- 1Institute for Marine and Antarctic Studies, University of Tasmania, Hobart, TAS, Australia
- 2Department of Climate Change, Energy, the Environment, and Water, Australian Antarctic Division, Kingston, TAS, Australia
- 3Australia Antarctic Program Partnership, University of Tasmania, Hobart, TAS, Australia
The relationships between flying Antarctic seabirds and their at-sea environments remain poorly understood, particularly outside of the breeding season. Improving our knowledge of how these marine predators use their habitat is a critical step towards conservation of these species. We tracked 27 adult Snow Petrels from two large breeding colonies in East Antarctica during the Austral winter (non-breeding period) – when they are primarily at sea away from their nesting sites. During this time, Snow Petrel habitat use was most associated with bathymetry (> 5000 m), low sea-surface height, relatively close distance to the southern boundary of the Antarctic Circumpolar Current, relatively close distance to the ice-edge, relatively high sea-ice concentration, and low sea-surface temperature. Individuals displayed various movement patterns: 20 birds occupied overlapping winter areas that ranged broadly (up to 2000 km) from their breeding sites. The remaining birds ventured far beyond their breeding sites – reaching a maximal distance from the colony of 5,268 km. One individual circumnavigated Antarctica. Daily activity patterns were related to day length, with peak activity occurring near dawn and dusk. Nocturnal activity increased from March until August/September. Key results reveal and depict Snow Petrel habitat maps for the non-breeding period in the East Antarctic region.
1 Introduction
Understanding ecological interactions and habitat use are fundamental tenets in spatial ecology (Cox et al., 2018). Such an understanding is important for decision makers considering the use of resources in ecosystem-based management (Hawkes et al., 2011). However, gaining insights into ecosystem interactions can be difficult in marine settings, where most fauna are difficult to observe (Furness and Camphuysen, 1997; Roncon et al., 2018; Riaz et al., 2021). To overcome this obstacle, tracking devices that collect data in situ are often deployed on highly mobile marine predators (Bondavalli and Ulanowicz, 1999; Hazen et al., 2019; Hindell et al., 2020). Such predators are often active across large expanses of habitat, where they hunt prey from multiple trophic levels, and subsequently amplify trophic information across multiple spatiotemporal dimensions (Hazen et al., 2019).
In the Southern Ocean, seabirds are top-order predators, many of whom spend most of their life at sea, only returning to land (or in the case of Emperor Penguins (Aptenodytes forsteri), sea-ice) to breed (Brown, 1980; del Hoyo, 2020). This life strategy – shared with seals – makes seabirds more accessible to study, and in situ data collection more feasible, than other marine taxa. It also allows researchers to deploy and retrieve tracking devices from a reliable point of origin such as a breeding colony (Hipfner et al., 2020). Tracking devices can remotely collect large quantities of data over extended periods of time (Jaeger et al., 2017). This makes them particularly valuable in marine ecology, as synoptic, at-sea observations of marine species requires ship-time, and the capacity for this is generally limited (Viola et al., 2022). This limitation is amplified at high latitudes such as in the Southern Ocean where environmental conditions are harsh and extended marine-based field work is logistically difficult or hazardous (Poland et al., 2003).
Many seabirds travel distances of thousands, tens of thousands (MacLeod et al., 2008; Rayner et al., 2008; Pinet et al., 2011), or hundreds of thousands of kilometers every year (Weimerskirch et al., 2015). The at-sea distribution of seabirds varies seasonally (Menkhorst et al., 2017) with birds travelling to areas remote from their breeding sites during the non-breeding season (González-Solís et al., 2007; Hedd et al., 2012; Gilg et al., 2013). Variation in conditions encountered during the non-breeding season can affect important demographic parameters like breeding success and quality of parental care (Webster and Marra, 2005; Reudink et al., 2009; Puskic et al., 2019). And when considering species with high migratory connectivity — the degree to which migratory movements of an individual or a population are linked spatially and temporally between and within seasons — demographic parameters associated with gene flow and natal dispersal become important considerations (Steiner and Gaston, 2005). As seabirds may be susceptible to benefits and stressors not encountered in their terrestrial breeding territory (Davis et al., 2016), a thorough understanding of at-sea distribution is vital to their conservation. Resource managers (such as fisheries targeting the prey fields of important marine predators) need to remain cognisant of at-sea predator distributions throughout the year, and not just during the breeding season. Tracking seabirds throughout the non-breeding period is an important step in addressing these concerns.
Despite the advantages and importance of studying seabirds, and their global recognition as bio-indicators of ecosystem health (Burger and Gochfeld, 2004; Piatt et al., 2007; Hazen et al., 2019), there is a paucity of literature on Antarctic flying seabirds when compared to seabird species in temperate and equatorial regions, but also when compared to other Antarctic vertebrate fauna (i.e., pinnipeds, cetaceans, and penguins) (Ainley et al., 2012; Roncon et al., 2018). Antarctic ecosystems host a suite of flying and non-flying seabird species (Menkhorst et al., 2017; Harrison et al., 2021). Many of the flying seabirds belong to the family Procellariidae, of which the most diverse guild (in terms of body size) is the fulmarine petrels (Harrison et al., 2021).
The smallest fulmarine petrel is the Snow Petrel (Pagodroma nivea), whose body mass ranges from< 250 to nearly 500 g (Moe et al., 2007). Snow Petrels occupy a circumpolar breeding distribution (Croxall et al., 1995), and along with Antarctic Petrels (Thalassoica antarctica) and South Polar Skuas (Catharacta maccormicki), are the most southerly breeding birds (Weathers et al., 2000). Much of the recent work regarding Snow Petrels has focused on breeding ecology, breeding sites, or understanding historical conditions using paleo-ecological deposits (Amundsen, 1995; Barbraud and Chastel, 1999; Einoder et al., 2014; Younger et al., 2016; Berg et al., 2019; Carrea et al., 2019; McClymont et al., 2021). Snow Petrels breed during the austral summer, often in small colonies or loose aggregations, along the coast of Antarctica and surrounding islands (Olivier et al., 2004). However, some birds breed in mountain ranges deep inland, up to 440 km from the coast (Goldsworthy and Thomson, 2000; Thor and Low, 2011).
Whilst seemingly abundant, historical attempts to estimate the global population size for Snow Petrels have been inconclusive, and hence, a comprehensive measure of their abundance remains unknown (Croxall et al., 1995). A sound ecological understanding of Snow Petrels — and many other petrel species — remains elusive. Despite concerted efforts to gather data on these seabirds in the 1980s, 1990s and early 2000s, the number of published datasets that exist for petrels in the Antarctic regions is small (Olivier et al., 2004). Moreover, early records are usually anecdotal, making it difficult to identify changes in population size and patterns over time (Olivier et al., 2004). To date, much of what we understand about Snow Petrel marine habitat use is derived from shipboard surveys in the 1980’s and 1990’s (Ainley et al., 1993; Ribic et al., 2011). Shipboard data is extremely beneficial to our understanding of the pelagic distribution of seabirds, and many seabird communities have been described via this method (Ainley et al., 2012).
However, it is well established that integrating data from tracking studies with observational data substantially improves our understanding of the pelagic distribution of seabirds (Priddel et al., 2014). Occasional sightings near research stations in winter suggest that some individuals remain in Antarctica, either relatively close to their breeding sites, or inland, during the non-breeding period (AAD personnel, pers. comms.). Yet, the non-breeding distribution of these birds is still poorly documented. To date, only one tracking study of birds breeding at Île des Pétrels, Pointe Géologie archipelago (66.67°S, 140.02°E), examined the movements of Snow Petrels during the non-breeding period (Delord et al., 2016). For all other colonies/breeding sites (> 195, based off estimates from Croxall et al., 1995), it remains unclear where important areas are during winter, or to what extent populations overlap and individuals share foraging habitat. Increasing our understanding of Snow Petrel habitat requirements will help create a baseline from which potentially threatening changes can be predicted and determined.
Hereby, to improve our understanding of how Snow Petrels use habitat during the non-breeding period, we deployed geolocators and concurrently tracked post-breeding Snow Petrels from two widely separated breeding sites in East Antarctica. Our aims were to determine their habitat use and foraging activity patterns throughout the non-breeding period, and to develop habitat suitability maps for the non-breeding period relevant to Snow Petrels in the East Antarctic region.
2 Methods
2.1 Study sites and field methods
Geolocator tracking devices (MK 18L geolocators (GLS), British Antarctic Survey) were fitted to incubating adult Snow Petrels at two widely separated breeding locations in East Antarctica: Béchervaise Island (67.59°S, 62.82°E), Mac. Robertson Land, and Filla Island (68.81°S, 77.83°E) in Prydz Bay, Princess Elizabeth Land (Figure 1). At Béchervaise Island, the birds were equipped with GLS devices during the incubation stage from the 17th – 20th of January 2011 (n = 14), and from the 24th – 25th of January 2012 (n = 14). At Filla Island, devices were fitted from the 11th – 13th January 2011 (n = 10). Devices were attached with small cable ties to 12 mm wide, soft, double-sided Velcro©. The Velcro band was then wrapped around the birds’ tarsi, and the end of the band was secured with an industrial strength superglue, Loctite 401. The combined weight of the device, cable ties, and Velcro was three grams, equivalent to ~1% of the birds’ mean body mass. At Filla Island, eight of ten birds were recaptured in the following season and the devices retrieved. At Béchervaise Island, eight of 14 tags deployed in January 2011 were retrieved in December 2011, while two were recovered in December 2012. Nine of 14 birds equipped in January 2012 were recaptured in December 2012. All recovered tags delivered data.
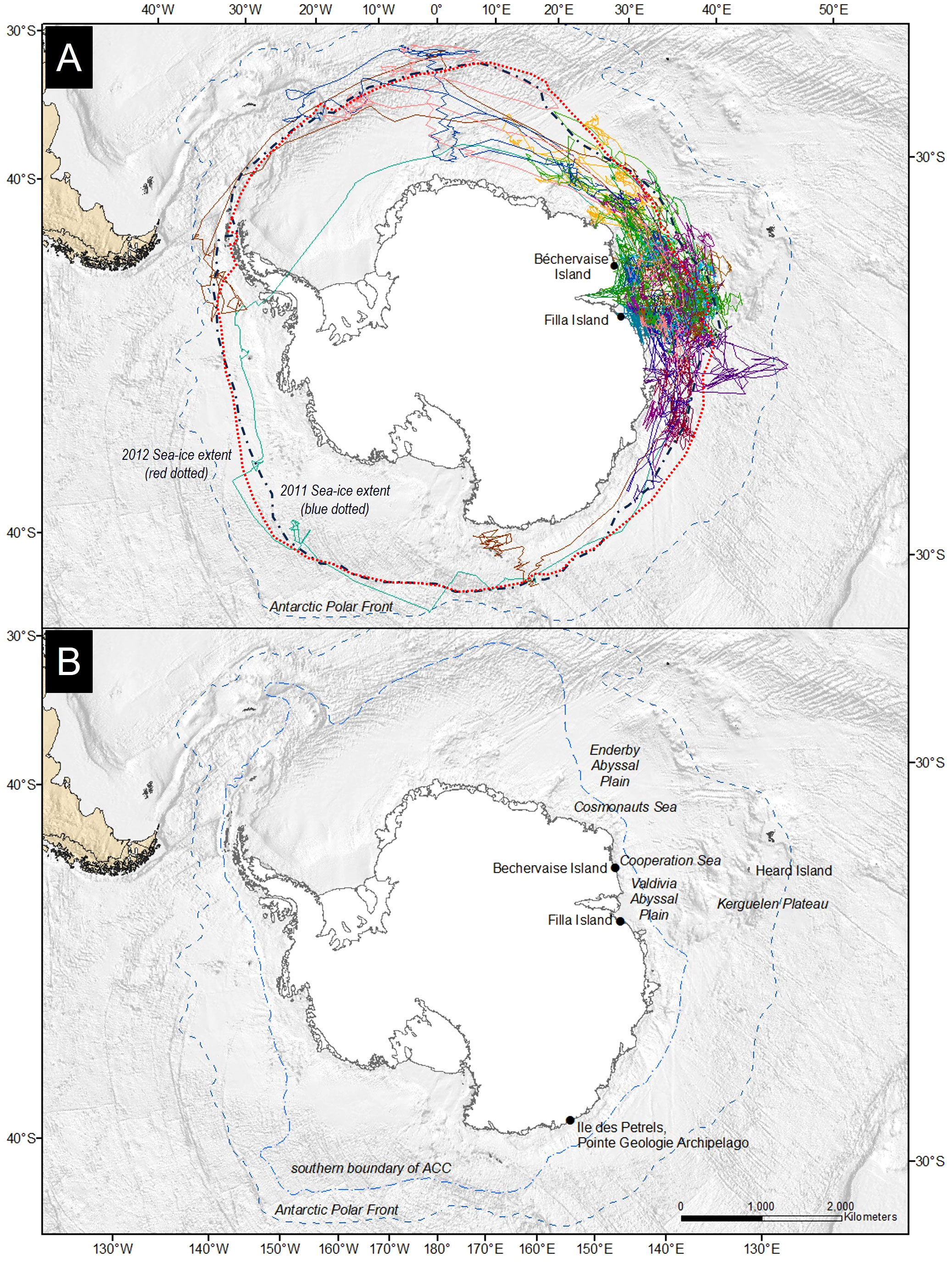
Figure 1 (A) Tracks of Snow Petrels Pagodroma nivea from Béchervaise and Filla islands in 2011 and 2012. Each colored solid line represents a tracked individual. The Antarctic Polar Front (or Antarctic Convergence) is shown as a dashed line. (B) Location of important sites and features mentioned within this manuscript. The southern boundary of the Antarctic Circumpolar Current (ACC) (light blue) and the Antarctic Polar Front (dark blue) are labelled and shown as dashed lines. The 2011 (blue) and 2012 (red) maximal sea-ice extents are added as dotted contours and labelled in (A).
