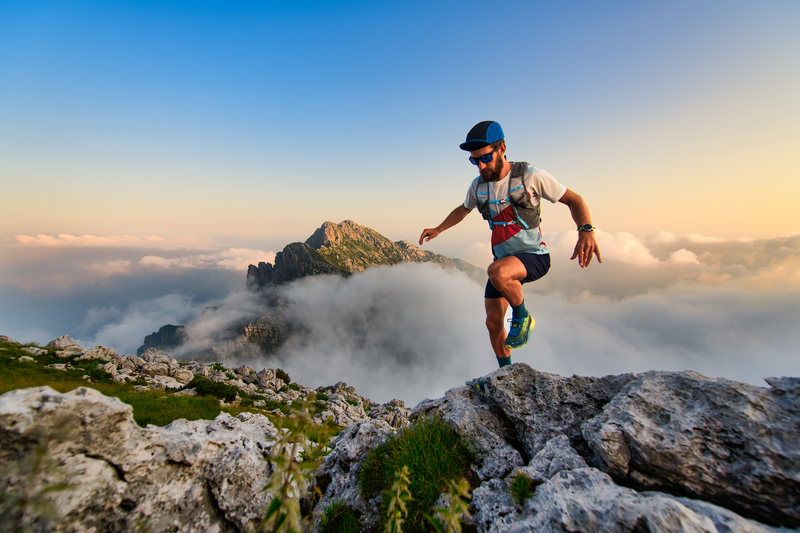
95% of researchers rate our articles as excellent or good
Learn more about the work of our research integrity team to safeguard the quality of each article we publish.
Find out more
ORIGINAL RESEARCH article
Front. Mar. Sci. , 05 October 2023
Sec. Marine Fisheries, Aquaculture and Living Resources
Volume 10 - 2023 | https://doi.org/10.3389/fmars.2023.1242793
This article is part of the Research Topic Fish Behaviour and Welfare View all 11 articles
Aquaculture systems, replete with equipment noise originating from aerators, pumps, feeders, and filtration systems, are known to exert substantial influence on fish behavior and growth. In this research, the focus was directed towards comprehending the impacts of aerator noise on the swimming, feeding, and growth progression of largemouth bass. In the course of a 50-day experimental period, the bass population was segmented into two groups: aerator noise (90.3dB re μPa RMS-1) and ambient noise (70.4dB re μPa RMS-1). The findings indicated discernible disparities in the swimming behavior and feeding between the two groups. Specifically, bass in the noise group maintained greater average angular distance and physical separation from their nearest counterparts than the bass in the ambient group, which were 43.61 ± 1.89° and 85.47 ± 1.72mm for the ambient group and 48.32 ± 0.49° and 97.01 ± 0.57mm for the noise group. Furthermore, the feeding kinetic energy was markedly lower in the noise group as compared to the ambient group. For the first time, the Shannon-Wiener diversity index was leveraged to gauge the diversity of fish swimming behavior, with the results signifying the diversity index of the noise group was 2.69 ± 0.07 higher than that of the ambient group, which was 2.51 ± 0.02. Lastly, the noise group demonstrated compromised growth performance, with a significantly lower average weight as opposed to the ambient group, along with marked variations in the specific growth rate. These findings offer a telling revelation about the profound impacts of aerator noise on the behavioral and growth of largemouth bass, thereby forming a valuable referential base for future research centered on the effects of noise pollution on aquatic organisms.
As the global populace continues to expand, the imperative of food security and sustainable development is gaining paramount importance (Godfray et al., 2010). Aquaculture, an escalating segment of food production, is playing an increasingly significant role in maintaining global food security and bolstering economic growth (Subasinghe et al., 2009). As per a United Nations Food and Agriculture Organization (FAO) report, nearly half of the world’s fishery yield is derived from aquaculture, with the prospect of this fraction growing in the foreseeable future (Canton, 2021). Aquaculture not only offers an abundant supply of animal and plant protein but also contributes to mitigating the overexploitation of marine resources and environmental degradation induced by conventional fishing methods (Naylor et al., 2000; Diana, 2009). In light of technological advances and improved aquaculture management, the industry is progressively adopting more ecofriendly and resource-efficient practices.
For instance, through deploying recirculating aquaculture systems (RAS), multitrophic aquaculture models, and precision feeding strategies, we can alleviate environmental pollution associated with aquaculture while augmenting resource utilization efficiency (Buck et al., 2018; Tang and Liu, 2018; Naylor et al., 2021). Amidst rapid advancements in aquaculture technology, the deployment of aquaculture equipment has become increasingly prevalent. While such equipment is instrumental in enhancing aquaculture productivity, promoting environmental quality, and ensuring product integrity, the escalating prevalence of noise pollution within the industry necessitates heightened attention (Bart et al., 2001; Slabbekoorn et al., 2010; Todd et al., 2021).
Noise emissions from various mechanical activities can have detrimental effects on animal health (Craven et al., 2009; New et al., 2014; Kunc et al., 2016; Slabbekoorn et al., 2019), compromising auditory abilities, inducing physiological stress (Popper, 2003; Halvorsen et al., 2012; Mancera et al., 2017), disrupting communication and behavior (Sarà et al., 2007; Gil et al., 2015; Gendron et al., 2020), and increasing stress-response hormones (Wale et al., 2013a; Hawkins and Chapman, 1975; Smith et al., 2004; Duarte et al., 2015), potentially impacting animal health and survival (Popper, 2003; Purser and Radford, 2011), especially in captive settings like farms and reserves (Popper and Hawkins, 2019; Sherwen and Hemsworth, 2019; Duarte et al., 2023). These noise-induced effects may manifest as reduced feeding behavior due to heightened vigilance (Evans et al., 2018; Giordano et al., 2022), leading to diverted attention (Mendl, 1999; Chan and Blumstein, 2011), potentially causing misjudgments in prey identification and feeding responses (Purser and Radford, 2011; Holles et al., 2013). Additionally, noise could obscure feeding-related acoustic signals (Brumm and Slabbekoorn, 2005; Zhang et al., 2021), resulting in missed feeding opportunities (Schaub et al., 2008; Siemers and Schaub, 2011) and altering food detection and classification during foraging (Lupien and McEwen, 1997; De Kloet et al., 1999).
