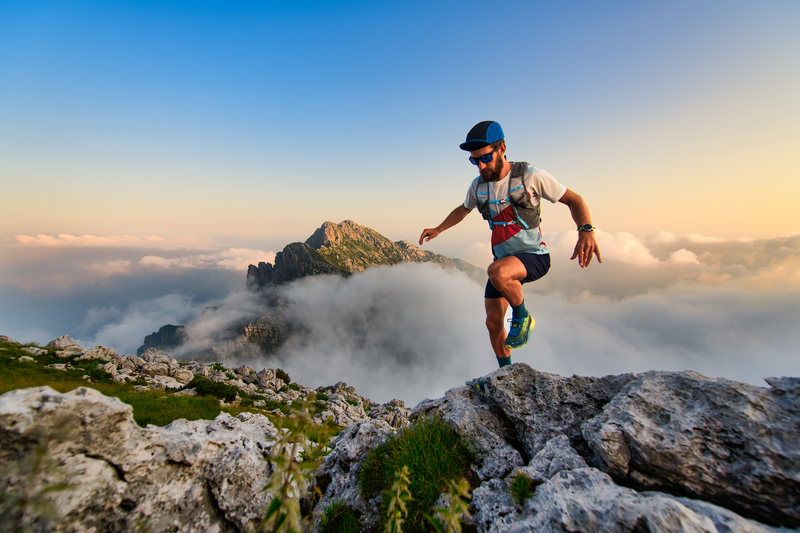
94% of researchers rate our articles as excellent or good
Learn more about the work of our research integrity team to safeguard the quality of each article we publish.
Find out more
ORIGINAL RESEARCH article
Front. Mar. Sci. , 21 July 2023
Sec. Marine Pollution
Volume 10 - 2023 | https://doi.org/10.3389/fmars.2023.1215251
This article is part of the Research Topic Coastal Environmental Quality and Marine Biodiversity Assessment, volume II View all 10 articles
The Korea Strait (KS) is a crucial marine passage for transporting heat, salt, and materials from the South Sea to the East Sea. The Tsushima Warm Water (TWW) and Korea Strait Bottom Cold Water (KSBCW) are major water masses that flow across the strait, but their effects on prokaryotic communities have been unclear. We used high-throughput sequencing to study the impact of TWW and KSBCW on prokaryotic composition and metabolic changes in the upper (0–50m; UL), middle (50–75m; ML), and bottom (75–150m; BL) layers during the fall of 2018 and 2019. The results showed that the UL had a freshwater influence from Changjiang Diluted Water in 2019, altering prokaryotic compositions and metabolic potentials. The KSBCW in the BL transported new bacterial communities with unique metabolic characteristics. Key genes involved in carbon metabolism had water mass impacts, preferring lower saline and temperature environments, and carbon fixation potential shifted from phototrophs in 2018 to chemotrophs in 2019. Temperature changes induced acclimation processes producing heat- and cold-shock genes/proteins. Our findings indicate that the freshwater influence and KSBCW modified the prokaryotic composition and metabolic function differentially. These results are important in understanding the relationship between water masses and ongoing environmental changes in this understudied region.
Prokaryotes are critical components of the ocean ecosystem, accounting for 70% of the total biomass on the ocean surface and 75% of the total biomass in the deep ocean (Fuhrman et al., 1989; Arístegui et al., 2009). They significantly contribute to ocean biogeochemical cycles via energy transmission and organic matter remineralization (Mason et al., 2003). Previous studies have found that prokaryotic abundance on the surface differs from that in the deep ocean (Tseng et al., 2015; Zorz et al., 2019; references therein). For example, Flavobacteria, Cyanobacteria, and Alphaproteobacteria are the dominant groups in the upper layer, whereas Alpha, Gamma, and Deltaproteobacteria dominate the middle layer (Walsh et al., 2016). Increased data have demonstrated that the richness of prokaryotes also varies with depth, that is, some research on bacteria describes increasing richness with depth (Walsh et al., 2016), whereas other studies detect declines (Bryant et al., 2016). This is because depth is closely related to the physical and biogeochemical properties of the water columns under unique conditions (Stocker, 2012). Thus, in-depth research on prokaryotic organisms is valuable (Wang et al., 2021a), as knowledge of their metabolic processes allows us to build ecological and biogeochemical models (Ducklow, 2000; Del Giorgio and Williams, 2005).
Physical mixing among different water masses is a crucial factor that influences the prokaryotic composition in the ocean (Venkatachalam et al., 2017). Several studies have revealed that the dynamics of different water masses, each with its physicochemical properties, produce differences in prokaryotic physiology and composition (Shade et al., 2008; Varela et al., 2008; Celussi et al., 2010; Galand et al., 2010; Laque et al., 2010). Physical properties such as temperature (T) and salinity (S) gradients in water masses are important driving factors in determining the prokaryotic population due to their biological mediation within cells. These properties affect prokaryotic photosynthetic activity, carbon, nitrogen, and sulfur metabolism, and other functional processes in various regions including the Western Sub-Arctic (Li et al., 2018), North Atlantic (Agogué et al., 2008), Eastern North Atlantic (Varela et al., 2008), Arctic Ocean (Galand et al., 2009), and Beaufort Sea (Fu et al., 2019). Water masses at the same location can be vertically classified into different types based on their T-S relationship. In the Southern Ocean, for example, the upper layer occupied by the Antarctic Surface Water that features warmer and less S conditions, while the middle layer occupied by the Winter Water with colder and less-S characteristics, and the deep layer occupied by the Circumpolar Deep Water that has warmer and high-S conditions (Randall-Goodwin et al., 2014).
The Korea Strait (KS) is a shallow channel (bottom depth <~200m) with a length of around 330 km and a breadth of approximately 140 km (Teague et al., 2006). It is situated between Korea and Japan and is separated into western and eastern channels by Tsushima Island. It serves as the sole pathway for transporting heat, salt, and materials from the South Sea to the East Sea (Figure 1A). Comprehending the inflow mechanism of the Korea Strait (KS) is vital in assessing and predicting alterations in the circulation of the East Sea (Teague et al., 2006). The major water masses flowing across the KS are Tsushima Warm Water (TWW) and Korea Strait Bottom Cold Water (KSBCW). The TWW, a branch of the Kuroshio Current, is the primary surface to mid-layer current (Teague et al., 2006) flowing northward from the Pacific Ocean to the East Sea (Lee et al., 2010), with an annual mean transport of ~2.5 Sv [1 Sv:106 m3 s -1] (Na et al., 2009). TWW is distinguished into two water types based on T-S relationship: Tsushima Surface Water (TSW; T>20°C and S<34.0 PSU) and Tsushima Middle Water (TMW; T:12–17°C and S>34.2 PSU) (Chang and Isobe, 2003; Moon et al., 2009). The KSBCW (T<10°C and S>34.0 PSU) is inhabited by a cold underwater running beneath the TMW in the western channel (Kim and Lee, 2004) originating from the East Sea and flowing southward along the Korean coast (Lee et al., 2010), with a monthly mean transport of approximately 0.35 Sv (Kim et al., 2006). In KS, approximately half of the transport was contained in the upper 50m and 10% occur below 100m, while the remaining was transported between ~50–100m (Teague et al., 2006).
Figure 1 (A) Sample collecting points from the Korea Strait. (B) The potential temperature and salinity distribution during fall 2018; (C) the potential temperature and salinity distribution during fall 2019. Tsushima Surface Water (TSW; T>20°C and S<34.0 PSU), Tsushima Middle Water (TMW; T: 12–17°C and S>34.2 PSU), and Korea Strait Bottom Cold Water (KSBCW; T<10°C and S>34 PSU).
Previous research has revealed that KS is the most dynamic environment due to its topography, water mass, and river discharge (i.e., from Changjiang River) (Lee et al., 2010). The annual freshwater (FW) discharge from the Changjiang River to the KS has been estimated to be 9.25×1011 m3 yr-1 (Tian et al., 1993), which alters the hydrographic conditions (low S and high T) and modifies biological processes in the receiving region (Liu et al., 2003; Jiao et al., 2007; Onitsuka et al., 2007; Yoon et al., 2022). Yang et al. (2000) demonstrated that the phytoplankton ecosystem changes along the KS because of the influence of T variation by TWW and excessive nutrient loading by FW. Regarding bacterial dynamics in KS, there have been few studies on organic matter production as a result of changes in hydrographic conditions (Chung and Kang, 1995; Kang and Kang, 2002). Lee and Choi (2021) and Kim et al. (2022) showed that the composition of distinct water masses in the East Sea’s Ulleung basin, i.e., TWW, has a major impact on bacterial production and diversity. Similarly, Ichinomiya et al. (2019) found that fluctuations in T and S of the TWW in the northern Japan basin significantly influence microbial abundance and diversity. Given that over 90% of the world’s ocean floor has a temperature of less than approximately 4°C (Levitus and Boyer, 1994), microorganisms inhabiting this environment must have adapted to the cold conditions. This has resulted in unique metabolic activity and species diversity compared to those found in the upper layers of the ocean (Knoblauch and Jørgensen, 1999). Similarly, the bottom cold water mass from the Yellow Sea with higher S and lower T (similar to the KSBCW characteristics) exhibited different prokaryotic dominance in the bottom layer compared to that observed in the surface layer (Wang et al., 2021a).
Despite the distinct characteristics of warm water mass (i.e., TWW) in the surface layer and cold-water mass (i.e., KSBCW) in the bottom layers of KS, no studies have been conducted to define these water mass impacts on prokaryotic composition and distribution (surface to bottom) that change over time. Furthermore, the absence of genomic information in KS hampered our knowledge of the impact of different water masses on prokaryotic metabolic processes to build a biogeochemical model of this important location. In this study, therefore, we aimed to (1) identify and characterize the different water masses from the surface to bottom layers during the fall of 2018 and 2019, (2) find their unique driving factors in determining the prokaryotic composition and distribution, and (3) investigate the response of metabolic potential according to the composition of different water masses.
