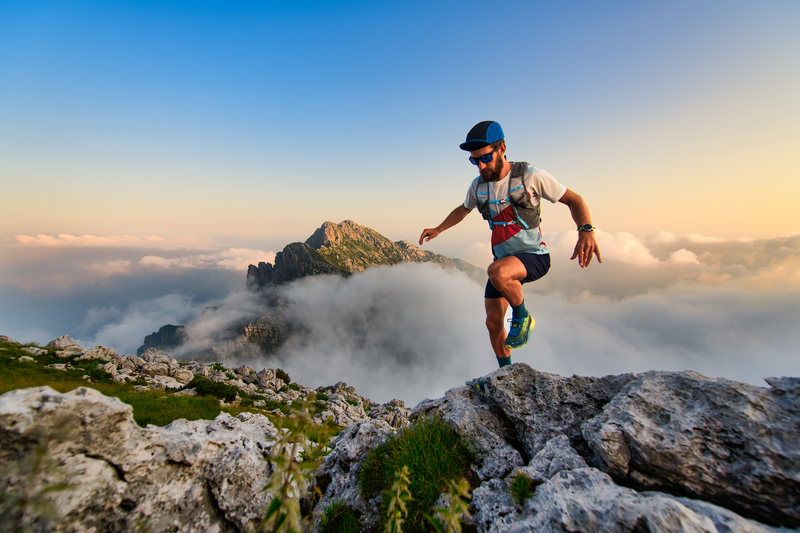
94% of researchers rate our articles as excellent or good
Learn more about the work of our research integrity team to safeguard the quality of each article we publish.
Find out more
ORIGINAL RESEARCH article
Front. Mar. Sci. , 09 August 2023
Sec. Marine Conservation and Sustainability
Volume 10 - 2023 | https://doi.org/10.3389/fmars.2023.1213424
Demand for critical minerals is projected to double or even triple by 2030 as the global energy and transportation sectors transition away from fossil fuels toward renewable energy. This anticipated demand raises interest in new critical mineral resources, including marine minerals, to supplement future supplies. While the environmental benefits of transitioning to green energy are many, there are inherent environmental costs. In this study, we compiled broad-scale datasets of prominent environmental features and human uses within four deep ocean regions of the U.S. Exclusive Economic Zone where marine minerals are predicted to occur, called prospective regions: they are the Blake Plateau, the Hawaiian Islands, Offshore California, and the Gulf of Alaska. We generated a normalized metric to score regions based on the co-occurrence of either environmental or human use variables within hexagonal grid cells, allowing us to assess the occurrence intensity and spatial patterns of environmental and/or human use variables within each region. Driven by the presence of threatened species and total species richness, the Blake Plateau and the Hawaiian Islands have the highest environmental occurrence scores, followed by Offshore California and the Gulf of Alaska. Offshore California is the busiest shipping region, followed by the Blake Plateau, the Gulf of Alaska and Hawaiian Islands, and subsea cables are densest in Offshore California, followed by Gulf of Alaska and Hawaiian Islands. The Gulf of Alaska has the largest reported fisheries landings. The sensitivities of biota and human uses to mining are highest for benthic or sessile species and infrastructure on or near ferromanganese crusts or manganese nodules that would be directly and physically altered through crust extraction or nodule removal. This study illustrates the usefulness of comprehensive, spatially explicit risk assessments to inform deep sea mining management and minimize ecological harm and human use conflicts. We assert that the relatively straightforward GIS-based methodology tested here can be applied and iterated upon elsewhere.
Greenhouse gas emissions (GHGs) resulting from the burning of fossil fuels are inarguably responsible for the Earth’s rapidly changing and warming climate (Pörtner et al., 2022). Consequently, governments have agreed to cut greenhouse gas emissions (GHGs) to stabilize climate change and its impacts, prompting a transition away from fossil fuels toward massive investments in low GHG solutions, most notably renewable energy technologies (renewables) (Gielen et al., 2019). The energy and transportation sectors require various minerals to develop renewables, and deep-sea mining (DSM) is in the exploratory stages of supplying some of these critical minerals (Katona et al., 2021; IEA, 2022).
Seafloor locations rich in critical minerals have been most intensively studied and considered for their resource potential in the “Area” (i.e., the seabed beyond national jurisdictions). The International Seabed Authority (ISA) oversees development of the legal and regulatory framework for DSM in the Area (United Nations Convention on the Law of the Sea, 1982). Exploration contracts have been active since 2001, and the ISA is currently developing exploitation regulations. DSM may move from the exploration stage to the exploitation stage in the Area within the next few years (Duncombe, 2022). In 2021, the Republic of Nauru expressed an initial intent to submit a plan of work for exploitation for polymetallic nodule fields in the Pacific Ocean in 2023, which accelerated the ISA’s timetable to adopt suitable exploitation regulations. Following these developments, calls increased for heightened environmental protection and for further information regarding the potential environmental and social impacts of DSM (Smith et al., 2020; Katona et al., 2021). The need for more environmental data has caused some countries to call for temporary pauses or moratoriums on DSM until more is known (Global Parliamentary Declaration Calling for a Moratorium on Deep Seabed Mining, 2022).
International development of critical minerals markets has already sparked the United States (U.S.) Government’s interest in accessing critical minerals domestically (Presidential Executive Order 13953 2020; Nassar et al., 2020). Although the U.S. Government has not named seabed prospecting as a likely domestic minerals source, marine minerals are known or predicted to occur in the territorial seabed of individual nations, including the United States (Cronan, 1980; Mizell et al., 2022). The United States is not a signatory of the United Nations Convention on the Law of the Sea (UNCLOS) and therefore cannot currently obtain exploration contracts for DSM in the Area through the ISA. If the U.S. were to seek critical minerals from seabed environments, it would be limited to DSM exploration in the U.S. exclusive economic zone (EEZ) or EEZs of countries with their own regulatory framework, such as the Cook Islands.
Emerging information shows overlaps in the U.S. EEZ among the locations of prospective marine mineral occurrence, benthic and abyssal ecosystems and environmental features, and human uses. The U.S. Geological Survey has identified regions of prospective occurrences for ferromanganese crusts and nodules in the U.S. EEZ (Mizell et al., 2022), including the East and West Coasts, the Subarctic and Arctic regions around Alaska, the Hawaiian Islands, and the Pacific Island Territories. Little data exists to ground-truth ferromanganese crust and nodule occurrence and associated resource potential. Publicly accessible databases or those available upon request record the locations of unique and threatened benthic features such as octocorals and seamounts, and areas of fishing, maritime shipping, and submarine cables (Table 1).
Table 1 Description of occurrence variables and summary metrics for both environmental features and human uses.
Proposed DSM mining activities are expected to cause seafloor disturbance, substrate removal, and suspension and transport of previously stable sediment and mineral material (Smith et al., 2020; Vonnahme et al., 2020). Studies of projected, modeled, and measured impacts of ferromanganese crust and nodule extraction on benthic organisms exist for the general habitats of abyssal plains and seamounts (Gollner et al., 2017; Jones et al., 2017; Simon-Lledó et al., 2019; Kaikkonen et al., 2021; Muñoz-Royo et al., 2021), as well as for specific regions in international waters, namely the Clarion Clipperton Zone (CCZ), the most well studied manganese nodule region with targeted DSM interest (Weaver et al., 2022). For example, experimental field studies and remote sensing analyses conducted in the abyssal basins of the Area in the Pacific Ocean demonstrate that nodule extraction has negative impacts on faunal biomass and density (Vanreusel et al., 2016; Vonnahme et al., 2020). The decades-long lack of documented recovery of benthic fauna in the CCZ following disturbances analogous to seabed mining raises important questions about the potential for broader ecosystem impacts of ore extraction on population connectivity, food webs and carbon cycling (Amon et al., 2022). However, spatially explicit analyses of potential conflicts between ecosystem services or human uses and DSM for most regions are largely absent from publicly available literature (van der Grient and Drazen, 2021), and this is certainly the case for the U.S. EEZ.
Strategic environmental assessments and mapping tools are among the approaches used to analyze and minimize potential impacts of DSM on ecosystem services, ranging from provisioning services (e.g., fish catch) to regulating services (e.g., climate regulation) to cultural services (e.g., educational) (Le et al., 2017). However, the mapping of ecosystem services in marine environments (Mangi et al., 2011), much less the deep sea, remains a challenge due the complexities of a highly dynamic environment where biotic and abiotic systems are difficult to define in space and time (Buonocore et al., 2021). Therefore, templates for examining the spatial distributions, interactions, and impact tradeoffs among services and human activities are more limiting and less straightforward in marine ecosystems than they are in a terrestrial context (Buonocore et al., 2021). Therefore, decision support tools relying on spatial analyses for evaluation and mitigation of possible ecosystem impacts are a priority before large -and -commercial scale DSM proceeds.
