- 1Earth Institute & School of Biology and Environmental Science, University College Dublin, Dublin, Ireland
- 2School of Civil and Environmental Engineering, University of New South Wales, Sydney, NSW, Australia
- 3Department of Biology, University of Pisa, Pisa, Italy
- 4Department of Biological Sciences, University of Alberta, Edmonton, AB, Canada
Understanding the difficult to predict interactive effects of anthropogenic stressors is recognized as one of the major challenges facing environmental scientists and ecosystem managers. Despite burgeoning research, predicting stressor interactions is still difficult, in part because the same two stressors can interact, or not, depending on their intensities. While laboratory experiments have provided useful insights about how organisms respond to serial doses of single stressors, we lack ‘response-surface’ field experiments in which naturally occurring assemblages are exposed to multiple types and concentrations of stressors. Here we used a field-based dosing system combined with a ‘response-surface’ design to test the individual and combined effects of two stressors (copper and chlorpyrifos) at five concentrations of each, for a total of 25 replicated treatments (n=4). After six weeks of dosing, chemical uptake and impacts at several levels of biological organization in mussel assemblages were measured. Stressor combinations produced interactive effects that would not have been revealed without using this replicated ‘response-surface approach’. Results show that non-additive effects of multiple stressors may be more complex and more common than previously thought. Additionally, our findings suggest that interactive effects of multiple stressors vary across levels of organization which has implications for monitoring and managing the chemical, biological and ecological impacts of priority pollutants in the real world.
Introduction
Set against a backdrop of growing human-populations, most habitats are affected by more than one anthropogenic pressure (Halpern et al., 2008; Barnosky et al., 2011; Bennett et al., 2015; Halpern et al., 2015; Tilman et al., 2017) and are rarely subject to the actions of single stressors/disturbances (Folt et al., 1999; Crain et al., 2008). Research to understand the effects of multiple stressors on biological and ecological processes has been increasing in recent years (Underwood, 1980; Vinebrooke et al., 2004; Blake and Duffy, 2010; Darling et al., 2013; MacDougall et al., 2013; Côté et al., 2016; Galic et al., 2018; Mayer-Pinto et al., 2020; Jackson et al., 2021). At its most simple, multiple stressors can cause chemical, biological and ecological impacts in three ways depending on whether the combined effect of both stressors is smaller (antagonistic interaction), equal (additive) or greater than (synergistic interaction) the sum of the effects of the individual stressors (Folt et al., 1999; Crain et al., 2008; Lyons et al., 2015). Despite this simplicity, there is still debate about the terminology used to describe combined effects, how prolific each non-additive effect is and whether their frequency and magnitude modify their effects (Piggott et al., 2015; Côté et al., 2016; Thompson et al., 2018; Orr et al., 2020).
In part, this is because it is difficult to predict the effects of multiple stressors because those effects can vary depending on the intensity of each stressor involved, with some synergistic effects at smaller concentrations and others antagonistic at larger concentrations (Chen et al., 2004; Coors and De Meester, 2008; Townsend et al., 2008). Yet, little of that work has, however, considered more than one or two concentrations/intensities of each stressor with research mainly having been performed in laboratory settings with only 10-20% of studies being undertaken in the field with natural-occurring assemblages (Crain et al., 2008; Mayer-Pinto et al., 2020). In addition, field experiments with the ability to test multiple pairwise comparisons have been rare (Côté et al., 2016) despite calls for their implementation (Strain et al., 2014; Bennett et al., 2015; Lyons et al., 2015; Côté et al., 2016; Orr et al., 2020; Rindi et al., 2022). This is predominantly because the large complex experiments required have been very difficult to run in the field. A recent review on the sub-lethal effects of multiple contaminants on habitat-forming species, revealed ~40% of publications were poorly designed with insufficient replication and/or appropriate controls (Mayer-Pinto et al., 2020). A suitable experimental design for such research is the ‘response-surface’ design (Inouye, 2001; Inouye, 2005; Rosenblatt and Schmitz, 2016). ‘Response-surface’ designs test the relationship between the response and a number of input (predictor) variables across a range of levels (Box and Wilson, 1951; Inouye, 2005; Lin and Peterson, 2006; Varaksin et al., 2018). Response-surface experiments have tended to be done in the laboratory with individual species or simplified assemblages, however, they have been criticized for lacking replicate combinations of treatments (Benedetti-Cecchi, 2005) and environmental relevance (Mayer-Pinto et al., 2010). This makes it difficult to examine how chemical and biological effects on organisms can be translated into ecological effects on assemblages, which are necessary to understand the effects of multiple stressors on the ecosystem (Attrill and Depledge, 1997). Wildlife exposed to multiple stressors, and not examined using appropriate experiments, surveys and modelling can fail to identify and manage declines in populations (Munns, 2006; Browne et al., 2015; Côté et al., 2016; Rosenblatt and Schmitz, 2016). Therefore, if we are to improve legislation and guidelines so they protect habitats and the populations of species they contain, as advocated in the literature recently (Orr et al., 2020; Jackson et al., 2021), appropriately designed manipulative field experiments are needed to test the effects of multiple stressors and their interactions, across a range of intensities/concentrations and across different levels of organization within the ecosystem. Here we present a ‘response-surface’ field with replicated combinations of treatments to (a) test whether the responses of mussels (copper accumulation, cellular viability) and their assemblages (filtration, respiration) interact additively, synergistically, or antagonistically to variations in the concentration of two stressors. We hypothesize that the combined influence of stressors (copper and the biocide chlorpyrifos) will vary depending on their relative concentrations, with maximal combined influences not necessarily being predictable based on individual dose-response curves and (b) understand if individual and combined effects of multiple stressors at a range of concentrations are consistent among chemical, biological, and ecological responses derived from different levels of biological organization.
Materials and methods
Location, duration and nature of experiment
The experiment ran for 6 weeks (19th August to 30th September 2011) on the floating pontoons at Malahide marina. The marina is in Malahide estuary located on the East coast of Ireland (Lat: 53° 27’ 12” Long: 06° 9’ 5), which has a tidal range of 2-4 m and a salinity range of 18 – 34. Here sub-tidal mussel assemblages (Mytilus edulis) attached to pontoons were exposed continuously for 6 weeks to different concentrations of copper (5, 50, 423, 1269 µgL-1) and/or the biocide chlorpyrifos (0.1, 25, 250, 5000 µgL-1) that are known to cause sub-lethal/lethal effects on mussels (see Table S1) using a programmable dosing system (Browne et al., 2016). In addition, replicated control plots that were not exposed to either stressor plots were also established. As such, there was 25 treatments in total (including the controls). Each of these specific concentrations of contaminants were delivered continuously over the duration of the experiment, from one of ten 640 L tanks, to four replicated experimental plots of each treatment via tubing connected to a pump, placed in each tank that was controlled by a digital timer (Browne et al., 2016). To standardize mussel assemblages in experimental plots, a 10 cm wide corer marked to a depth of 15 cm was used to standardize the size of assemblages, only assemblages that were 15 cm in depth were selected and the surrounding assemblage (outside the core) was cleared using a scraper to a distance of 15 cm. Plots were separated by a minimum of 1.5 m, which has previously been shown to ensure independence by preventing cross-contamination of experimental plots (Browne et al., 2016; Brooks and Crowe, 2018; 2019). All treatments were randomly allocated to plots and marked using printed codes fixed to the pontoon.
After dosing plots, rates of respiration and filtration of assemblages were measured and the hemocellular viability and concentrations of copper of three individual mussels from each plot was quantified at the end of the experiment using established techniques (Browne et al., 2016; see below for detailed protocols for each response variable).