Seawater samples from 0 to 150 m were collected at four stations for physicochemical and sequencing analyses using R/V Nara in October 2018 and 2019 from KS. Seawater was collected in Niskin bottles on a rosette sampler. At all stations, the T, S, and dissolved oxygen (DO) profiles were determined using an SBE 911 Plus (CTD; Sea-Bird Electronics, USA) sensor system attached to a rosette sampler. The accuracies of T, conductivity, and DO sensors were ± 0.001°C, ± 0.0003 S m-1, and ± 2% of the full-scale range, respectively. To determine the nutrient concentrations of nitrite (NO2−) and nitrate (NO3−), seawater samples were collected in 15 ml pre-acid rinsed plastic bottles and placed at –20°C until further analysis. Nitrite (NO2−) and nitrate (NO3−) were analyzed in the laboratory using a continuous flow auto-analyzer (QuAAtro 39, Seal Analytical, UK). The analytical precision of the nutrients was greater than 1%. Nutrient measurements were performed using the same method as that used by Hydes et al. (2010) in the GO-SHIP protocol, and reference materials (Kanso RMNS) were used to track the performance of the system and the consistency of the nutrient data. The sum of nitrite and nitrate measured in this study was defined as dissolved inorganic nitrogen (DIN). For DNA extraction, 2 L of seawater was filtered through 0.22 μm cellulose ester membrane filters (Millipore, Ireland) to capture bacterial cells. The filtered samples were immediately frozen and stored at −80°C until DNA extraction. A total of 24 samples for sequencing analysis were obtained during the study period (4 stations × 3 depths × 2 years) across the upper layer (UL; <50m), middle layer (ML; ~50-75m), and bottom layer (BL; >75m).
DNA was extracted from 24 genome samples using a DNA isolation kit (Qiagen, Germany) and cross-checked using a NanoDrop (Thermo Scientific, USA). After the quality check (Phred > = 20) (Bokulich et al., 2013), a 16S rRNA library was prepared to target the V3 and V4 hypervariable regions (Co. 3BIGS, South Korea, https://3bigs.com) based on RNA genes in chromosomes. In brief, 50 ng of genomic DNA was used to amplify the 16S rRNA gene v3 region for 26 cycles using a PCR kit. The forward and reverse primer concertation was kept at 0.2 µm each. The PCR was validated using positive control and non-template control samples. The Illumina adapted sequences were: forward, 5′- TCGTCGGCAGCGTCAGATGTGTATAAGAGACAGTCGTCGGCAGCGTCAGATGTGTATAAGAGACAGCCTACGGGNGGCWGCAG, and reverse, 5′- GTCTCGTGGGCTCGGAGATGTGTATAAGAGACAGGTCTCGTGGGCTCGGAGATGTGTATAAGAGACAGGACTACHVGGGTATCTAATCC-3. The 16s rRNA sequencing aimed to cover the high coverage diversity in this study following the protocol described by (Chakravorty et al., 2007; Klindworth et al., 2013). The extracted samples were analyzed and amplified using Illumina MiSeq (MiSeq control software v2.4.1.3) and real-time analysis (v1.18.54.0). 16S rRNA targeting was performed using a quantitative kit for the DNA samples. Primers were prepared for all DNA fragments and subjected to quality checks using a Bioanalyzer trace system, followed by clustering. The paired-end reads were demultiplexed, trimmed, and quality-filtered before assembling the reads using QIIME1.9.1. (Price et al., 2009) and USEARCH7 was used to detect or filter OTUs. The National Center for Biotechnology Information (NCBI), and SILVA 138.1 databases were used to determine the similarity (97%) of OTUs using UCLUST and close reference analyses. The OTU table was normalized to the 16S copy number abundance by dividing each OTU. After filtration, the generated OTU table using the Biological Observation Matrix format was clustered into OTU based on ≥97% similarity threshold using the Python Nearest Alignment Space Termination method (PyNAST) (Caporaso et al., 2010). We performed comparative OTU assignments with the database in terms of phylum, class, order, family, and genus separately, using RDP classifiers. Permutational multivariate analysis of variance (PERMANOVA) was used to observe significant differences among groups. The relative abundances of individual taxonomic classifications were estimated using STAMP (V2.1.3). The generated 16S rRNA data were submitted to the NCBI Sequence Read Archive (accession no. PRJNA898851).
The predictive functional genes/potential of the prokaryotic communities was obtained using the Silva-Tax4Fun (Aßhauer et al., 2015). The SILVA-labeled OTU tables were used as an input file in Tax4Fun, which is an open-source R package. The SILVA-labeled OTUs are transformed into prokaryotic KEGG organisms in Tax4Fun, and the 16S rRNA copy number from the NCBI genome annotation is used to further standardize the data. The prokaryotic communities were assigned to their predictive functions by linearly combining the normalized taxonomic abundances into the precomputed association matrix of KEGG Ortholog reference profiles to Silva-defined microorganisms constructed by Tax4Fun (Wemheuer et al., 2020). The difference in the abundance of functional genes among the samples was tested with the Tukey-Kramer test (STAMP V2.1.3.) following the protocol described by (Parks et al., 2014).
The surface S data used in this study for October 2018 and 2019 were obtained from Ocean Color, NASA (https://oceancolor.gsfc.nasa.gov/). The physiochemical parameters were drawn using Ocean Data View (v 5.6.3) and correlation analysis was performed using R software (v1.3.109).
We used a T-S diagram to determine the physiochemical characteristics for water mass analysis during the fall of 2018 and 2019 (Figures 1B, C). Based on T-S diagram, two different water masses (i.e., TSW and TMW) in fall 2018 (Figure 1B) and four different water masses (i.e., FW, TSW, TMW, and KSBCW) in fall 2019 (Figure 1C) were likely to have participated in the physical mixing process in the western channel of KS. In particular, TSW with high T and low S (T>20°C and S<34.0 PSU) and TMW with low T and high S (~12<T<17°C and S>34.0 PSU) were observed in both years. However, compared to the fall of 2018, the BL of 2019 showed unique KSBCW characteristics with lower T and higher S (T<10°C and S>34.0 PSU), and the UL of 2019 showed lower S (<32.0 PSU) due to FW input. These results collectively suggest that during the fall of 2018, TSW influences across the UL (<50m), and TMW extends from ML to BL (~50m–140m), whereas during the fall of 2019, the UL was dominated by TSW with FW influence, ML by TMW, and BL (>75m) by the KSBCW.
In detail, during the fall of 2018, the UL showed a warmer T range of 20.40–21.42°C and a S range of 32.99–33.46 PSU (Figures 2A, B), while compared to the UL, the ML (~40–70m) T was reduced (12.11–20.42°C) and the S was elevated (33.31–34.46 PSU). This suggests that the higher T and lower S in the UL of 2018 were influenced by the TSW. Unlike in 2018, stations 1 and 2 in the UL of 2019 showed the different temperature (Figure 2E) and lower range of S (<32 PSU) than those observed at the same stations in 2018 (>33 PSU) (Figures 2B, F). This low-S water indicates FW influence from the Changjiang Diluted Water (CDW) from the East China Sea (ECS) (Figure S1) (Chang and Isobe, 2003; Gomes et al., 2018; Kim et al., 2022; Yoon et al., 2022). Similar to the S variation, the UL T in the fall of 2019 was higher (21.76–24.26°C) than that observed in the fall of 2018. The T in the BL (70–140 m) also varied between 2018 and 2019. Specifically, the T in BL ranged from 11.71 to 16.01°C in 2018, while the T in 2019 ranged from 4.97 to 18.22°C. In particular, the low-cold water of the KSBCW (T<10°C) was dominant in the BL during the fall of 2019. The DO profile also differed between the fall of 2018 and 2019 (Figures 2C, G). For example, in the fall of 2018, the UL DO was influenced by TSW with a range of 126.88–150.89 μmol L-1, while in the fall of 2019, TSW along with FW influences increased DO ranges from 203.61–248.61 μmol L-1. Similarly, the ML DO in the fall of 2018 ranged from 91.08–139.43 μmol L-1, whereas the DO in the fall of 2019 was higher with a range of 119.21–215.71 μmol L-1. DIN distribution in UL ranged from 1.28–13.57 μmol L-1 in the fall of 2018 (Figure 2D), while in the fall of 2019, it showed a reduction ranging from 0.88–4.93 μmol L-1 (Figure 2H). On the opposite, the BL in fall 2019 detected with higher DIN (11.80–21.45 μmol L-1) than those in fall 2018 BL (2.28–15.92 μmol L-1), indicating that KSBCW transported higher nutrients to the KS from the East Sea during fall 2019. Taken together, during the fall of 2018, the UL was occupied by TSW (i.e., low-S and high-T characteristics), and the ML/BL was dominated by TMW (i.e., high-S characteristics). The UL was influenced by a mixture of FW (Sts. 1–2) and TSW (Sts. 3–4), the ML was dominated by TMW, and the BL was occupied by the KSBCW (i.e., low-T characteristics) during the fall of 2019 (Table 1).
Figure 2 Distribution of temperature, salinity, dissolved oxygen (DO), and dissolved inorganic nitrogen (DIN) from sampled depths at different layers. (A–D) samples collected from 2018; (E–H) samples collected from 2019. While squares indicate the samples obtained for genomic analysis.
Table 1 Characteristics of water masses identified during the fall of 2018 and 2019 in the Korea Strait.
After assembly, 251,120 OTUs were identified from samples collected in the fall of 2018, whereas 2,211,501 OTUs were identified in samples collected in the fall of 2019 (Supplementary Table 1). From the 2018 samples, 46 classes, 93 orders, 173 families, and 389 genera were identified, while 39 classes, 85 orders, 148 families, and 350 genera were identified in the 2019 samples. This shows that while there were more OTUs in the fall of 2019 (i.e., high diversity), the prokaryotic abundance was greater (i.e., high density) in the fall of 2018. In particular, the phylum Proteobacteria accounted for 57% of total species in fall 2018 and 52% in fall 2019. However, Bacteroidetes accounted for 12% in the fall of 2018 and 27% in the fall of 2019, and Actinobacteria accounted for 7% in the fall of 2018 and 8% in the fall of 2019. Notably, cyanobacteria accounted for 6.5% of the total abundance in the fall of 2018, nearly twice compared to the fall of 2019 (3.65%).