To advance evidence-based decision making about marine mineral prospecting within the U.S. EEZ, this paper presents a spatial analysis of areas of prospective mineral occurrences in the U.S. EEZ in relation to the presence of marine habitats, species, and human uses from publicly available databases. A core objective was building a normalized metric to score regions based on the co-occurrence of either environmental or human use variables so that occurrence intensity and patterns can be evaluated in the context of mining locations and impacts. Our analysis was limited to a relatively small pool of datasets that we could obtain for all regions, shedding light on critical knowledge gaps in the deep sea requiring urgent attention. This study also provides a methodology for rapid primary spatial conflict analysis for deep-sea activities using GIS that can be applied elsewhere or built on as relevant datasets become more detailed.
To follow is a description of how we constructed this GIS-based methodology, the results of its application in four regions within the U.S. EEZ, and a discussion of the implications of potential ecosystem and human use impacts in regions prospective for manganese nodules and ferromanganese crusts should mining ever occur there. We end with a summary of the study’s limitations and highlight research priorities needed to close key information gaps and strengthen the utility of this tool for understanding and minimizing potential conservation and human use impacts associated with seabed mining.
To gain a preliminary understanding of non-mineral marine resources that could be impacted by DSM, we analyzed environmental and human use variables that occur in prospective mineral occurrence regions (PMORs) for ferromanganese crusts and manganese nodules located in the U.S. EEZ (Mizell et al., 2022). We compiled broad-scale datasets of prominent environmental features (e.g., species distributions) and human uses (e.g., fisheries) within PMORs and then generated a normalized metric to score regions based on the co-occurrence of either environmental or human use variables. Results highlight the areas richest in resources and uses that are also potentially at risk from DSM impacts.
Empirical data confirming the presence of critical minerals in the U.S. EEZ marine environment remains sparse, and, to date, no extensive exploration for DSM has occurred in U.S. waters or the Area (USGS, 2022). However, the oceanographic and geographic criteria required for the formation of ferromanganese (crust and nodule) marine minerals indicate PMORs where marine minerals likely occur (Hein et al., 2009; Mizell et al., 2022). For ferromanganese minerals, formation criteria include factors such as substrate availability, ocean sedimentation rates, and surface primary productivity. The US Geological Survey has identified PMORs present in and around the U.S. EEZ that can serve as a first-order spatial outline of regions with marine mineral resource potential (Mizell et al., 2022). While the potential occurrence of one or more minerals in PMORs exists, marine minerals are not guaranteed to be present in a PMOR or to contain metal or mineral concentrations high enough to be of commercial interest. In addition, we note that because large expanses of seafloor have uniform geographic and oceanographic characteristics, prospective regions represent seafloor areas that may be far larger than any actual exploration or commercial exploitation footprint would likely be in any of these areas. For example, ISA allocates to each contractor an area of 3,000 km2 for the exploration of ferromanganese crusts (ISA, 2023). However, the size of contracted exploitation areas for crusts will be considerably smaller because conditions favorable for a viable commercial mining operation will be limited within exploration blocks (Hein et al., 2009). We do not suggest that entire PMORs would be mined but they are useful to create an outer boundary in spatial analyses large enough to enclose the possible area of environmental impact. We recognize that some impacts such as noise and sediment plumes might travel distances beyond defined PMOR boundaries. PMORs of various sizes are indicated within most regions of the U.S. EEZ including the East and West Coasts, the Subarctic and Arctic regions around Alaska, the Hawaiian Islands, and the Pacific Territories.
We limited our study area to four areas containing PMORs within the U.S. EEZ for which there was sufficient data on species distributions, seafloor habitats, and human uses and infrastructure to draw some preliminary conclusions: the Blake Plateau ferromanganese crust PMOR off the coast of the Carolinas (32,271 km2), PMOR for ferromanganese crusts in the offshore region of California (217,119 km2), PMORs for ferromanganese crusts in the Gulf of Alaska (60,488 km2 total), and PMORs for ferromanganese crusts and manganese nodules in the Hawaiian Islands (184,116 km2 ferromanganese crusts and 188,080 km2 manganese nodules; Figure 1).
Figure 1 The Blake Plateau, offshore California, Hawaiian Islands, and Gulf of Alaska prospective mineral occurrence regions (PMORs). Areas of PMORs adapted from data collected by the USGS (Mizell et al., 2022). Ocean Conservancy modified the Hawaiian Islands POMR in consultation with USGS. United States Exclusive Economic Zone (EEZ) is shown and is provided by the Flanders Marine Institute. Basemaps provided by ESRI.
We utilized a combination of national- and global-scale datasets to assess the occurrence of both environmental variables and human uses within PMORs (Table 1). Environmental variables were defined as any environmentally significant characteristics or features present in an area, including the following: seabed topography, habitat suitability for deep-sea corals, threatened species distributions, and marine animal species richness. We did not evaluate ecosystem function, or the interaction among environmental variables that drive biophysical processes such as predator-prey dynamics or carbon cycling, due to a paucity of information in regions analyzed. Human use variables were defined as any type of documented human use within a given PMOR, including fisheries, shipping, and submarine cables (Table 1).
We divided PMORs into grids composed of 2,500 square kilometer-sized hexagonal grid cells. Hexagonal grids are commonly used when capturing heterogeneous spatial trends over large areas and help minimize edge effects in geospatial analyses (Birch et al., 2007). We tested varying hexagon area sizes until finding a size that successfully captured the spatial variability of our input variables. To test this, we started with larger-sized hexagonal cells (5,000 square kilometers) and calculated the average occurrence of each variable across all hexagonal cells. Then, we repeated the process on smaller cell sizes, working in reductions of 500 square kilometers until the average occurrence and spread (standard deviation) of a variable stabilized and no longer changed with diminishing hexagonal grid size.
See Table 2 for an example of the grid cell size selection process we applied to one variable, mean fisheries landings. Here, mean fisheries landings, and associated standard deviations, were calculated per grid cell for varying hexagon sizes. We found that, similar to other variables, mean fisheries landings and standard deviations remained approximately the same between 2,500 and 1,500 square kilometers. As values for mean fisheries landings, and all other variables, stabilized at the 2,500-square kilometer size with no meaningful difference below this size, we elected to use this hexagonal cell size for our analysis. This hexagonal grid cell structure allowed us to then capture the spatial heterogeneity and variability of our input variables.
Then, for each hexagonal grid cell, we calculated summary metrics for each variable to assess the occurrence intensity and spatial patterns of environmental and/or human use variables within each PMOR. Subsequently, we converted the summary metrics for all of our variables to a normalized score ranging from 0 to 1 using a modified form of the minimum-maximum normalization equation (equation 1):
where x’ is the normalized value of the variable, x is the recorded value for a variable in a grid cell, and max(x) is the maximum recorded value for that variable across all PMORs. This normalization method allowed all data to occupy a standard range where a value of 0 represented no recorded occurrence of a variable and 1 represented the highest occurrence of that variable. It is important to note that we determined the minimum and maximum values by the lowest and highest values present for a variable across all PMORs. We chose this normalization method as it offered the simplest pathway to scoring the occurrence of different variables while 1) preserving the data’s structure such that even slight differences between locations would remain observable, and 2) detecting areas where a certain variable may disproportionately occur relative to all other PMORs. To provide an example, if a grid cell in the Gulf of Alaska scored close to a 1 for fisheries, that cell had a relatively high occurrence of fisheries not only within the Gulf of Alaska PMOR, but across all PMORs as well. Such a methodology thus allowed us to identify the variables that may co-occur unevenly within the various PMORs. While we make absolute comparisons among the four regions across variables, we acknowledge that each region’s score is contextual; a comparatively lower score for fisheries in the Blake Plateau, for example, does not mean fisheries are unimportant in that region. We considered categorizing the data into discrete levels, such as low or high occurrence but decided against this approach as it would have eliminated the ability to identify the specific score variations among adjacent, but similarly scored areas.
Following data normalization, we summed the occurrence scores of all variables to produce two summary occurrence scores: an environmental variable score (range: 0 - 11) and a human use variable score (range: 0 - 3). Notably, this method weighed all variables equally such that no variable was considered more or less important in the overall score, avoiding biases in the treatment of variables. The environmental and human use variables used in this analysis were selected based on data availability and consistency across regions, and we acknowledge that they underrepresent the biodiversity, habitats or ecosystem services found in PMORs. We mapped these occurrence scores to visually identify spatial patterns among areas within PMORs and highlight those areas that displayed relatively high or low environmental and/or human use variable scores.
