- 1National Research Council (CNR), Roma, Italy
- 2IRBIM, Istituto per le Risorse Biologiche e le Biotecnologie Marine, Ancona, Italy
- 3National Institute of Oceanography and Experimental Geophysics (Italy), Trieste, Italy
- 4Commonwealth Scientific and Industrial Research Organisation (CSIRO), Canberra, ACT, Australia
- 5CSIRO Oceans and Atmosphere, Horbart, TAS, Australia
- 6Department of Mathematics and Statistics, Dalhousie University, Halifax, NS, Canada
- 7Leibniz Centre for Tropical Marine Research (LG), Bremen, Germany
Editorial on the Research Topic
Data-limited research in stock assessment to increase the understanding of fisheries resources and inform and improve management efforts
Management thinker Peter Drucker is often quoted as saying “You can’t manage what you can’t measure.” Drucker means that you cannot know whether or not you are successful unless success is defined and monitored. Such a quote is fully applicable to fishery science because only when we can estimate the status of stocks can we provide meaningful and successful management advice: that which gets measured gets managed. However, an increasing share of fishers’ income is derived from fish from stocks whose status remains unassessed. In such situations, a simple rough model might be more useful than no model at all.
The main reasons for the lack of assessment and associated formal harvest control rules are often associated to:
– lack of (quality) data to reliably inform a fully integrated stock assessment.
– limited capacity and funding.
– associated fishery characteristics, including inconsistent targeting practices, numerous unregulated operators, or profound cultural issues.
– the challenge of selecting from numerous possibilities and the most appropriate assessment and management options given the fishery’s context.
However, many methods have been developed to assist in the assessment of the status of so-called data-limited stocks. Although not based on complex integrated models increasingly used in stock assessments, data-limited assessment methods, particularly when paired with precautionary harvest control rules, provide a reliable understanding of the stock status and might be used to achieve fishery sustainability.
A brief search on the Scopus database (www.scopus.com) highlighted approximately 360 documents produced between 1993 and 2023 pertaining to this area of research (TITLE-ABS-KEY [(“data-limited” OR “data poor”) AND “stock assessment”)]. The bibliographic analysis showed an exponential increase with time, especially for “data-limited” approaches (Figure 1). These studies regarded mainly northern hemisphere countries (Figure 2).
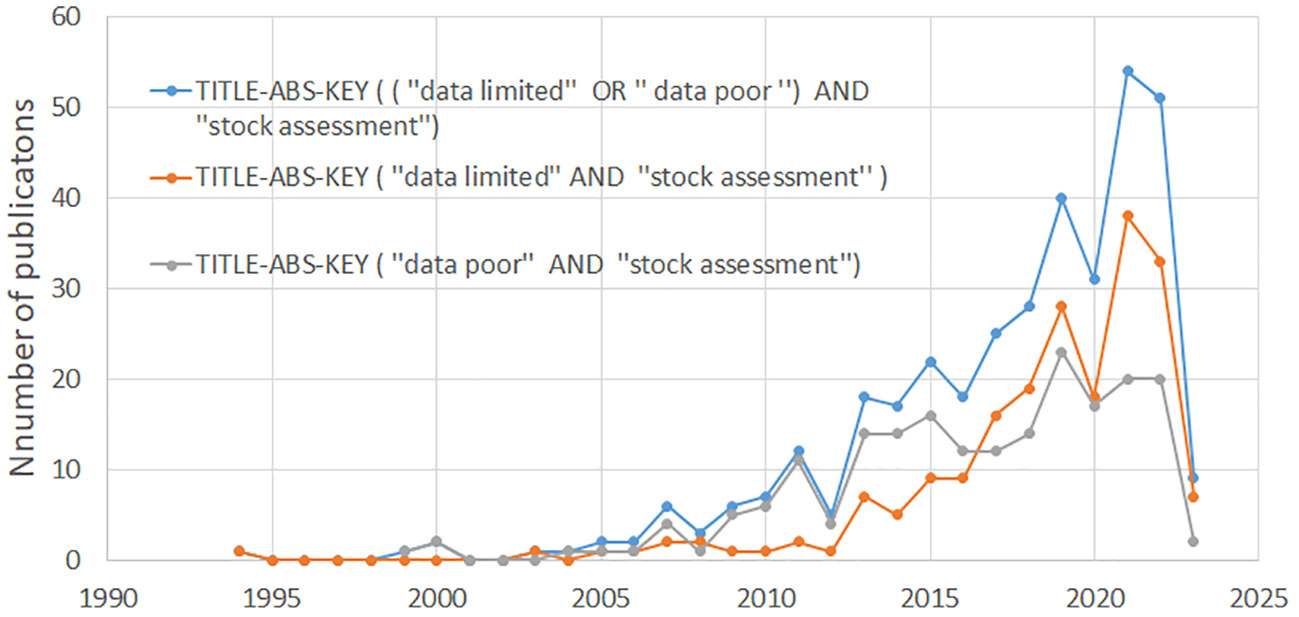
Figure 1 Number of publications by year relevant to this research topic. Source: www.scopus.com.