Noise interference can disrupt animals’ ability to accurately discern food quantity and quality (Kight and Swaddle, 2011), potentially leading to reduced foraging efficiency (Schaub et al., 2008), contrary to optimal foraging theory (MacArthur and Pianka, 1966). Alternatively, this could also be attributed to noise-induced stress, suppressing appetite and activity, and consequently reducing foraging behavior (Mendl, 1999; Charmandari et al., 2005; Williams et al., 2015; Zhang et al., 2022). For instance, Gendron et al. (2020) discovered that noise-exposed Pseudopleuronectes americanus larvae exhibited significantly shorter feeding durations and smaller stomach capacities than controls. Purser and Radford (2011) observed decreased foraging performance in Gasterosteus aculeatus due to increased feeding errors. Likewise, Voellmy et al. (2014) reported on the impact of noise on Phoxinus phoxinus feeding success. These investigations collectively suggest that noise pollution can significantly affect animal feeding behavior, consequently influencing their growth and health indices.
Noise disturbances can not only alter fish feeding behavior but also significantly impact their swimming behavior and spatial distribution (De Vincenzi et al., 2015; Sabet et al., 2016; Simpson et al., 2016; Mickle et al., 2019; Hang et al., 2021). For instance, Hanache et al. (2020) reported that Phoxinus phoxinus, when exposed to noise, allocated less time to foraging in low food density conditions, exhibited changes in spatial distribution, and demonstrated decreased cohesiveness in their swimming. Similarly, noise-affected tuna schools have been observed to alter their coordinated unidirectional swimming structure, increase vertical movements towards the water surface or bottom, and exhibit uncoordinated swimming behavior in more dispersed groups (Sarà et al., 2007).
Largemouth bass (Micropterus salmoides), an economically significant freshwater species known for its fast growth rate, high nutritional value, and strong environmental adaptability (Bai and Li, 2018), holds global economic importance in aquaculture. While previous research has explored the effects of noise on fish feeding and swimming behavior, this study takes approach by introducing the Shannon-Wiener Diversity Index (SWDI) analysis method, extending investigation to encompass group behavior. Specifically, this study will assess the impact of oxygenation equipment noise on feeding and swimming behaviors of largemouth bass school, and quantify the differences in noise impacts on fish school behavior. Through a comprehensive analysis of the findings, this study aims to offer scientific insights to the aquaculture industry, mitigate adverse environmental impacts, and bolster the sustainable development of the industry.
In this study, 200 largemouth bass were procured, each with an average body length of 11.17 ± 0.36cm and a weight of 14.37 ± 2.13g, from Hangzhou Jianfeng Agricultural Development Co., Ltd. Before initiating the experiment, the fish were temporarily housed in a RAS for environmental acclimatization. The water parameters in the holding pool were sustained at certain levels to ensure optimal conditions for the fish. Specifically, the temperature was kept steady at around 25°C, within a margin of ±1°C. Similarly, the pH was 7.2 ± 0.8. Additionally, the concentration of dissolved oxygen was ensured to be 6.5 ± 1mg l-1. Monitoring was also carried out on the levels of ammonia nitrogen and nitrite, with both being kept below 0.3mg l-1 and 0.5mg l-1, respectively. Lighting for the RAS system was provided by full-spectrum LED lights with a 24-hour photoperiod. The fish were fed with floating feed (Fujian Tianma Science and Technology Group Co., Ltd.) at 8:00 a.m. and 8:00 p.m. daily. These preliminary steps were implemented to ensure optimal environmental and feeding conditions for the smooth progression of the subsequent experiment.
The experimental setup incorporated two sets of RAS (Figure 1), each containing three aquaculture pools made of PVC with an internal diameter of 1 meter and a height of 0.8 meters. The biological filter pool, a 1*0.5*0.8m PVC tank, facilitated ammonia removal, UV sterilization, and solid particle filtration, offering a controlled environment for the fish. The soundscape management equipment included a type 8130 hydrophone (Denmark Brüel & Kjær Co. Ltd.), a player, an AVANT MI-2004 power amplifier, and a US-0150 underwater loudspeaker (Hangzhou ECON Technology Co. Ltd., China), collectively used for noise soundscape collection and provision during the experiment. Additionally, the setup comprised a behavior video capture system (Hikvision, Hangzhou) to record the fish group’s feeding and swimming patterns.
The experimental system was established in a quiet laboratory with measures like wrapping the stainless steel frame with blackout cloth, padding the culture pool’s bottom with a PVC shock-absorbing tray, employing liquid oxygen for oxygen supply, using a fixed biological bed, and wrapping PVC pipes with sound-absorbing materials to mitigate interference from environmental noise and system operational noise.
In this study, a noise group was established and subjected to a sound level of 90.3dB re μPa RMS-1, while an ambient control group with a sound level of 70.4dB re μPa RMS-1 was also established (Figure 2). The noise sample was collected from the large-scale aquaculture facility of Suzhou Jinchengfu Fisheries Co., Ltd. and effectively represents the acoustic characteristics encountered by fish in a closed RAS. The aeration equipment sampled was a GRB-200A three-lobe roots blower (GSD Industrial Co.,Ltd.) with an air volume capacity of 50m³ min-1 and a pressure of 78.43kPa. To evaluate the acoustic impact of the aeration machine within the fish pond, recordings of the soundscape were obtained when the aeration machine operated exclusively. For the ambient control group, the soundscape was recorded with the complete RAS in the shutdown state.
Each group contained three replicates of the aquaculture pool, each housing 20 fish. The entire experiment spanned 50 days. During the experiment, the recirculating water flow was set at 300L h-1, with a daily water change constituting 15% of the total volume. Fecal waste at the bottom of the fish pond was vacuumed daily. Concurrently, the parameters of the water quality were held in alignment with those established during the acclimation stage, and the fish were fed at 8:00 am and 8:00 pm each day.
To capture the feeding and swimming behavior of the largemouth bass, cameras and 24-hour constant LED lights were installed on the stainless steel frame carrying shade cloth, which was positioned directly above the culture tank. Recordings were made at six designated time points (3:00, 6:00, 9:00, 12:00, 15:00, 18:00) each day, with each session lasting ten minutes, to analyze the group swimming behavior. To analyze feeding behavior, video footage was captured every three days at 8:00 in the morning. The cameras were networked to a switch and recorder, facilitating both video storage and real-time monitoring.
For the analysis of group swimming behavior, the Mask RCNN technique was employed (Figure 3). From each video, 60 frames were extracted, and object instance segmentation was conducted on the experimental fish in the frames. This generated a segmentation mask for each experimental fish, enabling their identification and localization (He et al., 2020). To evaluate the cohesion of swimming, the distance between every experimental fish and its two nearest neighbors within the group was computed. A line connecting a fish’s head and tail represented its swimming direction. To evaluate the polarity of swimming, the angle formed by the swimming direction of an experimental fish and its two closest neighbors was utilized.
Figure 3 Quantitative Measurement of the Angle and Distance Between a Focal Fish and Its Nearest Neighbor.