The dominant 25 orders (92% of total abundance) revealed that relative abundance (i.e., specific abundance is divided by total abundance) varied among each layer, representing the different water mass influences (Figure 3). In UL 2018, for example, the Flavobacteriales showed 16.04% total abundance, which increased to 33.28% in 2019 UL with FW region (i.e., Sts. 1–2) and to 47.03% at high T region (i.e., Sts. 3–4) (Figure 3A). Similarly, Rhodobacterales in UL showed an increasing trend from 9.69% in 2018 to 18.41% in the FW region and 13.95% with a high T region in 2019. In contrast, the Pelagibacterales decreased from 14.63% during the fall of 2018 to 13.54% in the FW region and 6.64% in the high T region during the fall of 2019; Synechococcales decreased from 9.35% during the fall of 2018 to 2.10% in FW region and 5.23% in high T region during the fall of 2019. These variations might be the cause of environmental changes caused by the distribution of distinct water masses. The main orders (15 most abundant) were correlated with the environmental parameters (Figure 4). In the fall of 2018 UL, dominant communities such as Pelagibacterials, Flavobacteriales, and Synechococcales were positively correlated with T and DIN. In the fall of 2019, Pelagibacterials and Synechococcales were positive, while Flavobacteriales and Rhodobacterales were negatively correlated with S (at Sts. 1–2). However, under high T conditions (at Sts. 3–4) these Pelagibacterials and Synechococcus showed negative correlations, while other communities showed positive correlations with T. Together, this suggests that the UL prokaryotic composition is mainly driven by the T and DIN in fall 2018, and S and T in fall 2019.
Figure 3 Prokaryotic diversity and density in order level. The dominant orders encompass 92% of the total abundances are listed here. (A) Results obtained from 2018 and 2019 UL (upper layer); (B) results obtained from 2018 and 2019 ML (middle layer), and (C) results obtained from 2018 and 2019 BL (bottom layer). UL 1-2 represents FWI fresh water influence (Sts. 1–2), and (Sts. 2–4), represents high temperature condition. The description of abundance in order are shown in Supplementary Table 2.
Figure 4 Spearman correlation between the environmental parameters and the prokaryotic families. This correlation analysis was conducted with the major abundant 15 families, but, correlations with R values greater than or equal to 0.3 are displayed here. Red lines indicate positive correlations; dark blue lines indicate negative correlations; sky blue lines indicate negative correlations with environmental factors; the thicker the lines, the stronger the correlations. UL (upper layer), ML (middle layer), and BL (bottom layer).
The shallow shelf region is a transitional zone with significant anthropogenic runoff and strong impacts from diverse water masses (Agardi et al., 2005). Several variables, including S (Zäncker et al., 2018), T (Bachmann et al., 2018), nutrients (Raes et al., 2018), and DO availability (Aldunate et al., 2018), have been shown to have a major influence on bacterial populations in such regions. In this study, we found that T and S variables have a substantial influence on all layers (Figure 4), suggesting that water mass is the primary determinant of bacterial composition. Specifically, FW has a significant impact on the physical properties of seawater, causing changes in the bacterial population (Fu et al., 2019). Compared to UL 2018, the UL 2019 (Sts. 1–2) exhibited low-S waters (S<32.0 PSU), indicating a FW effect from the CDW from the ECS (Chang and Isobe, 2003; Gomes et al., 2018; Kim et al., 2022). The Changjiang River has the world’s third largest and the fifth-largest in FW discharge (Beardsley et al., 1985). According to previous studies, 70% of CDW is transferred annually via KS to the East Sea (Chang and Isobe, 2003; Senjyu et al., 2006; Moon et al., 2019). As a result, CDW influence plays a significant role in the large biological effects on the ECS and Korean coastal environment (Son and Choi, 2022) and alters phytoplankton composition (Yoon et al., 2022). Within the past ten years, the CDW has had the largest FW discharge in 2019-2020 followed by 2016 and 2017, but there was no significant FW discharge in 2018 (Son and Choi, 2022). Following this, the satellite observations of sea surface S show that the CDW influence from the ECS to the KS was significantly larger in fall 2019 than in fall 2018 (Figure S1). The contrasting T and S characteristics observed in the 2018 and 2019 UL samples were indicative of differences in the composition of the bacterial communities. Specifically, changes in Pelagibacterials and Synechococcales in the UL demonstrated how FW discharge distinguishes their distribution patterns and responds to environmental conditions. The populations of Pelagibacterials and Synechococcales showed a positive correlation with S in the fall of 2019 UL and a positive association with T in the fall of 2018 UL. When the S decreased in the fall of 2019 (Sts. 1–2), Flavobacteriales increased (33.28%) nearly double compared to the fall of 2018 (16.04%), and Synechococcales decreased almost four times (2.10%) in 2019 compared to the fall of 2018 (9.35%) UL. This suggests that S and T were the key factors in determining the dominant communities in the UL of KS, specifically T alone in the fall of 2018 and T and FW (S reduction mainly) in the fall of 2019.
Unlike UL, several communities in ML did not show much variation, i.e., the relative abundance of Pelagibacterales in the fall of 2018 showed 18.90%, which is similar to the fall of 2019 (16.26%), and those of Oceanospirillales showed 4.28% in 2018 and 5.08% in 2019, respectively. However, few communities in ML showed exceptional variation, such as Flavobacteriales and Synechococcales, which showed approximately four-fold elevation in fall 2019 compared to fall 2018. Although several community abundances were similar in both years of ML, they were differentially correlated with T variation (Figure 4). In BL, although dominant communities such as Pelagibacterales, Synechococcales, and Flavobacteriales showed similar abundances between the falls of 2018 and 2019, several new communities (i.e., Saprospirales, Clostridiales, Pseudomonadales, Nitrosomodales, Sphingomonadales, and Desulfomonadales) were detected in the fall of 2019 as high abundance; interestingly, all these communities were negatively correlated with T (<10°C), which is a unique feature of KSBCW.
The vertical structure of water masses has been considered another factor for microbial dispersal due to various hydrological qualities that function as barriers to mixing among distinct water masses (Matsuoka et al., 2012). The same bacterial groups respond differentially to different water masses vertically (Fu et al., 2019), and thus were isolated from each other due to their limited mobility (Spencer-Cervato and Thierstein, 1997). A time series study in Southern California found that when vertical stratification was weak during the winter, the bacterial composition was homogenized but became divergent vertically when surface water warmed up and stratified (Chow et al., 2013). In this study, the vertical structure of different water masses is caused not only by the T and S properties, which produce a mixing barrier but also by the diverse origins of the water mass at different depths. The TWW (TSW+TMW) originated in the warm equatorial Pacific area and flowed from the surface to the middle layer (Teague et al., 2006), whereas the cold bottom KSBCW originated in the East Sea (Lee et al., 2010). According to the “barrier to dispersal” theory (Spencer-Cervato and Thierstein, 1997), water masses from different origins would transport their source bacterial populations and organic materials to a new place, where they are modified by changes in environmental conditions but retain unique signatures in the absence of homogenization with other water sources. This is also supported by a previous study in the western channel of KS, where the vertical water-column structure of the TMW and KSBCW showed changes in phytoplankton composition due to vertically different T and S properties (Shon et al., 2008). Furthermore, the bacterial composition in TWW showed a horizontal similarity, but not in its vertical distribution in the East Sea (Lee and Choi, 2021). All these findings support our assumption that the observed several new communities and higher DIN in BL during the fall of 2019 might be brought along with the KSBCW and suggest that T and DIN were the primary factors. Taken together, it is observed that in UL compared to 2018, FW in 2019 modified the prokaryotic composition, whereas no significant change was observed in ML, but several new communities were brought to BL in 2019 along with KSBCW compared to 2018 without KSBCW. To better understand the metabolic potential of distinct prokaryotic communities driven by different water masses, we further explored their metabolic potential/functional characteristics in the following section.
Water mass intrusion, such as prokaryotic composition, affects photosynthetic characteristics (Figure S2). During the fall of 2018 and 2019, 46 genes from four sub-divisions were found, including 11 genes of photosystem I (PSI), 24 genes of photosystem II (PSII), 7 genes of the light-harvesting complex, and 4 genes of a reaction center. In comparison with ML and BL, the UL showed high relative abundances of photosynthesis genes; specifically, compared with the UL in the fall of 2019, the UL samples obtained in the fall of 2018 showed high gene abundance. In general, photosynthesis occurs mostly in the surface ocean than in deep environments, owing to light availability. Global ocean Synechococcus data suggest that high T have a vital influence on UL, where the majority of their population resides as a primary photosynthetic community (Zinser et al., 2007; Flombaum et al., 2013; Kent et al., 2016). In this study, we observed high Synechococcales abundance in the UL compared to ML and BL (Figure 3), which were similar to sequencing data from the Indian Ocean (Wang et al., 2021b) and Western Subarctic Pacific Ocean (Li et al., 2018). Similar to photosynthesis, compared to the 2019 UL samples, the 2018 UL samples showed high Synechococcales abundance (Figure 3A).
Different variables influence cyanobacteria in the upper ocean (Wang et al., 2021b), with the T being the greatest driver, followed by DIN, on their metabolic adaptability (Thompson et al., 2017). In this study, the T in 2019 UL (21.77–24.27°C) showed elevation compared to the T in 2018 UL (20.40–21.42°C), but we found that DIN was greater in 2018 UL (1.28-13.57 μmol L-1) than in 2019 UL (0.88-4.93 μmol L-1) and that our correlation analysis (Figure 4) revealed a positive interaction between DIN and Synechococcales in 2018 UL, which was not seen in 2019 UL. This suggests that although the T is a vital factor for Synechococcales, DIN has a greater influence on their composition because it tends to synthesize more sugar (Li et al., 2019) to create more proteins for phytoplankton adaption (Thangaraj and Sun, 2021) in the ocean environment (Zubkov et al., 2003). Shim and Yoon (2021) recently studied the long-term (20-year; 1999-2018) nitrate fluctuation in the East Sea and concluded that the TWW from the CDW, coupled with the input of other rivers (e.g., Changjiang, Nakdong, Taehwa, and Hyungsan), influences the nutrient concentration in the Kuroshio Current extension and Korean coastal environment (Asanuma et al., 2014; Yoon et al., 2022). Based on these earlier findings and our results, we hypothesized that although TSW influenced T more than photosynthetic properties in 2018 and 2019, DIN availability was a significant parameter from the surrounding rivers to determine the high photosynthetic potential in the UL of 2018.