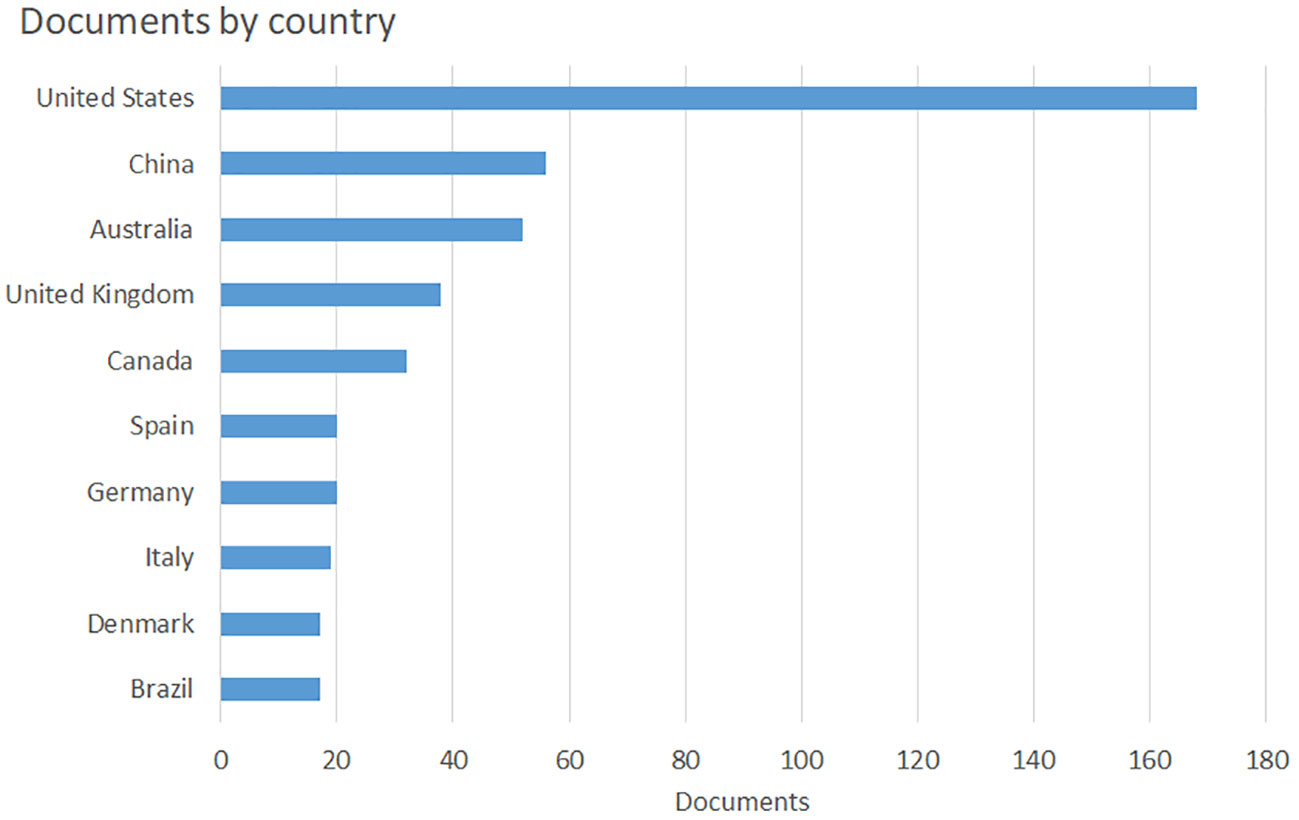
Figure 2 Number of publications by country relevant to this research topic (only countries with more than 15 documents are presented). Source: www.scopus.com.
The RT included 22 papers from various countries (two from the US, five from Med, and eight from China). The works of the RT are distributed mainly around several topics:
The first application of the data-limited approach to new species (e.g., Angelini et al., 2021; Falsone et al., 2021; Geraci et al., 2021; Shi et al., 2022; Tsikliras et al., 2021; Wang et al., 2021; Wang et al., 2022; Zhang et al., 2021; Zhang et al., 2022; Zhu et al., 2021).