The SWDI, an index commonly used in biodiversity research proposed by Claude Shannon and Norbert Wiener in the fields of information theory and ecology, measures the richness and evenness of species in an ecosystem. This study proposed to use the SWDI to evaluate the diversity of group swimming behavior. The average angle and distance during the experiment were divided into nine angle ranges (0-10°, 10-20°, 20-30°…, >90°) and eight distance ranges (<60mm, 60-70mm, 70-80mm…., >120mm), generating 72 unique angle-distance combinations. The frequency of each angle-distance combination was counted, and the relative frequency calculated. The SWDI for group behavior was then determined using the formula:
Where H represents the SWDI, and pi is the relative frequency of the ith angle-distance combination.
In analyzing group feeding behavior, this study implemented an Modified kinetic energy model (MKEM) to quantify the feeding intensity of the group (indicative of the group’s appetite). The MKEM model doesn’t require tracking or foreground segmentation. It facilitates the extraction and quantification of the spatial behavioral characteristics of the fish group, effectively describing the feeding behavior, independent of light environment and water quality (Wei et al., 2021). Greater intensity of water ripple fluctuations and alterations in the flow field in the reflective area, induced by feeding, result in higher feeding kinetic energy values (Figures 4A, B). Each feeding video clip in this experiment lasted 15 seconds, with a frame rate of 25fps, and the single-time feeding kinetic energy change is shown in Figure 5A. The feeding process was initiated when the feed was introduced. The same quantity of feed was given in each fish tank using a satiety feeding strategy, with leftover feed scooped out, dried, and weighed post-feeding to compute the feed intake quantity.
Figure 4 Feeding Kinetic Energy Analysis. (A) Snapshot of a Feeding Event: Visual depiction of largemouth bass during the feeding process. (B) Transformation of Feeding Snapshot into an Optical Flow Image: Conversion of the feeding snapshot into an optical flow image for further analysis.
Figure 5 Kinetic Energy Changes During Feeding. (A) Change in Kinetic Energy During a Single Feeding: Variation in kinetic energy throughout a singular feeding event. (B) Peak Feeding Kinetic Energy Changes in Noise and Ambient Groups: Comparison of peak feeding kinetic energy changes in both groups from Day 1 to Day 50 of the experiment.
Prior to the commencement of the experiment, a random sampling of 20 fish was undertaken to determine initial body length and weight. Over the course of the experiment, fish were weighed every 10 days. At the conclusion of the experiment, eight fish were randomly selected from each cultivation pond for growth evaluation, with the concurrent tally of fish in each group also recorded.
The Specific Growth Rate (SGR, % d-1) and Survival Rate (SR, %) were calculated as follows:
In these equations, BW1 and BW2 represent the initial and final body weights of each fish (in grams), while T1 and T2 denote the corresponding time intervals. Ni and Nf stand for the initial and final numbers of fish, respectively.
The data are presented as mean ± standard deviation (SD). A comparative analysis of growth, swimming, and feeding across different treatments was conducted using independent t-tests. For SWDI measured at multiple time points, comparisons were analyzed using repeated measures ANOVA. Prior to analysis, normality of the data distribution was assessed through the Kolmogorov-Smirnov test, and homogeneity of variances was examined using Levene’s Test. In cases where the assumptions were met, the threshold for statistical significance was set at P<0.05. All statistical analyses were performed using IBM SPSS Statistics version 22.0. Graphical representations were generated using SigmaPlot Version 14.0.
The results exhibited substantial disparities in swimming behavior between the largemouth bass in the ambient group and those exposed to noise. Throughout the experiment, the mean angle and distance between a focal fish and their nearest counterparts in the noise group were significantly greater than those in the ambient group (Figure 6), with average values of 48.32 ± 0.49° and 97.01 ± 0.57mm for the noise group, compared to 43.61 ± 1.89° and 85.47 ± 1.72mm for the ambient group (P<0.05).
Figure 6 Comparative Analysis of Swimming Behavior in the Noise and Ambient Groups. (A) The average angle between the nearest two fish in each group. (B) The average distance between the nearest two fish in each group.
When considering the diversity of swimming behavior, notable differences were also observed between the ambient and noise groups. An examination of various angle-distance combination categories (Figure 7) reveals that in the ambient group, the combination of an average angle of 30-40° and an average distance of 80-90mm was observed in 12.73% of fish, followed by the combination of 30-40° and 90-100mm, which accounted for 11.23% of observations. Conversely, the fish in the noise group demonstrated more dispersed swimming behavior. The highest proportion of observations in the noise group was in the combination of 30-40° and 100-110mm (9.09%), followed by 30-40° and 90-100mm (8.72%). Swimming behavior in the noise group was characterized by larger average distances.
Figure 7 Heat Map Depicting the Distribution of Largemouth Bass Swimming Behavior in Both Experimental Tanks: The heat map visualizes the variations in the swimming behavior of largemouth bass in response to the experimental conditions. Both the average angle (horizontal axis) and average distance (vertical axis) between fish are represented. (A) Ambient Group: Depicts the swimming behavior distribution under normal conditions. (B) Noise Group: Illustrates the altered swimming behavior under the influence of equipment noise.
The mean SWDI also differed significantly between the two groups throughout the experiment (Figure 8A), with the noise group (2.69 ± 0.07) being significantly higher than the ambient group (2.51 ± 0.02) (P<0.05). As depicted in Figure 8B, the SWDI of the fish population in the noise group exhibited an upward trend over time. The two groups started to show significant difference one month after noise intervention, and this disparity persisted until the conclusion of the experiment. The present study further analyzed the average SWDI at various time points (3/6/9/12/15/18 o’clock), revealing diurnal patterns in fish activity (Figure 8C). At 6:00 and 18:00, the diversity of fish swimming behavior was lower (P<0.05), suggesting more stable formations. While similar trends were observed in both the noise and ambient groups, the average SWDI for fish swimming behavior in the ambient group was significantly lower at 3/6/18 o’clock compared to the noise group (P<0.05).
Figure 8 Variation in Shannon-Wiener Diversity Index (SWDI) Reflecting Swimming Behavior Diversity in Largemouth Bass Populations Across Noise and Ambient Groups: This figure illustrates the diverse swimming behaviors of largemouth bass in response to both experimental conditions using the SWDI. (A) Mean SWDI Throughout the Experiment. (B) Mean SWDI for Each 10-Day Period. (C) Mean SWDI at Different Time Points of the Day.