Rationale for contaminants
Copper is a trace element used by many organisms to help sustain important physiological functions (Rainbow, 1997). Whilst it occurs naturally in aquatic habitats through geological processes (e.g. weathering, sedimentation), anthropogenic inputs of copper can come from runoff from agriculture, sewage, storm-water and mining (Makepeace et al., 1995; Srinivasan and Swain, 2007). It has also been used for the past two hundred years as the active agent in antifouling paints and there has been resurgence in its use in the last few years due to the banning of tributyl-tin (Lewis, 1998). Copper’s toxicity to invertebrates is well known and has been shown to cause sublethal effects in crabs, mussels and limpets (Brown et al., 2004). Equally copper can reduce recruitment by fouling assemblages (Johnston and Keough, 2000) and the cellular viability and attachment of limpets (Cartwright et al., 2006). Organophosphates such as chlorpyrifos are used globally as biocides in agriculture, aquaculture and marinas to reduce the abundance of pests on crops, artificial structures and fish (Lewis, 1998; Firbank et al., 2008). The smallest concentration of copper (5 µgL-1) and chlorpyrifos (0.1 µgL-1) were chosen to correspond to minimal concentrations allowed in European (Water Framework Directive, 2000/60) and North American aquatic habitats (EPA, 1980). The moderate concentrations of copper (50 and 423 µgL-1) and chlorpyrifos (25 and 250 µgL-1) used have been deemed to be sub-lethal, but environmentally realistic (Valkirs et al., 2003; Roberts et al., 2006; McKenzie et al., 2011; McKenzie et al., 2012). The highest concentration of copper (1269 µgL-1) and chlorpyrifos (5000 µgL-1) are at the larger range of values recorded in the environment (Makepeace et al., 1995; Huang et al., 2020) and within the range expected to produce biological impacts (Guzzella et al., 1997; Kim et al., 2004; Huang et al., 2020).
Protocols for responses
Cellular viability
The day after final dosing, three mussels were collected from each plot for analysis of cellular viability using established methods (Cartwright et al., 2006; Browne et al., 2008; Moreira et al., 2013). Briefly, hemolymph was withdrawn from the adductor muscle of each mussel using a syringe and a 50 μL aliquot was pipetted into each of two wells of a 96-well microtiter plate and agitated using a plate shaker (1400 rpm, 60 s). The plate was then left for 50 min to allow hemocytes to adhere at the bottom of wells. After the incubatory period, excess cells were discarded and the plates were washed with phosphate buffer solution. Neutral red dye (0.4%) was added and incubated in the dark for a minimum of 3 hours. Wells were then washed with phosphate buffer and an acidified solution (1% acetic acid, 20% ethanol) was added to resolubilize the dye. Dye was then quantified using a spectrophotometer at 550 nm absorbance and hemocytes quantified using a protein assay to quantify total protein in the hemolymph (Bradford, 1976) and enabled hemocellular viability to be quantified as optical density per gram of protein from replicate mussels.
Copper in tissue
Three mussels removed from each plot were left in (Instant Ocean prepared with 18MΩ cm conductivity water with < 0.015 ppm Cu) seawater for 24 hr so that they evacuated sediment from their gut. Mussels were then placed into pre-cleaned 100 mL polyethylene bags, labelled and frozen. All glassware and plastic containers were cleaned in a phosphate and soap-free degreasing detergent (10% solution of Decon 90), then rinsed in water (18 MΩ cm conductivity), followed by nitric acid (10% Aristar grade by VWR) bath for 24 hr using 18MΩ cm water and rinsed again in 18 MΩ cm water. Mussels were then dried in an environment free from particles then stored in bags until required. The frozen mussels were freeze-dried (Labconco, Freezone 6) in their storage containers for 3 days. The length, width and height of the shell-valves were measured to the nearest mm using callipers. Mussels were then shucked by removing the mussel from its shell and the mass of the freeze-dried mussel measured (Scalehouse, ALD114CM) and recorded. Each freeze-dried mussel was then transferred to a polyethylene container for digestion (20-25°C, 4 days) with concentrated nitric acid (10 mL) (Sigma-Aldrich). Samples were then transferred to boiling tubes in a heater (Skalar, Tecator 1016 Digester Heated Block) for digestion (100 °C, 48 hr). Finally, the acidic solutions containing digested mussels were then transferred to pre-cleaned polyethylene screw-top 100 mL bottles (Linnux, UK) and diluted to 10 mL with 18 MΩ cm water for storage prior to analysis. Concentrations of copper in the solutions containing the digested mussel were determined by Inductively-Coupled Mass Spectrometry (X Series 2 ICP-MS, Thermo Fisher Scientific, Hemel Hempstead, U.K.). This instrument was operated in ‘collision/reaction cell mode’, with 7% H2 in He as the collision/reaction gas, to negate the effect of polyatomic interferences, e.g. 23Na, 40Ar and 25Mg, 40Ar on 63Cu and 65Cu respectively. All digested mussels were diluted through series dilution a hundred-fold prior to analysis to give a final concentration of 10 mgL-1 in the diluted digests. Indium and Iridium were used as internal standards to account for instrumental drift. All analyses were done using ISO 9001:2008 protocols.
Respiration by assemblages
Respiration was measured using temporal changes in the concentration of dissolved oxygen (mgO2 L-1) in the dark by placing an opaque chamber over the assemblage (see Figure S1), using a hand-held probe (HQ20 Hach Lange Ltd portable LDO™, Loveland, USA). To ensure that measurements were taken at the correct time points, a linearity test was used to test how long it took the volume of water to be depleted of oxygen. Aqueous concentrations of oxygen were measured every two minutes for an hour to identify the period during which there was a linear decrease of oxygen in the water. On the basis of this test, measures of oxygen were taken after 10 and 20 minutes. The initial 10 minutes has been shown to also allow for acclimatization of the assemblages and to ensure photosynthesis had ceased after covering with an opaque chamber (Noël et al., 2010). The chamber was also fitted with a pump to circulate the water and help homogenize concentrations of oxygen, so measures were representative. Rates of oxygen uptake by the assemblages were estimated using the equation; R = Δ [O2] dark/Δt dark, where R is Respiration, Δ [O2] dark is the difference in concentration of dissolved oxygen between measures at the beginning and end of the dark period and Δt dark is the time difference between these measurements. R was derived on a per hour basis for each individual plot and expressed as mgO2 L-1hr-1 (Nielsen, 2001; Noël et al., 2010).
Filtration by assemblages
Rates at which assemblages cleared algal cells from the water was measured in-situ using purpose-built chambers (see Figure S1). Here 5 mL of an algal solution (Isocrysis galbana) was injected into the chamber to give a concentration of algal cells of 12 -15,000 per 0.5 mL-1 in the chamber. To ensure that algae remained suspended in solution, the chamber was fitted with a circulatory pump. After introduction of algae, 20 ml samples of seawater from within the chamber were taken at three time intervals: 0, 15 and 30 minutes respectively and stored in cool, dark conditions in the laboratory until analysis. The numbers of particles retained in samples were counted in the laboratory using flow-cytometry and filtration-rates calculated as the change in concentration through time using the equation; Filtration = V (loge C1 − loge C2)/t, where V is the volume of water in the chamber and C1 and C2 are the algal concentration at the beginning and end of the timed intervals (t) (Coughlan, 1969; Canty et al., 2007; Browne et al., 2008).