For environmental variable scores, a grid cell was classified as having a low or high occurrence score if its score was one standard deviation below or above the mean environmental variable score, respectively. For human use variable scores, a grid cell was classified as having a high occurrence score if its human use variable score was one standard deviation above the mean human use occurrence scores. However, a grid cell was classified as having a low human use occurrence score if its human use variable score was zero. The standard deviation of the human use scores were larger than the mean human use score, meaning that values below one standard deviation would have been negative. Therefore, we elected to classify areas as low human use if their scores were zero. (See Supplementary Information for environmental and human use variable occurrence data, raw scores for each variable, definitions of variables and the treatment of variables in the final scoring.)
The occurrence of each environmental and human use variable varied considerably within and among PMORs. For the purposes of illustrating the proportional occurrence of the different factors within each PMOR, we summed certain scores into broad singular scores. First, scores for all taxonomic groups, from class Mammalia to order Testudines, were totaled for a single total species richness score to demonstrate the proportion of a region’s total environmental score that was composed of that region’s overall species richness. Second, scores for vulnerable species, endangered species, and critically endangered species were tallied into a single threatened species score to demonstrate the proportion of a region’s total environmental score that was composed of the total number of threatened species present in that region. The quantification of taxa here is a relative measure of biodiversity and does not represent the ecosystem functioning roles they serve, either individually or collectively.
Environmental variable scores and human use variable scores varied spatially within and across all PMORs (Figures 2, 3). The mean environmental variable score for all PMORs was 3.17 (SD = 1.20). Grid cells were classified as having low or high environmental variable scores if they, respectively, scored below 1.97 or above 4.37. The mean human use variable score for all PMORs was 0.16 (SD = 0.21). Grid cells were classified as having low or high human use variable scores if they, respectively, scored 0 or above 0.37. (See Supplemental Information for detailed tables with the results for each variable by PMOR.)
Figure 2 Preliminary environmental occurrence maps for the Blake Plateau, offshore California, Hawaiian Islands, and Gulf of Alaska regions. Bar charts show the proportional occurrence of each variable that makes up each PMOR’s total environmental score. Occurrence categories are defined as: Low =<1 standard deviation (SD); Medium = within 1 SD; High = >1 SD. Marine protected areas (MPAs) are marked on maps. Mineral Occurrence areas (PMORs) adapted from data collected by the USGS (Mizell et al., 2022). MPA locations adapted from NOAA’s Marine Protected Areas Inventory (2020). The United States Exclusive Economic Zone (EEZ) is shown and is provided by the Flanders Marine Institute. Basemaps provided by ESRI. See Supplemental Information for maps (S1-S11) showing the scores for each environmental variable within each PMOR.
Figure 3 Preliminary human use maps for the Blake Plateau, offshore California, Hawaiian Islands, and Gulf of Alaska regions. Bar charts show the proportional occurrence of each variable that makes up each PMOR’s total human use score. Occurrence categories are defined as: Low = 0 recorded occurrence; Medium = above 0 but within 1 SD; High = >1 SD. Marine protected areas (MPAs) are marked on maps. Mineral Occurrence areas (PMORs) adapted from data collected by the USGS (Mizell et al., 2022). MPA locations adapted from NOAA's Marine Protected Areas Inventory (2020). The United States Exclusive Economic Zone (EEZ) is shown and is provided by the Flanders Marine Institute. Basemaps provided by ESRI. All maps are at different scales. See Supplemental Information for maps (S12-S14) showing the scores for each human use variable within each PMOR.
Of all the PMORs, the Blake Plateau region had the highest median environmental variable score (5.35; Figure 4A) and demonstrated a high environmental occurrence score across all of its grid cells (Figure 2). The Blake Plateau’s high environmental occurrence scores were driven by higher levels of octocoral suitability and species richness (Figure 2). Modeled octocoral suitability data utilized in our analyses were based on observed octocoral distribution presence data and 32 environmental variables favorable for octocoral occurrence, including bathymetric (e.g., depth and slope), physical (e.g., temperature), chemical (e.g., salinity), hydrological (e.g., current flow), and biological variables (e.g., primary productivity) (Yesson et al., 2012).
The Blake Plateau had a mean octocoral suitability of 21.29 which was approximately six times higher than the next highest mean octocoral suitability observed in any other PMOR (Table 3). Additionally, at least 5 critically endangered species were found to be present within all the Blake Plateau’s grid cells.
After the Blake Plateau PMOR, the next highest median environmental occurrence scores were found in the Hawaiian Islands PMOR (3.32) followed by the Offshore California (3.05) and Gulf of Alaska PMORs (1.52; Figure 4A). The Hawaiian Islands PMOR did not have any variable that demonstrated, on average, much higher occurrences than the other PMORs (Table 3) scoring the highest in the presence of fish species and endangered species by a slight margin (1-2 more species; Table 3). However, we found that the Hawaiian Islands PMOR had grid cells with notably high environmental variable scores, especially within the boundaries of the Papahānaumokuākea Marine National Monument (Figure 2). In fact, the Hawaiian Islands PMOR contained the seven highest environmental variable scores (Figure 4A). These notably high scores were found to be driven by much higher levels of total species richness (Figure 2). The Hawaiian Islands PMOR had the only grid cells to contain over 1,000 species.
In the Offshore California PMOR, we observed uniformly high environmental occurrence scores along the eastern boundary of the PMOR (Figure 2). However, similar to the Hawaiian Islands PMOR, this region did not have, on average, notably different occurrence scores for any environmental variable (Table 3). Finally, the Gulf of Alaska PMOR was found to have among the lowest environmental variable scores with a small number of grid cells not having low environmental variable scores (Figures 2, 4A). These were driven by a lower recorded number of threatened species with an average of approximately 9 threatened species found in each grid cell and no recorded presence of critically endangered species (Table 3). Additionally, species richness was also found to be the lowest in the Gulf of Alaska PMOR with grid cells containing, on average, approximately 214 species present (Figure 2, Table 3). Grid cells with relatively higher environmental variable scores in this PMOR were found to coincide with the occurrence or nearby presence of seamounts.
For human uses, the Offshore California PMOR had the highest median human use variable score (0.22; Figure 4B) and had its highest human use variable scores concentrated within the center of the PMOR (Figure 3). These were primarily driven by the high level of shipping transit that was observed in this PMOR with an average of approximately 500+ vessel tracks observed per grid cell (Table 4). Additionally, this PMOR had a larger presence of submarine cables with grid cells containing, on average, ~32 miles of cables (Table 4). Both mean values were over three times higher than the next highest mean values observed for each metric.
The next highest human use variable scores were observed in the Gulf of Alaska PMOR (median = 0.13) where grid cells demonstrating high human use were driven by a higher amount of reported fisheries landings within these grid cells (Table 4; Figure 3). In fact, this PMOR reported the highest mean fisheries landings per grid cell with approximately 83 metric tons of fish landed per grid cell (Table 4). The Gulf of Alaska PMOR also had moderate levels of submarine cables (~ 9 miles per grid cell) and shipping transit (~ 117 vessel tracks per grid cell). Interestingly, some grid cells with higher human use variable scores in this PMOR coincided with areas that also reported relatively high environmental variable scores for that PMOR (Figures 2, 3).
The Blake Plateau demonstrated the lowest averages of fisheries production (~25 metric tons) and submarine cables (6 miles) per grid cell but did demonstrate the second highest levels of shipping transit averaging approximately 170 vessel tracks per grid cell. Even with its lower levels of fisheries and infrastructure, the Blake Plateau PMOR still had a uniform occurrence of human uses throughout the PMOR (median = 0.08; Figure 3). On the other hand, the Hawaiian Islands PMOR (median = 0.04) had higher averages for fisheries (~37 metric tons) and submarine cables (~7 miles) but demonstrated the highest number of grid cells with no recorded human uses, all of which occurred within the Papahānaumokuākea Marine National Monument (Figure 3). Additionally, the Hawaiian Islands PMOR saw the least amount of shipping transit by far, recording only an average of approximately 1 vessel track per grid cell (Table 4). The few grid cells reporting high human use variable scores in this PMOR were also located within the marine national monument, but these high scores were found to be driven mostly by the presence of submarine cables.
Our spatially explicit analysis of the co-occurrence of PMORs with ecological features and human uses suggests that any future seabed mining in these locations would overlap with and potentially impact various habitats, species, ecosystem services, and existing infrastructure. To better understand the types or magnitudes of interactions among mineral prospecting, marine life, or human uses in PMORs, we discuss them here in the context of the potential disturbances specific to DSM of ferromanganese crusts or manganese nodules, and their corresponding general seafloor environments, seamounts vs abyssal plains, respectively.