With respect to feeding behavior, the largemouth bass in the ambient group exhibited a more robust feeding inclination, while those in the noise group displayed a diminished appetite (Figure 5B). Specifically, the feeding kinetic energy in both groups increased as the experiment unfolded, albeit at different rates. During the early stage of the experiment, the feeding kinetic energy of the noise group was lower than the ambient group, though the difference was not statistically significant. However, from the 27th day onwards, the feeding performance of the ambient group consistently outstripped that of the noise group, a trend that persisted until the experiment’s conclusion. Measurements taken on the 48th day indicated that the feeding kinetic energy of the ambient group stood at 1639.99 ± 24.30, compared to 1569.97 ± 22.53 for the noise group.
Moreover, the daily average feed intake of the largemouth bass in the ambient group exceeded that of the noise group, with the discrepancy widening over time. For most of the experimental period, the divergence in daily average feed intake between the two groups did not reach statistical significance, except on days 33, 47, 49, and 50 (Figure 9). Nevertheless, a marked contrast in total feed consumption was observed between the two groups (Figure 10), with the noise group’s intake (24.95 ± 1.58g) significantly lower than that of the ambient group (29.25 ± 0.86g) (P<0.05).
Figure 9 Comparison of Daily Feed Intake: This graph depicts the change in the average daily feed intake for both the Noise and Ambient groups over the course of the 50-day experimental period. Differences marked with different lowercase letters are considered significant.
Figure 10 Total Food Intake Comparison: This figure illustrates the overall contrast in food consumption between the Noise and Ambient groups throughout the entire experimental duration. Differences marked with different lowercase letters are considered significant.
The present study revealed that the SR remained 100% across all groups throughout the experiment, and that the noise generated by aeration equipment significantly impacted the growth performance of largemouth bass. At the experiment’s inception, the initial weight of fish across all groups was identical, averaging 11.06 ± 0.68 g. After 50 days of experimental treatment, the mean weight of largemouth bass in the ambient group (30.61 ± 1.51g) was significantly higher than that in the noise group (26.36 ± 1.45g) (P<0.05), indicating that aeration noise impeded growth. Figure 11A illustrates the weights of the fish measured at ten-day intervals throughout the experiment. The data show that during the experiment’s first month, there was no significant disparity in weight between the ambient and noise groups. However, from day 40 onwards, the weight of fish in the aerator noise group was persistently and markedly below the level in the ambient group, a trend that endured until the experiment’s end. Over the course of the experiment, the weight difference between the ambient and noise groups progressively increased (Figure 11A). The SGR of largemouth bass in the noise group and the ambient group also displayed significant divergence (Figure 11B), with the noise group’s SGR (3.38 ± 0.09) markedly lower than that of the ambient group (3.68 ± 0.08) (P<0.05).
Figure 11 Largemouth Bass Growth Comparison in Different Noise Environments. (A) Body Weight Change in Body Weight per 10 Days. (B) Specific Growth Rate (SGR) Comparison.
In this investigation, the profound influence of aeration equipment noise on the swimming patterns, feeding habits, and growth of largemouth bass was distinctly observed. Evidently, compared to those in the ambient group, the fish in the noise group exhibited substantial differences in swimming behavior, feeding vigor, and quantity of food consumed. The escalated swimming expenditure and diminished feeding intensity and quantity within a noisy environment adversely affected the growth performance of the largemouth bass in the noise group.
Acoustic signals constitute one of the primary channels through which fish garner information about their environment, with aquatic species relying on auditory data for communication, navigation, orientation, evasion, and foraging (Popper and Hawkins, 2019). Noise acts as a stimulus source that disrupts fish attention and interferes with fish behavior by masking acoustic information (Slabbekoorn et al., 2010). In terms of swimming behavior observed in this study, significant disparities arose between the ambient and aerator noise groups. The average distance and angle between each fish in the noise group and its nearest neighbor were greater, implying that the noise environment might have impeded the orientation navigation information of the largemouth bass or induced discomfort, thus compelling them to maintain a more significant distance and angle from other individuals.
The distance and angle between the closest individuals in a fish school can serve as metrics of the school’s clustering strategy, which enhances group collaboration and reduces swimming energy expenditure (Chen et al., 2016; Kent et al., 2019). In this study, the noise group fish had larger average distances and angles, indicative of lower fish school cohesion and polarity. This observation aligns with previous research findings. For instance, Sarà et al. (2007) found that noise could alter the swimming direction of tuna, leading to a relatively dispersed cluster structure and more chaotic swimming behavior in the school. Similarly, De Vincenzi et al. (2015) demonstrated that noise impacted the swimming activity and behavior distribution of the lesser spotted dogfish (Scyliorhinus canicula) in aquaculture ponds. McLaughlin and Kunc (2015) reported that noise exposure caused the european minnow (Phoxinus phoxinus) to modify its spatial distribution, with marked increases in social interaction and swimming distance. These findings resonate with the present observations: under the influence of the noise environment, the distribution of largemouth bass in the culture pond becomes more dispersed.
However, the research further points out that the noise environment not only affects the clustering behavior of largemouth bass schools but also affects the diversity of their swimming behavior. It was discovered that, compared to the ambient group, the diversity of swimming behavior among the largemouth bass in the noise group was higher. This could be due to the largemouth bass being distracted by the disruption of aeration equipment noise, or the occlusion of orientation and navigation acoustic information by noise. Additionally, this might be a response strategy of the largemouth bass to the noise environment, in which behavioral diversity is increased to adapt to the noisy conditions. A higher SWDI may indicate a more diversified distance and angle between fish in a school and more irregular formations during swimming. In some instances, a greater diversity of swimming behavior may correlate with increased swimming energy expenditure (Webb, 1984). For example, irregular formations that lead to larger distances between individuals could cause fish to expend more energy while adjusting their position and speed during swimming. Maintaining this state of elevated energy expenditure over an extended period could potentially affect individual growth performance within the fish school (Jobling et al., 1993). The application of the SWDI to assess the behavior of largemouth bass schools is a novel contribution of this study. Although this index is often used to evaluate the diversity of biological communities (Spellerberg and Fedor, 2003; Thukral, 2017), it is infrequently applied within behavioral ecology. The study’s results indicate that this index effectively captures the diversity of swimming behavior in largemouth bass schools, thereby introducing a novel research tool in behavioral ecology. The reliability of the findings was ensured through meticulous experimental design and data analysis methodologies. For example, the swimming behavior of fish schools was continuously monitored throughout the experiment, encompassing comprehensive classification of angle-distance combinations and rigorous statistical analyses. Additionally, regular inspection and maintenance of experimental equipment and data training models were conducted to ensure the stability of the noise level and the accuracy of the algorithm. As a result, the credibility of the findings is held with a high level of confidence.