Several genes encoding various carbon-fixing pathways were identified during the study period (Figures 5, 6). Among these, certain Calvin cycle genes had similar abundances between fall 2018 and 2019 from UL to BL (Figures 5), and did not show significant variation regardless of different water mass compositions, that is, ribulose 1,5-bisphosphate (RuBisCO), a key carbon fixation gene, phosphoribulokinase (PRK), which catalyzes ATP-dependent phosphorylation, phosphoglycerate kinase (PK) involved in carbohydrate degradation, and glycerol-3-phosphate dehydrogenase (GPDH) associated with redox conversion. However, fewer genes showed a notable variation only in the UL, such as the aldolase enzyme, which helps in sugar breakdown and energy generation, revealing that a high abundance was in the UL (34%; occupied by TSW) in the fall of 2018 than those compared its abundances in the fall of 2019 UL (30%; occupied by FW and TSW). Similarly, the ribose 5-P isomerase, involved in energy conversion, was abundant in UL (34% in 2019) and (27% in 2018). We analyzed these specific gene abundances in the fall of 2019 to understand FW influence (Figure S3) and noticed that the Sts. 1–2 influenced from the FW increased aldolase levels compared to the Sts. 3–4 under high-T conditions by TSW influence. In contrast, FW region decreased ribose 5-P isomerase activity compared to that observed at higher T. In addition, several other key genes were differentially modified by FW, either by increasing fructose bisphosphate (FBP) and transketolase (TKT) or decreasing sedoheptulose bisphosphates (SBPase) and ribose 5-p isomerase. We observed that the relative abundance of these key genes in Sts. 3–4 (with TSW) was similar in the fall of 2018 and 2019, regardless of T variation. This suggests that, although the UL T (Sts. 3–4) driven by TSW in the fall of 2019 is higher than that in the fall of 2018, it does not have a significant impact on Calvin cycle genes; however, the FW (Sts. 1–2) significantly altered the abundance of these genes.
Figure 5 The relative abundance of genes/enzymes involved in the Calvin cycle (the reductive pentose phosphate pathway). The bar chart represents samples obtained from 2018; the pie chart represents samples obtained from 2019. UL (upper layer), ML (middle layer), and BL (bottom layer). The description, of abundance and function of each gene shown here, are given in Supplementary Table 3. The carboxylation steps involving (RuBisCO) Ribulose 1,5-bisphosphate carboxylase oxygenase is highlighted in the red bubble with inorganic carbon as the source of carbon dioxide (CO2) in yellow. Known/suspected enzymes control or limiting over Calvin cycle activity are shown in purple text. Oxygenation of RuBisCO is shown as a dashed arrow and canonical phosphoglycerate-process photorespiratory pathways are shown as brown text. Reactions generating or requiring ATP or NADPH are highlighted in a circle, although NADH may be substituted for NADPH in some organisms in some reactions. The entry and exit are shown in yellow circles and oxygen is shown in red. Major points for the intermediate existence of the Calvin cycle are shown in red arrows.
Figure 6 The relative abundance of genes/enzymes involved in multiple/alternative carbon fixation pathways. The bar chart represents samples obtained from 2018; the pie chart represents samples obtained from 2019. UL (upper layer), ML (middle layer), and BL (bottom layer). Schematic of intermediates and enzymes of four of the five (non-Calvin cycle) alternative pathways utilized by chemolithotroph and autotrophic microorganisms. All pathways use the common strategy of cycling between acetyl-CoA (circumference) and succinyl-CoA (center) to form the C2 and C3 carbon products that exit the cycle (acetyl-CoA and pyruvate; shown in blue). These metabolic pathways overlap partially one another concerning enzymes and intermediate usage, which allows them to represent spoked on a circle, with centered on succinyl-CoA. The incorporation of carboxylation steps is indicated by yellow circles (carbon dioxide) and green circles (bicarbonate). The enzymes requiring reactions or co-factors with complete or partial oxygen sensitivity are indicated in red, although some oxygen-resistant alternative enzymes exist for some of these reactions. The description of the abundance and function of each gene shown here are given in Supplementary Table 3.
In addition to the Calvin cycle, various alternative carbon fixation mechanisms that can efficiently fix carbon instead of the Calvin cycle were identified (Figure 6). These alternative pathways are similar in that they insert inorganic carbon into existing carbon backbones and fix carbon through succinyl-CoA/acetyl-CoA cycles, and the enzymes involved in these alternative cycles largely overlap. The need for energy (ATP) and reducing equivalents to fix a carbon molecule are the primary techniques used to determine the variations in these carbon cycle differentiations. These alternative carbon fixation pathways have two features: i) lower ATP required for carbon fixation than the Calvin cycle, and ii) carboxylation enzymes that use bicarbonate rather than dissolved CO2 (Bar-Even et al., 2012; Boyle and Morgan, 2011).
The 3-hydroxy propionate (3-HOP) cycle has a carboxylation enzyme, acetyl-CoA carboxylase, which uses bicarbonate as a substrate. In the UL, this enzyme revealed significant change from the fall of 2018 (8%) to the fall of 2019 (29%). This enzyme is oxygen-insensitive and does not catalyze oxygenation processes, such as RuBisCO (Zarzycki et al., 2009), allowing for high primary productivity (Boyle and Morgan, 2011; Bar-Even et al., 2012). According to Könneke et al. (2014), the chemoautotrophic carbon fixation pathway (for archaea:3-HP/4-HB) is more energy-efficient than the Calvin cycle. In this study, the hydroxy-propionate (involved in the protein interaction) gene in the UL was more prevalent in the fall of 2019 (90%) compared to the fall of 2018 (74%). Furthermore, in UL 2019, methyl malonyl-CoA epimerase (converts succinate to propionate) (65%) and methyl malonyl-CoA mutase (pyruvate to propanoate) (76%), were more abundant than those in 2018 (46% and 40%, respectively). Because these genes were superficially abundant in the fall of 2019, we aimed to explore the role of FW influence (Figure S4) and noticed that S reduction (Sts. 1–2) increased in abundance compared with high T conditions (Sts. 3–4), suggesting that the increased abundance of all these enzymes in the fall of 2019 was mainly modified by S variation rather than T.
Unlike UL, most gene abundances in ML 2018 and 2019 did not show significant changes. However, certain functional genes, such as 4-hydroxybutyryl-CoA synthetase and 3-hydroxybutyryl-Coa, were abundant in 2019 BL samples, which had a lower T (<5°C) than the T range of >12°C in 2018. We also noticed that RuBisCO was more abundant in the BL in 2019 than in the BL in 2018. This implies that these enzymes are susceptible to cooler T in prokaryotic metabolism. Variations in these carbon fixation pathways (Calvin and others) revealed that carbon fixation by phototrophs and chemotrophs was not completely different between 2018 and 2019. However, the S and T sensitivity of certain key genes indicated differing water mass influences: preference for lower S/higher T (UL) and lower T conditions (BL).
The T is a vital physicochemical parameter that influences all biological processes through enzymatic reactions (Franks et al., 1990). T changes can cause prokaryotes to respond in multiple ways to a new environment to maintain consistent metabolic activity and trigger several adaptive modifications (Jaenicke and Sterner, 2006). The maintenance of overall metabolic rates with the fewest changes in molecular composition would activate a less energy-demanding mechanism (Rabus et al., 2002). This might explain why various carbon metabolisms were observed in this study because these multiple carbon pathways have lower energy needs and rely on available bicarbonate raters rather than CO2, such as 3-HP/4-HB, which is a more energy-efficient metabolism than the Calvin cycle. A recent study spanning over 1000 km in the Baltic Sea demonstrated that microbial communities and associated functional gene abundances can be altered not only by T but also by S variation (Broman et al., 2022). They revealed that S was the primary driver of functional gene modifications related to the carbon and nitrogen cycles. Their findings support our hypothesis that S reduction through FW input would greatly modify the functional capabilities of prokaryotic communities in the fall of 2019 UL compared to that in the fall of 2018. Our assumption is also in agreement with studies that have found that S influences the gene abundance of microbial communities in systems such as lakes and estuarine environments (Dai et al., 2018; Yang et al., 2023). For example, a study conducted in the Hangzhou Bay estuary identified S as one of the most influential variables for amino acid catabolism related to gene abundances in nitrogen, sulfur, and phosphorus pathways in prokaryotes (Dai et al., 2018). In our study, the major carbon pathways that changed gene abundance were seemingly controlled by the S variation in the UL of the fall of 2019 compared to that of the fall of 2018. Additionally, S variation has been shown to promote key genes in carbon metabolism (Figures S3, S4), which were more prevalent in the fall of 2019 (Sts. 1–2). Taken together, our results indicate that S variation via FW input has a larger impact on the UL of fall 2019 than relatively stable parameters, such as T, which is similar to earlier observations between S and other environmental parameters (Seidel et al., 2021; Broman et al., 2022).
RuBisCO possesses less sensitivity and higher velocity, which favors a CO2-rich environment, such as rising CO2 levels (Zhu et al., 2010). The RuBisCO gene in the deep ocean was recently identified in high abundance in the Synechococcus community with oxygen-rich surface water exposed to high light conditions (Han et al., 2022). Notably, with lower oxygen levels in the deep ocean, there are numerous Calvin cycle genes, including that abundant RuBisCO in chemoautotrophs, such as Nitrosomonas, Nitrosospira, and Acidomicrobium found (Han et al., 2022). Although large amounts of RuBisCO were found in the UL, they were found in higher abundances in the BL, which had lower oxygen levels than the UL in both years (Figures 3C, G). In particular, high abundances of RuBisCO were noticed in the 2019 BL, which had a lower T and higher DO compared to the 2018 BL. This pattern illustrates that, regardless of the DO reduction in BL lower T >10°C in 2019, which is a unique feature of the KSBCW in this region, the carbon fixation shifted from photoautotrophs to chemoautotrophs compared to that in 2018. In particular, archaeal communities are sensitive to high oxygen concentrations and can maintain higher enzyme activity under low DO and T conditions (Demirci et al., 2020). These large abundances of chemotrophic carbon-fixing genes in BL in 2019 reduced oxygen conditions and could explain the deoxygenated habitat of this archaeal colony. Furthermore, in this study, most enzymes in the archaeal carbon fixation pathway were shown to be T sensitive and so prevalent in BL (specifically in 2019), which could be the reason for their preference for cooler environments (Danovaro et al., 2017). Our results are consistent with those of recent Bohai Sea metagenome and metatranscriptome studies, which found 6 to 33-fold increases in these genes with decreased oxygen concentration (Han et al., 2022). The abundance of Synechococcales and numerous genes in the Calvin cycle in the UL indicates that photoautotrophic carbon fixation at the UL and chemoautotrophic carbon fixation at the BL were more prevalent during this study. In particular, compared to 2018, the UL 2019 was relatively different due to FW influence, and compared to 2018, the BL in 2019 was different (promoting chemoautotroph carbon fixation) primarily driven by lower T.