Statistical analysis
Data for all four response variables were assessed individually using generalized additive models (GAMs; Hastie and Tibshirani, 1990; Wood, 2006) within the mgcv package in R v.3.03 (R Development Core Team, 2014). After examining the data and refining the model, the predictor variables, concentrations of copper and biocide concentration were log transformed (log+1 for copper and log+0.01 for biocide) to create equidistance between the ranges of concentrations and reduce overestimation between data in the model (Wood, 2006). The optimum degree of smoothing of the model was defined by minimizing the generalized cross validation (GCV) scores of the smoothing parameters (i.e., knots and splines), which reduced over-fitting of the model to the data (Gu and Wahba, 1991; Wood, 2006). Additionally, to assess interactive effects between stressors (predictor variables), a tensor product was also included in the model. The data for the four response variables were assessed using graphical diagnostics for normality, homogeneity and model fit. One replicate was removed from the cellular viability dataset. Its estimate (1.34 optical density g -1 hemocyte-protein) was larger in comparison to all other data, so we concluded that an error had been made when recording the data. After validating the model, response-surfaces of the GAMs for each of the four response variables were plotted to visualize and assess the relationships between both stressors.
Results
Cellular viability
The hemocellular viability of mussels decreased with log-copper concentration in the single stressor plots (Figure 1; Table 1A). Concomitantly, mussels exposed to an increase in concentration of the biocide chlorpyrifos saw an asymptotic decrease in cellular viability (Figure 1; Table 1A). It appears, however, there was an interactive effect across the response-surface, when copper and chlorpyrifos where combined, such that an increasingly strong antagonism developed as the concentration of both stressors increased: at larger concentrations, each stressor reversed the effect of the other, although at large concentrations of copper the relationship was hump-shaped. The strength of the antagonistic interaction was strongest at intermediate concentrations of chlorpyrifos and large concentrations of copper, with no apparent visual difference between the cellular viability of mussels at those concentrations and those in control plots that were not exposed to either stressor (Figures 1A, B). Although the model explained a relatively small amount of deviance of the dataset (Table 1A, 17% deviance explained), the model was supported statistically (Table 1A).
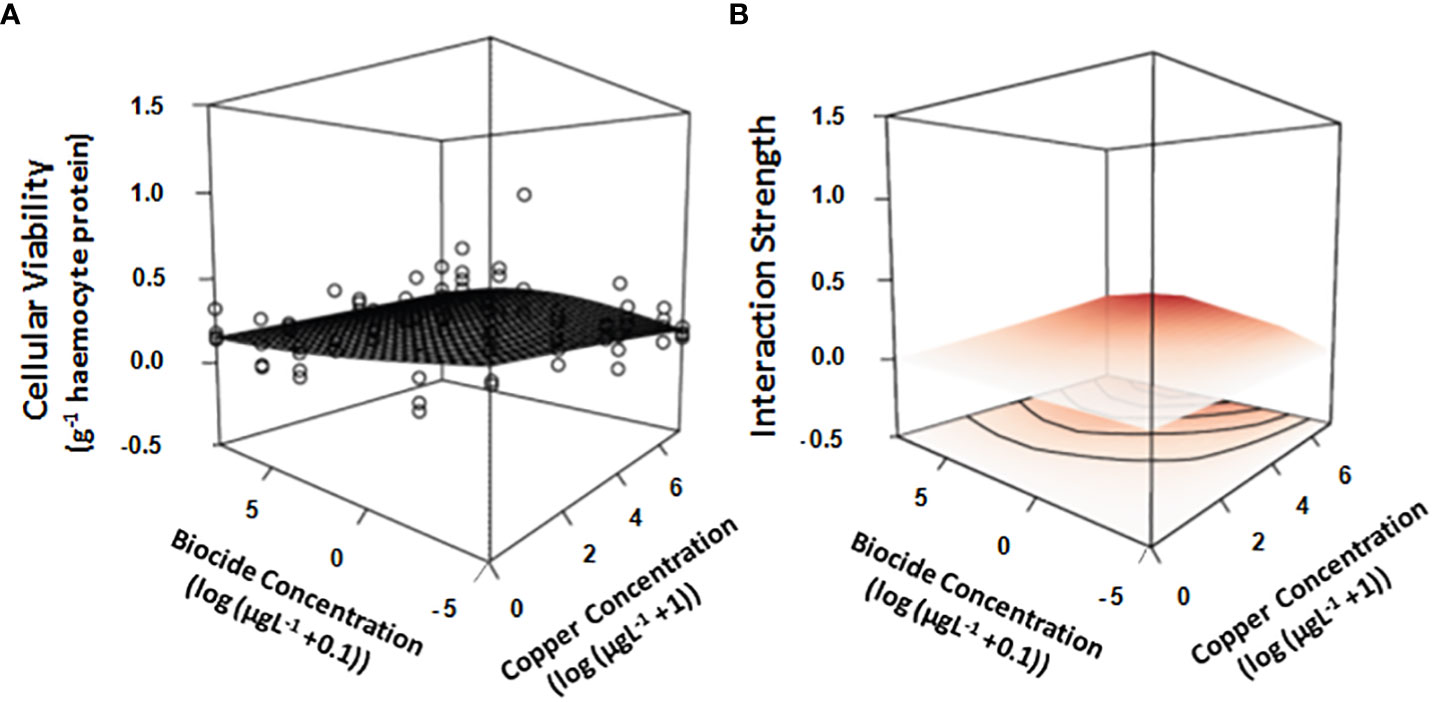
Figure 1 Overlay of the GAM generated response-surface on (A) the real data obtained by measuring the cellular viability (optical density g -1 hemocyte protein) of mussels exposed to increasing concentrations of two stressors (Copper & the biocide chlorpyrifos). Also shown is the interaction strength across the response surface (B), where color is indicative of whether the interaction is either positive (red), negative (blue) or neutral (white). Concentrations of both stressors are log-transformed (log+1 for copper & log+0.01 for the biocide chlorpyrifos). All treatments and controls were replicated (n = 4).
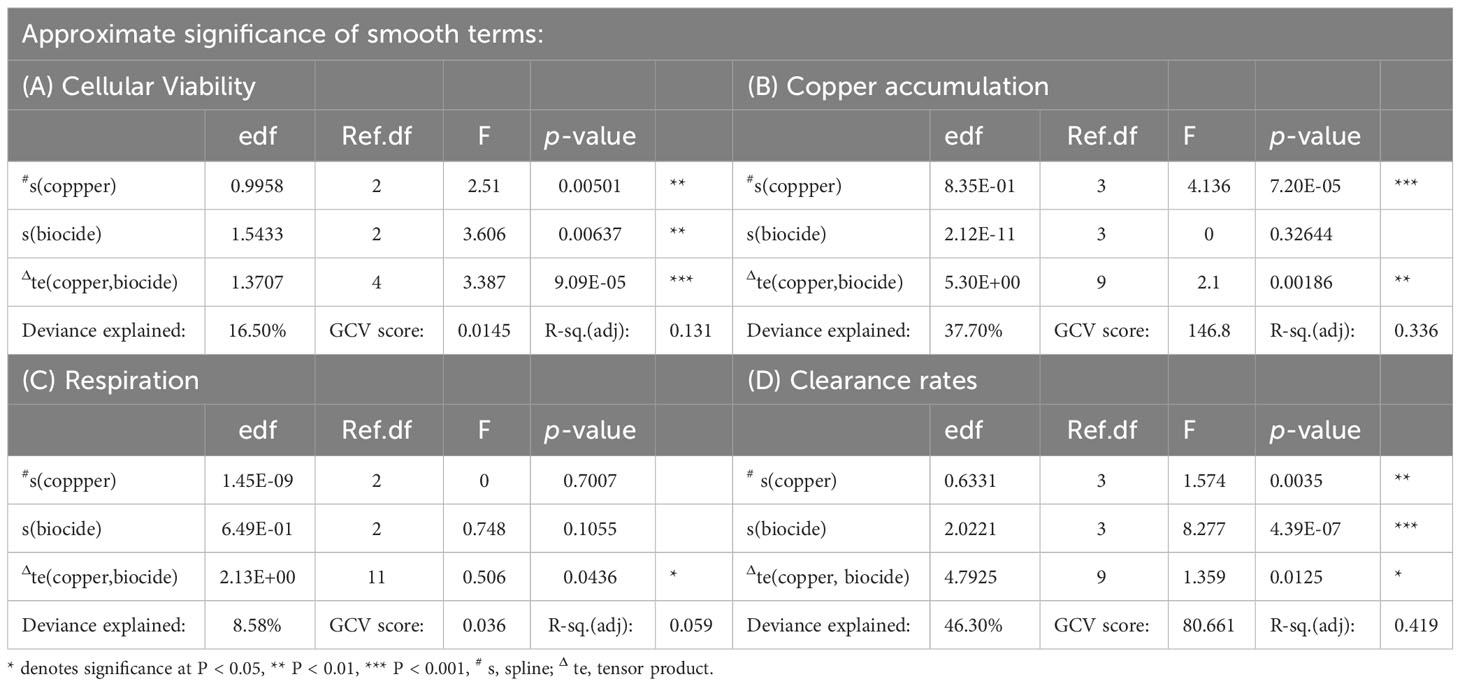
Table 1 Analyses of GAM (Generalized Additive Model) response-surface generated from (A) cell viability data (optical density g -1 hemocyte protein), (B) copper accumulation data (µg g -1 mussel tissue), (C) respiration data (mgO2L-1hr -1) and (D) clearance data (Lhr-1) of mussels/mussel assemblages exposed to increasing concentrations of two stressors (Copper & the biocide chlorpyrifos).