2.2 Data analyses
Analyses were limited to data collected from the 1st of March — when a clear day-night cycle occurs at the latitudes under study, and all birds had left the colonies — until the 31st of October, just prior to the birds’ return in early November (and subsequent tag retrieval). All data handling and processing occurred in Microsoft Excel (Microsoft Corporation 2018) and in the R statistical environment (R Core Team 2021) unless stated otherwise.
2.3 Tracking data
The geolocation tags recorded light levels every 10 minutes. The positions of individual birds were estimated using the light-level data twice daily (dawn and dusk) with the SGAT and BAStag packages (Sumner et al., 2009; Lisovski and Hahn, 2012). These packages apply a threshold method that determines twilight as the time at which the ambient light levels cross a predefined threshold (Sumner et al., 2009; Wotherspoon et al., 2013).
The GLS tags recorded saturation status (wet or dry) at three-second intervals and stored the data in five-minute bins. We assumed that the tags were always wet while a bird was feeding but dried quickly once a bird became airborne. Prolonged ‘wet’ activity was most likely associated with resting and/or preening on the ocean surface, but we acknowledge some feeding may have occurred when the sensor read wet over this time. ‘Dry’ activity included flying but also resting on a dry surface.
2.4 Distances travelled, at-sea movements, and activity patterns
To compare differences in distances travelled by Snow Petrels from the two deployment sites, Welch’s t-tests were used. All means are given ± 1 SE, and the level of significance was set at p = 0.05 unless stated otherwise. The proportions of wet/dry activities were calculated per day per bird and summarised by month, as well as day. The loggers recorded time in UT (universal time). For the examination of cathemeral patterns, these data were adjusted to local times relative to the deployment location.
2.5 Habitat suitability, preference, and use
Throughout this manuscript, we use the following terms to describe subtly different, but related, conditions: ‘Habitat’ refers to the polar environment surrounding Snow Petrels; ‘habitat use’ refers to the environmental conditions in which tracked Snow Petrels were recorded (i.e. the habitats that they were observed to make use of); ‘available habitat’ refers to the environmental conditions that were accessible to (within flying distance of) the birds, and thus they could potentially have made use of; ‘habitat preference’ is a measure of habitat use relative to availability, whereby “preferred habitats” are environmental conditions that are used more often than would be expected according to their availability (the animals “prefer” to use these habitats). Note that habitat preference is based only on the environmental conditions of the area in question, and does not consider whether it is physically accessible to the animals. ‘Habitat suitability’ is a modified version of habitat preference that additionally accounts for the accessibility of the area in question. A given area might therefore have a high habitat preference value (indicating that it contains environmental conditions preferred by the animals), but a low habitat suitability if it is located far from the colony and therefore not readily accessible to the animals.
Tracks of geographic positions and expected flight activities (foraging/resting/travelling) were generated by a state-space model using the BSAM package (Jonsen et al., 2015). Once the track of each bird had been established, 50 random tracks were simulated for each observed track, using the availability package (Raymond et al., 2015; Reisinger et al., 2018). These simulated tracks provide an estimate of available geographic space from which available habitat (environmental conditions) can be inferred. By comparing the environmental conditions associated with simulated tracks to those of actual tracks, the model seeks to quantify preference. Explicitly, the model identifies Snow Petrel preference for environmental variables by comparing environmental conditions at known locations with a continuous array of points in space and time that reflect available habitat [see eqn. 1 below, Aarts et al. (2012)].
Equation 1:
Here, habitat i denotes a unique point in environmental space X. Usage fu(Xi) and availability fa(Xi) are defined as two continuous probability density functions. Preference w(Xi) is related to the ratio of habitat use over habitat availability . The denominator K is a normalizing constant ensuring that fu(X) integrates to 1 over all of X (Aarts et al., 2012). Put simply, the model compares simulated tracks to actual tracks and uses the environmental differences between them to infer habitat preference.
To ensure that simulated tracks remained in biologically plausible areas, a conservative limit of 40°S (Menkhorst et al., 2017) was set as the northern latitudinal boundary to the simulated tracks. A land mask was also used to constrain simulated tracks to remain over oceanic areas. Environmental variables were then sourced to match true and simulated tracks at values closest to the track date, time, latitude, and longitude. Variables included oceanic parameters such as sea-surface temperature, wind, eddy kinetic energy, and bathymetry that could be ecologically important (Hindell et al., 2020; Skov et al., 2021; Warwick-Evans et al., 2021), as well as Antarctic-specific variables such as sea-ice concentration, distance to open-water, distance to ice-edge, and ice category. Ice category information was based on Fraser et al. (2020) and allocates a value under the following classifications: 0 = pack ice or ocean; 1 = continent; 2 = islands; 3 = ice shelf; 4 = fast ice; 5 = manually derived fast ice edge; and 6 = automatically (i.e., reduced subjectivity) derived fast ice edge. Additionally, a suite of variables was acquired and used as a proxy for large environmental processes. These variables are described in detail in Table 1. Upon completing these steps, there were > 4.5 million data points with relevant environmental information for each point/simulated point.
Following Reisinger et al. (2018), we then used a case-control design that assigned location estimates from real tracks a value of 1, and those from simulated tracks (habitat available to any given individual Snow Petrel) a value of 0. Next, we used boosted regression trees (BRTs) to discriminate between real and simulated tracks based on their environmental covariates. Boosting is an iterative technique that adjusts the weight of an observation based on previous iterations (Boehmke and Greenwell, 2019). This approach vastly improves the predictive performance of a model (Boehmke and Greenwell, 2019), and works well for distribution modelling (Elith et al., 2008).
The output of this model (w(Xi) from equation 1, above), is an estimate of the habitat preference of the environment Xi, associated with the ith data point (Aarts et al., 2008). We used univariate and bivariate partial effects plots to understand the influence of each predictor variable on habitat preference. The birds were expected to vary their habitat preferences over the course of the winter season, but the standard method used for assessing variable importance in BRTs (the sum of the squared improvements over all internal nodes of the trees for which that variable was chosen as the split variable, see e.g. Friedman, 2001) does not easily allow changes in importance to be assessed over time. We therefore generated a bivariate interaction between day of year and each of the other predictor variables and, following the approach used by the vip package in R (Greenwell et al., 2022), calculated the standard deviation of each partial effect for each distinct day of year. This gives a time-varying measure of the importance of each predictor variable. At a time (day of year) when a predictor variable has no influence on the model output, its partial effect will be flat and standard deviation will be zero. The larger a variable’s influence at other times, the greater the range of its partial effect and therefore the larger its standard deviation. Standard deviations were calculated on the response (probability) scale, and so these variable importance are comparable between predictor variables. The steps described above yield a model that statistically describes the difference between the habitats used by the animals and those available to them, thus giving an indication of habitat preference. We wanted to be able to predict from those models using a regional grid of environmental data to obtain a spatial depiction of suitable habitat in the region. To do so, it is also necessary to account for accessibility.
During the breeding period the birds are biologically constrained in terms of how far from their chick they can travel. A grid cell that is far from the colony is less likely to be suitable than one close to the colony, even if the cells contain identical environmental conditions. This distance constraint will likely change over time, becoming less pronounced as the non-breeding period progresses. However, the habitat preference models described above are fitted on training data from grid cells that are all available to the animals (i.e., all data came from cells that were visited by either a real or simulated track). The habitat preference models do not account for the fact that when predicting on new data, some of the cells in the new data might not be accessible to the animals. Accordingly, we fitted a second set of binomial models in a similar fashion to Reisinger et al. (2018), that assume accessibility to be a function solely of distance from the colony. A grid cell was deemed to be accessible if it contained any tracks (real or simulated), and inaccessible if it did not. Probability of accessibility was fitted as a non-increasing function of distance from colony, using the scam package in R (Pya, 2022).
A regional grid for each environmental variable was constructed by first acquiring data (on the native grid of the variable) over the circumpolar region south of 40°S, for the same variables used in fitting the models (Table 1). For time-varying variables, data were acquired for the relevant dates and averaged for each day over the winter period of each respective year. Each regional layer was resampled at a resolution of 0.1 degree to create a set of consistent, regional environmental layers.
Regional maps of suitable habitat were then produced by using the habitat preference models to infer habitat preference over the region using the regional environmental layers. The accessibility models were used to infer the accessibility of each grid cell in the regional grid, and the habitat suitability was calculated as the product of those two (Reisinger et al., 2018; Hindell et al., 2020):
Equation 2:
The habitat suitability values obtained by the procedure described above are not necessarily intuitive to interpret. The mean value of habitat preference is arbitrary and is controlled by the number of simulated tracks that were generated, because this controls the number of zeros in the training data (the number of simulated track points) relative to the number of ones (the number of real track points). To provide more intuitive plots, we rescaled the habitat suitability values following the percentile procedure of Raymond et al. (2015) and Hindell et al. (2020). Habitat suitability values above 80, for example, represent the top 20% (by area) of most suitable habitat.
We assumed that the responses of birds to environmental features were the same regardless of colony or year, meaning that inter-annual or inter-colony differences in habitat usage are the result of differences in environmental conditions, rather than different responses to environmental conditions. We therefore fitted a single model to all data and present habitat suitability maps for these combined data. Notably, we also fitted separate models by year and colony, and present these outputs in the Supplementary Material. Encouragingly, the influence assigned to environmental variables on Snow Petrel presence by the boosted regression models did not show substantial differences between the inter-colony, inter-annual, or combined models.
3 Results
3.1 Deployment duration and distances travelled
Snow Petrels from Béchervaise Island were tracked for 330 ± 12 days. Two individuals were recaptured two years post-deployment after 695 and 701 days, respectively. At Filla Island, the petrels were recaptured after 361 ± 6 days. At both locations, all Snow Petrels had returned to their nests by the 10th of November. One individual performed a complete circumpolar navigation. Tracks are shown in Figure 1. The Snow Petrels from Béchervaise Island travelled on average 86,192 ± 2670 km and 106,077 ± 11,607 km in 2011 and 2012, respectively (Table 2). The difference was not statistically significant (Welch’s t-test, t11,916 = 2.17, p = 0.98). The birds from Filla Island travelled 107,094 ± 16,735 km in 2011. This was not significantly different from the distances of Snow Petrels from Béchervaise Island in 2011 (t7,840 = 10.32, p = 1.0) (Table 2).