While this study primarily aimed to investigate the distinct impact of noise generated by oxygenation equipment on largemouth bass behavior, an additional insight emerged regarding the general behavioral patterns of fish schools. By analyzing the diversity of fish school behavior at different times throughout the day, the present study observed that fish schools maintain a more regular swimming formation prior to feeding times (6:00, 18:00). Post-feeding, fish schools may exhibit a greater diversity of behavior. Once satiated, they might be more inclined to engage in other activities such as reproduction, territorial protection, and social interactions (Ward et al., 2006). At this juncture, the fish school may become more dispersed, reducing the need for foraging, and individuals within the school may have greater freedom of movement. Under conditions of scarcity, the behavior of fish schools may be more uniform. This is due to the need for cooperation in the search for food in the face of reduced availability, causing the fish to form more compact groups. This scenario leads to an increase in fish school cohesion, with the tighter assembly being more effective in food foraging (Keenleyside, 2012). Starving fish schools might rely more heavily on the guidance of a leader (Reebs, 2000), subsequently reducing behavioral diversity.
In this study, it was observed that largemouth bass in the ambient group displayed a stronger feeding inclination, while those in the noise group demonstrated a significantly diminished appetite. This discrepancy might be attributed to noise distractions affecting the largemouth bass’s focus, indirectly impacting their feeding desire and resulting in decreased food consumption. These findings align with those of Voellmy et al. (2014), who verified the disruptive influence of sound on fish feeding, showing that significantly more individuals in the control group obtained food, indicating that fish foraging activities were hindered under experimental conditions. Purser and Radford (2011) demonstrated that the stickleback fish, Gasterosteus aculeatus, exposed to noise, increased the frequency of attacks on non-food items due to misidentifying them as food, leading to a decline in its foraging performance. Gendron et al. (2020) found that the winter flounder, Pseudopleuronectes americanus, preyed less frequently and had a relatively reduced stomach capacity under noise conditions. Wale et al. (2013b) reported that noise affected the feeding behavior and performance of crabs in a tank-based controlled experiment. While the mentioned studies involve different fish species, the present study focus on largemouth bass contributes to understanding of how noise pollution can impact feeding behavior in this particular species.
It’s worth noting that this research also observed that the feeding kinetic energy in both groups was initially low, but increased as the experiment progressed with the growth and increased food consumption of the largemouth bass. In the noise group, the increase in feeding kinetic energy may reflect an adaptive response, suggesting that, after a period of acclimation to the noise environment, largemouth bass could adjust their behavior to cope with the noise conditions (Dooling et al., 2015). Nonetheless, despite these adaptive shifts, the feeding performance of the largemouth bass in the noise-exposed group continued to be significantly inferior compared to the ambient group. This diminished performance might be attributed to the persistent interference and suppression induced by the noisy environment on the largemouth bass’s feeding conduct and appetite.
This investigation underscores the significant influence of aeration equipment noise on largemouth bass growth performance. Largemouth bass in the noise-exposed group exhibited a considerably lower average weight and slower growth rate compared to the ambient group. This discrepancy may stem from the noise-induced reduction in feeding desire and intake, leading to decreased energy consumption. Additionally, the less cohesive and polarized swimming patterns observed in the noise group could contribute to heightened energy expenditure during swimming activities. These findings align with prior research. Lagardčre (1982) reported elevated noise levels in aquaculture ponds leading to increased metabolic rates in prawns and reduced growth performance. Banner and Hyatt (1973) observed decreased SR and growth performance in carp exposed to noise. Similarly, Wysocki et al. (2007) and Davidson et al. (2009) noted short-term negative effects of noise on rainbow trout growth performance in RAS, with potential adaptability over time. Hang et al. (2021) also explored the negative effects of noise on largemouth bass growth performance in RAS systems, this study uniquely focuses on analyzing the specific impact of aeration equipment noise, which plays a prominent role in the soundscape of intensive aquaculture settings, on the diversity of fish school behavior, providing additional evidence to better assess the impact of noise on fish ecology and welfare. Furthermore, this research expands the understanding by directly linking noise to the total feeding quantity of largemouth bass schools, providing concrete evidence for the reduction in feeding desire and intake caused by noise.
However, the study presents certain limitations. For instance, the sample size employed is relatively modest, potentially lacking comprehensive representation of the entire largemouth bass population. Furthermore, the experiment’s duration is comparatively brief, and variations between the experimental conditions and actual production environments may impact the validity of the results. It is also important to acknowledge the influence of the 24-hour photoperiod with full-spectrum LED lights on fish behavior. While this lighting condition was chosen to ensure consistent visibility for our observations, it may have disrupted the natural diurnal rhythms of the tested fish. This is a notable limitation as it could have affected the baseline behavior of largemouth bass, potentially confounding the results. Additionally, the methodologies and instruments implemented for sound environment assessment may inherently possess constraints. While sound pressure was utilized to characterize the noise environment, measurement of the particle motion component remained beyond the scope of this study. Notwithstanding these limitations, the research elucidates the noteworthy impact of noise on the behavior and growth of largemouth bass within the specific experimental framework. Such insights are crucial for understanding the repercussions of noise pollution on fish ecology, contributing to the enhancement of fish welfare and potentially influencing fish production. These findings also provide a foundation for future investigations into the impacts of noise on largemouth bass and other fish species.
This investigation has illuminated the influence of aeration equipment noise on the swimming behavior, feeding habits, and growth progression of largemouth bass populations. The results from the experimental trials indicate that largemouth bass residing in noisy environments, when contrasted with the ambient group, display substantial disparities in swimming behavior, characterized by larger average angles and distances from the nearest neighbor fish. Moreover, these bass experience diminished appetite, decreased food consumption, and compromised growth performance. Additionally, the present study has pioneered the use of the SWDI to appraise the diversity of fish swimming behavior, thereby offering a novel evaluation instrument for fish behavior studies. Going forward, it is crucial to delve deeper into the precise mechanisms by which noise impacts largemouth bass and other aquatic organisms, aiming to provide scientific substantiation for the mitigation and regulation of noise pollution. Simultaneously, these research findings assume paramount importance in addressing the challenges posed to fish welfare within aquaculture settings. Efforts to minimize noise pollution within aquacultural settings hold the potential to elevate farming efficiency, foster animal welfare, and establish a robust framework for the sustainable advancement of aquaculture practices.
The original contributions presented in the study are included in the article/supplementary material. Further inquiries can be directed to the corresponding author.
The animal study was approved by Experimental Animal Welfare Ethics Committee of Zhejiang University. The study was conducted in accordance with the local legislation and institutional requirements.
YZ conceived the study, carried out field work, analyzed data, and wrote the majority of the manuscript. AS, SH, HZ carried out filed work. WX, JZ, SZ conceived the study, analyzed data and revised the manuscript All authors contributed to the article and approved the submitted version.