Copper in tissue
Copper in the mussels from plots exposed to only biocide did not differ significantly from those in the controls (Figure 2A; Table 1B). In contrast, there was relatively log-linear increases in copper found in mussels exposed to increasing concentrations of copper (Figure 2A; Table 1B). The monotonic relationship between the concentrations of copper added to mussels and those found in their tissues was unaffected at the highest concentration of biocide, however, at the smallest concentrations of chlorpyrifos and large concentrations of copper, there was a synergistic interaction between the stressors (Figure 2A; Table 1B). The strength of that interaction was strongest in mussels exposed to both small concentrations of chlorpyrifos and large concentrations of copper. Here mussels contained more copper; twice as much as mussels exposed to the highest concentration of copper (Figure 2B; Table 1B). The interactive effects of copper and chlorpyrifos provided a good fit to the data, explaining 38% of the deviance (Figures 2A, B; Table 1B).
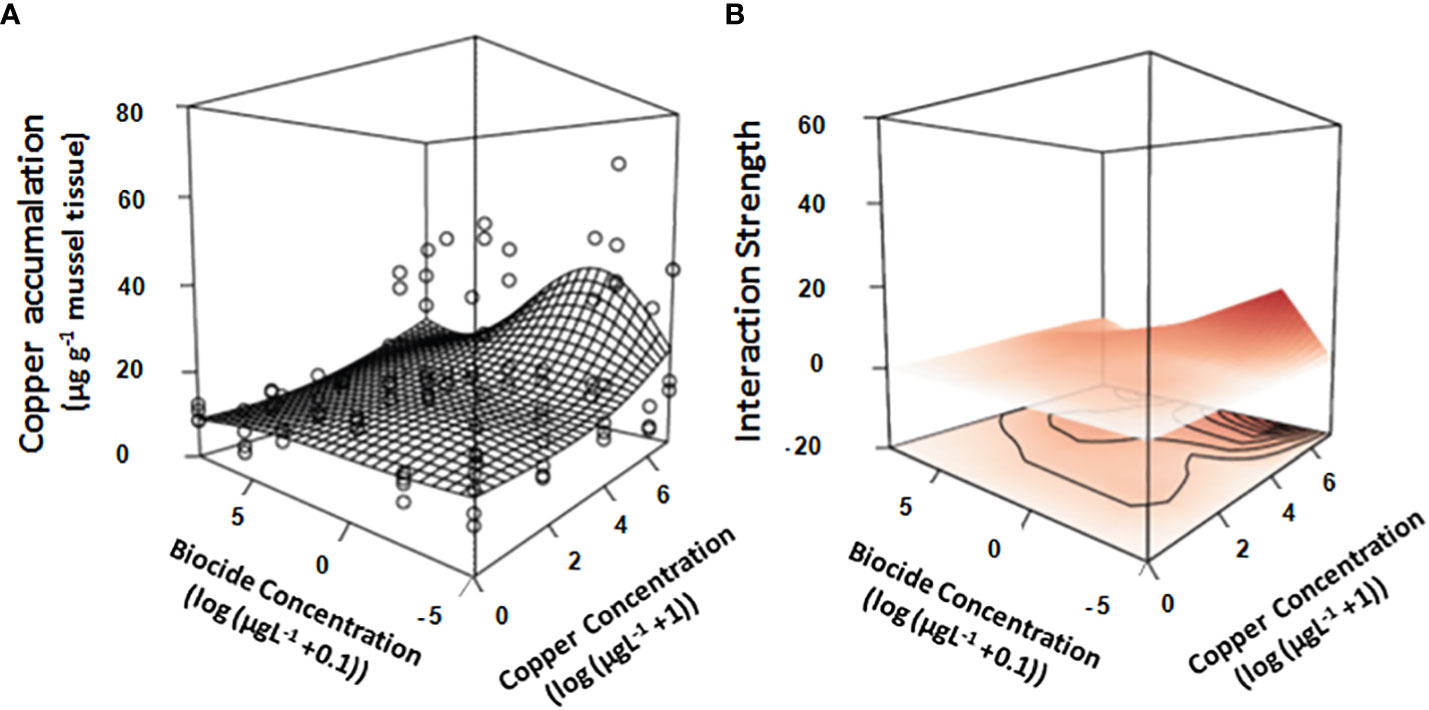
Figure 2 Overlay of the GAM generated response-surface on (A) the real data obtained by measuring the accumulation of copper in mussels (µg g -1 mussel tissue) exposed to increasing concentrations of two stressors (Copper & the biocide chlorpyrifos). Also shown is the interaction strength across the response surface (B), where color is indicative of whether the interaction is either positive (red), negative (blue) or neutral (white). Concentrations of both stressors are log-transformed (log+1 for copper & log+0.01 for the biocide chlorpyrifos). All treatments and controls were replicated (n = 4).
Respiration by assemblages
Respiration by mussel assemblages varied greatly (Figure 3A) with the fitted model explaining 9% of the deviance contained in the data (Table 1C) but there still appeared to be an interactive effect reflected in the model visualization (Figures 3A, B) and in the significance of the tensor product (Table 1C). Greater respiration occurred in assemblages exposed to small concentrations of chlorpyrifos with and without small concentrations of copper, however, the ‘response-surface’ flattened at larger concentrations of copper due to an antagonistic interaction between the two contaminants (Figures 3A, B). On its own, copper had little effect, though our model predicts slightly elevated respiration with increasing concentrations of copper (Figure 3A).