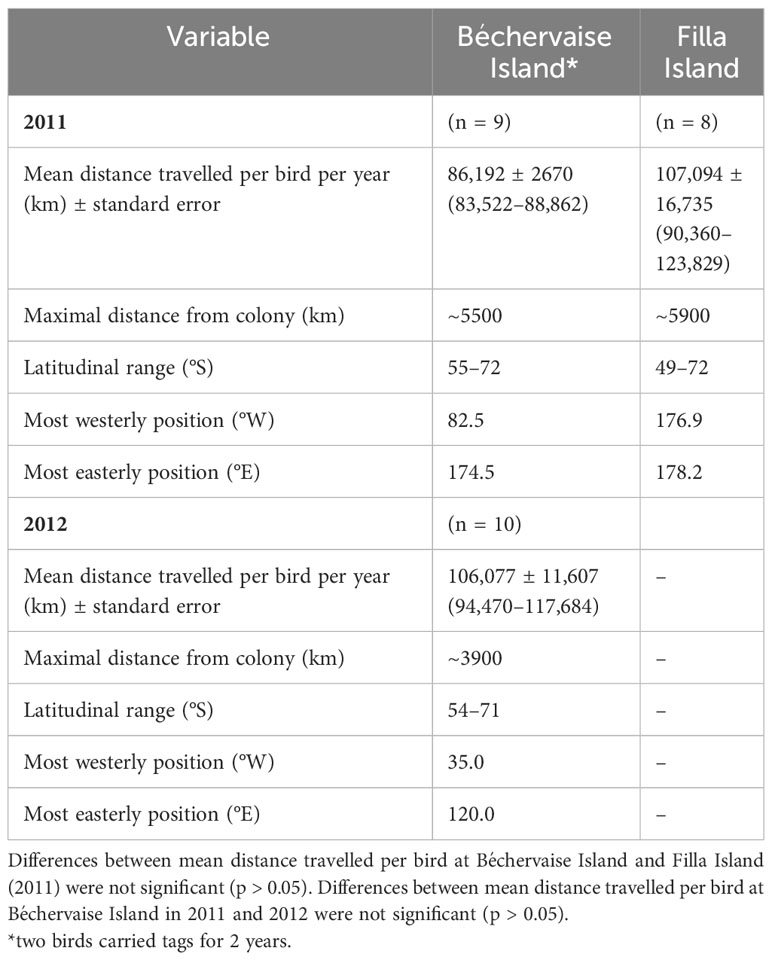
Table 2 Characteristics of winter movements of Snow Petrels Pagodroma nivea from two colonies in East Antarctica.
3.2 At-sea movements
In 2011, the main area used by Snow Petrels from Béchervaise in the non-breeding period covered waters near the southern Kerguelen Plateau and the Valdivia Abyssal Plain (Figure 1, but see also Supplementary Material). Generally, despite covering greater distance, and hosting the greatest vagrant within this study (Table 2), Snow Petrels from Filla Island remained closer to their breeding colony than birds from Béchervaise Island (Figure 2). For birds from both colonies in each year, the monthly distribution of individuals tended to remain within eastern longitudes, and at latitudes south of 54° (Figure 2).
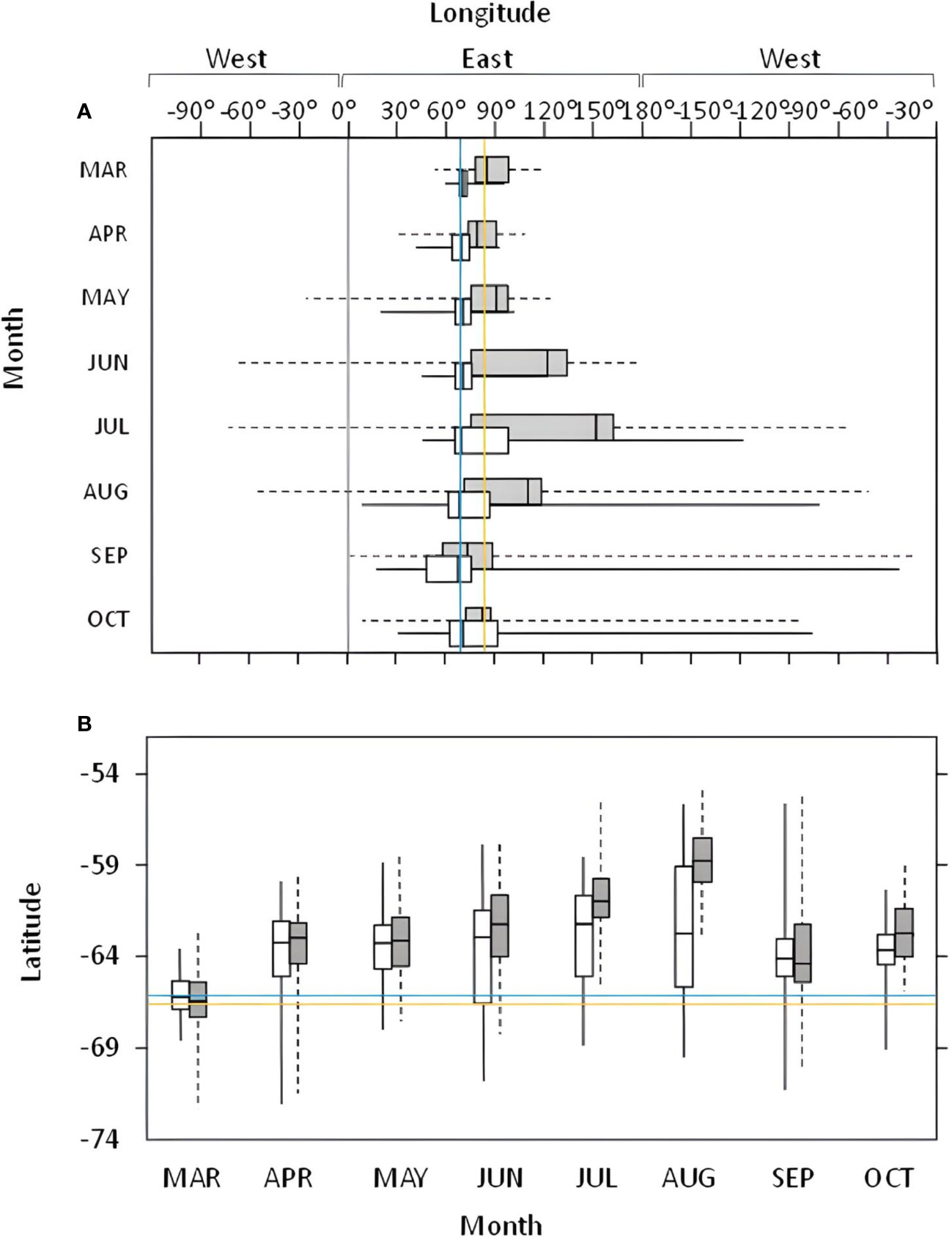
Figure 2 Box plots of monthly longitudinal (A) and latitudinal (B) distribution of Snow Petrels Pagodroma nivea from Béchervaise (grey), and Filla (white) islands in 2011. Colony locations are shown by the blue (Béchervaise), and yellow (Filla) lines. The 0-degree longitude line is shown in grey.
3.3 Activity patterns
Tags remained dry for 55–73% of the time of deployment. Immersion/moisture sensor data alternating between wet and dry were attributed to feeding activity (herein: foraging), and accounted for 21–35% of recordings. The least time was spent with immersed sensors (wet) (7–18%), and such activity was attributed to rafting (resting on the water), preening, or extended feeding events. For all individuals, foraging times were shortest in March to May ranging from 21–27% for birds from Béchervaise Island, and from 22–39% for birds from Filla Island. In the remaining months, foraging status ranged from 30–32% and 31–35% at Béchervaise and Filla, respectively.
Foraging by birds from Filla occurred 43 ± 12% of the time during diurnal hours (57 ± 12% nocturnal). This was slightly lower (higher) than the foraging efforts observed for birds from Béchervaise 48 ± 12% diurnal (52 ± 12% nocturnal) (Figure 3). The diel differences between colony foraging were not statistically significant (ANOVA (day), F = 0.791, df =15, p= 0.389; ANOVA (night), F = 0.856, df =15, p = 0.371). However, throughout the tracking period, foraging activity changed for both colonies. From March until July, foraging activity was predominantly nocturnal (50–75%) and was highest in June and July. Petrels from Béchervaise switched to a diurnal pattern in August while the petrels from Filla did so in September (Figure 3).
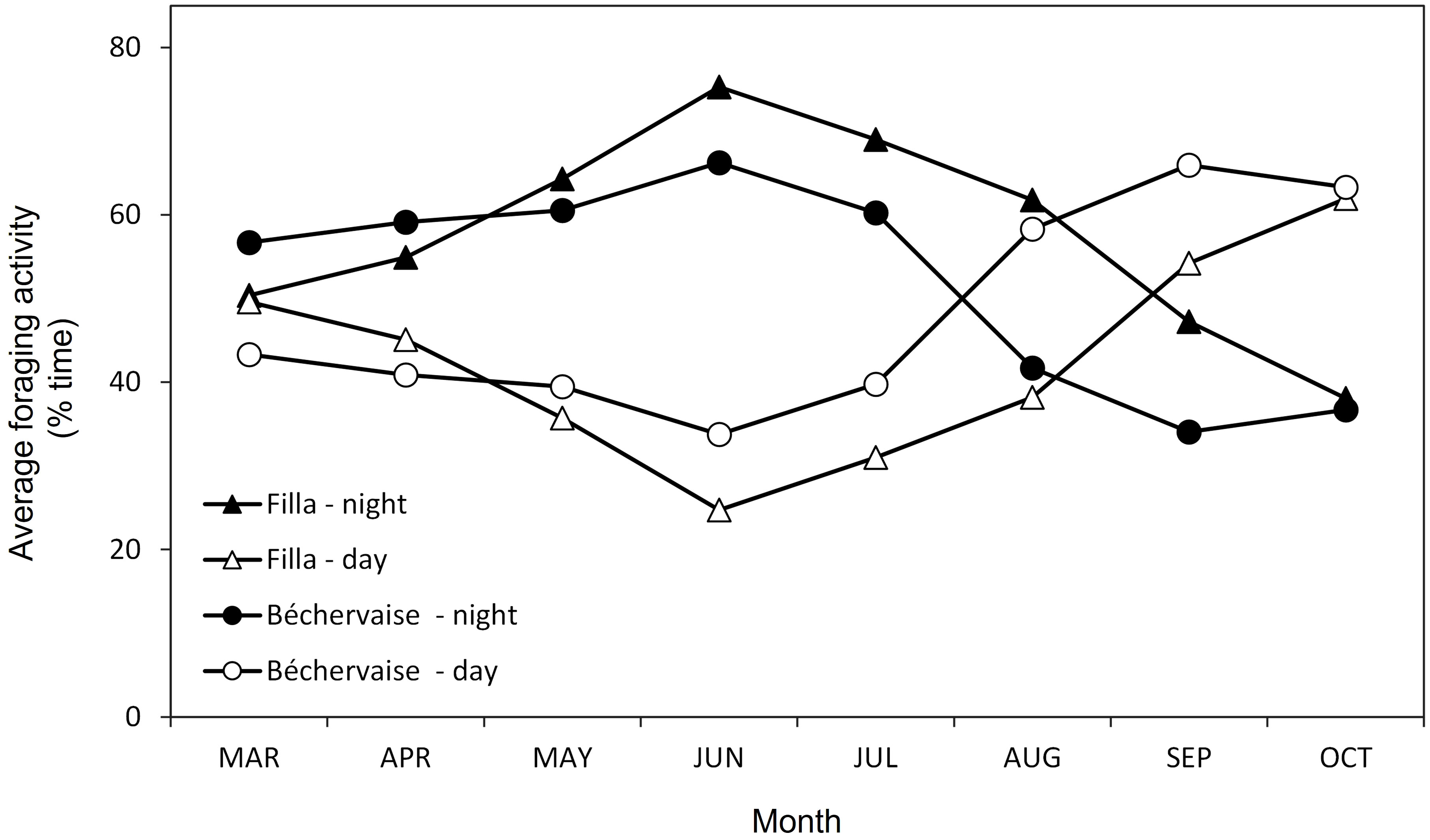
Figure 3 Monthly percentages of diurnal and nocturnal foraging activities of Snow Petrels Pagodroma nivea from Filla and Béchervaise islands in 2011.