This study was supported by the National Key R&D Program of China (Grant No. 2022YFD2001700), the Key Program of Science and Technology of Zhejiang Province (Grant No. 2023C02050), and the National Modern Agriculture Industrial Technology System Special Project-the National Technology System for Conventional Freshwater Fish Industries (Grant No. CARS-45-26).
We grateful thank Nantian Peng and Yanfeng Zhang for their technical support during sampling and experiments.
The authors declare that the research was conducted in the absence of any commercial or financial relationships that could be construed as a potential conflict of interest.
All claims expressed in this article are solely those of the authors and do not necessarily represent those of their affiliated organizations, or those of the publisher, the editors and the reviewers. Any product that may be evaluated in this article, or claim that may be made by its manufacturer, is not guaranteed or endorsed by the publisher.
Bai J., Li S. (2018). evelopment of largemouth bass (Micropterus salmoides) culture," in Aquaculture in China. Eds. J. Gui, Q. Tang, Z. Li, J. Liu and S. S. De Silva. doi: 10.1002/9781119120759.ch4_5
Banner A., Hyatt M. (1973). Effects of noise on eggs and larvae of two estuarine fishes. Trans. Am. Fisheries Soc. 102 (1), 134–136. doi: 10.1577/1548-8659(1973)102<134:EONOEA>2.0.CO;2
Bart A. N., Clark J., Young J., Zohar Y. (2001). Underwater ambient noise measurements in aquaculture systems: a survey. Aquacultural Eng. 25 (2), 99–110. doi: 10.1016/S0144-8609(01)00074-7
Brumm H., Slabbekoorn H. (2005). Acoustic communication in noise. Adv. Study Behav. 35, 151–209. doi: 10.1016/S0065-3454(05)35004-2
Buck B. H., Troell M. F., Krause G., Angel D. L., Grote B., Chopin T. (2018). State of the art and challenges for offshore integrated multi-trophic aquaculture (IMTA). Front. Mar. Sci. 5, 165. doi: 10.3389/fmars.2018.00165
Canton H. (2021). “Food and agriculture organization of the United Nations—FAO,” in The Europa directory of international organizations 2021 (Rome, Italy: Routledge), 297–305.
Chan A. A. Y. H., Blumstein D. T. (2011). Attention, noise, and implications for wildlife conservation and management. Appl. Anim. Behav. Sci. 131 (1-2), 1–7. doi: 10.1016/j.applanim.2011.01.007
Charmandari E., Tsigos C., Chrousos G. (2005). Endocrinology of the stress response. Annu. Rev. Physiol. 67, 259–284. doi: 10.1146/annurev.physiol.67.040403.120816
Chen S. Y., Fei Y. H. J., Chen Y. C., Chi K. J., Yang J. T. (2016). The swimming patterns and energy-saving mechanism revealed from three fish in a school. Ocean Eng. 122, 22–31. doi: 10.1016/j.oceaneng.2016.06.018
Craven A., Carton A. G., McPherson C. R., McPherson G. (2009). Determining and quantifying components of an aquaculture soundscape. Aquacultural Eng. 41 (3), 158–165. doi: 10.1016/j.aquaeng.2009.07.003
Davidson J., Bebak J., Mazik P. (2009). The effects of aquaculture production noise on the growth, condition factor, feed conversion, and survival of rainbow trout, Oncorhynchus mykiss. Aquaculture 288 (3-4), 337–343. doi: 10.1016/j.aquaculture.2008.11.037
De Kloet E. R., Oitzl M. S., Joëls M. (1999). Stress and cognition: are corticosteroids good or bad guys? Trends Neurosci. 22 (10), 422–426. doi: 10.1016/s0166-2236(99)01438-1
De Vincenzi G., Filiciotto F., Maccarrone V., Mazzola S., Buscaino G. (2015). Behavioural responses of the European spiny lobster, Palinurus elephas (Fabriciu), to conspecific and synthetic sounds. Crustaceana 88 (5), 523–540. doi: 10.1163/15685403-00003430
Diana J. S. (2009). Aquaculture production and biodiversity conservation. Bioscience 59 (1), 27–38. doi: 10.1525/bio.2009.59.1.7
Dooling R. J., Leek M. R., Popper A. N. (2015). Effects of noise on fishes: What we can learn from humans and birds. Integr. zoology 10 (1), 29–37. doi: 10.1111/1749-4877.12094
Duarte M. H. L., Sousa-Lima R. S., Young R. J., Farina A., Vasconcelos M., Rodrigues M., et al. (2015). The impact of noise from open-cast mining on Atlantic forest biophony. Biol. Conserv. 191, 623–631. doi: 10.1016/j.biocon.2015.08.006
Duarte R. H. L., de Oliveira Passos M. F., Beirão M. V., Midamegbe A., Young R. J., de Azevedo C. S. (2023). Noise interfere on feeding behaviour but not on food preference of saffron finches (Sicalis flaveola). Behav. Processes 206, 104844. doi: 10.1016/j.beproc.2023.104844
Evans J. C., Dall S. R., Kight C. R. (2018). Effects of ambient noise on zebra finch vigilance and foraging efficiency. PloS One 13 (12), e0209471. doi: 10.1371/journal.pone.0209471
Gendron G., Tremblay R., Jolivet A., Olivier F., Chauvaud L., Winkler G., et al. (2020). Anthropogenic boat noise reduces feeding success in winter flounder larvae (Pseudopleuronectes americanus). Environ. Biol. Fishes 103, 1079–1090. doi: 10.1007/s10641-020-01005-3
Gil D., Honarmand M., Pascual J., Pérez-Mena E., Macías Garcia C. (2015). Birds living near airports advance their dawn chorus and reduce overlap with aircraft noise. Behav. Ecol. 26 (2), 435–443. doi: 10.1093/beheco/aru207
Giordano A., Hunninck L., Sheriff M. J. (2022). Prey responses to predation risk under chronic road noise. J. Zoology 317 (2), 147–157. doi: 10.1111/jzo.12968
Godfray H. C. J., Beddington J. R., Crute I. R., Haddad L., Lawrence D., Muir J. F., et al. (2010). Food security: the challenge of feeding 9 billion people. science 327 (5967), 812–818. doi: 10.1126/science.1185383
Halvorsen M. B., Casper B. M., Matthews F., Carlson T. J., Popper A. N. (2012). Effects of exposure to pile-driving sounds on the lake sturgeon, Nile tilapia and hogchoker. Proc. R. Soc. B: Biol. Sci. 279 (1748), 4705–4714. doi: 10.1098/rspb.2012.1544
Hanache P., Spataro T., Firmat C., Boyer N., Fonseca P., Médoc V. (2020). Noise-induced reduction in the attack rate of a planktivorous freshwater fish revealed by functional response analysis. Freshw. Biol. 65 (1), 75–85. doi: 10.1111/fwb.13271
Hang S., Zhao J., Ji B., Li H., Zhang Y., Peng Z., et al. (2021). Impact of underwater noise on the growth, physiology and behavior of Micropterus salmoides in industrial recirculating aquaculture systems. Environ. pollut. 291, 118152. doi: 10.1016/j.envpol.2021.118152
Hawkins A. D., Chapman C. J. (1975). Masked auditory thresholds in the cod, Gadus morhua L. J. Comp. Physiol. 103 (2), 209–226. doi: 10.1007/BF00617122
He K., Gkioxari G., Dollâr P., Girshick R. (2020). R-CNN mask 2020. IEEE Trans. Pattern Anal. Mach. Intell. 42, 386–397. doi: 10.1109/TPAMI.2018.2844175
Holles S., Simpson S. D., Radford A. N., Berten L., Lecchini D. (2013). Boat noise disrupts orientation behaviour in a coral reef fish. Mar. Ecol. Prog. Ser. 485, 295–300. doi: 10.3354/meps10346
Jobling M., Baardvik B. M., Christiansen J. S., Jørgensen E. H. (1993). The effects of prolonged exercise training on growth performance and production parameters in fish. Aquaculture Int. 1, 95–111. doi: 10.1007/BF00692614
Keenleyside M. H. (2012). Diversity and adaptation in fish behaviour Vol. 11 (Berlin, Germany: Springer Science & Business Media).