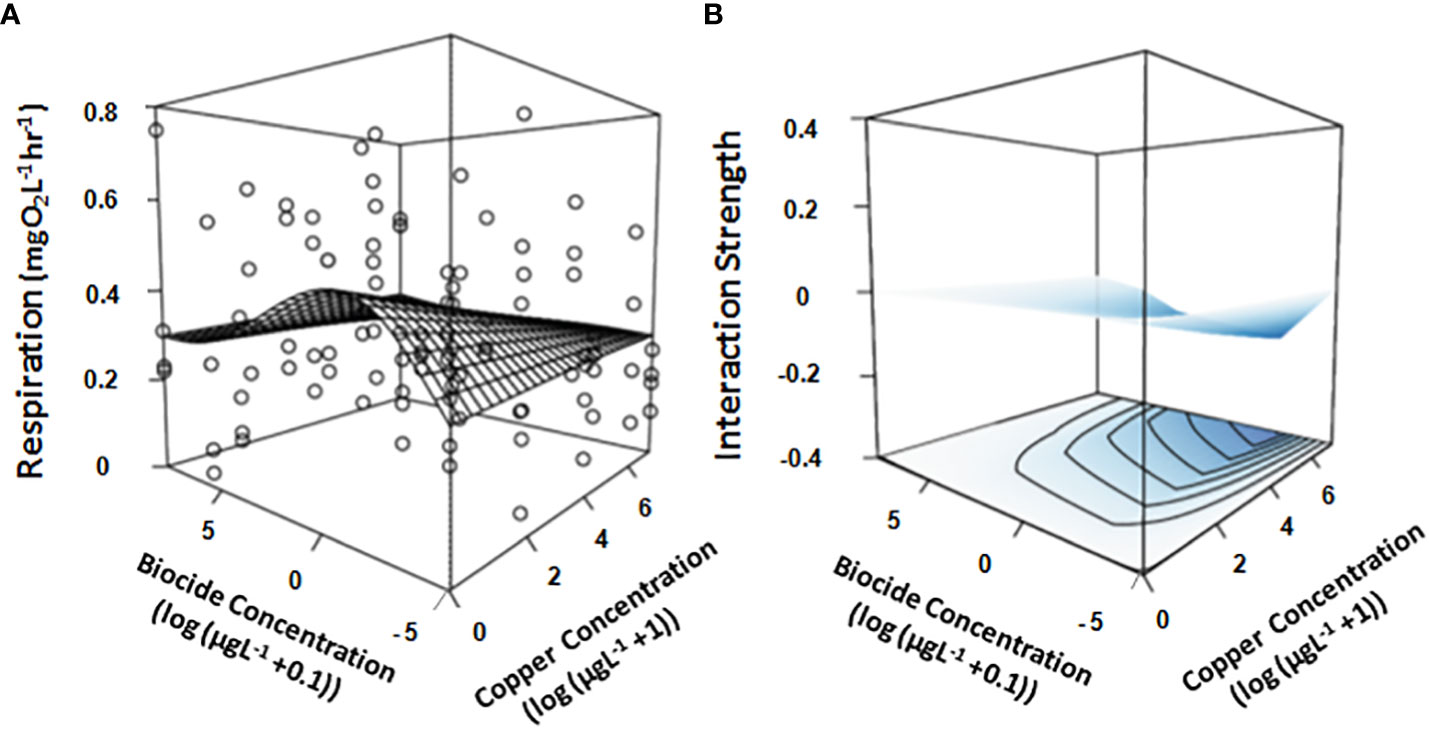
Figure 3 Overlay of the GAM generated response-surface on (A) the real data obtained by measuring the respiration (mgO2L-1hr -1) of mussel assemblages exposed to increasing concentrations of two stressors (Copper & the biocide chlorpyrifos). Also shown is the interaction strength across the response surface (B), where color is indicative of whether the interaction is either positive (red), negative (blue) or neutral (white). Concentrations of both stressors are log-transformed (log+1 for copper & log+0.01 for biocide chlorpyrifos). All treatments and controls were replicated (n = 4).
Filtration by assemblages
In the absence of copper, filtration by assemblages decreased monotonically with increasing concentrations of biocide (Figure 4A; Table 1D), with assemblages exposed to large concentrations filtering 70% less compared to controls. In contrast, there was a non-monotonic (U-shaped) relationship between filtering by assemblages and the log-transformed concentration of copper without chlorpyrifos (Figure 4A). At weak concentrations of copper there was an almost log-linear decrease in filtering by mussel assemblages, however, that effect was greatest (i.e. asymptotic) at intermediate concentrations (Figure 4B). At large concentrations there was only a small difference between filtering by assemblages at the large concentrations of copper and mussel assemblages in control plots (Figures 4A, B, Table 1). At the highest concentration of copper, a synergistic interaction with chlorpyrifos caused larger reductions to filtering than assemblages exposed to no copper (Figures 4A, B, Table 1D). There was clear evidence of interactions between the effects of copper and chlorpyrifos, with the two stressors appearing to synergistically enhance and antagonistically diminish filtering by assemblages depending upon their concentration (Figures 4A, B, Table 1D), which is reflected in terms of the deviance explained (46%) and is supported statistically (Table 1D).
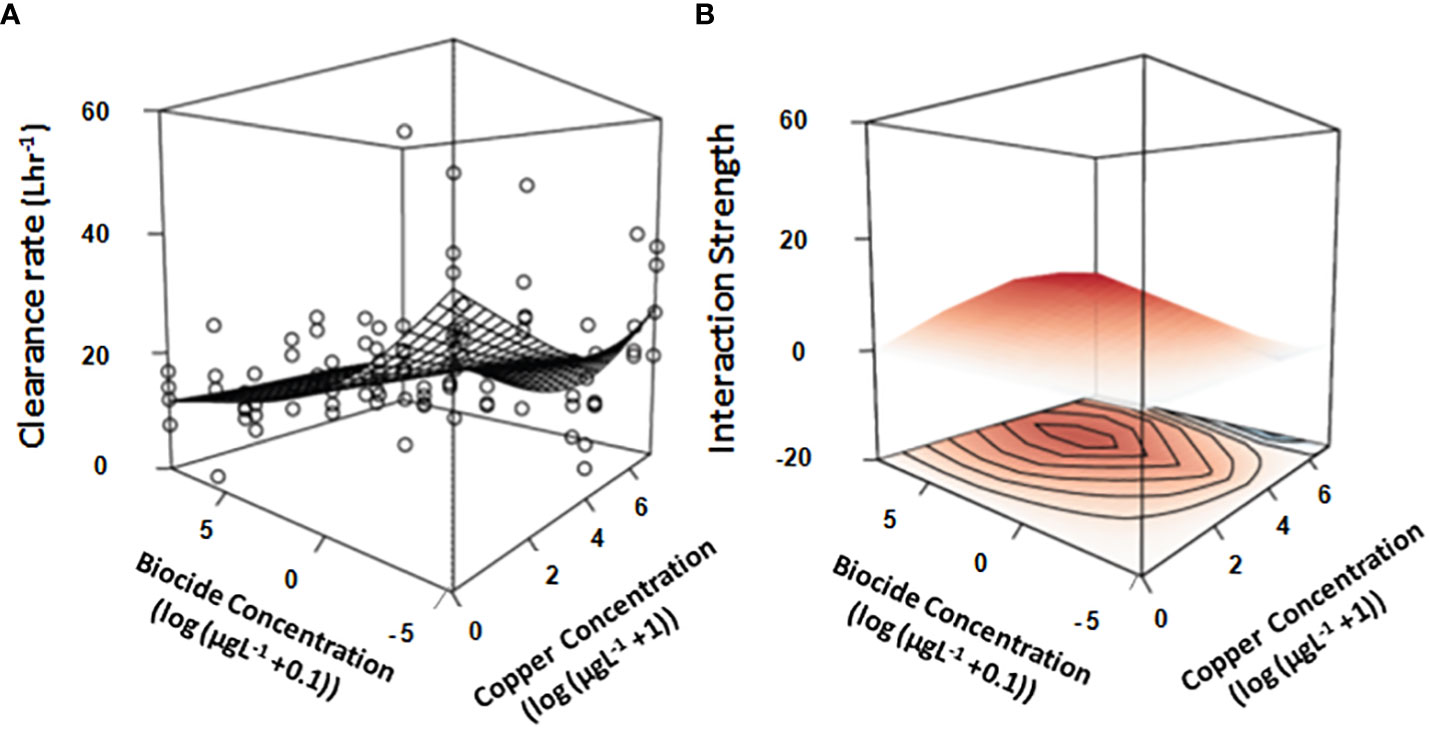
Figure 4 Overlay of the GAM generated response-surface on (A) the real data obtained by measuring the clearance rate (Lhr-1) of mussel assemblages exposed to increasing concentrations of two stressors (Copper & the biocide chlorpyrifos). Also shown is the interaction strength across the response surface (B), where color is indicative of whether the interaction is either positive (red), negative (blue) or neutral (white). Concentrations of both stressors are log-transformed (log+1 for copper & log+0.01 for biocide chlorpyrifos). All treatments and controls were replicated (n = 4).