3.4 Habitat suitability, preference, and use
The rank importance (ranked by relative importance of variable) of environmental predictors were similar between years and colonies. Bathymetry, sea-surface height, distance to the southern boundary of the Antarctic Circumpolar Current, distance to the ice-edge, sea-ice concentration, and sea-surface temperature were broadly found to be important predictors for single colony models, and the combined model (Table 3). Notably, the partial plots for variable contribution (see Supplementary Material) show that bathymetric depths greater than 5000 meters were associated with more preferred habitat. Such deep features are not habitat features of direct relevance to a surface-feeding species, but rather are indicating the effect of depth on other environmental processes (such as circulation and subsequent sea-ice coverage, Sun et al., 2022).
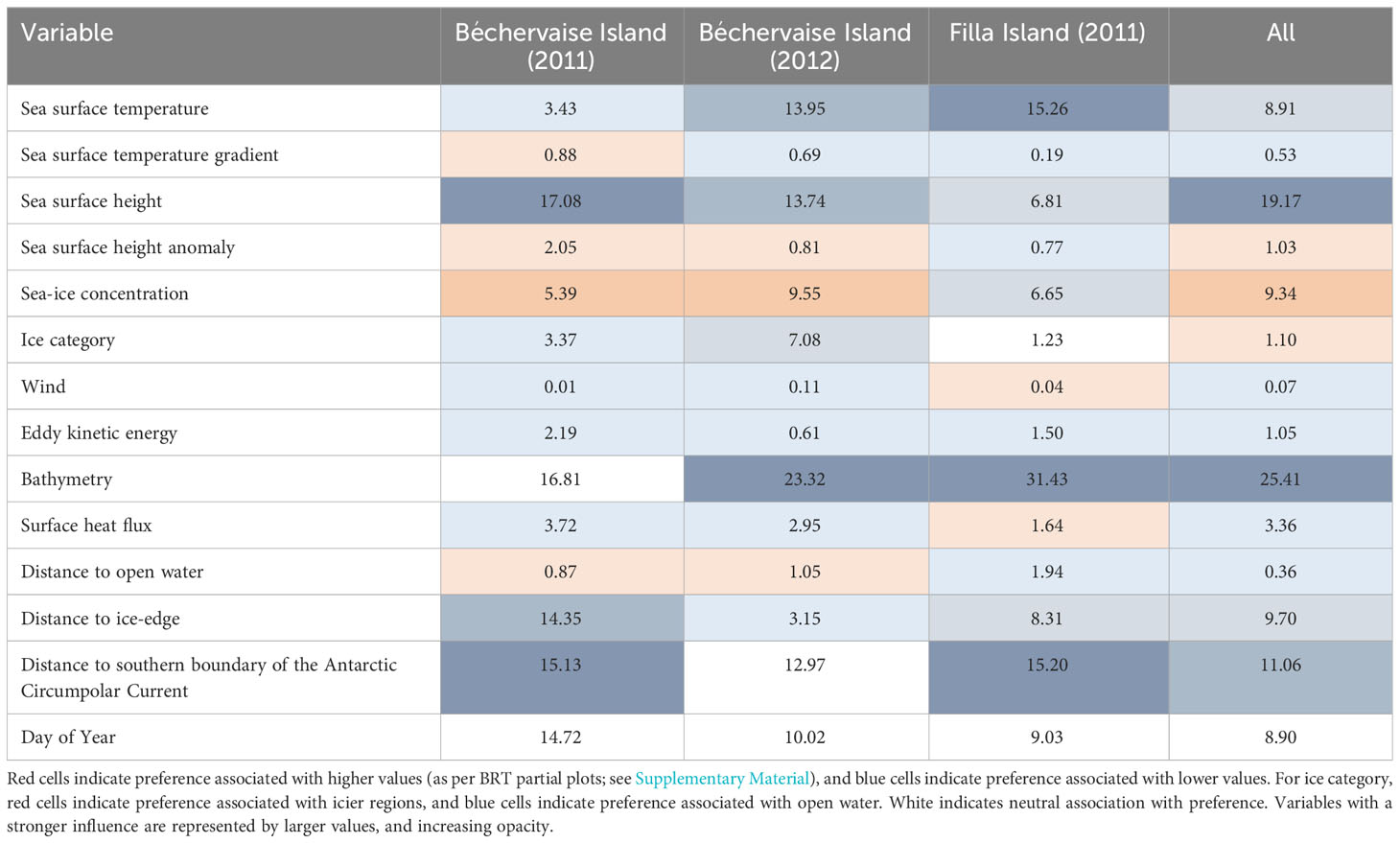
Table 3 Influence of environmental variables on Snow Petrel (Pagodroma nivea) habitat preference as determined by boosted regression tree (BRT) analyses.
The contribution of environmental variables changed temporally, and the importance of some variables, such as distance to the southern boundary of the Antarctic Circumpolar Current were found to be most important between April and May, before sharply dropping off in the later months (Figure 4). Other variables, such as distance to ice edge were consistently important during the non-breeding period (Figure 4).
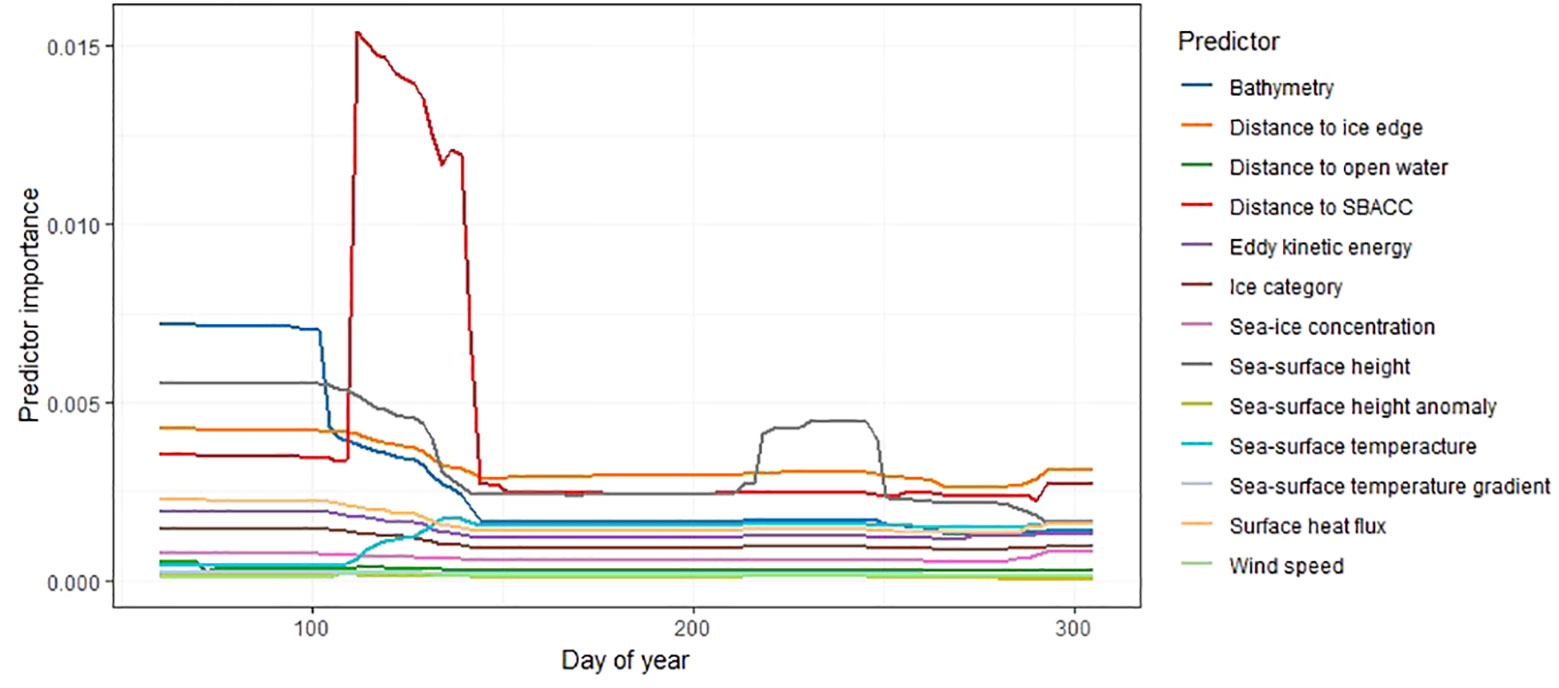
Figure 4 Relative importance of each environmental variable (habitat use) by day of year for all colonies. Environmental variables are shown in the legend on the right, where SBACC refers to distance to the southern boundary of the Antarctic Circumpolar Current. The y-axis values describe the standard deviation of the partial effect for each distinct value of day of year (where 1 would be the 1st of January, and 365 would be the 31st of December – note that dates are restricted from March to October).
Habitat suitability maps for Snow Petrels in the non-breeding period (Figure 5) show that suitable habitat was circumpolar, but with the higher values tending to be in areas closer to breeding sites. In June, suitable cells were observed further away from the coast, and continued to expand around west Antarctica until the end of the non-breeding cycle in October. The maps signify waters over the Kerguelen Plateau, and the Enderby and Valdivia Abyssal Plains as areas highly associated with Snow Petrel habitat suitability (a trend consistent with Figure 2). Highly suitable cells tend to be seen around the Cosmonaut Sea in earlier months, before shifting around to the Cooperation Sea in the later months. Maps and outputs for individual colonies are presented in the Supplementary Material.
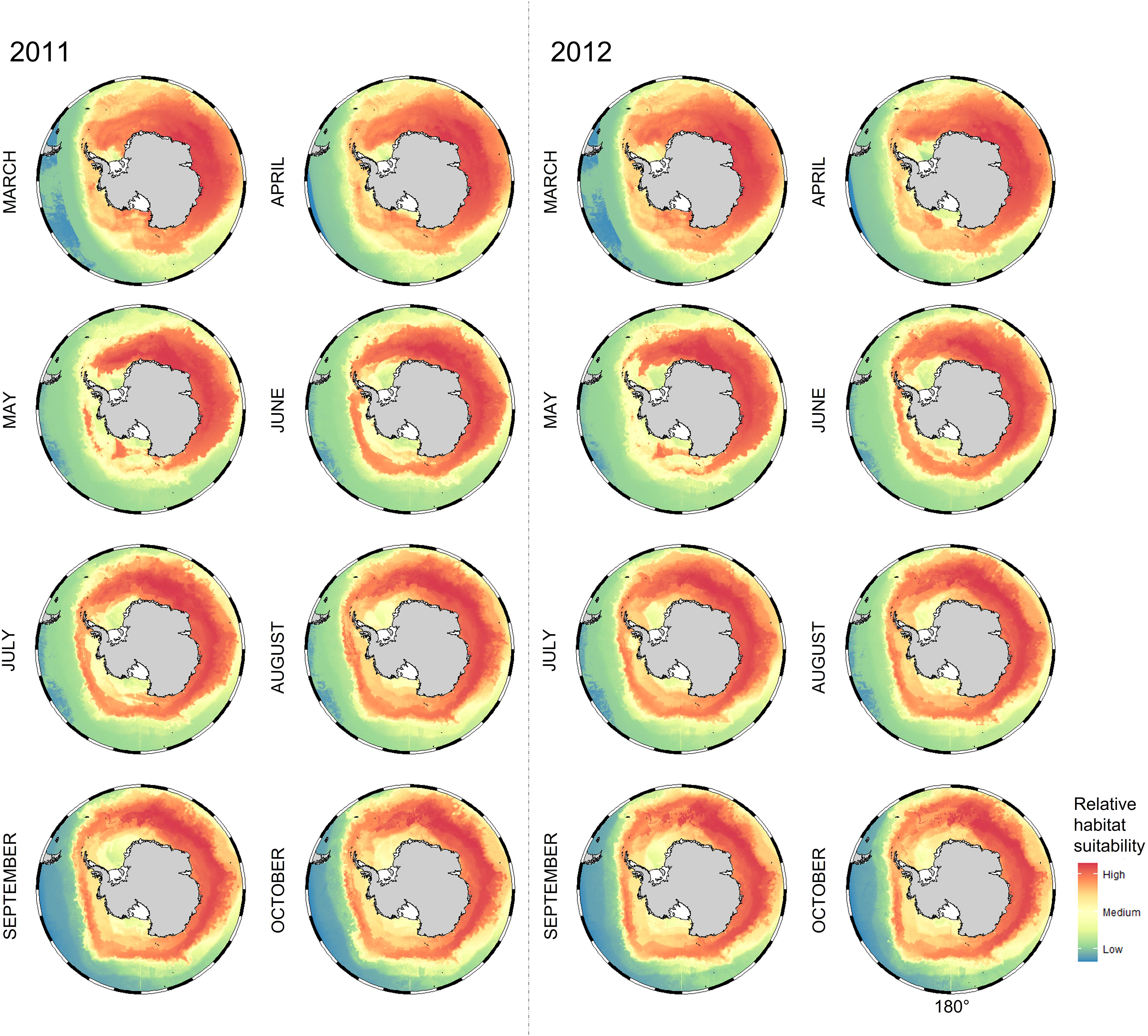
Figure 5 Monthly non-breeding habitat suitability maps for Snow Petrels (Pagodroma nivea) breeding at Béchervaise and Filla Islands in 2011 (left of dotted line) and 2012 (right of dotted line). Redder colors indicate higher habitat suitability. Preferred habitat (i.e. with appropriate environmental conditions) occurs elsewhere around Antarctica but is less likely to be suitable for Snow Petrels from Filla Island and Béchervaise Island given the distance to their respective breeding sites. The numerical values for the legend correspond as follows: High = 80%, Medium = 50%, and Low = 20%.