Manganese nodules occur as fields of individual spheroidal concretions sitting at the surface of soft sediments in the abyssal plains of ocean basins, ranging from 4,000 - 6,000 m deep (Mizell and Hein, 2021). The primary environmental impacts from manganese nodule mining based on current mining plans and technology prototypes have been currently theorized to be: removal of substrate (nodules) for benthic fauna (Vanreusel et al., 2016; Stratmann et al., 2021) and a high probability of direct mortality of immobile epifauna and infauna (Kaikkonen et al., 2021), disturbance of seafloor sediment, transport of benthic plumes and sediment resettling (e.g. Orcutt et al., 2020), noise and light and their potential to alter animal behavior (Williams et al., 2022, Levin et al., 2016), and release of sediment and mineral-rich fluids into the water column from surface spills, riser pipe leaks, or dewatering operations (Conley et al., 2018; Christiansen et al., 2020; Drazen et al., 2020; Muñoz-Royo et al., 2021).
The Clarion-Clipperton Zone (CCZ), encompasses a large abyssal plain area of the equatorial Pacific Ocean and contains vast fields of polymetallic nodules rich in manganese, nickel, copper, cobalt, and trace amounts of other metals that are of interest for mining (ISA, 2010). The south-southeast section of the Hawaiian Islands PMOR is located near the boundary of the CCZ and therefore may also contain manganese nodules with mineral resource potential and may experience impacts from nodule mining in the CCZ, though samples to confirm nodule presence in this PMOR have not been collected. However, the effects of mining in this section of the CCZ might or might not reach the PMOR, independent of the presence of nodules.
If manganese nodule mining were to occur in the CCZ or Hawaii EEZ, benthic and pelagic organisms in the Hawaiian Islands PMOR would be potentially exposed to seabed alteration and sediment plumes. Our analysis shows relatively high species richness in ray-finned fishes, crustaceans, sharks, and sea turtles and high numbers of endangered species in the Hawaiian Islands PMOR compared to the other U.S. PMORs studied, raising concerns about DSM impacts on these taxa. Some fisheries in the Hawaiian Islands’ PMOR, particularly Hawaii’s $100 million bigeye (ahi) tuna fishery, could face risk because of mining-induced ecosystem impacts. The shallower waters over the CCZ host the highest reported catches of tuna compared to other global areas contracted by ISA for mining exploration (van der Grient and Drazen, 2021). Dewatering plumes and surface spills that may result from mining activities could directly or indirectly interfere with tunas depending on the depth of discharge in the water column (van der Grient and Drazen, 2021). Bigeye tuna can occur as deep as 300 to 400 m, potentially increasing their exposure to vertically moving sediments or reducing foraging opportunities due to midwater food web impacts (Musyl et al., 2003).
For the Hawaii regions specifically, the eastward Subtropical Countercurrent and Hawaiian Lee Countercurrent, characterized as surface currents occurring at depths of approximately 150 m, overlap with two areas of prospective mineral occurrences in the Hawaiian Islands PMOR (Liu et al., 2003; Toonen et al., 2011). Beyond the direct footprint of nodule extraction sites, the potential for water chemistry changes, introduction of contaminants and transport of sediment and mineral-rich plumes associated with wastewater releases is of concern for DSM. Theoretical discharges of water collected at depth is expected to differ in temperature, salinity and oxygen concentrations depending on release depth and could contain higher metal concentrations resulting from fragmented nodules (Fuchida et al., 2018; United Nations Environment Programme Finance Initiative, 2022).
Discharge depth will influence the distance of sediment dispersal; a shallow-water discharge is more likely to be entrained in the surficial Subtropical Countercurrent and Hawaiian Lee Countercurrent. In addition, modeling shows that transport potential will depend on the size of the sediment particles, with finer and smaller particles traveling farther (Baeye et al., 2022). Hypothetically, fine sediment could take as long as a year to settle on the seabed and might be carried distances of several hundreds to thousands of kilometers before settling (Ouillon et al., 2022). Under the right circumstances, these two currents could elevate the likelihood of sediment advection beyond the immediate mining footprint into surrounding waters and possibly beyond, potentially impacting pelagic species by interfering with feeding, respiration, or sensory functions (e.g., diel vertical migrations cued by sunlight) or by smothering shallower-water benthos through re-sedimentation (Niner et al., 2018). Researchers acknowledge the complexities and vagaries in plume dynamics, underscoring the need for further spatially-explicit plume modeling (e.g. Muñoz-Royo et al., 2022; Weaver et al., 2022). Indeed, modeling to show space use conflicts within the broader mining impact footprint would be a useful follow on to the initial mineral occurrence overlap analyses presented here.
Ferromanganese crusts occur as thick coatings that form on exposed rock surfaces of seamounts, ridges, and plateaus throughout ocean basins, which allows them to occur at a much broader range of depths than manganese nodules, typically between 800 and 4,000 m (Hein and Petersen, 2013; Mizell and Hein, 2021). Extraction technologies are still in the research and development phase for ferromanganese crusts, yet general impacts of ferromanganese crust mining are expected to be substrate removal and associated habitat destruction and mortality to immobile or slow-moving organisms, light and noise pollution, sediment plume generation, and accidental wastewater discharges throughout the water column (Bluhm, 2001; Clark and Smith, 2013, Levin et al., 2016, Christiansen et al., 2020). Slow-growing, cold-water corals, sponges, and other sessile organisms common to seamount habitats are highly vulnerable to damage or removal during ferromanganese crust extraction. In the water column around seamounts, ocean currents interact with seamounts to create upwelling that delivers nutrients to surface waters. This upwelling of nutrients drives phytoplankton growth that in turn supports higher diversity and biomass of pelagic species compared to other oceanic areas (Morato et al., 2010), such as aggregations of surface sharks, seabirds, and marine mammals (Pitcher et al., 2007). Field experiments show that the open ocean surface water disposal of mine tailings interfered with photosynthesis and phytoplankton growth and diversity (Choi et al., 2022). As such, impaired primary production could impact the base of the pelagic food web and potentially cascade through higher trophic levels with unknown consequences for predators. Metal- and sediment-heavy plumes and noise generated by mining machinery or dewatering plumes could inhibit the physiology (gill function), predation (visual contact) or communication abilities of some taxa, including fishes and marine mammals, drawn to seamounts to feed (Drazen et al., 2020; Thompson et al., 2023).
There are more PMORs for ferromanganese crusts than nodules in the four U.S. regions analyzed in this study (Mizell et al., 2022). To put into perspective the amount of seafloor area within the U.S. EEZ that may experience impacts from DSM for ferromanganese crusts, we can consider the predicted mine site footprint. Hein et al. (2009) calculated that the optimal area needed to support a 20-year commercially viable ferromanganese crust mine site atop an average-size guyot in the north-equatorial Pacific is approximately 260 km2. Assuming the ferromanganese crust characteristics used to generate this estimate are similar to those in the Hawaiian Islands PMOR (372,196 km2), which has not been validated, a single mine site would encompass 0.07% of total seafloor area outlined by the PMOR. The relatively small amount of area that this theoretical mine site encompasses as a fraction of the PMORs highlights the importance of careful spatial analyses to determine where environmental impact can be minimized in PMORs if mining were pursued.
A primary human use concern for DSM of ferromanganese crusts is its impact on fisheries. In open ocean settings, seamounts, and other seafloor edifices, upon which ferromanganese crusts grow, are among the most productive fishery habitats, accounting for high species diversity and fish biomass (Watson et al., 2007). PMORs within the Gulf of Alaska, on average, reported the highest fisheries landings at 83 metric tons, which was more than twice that of the next highest. We assume that seamount-associated demersal fishes would be most susceptible to theoretical DSM considering their long lifespans, late age at sexual maturity, slow growth, and spatial overlap with prospective occurrences of ferromanganese crusts (Morato et al., 2006). Sablefish (black cod), supporting an Alaskan-wide fishery valued at $166 million, is the dominant finfish species on Gulf of Alaska seamounts, with older, larger sablefish migrating to seamounts from the continental slope (Maloney, 2004; Kapur et al., 2021). Physical damage to seamount habitats, noise, and increased turbidity from excavation or wastewater discharge are the most likely mining-related disturbances to affect populations comprised largely of more mature, male sablefish (Maloney, 2004). Seamounts in the Hawaiian archipelago are known to aggregate yellowfin, bigeye, or albacore tuna, likely contributing to increased fishing opportunities or higher catches in the region (Yasui, 1986; Sibert et al., 2000). Therefore, DSM in the Hawaiian Islands could potentially affect tuna species and related fisheries through acute or ambient impacts.