Discussion
Predicting the combined effects of stressors based on knowledge of their individual effects is fraught with uncertainty; particularly when more recent knowledge suggests that non-additive effects may outweigh additive effects (Strain et al., 2014; Piggott et al., 2015; Côté et al., 2016). Using a ‘response-surface’ field experiment this study reveals a prevalence of non-additive effects between stressors that varied depending on their concentration, and on the responses of the mussels and assemblages measured. For the cellular level response variable, there were antagonistic interactions between increasing concentrations of both stressors that peaked at intermediate concentrations of chlorpyrifos and the highest concentration of copper such that the cellular viability of mussels at those concentrations were similar to the controls (Figures 1A, B). In terms of copper in the tissue of mussels, there was a synergistic interaction in which mussels exposed to large concentrations of copper and intermediate concentrations of chlorpyrifos accumulated significantly more copper than mussels exposed to the highest concentration of copper only (Figures 2A, B). Additionally for filtration, there was evidence of two non-additive effects with filtering by mussel assemblages exposed from intermediate to larger concentrations of chlorpyrifos across the gradient of copper concentrations being reduced to the same extent as the single stressor with the greatest effect; in this instance the intermediate to highest concentrations of chlorpyrifos (Figures 4A, B). This particular type of non-additive effect is suggestive of one that is predicted under the “simple comparative/dominance model” where a single stressor dominates the other stressor in determining their combined effect (Folt et al., 1999; Côté et al., 2016). Additionally, there was a synergistic interaction between small to intermediate concentrations of chlorpyrifos and the highest concentration of the copper, which reduced filtering by assemblages when compared to the controls (Figures 4A, B). In terms of respiration by assemblages, there was a significant interaction between copper and chlorpyrifos (Table 1C; Figures 3A, B), however, given the small amount of deviance explained for the model (9%) and considering that p-values in a GAM are only approximate, caution must be taken in the interpretation.
One possible explanation for the antagonistic interaction between stressors occurring for cellular viability of mussels could be due to exogenous (direct) interactions between the stressors in the environment (i.e. in the water-column before entering the organism) (Lyons et al., 2015). These types of chemical interactions are thought to occur in aquatic environments between these contaminants, where chlorpyrifos may chelate copper-ions (Meikle and Youngson, 1978). Others, however, believe that copper’s speciation is not only indicative of its toxicity but also indicative of its bioavailability (Hall and Anderson, 1999). Although some more benign species of copper can still be assimilated into the tissues of bivalves through feeding or respiration, this is thought to be at much smaller concentrations than species of copper that are thought to be more toxic (Pan and Wang, 2009). As such, if there was an exogenous interaction between the stressors, one would have expected to observe a reduced uptake of both stressors when combined. While we were unable to quantify chlorpyrifos in the tissues of mussels, there was a synergistic interaction between intermediate chlorpyrifos-concentrations and increasing copper-concentrations, which resulted in mussels at those mixtures accumulating more copper in their tissue than mussels exposed to the highest concentration of copper alone (Figure 2A).
In fact, Dondero et al. (2011) reported similar antagonistic interactions between increasing concentrations of this chlorpyrifos and another metal (nickel) on cellular responses in mussels; however, the authors found no evidence of exogenous chemical reactions. Instead, the authors found that the antagonism was due to the stress-response of the species. They used molecular techniques to identify whether there were more genes associated with metals (metallothioneins). This latter finding may be caused by an endogenous antagonistic interaction between the contaminants, where an organism responds to different contaminants through a general response (i.e. inflammation; Browne et al., 2015) that may include the rapid synthesis of proteins (e.g. enzymes, hormones) which may detoxify contaminants (Lyons et al., 2015). This may lend some weight to an argument that an endogenous (indirect) interaction occurred in our experiment, in that copper was present at larger concentrations in mussels exposed to intermediate levels of chlorpyrifos than it was in all other stressor treatments (Figure 2). Indeed, the potential for exogenous and endogenous interactions has been shown for other habitats and other stressors and those interactions also vary between being synergistic or antagonistic. An example of evidence of a synergistic exogenous interaction is one where the toxicity of certain polycyclic aromatic hydrocarbons is known to be enhanced on exposure to sunlight; in particular ultraviolet radiation (Oris and Giesy, 1985). Furthermore, the enhanced toxicity of polycyclic aromatic hydrocarbons has been shown to cause lethal effects to organisms at concentrations an order of magnitude smaller than those that are toxic without ultraviolet radiation (Ankley et al., 2010).
In contrast, examples of antagonistic exogenous interactions can occur with a range of metals in water where the toxicity and bioavailability of the metal are thought to depend on abiotic factors such as pH, temperature, salinity, “minerality-hardness” and concentrations of organic matter (Kiaune and Singhasemanon, 2011).
Such endogenous interactions can be even more complex because they may depend on biochemical and physiological processes in an organism (Lyons et al., 2015). For a range of aquatic species exposed to combinations of increased temperature and different chemical stressors there have been a mix of antagonistic or synergistic interactions. In particular, the direction of the interaction was dependent on not only the identity of the chemical stressor and the intensity of warming, but also the ability of either stressor type to rapidly activate a stress response; for example, the synthesis of “heat shock” proteins, which have also been shown to play a role in reducing toxicity of chemical stressors (reviewed in Holmstrup et al., 2010). Additionally, increased temperature combined with a non-ionic organic compound antagonistically effected freshwater sponges (Müller et al., 1995), but copper and warmer temperatures interacted synergistically in zebra mussels (Rao and Khan, 2000).
The interactive effect of copper and chlorpyrifos on the viability of hemocytes was not observed with filtration (Figure 4A). In fact, for filtering by assemblages our analysis indicates there were two non-additive interaction effects; a synergistic interaction as predicted under the additive model (Folt et al., 1999) and an indication that an interaction as predicted under the simple “comparative/dominance model” occurred (Folt et al., 1999; Côté et al., 2016). Firstly, there was a synergistic interaction at small to intermediate concentrations of chlorpyrifos and large concentrations of copper which reduced the filtering rate of assemblages compared to the controls that were not exposed to either stressor. Secondly, at larger concentrations of chlorpyrifos the addition of copper (across all concentrations) did little to change the effect of the chlorpyrifos, which in this instance determined the effect across the ‘response-surface’ (Figure 4). Indeed, one possible explanation for the synergistic effect of stressors on the filtering by assemblages in our experiment, could be related to the synergistic increase in copper accumulating in mussels at these concentrations (Figure 2). As explained above the mode of action for both these contaminants can be different, yet despite those differences the individual effects can combine to overwhelm the organisms at particular concentrations of both stressors and hence reveal synergistic effects or ‘surprises’ (Paine et al., 1998) at higher levels of biological organization. Previous experiments have shown that when mussels are exposed to large doses of chemical stressors, they can close their valves (Kramer et al., 1989), which may explain the increased copper in mussels exposed to small rather than larger concentrations of chlorpyrifos. Chlorpyrifos can be more toxic at smaller doses than copper (Serrano et al., 1997). Subsequently the effects of chlorpyrifos may reduce the organism’s ability to detect larger doses of copper and hence may ingest and store more copper when exposed to copper combined with small to intermediate doses of chlorpyrifos (Figure 2), which is then translated to greater rates of filtering by assemblages.