The application of several data-limited approaches for comparison to the same species (e.g., Meissa et al., 2021; Simard et al., 2021; Zheng et al., 2022).
The development of the application of complex approaches adapted for data-limited situations (e.g., Harford et al., 2021; Mannini et al., 2020; Omori et al., 2021; Rudd et al., 2021; Sá́nchez-Maroño et al., 2021).
The assessment and forecasting approaches for data-limited species (e.g., Armelloni et al., 2021; Pantazi et al., 2020).
how data-limited stocks can undermine a formal management process (e.g., Kell et al., 2022).
The formal management harvest control rules for data-limited fisheries (e.g., Sanchez-Marono et al., 2021; Xia et al., 2021).
From the analysis of the keywords used in the 22 published manuscripts, the heterogeneity in covered topics is evident. However, the most used methodologies within the data-limited paradigm are production models (cited in 16 manuscripts) and length-based approaches (cited in six manuscripts).
Overall, this Research Topic provided a ground for discussing the potential of data-poor methods to be applied in fishery assessments as well as limitations on their use. Moreover, the studies covered a management perspective with a clear objective of resource conservation, sustainable exploitation, economic viability, and a combination of these and other aims. Although many of the data-poor studies in the present RT concentrate on the assessment of the status of biological resources, the overall conclusion is that the proper management of data-limited fisheries has specific research needs to be developed in the following years. These would focus on the application of artificial intelligence in stock assessment methodologies and the implementation of data collection programs dedicated to the understanding of specific parameters (e.g., carrying capacity). Such needs have also to take into account the state of the art depicted in the 22 scientific studies collected under this RT.
Author contributions
All authors listed have made a substantial, direct, and intellectual contribution to the work and approved it for publication.
Conflict of interest
The authors declare that the research was conducted in the absence of any commercial or financial relationships that could be construed as a potential conflict of interest.
Publisher’s note
All claims expressed in this article are solely those of the authors and do not necessarily represent those of their affiliated organizations, or those of the publisher, the editors and the reviewers. Any product that may be evaluated in this article, or claim that may be made by its manufacturer, is not guaranteed or endorsed by the publisher.
References
Angelini S., Armelloni E. N., Costantini I., De Felice A., Isajlović I., Leonori I., et al. (2021). Understanding the dynamics of ancillary pelagic species in the Adriatic Sea. Front. Mar. Sci. 8. 728948. doi: 10.3389/fmars.2021.728948
Armelloni E. N., Scanu M., Masnadi F., Coro G., Angelini S., Scarcella G. (2021). Data Poor Approach for the assessment of the main target species of rapido trawl fishery in adriatic sea. Front. Mar. Sci. 8, 552076. doi: 10.3389/fmars.2021.552076
Falsone F., Scannella D., Geraci M. L., Gancitano V., Vitale S., Fiorentino F. (2021). How fishery collapses: the case of lepidopus caudatus (Pisces: trichiuridae) in the strait of Sicily (Central Mediterranean). Front. Mar. Sci. 7, 584601. doi: 10.3389/fmars.2020.584601
Geraci M. L., Falsone F., Gancitano V., Scannella D., Fiorentino F., Vitale S. (2021). Assessing cephalopods fisheries in the strait of Sicily by using poor data modeling. Front. Mar. Sci. 8, 584657. doi: 10.3389/fmars.2021.584657
Harford W. J., Amoroso R., Bell R. J., Caillaux M., Cope J. M., Dougherty D., et al. (2021). Multi-indicator harvest strategies for data-limited fisheries: a practitioner guide to learning and design. Front. Mar. Sci. 8, 757877. doi: 10.3389/fmars.2021.757877
Kell L. T., Sharma R., Winker H. (2022). Artefact and artifice: Evaluation of the skill of catch-only methods for classifying stock status. Front. Mar. Sci. 9, 762203. doi: 10.3389/fmars.2022.762203
Mannini A., Pinto C., Konrad C., Vasilakopoulos P., Winker H. (2020). “The elephant in the room”: exploring natural mortality uncertainty in statistical catch at age models. Front. Mar. Sci. 7, 585654. doi: 10.3389/fmars.2020.585654