Sessile or benthic biota found on or near seamounts are the most vulnerable to the excavation of ferromanganese crusts for minerals because: 1) they are less able to avoid the direct, physical impacts of mining activities; and 2) the extraction of crust would kill sessile organisms attached to the crust or eliminate their habitat and that used by demersal species. The taxa of greatest concern are octocorals, polychaetes, sponges, infauna, crustaceans, and demersal fishes as these slow-moving bottom dwellers presumably will be disproportionately impacted. Microbial diversity and communities living in or on crust sediments might be altered in ways that affect carbon cycling (Orcutt et al., 2015). Models show the Blake Plateau POMR has the highest suitability for octocorals, followed by the Gulf of Alaska, Offshore California, and the Hawaiian Islands. The PMORs ranking first for bony fish (Actinopterygii) and crustacean (Malacostraca) species richness are the Hawaiian Islands, followed by the Blake Plateau. Offshore California has the third highest species richness for bony fishes and crustaceans, while the Gulf of Alaska ranks fourth in species richness for both taxa. However, a more complete understanding of the density of these populations and of ecosystem dynamics at each PMOR is needed to fully evaluate the extent to which different taxa would be impacted by mining activities.
Parts of the Hawaiian Islands and Gulf of Alaska PMORs with ferromanganese crusts receive special protections due to their ecologically sensitive habitats. The Papahanaumokuakea Marine National Monument, encompassing a 2,170-kilometer-long segment of the Hawaiian archipelago, overlaps with the Hawaiian Islands PMOR. The Monument is ecologically significant for its concentration of endangered and threatened species and is culturally important to the Native Hawaiian community. Mining is prohibited inside the Monument, but commercial mining might be permissible in the unprotected, adjacent seamounts. It is not known whether and to what extent sediment plumes originating outside the monument would affect marine life moving into and out of the protected area, highlighting a research gap and priority for further study.
The Alaska Seamount Habitat Protection Area overlaps with the Gulf of Alaska PMOR. The North Pacific Fishery Management Council banned bottom trawling in the protected area after explorations in the 2000s reported large assemblages of demersal fish and invertebrates along with then newly discovered corals and sponges (Hoff and Stevens, 2005; Stone and Shotwell, 2007). We can use bottom trawl fishing and its impacts on seamount benthic fauna as an analog to seamount crust extraction in that both activities are or, in the case of theoretical DSM, would be in direct physical contact with the substrate. The most important differences between the two activities are intensity and duration; entire excavation of the crust for ore extraction would be a single comparatively more intense event whereas trawling scours the same surface areas over many decades. Studies from Tasmania comparing trawled and untrawled seamounts show stark differences in the presence and density of matrix-forming corals. The absence of the coral Solensomilia variabilis from trawled seamounts combined with historical reports of high bycatch of the same coral species in nets strongly points to trawl gear mechanical damage as the cause (Althaus et al., 2009). Impacts from commercial-scale mining of seamount crusts would arguably be more acute than bottom trawling as the substrate used by corals and other sessile organisms would be completely removed, likely irreversibly altering benthic communities (Gollner et al., 2017; Washburn et al., 2019).
Other human uses analyzed for their spatial overlap with areas of higher mineral occurrence potential across all PMORs were shipping and transoceanic submarine cables, the latter likely associated with telecommunications infrastructure. The Offshore California ferromanganese crust PMOR had the highest ship traffic levels, which were three times that of the next busiest, the Blake Plateau ferromanganese crust PMOR, followed by the Gulf of Alaska ferromanganese crust and Hawaiian Island ferromanganese crust and nodule PMORs (Table 4). Statistically, the number of incidents, including collisions, is correlated with vessel traffic, with more incidents occurring in areas of higher vessel activity (Rawson et al., 2019; Rawson and Brito, 2021). Introducing additional survey, mining, and support vessels to PMORs with high numbers of container vessels and oil tankers, particularly in the densely trafficked Offshore California ferromanganese crust PMOR, could increase the risk of vessel collisions and oil spills.
The Offshore California ferromanganese crust PMOR had more subsea cables on a per-kilometer basis than any other PMOR, with the second, third and fourth highest cable densities documented, respectively, in the Gulf of Alaska ferromanganese crust, Hawaiian Islands ferromanganese crust and nodule, and Blake Plateau ferromanganese crust PMORs. We are not aware of any research in the public domain specifying the susceptibility of subsea cables to DSM. However, physical harm to the benthos, and cable infrastructure specifically, from anthropogenic activities, particularly fishing, is well documented; more than 40 percent of reported undersea cable damage is associated with commercial fisheries that encounter the seafloor through bottom-tending gear (Communications Security, Reliability and Interoperability Council, 2014; Clark et al., 2015). Considering that DSM’s direct physical footprint on the seabed would be analogous to, if not more permanent than, bottom trawling (Miller et al., 2018) it is important to identify the spatial overlap of potential mining locations and existing telecommunications infrastructure before nodule or crust removal commences.
This analysis and the conclusions drawn from it were limited by the number and resolution of spatially explicit datasets available. We relied on coarser-scale national and global datasets in the absence of more granular data, and most input data were based on models rather than direct empirical data. Despite having few empirical data, we nevertheless identified areas of higher potential conflict at the resolution of our analysis.
Erring on the side of simplicity, we did not weigh the relative risk of DSM to each environmental attribute and human use analyzed. The data normalization and scoring methods were also heavily influenced by outliers despite efforts to minimize this dependence, such as utilizing only databases that had at least one data point in each of the PMORs analyzed. Outliers (e.g., one grid cell area containing much higher species richness than all other areas) sometimes caused a disproportionately high or low value for a variable in one area which inflated or deflated the score difference among other areas. Occurrences of specific environmental variables or human uses were greater in some PMORs than others (Figures 2, 3). The lack of occurrence of one or more variables in a PMOR may be an artifact of the datasets used, and gaps in data, either observational or modeled, are possible. Thus, some caution is warranted in interpreting findings. We compared the occurrence of variables relative to all PMORs, but evaluations of individual PMORs and the data unique to each is another approach worth considering. For example, analyzing in more detail fishery catches attributed to a specific PMOR, rather than comparing catch levels across PMORs, would clarify the relative importance of DSM’s potential disruption to the fishery in that PMOR.
Our PMOR-specific fisheries data may be biased due to the inclusion of catch data centered just outside the U.S. EEZ where American, and potentially foreign, fishing fleets also operate. We decided it was appropriate to include fisheries data from areas immediately outside the U.S. EEZ for two reasons: 1) fish populations are spatially diffuse and are not constrained by geopolitical boundaries; and 2). Modeled sediment plumes, in the case of nodule mining in the CCZ, can travel 1400 km from the source (Muñoz-Royo et al., 2021), potentially exposing fish or their prey species to sediment particles beyond PMOR boundaries.
We also recognize that data availability is influenced by some level of sampling bias. For example, the Papahanaumokuakea Marine National Monument is a commonly visited region for studying benthic diversity due to its national designation, accessibility, and other variables, which generates a higher density of ecosystem data than less explored or more remote regions. More generally, physical proximity to ocean ecosystems determines sampling opportunity, generating an abundance of data in some ocean regions and disparities in others that can potentially downplay the biological diversity and importance of under-sampled areas. For example, shipping routes represent 2% of ocean area, but account for 18% of data collected and 41% of species, while sampling coverages in the deep-sea total< 0.6% across 10 major taxonomic groups at depths of 1,000 and 1,800 m and<0.02% at depths below 1800 m (Hughes et al., 2021). Similarly, latitudinal sampling bias generates differences in data availability, with less sampling near the equator than the mid-latitudes of the northern hemisphere, and this latitudinal gradient is also true for the deep-sea (Menegotto and Rangel, 2018). Marine ecosystem data richness and abundance can greatly affect spatial analyses by under or overstating potential conflicts or impacts. It is abundantly clear that unprotected, remote, or less accessible marine regions remain the highest priorities for further scientific exploration and data collection.
While our GIS methodology is useful for a rapid, preliminary assessment and provides a foundation for future DSM spatial conflict analyses, further assessment is warranted that involves finer-resolution data and uses datasets without biases arising from observation density or unexplainable outliers.
In the future, further spatially explicit analyses are needed to inform policy, management, and planning efforts. This information as it related to the U.S. EEZ is particularly timely, as recently established federal policies incentivize mineral development from domestic sources (Inflation Reduction Act, 2022). California, joining Oregon and Washington, preemptively banned hard mineral mining in their state territorial waters, citing the unacceptably high risk this activity poses to biodiversity, the sovereignty and rights of Native American tribes, fishing, and tourism (California Seabed Mining Prevention Act, 2022). State bans show that for these regions, the costs of mining are thought to outweigh its benefits to society.