Beds of bivalves are some of the most important and dominant habitat-forming species in aquatic systems, as they provide living space and favorable conditions for other species, which facilitates greater biodiversity (Norling and Kautsky, 2007). Mussel beds have diverse assemblages (O’Connor and Crowe, 2007), and their filtering can play a major role in assimilating and recycling sediment, nutrients and energy from the water-column (Newell, 2004). They also provide an important source of food for scavengers/predators (e.g. crabs, starfish, birds, humans) and underpin a vast array of both aquatic and terrestrial food webs (Paine, 1966; Norling and Kautsky, 2007). As such, the negative effects of stressors as described in the current experiment could have serious implications for the functions of mussels beds. In fact, loss of dominant species due to multiple stressors from higher trophic levels is predicted to have a greater impact than loss of a species from other trophic levels and may change the productivity and other useful functions of organisms and assemblages (Hughes and Connell, 1999; Coors and De Meester, 2008; Kendrick et al., 2019).
In this experiment we found possible evidence of a simple “comparative/dominance model” interaction between the stressors tested (Folt et al., 1999). In these types of interactions, the interaction is dominated by the effect of a single stressor (Folt et al., 1999). In these interactions it would appear that at sufficiently large doses/concentrations of one stressor it makes no difference to add the other stressor. Whilst this sort of outcome is valuable to determine managerial approaches for two chemical stressors, as given this result a manager or policymaker could try to remove the worst stressor from the ecosystem. If, however, the more harmful stressor is one that would be expected to occur under climatic change such as increased temperature, acidification, etc., it may not be possible to modify its effects (Harley et al., 2006; Russell et al., 2009; Cheng et al., 2015). These sorts of scenarios could be examined using a ‘response-surface’ design and provide useful information to managers and policymakers who wish to avoid harmful multiple stressor scenarios, something that has previously been called for in the literature (Kroeker et al., 2017; Varaksin et al., 2018), but remains rare (but see Rindi et al., 2022 for experimental approaches on biofilms) due to the complexity of the task and remains a knowledge-gap (Orr et al., 2020; Jackson et al., 2021).
Conclusion
Our results highlight that non-additive effects may be even more complex and may be even more common than previously thought (Crain et al., 2008; Darling and Côté, 2008; Holmstrup et al., 2010), particularly given that those interactions (or lack thereof) can vary substantially across different response variables and across varying concentrations of the stressors. In the current experiment, if we had used a binary or two factor by two level experimental design, we may have reached erroneous conclusions in terms of the existence, strength and direction of the interactions between stressors. While our results show the value of using ‘response-surface’ designs to examine the effects of multiple stressors across a range of intensities, these designs are limited by the number of stressors and organismal responses that can be investigated. So to ensure causal inferences gained from response-surface experimental designs are environmentally relevant will require that the stressors and responses (biological and ecological) chosen are based on those found to correlate in environmental surveys. Using linked surveys and experiments in this way is crucial to understand cause-effect relationships between stressors and biota is crucial to restoring, rehabilitating and preserving biota and their habitats (Underwood, 1980; Underwood and Peterson, 1988; Burton and Johnston, 2010).
The variation in the chemical, biological and ecological outcomes we observed with mussel beds exposed to different concentrations of copper and/or chlorpyrifos across levels of biological organization has important implications for monitoring and managing the impacts and risks of priority pollutants to the ecosystem. As such depending upon the assay used there is a possibility of concluding there was no effect, when in fact based on our findings there was a substantial effect. This potential for ambiguity across those levels of organization further highlights the need for more careful selection of assays and experiments to determine the nature, magnitude and mechanism of impacts of multiple contaminants and other stressors to the ecosystem (Underwood, 1980; Underwood and Peterson, 1988; Adams, 2005; Martínez-Crego et al., 2010; Browne et al., 2015). That said, this ambiguity in the response variables may be driven in part by experimental procedures used to measure respiration and filtration as we cannot be completely certain that the chambers used were sealed fully against the pontoon-surface. Equally that ambiguity may to some extent be explained by differences in the mechanism and timing of toxicity (i.e. adverse-outcome pathway; Kramer et al., 2011; Browne et al., 2015) for the various combinations of contaminants at different levels of biological organization.
There is a growing consensus that many, if not all terrestrial and aquatic habitats are impacted in some way by multiple anthropogenic stressors (Halpern et al., 2015; Lyons et al., 2015). As such there is a pressing need to understand and quantify those effects, if we are to preserve biodiversity and the valuable services they provide (Adger, 2006; Crain et al., 2008; Clements and Rohr, 2009). Experiments, such as the one described here, have the potential to unravel some of the observed complexities surrounding the effects and interactions of multiple stressors. Potential improvements would be to include more response-variables, particularly those at levels of biological organization that may be dampened by inter- and intra-specific interactions (Harley et al., 2006; Crain et al., 2008; Bennett et al., 2015). In addition, experiments incorporating larger temporal and spatial scales could test if the types of interaction vary or are general through time and/or space, or indeed may vary due to the timing/frequency and duration of exposure to the stressors themselves. Understanding how multiple stressors interact to impact the ecosystem remains a challenge but this challenge needs to be met to identify and manage harmful anthropogenic activities so that they have smaller ecological impacts.
Data availability statement
The original contributions presented in the study are included in the article/Supplementary Material. Further inquiries can be directed to the corresponding author.
Author contributions
PB, MB, LB-C and TC conceived the study. PB and MB set up and ran the experiment, while DL assisted PB, MB and others with field-measurements on the final day of the experiment. PB organized the database. PB, LB-C and DL performed the statistical analysis, PB and DL created the figures. TC supervised PB’s PhD of which this study was part of. PB wrote the first draft of the manuscript. All others contributed to manuscript revision and approved the submitted version.
Funding
PB was supported by IRC as part of the UCD Graduate Research Education Program. MB was supported by an IRCSET Fellowship.
Acknowledgments
Sincere thanks to R. Clough and A. Fisher (Plymouth University) for providing access to instruments for the analysis of copper in tissue samples. Thanks to Jen Coughlan, Donal Lennon, and the technical staff at SBES. Many thanks to members of the MarBEE group (Silvia Saloni, Claire Golléty, & Sergio Mascolino) and those from SBES (Bas Boots, Emma Boston, Judith Kochmann, Paul O’Callaghan & Phil Fanning) for assistance in the field. We thank D. Offer and the staff at Malahide Marina for access, storage, and facilities to do this experiment.
Conflict of interest
The authors declare that the research was done with no commercial or financial relationships that could be construed as a potential conflict of interest.
Publisher’s note
All claims expressed in this article are solely those of the authors and do not necessarily represent those of their affiliated organizations, or those of the publisher, the editors and the reviewers. Any product that may be evaluated in this article, or claim that may be made by its manufacturer, is not guaranteed or endorsed by the publisher.