4 Discussion
This work presents an important step in our understanding of Snow Petrel habitat suitability throughout the non-breeding period for two large colonies in East Antarctica. It provides habitat suitability maps for Snow Petrels during the non-breeding period, and contributes to knowledge of their at-sea winter habitat requirements. The mean distances travelled by adult Snow Petrels were similar across years and between colonies, and in all cases, the mean distance travelled by individuals during the non-breeding period exceeded 80,000 km (Table 2). Individual tracks suggest a wide range of movement strategies throughout the winter – with four of 27 individuals reaching west Antarctica, and one individual performing a complete circumpolar navigation (Figure 1). To our knowledge, this is the first record of a Snow Petrel completing such a feat, though it is not uncommon for other, larger, Antarctic seabirds such as Wandering Albatross (Diomedea exulans) to perform single, double, or even triple circumnavigations over the course of a year (Weimerskirch et al., 2015).
Bathymetry, sea-surface height, distance to the southern boundary of the Antarctic Circumpolar Current, distance to the ice-edge, sea-ice concentration, and sea-surface temperature were important determinants of Snow Petrel habitat preference (Table 3, Figure 4). Areas between the breeding colonies, Cosmonauts Sea, Cooperation Sea, and the southern Kerguelen Plateau were particularly important regions. However, suitable habitat was observed around the entire continent (Figure 5). Given suitability was informed by presence data, this observation suggests Snow Petrels are highly mobile and have generalist habitat requirements throughout the winter, and likely contributes to their existence as the singular species within their genus with a circumpolar distribution [i.e., there has been a lack of environmental pressure to promote speciation. We note that there are two sub-species, but this is subject to debate (Carrea et al., 2019; Kim and Kim, 2020)].
The only other study to assess habitat use by Snow Petrels during the non-breeding period was conducted at Pointe Géologie, Adélie Land, East Antarctica – roughly 2,500 kilometers to the east of Filla Island (Figure 1). The Snow Petrels from Pointe Géologie remained in ice-associated waters, and moved towards similar geographic regions as those described in this paper throughout the non-breeding period (Delord et al., 2016). Notably, the previous study used immersion sensors to understand Snow Petrel activity patterns (Delord et al., 2016). Though our values differ slightly (due to differences in method), our findings on immersion and diurnal activity align with the French study (Figure 3). Snow Petrels from both colonies were predominantly active during nocturnal hours throughout the non-breeding period (Figure 3), and remained in ice-associated waters (Figure 1). Petrels from Béchervaise Island switched to a diurnal pattern in August, and petrels from Filla Island did so in September (Figure 3). This earlier change in cathemeral activity is possibly because birds from Béchervaise Island tended to be farther north than those from Filla Island (Figure 2). Snow Petrels are known to be particularly active during crepuscular hours. It is believed that such activity is associated with the vertical movement of prey (such as Antarctic Krill (Euphausia superba)) towards the sea surface during hours of darkness (Fraser et al., 1989; Delord et al., 2016). It is likely that the nocturnal activity observed in Snow Petrels from Béchervaise, and Filla Islands is also in response to this, though we expect greater foraging success during twilight as Snow Petrels are visual predators and it is slightly lighter at dawn/dusk than during the night.
The comparatively lower foraging activity of Snow Petrels during autumn (March/April, Figure 3) may be related to their complex and protracted annual moult that occurs in several phases. Their primary feathers are moulted in January; by March, all wing and tail feathers have been replaced; flight feathers are replaced continuously without impairing the ability to fly (allowing adults to feed their chicks); and the final phase is the post-breeding moult in late March/April when the body feathers are replaced (Maher, 1962). During this final phase, Snow Petrels may limit their exposure to water as body feathers are crucial for thermoregulation and, at least in some terrestrial birds, the disorder of plumage caused by moult can induce heat loss and increased energy demands (Schieltz and Murphy, 1997). Although the Snow Petrel plumage is very dense and heat loss may not be severe, a disruption to the integrity of the plumage is likely.
Given the foraging potential associated with the Kerguelen Axis (the area extending from the Kerguelen Plateau, over the BANZARE Bank south to east Antarctica) (Constable and Swadling, 2020), and its proximity to our study sites, it is unsurprising that most of the Snow Petrels in this study remained relatively close (< 1000 km) to their breeding area (and thus the Kerguelen region) throughout the non-breeding period (Figures 1, 2, 5). Throughout winter, chlorophyll-a levels are particularly low in the Southern Ocean (Sokolov and Rintoul, 2007). In summer studies, chlorophyll-a levels are routinely used as a proxy for primary productivity in the ocean – where planktivorous species (such as krill, zooplankton, and small fish) are present within their prey fields (Hammond et al., 2020; Hellessey et al., 2020). This is then used to infer biological activity within a region, and it is expected that tertiary consumers (such as Snow Petrels) will follow the secondary consumers (such as planktivores) to the prey fields (Santora et al., 2009). We did not include ocean color as a predictor in the habitat preference models, because satellite-derived chlorophyll-a concentration is not available during times of darkness, or in areas covered by sea ice or cloud, which constituted the majority of our study. Nevertheless, though reduced, phytoplankton production persists in areas where the Antarctic Circumpolar Current interacts with large topographic features such as the Kerguelen Islands (Sokolov and Rintoul, 2007). Thus, the Kerguelen Axis is one of the most important locations for primary production in the region (Arrigo et al., 2008). If chlorophyll-a levels in the Southern Ocean decrease during winter, while areas near the breeding colonies remain productive, it seems unnecessary for a bird to expend additional energy to reach a more distant foraging area. It is more practical for them to rely on a nearby and dependable food source. However, it should be noted that this theory may not apply uniformly to all Snow Petrel colonies, given the variations in conditions between breeding sites and the fact that the south-polar region is not homogeneous (Subramaniam et al., 2022).
The early peak (around April 10th/Day 100) in the importance of distance to the southern boundary of the Antarctic Circumpolar Current (SBACC, Figure 4) is driven by birds from Béchervaise Island in 2011 (see Supplementary Figure 1; herein Figure S1). Many of the environmental predictors deemed to be important for habitat preference are shared between the single colony models and the combined model (Table 3). However, there are temporal differences between colonies regarding the time of year in which each variable is most important (Figure S1). For birds from Béchervaise Island in 2011, SBACC was important throughout the non-breeding period, but most important in the earlier months (Figure S1). This may be associated with the biological productivity, and subsequent foraging potential, linked to the Antarctic Circumpolar Current (Ito et al., 2005; Ribic et al., 2011). We suspect the proximity to SBACC may be more important at the start of the non-breeding period as local (closer) foraging areas could be depleted by foraging effort related to the chick-rearing stages – where shorter flights and larger catches are required to maintain proximity to, and satiety of, newly hatched Snow Petrel chicks. Such localized prey depletion has been observed around breeding areas for other Antarctic seabirds such as the Macaroni Penguin (Eudyptes chrysolophus) (Bedford et al., 2015). However, the quantification of prey-fields is an emerging area of research, and not yet applicable to the areas described within this manuscript.
Bathymetry was overwhelmingly the most important environmental predictor throughout the year for birds from Filla Island in 2011(Figure S1). Bathymetry has previously been recognized as an important environmental feature for other groups of seabirds with variable foraging strategies, such as sulids (Young et al., 2015). It is thought that individual birds may seek out distinct bathymetric features known to increase productivity, or oceanographic features that harbor prey due to bathymetric variability (Young et al., 2015). The same may be true for Snow Petrels.
The temporal importance of environmental predictors was most variable when modelled for birds from Béchervaise Island in 2012 (Figure S1). Almost all variables were highly important at one point of the year, but bathymetry, distance to open water, and sea-surface height were the strongest predictors for habitat preference. These variables are associated with the Antarctic Circumpolar Current and are likely representing preference for biological productivity associated with the current (Sokolov and Rintoul, 2009).
Despite the increase in sea-ice concentrations from March to September, monthly activity remained relatively constant throughout the non-breeding period for birds from both colonies (Figure 3). This suggests that Snow Petrels remain to the north of the sea ice zone, utilize gaps in the sea-ice to forage, or do both. Our “distance to open water” predictor was based on gridded sea ice data (Table 1). The grid size (12.5 km) is sufficient to detect large gaps in the sea-ice, but too coarse to detect leads that are usable by highly mobile flying birds. Notably, we initially conducted analyses using daily leads data (Reiser et al., 2020), but we did not find a strong association between the presence of Snow Petrels and the presence of leads. Consequently, we excluded the leads data from the final results. However, we are confident that future studies, with improved tracking accuracy, will be able to incorporate leads data as an environmental variable. Although our model included a range of sea-ice variables, the mismatch between the spatial resolution of current satellite sensors and the tracking inaccuracies of GLS devices may explain the unexpectedly weak associations between sea-ice variables and the tracking data.
To clarify, the use of GLS tracking devices allowed us to monitor the birds continuously throughout the study period without being limited by battery capacity. However, these devices have relatively poor location accuracy (~186 ± 114 km for flying birds; Phillips et al., 2004). Nonetheless, the scale of our study far exceeded the scale of inaccuracy, and in conjunction with our quantitative approach (Elith et al., 2008; Reisinger et al., 2018; Hindell et al., 2020), the accuracy of GLS devices is unlikely to have significantly impacted the results. Therefore, our findings provide a broad indication of distribution and habitat suitability rather than a detailed account of fine-scale movement dynamics (Quillfeldt et al., 2017).
Future studies in the region will benefit from advancements in battery technology, enabling the use of smaller GPS tracking devices that offer higher accuracy compared to GLS devices (Bernard et al., 2021). These devices will allow researchers to track animals with GPS-scale resolution for extended periods, further enhancing the understanding of animal movements.