The National Environmental Policy Act (42 U.S.C. § 4321 et seq.), the Endangered Species Act (16 U.S.C. § 1531 et seq.), Marine Mammal Protection Act (16 U.S.C. § 1361 et seq.), and other relevant U.S. laws require assessments of deep-sea mining impacts and measures to avoid or mitigate impacts on sensitive species or ecosystems. Therefore, biological, social, and cultural data will be collected as a prerequisite for any seabed mining the U.S. EEZ. Regulators must be reasonably confident that the data are sufficiently robust to identify the full range of potential or likely impacts that in turn are needed to trigger requirements to avoid or mitigate ecological and social harm (Endangered Species Act, 16 U.S.C. § 1533(b)(1)(A); Magnuson-Stevens Fishery Conservation and Management Act, 16 U.S.C. § 1851(a)(2); and National Environmental Policy Act, 40 CFR Parts 1508.1(s), 1508.20.).
Data gaps remain a barrier to DSM impact analyses within the U.S. EEZ where ecosystem knowledge and baseline data on biodiversity as well as confirmed mineral presence and composition remains sparse. The paucity of baseline empirical or observational data, particularly in the deep, abyssal mineral-associated ecosystems, is an obstacle to evaluating potential for DSM, as well as the potential for evaluating DSM impacts and to interpreting actual impacts if or when commercial DSM occurs (Jones et al., 2017; Kaikkonen et al., 2021). Our GIS analysis suggests an urgent need for collecting environmental, human use, and marine mineral data at a finer spatial scale than what currently exists and making it available on public databases. Particular data gaps that could influence this sort of analysis are the incomplete information on migratory routes for some taxa (e.g., whales), acoustic surveys for biomass assessments, and deep-sea coral observations. While these data do exist, they were either not uniformly available across all PMORs or were collected using different methodologies such that they could not be integrated or combined for our analysis.
Surveys and analyses are needed to account for the distribution of species in relation to potential DSM sites. This specificity can be used to determine levels of confidence in how DSM will differentially affect benthic or pelagic organisms (van Doorn et al., 2022). For example, benthic ecosystems are proposed to be subject to the greatest impacts via seabed destruction and habitat loss, but surface water or mesopelagic ecosystems may be more susceptible to sediment discharge (Spearman et al., 2020). Additionally, a better understanding of how DSM may interfere with other human uses or existing infrastructure is critical to assessing and predicting potential DSM conflicts. Further research should not be constrained just to DSM sites as mining activities will likely impact surrounding areas (Spearman et al., 2020; Katona et al., 2021; Williams et al., 2022). Empirical data on the relative abundance of critical minerals, presence of environmental features, and human uses are urgently needed to inform future risk assessments and DSM management.
The raw data supporting the conclusions of this article will be made available by the authors, without undue reservation.
AG conducted data search, co-developed geospatial analyses methods, conducted the geospatial analyses, wrote initial drafts of manuscript, and contributed to manuscript review. CR co-conceived the geospatial analysis with Ocean Conservancy colleague, provided early and frequent guidance on the GIS approach and subsequent refinements, initially drafted the discussion and managed the co-author review process. JM provided input on parameters and information sources for the analysis, particularly on emerging or future human uses, provided feedback on the initial analysis outputs, and results and reviewed an initial manuscript draft. JD provided technical guidance for the GIS analysis and developed some of the maps used in the manuscript. SC co-conceived the geospatial analysis, reviewed draft manuscripts and recommended changes to strengthen the rationale and framing of the analysis and its findings. GL provided strategic advice on initial project scope, framing and analysis, reviewed and edited various iterations of the manuscript, and sought outside review by subject matter experts before formal submission. All authors contributed to the article and approved the submitted version.
AG’s participation in this study was funded by the Climate Adaptation Science (CAS) program at Utah State University which is supported by the National Science Foundation (NSF) under grant no. 1633756.
We thank Dr. Lisa Levin, Scripps Institution of Oceanography for early input on geographies of interest for the geospatial analysis, Dr. Kira Mizell, U.S. Geological Survey, for her expertise in prospective mineral occurrences in the U.S. EEZ and input on the spatial analysis, Dr. Jesse van der Grient, South Atlantic Environmental Research Institute (SAERI), for reviewing the draft manuscript. We are also grateful to the two reviewers whose comments helped us improve this manuscript. Any use of trade, firm, or product names is for descriptive purposes only and does not imply endorsement by the U.S. Government.
The authors declare that the research was conducted in the absence of any commercial or financial relationships that could be construed as a potential conflict of interest.
All claims expressed in this article are solely those of the authors and do not necessarily represent those of their affiliated organizations, or those of the publisher, the editors and the reviewers. Any product that may be evaluated in this article, or claim that may be made by its manufacturer, is not guaranteed or endorsed by the publisher.
The scientific results and conclusions, as well as any views or opinions expressed herein, are those of the author(s) and do not necessarily reflect those of National Oceanic and Atmospheric Administration or the Department of Commerce.
The Supplementary Material for this article can be found online at: https://www.frontiersin.org/articles/10.3389/fmars.2023.1213424/full#supplementary-material
Althaus F., Williams A., Schlacher T., Kloser R., Green M., Barker B., et al. (2009). Impacts of bottom trawling on deep-coral ecosystems of seamounts are long-lasting. Mar. Ecol. Prog. Ser. 397, 279–294. doi: 10.3354/meps08248
Amon D. J., Gollner S., Morato T., Smith C. R., Chen C., Christiansen S., et al. (2022). Assessment of scientific gaps related to the effective environmental management of deep-seabed mining. Mar. Policy 138. doi: 10.1016/j.marpol.2022.105006
Baeye M., Purkiani K., de Stigter H., Gillard B., Fettweis M., Greinert J. (2022). Tidally driven dispersion of a deep-sea sediment plume originating from seafloor disturbance in the DISCOL area (SE-Pacific Ocean). Geosciences 12 (1), 8. doi: 10.3390/geosciences12010008
Birch C. P. D., Oom S. P., Beecham J. A. (2007). Rectangular and hexagonal grids used for observation, experiment and simulation in ecology. Ecol. Model. 206, 347–359. doi: 10.1016/j.ecolmodel.2007.03.041
Bluhm H. (2001). Re-establishment of an abyssal megabenthic community after experimental physical disturbance of the seafloor. Deep Sea Res. Part II Top. Stud. Oceanogr. 48, 3841–3868. doi: 10.1016/S0967-0645(01)00070-4
Buonocore E., Grande U., Franzese P. P., Russo G. F. (2021). Trends and evolution in the concept of marine ecosystem services: an overview. Water 13, 15. doi: 10.3390/w13152060
California Seabed Mining Prevention Act (2022). AB-1832, Chapter 433 (Sept. 19, 2022). (State of California, United States).
Choi D. H., Yoo J., Yang W., Noh J. H., Lee Y., Ra K., et al. (2022). Potential short-term effects of mine tailings on phytoplankton assemblages in the open ocean. J. Mar. Sci. Eng. 1(1162). doi: 10.3390/jmse10081162
Christiansen B., Denda A., Christiansen S. (2020). Potential effects of deep seabed mining on pelagic and benthopelagic biota. Mar. Policy 114. doi: 10.1016/J.MARPOL.2019.02.014
Clark M. R., Althaus F., Schlacher T. A., Williams A., Bowden D. A., Rowden A. A. (2015). The impacts of deep-sea fisheries on benthic communities: a review. ICES J. Mar. Sci. 73 (1), i51–i69. doi: 10.1093/icesjms/fsv123
Clark M., Smith S. (2013). “Environmental management considerations,” in Deep Sea Minerals: Cobalt-rich Ferromanganese Crusts, a Physical, Biological, Environmental, and Technical Review. Eds. Baker E., Beaudoin Y. (Noumea, New Caledonia: Secretariat of the Pacific Community), 23–40.
Communications Security, Reliability and Interoperability Council (2014) Final Report: Protection of Submarine Cables Through Spatial Separation. Working Group 8 Submarine Cable Routing and Landing. Available at: https://transition.fcc.gov/pshs/advisory/csric4/CSRIC_IV_WG8_Report1_3Dec2014.pdf (Accessed January 31, 2023).
Conley K. R., Lombard F., Sutherland K. R. (2018). Mammoth grazers on the ocean’s minuteness: A review of selective feeding using mucous meshes. Proc. Biol. Sci. 285. doi: 10.1098/rspb.2018.0056
Drazen J. C., Smith C. R., Gjerde K. M., Haddock S. H. D., Carter G. S., Choy, et al. (2020). Midwater ecosystems must be considered when evaluating environmental risks of deep-sea mining. PNAS 117 (3), 17455–17460. doi: 10.1073/pnas.2011914117
Fuchida S., Ishibashi J. I., Shimada K., Nozaki T., Kumagai H., Kawachi M., et al. (2018). Onboard experiment investigating metal leaching of fresh hydrothermal sulfide cores into seawater. Geochem. Trans. 19 (1). doi: 10.1186/s12932-018-0060-9
Gielen D., Boshell F., Saygin D., Bazilian M. D., Wagner N., Gorini R., et al. (2019). The role of renewable energy in the global energy transformation. Energy Strategy Rev. 24, 38–50. doi: 10.1016/j.esr.2019.01.006
Global Parliamentary Declaration Calling for a Moratorium on Deep Seabed Mining (2022). Available at: https://www.pgaction.org/ilhr/oceans/call-for-moratorium-on-deep-seabed-mining.html (Accessed March 20, 2023).