Meissa B., Dia M., Baye B. C., Bouzouma M., Beibou E., Roa-Ureta R. H. (2021). A comparison of three data-poor stock assessment methods for the pink spiny lobster fishery in Mauritania. Front. Mar. Sci. 8, 714250. doi: 10.3389/fmars.2021.714250
Omori K. L., Tribuzio C. A., Babcock E. A., Hoenig J. M. (2021). Methods for identifying species complexes using a novel suite of multivariate approaches and multiple data sources: a case study with gulf of Alaska rockfish. Front. Mar. Sci. 8, 663375. doi: 10.3389/fmars.2021.663375
Pantazi V., Mannini A., Vasilakopoulos P., Kapiris K., Megalofonou P., Kalogirou S. (2020). That’s all I know: inferring the status of extremely data-limited stocks. Front. Mar. Sci. 7, 583148. doi: 10.3389/fmars.2020.583148
Rudd M. B., Cope J. M., Wetzel C. R., Hastie J. (2021). Catch and length models in the stock synthesis framework: Expanded application to data-moderate stocks. Front. Mar. Sci. 8, 663554. doi: 10.3389/fmars.2021.663554
Sánchez-Maroño S., Uriarte A., Ibaibarriaga L., Citores L. (2021). Adapting simple index-based catch rules for data-limited stocks to short-lived fish stocks’ characteristics. Front. Mar. Sci. 8, 662942. doi: 10.3389/fmars.2021.662942
Shi Y., Zhang X., He Y., Fan W., Tang F. (2022). Stock assessment using length-based Bayesian evaluation method for three small pelagic species in the Northwest pacific ocean. Front. Mar. Sci. 9, 775180. doi: 10.3389/fmars.2022.775180
Simard N. S. M., Militz T. A., Kinch J., Southgate P. C. (2021). From past to present: construction of a dataset documenting mother-of-Pearl exports from a pacific island nation, Papua new Guinea. Front. Mar. Sci. 8, 762610. doi: 10.3389/fmars.2021.762610
Tsikliras A. C., Touloumis K., Pardalou A., Adamidou A., Keramidas I., Orfanidis G. A., et al. (2021). Status and exploitation of 74 un-assessed demersal fish and invertebrate stocks in the Aegean Sea (Greece) using abundance and resilience. Front. Mar. Sci. 7, 578601. doi: 10.3389/fmars.2020.578601
Wang L., Lin L., Liu Y., Zhai L., Ye S. (2022). Fishery dynamics, status, and rebuilding based on catch-only data in coastal waters of China. Front. Mar. Sci. 8, 757503. doi: 10.3389/fmars.2021.757503
Wang Y. C., Liang C., Xian W., Wang Y. B. (2021). Using the LBB method for the assessments of seven fish stocks from the Yangtze estuary and its adjacent waters. Front. Mar. Sci. 8, 679299. doi: 10.3389/fmars.2021.679299
Xia M., Carruthers T., Kindong R., Dai L., Geng Z., Dai X., et al. (2021). How can information contribute to management? value of information (VOI) analysis on Indian ocean striped marlin (Kajikia audax). Front. Mar. Sci. 8, 646174. doi: 10.3389/fmars.2021.646174
Zhang K., Li J., Hou G., Huang Z., Shi D., Chen Z., et al. (2021). Length-based assessment of fish stocks in a data-poor, jointly exploited (China and Vietnam) fishing ground, northern south China Sea. Front. Mar. Sci. 8, 718052. doi: 10.3389/fmars.2021.718052
Zhang Z., Wang Y., Liu S., Liang C., Xian W. (2022). Assessing the distribution and sustainable exploitation of lophius litulon in marine areas off Shandong, China. Front. Mar. Sci. 9, 759591. doi: 10.3389/fmars.2022.759591
Zheng L., Wang Y., Liu S., Liang C., Xian W. (2022). Using data-limited methods to assess the status of bartail flathead platycephalus indicus stocks in the bohai and yellow seas. Front. Mar. Sci. 8, 759465. doi: 10.3389/fmars.2021.759465
Keywords: stock assessment, data limited, fishery management, data poor approach, harvest control rule
Citation: Scarcella G, Libralato S, Dowling NA, Flemming JM and Wolff M (2023) Editorial: Data-limited research in stock assessment to increase the understanding of fisheries resources and inform and improve management efforts. Front. Mar. Sci. 10:1193307. doi: 10.3389/fmars.2023.1193307
Received: 24 March 2023; Accepted: 07 April 2023;
Published: 20 April 2023.
Edited and Reviewed by:
Stelios Katsanevakis, University of the Aegean, GreeceCopyright © 2023 Scarcella, Libralato, Dowling, Flemming and Wolff. This is an open-access article distributed under the terms of the Creative Commons Attribution License (CC BY). The use, distribution or reproduction in other forums is permitted, provided the original author(s) and the copyright owner(s) are credited and that the original publication in this journal is cited, in accordance with accepted academic practice. No use, distribution or reproduction is permitted which does not comply with these terms.
*Correspondence: Giuseppe Scarcella, Z2l1c2VwcGUuc2NhcmNlbGxhQGNuci5pdA==