This study revealed key genes involved in nitrogen fixation (nifH), ammonium assimilation (glnA) ammonium oxidation (amoA), hydroxylamine oxidation (hao), and nitrite oxidation (nxrAB) (Figure 7), and showed that nifH, amoA, hao, and nxrB in UL of both years had significant changes. Specifically, nifH gene abundance in the 2019 UL was greater than that in 2018. Similarly, hao, nxrB genes showed a slight elevation in the UL in 2019 compared to the 2018 UL. In contrast, amoA and glnA in the UL were highly abundant in 2018 compared to 2019. This suggests that compared to the UL in 2018, the metabolic potential related to nitrogen fixation (nifH) and nitrification (hao + nxrB) was greater in 2019, whereas ammonium oxidation (amoA) and assimilation (glnA) were lower. As these gene abundances varied in UL 2019 compared to UL 2018, we estimated their individual abundances based on water mass differentiation and found that FW influence (at Sts. 1–2) in 2019 increased nitrogen fixation and nitrification (i.e., hydroxylamine oxidation and nitrite oxidation) potentials and decreased ammonia oxidation and assimilation potential (Figure S5). This suggests that the metabolic potential of the nitrogen cycle in the fall of 2019 was significantly modified by FW input compared to 2018. Our results are similar to those of a recent proteome investigation by Ilgrande et al. (2018) on nitrifying communities and their metabolic potential for S variation. They found that S changes could reduce ammonium and nitrite oxidation potential to 42%, and other metabolic processes related to the nitrogen cycle were positively influenced. Liu et al. (2022) showed that S changes in response to different nutrient-cycling genes and found that decreasing S can modify nitrogen metabolic genes in aquatic environments.
Figure 7 The relative abundance of genes/enzymes involved in the nitrification process. The bar chart represents samples from 2018, and the pie chart represents samples from 2019. The description of the abundance and function of each gene represents shown here are given in Supplementary Table 3.
Sulfur oxidation and reduction were identified (Figure S6), with oxidation genes (Sox A, B,X,Y,Z) being the most abundant in the UL in both years. Compared to 2018, the 2019 samples had slightly higher UL abundances and lower BL abundances. However, unlike nitrogen metabolism, the Sts. 1–2 (i.e., the region of FW influence) did not show the individual genes involved in sulfur metabolism; however, the Sts. 3–4 showing high T by TSW increased in abundance (Figure S7). This variation demonstrates the effect of T on the sulfur oxidation potential. For example, the UL T values in 2019 were higher than those in 2018. Similar to sulfur oxidation, T fluctuations influenced the sulfur reduction genes between 2018 and 2019. Interestingly, the dsrA,B genes were abundant in both years BL, specifically in 2019 BL, showing that these functional genes were dominant in the KSBCW and were susceptible to warmer environments driven by TWW.
These findings are in line with those from the Okhotsk Sea (near our study area) (Li et al., 2018) and the South Atlantic Subtropical gyre (Murillo et al., 2014). An incubation experiment in the Arctic seawater (0°C, 10°C, and 20°C) revealed unique T responses for sulfate oxidation and reduction potential, implying differing characterization of sulfur-oriented bacterial populations (Robador et al., 2009). This shows that the respiration rates of sulfur-oriented bacteria increased significantly at lower T, which might explain the high abundance of dsrA,B genes found in this study. Comparative investigations between psychrophilic and mesophilic bacteria have revealed that under cold T, bacteria have a high enzymatic level for a long time to adapt (Feller and Gerday, 2003). Other studies have demonstrated that electron transfer coupling is less effective at lower T (Rabus et al., 2002), which is consistent with the current findings, with most oxidation and reduction gene abundance. Alphaproteobacteria play an important role in sulfur metabolism and have abundant sulfur oxidation and reduction genes (Li et al., 2018). Alphaproteobacteria were more abundant in the UL samples in this investigation (Supplemental Table 2), which might explain the increased abundance of sulfur reduction and oxidation. Our findings agree with a previous study that discovered sulfur-oxidizing Alphaproteobacteria with chemolithotroph lifestyles in surface water at higher T (Meyer and Kuever, 2007). Acclimation to low T does not fully compensate for the thermodynamic restrictions imposed on complex biochemical processes, such as respiratory energy generation.
This study found both heat and cold shock genes/proteins in response to T fluctuations driven by the difference in the composition of water masses (Figure 8). In this study, heat shock proteins were more abundant in the UL in both 2018 and 2019 compared to the other layers. However, compared to 2018, samples from 2019 showed higher abundance, with T ranges in the UL of 2019 being higher than those in 2018. Similar to other results, we estimated the FW influence of these heat shock protein abundances in the fall of 2019 and found no evidence that S variation may alter these gene abundances (Figure S8). Increasing T in the bacterial environment has been reported to harm protein synthesis in cellular processes (Maleki et al., 2016). In bacteria, heat shock proteins increase protein synthesis mechanisms (De Maio, 1999) to adjust to T changes (Maleki et al., 2016). In particular, when bacteria are exposed to higher T, the DnaK gene, which plays a role in repairing thermally damaged proteins for cell survival, increases (Yusof et al., 2022). Our DnaK gene was found to be highly prevalent in the UL in both years of this study. More specifically, as compared with the 2018 UL, this gene was shown to be higher (14% higher) in 2019. Similarly, other heat shock genes (clpS and HspQ) found in this study were shown to be greater (11%-115%) in 2019 compared to 2018. This implies that in comparison to 2018, prokaryotes created more heat shock proteins (in abundance) in 2019 to repair their damaged proteins to survive. Our results also demonstrate that when the T in the study area is high, these heat shock proteins increase in prokaryotes, which could be considered biomarkers of this region to increase surface ocean T. Cold shock genes are generated in cold environments in response to T changes to preserve bacteria’s optimal growth conditions (Keto-Timonen et al., 2016). Bacterial enzymatic activity is reduced under these conditions, which affects transcription and translation mechanisms (Phadtare and Inouye, 2004). Cold shock genes assist cells in overcoming these alterations by altering their nucleic acid components (Phadtare and Severinov, 2010). After cold shock genes are created, enzymatic activity in bacteria accelerates, allowing cells to develop at a lower T and even at a slower phase (Ermolenko & Makhatadze, 2002). We found that the high abundance of the cspA, cpA, and desR cold genes in the BL was evident in fall 2019 compared to fall 2018, owing to the intrusion of KSBCW (<10°C), which could be considered as biomarkers of this specific water mass in this region.
Figure 8 The relative abundance of cold and heat shock genes/proteins in bacterial metabolism. The bar chart represents samples from 2018, and the pie chart represents samples from 2019. UL (upper layer), ML (middle layer), and BL (bottom layer). The description of the abundance and function of each gene represents showed here are given in Supplementary Table 3.
The Changjiang River is known as a hotspot with a yearly T increase of approximately 3.5°C and an anticipated 30% increase in annual precipitation (Gu et al., 2015) due to climate change, which is expected to boost FW flow to ECS via CDW (Liu et al., 2021). Previous investigations found that approximately 70% of the ECS FW is transferred annually through KS (Senjyu et al., 2006; Moon et al., 2019; Yoon et al., 2022) which might change the environmental conditions of KS in the future. According to a recent study, the Kuroshio current extension is sensitive to global climate change and has the potential to warm significantly in the future (Lam et al., 2021), in addition to increasing warming TWW into the East Sea via the KS. Collectively, we anticipate large physical and biogeochemical changes in future KS based on significant increases in FW input from the Changjiang River and surface warming from the TWW. The results of this study, describing FW influence and T variation in prokaryotic composition, and their functional capabilities from genome abundances to biomarkers potential to different water masses from UL to BL, could be used as a reference for future investigations.
In this study, we compared the prokaryotic communities from different layers of the KS between the fall of 2018 and 2019 to investigate how different water masses influenced their composition and metabolic potential. Our results showed that the S and T of the water masses played an important role in regulating the prokaryotic population and metabolic modifications, especially in the UL and BL in 2019, which differed from those in 2018 due to the difference in the composition of water masses. We observed that the FW input from the ECS into the 2019 UL had a significant impact on altering the prokaryotic population from high density in 2018 to high diversity in 2019 than the relatively stable variable of T in 2018. In the 2019 BL, the lower T driven by the KSBCW brought several new communities from its origin to the KS, which was different from the BL in 2018. We also investigated the functional genes and found that they were influenced by different water mass conditions, including a preference for lower S and lower/higher T. Specifically, compared to 2018, the UL in 2019 promoted phototroph carbon fixation, whereas the BL in 2019 promoted chemoautotroph carbon fixation driven by KSBCW (T<10°C). We identified several biomarker genes based on the T variation in different water mass compositions from the UL to the BL in the study region. This study is the first attempt to demonstrate the effect of different water masses on the prokaryotic composition and metabolic/functional potential in the KS. Our findings provide a baseline understanding of the biogeochemical pattern in this understudied region and highlight the need for more comprehensive studies that consider not only the distinct water masses from the UL to the BL but also the effects of climate change, such as warming and acidification, to predict the biogeochemical pattern in the future.
The datasets presented in this study can be found in online repositories. The names of the repository/repositories and accession number(s) can be found in the article/Supplementary Material.
ST and I-NK developed the concept and design of the article. H-RK and I-NK conducted sampling. I-NK, ST and H-RK conducted measurements and analysis. ST, and I-NK wrote the manuscript. S-YK, H-KJ, and J-HK discussed the results and commented on the article.
This research was supported by the National Institute of Fisheries Science, Ministry of Oceans and Fisheries, Korea (R2023008) and the National Research Foundation of Korea (NRF) funded by the Korean government (MSIT) (NRF-2022R1A2C1008475). This study was also supported by a grant from the Korea Institute of Ocean Science and Technology (KIOST) (PEA008A) and Korea Institute of Marine Science and Technology Promotion (KIMST) funded by the Ministry of Oceans and Fisheries (MOF), Korea, titled “Development of Risk Managing Technology Tackling Ocean and Fisheries Crisis around Korean Peninsula by Kuroshio Current” (RS-2023-00256330).