Gollner S., Kaiser S., Menzel L., Jones D. O., Brown A., Mestre N. C., et al. (2017). Resilience of benthic deep-sea fauna to mining activities. Mar. Environ. Res. 129, 76–101. doi: 10.1016/j.marenvres.2017.04.010
Hein J. R., Conrad T. A., Dunham R. E. (2009). Seamount characteristics and mine-site model applied to exploration- and mining-lease-block selection for cobalt-rich ferromanganese crusts. Mar. Georesour. Geotechnol. 27, 160–176. doi: 10.1080/10641190902852485
Hein J. R., Petersen S. (2013). “The geology of cobalt-rich ferromanganese crusts,” in Deep Sea Minerals: Cobalt-rich Ferromanganese Crusts, a Physical, Biological, Environmental, and Technical review. Eds. Baker E., Beaudoin Y. (Noumea, New Caledonia: Secretariat of the Pacific Community), 7–14.
Hoff G. R., Stevens B. (2005). Faunal assemblages structure on the Patton Seamount (Gulf of Alaska, USA). Alaska Fish. Res. Bull. 11, 27–35.
Hughes A. C., Orr M. C., Ma M., Costello M. J., Waller J., Provoost P., et al. (2021) Sampling biases shape our view of the natural world. Ecography 44 (9). doi: 10.1111/ecog.05926
IEA (2022) The Role of Critical Minerals in Clean Energy Transitions. Available at: www.iea.org (Accessed October 26, 2022).
ISA (2010). A Geologic Model for Polymetallic Nodule Deposits in the Clarion–Clipperton Fracture Zone. Technical Study No. 6 (Kingston, Jamaica: International Seabed Authority).
ISA (2023) Explortation Areas. Available at: www.isa.org.jm/exploration-contracts/exploration-areas/ (Accessed March 22, 2023).
Jones D. O. B., Kaiser S., Sweetman A. K., Smith C. R., Menot L., Vink A., et al. (2017). Biological responses to disturbance from simulated deep-sea polymetallic nodule mining. PloS One 12 (2). doi: 10.1371/journal.pone.0171750
Kaikkonen L., Helle I., Kostamo K., Kuikka S., Törnroos A., Nygård H., et al. (2021). Causal approach to determining the environmental risks of seabed mining. Environ. Sci. Technol. 55 (13), 8502–8513. doi: 10.1021/acs.est.1c01241
Kapur M. S., Lee Q., Correa G. M., Haltuch M., Gertseva V., Hamel O. S. (2021). DRAFT Status of Sablefish (Anoplopoma fimbria) along the US West coast in 2021 (Portland: Pacific Fisheries Management Council).
Katona S., Paulikas D., Stone G. S. (2021). Ethical opportunities in deep-sea collection of polymetallic nodules from the Clarion-Clipperton Zone. Integr. Environ. Assess. Manage. 18 (3), 634–654. doi: 10.1002/ieam.4554
Le J. T., Levin L. A., Carson R. T. (2017). Incorporating ecosystem services into environmental management of deep-seabed mining. Deep-Sea Res. II 137, 486–503. doi: 10.1016/j.dsr2.2016.08.007
Levin L., Mengerink K., Gjerde K. M., Rowden A. A., Van Dover C. L., Clark M. R., et al. (2016). Defining “serious harm” to the marine environment in the context of deep-seabed mining. Mar. Policy 74. doi: 10.1016/j.marpol.2016.09.032
Liu Q., Wang S., Wang Q., Wang W. (2003). On the formation of Subtropical Countercurrent to the west of the Hawaiian Islands. J. Geophys. Res. Oceans 108 (C5). doi: 10.1029/2002JC001366
Maloney N. B. (2004). Sablefish, Anoplopoma fimbria, populations on Gulf of Alaska seamounts. Mar. Fish. Rev. 66, 1–12.
Mangi S. C., Davis C. E., Payne L. A., Austen M. C., Simmonds D., Beaumont N. J., et al. (2011). Valuing the regulatory services provided by marine ecosystems. Environmetrics 22, 686–698. doi: 10.1002/env.1095
Marine Protected Areas Inventory. (2020). The MPA Inventory. Available at https://marineprotectedareas.noaa.gov/dataanalysis/mpainventory/ (Accessed July 18, 2023).
Menegotto A., Rangel T. F. (2018). Mapping knowledge gaps in marine diversity reveals a latitudinal gradient of missing species richness. Nat. Commun. 9, 4713. doi: 10.1038/s41467-018-07217-7
Miller K. A., Thompson K. F., Johnston P., Santillo D. (2018). An overview of seabed mining including the current state of development, environmental impacts, and knowledge gaps. Front. Mar. Sci. 4. doi: 10.3389/fmars.2017.00418
Mizell K., Hein J. R. (2021). “Ocean floor manganese deposits,” in Encyclopedia of Geology (Second Edition). Eds. Alderton D., Elias S. A. (Academic Press), 993–1001. doi: 10.1016/B978-0-08-102908-4.00030-8
Mizell K., Hein J. R., Au M., Gartman A. (2022). “Estimates of metals contained in abyssal manganese nodules and Ferromanganese crusts in the global ocean based on regional variations and genetic types of nodules,” in Perspectives on Deep-Sea Mining. Ed. Sharma (New York, NY: Springer), 53–80. doi: 10.1007/978-3-030-87982-2_3
Morato T., Cheung W. W. L., Pitcher T. J. (2006). Vulnerability of seamount fish to fishing: fuzzy analysis of life-history attributes. J. Fish Biol. 68, 209–221. doi: 10.1111/j.0022-1112.2006.00894.x
Morato T., Hoyle S. D., Allain V., Nicol S. J. (2010). Seamounts are hotspots of pelagic biodiversity in the open ocean. Proc. Natl. Acad. Sci. 107, 9707–9711. doi: 10.1073/pnas.0910290107
Muñoz-Royo C., Ouillon R., Mousadik S., Alford M. H., Peacock T. (2022). An in situ study of abyssal turbidity-current sediment plumes generated by a deep seabed polymetallic nodule mining preprototype collector vehicle. Sci. Adv. 8 (38). doi: 10.1126/sciadv.abn1219
Muñoz-Royo C., Peacock T., Alford M. H., Smith J. A., Boyer L. A., Kulkarni C. S. (2021). Extent of impact of deep-sea nodule mining midwater plumes is influenced by sediment loading, turbulence and thresholds. Commun. Earth Environ. 2, 148. doi: 10.1038/s43247-021-00213-8
Musyl M. K., Brill R. W., Boggs C. H., Curran D. S., Kazama T. K., Seki M. P. (2003). Vertical movements of bigeye tuna (Thunnus obesus) associated with islands, buoys, and seamounts near the main Hawaiian Islands from archival tagging data. Fish. Oceanogr. 12 (3), 152–169. doi: 10.1046/j.1365-2419.2003.00229.x
Nassar N. T., Alonso E., Brainard J. L. (2020). Investigation of U.S. Foreign Reliance on Critical Minerals—U.S. Geological Survey Technical Input Document in Response to Executive Order no. 13953 signed September 30, 2020 (Ver. 1.1, December 7, 2020): U.S. Geological Survey Open-File Report 2020–1127. (U.S. Geological Survey). doi: 10.3133/ofr20201127
Niner H. J., Ardon J. A., Escobar E. G., Gianni M., Jaeckel A., Jones D. O. B., et al. (2018). Deep-sea mining with no net loss of biodiversity – an impossible aim. Front. Mar. Sci. 5. doi: 10.3389/fmars.2018.00053
Orcutt B. N., Bradley J. A., Brazelton W. J., Estes E. R., Goordial, Huber J. M., et al. (2020). Impacts of deep-sea mining on microbial ecosystem services. Limnol. Oceanogr. 65 (7), 1489–1510. doi: 10.1002/LNO.11403
Orcutt B. N., Sylvan J. B., Rogers D. R., Delaney J., Lee R. W., Girguis P. R. (2015). Carbon fixation by basalt-hosted microbial communities. Front. Microbiol. 6 (904). doi: 10.3389/fmicb.2015.00904
Ouillon R., Muñoz-Royo C., Alford M., Peacock T. (2022). Advection-diffusion settling of deep-sea mining sediment plumes. Part 1: Midwater plumes. Flow 2 (E22). doi: 10.1017/flo.2022.20
Pitcher T. J., Morato T., Hart P. J. B., Clark M. R., Haggan N., Santos R. S. (2007). Seamounts: ecology, fisheries, and conservation. Blackwell Fisheries and Aquatic Resources Series 12 (Oxford: Blackwell Publishing).
