marine science. Front. Mar. Sci. 10:1296334. doi: 10.3389/fmars.2023.1296334
Received: 18 September 2023; Accepted: 29 September 2023;
Published: 05 October 2023.
Edited and Reviewed by:
Hervé Claustre, Centre National de la Recherche Scientifique (CNRS), FranceCopyright © 2023 He, Du and Xiao. This is an open-access article distributed under the terms of the Creative Commons Attribution License (CC BY). The use, distribution or reproduction in other forums is permitted, provided the original author(s) and the copyright owner(s) are credited and that the original publication in this journal is cited, in accordance with accepted academic practice. No use, distribution or reproduction is permitted which does not comply with these terms.
*Correspondence: Junyu He, anhnemhlanVueXVAMTYzLmNvbQ==
Disclaimer: All claims expressed in this article are solely those of the authors and do not necessarily represent those of their affiliated organizations, or those of the publisher, the editors and the reviewers. Any product that may be evaluated in this article or claim that may be made by its manufacturer is not guaranteed or endorsed by the publisher.
Research integrity at Frontiers
Learn more about the work of our research integrity team to safeguard the quality of each article we publish.