It is possible that Snow Petrels spend the majority of their sea-time in productive areas where they forage successfully, but this interpretation is limited to nesting birds (a common limitation in seabird tracking studies (Priddel et al., 2014), as those are the individuals accessible to researchers (i.e., juveniles, pre-breeders, and non-breeders are generally not constrained to a nest and are therefore extremely difficult to retrieve from the same location when attaching/detaching tracking devices). State-space modelling of GPS tracking data would allow researchers to identify specific “foraging only” regions [i.e., environmental features actively targeted by the birds; Halpin et al. (2022)]. Moreover, with the emergence of circumpolar databases that increasingly provide information on mesopelagic fish species (Woods et al., 2022), and krill (Atkinson et al., 2017), relevant seabird studies whose data overlap with prey data could gain valuable insight on which prey species are most commonly associated with seabird presence. Ideally, these foraging areas are regions that should be considered in conservation management planning (Thaxter et al., 2012; Veit and Harrison, 2017). Other species of seabird, such as penguins and shearwaters, associate with areas of subsurface productivity influenced by topographic features (Raymond et al., 2010; Kokubun et al., 2015; Riaz et al., 2021), and it is possible that Snow Petrels do the same. Subsurface productivity is not well detected by the satellite chlorophyll-a sensors currently available, but could also be considered in future works. Interestingly, the ‘knots’ observed in the flight paths of tracked Snow Petrels (Figure 1), may indicate the use of icebergs for roosting and temporary foraging mounts. Snow Petrels are well known to roost on icebergs (Brown, 1966), and increased primary productivity has been shown to occur around icebergs (Hopwood et al., 2019; Liniger et al., 2020; Smith et al., 2007). Such enhanced primary productivity could lead to hotspots of bountiful prey, distant from breeding locations.
Our study presents the first detailed analysis of environmental variables associated with Snow Petrel presence throughout the winter in East Antarctica using colonies near Mawson (Béchervaise) and Davis (Filla) stations. Congruent with other ecological studies in the region (Bestley et al., 2018; Hindell et al., 2020), our research demonstrates the great biological importance of the Kerguelen axis. The Kerguelen region is subject to both research and commercial fishing activity (Hill et al., 2017; Tixier et al., 2019; Subramaniam et al., 2022). Preliminary studies show little impact from commercial fishing activities on ecosystem interactions (Subramaniam et al., 2020). However, more research is needed to disentangle the influence of human activity and natural variation in the region on ecosystems and their inhabitants such as Snow Petrels (Subramaniam et al., 2022). The single most important habitat feature for fulmarine petrels (including Snow Petrels) to persist on Earth’s coldest continent is an abundance of food (Weathers et al., 2000). Although Antarctic Petrels (Thalassoica antarctica) and Snow Petrels are better insulated than lower-latitude species, their best adaptation to dealing with cold environments is an increased metabolic rate (Weathers et al., 2000). When combined with plasticity in behavior, and variations between individuals regarding habitat use, Snow Petrels may be able to overcome challenges associated with anthropogenic climate change provided their prey fields remain plentiful. Once a more comprehensive understanding of Snow Petrel at-sea habitat use has been realized, we also recommend that future studies seek to explore how the suitability of Snow Petrel habitat will change under different climate scenarios. In any case, to give the Snow Petrel the best possible chance of adapting to climate change, we must ensure it has time to do so. As with other wide-ranging species, protecting their prey availability and suitable nesting habitat will contribute to their conservation (Guixé and Arroyo, 2011).
Data availability statement
The datasets presented in this study can be found in online repositories. The names of the repository/repositories and accession number(s) can be found below: http://dx.doi.org/doi:10.4225/15/518831951455A Wienecke, B. (2013) GLS tag deployments on Snow petrels (Pagodroma nivea) in 2011 and 2012 from Bechervaise Island, Mawson Coast and Filla Island, Rauer Group, Ver. 1, Australian Antarctic Data Centre - ASAC_2722_SP_GLS.
Ethics statement
All applicable international, national, and/or institutional guidelines for the care and use of animals were followed. All animal handling and attachment of device procedures were approved by the Australian Government Antarctic Animal Ethics Committee through Australian Antarctic Science (AAS) projects # 2722 and 4087. The study was conducted in accordance with the local legislation and institutional requirements.
Author contributions
BV: Conceptualization, Data curation, Formal Analysis, Funding acquisition, Investigation, Methodology, Project administration, Resources, Software, Validation, Visualization, Writing – original draft, Writing – review & editing. BW: Conceptualization, Formal Analysis, Investigation, Project administration, Resources, Supervision, Validation, Visualization, Writing – review & editing. CG: Formal Analysis, Investigation, Methodology, Software, Validation, Writing – review & editing. SC: Funding acquisition, Investigation, Project administration, Resources, Supervision, Validation, Writing – review & editing. BR: Data curation, Formal Analysis, Investigation, Methodology, Resources, Software, Validation, Visualization, Writing – review & editing. CS: Conceptualization, Funding acquisition, Investigation, Project administration, Resources, Supervision, Validation, Writing – review & editing. MS: Data curation, Investigation, Resources, Software, Validation, Writing – review & editing. PV: Investigation, Supervision, Validation, Writing – review & editing. SW: Data curation, Formal Analysis, Investigation, Methodology, Software, Validation, Visualization, Writing – review & editing. LE: Conceptualization, Funding acquisition, Investigation, Project administration, Resources, Supervision, Validation, Writing – review & editing.
Funding
The author(s) declare financial support was received for the research, authorship, and/or publication of this article. This work was funded by the Australian Antarctic Division AAS projects # 2722 and 4087 and a post- graduate scholarship for BV from University of Tasmania.
Acknowledgments
We thank S. Doust, J. McInnes, H. Achurch, and L. Einoder for assistance in the field, and the ATAAMS team for early discussions surrounding this work. We extend thanks to N. Kelly, D. Fierro-Arcos, T. Travers, D. Green, A. Henderson, B. Woods, A. Fraser, J. Lieser, and J. Cleeland for fruitful discussions, and technical assistance with this work. We also thank S. Willmes for providing us with daily leads data for initial models that were not included in the final output. Finally, we thank the Australian Antarctic Division for funding and logistics support to deliver this work as a contribution to AAS projects # 2722, 4087, and 4518, and the Integrated Digital East Antarctica program. The work was carried out according to permits and conditions issued under the Australian Antarctic Treaty (Environmental Protection) Act 1980 (Permit numbers ATEP-4087-2011/12 and ATEP-4087-2012/13). This research was supported by use of the Nectar Research Cloud, a collaborative Australian research platform supported by the NCRIS-funded Australian Research Data Commons (ARDC).
Conflict of interest
The authors declare that the research was conducted in the absence of any commercial or financial relationships that could be construed as a potential conflict of interest.
Publisher’s note
All claims expressed in this article are solely those of the authors and do not necessarily represent those of their affiliated organizations, or those of the publisher, the editors and the reviewers. Any product that may be evaluated in this article, or claim that may be made by its manufacturer, is not guaranteed or endorsed by the publisher.
Supplementary material
The Supplementary Material for this article can be found online at: https://www.frontiersin.org/articles/10.3389/fmars.2023.1278229/full#supplementary-material
References
Aarts G., Fieberg J., Matthiopoulos J. (2012). Comparative interpretation of count, presence–absence and point methods for species distribution models. Methods Ecol. Evol. 3 (1), 177–187. doi: 10.1111/j.2041-210X.2011.00141.x
Aarts G., MacKenzie M., McConnell B., Fedak M., Matthiopoulos J. (2008). Estimating space-use and habitat preference from wildlife telemetry data. Ecography 31, 140–160. doi: 10.1111/j.2007.0906-7590.05236.x
Ainley D. G., Ribic C. A., Spear L. B. (1993). Species-Habitat Relationships among Antarctic Seabirds: A Function of Physical or Biological Factors? Condor 95 (4), 806–816. doi: 10.2307/1369419
Ainley D. G., Ribic C. A., Woehler E. J. (2012). Adding the ocean to the study of seabirds: a brief history of at-sea seabird research. Mar. Ecol. Prog. Ser. 451, 231–243. doi: 10.3354/meps09524
Amundsen T. (1995). Egg Size and Early Nestling Growth in the Snow Petrel. Condor 97 (2), 345–351. doi: 10.2307/1369020
Arrigo K. R., van Dijken G. L., Bushinsky S. (2008). Primary production in the Southern Ocean 1997–2006. J. Geophysical Res.: Oceans 113 (C8). doi: 10.1029/2007JC004551
Atkinson A., Hill S. L., Pakhomov E. A., Anadon R., Chiba S., Daly K. L., et al. (2017). KRILLBASE: a circumpolar database of Antarctic krill and salp numerical densities 1926-2016. 9 (1), 193–210. doi: 10.5194/essd-9-193-2017
Barbraud C., Chastel O. (1999). Early body condition and hatching success in the snow petrel Pagodroma nivea. Polar Biol. 21 (1), 1–4. doi: 10.1007/s003000050326
Bedford M., Melbourne-Thomas J., Corney S., Jarvis T., Kelly N., & Constable A. (2015). Prey-field use by a Southern Ocean top predator: enhanced understanding using integrated datasets. Mar. Ecol. Prog. Ser. 526, 169–181. doi: 10.3354/meps11203
Berg S., White D., Hermichen W.-D., Emmerson L. (2019). Late Holocene colonisation of snow petrels (Pagodroma nivea) of the Prince Charles Mountains, Antarctica. Polar Biol. 42, 1167–1173. doi: 10.1007/s00300-019-02509-0
Bernard A., Rodrigues A. S. L., Cazalis V., Grémillet D. (2021). Toward a global strategy for seabird tracking. Conserv. Lett. 14 (3), e12804. doi: 10.1111/conl.12804
Bestley S., Raymond B., Gales N. J., Harcourt R. G., Hindell M. A., Jonsen I. D., et al. (2018). Predicting krill swarm characteristics important for marine predators foraging off East Antarctica. Ecography 41 (6), 996–1012. doi: 10.1111/ecog.03080
Boehmke B., Greenwell B. (2019). Hands-on machine learning with R (1 ed.) (New York, USA: Chapman and Hall/CRC).
Bondavalli C., Ulanowicz R. E. (1999). Unexpected effects of predators upon their prey: the case of the American alligator. Ecosystems 2 (1), 49–63. doi: 10.1007/s100219900057
Brown D. A. (1966). Breeding biology of the snow petrel pagodroma nivea (forster). ANARE Sci. Rep. 89, 1–63.
Brown R. G. B. (1980). “Seabirds as Marine Animals,” in Behavior of Marine Animals: Current Perspectives in Research. Marine Birds. Eds. Burger J., Olla B. L., Winn H. E. (US: Springer), 1–39.
Burger J., Gochfeld M. (2004). Marine Birds as Sentinels of Environmental Pollution. EcoHealth 1 (3), 263–274. doi: 10.1007/s10393-004-0096-4
Carrea C., Burridge C. P., Wienecke B., Emmerson L. M., White D., Miller K. J. (2019). High vagility facilitates population persistence and expansion prior to the Last Glacial Maximum in an antarctic top predator: The Snow petrel (Pagodroma nivea). J. Biogeography 46 (2), 442–453. doi: 10.1111/jbi.13513
Clarke A., Barnes D. K. A., Hodgson D. A. (2005). How isolated is Antarctica? Trends Ecol. Evol. 20 (1), 1–3. doi: 10.1016/j.tree.2004.10.004
Constable A., Swadling K. M. (2020). Ecosystem drivers of food webs on the Kerguelen Axis of the Southern Ocean. Deep Sea Res. Part II: Topical Stud. Oceanography 174, e104790. doi: 10.1016/j.dsr2.2020.104790
Cordero Quirós N., Jacox M. G., Pozo Buli M., Bograd S. J. (2022). Future Changes in Eddy Kinetic Energy in the California Current System From Dynamically Downscaled Climate Projections. Geophysical Res. Lett. 49 (21), e2022gl099042. doi: 10.1029/2022GL099042
Cox S. L., Embling C. B., Hosegood P. J., Votier S. C., Ingram S. N. (2018). Oceanographic drivers of marine mammal and seabird habitat-use across shelf-seas: A guide to key features and recommendations for future research and conservation management. Estuarine Coast. Shelf Sci. 212, 294–310. doi: 10.1016/j.ecss.2018.06.022
Cropper T. E., Hanna E., Bigg G. R. (2014). Spatial and temporal seasonal trends in coastal upwelling off Northwest Africa 1981–2012. Deep Sea Res. Part I: Oceanographic Res. Papers 86, 94–111. doi: 10.1016/j.dsr.2014.01.007
Croxall J., Steele W., McInnes S., Prince P. (1995). Breeding distribution of the Snow Petrel Pagodroma nivea. Mar. Ornithol. 23, 69–100.