We would like to thank the captain and crew members of the R/V Nara for their endless support during the fall 2018 and 2019 cruises. We express our gratitude to Dr. Dae-Hyun Kim for his valuable assistance with the processing of CTD data.
The authors declare no conflicts of interest and the research was conducted in the absence of any commercial or financial relationships that could be construed as a potential conflict of interest.
All claims expressed in this article are solely those of the authors and do not necessarily represent those of their affiliated organizations, or those of the publisher, the editors and the reviewers. Any product that may be evaluated in this article, or claim that may be made by its manufacturer, is not guaranteed or endorsed by the publisher.
The Supplementary Material for this article can be found online at: https://www.frontiersin.org/articles/10.3389/fmars.2023.1215251/full#supplementary-material
KS, Korea Strait; TWW, Tsushima Warm Water; TSW, Tsushima Surface Water; TMW, Tsushima Mid Water; KSBCW, Korea Strait Bottom Cold Water; UL, Upper Layer; ML, Middle Layer; BL, Bottom Layer; T, Temperature; S, Salinity; FW, Fresh Water; CDW, Changjiang Diluted Water; ECS, East China Sea.
Aßhauer K. P., Wemheuer B., Daniel R., Meinicke P. J. B. (2015). Tax4Fun: predicting functional profiles from metagenomic 16S rRNA data. Bioinformatics 31, 2882–2884. doi: 10.1093/bioinformatics/btv287
Agardi T., Alder J., Dayton P., Curran S., Kitchingman A., Wilson M., et al. Millennium ecosystem assessment: ecosystems and human well-being (2005) (Washington, DC: Current State and Trends Island Press).
Agogué H., Brink M., Dinasquet J., Herndl G. J. (2008). Major gradients in putatively nitrifying and non-nitrifying archaea in the deep north Atlantic. Nature 456, 788–791. doi: 10.1038/nature07535
Aldunate M., de la Iglesia R., Bertagnolli A. D., Ulloa O. (2018). Oxygen modulates bacterial community composition in the coastal upwelling waters off central Chile. Deep Sea Res. Part II: Topical Stud. Oceanography 156, 68–79. doi: 10.1016/j.dsr2.2018.02.001
Arístegui J., Gasol J. M., Duarte C. M., Herndld G. (2009). Microbial oceanography of the dark ocean's pelagic realm. Limnology Oceanography 54, 1501–1529. doi: 10.4319/lo.2009.54.5.1501
Asanuma I., Zhang X.-G., Zhao C., Huang B., Hasegawa D. (2014). Nutrients distribution in the coastal water of East Asia relative to the kuroshio. Landscape Ecol. Eng. 10, 191–199. doi: 10.1007/s11355-013-0242-7
Bachmann J., Heimbach T., Hassenrück C., Kopprio G. A., Iversen M. H., Grossart H. P., et al. (2018). Environmental drivers of free-living vs. particle-attached bacterial community composition in the Mauritania upwelling system. Front. Microbiol. 9, 2836. doi: 10.3389/fmicb.2018.02836
Bar-Even A., Noor E., Milo R. (2012). A survey of carbon fixation pathways through a quantitative lens. J. Exp. Bot. 63, 2325–2342. doi: 10.1093/jxb/err417
Beardsley R., Limeburner R., Yu H., Cannon G. (1985). Discharge of the changjiang (Yangtze river) into the East China sea. Continental Shelf Res. 4, 57–76. doi: 10.1016/0278-4343(85)90022-6
Bokulich N. A., Subramanian S., Faith J. J., Gevers D., Gordon J. I., Knight R., et al. (2013). Quality-filtering vastly improves diversity estimates from illumina amplicon sequencing. Nat. Methods 10, 57–59. doi: 10.1038/nmeth.2276
Boyle N. R., Morgan J. A. (2011). Computation of metabolic fluxes and efficiencies for biological carbon dioxide fixation. Metab. Eng. 13, 150–158. doi: 10.1016/j.ymben.2011.01.005
Broman E., Izabel-Shen D., Rodríguez-Gijón A., Bonaglia S., Garcia S. L., Nascimento F. J. (2022). Microbial functional genes are driven by gradients in sediment stoichiometry, oxygen, and salinity across the Baltic benthic ecosystem. Microbiome 10 (1), 1–17. doi: 10.1186/s40168-022-01321-z
Bryant J. A., Clemente T. M., Viviani D. A., Fong A. A., Thomas K. A., Kemp P., et al. (2016). Diversity and activity of communities inhabiting plastic debris in the north pacific gyre. MSystems 1, e00024–e00016. doi: 10.1128/mSystems.00024-16
Caporaso J. G., Kuczynski J., Stombaugh J., Bittinger K., Bushman F. D., Costello E. K., et al. (2010). QIIME allows analysis of high-throughput community sequencing data. Nat. Methods 7, 335–336. doi: 10.1038/nmeth.f.303
Celussi M., Bergamasco A., Cataletto B., Umani S. F., Del Negro P. (2010). Water masses’ bacterial community structure and microbial activities in the Ross Sea, Antarctica. Antarctic Sci. 22, 361–370. doi: 10.1017/S0954102010000192
Chakravorty S., Helb D., Burday M., Connell N., Alland D. (2007). A detailed analysis of 16S ribosomal RNA gene segments for the diagnosis of pathogenic bacteria. J. microbiological Methods 69, 330–339. doi: 10.1016/j.mimet.2007.02.005
Chang P. H., Isobe A. (2003). A numerical study on the changjiang diluted water in the yellow and East China seas. J. Geophysical Research: Oceans 108, (C9). doi: 10.1029/2002JC001749
Chow C.-E. T., Sachdeva R., Cram J. A., Steele J. A., Needham D. M., Patel A., et al. (2013). Temporal variability and coherence of euphotic zone bacterial communities over a decade in the southern California bight. ISME J. 7, 2259–2273. doi: 10.1038/ismej.2013.122
Chung I.-K., Kang Y.-H. (1995). The ultrastructure of the chlorococcalean picoplankton isolated from the western channel of the Korea strait. J. Korean Soceity Oceanography 30, 529–536.
Dai T., Zhang Y., Ning D., Su Z., Tang Y., Huang B., et al. (2018). Dynamics of sediment microbial functional capacity and community interaction networks in an urbanized coastal estuary. Front. Microbiol. 9, 2731. doi: 10.3389/fmicb.2018.02731
Danovaro R., Corinaldesi C., Dell’Anno A., Snelgrove P. V. (2017). The deep-sea under global change Vol. 27 (Curr. Biol), R461–R465. doi: 10.1016/j.cub.2017.02.046
Del Giorgio P. A., Williams P. L. B. (2005). The global significance of respiration in aquatic ecosystems: from single cells to the biosphere. Respiration Aquat. Ecosyst. 1. doi: 10.1093/acprof:oso/9780198527084.003.0014
De Maio A. J. S. (1999). Heat shock proteins: facts, thoughts, and dreams. Shock 11, 1–12. doi: 10.1097/00024382-199901000-00001
Demirci H., Tolar B. B., Doukov T., Petriceks A., Pal A., Yoshikuni Y., et al. (2020). Structural adaptation of oxygen tolerance in 4-hydroxybutyrl-CoA dehydratase, a key enzyme of archaeal carbon fixation. bioRxiv, 2020–02. doi: 10.1101/2020.02.05.935528
Ducklow H. (2000). Bacterial production and biomass in the oceans. Microbial Ecol. oceans 1, 85–120.
Ermolenko D., Makhatadze G. (2002). Bacterial cold-shock proteins. Cell. Mol. Life Sci. CMLS 59, 1902–1913. doi: 10.1007/PL00012513
Feller G., Gerday C. (2003). Psychrophilic enzymes: hot topics in cold adaptation. Nat. Rev. Microbiol. 1, 200–208. doi: 10.1038/nrmicro773
Flombaum P., Gallegos J. L., Gordillo R. A., Rincón J., Zabala L. L., Jiao N., et al. (2013). Present and future global distributions of the marine cyanobacteria prochlorococcus and synechococcus. Proc. Natl. Acad. Sci. 110, 9824–9829. doi: 10.1073/pnas.1307701110
Franks F., Mathias S., Hatley R. (1990). Water, temperature and life. Philos. Trans. R. Soc. London. B Biol. Sci. 326 (1237), 517–533. doi: 10.1098/rstb.1990.0029
Fu Y., Rivkin R. B., Lang A. S. (2019). Effects of vertical water mass segregation on bacterial community structure in the Beaufort Sea. Microorganisms 7, 385. doi: 10.3390/microorganisms7100385
Fuhrman J. A., Sleeter T. D., Carlson C. A., Proctor L. M. (1989). Dominance of bacterial biomass in the Sargasso Sea and its ecological implications. Mar. Ecol. Prog. Ser., 207–217. doi: 10.3354/meps057207
Galand P. E., Casamayor E. O., Kirchman D. L., Lovejoy C. (2009). Ecology of the rare microbial biosphere of the Arctic ocean. Proc. Nat. Acad. Sci. 106 (52), 22427–22432. doi: 10.1073/pnas.0908284106
Galand P. E., Potvin M., Casamayor E. O., Lovejoy C. (2010). Hydrography shapes bacterial biogeography of the deep Arctic ocean. ISME J. 4, 564–576. doi: 10.1038/ismej.2009.134
Gomes H. D. R., Xu Q., Ishizaka J., Carpenter E. J., Yager P. L., Goes J. I. (2018). The influence of riverine nutrients in niche partitioning of phytoplankton communities–a contrast between the Amazon river plume and the ChangJiang (Yangtze) river diluted water of the East China Sea. Front. Mar. Sci. 5, 343. doi: 10.3389/fmars.2018.00343
Gu H., Yu Z., Wang G., Wang J., Ju Q., Yang C., et al. (2015). Impact of climate change on hydrological extremes in the Yangtze river basin, China. Stochastic Environ. Res. Risk Assess. 29, 693–707. doi: 10.1007/s00477-014-0957-5
Han Y., Zhang M., Chen X., Zhai W., Tan E., Tang K., and (2022). Transcriptomic evidences for microbial carbon and nitrogen cycles in the deoxygenated seawaters of bohai Sea. Environ. Int. 158, 106889. doi: 10.1016/j.envint.2021.106889
Hydes D., Aoyama M., Aminot A., Bakker K., Becker S., Coverly S., et al. (2010). Recommendations for the determination of nutrients in seawater to high levels of precision and inter-comparability using continuous flow analysers (GO-SHIP (Unesco/IOC).