Kent M. I., Lukeman R., Lizier J. T., Ward A. J. (2019). Speed-mediated properties of schooling. R. Soc. Open Sci. 6 (2), 181482. doi: 10.1098/rsos.181482
Kight C. R., Swaddle J. P. (2011). How and why environmental noise impacts animals: an integrative, mechanistic review. Ecol. Lett. 14 (10), 1052–1061. doi: 10.1111/j.1461-0248.2011.01664.x
Kunc H. P., McLaughlin K. E., Schmidt R. (2016). Aquatic noise pollution: implications for individuals, populations, and ecosystems. Proc. R. Soc. B: Biol. Sci. 283 (1836), 20160839. doi: 10.1098/rspb.2016.0839
Lagardčre J. P. (1982). Effects of noise on growth and reproduction of Crangon crangon in rearing tanks. Mar. Biol. 71, 177–185. doi: 10.1007/BF00394627
Lupien S. J., McEwen B. S. (1997). The acute effects of corticosteroids on cognition: integration of animal and human model studies. Brain Res. Rev. 24 (1), 1–27. doi: 10.1016/S0165-0173(97)00004-0
MacArthur R. H., Pianka E. R. (1966). On optimal use of a patchy environment. Am. Nat. 100 (916), 603–609. doi: 10.1086/282454
Mancera K. F., Lisle A., Allavena R., Phillips C. J. (2017). The effects of mining machinery noise of different frequencies on the behaviour, faecal corticosterone and tissue morphology of wild mice (Mus musculus). Appl. Anim. Behav. Sci. 197, 81–89. doi: 10.1016/j.applanim.2017.08.008
McLaughlin K. E., Kunc H. P. (2015). Changes in the acoustic environment alter the foraging and sheltering behaviour of the cichlid Amititlania nigrofasciata. Behav. processes 116, 75–79. doi: 10.1016/j.beproc.2015.04.012
Mendl M. (1999). Performing under pressure: stress and cognitive function. Appl. Anim. Behav. Sci. 65 (3), 221–244. doi: 10.1016/S0168-1591(99)00088-X
Mickle M. F., Harris C. M., Love O. P., Higgs D. M. (2019). Behavioural and morphological changes in fish exposed to ecologically relevant boat noises. Can. J. Fisheries Aquat. Sci. 76 (10), 1845–1853. doi: 10.1139/cjfas-2018-0258
Naylor R. L., Goldburg R. J., Primavera J. H., Kautsky N., Beveridge M. C., Clay J., et al. (2000). Effect of aquaculture on world fish supplies. Nature 405 (6790), 1017–1024. doi: 10.1038/35016500
Naylor R. L., Hardy R. W., Buschmann A. H., Bush S. R., Cao L., Klinger D. H., et al. (2021). A 20-year retrospective review of global aquaculture. Nature 591 (7851), 551–563. doi: 10.1038/s41586-021-03308-6
New L. F., Clark J. S., Costa D. P., Fleishman E., Hindell M. A., Klanjšček T., et al. (2014). Using short-term measures of behaviour to estimate long-term fitness of southern elephant seals. Mar. Ecol. Prog. Ser. 496, 99–108. doi: 10.3354/meps10547
Popper A. N. (2003). Effects of anthropogenic sounds on fishes. Fisheries 28 (10), 24–31. doi: 10.1577/1548-8446(2003)28[24:EOASOF]2.0.CO;2
Popper A. N., Hawkins A. D. (2019). An overview of fish bioacoustics and the impacts of anthropogenic sounds on fishes. J. fish Biol. 94 (5), 692–713. doi: 10.1111/jfb.13948
Purser J., Radford A. N. (2011). Acoustic noise induces attention shifts and reduces foraging performance in three-spined sticklebacks (Gasterosteus aculeatus). PloS One 6 (2), e17478. doi: 10.1371/journal.pone.0017478
Reebs S. G. (2000). Can a minority of informed leaders determine the foraging movements of a fish shoal? Anim. Behav. 59 (2), 403–409. doi: 10.1006/anbe.1999.1314
Sabet S. S., Wesdorp K., Campbell J., Snelderwaard P., Slabbekoorn H. (2016). Behavioural responses to sound exposure in captivity by two fish species with different hearing ability. Anim. Behav. 116, 1–11. doi: 10.1016/j.anbehav.2016.03.027
Sarà G., Dean J. M., d’Amato D., Buscaino G., Oliveri A., Genovese S., et al. (2007). Effect of boat noise on the behaviour of bluefin tuna Thunnus thynnus in the Mediterranean Sea. Mar. Ecol. Prog. Ser. 331, 243–253. doi: 10.3354/meps331243
Schaub A., Ostwald J., Siemers B. M. (2008). Foraging bats avoid noise. J. Exp. Biol. 211 (19), 3174–3180. doi: 10.1242/jeb.022863
Sherwen S. L., Hemsworth P. H. (2019). The visitor effect on zoo animals: Implications and opportunities for zoo animal welfare. Animals 9 (6), 366. doi: 10.3390/ani9060366
Siemers B. M., Schaub A. (2011). Hunting at the highway: traffic noise reduces foraging efficiency in acoustic predators. Proc. R. Soc. B: Biol. Sci. 278 (1712), 1646–1652. doi: 10.1098/rspb.2010.2262
Simpson S. D., Radford A. N., Nedelec S. L., Ferrari M. C., Chivers D. P., McCormick M. I., et al. (2016). Anthropogenic noise increases fish mortality by predation. Nat. Commun. 7 (1), 10544. doi: 10.1038/ncomms10544
Slabbekoorn H., Bouton N., van Opzeeland I., Coers A., ten Cate C., Popper A. N. (2010). A noisy spring: the impact of globally rising underwater sound levels on fish. Trends Ecol. Evol. 25 (7), 419–427. doi: 10.1016/j.tree.2010.04.005
Slabbekoorn H., Dalen J., de Haan D., Winter H. V., Radford C., Ainslie M. A., et al. (2019). Population-level consequences of seismic surveys on fishes: An interdisciplinary challenge. Fish Fisheries 20 (4), 653–685. doi: 10.1111/faf.12367