Davis S. E., Maftei M., Mallory M. L. (2016). Migratory Connectivity at High Latitudes: Sabine’s Gulls (Xema sabini) from a Colony in the Canadian High Arctic Migrate to Different Oceans. PloS One 11 (12), e0166043. doi: 10.1371/journal.pone.0166043
Delord K., Pinet P., Pinaud D., Barbraud C., De Grissac S., Lewden A., et al. (2016). Species-specific foraging strategies and segregation mechanisms of sympatric Antarctic fulmarine petrels throughout the annual cycle. Ibis 158 (3), 569–586. doi: 10.1111/ibi.12365
Einoder L. D., Emmerson L. M., Southwell D. M., Southwell C. J. (2014). Cavity characteristics and ice accumulation affect nest selection and breeding in snow petrels Pagodroma nivea. Mar. Ornithol. 42, 175–182.
Elith J., Leathwick J. R., Hastie T. (2008). A working guide to boosted regression trees. J. Anim. Ecol. 77 (4), 802–813. doi: 10.1111/j.1365-2656.2008.01390.x
Emmerson L., Southwell C., Clarke J., Tierney M., Kerry K. (2015). Adélie penguin response parameters signal reduced prey accessibility: implications for predator–prey response curves. Mar. Biol. 162, 1187–1200. doi: 10.1007/s00227-015-2661-5
Fraser A. D., Massom R. A., Ohshima K. I., Willmes S., Kappes P. J., Cartwright J., et al. (2020). High-resolution mapping of circum-Antarctic landfast sea ice distribution 2000–2018. Earth Syst. Sci. Data 12 (4), 2987–2999. doi: 10.5194/essd-12-2987-2020
Fraser W. R., Pitman R. L., Ainley D. G. (1989). Seabird and fur seal responses to vertically migrating winter krill swarms in Antarctica. Polar Biol. 10 (1), 37–41. doi: 10.1007/BF00238288
Friedman J. H. (2001). “Greedy Function Approximation: A Gradient Boosting Machine,”. Ann. Stat 29 (5), 1189–1232. doi: 10.1214/aos/1013203451
Furness R. W., Camphuysen K. (1997). Seabirds as monitors of the marine environment. ICES J. Mar. Sci. 54 (4), 726–737. doi: 10.1006/jmsc.1997.0243
Gilg O., Moe B., Hanssen S. A., Schmidt N. M., Sittler B., Hansen J., et al. (2013). Trans-Equatorial Migration Routes, Staging Sites and Wintering Areas of a High-Arctic Avian Predator: The Long-tailed Skua (Stercorarius longicaudus). PloS One 8 (5), e64614. doi: 10.1371/journal.pone.0064614
Goldsworthy P., Thomson P. (2000). An extreme inland breeding locality of snow petrels (Pagodroma nivea) in the southern Prince Charles Mountains, Antarctica. Polar Biol. 23, 717–720. doi: 10.1007/s003000000146
González-Solís J., Croxall J. P., Oro D., Ruiz X. (2007). Trans-equatorial migration and mixing in the wintering areas of a pelagic seabird. Front. Ecol. Environ. 5 (6), 297–301. doi: 10.1890/1540-9295(2007)5[297:TMAMIT]2.0.CO;2
Greenwell B., Boehmke B., Gray B. (2022). Package 'vip'. R Package version 0.3.2 (Auckland, New Zealand: CRAN).
Guixé D., Arroyo B. (2011). Appropriateness of Special Protection Areas for wide-ranging species: the importance of scale and protecting foraging, not just nesting habitats. Anim. Conserv. 14 (4), 391–399. doi: 10.1111/j.1469-1795.2011.00441.x
Halpin L. R., Mott R., Clay T. A., Humphries G. R. W., Chatwin T. A., Carlile N., et al. (2022). Predicting the Foraging Habitats of Sympatrically Breeding Gadfly Petrels in the South Pacific Ocean. Front. Mar. Sci. 9. doi: 10.3389/fmars.2022.853104
Hammond M. L., Beaulieu C., Henson S. A., Sahu S. K. (2020). Regional surface chlorophyll trends and uncertainties in the global ocean. Sci. Rep. 10 (1), 15273. doi: 10.1038/s41598-020-72073-9
Harrison P., Perrow M., Larsson H. (2021). Seabirds: The New Identification Guide (Barcelona, Spain: Lynx Edicions).
Hawkes L. A., Witt M. J., Broderick A. C., Coker J. W., Coyne M. S., Dodd M., et al. (2011). Home on the range: spatial ecology of loggerhead turtles in Atlantic waters of the USA. Diversity Distributions 17 (4), 624–640. doi: 10.1111/j.1472-4642.2011.00768.x
Hazen E. L., Abrahms B., Brodie S., Carroll G., Jacox M. G., Savoca M. S., et al. (2019). Marine top predators as climate and ecosystem sentinels. Front. Ecol. Environ. 17 (10), 565–574. doi: 10.1002/fee.2125
Hedd A., Montevecchi W. A., Otley H., Phillips R. A., Fifield D. A. (2012). Trans-equatorial migration and habitat use by sooty shearwaters Puffinus griseus from the South Atlantic during the nonbreeding season. Mar. Ecol. Prog. Ser. 449, 277–290. doi: 10.3354/meps09538
Hellessey N., Johnson R., Ericson J. A., Nichols P. D., Kawaguchi S., Nicol S., et al. (2020). Antarctic krill lipid and fatty acid content variability is associated to satellite derived chlorophyll-a and sea surface temperatures. Sci. Rep. 10 (1), 1–11. doi: 10.1038/s41598-020-62800-7
Hill N. A., Foster S. D., Duhamel G., Welsford D., Koubbi P., Johnson C. R. (2017). Model-based mapping of assemblages for ecology and conservation management: A case study of demersal fish on the Kerguelen Plateau. Diversity Distributions 23 (10), 1216–1230. doi: 10.1111/ddi.12613
Hindell M. A., Reisinger R. R., Ropert-Coudert Y., Hückstädt L. A., Trathan P. N., Bornemann H., et al. (2020). Tracking of marine predators to protect Southern Ocean ecosystems. Nature 580 (7801), 87–92. doi: 10.1038/s41586-020-2126-y
Hipfner J. M., Prill M. M., Studholme K. R., Domalik A. D., Tucker S., Jardine C., et al. (2020). Geolocator tagging links distributions in the non-breeding season to population genetic structure in a sentinel North Pacific seabird. PloS One 15 (11), e0240056. doi: 10.1371/journal.pone.0240056
Hopwood M. J., Carroll D., Höfer J., Achterberg E. P., Meire L., Le Moigne F. A. C., et al. (2019). Highly variable iron content modulates iceberg-ocean fertilisation and potential carbon export. Nat. Commun. 10, 5261. doi: 10.1038/s41467-019-13231-0
Ito T., Parekh P., Durkiewicz S., & Follows M. J. (2005). The Antarctic Circumpolar Productivity Belt. Geophysical Res. Lett. 32 (13), L13604. doi: 10.1029/2005GL023021
Jaeger A., Feare C. J., Summers R. W., Lebarbenchon L., Larose C. S., Le Corre M. (2017). Geolocation Reveals Year-Round at-Sea Distribution and Activity of a Superabundant Tropical Seabird, the Sooty Tern Onychoprion fuscatus. Front. Mar. Sci. 394 (4). doi: 10.3389/fmars.2017.00394
Jonsen I., Bestley S., Wotherspoon S., Sumner M., Flemming J. (2015). bsam: Bayesian state-space models for animal movement. R package. R Foundation for Statistical Computing version 0.43, 1 (Auckland, New Zealand: CRAN).
Kim J.-U., Kim J.-H. (2020). Complete mitochondrial genome of the snow petrel, Pagodroma nivea. Mitochondrial DNA Part B. 5 (3), 3337–3338. doi: 10.1080/23802359.2020.1820389
Kokubun N., Lee W. Y., Kim J. H., Takahashi A. (2015). Chinstrap penguin foraging area associated with a seamount in Bransfield Strait, Antarctica. Polar Sci. 9 (4), 393–400. doi: 10.1016/j.polar.2015.10.001
Liniger G., Strutton P. G., Lannuzel D., Moreau S. (2020). Calving event led to changes in phytoplankton bloom phenology in the Mertz Polynya, Antarctica. J. Geophys. Res.: Oceans 125 (11), e2020JC016387. doi: 10.1029/2020JC016387
Lisovski S., Hahn S. (2012). GeoLight – processing and analysing light-based geolocator data in R. Methods Ecol. Evol. 3 (6), 1055–1059. doi: 10.1111/j.2041-210X.2012.00248.x
MacLeod C., Adams J., Lyver P. (2008). At-sea distribution of satellite-tracked Grey-faced Petrels, Pterodroma macroptera gouldi, captured on the Ruamaahua (Aldermen) Islands, New Zealand. Papers Proc. R. Soc. Tasmania 142 (1), 73–88. doi: 10.26749/rstpp.142.1.73
Maher W. J. (1962). Breeding Biology of the Snow Petrel near Cape Hallett, Antarctica. Condor 64 (6), 488–499. doi: 10.2307/1365472
Malpress V., Bestley S., Corney S., Welsford D., Labrousse S., Sumner M., et al. (2017). Bio-physical characterisation of polynyas as a key foraging habitat for juvenile male southern elephant seals (Mirounga leonina) in Prydz Bay, East Antarctica. PloS One 12 (9), e0184536. doi: 10.1371/journal.pone.0184536
McClymont E. L., Bentley M. J., Hodgson D. A., Spencer-Jones C. L., Wardley T., West M. D., et al. (2021). Summer sea-ice variability on the Antarctic margin during the last glacial period reconstructed from snow petrel (Pagodroma nivea) stomach-oil deposits. Clim. Past Discuss 2021, 1–39. doi: 10.5194/cp-2021-134
Menkhorst P., Rogers D., Clarke R., Davies J., Marsack P., Franklin K. (2017). The Australian Bird Guide (Clayton, Australia: CSIRO Publishing).
Moe B., Angelier F., Bech C., Chastel O. (2007). Is basal metabolic rate influenced by age in a long-lived seabird, the snow petrel? J. Exp. Biol. 210 (19), 3407–3414. doi: 10.1242/jeb.005090
Olivier F., Lee A. V., Woehler E. J. (2004). Distribution and abundance of snow petrels Pagodroma nivea in the Windmill Islands, East Antarctica. Polar Biol. 27 (5), 257–265. doi: 10.1007/s00300-004-0595-3
Park Y.-H., Durand I. (2019). Altimetry-drived Antarctic Circumpolar Current fronts (Plouzané, France: SEANOE).