Pörtner H.-O., Roberts D. C., Tignor M., Poloczanska E. S., Mintenbeck K., Alegría A., et al. (2022). Climate Change 2022: Impacts, Adaptation, and Vulnerability,” in Contribution of Working Group II to the Sixth Assessment Report of the Intergovernmental Panel on Climate Change (Cambridge, UK and New York, NY, USA: Cambridge: Cambridge University Press. Presidential Executive Order 13953).
Rawson A., Brito M. (2021). A critique of the use of domain analysis for spatial collision risk assessment. Ocean Eng. 219. doi: 10.1016/j.oceaneng.2020.108259
Rawson A., Sabeur Z., Correndo G. (2019). “Spatial challenges of maritime risk analysis using big data,” in Proceedings of the 8th International Conference on Collision and Grounding of Ships and Offshore Structures. Ed. Soares C., Guedes (Boca RAton, FL: CRC Press/Balkema), 275–283.
Sibert J., Holland K., Itano D. (2000). Exchange rates of yellowfin and bigeye tunas and fishery interaction between Cross seamount and near-shore fads in Hawaii. Aquat. Living Resour. 13, 93–105. doi: 10.1016/S0990-7440(00)01057-3
Simon-Lledó E., Bett B. J., Huvenne V. A. I., Köser K., Schoening T., Greinert J., et al. (2019). Biological effects 26 years after simulated deep-sea mining. Sci. Rep. 9 (1). doi: 10.1038/s41598-019-44492-w
Smith C. R., Tunnicliffe V., Colaço A., Drazen J. C., Gollner S., Levin L. A., et al. (2020). Deep-sea misconceptions cause underestimation of seabed-mining impacts. Trends Ecol. Evol. 35 (10), 853–857. doi: 10.1016/j.tree.2020.07.002
Spearman J., Taylor J., Crossouard N., Cooper A., Turnbull M., Lee M., et al. (2020). Measurement and modelling of deep sea sediment plumes and implications for deep sea mining. Sci. Rep. 10. doi: 10.1038/s41598-020-61837-y
Stone R. P., Shotwell S. K. (2007). “State of deep coral ecosystems in the Alaska region: Gulf of Alaska, Bering Sea and the Aleutian islands,” in The State of Deep Coral Ecosystems of the United States. Eds. Lumsden S. E., Hourigan T. F., Bruckner A. W., Dorr G. (Silver Spring, Maryland: National Oceanic Atmospheric Administration), 65–108.
Stratmann T., Soetaert K., Kersken D., van Oevelen. D. (2021). Polymetallic nodules are essential for food-web integrity of a prospective deep-seabed mining area in Pacific abyssal plains. Sci. Rep. 11. doi: 10.1038/s41598-021-91703-4
Thompson K. G., Miller K. A., Wacker J., Derville S., Laing C., Santillo D., et al. (2023). Urgent assessment needed to evaluate potential impacts on cetaceans from deep seabed mining. Front. Mar. Sci. 10. doi: 10.3389/fmars.2023.1095930
Toonen R. J., Andrews K. R., Baums I. B., Bird C. E., Concepcion G. T., Daly-Engel T. S., et al. (2011). Defining boundaries for ecosystem-based management: A multispecies case study of marine connectivity across the Hawaiian archipelago. J. Mar. Biol. 2011, 13. doi: 10.1155/2011/460173
United Nations Convention on the Law of the Sea (1982). 1833 UNTS 3 (entered into force 16 November 1994); Agreement Relating to the Implementation of Part XI of the United Nations Convention on the Law of the Sea of 10 December 1982, 28 July 1994, 1836 UNTS 3. (Secretary-General of the United Nations).
United Nations Environment Programme Finance Initiative (2022) Harmful Marine Extractives: Understanding the risks & impacts of financing non-renewable extractive industries - Offshore Oil and Gas. Available at: https://wedocs.unep.org/20.500.11822/40149 (Accessed February 2, 2023).
USGS (2022) Global Marine Mineral Resources. Available at: https://www.usgs.gov/centers/pcmsc/science/global-marine-mineral-resources (Accessed June 7, 2023).
van der Grient J. M. A., Drazen J. C. (2021). Potential spatial intersection between high-seas fisheries and deep-sea mining in international waters. Mar. Policy 129. doi: 10.1016/j.marpol.2021.104564
van Doorn E., Laugesen J., Haeckel M., Mestre N., Skjeret F., Vink A. (2022). “Risk assessment for deep-seabed mining,” in Perspectives on Deep-Sea Mining. Ed. Sharma (New York, NY: Springer), 497–526. doi: 10.1007/978-3-030-87982-2_3
Vanreusel A., Hilario A., Ribeiro P. A., Menot L., Arbizu P. M. (2016). Threatened by mining, polymetallic nodules are required to preserve abyssal epifauna. Sci. Rep. 6 (1). doi: 10.1038/srep26808
Vonnahme T. R., Molari M., Janssen F., Wenzhöfe F., Haeckel M., Titschack J., et al. (2020). Effects of a deep-sea mining experiment on seafloor microbial communities and functions after 26 years. Sci. Adv. 29;6 (18). doi: 10.1126/sciadv.aaz5922
Washburn T. W., Turner P. J., Durden J. M., Jones D. O. B., Weaver P., Van Dovers C. L. (2019). Ecological risk assessment for deep-sea mining. Ocean Coast. Manage. 176, 24–39. doi: 10.1016/j.ocecoaman.2019.04.014
Watson R., Kitchingman A., Cheung W. W. (2007). “Catches from world seamount fisheries,” in Seamounts: Ecology, Fisheries and Conservation. Eds. Pitcher T. P., Morato, Hart P. J. B., Clark M. R., Haggan N., Santos R. S. (Oxford: Blackwell), 400–412.
Weaver P. P. E., Aguzzi J., Boschen-Rose R. E., Colaço A., de Stigter H., Gollner S., et al. (2022). Assessing plume impacts caused by polymetallic nodule mining vehicles. Mar. Policy 139. doi: 10.1016/j.marpol.2022.105011
Williams R., Erbe C., Duncan A., Nielsen K., Washburn T., Smith C. (2022). Noise from deep-sea mining may span vast ocean areas. Science 377, 157–158. doi: 10.1126/science.abo2804
Yasui M., Uchida R. N., Hayasi S., Boehlert G. W. (eds.). (1986). Albacore Thunnus alalunga pole-and-line fishery around the Emperor Seamounts. Environment and Resources of Seamounts in the North Pacific NOAA Technical Report NMFS. pp. 37–40.
Keywords: critical minerals, ferromanganese crusts, manganese nodules, climate change, ecosystem services, human uses, ocean conservation
Citation: González Ortiz AA, Robbins CS, Morris JA Jr., Cooley SR, Davies J and Leonard GH (2023) An initial spatial conflict analysis for potential deep-sea mining of marine minerals in U.S. Federal Waters. Front. Mar. Sci. 10:1213424. doi: 10.3389/fmars.2023.1213424
Received: 27 April 2023; Accepted: 10 July 2023;
Published: 09 August 2023.
Edited by:
Carolyn J. Lundquist, National Institute of Water and Atmospheric Research (NIWA), New ZealandReviewed by:
Damianos Chatzievangelou, Institute of Marine Sciences (CSIC), SpainCopyright © 2023 González Ortiz, Robbins, Morris, Cooley, Davies and Leonard. This is an open-access article distributed under the terms of the Creative Commons Attribution License (CC BY). The use, distribution or reproduction in other forums is permitted, provided the original author(s) and the copyright owner(s) are credited and that the original publication in this journal is cited, in accordance with accepted academic practice. No use, distribution or reproduction is permitted which does not comply with these terms.
*Correspondence: Christopher S. Robbins, Y3JvYmJpbnNAb2NlYW5jb25zZXJ2YW5jeS5vcmc=
†These authors have contributed equally to this work and share first authorship
Disclaimer: All claims expressed in this article are solely those of the authors and do not necessarily represent those of their affiliated organizations, or those of the publisher, the editors and the reviewers. Any product that may be evaluated in this article or claim that may be made by its manufacturer is not guaranteed or endorsed by the publisher.
Research integrity at Frontiers
Learn more about the work of our research integrity team to safeguard the quality of each article we publish.