Smith M. E., Kane A. S., Popper A. N. (2004). Noise-induced stress response and hearing loss in goldfish (Carassius auratus). J. Exp. Biol. 207 (3), 427–435. doi: 10.1242/jeb.00755
Spellerberg I. F., Fedor P. J. (2003). A tribute to claude shanno–2001) and a plea for more rigorous use of species richness, species diversity and the ‘Shannon–Wiener’Index. Global Ecol. biogeography 12 (3), 177–179. doi: 10.1046/j.1466-822X.2003.00015.x
Subasinghe R., Soto D., Jia J. (2009). Global aquaculture and its role in sustainable development. Rev. aquaculture 1 (1), 2–9. doi: 10.1111/j.1753-5131.2008.01002.x
Tang Q., Liu H. (2018). Development strategies and prospects – driving forces and sustainable development of Chinese qquaculture," in Aquaculture in China. Eds. J. Gui, Q. Tang, Z. Li, J. Liu and S. S. De Silva. doi: 10.1002/9781119120759.ch8_1
Thukral A. K. (2017). A review on measurement of Alpha diversity in biology. Agric. Res. J. 54 (1), 1–10. doi: 10.5958/2395-146X.2017.00001.1
Todd V. L., Williamson L. D., Jiang J., Cox S. E., Todd I. B., Ruffert M. (2021). Prediction of marine mammal auditory-impact risk from Acoustic Deterrent Devices used in Scottish aquaculture. Mar. pollut. Bull. 165, 112171. doi: 10.1016/j.marpolbul.2021.112171
Voellmy I. K., Purser J., Flynn D., Kennedy P., Simpson S. D., Radford A. N. (2014). Acoustic noise reduces foraging success in two sympatric fish species via different mechanisms. Anim. Behav. 89, 191–198. doi: 10.1016/j.anbehav.2013.12.029
Wale M. A., Simpson S. D., Radford A. N. (2013a). Size-dependent physiological responses of shore crabs to single and repeated playback of ship noise. Biol. Lett. 9 (2), 20121194. doi: 10.1098/rsbl.2012.1194
Wale M. A., Simpson S. D., Radford A. N. (2013b). Noise negatively affects foraging and antipredator behaviour in shore crabs. Anim. Behav. 86 (1), 111–118. doi: 10.1016/j.anbehav.2013.05.001
Ward A. J., Webster M. M., Hart P. J. (2006). Intraspecific food competition in fishes. Fish Fisheries 7 (4), 231–261. doi: 10.1111/j.1467-2979.2006.00224.x
Webb P. W. (1984). Body form, locomotion and foraging in aquatic vertebrates. Am. zoologist 24 (1), 107–120. doi: 10.1093/icb/24.1.107
Wei D., Bao E., Wen Y., Zhu S., Ye Z., Zhao J. (2021). Behavioral spatial-temporal characteristics-based appetite assessment for fish school in recirculating aquaculture systems. Aquaculture 545, 737215. doi: 10.1016/j.aquaculture.2021.737215
Williams R., Wright A. J., Ashe E., Blight L. K., Bruintjes R., Canessa R., et al. (2015). Impacts of anthropogenic noise on marine life: Publication patterns, new discoveries, and future directions in research and management. Ocean Coast. Manage. 115, 17–24. doi: 10.1016/j.ocecoaman.2015.05.021
Wysocki L. E., Davidson J. W. III, Smith M. E., Frankel A. S., Ellison W. T., Mazik P. M., et al. (2007). Effects of aquaculture production noise on hearing, growth, and disease resistance of rainbow trout Oncorhynchus mykiss. Aquaculture 272 (1-4), 687–697. doi: 10.1016/j.aquaculture.2007.07.225
Zhang X., Guo H., Chen J., Song J., Xu K., Lin J., et al. (2021). Potential effects of underwater noise from wind turbines on the marbled rockfish (Sebasticus marmoratus). J. Appl. Ichthyology 37 (4), 514–522. doi: 10.1111/jai.14198
Keywords: aerator noise, largemouth bass, swimming behavior, feeding, growth
Citation: Zhang Y, Shitu A, Hang S, Ye Z, Zhao H, Xu W, Zhao J and Zhu S (2023) The effects of aerator noise on the swimming, feeding, and growth of Micropterus salmoides. Front. Mar. Sci. 10:1242793. doi: 10.3389/fmars.2023.1242793
Received: 19 June 2023; Accepted: 14 September 2023;
Published: 05 October 2023.
Edited by:
Rosario Martínez-Yáñez, University of Guanajuato, MexicoReviewed by:
Zonghang Zhang, Shantou University, ChinaCopyright © 2023 Zhang, Shitu, Hang, Ye, Zhao, Xu, Zhao and Zhu. This is an open-access article distributed under the terms of the Creative Commons Attribution License (CC BY). The use, distribution or reproduction in other forums is permitted, provided the original author(s) and the copyright owner(s) are credited and that the original publication in this journal is cited, in accordance with accepted academic practice. No use, distribution or reproduction is permitted which does not comply with these terms.
*Correspondence: Songming Zhu, emh1c21Aemp1LmVkdS5jbg==
Disclaimer: All claims expressed in this article are solely those of the authors and do not necessarily represent those of their affiliated organizations, or those of the publisher, the editors and the reviewers. Any product that may be evaluated in this article or claim that may be made by its manufacturer is not guaranteed or endorsed by the publisher.
Research integrity at Frontiers
Learn more about the work of our research integrity team to safeguard the quality of each article we publish.