Phillips R. A., Silk J. R. D., Croxall J. P., Afanasyev V., Briggs D. R. (2004). Accuracy of geolocation estimates for flying seabirds. Mar. Ecol. Prog. Ser. 266, 265–272. doi: 10.3354/meps266265
Piatt J. F., Sydeman W. J., Wiese F. (2007). Seabirds as indicators of marine ecosystems. Mar. Ecol. Prog. Ser. 352, 199–204. doi: 10.3354/meps07070
Pinet P., Jaquemet S., Pinaud D., Weimerskirch H., Phillips R. A., Le Corre M. (2011). Migration, wintering distribution and habitat use of an endangered tropical seabird, Barau’s petrel Pterodroma baraui. Mar. Ecol. Prog. Ser. 423, 291–302. doi: 10.3354/meps08971
Poland J. S., Riddle M. J., Zeeb B. A. (2003). Contaminants in the Arctic and the Antarctic: a comparison of sources, impacts, and remediation options. Polar Rec. 39 (4), 369–383. doi: 10.1017/S0032247403002985
Post E., Bhatt U. S., Bitz C. M., Brodie J. F., Fulton T. L., Hebblewhite M., et al. (2013). Ecological Consequences of Sea-Ice Decline. Science 341 (6145), 519–524. doi: 10.1126/science.1235225
Priddel D., Carlile N., Portelli D., Kim Y., O'Neill L., Bretagnolle V., et al. (2014). Pelagic distribution of Gould's Petrel (Pterodroma leucoptera): linking shipboard and onshore observations with remote-tracking data. Emu 114 (4), 360–370. doi: 10.1071/MU14021
Puskic P., Lavers J. L., Adams L. R., Grünenwald M., Hutton I., & Bond A. L. (2019). Uncovering the sub-lethal impacts of plastic ingestion by shearwaters using fatty acid analysis. Conserv. Physiol. 7 (1), e107. doi: 10.1093/conphys/coz017
Quillfeldt P., Engler J. O., Silk J. R. D., Phillips R. A. (2017). Influence of device accuracy and choice of algorithm for species distribution modelling of seabirds: a case study using black-browed albatrosses. J. Avian Biol. 48 (12), 1549–1555. doi: 10.1111/jav.01238
Raymond B., Lea M. A., Patterson T., Andrews-Goff V., Sharples R., Charrassin J.-B., et al. (2015). Important marine habitat off East Antarctica revealed by two decades of multi-species predator tracking. Ecography 38 (2), 121–129. doi: 10.1111/ecog.01021
Raymond B., Shaffer S. A., Sokolov S., Woehler E. J., Costa D. P., Einoder L., et al. (2010). Shearwater Foraging in the Southern Ocean: The Roles of Prey Availability and Winss. PloS One 5 (6), e10960. doi: 10.1371/journal.pone.0010960
Rayner M. J., Hauber M. E., Clout M. N., Seldon D. S., Van Dijken S., Bury S., et al. (2008). Foraging ecology of the Cook’s petrel Pterodroma cookii during the austral breeding season: a comparison of its two populations. Mar. Ecol. Prog. Ser. 370, 271–284. doi: 10.3354/meps07660
Reiser F., Willmes S., Heinemann G. (2020). A New Algorithm for Daily Sea Ice Lead Identification in the Arctic and Antarctic Winter from Thermal-Infrared Satellite Imagery. Remote Sens. 12 (12), 1957. doi: 10.3390/rs12121957
Reisinger R. R., Raymond B., Hindell M. A., Bester M. N., Crawford R. J. M., Davies D., et al. (2018). Habitat modelling of tracking data from multiple marine predators identifies important areas in the Southern Indian Ocean. Diversity Distributions 24 (4), 535–550. doi: 10.1111/ddi.12702
Reudink M. W., Marra P. P., Kyser T. K., Boag P. T., Langin K. M., Ratcliffe L. M. (2009). Non-breeding season events influence sexual selection in a long-distance migratory bird. Proc. R. Soc. B.: Biol. Sci. 276 (1662), 1619–1626. doi: 10.1098/rspb.2008.1452
Riaz J., Bestley S., Wotherspoon S., Emmerson L. (2021). Horizontal-vertical movement relationships: Adélie penguins forage continuously throughout provisioning trips. Movement Ecol. 9 (1), 43. doi: 10.1186/s40462-021-00280-8
Ribic C. A., Ainley D. G., Ford R. G., Fraser W. R., Tynan C. T., Woehler E. J. (2011). Water masses, ocean fronts, and the structure of Antarctic seabird communities: Putting the eastern Bellingshausen Sea in perspective. Deep Sea Res. Part II 58 (13-16), 1695–1709. doi: 10.1016/j.dsr2.2009.09.017
Roncon G., Bestley S., McMahon C. R., Wienecke B., Hindell M. A. (2018). View From Below: Inferring Behavior and Physiology of Southern Ocean Marine Predators From Dive Telemetry [Review]. Front. Mar. Sci. 5 (464). doi: 10.3389/fmars.2018.00464
Santora J. A., Reiss C. S., Cossio A. M., Veit R. R. (2009). Interannual spatial variability of krill (Euphausia superba) influences seabird foraging behavior near Elephant Island, Antarctica. Fisheries Oceanography 18 (1), 20–35. doi: 10.1111/j.1365-2419.2008.00490.x
Schieltz P. C., Murphy M. E. (1997). The contribution of insulation changes to the energy cost of avian molt. Can. J. Zool. 75 (3), 396–400. doi: 10.1139/z97-049
Skov H., Theophilus T. Z. E., Heinänen S., Fauchald P., Madsen M., Mortensen J. B., et al. (2021). Real-time predictions of seabird distribution improve oil spill risk assessments. Mar. pollut. Bull. 170, 112625. doi: 10.1016/j.marpolbul.2021.112625
Smith K., Robison B. H., Helly J. J., Kauffman R. S., Ruhl H. A., Shaw T. J., et al. (2007). Free-drifting icebergs: hot spots of chemical and biological enrichment in the weddell sea. Science. 317(5837), 478–482. doi: 10.1126/science.1142834
Sokolov S., Rintoul S. R. (2007). On the relationship between fronts of the Antarctic Circumpolar Current and surface chlorophyll concentrations in the Southern Ocean. J. Geophysical Res.: Oceans 112 (C7). doi: 10.1029/2006JC004072
Sokolov S., Rintoul S. (2009). Circumpolar structure and distribution of the Antarctic Circumpolar Current fronts: 2. Variability and relationship to sea surface height. J. Geophysical Res.: Oceans 114 (C11). doi: 10.1029/2008JC005248
Spear L. B., Ainley D. G. (2008). Flight speed of seabirds in relation to wind speed and direction. Ibis 139 (2), 234–251. doi: 10.1111/j.1474-919X.1997.tb04621.x
Steiner U. K., Gaston A. J. (2005). Reproductive consequences of natal dispersal in a highly philopatric seabird. Behav. Ecol. 16 (3), 634–639. doi: 10.1093/beheco/ari035
Subramaniam R. C., Corney S. P., Melbourne-Thomas J., Péron C., Ziegler P., Swadling K. M. (2022). Spatially explicit food web modelling to consider fisheries impacts and ecosystem representation within Marine Protected Areas on the Kerguelen Plateau. ICES J. Mar. Sci. 79 (4), 1327–1339. doi: 10.1093/icesjms/fsac056
Subramaniam R. C., Melbourne-Thomas J., Corney S. P., Alexander K. A., Péron C., Ziegler P., et al. (2020). Time-dynamic food web modelling to explore environmental drivers of ecosystem change on the Kerguelen Plateau. Front. Mar. Sci. 7, 1–20. doi: 10.3389/fmars.2020.00641
Sumner M. D., Wotherspoon S. J., Hindell M. A. (2009). Bayesian Estimation of Animal Movement from Archival and Satellite Tags. PloS One 4 (10), e7324. doi: 10.1371/journal.pone.0007324
Sun C., Liu C., Wang Z., Yan L., Tao T., Qin Q., et al. (2022). ) On the influences of the continental shelf bathymetry correction in Prydz Bay, East Antarctica. Front. Mar. Sci. 9, e1641. doi: 10.3389/fmars.2022.957414
Talley L., Pickard G. L., Emery W. J., Swift J. H. (2011). Descriptive Physical Oceanography. Sixth Edition (Oxford, UK: Elsevier Publishing).
Tamura T., Ohshima K. I., Nihashi S. (2008). Mapping of sea ice production for Antarctic coastal polynyas. Geophysical Res. Lett. 35 (7). doi: 10.1029/2007GL032903
Thaxter C. B., Lascelles B., Sugar K., Cook A. S. C. P., Roos S., Bolton M., et al. (2012). Seabird foraging ranges as a preliminary tool for identifying candidate Marine Protected Areas. Biol. Conserv. 156, 53–61. doi: 10.1016/j.biocon.2011.12.009
Thor G., Low M. (2011). The persistence of the snow petrel (Pagodroma nivea) in Dronning Maud Land (Antarctica) for over 37,000 years. Polar Biol. 34 (4), 609–613. doi: 10.1007/s00300-010-0912-y
Tittensor D., Mora C., Jetz W., Lotze H. K., Ricard D., Vanden Berghe E., et al. (2010). Global patterns and predictors of marine biodiversity across taxa. Nature 466, 1098–1101. doi: 10.1038/nature09329
Tixier P., Welsford D., Lea M.-A., Hindell M. A., Guinet C., Janc A., et al. (2019). Fisheries interaction data suggest variations in the distribution of sperm whales on the Kerguelen Plateau. The Kerguelen Plateau: marine ecosystem and fisheries. Proceedings of the Second Symposium. Available at: https://figshare.utas.edu.au/articles/conference_contribution/Fisheries_interaction_data_suggest_variations_in_the_distribution_of_sperm_whales_on_the_Kerguelen_Plateau/23099726
Veit R. R., Harrison N. M. (2017). Positive Interactions among Foraging Seabirds, Marine Mammals and Fishes and Implications for Their Conservation [Review]. Front. Ecol. Evol. 5. doi: 10.3389/fevo.2017.00121
Viola B. M., Sorrell K. J., Clarke R. H., Corney S. P., Vaughan P. M. (2022). Amateurs can be experts: A new perspective on collaborations with citizen scientists. Biol. Conserv. 274, e109739. doi: 10.1016/j.biocon.2022.109739
Vivier F., Kelly K. A., Thompson L. (1999). Contributions of wind forcing, waves, and surface heating to sea surface height observations in the Pacific Ocean. J. Geophysical Res. Oceans 104 (C9), 20767–20788. doi: 10.1029/1999JC900096
Warwick-Evans V., Santora A., Waggitt J. J., Trathan P. N. (2021). Multi-scale assessment of distribution and density of procellariiform seabirds within the Northern Antarctic Peninsula marine ecosystem. ICES J. Mar. Sci 78 (4), 1324–1339. doi: 10.1093/icesjms/fsab020
Weathers W., Gerhart K., Hodum P. (2000). Thermoregulation in Antarctic fulmarine petrels. J. Comp. Physiol. B. 170 (8), 561–572. doi: 10.1007/s003600000134
Webster M. S., Marra P. P. (2005). “The importance of understanding migratory connectivity and Seasonal Interactions,” in Birds of Two Worlds: The Ecology and Evolution of Migration. Eds. Greenberg R. S., Marra P. P. (Baltimore, USA: JHU Press), 199–209.
Weimerskirch H., Delord K., Guitteaud A., Phillips R. A., Pinet P. (2015). Extreme variation in migration strategies between and within wandering albatross populations during their sabbatical year, and their fitness consequences. Sci. Rep. 5, 8853–8853. doi: 10.1038/srep08853
Woods B., Trebilco R., Walters A., Hindell M., Duhamel G., Flores H., et al. (2022). Myctobase, a circumpolar database of mesopelagic fishes for new insights into deep pelagic prey fields. Sci. Data 9 (1), 404. doi: 10.1038/s41597-022-01496-y
Wotherspoon S., Sumner M., Lisovski S. (2013). BAStag: Basic data processing for light based geolocation archival tags. R Package version 0.1-3 (Auckland, New Zealand: CRAN).
Young H. S., Maxwell S. M., Conners M. G., Shaffer S. A. (2015). Pelagic marine protected areas protect foraging habitat for multiple breeding seabirds in the central Pacific. Biol. Conserv. 181, 226–235. doi: 10.1016/j.biocon.2014.10.027
Keywords: fulmarine petrels, GLS tracking, GLS tags, geolocation, seabirds, polar ecosystems, machine learning, boosting
Citation: Viola B, Wienecke B, Green C-P, Corney S, Raymond B, Southwell C, Sumner MD, Virtue P, Wotherspoon S and Emmerson L (2023) Marine distribution and habitat use by Snow Petrels Pagodroma nivea in East Antarctica throughout the non-breeding period. Front. Mar. Sci. 10:1278229. doi: 10.3389/fmars.2023.1278229
Received: 16 August 2023; Accepted: 10 October 2023;
Published: 23 October 2023.
Edited by:
Matt Rayner, Auckland Museum, New ZealandReviewed by:
Akinori Takahashi, National Institute of Polar Research, JapanDavid Ainley, H.T. Harvey & Associates, United States
Copyright © 2023 Viola, Wienecke, Green, Corney, Raymond, Southwell, Sumner, Virtue, Wotherspoon and Emmerson. This is an open-access article distributed under the terms of the Creative Commons Attribution License (CC BY). The use, distribution or reproduction in other forums is permitted, provided the original author(s) and the copyright owner(s) are credited and that the original publication in this journal is cited, in accordance with accepted academic practice. No use, distribution or reproduction is permitted which does not comply with these terms.
*Correspondence: Benjamin Viola, benjamin.viola@utas.edu.au; benjamin.viola@aad.gov.au