Ichinomiya M., Yamada K., Nakagawa Y., Nishino Y., Kasai H., Kuwata A. (2019). Parmales abundance and species composition in the waters surrounding Hokkaido, north Japan. Polar Sci. 19, 130–136. doi: 10.1016/j.polar.2018.08.001
Ilgrande C., Leroy B., Wattiez R., Vlaeminck S. E., Boon N., Clauwaert P. (2018). Metabolic and proteomic responses to salinity in synthetic nitrifying communities of nitrosomonas spp. and nitrobacter spp. Front. Microbiol. 9, 2914. doi: 10.3389/fmicb.2018.02914
Jaenicke R., Sterner R. (2006). Life at high temperatures. prokaryotes, 167–209. doi: 10.1007/0-387-30742-7_7
Jeong W. G., Cho S. M. (2018). Estimation of primary production of the waters around rack oyster farm at wando, Korea. Fisheries Aquat. Sci. 21 (1), 1–7. doi: 10.1186/s41240-018-0086-z
Jiao N., Zhang Y., Zeng Y., Gardner W. D., Mishonov A. V., Richardson M. J., et al. (2007). Ecological anomalies in the East China Sea: impacts of the three gorges dam? Water Res. 41, 1287–1293. doi: 10.1016/j.watres.2006.11.053
Kang H., Kang D.-S. (2002). Contribution of marine microbes to particulate organic matter in the Korea strait. J. korean Soc. oceanography 37, 35–44.
Kent A. G., Dupont C. L., Yooseph S., Martiny A. C. (2016). Global biogeography of prochlorococcus genome diversity in the surface ocean. ISME J. 10, 1856–1865. doi: 10.1038/ismej.2015.265
Keto-Timonen R., Hietala N., Palonen E., Hakakorpi A., Lindström M., Korkeala H. (2016). Cold shock proteins: a minireview with special emphasis on csp-family of enteropathogenic yersinia. Front. Microbiol. 7, 1151. doi: 10.3389/fmicb.2016.01151
Kim I.-N., Lee T.-S. (2004). Physicochemical properties and the origin of summer bottom cold waters in the Korea strait. Ocean Polar Res. 26, 595–606. doi: 10.4217/OPR.2004.26.4.595
Kim H.-R., Lim J.-H., Kim J.-H., Thangaraj S., Kim I.-N. (2022). Physical process controlling the surface bacterial community composition in the ulleung basin of East Sea. Front. Mar. Sci. 9, 841492. doi: 10.3389/fmars.2022.841492
Kim Y. H., Kim Y. B., Kim K., Chang K. I., Lyu S. J., Cho Y. K., et al. (2006). Seasonal variation of the Korea strait bottom cold water and its relation to the bottom current. Geophys. Res. Lett. 33 (24). doi: 10.1029/2006GL027625
Klindworth A., Pruesse E., Schweer T., Peplies J., Quast C., Horn M., et al. (2013). Evaluation of general 16S ribosomal RNA gene PCR primers for classical and next-generation sequencing-based diversity studies. Nucleic Acids Res. 41, e1–e1. doi: 10.1093/nar/gks808
Knoblauch C., Jørgensen B. B. (1999). Effect of temperature on sulphate reduction, growth rate and growth yield in five psychrophilic sulphate-reducing bacteria from Arctic sediments. Environ. Microbiol. 1, 457–467. doi: 10.1046/j.1462-2920.1999.00061.x
Könneke M., Schubert D. M., Brown P. C., Hügler M., Standfest S., Schwander T., et al. (2014). Ammonia-oxidizing archaea use the most energy-efficient aerobic pathway for CO2 fixation. Proc. Natl. Acad. Sci. 111, 8239–8244. doi: 10.1073/pnas.1402028111
Lam A. R., Macleod K. G., Schilling S. H., Leckie R. M., Fraass A. J., Patterson M. O., et al. (2021). Pliocene to earliest pleistocene (5–2.5 ma) reconstruction of the kuroshio current extension reveals a dynamic current. Paleoceanography Paleoclimatology 36, e2021PA004318. doi: 10.1029/2021PA004318
Laque T., Farjalla V. F., Rosado A. S., Esteves F. A. (2010). Spatiotemporal variation of bacterial community composition and possible controlling factors in tropical shallow lagoons. Microbial Ecol. 59, 819–829. doi: 10.1007/s00248-010-9642-5
Lee J.-K., Choi K.-H. (2021). Bacterial communities from the water column and the surface sediments along a transect in the East Sea. J. Mar. Life Sci. 6, 9–22.
Lee Y.-W., Park H.-J., Choy E.-J., Kim Y.-S., Kang C.-K. (2010). Temporal variation of phytoplankton community related to water column structure in the Korea strait. Ocean Polar Res. 32, 321–329. doi: 10.4217/OPR.2010.32.3.321
Levitus S., Boyer T. (1994). World ocean atlas 1994 volume 4: temperature Vol. 4 (Washington: DC, US Department of Commerce, NOAA Atlas NESDIS).
Li Y., Jing H., Xia X., Cheung S., Suzuki K., Liu H. (2018). Metagenomic insights into the microbial community and nutrient cycling in the western subarctic pacific ocean. Front. Microbiol. 9, 623. doi: 10.3389/fmicb.2018.00623
Li Y.-Y., Chen X.-H., Xue C., Zhang H., Sun G., Xie Z.-X., et al. (2019). Proteomic response to rising temperature in the marine cyanobacterium synechococcus grown in different nitrogen sources. Front. Microbiol. 10, 1976 doi: 10.3389/fmicb.2019.01976
Liu Z., Gan J., Wu H., Hu J., Cai Z., Deng Y. (2021). Advances on coastal and estuarine circulations around the changjiang estuary in the recent decades 2000–2020). Front. Mar. Sci. 8, 615929. doi: 10.3389/fmars.2021.615929
Liu Q., Yang J., Wang B., Liu W., Hua Z., Jiang H. (2022). Influence of salinity on the diversity and composition of carbohydrate metabolism, nitrogen and sulfur cycling genes in lake surface sediments. Front. Microbiol. 13, 1019010. doi: 10.3389/fmicb.2022.1019010
Liu S. M., Zhang J., Chen H., Wu Y., Xiong H., Zhang Z. (2003). Nutrients in the changjiang and its tributaries. Biogeochemistry 62, 1–18. doi: 10.1023/A:1021162214304
Matsuoka A., Bricaud A., Benner R., Para J., Sempéré R., Prieur L., et al. (2012). Tracing the transport of colored dissolved organic matter in water masses of the southern Beaufort Sea: relationship with hydrographic characteristics. Biogeosciences 9 (3), 925–940. doi: 10.5194/bg-9-925-2012
Maleki F., Khosravi A., Nasser A., Taghinejad H., Azizian M. (2016). Bacterial heat shock protein activity. J. Clin. Diagn. research: JCDR 10, BE01. doi: 10.7860/JCDR/2016/14568.7444
Mason R. L., Gunst R. F., Hess J. L. (2003). Statistical design and analysis of experiments: with applications to engineering and science (John Wiley & Sons).
Meyer B., Kuever J. (2007). Molecular analysis of the diversity of sulfate-reducing and sulfur-oxidizing prokaryotes in the environment, using aprA as functional marker gene. Appl. Environ. Microbiol. 73, 7664–7679. doi: 10.1128/AEM.01272-07
Moon J. H., Hirose N., Yoon J. H., Pang I. C. (2009). Effect of the along-strait wind on the volume transport through the Tsushima/Korea strait in September. J. oceanography 65, 17–29. doi: 10.1007/s10872-009-0002-3
Moon J.-H., Kim T., Son Y. B., Hong J.-S., Lee J.-H., Chang P.-H., et al. (2019). Contribution of low-salinity water to sea surface warming of the East China Sea in the summer of 2016. Prog. oceanography 175, 68–80. doi: 10.1016/j.pocean.2019.03.012
Moon C. H., Yang H. S., Lee K. W. (1996). Regeneration processes of nutrients in the polar front area of the East Sea 1. relationships between water mass and nutrient distribution pattern in autumn. Korean J. Fisheries Aquat. Sci. 29 (4), 503–526.
Murillo A. A., Ramírez-Flandes S., Delong E. F., Ulloa O. (2014). Enhanced metabolic versatility of planktonic sulfur-oxidizing γ-proteobacteria in an oxygen-deficient coastal ecosystem. Front. Mar. Sci. 1, 18. doi: 10.3389/fmars.2014.00018
Na H., Isoda Y., Kim K., Kim Y. H., Lyu S. J. (2009). Recent observations in the straits of the East/Japan Sea: a review of hydrography, currents and volume transports. J. Mar. Syst. 78, 200–205. doi: 10.1016/j.jmarsys.2009.02.018
Onitsuka G., Yanagi T., Yoon J. H. (2007). A numerical study on nutrient sources in the surface layer of the Japan Sea using a coupled physical-ecosystem model. J. Geophysical Research: Oceans 112, (C5). doi: 10.1029/2006JC003981
Parks D. H., Tyson G. W., Hugenholtz P., Beiko R. G. (2014). STAMP: statistical analysis of taxonomic and functional profiles. Bioinformatics 30, 3123–3124. doi: 10.1093/bioinformatics/btu494
Phadtare S., Inouye M. (2004). Genome-wide transcriptional analysis of the cold shock response in wild-type and cold-sensitive, quadruple-csp-deletion strains of escherichia coli. J. bacteriology 186, 7007–7014. doi: 10.1128/JB.186.20.7007-7014.2004
Phadtare S., Severinov K. (2010). RNA Remodeling and gene regulation by cold shock proteins. RNA Biol. 7, 788–795. doi: 10.4161/rna.7.6.13482
Price M. N., Dehal P. S., Arkin A. P. (2009). FastTree: computing large minimum evolution trees with profiles instead of a distance matrix. Mol. Biol. Evol. 26, 1641–1650. doi: 10.1093/molbev/msp077
Rabus R., Brüchert V., Amann J., Könneke M. (2002). Physiological response to temperature changes of the marine, sulfate-reducing bacterium desulfobacterium autotrophicum. FEMS Microbiol. Ecol. 42, 409–417. doi: 10.1111/j.1574-6941.2002.tb01030.x
Raes E. J., Bodrossy L., Van De Kamp J., Bissett A., Ostrowski M., Brown M. V., et al. (2018). Oceanographic boundaries constrain microbial diversity gradients in the south pacific ocean. Proc. Natl. Acad. Sci. 115, E8266–E8275. doi: 10.1073/pnas.1719335115
Randall-Goodwin E., Meredith M., Jenkins A., Sherrell R., Abrahamsen E. (2014). Water mass structure and freshwater distributions in the amundsen Sea polynya, Antarctica. Elem Sci. Anth. doi: 10.12952/journal.elementa.000065
Robador A., Brüchert V., Jørgensen B. B. (2009). The impact of temperature change on the activity and community composition of sulfate-reducing bacteria in arctic versus temperate marine sediments. Environ. Microbiol. 11, 1692–1703. doi: 10.1111/j.1462-2920.2009.01896.x
Seidel L., Broman E., Turner S., Ståhle M., Dopson M. (2021). Interplay between eutrophication and climate warming on bacterial communities in coastal sediments differs depending on water depth and oxygen history. Sci. Rep. 11 (1), 23384. doi: 10.1038/s41598-021-02725-x
Senjyu T., Enomoto H., Matsuno T., Matsui S. (2006). Interannual salinity variations in the tsushima strait and its relation to the changjiang discharge. J. Oceanography 62, 681–692. doi: 10.1007/s10872-006-0086-y
Shade A., Jones S. E., Mcmahon K. D. (2008). The influence of habitat heterogeneity on freshwater bacterial community composition and dynamics. Environ. Microbiol. 10, 1057–1067. doi: 10.1111/j.1462-2920.2007.01527.x
Shim M. J., Yoon Y. Y. (2021). Long-term variation of nitrate in the East Sea, Korea. Environ. Monit. Assess. 193, 1–13. doi: 10.1007/s10661-021-09425-z
Shon D.-H., Shin K.-S., Jang P.-G., Kim Y.-O., Chang M., Kim W.-S. (2008). Effect of thermal stratification and mixing on phytoplankton community structure in the western channel of the Korea strait. Ocean Polar Res. 30, 261–275. doi: 10.4217/OPR.2008.30.3.261
Son Y. B., Choi J. (2022). Mapping the changjiang diluted water in the East China Sea during summer over the a 10-year period summer season using GOCI satellite sensor data. Front. Mar. Sci. 9, 1024306.
Spencer-Cervato C., Thierstein H. R. (1997). First appearance of globorotalia truncatulinoides: cladogenesis and immigration. Mar. Micropaleontology 30, 267–291. doi: 10.1016/S0377-8398(97)00004-2
Stocker R. (2012). Marine microbes see a sea of gradients. Science 338, 628–633. doi: 10.1126/science.1208929
Teague W. J., Ko D. S., Jacobs G. A., Perkins H. T., Book J. W., Smith S. R., et al. (2006). Currents through the Korea/Tsushima strait: a review of LINKS observations. Oceanography 19 (3), 50. doi: 10.5670/oceanog.2006.43
Thangaraj S., Sun J. (2021). Transcriptomic reprogramming of the oceanic diatom skeletonema dohrnii under warming ocean and acidification. Environ. Microbiol. 23, 980–995. doi: 10.1111/1462-2920.15248
Thompson L. R., Williams G. J., Haroon M. F., Shibl A., Larsen P., Shorenstein J., et al. (2017). Metagenomic covariation along densely sampled environmental gradients in the red Sea. ISME J. 11, 138–151. doi: 10.1038/ismej.2016.99
Tian R., Hu F., Martin J. (1993). Summer nutrient fronts in the changjiang (Yantze river) estuary. Estuarine Coast. Shelf Sci. 37, 27–41. doi: 10.1006/ecss.1993.1039
Tseng C.-H., Chiang P.-W., Lai H.-C., Shiah F.-K., Hsu T.-C., Chen Y.-L., et al. (2015). Prokaryotic assemblages and metagenomes in pelagic zones of the south China Sea. BMC Genomics 16, 1–16. doi: 10.1186/s12864-015-1434-3
Varela M. M., Van Aken H. M., Sintes E., Herndl G. J. (2008). Latitudinal trends of crenarchaeota and bacteria in the meso-and bathypelagic water masses of the Eastern north Atlantic. Environ. Microbiol. 10, 110–124. doi: 10.1111/j.1462-2920.2007.01437.x
Venkatachalam S., Ansorge I. J., Mendes A., Melato L. I., Matcher G. F., Dorrington R. A. (2017). A pivotal role for ocean eddies in the distribution of microbial communities across the Antarctic circumpolar current. PloS One 12, e0183400. doi: 10.1371/journal.pone.0183400
Walsh E. A., Kirkpatrick J. B., Rutherford S. D., Smith D. C., Sogin M., D'hondt S. (2016). Bacterial diversity and community composition from seasurface to subseafloor. ISME J. 10, 979–989. doi: 10.1038/ismej.2015.175
Wang Y., Hu X., Sun Y., Wang C. (2021a). Influence of the cold bottom water on taxonomic and functional composition and complexity of microbial communities in the southern yellow Sea during the summer. Sci. Total Environ. 759, 143496. doi: 10.1016/j.scitotenv.2020.143496
Wang Y., Liao S., Gai Y., Liu G., Jin T., Liu H., et al. (2021b). Metagenomic analysis reveals microbial community structure and metabolic potential for nitrogen acquisition in the oligotrophic surface water of the Indian ocean. Front. Microbiol. 12, 518865. doi: 10.3389/fmicb.2021.518865
Wemheuer F., Taylor J. A., Daniel R., Johnston E., Meinicke P., Thomas T., et al. (2020). Tax4Fun2: prediction of habitat-specific functional profiles and functional redundancy based on 16S rRNA gene sequences. Environ. Microbiol. 15 (1), 1–12. doi: 10.1186/s40793-020-00358-7
Yang J.-S., Choi H.-Y., Jeong H.-J., Jeong J.-Y., Park J.-K. (2000). The outbreak of red tides in the coastal waters off kohung, chonnam, Korea: 1. physical and chemical characteristics in 1997. J. Of Korean Soc. Of Oceanography 5, 16–26.
Yang C., Yang S., Vigier N. (2023). Li Isotopic variations of particulate non-silicate phases during estuarine water mixing. Geochimica Cosmochimica Acta 354, 229–239. doi: 10.1016/j.gca.2023.06.020
Yoon J. N., Lee M., Jin H., Lim Y. K., Ro H., Park Y. G., et al. (2022). Summer distributional characteristics of surface phytoplankton related with multiple environmental variables in the Korean coastal waters. J. Mar. Sci. Eng. 10 (7), 850. doi: 10.3390/jmse10070850
Yusof N. A., Masnoddin M., Charles J., Thien Y. Q., Nasib F. N., Wong C. M. V. L., et al. (2022). Can heat shock protein 70 (HSP70) serve as biomarkers in Antarctica for future ocean acidification, warming and salinity stress? Polar Biol. 45 (3), 371–394. doi: 10.1007/s00300-022-03006-7
Zäncker B., Cunliffe M., Engel A. (2018). Bacterial community composition in the sea surface microlayer off the Peruvian coast. Front. Microbiol. 9, 2699. doi: 10.3389/fmicb.2018.02699
Zarzycki J., Brecht V., Müller M., Fuchs G. (2009). Identifying the missing steps of the autotrophic 3-hydroxypropionate CO2 fixation cycle in chloroflexus aurantiacus. Proc. Natl. Acad. Sci. 106, 21317–21322. doi: 10.1073/pnas.0908356106
Zhu X.-G., Long S. P., Ort D. R. (2010). Improving photosynthetic efficiency for greater yield. Annu. Rev. Plant Biol. 61, 235–261. doi: 10.1146/annurev-arplant-042809-112206
Zinser E. R., Johnson Z. I., Coe A., Karaca E., Veneziano D., Chisholm S. W. J. (2007). Influence of light and temperature on prochlorococcus ecotype distributions in the Atlantic ocean. Limnology Oceanography 52, 2205–2220. doi: 10.4319/lo.2007.52.5.2205
Zorz J., Willis C., Comeau A. M., Langille M. G., Johnson C. L., Li W. K., et al. (2019). Drivers of regional bacterial community structure and diversity in the Northwest Atlantic ocean. Front. Microbiol. 10, 281. doi: 10.3389/fmicb.2019.00281
Zubkov M. V., Fuchs B. M., Tarran G. A., Burkill P. H., Amann R. J. A., Microbiology E. (2003). High rate of uptake of organic nitrogen compounds by prochlorococcus cyanobacteria as a key to their dominance in oligotrophic oceanic waters. Appl. Environ. Microbiol. 69, 1299–1304. doi: 10.1128/AEM.69.2.1299-1304.2003
Keywords: prokaryotes, bacteria, water mass, carbon metabolism, stress genes, Korea Strait, Tsushima Warm Water, Korea Strait Bottom Cold Water
Citation: Thangaraj S, Kim H-R, Kim S-Y, Jung H-K, Kim J-H and Kim I-N (2023) Water mass structure determine the prokaryotic community and metabolic pattern in the Korea Strait during fall 2018 and 2019. Front. Mar. Sci. 10:1215251. doi: 10.3389/fmars.2023.1215251
Received: 01 May 2023; Accepted: 03 July 2023;
Published: 21 July 2023.
Edited by:
Dilip Kumar Jha, National Institute of Ocean Technology, IndiaReviewed by:
Gopalakrishnan Thilagam, Pachaiyappa’s College for Men, IndiaCopyright © 2023 Thangaraj, Kim, Kim, Jung, Kim and Kim. This is an open-access article distributed under the terms of the Creative Commons Attribution License (CC BY). The use, distribution or reproduction in other forums is permitted, provided the original author(s) and the copyright owner(s) are credited and that the original publication in this journal is cited, in accordance with accepted academic practice. No use, distribution or reproduction is permitted which does not comply with these terms.
*Correspondence: Il-Nam Kim, aWxuYW1raW1AaW51LmFjLmty
Disclaimer: All claims expressed in this article are solely those of the authors and do not necessarily represent those of their affiliated organizations, or those of the publisher, the editors and the reviewers. Any product that may be evaluated in this article or claim that may be made by its manufacturer is not guaranteed or endorsed by the publisher.
Research integrity at Frontiers
Learn more about the work of our research integrity team to safeguard the quality of each article we publish.