Supplementary material
The Supplementary Material for this article can be found online at: https://www.frontiersin.org/articles/10.3389/fmars.2023.1169677/full#supplementary-material
References
Adams S. M. (2005). Assessing cause and effect of multiple stressors on marine systems. Mar. pollut. Bull. 51 (8-12), 649–657. doi: 10.1016/j.marpolbul.2004.11.040
Adger W. N. (2006). Vulnerability. Global Environ. Change 16 (3), 268–281. doi: 10.1016/j.gloenvcha.2006.02.006
Ankley G. T., Jensen K. M., Kahl M. D., Durhan E. J., Makynen E. A., Cavallin J. E., et al. (2010). Use of chemical mixtures to differentiate mechanisms of endocrine action in a small fish model. Aquat. Toxicol. 99 (3), 389–396. doi: 10.1016/j.aquatox.2010.05.020
Attrill M. J., Depledge M. H. (1997). Community and population indicators of ecosystem health: targeting links between levels of biological organisation. Aquat. Toxicol. 38 (1-3), 183–197. doi: 10.1016/S0166-445X(96)00839-9
Barnosky A. D., Matzke N., Tomiya S., Wogan G. O., Swartz B., Quental. T. B., et al. (2011). Has the Earth’s sixth mass extinction already arrived? Nature 471 (7336), 51–57. doi: 10.1038/nature09678
Benedetti-Cecchi L. (2005). The importance of the variance around the mean effect size of ecological processes: reply. Ecology 86 (1), 265–268. doi: 10.1890/04-1164
Bennett S., Wernberg T., De Bettignies T., Kendrick G. A., Anderson R. J., Bolton J. J., et al. (2015). Canopy interactions and physical stress gradients in subtidal communities. Ecol. Lett. 18 (7), 677–686. doi: 10.1111/ele.12446
Blake R. E., Duffy J. E. (2010). Grazer diversity affects resistance to multiple stressors in an experimental seagrass ecosystem. Oikos 119 (10), 1625–1635. doi: 10.1111/j.1600-0706.2010.18419.x
Box G. E. P., Wilson K. B. (1951). On the experimental attainment of optimum conditions. J. R. Stat. Soc. Ser. B. 13 (1), 1–45. doi: 10.1111/j.2517-6161.1951.tb00067.x
Bradford M. M. (1976). A rapid and sensitive method for the quantitation of microgram quantities of protein utilizing the principle of protein-dye binding. Anal. Biochem. 72 (1-2), 248–254. doi: 10.1006/abio.1976.9999
Brooks P. R., Crowe T. P. (2018). Density and biotic interactions modify the combined effects of global and local stressors. Oikos 127 (12), 1746–1758. doi: 10.1111/oik.04459
Brooks P. R., Crowe T. P. (2019). Combined effects of multiple stressors: New insights into the influence of timing and sequence. Front. Ecol. Evol. 7, 387. doi: 10.3389/fevo.2019.00387
Brown R. J., Galloway T. S., Lowe D., Browne M. A., Dissanayake A., Jones M. B., et al. (2004). Differential sensitivity of three marine invertebrates to copper assessed using multiple biomarkers. Aquat. Toxicol. 66 (3), 267–278. doi: 10.1016/j.aquatox.2003.10.001
Browne M. A., Dissanayake A., Galloway T. S., Lowe D. M., Thompson R. C. (2008). Ingested microscopic plastic translocates to the circulatory system of the mussel, mytilus edulis (L.). Environ. Sci. Technol. 42 (13), 5026–5031. doi: 10.1021/es800249a
Browne M. A., Underwood A. J., Chapman M. G., Williams R., Thompson R. C., van Franeker J. A. (2015). Linking effects of anthropogenic debris to ecological impacts. Proc. R. Soc. B: Biol. Sci. 282 (1807), 20142929. doi: 10.1098/rspb.2014.2929
Browne M. A., Brooks P. R., Clough R., Fisher A. S., Mayer Pinto M., Crowe T. P. (2016). Simulating regimes of chemical disturbance and testing impacts in the ecosystem using a novel programmable dosing system. Methods Ecol. Evol. 7 (5), 609–618. doi: 10.1111/2041-210X.12521
Burton G. A., Johnston E. L. (2010). Assessing contaminated sediments in the context of multiple stressors. Environ. Toxicol. Chem. 29 (12), 2625–2643. doi: 10.1002/etc.332
Canty M. N., Hagger J. A., Moore R. T. B., Cooper L., Galloway T. S. (2007). Sublethal impact of short term exposure to the organophosphate pesticide azamethiphos in the marine mollusc mytilus edulis. Mar. pollut. Bull. 54 (4), 396–402. doi: 10.1016/j.marpolbul.2006.11.013
Cartwright S. R., Coleman R. A., Browne M. A. (2006). Ecologically relevant effects of pulse application of copper on the limpet Patella vulgata. Mar. Ecol. Prog. Ser. 326, 187–194. doi: 10.3354/meps326187
Chen C. Y., Hathaway K. M., Folt C. L. (2004). Multiple stress effects of Vision® herbicide, pH, and food on zooplankton and larval amphibian species from forest wetlands. Environ. Toxicol. Chem.: Int. J. 23 (4), 823–831. doi: 10.1897/03-108
Cheng B. S., Bible J. M., Chang A. L., Ferner M. C., Wasson K., Zabin C. J., et al. (2015). Testing local and global stressor impacts on a coastal foundation species using an ecologically realistic framework. Global Change Biol. 21 (7), 2488–2499. doi: 10.1111/gcb.12895
Clements W. H., Rohr J. R. (2009). Community responses to contaminants: using basic ecological principles to predict ecotoxicological effects. Environ. Toxicol. Chem.: Int. J. 28 (9), 1789–1800. doi: 10.1897/09-140.1
Coors A., De Meester L. (2008). Synergistic, antagonistic and additive effects of multiple stressors: predation threat, parasitism and pesticide exposure in Daphnia magna. J. Appl. Ecol. 45 (6), 1820–1828. doi: 10.1111/j.1365-2664.2008.01566.x
Coughlan J. (1969). The estimation of filtering rate from the clearance of suspensions. Mar. Biol. 2 (4), 356–358.
Côté I. M., Darling E. S., Brown C. J. (2016). Interactions among ecosystem stressors and their importance in conservation. Proc. R. Soc. B: Biol. Sci. 283 (1824), 20152592. doi: 10.1098/rspb.2015.2592
Crain C. M., Kroeker K., Halpern B. S. (2008). Interactive and cumulative effects of multiple human stressors in marine systems. Ecol. Lett. 11 (12), 1304–1315. doi: 10.1111/j.1461-0248.2008.01253.x
Darling E. S., Côté I. M. (2008). Quantifying the evidence for ecological synergies. Ecol. Lett. 11 (12), 1278–1286. doi: 10.1111/j.1461-0248.2008.01243.x
Darling E. S., McClanahan T. R., Côté I. M. (2013). Life histories predict coral community disassembly under multiple stressors. Global Change Biol. 19 (6), 1930–1940. doi: 10.1111/gcb.12191
Dondero F., Banni M., Negri A., Boatti L., Dagnino A., Viarengo A. (2011). Interactions of a pesticide/heavy metal mixture in marine bivalves: a transcriptomic assessment. BMC Genomics 12 (1), 1–18. doi: 10.1186/1471-2164-12-195
Firbank L. G., Petit S., Smart S., Blain A., Fuller R. J. (2008). Assessing the impacts of agricultural intensification on biodiversity: a British perspective. Philos. Trans. R. Soc B 363, 777–787. doi: 10.1098/rstb.2007.2183
Folt C. L., Chen C. Y., Moore M. V., Burnaford J. (1999). Synergism and antagonism among multiple stressors. Limnol. Oceanogr. 44 (3part2), 864–877. doi: 10.4319/lo.1999.44.3_part_2.0864
Galic N., Sullivan L. L., Grimm V., Forbes V. E. (2018). When things don’t add up: quantifying impacts of multiple stressors from individual metabolism to ecosystem processing. Ecol. Lett. 21 (4), 568–577. doi: 10.1111/ele.12923
Gu C., Wahba G. (1991). Minimizing GCV/GML scores with multiple smoothing parameters via the Newton method. SIAM J. Sci. Stat. Computing 12 (2), 383–398. doi: 10.1137/0912021
Guzzella L., Gronda A., Colombo L. (1997). Acute toxicity of organophosphorus insecticides to marine invertebrates. Bull. Environ. contam. Toxicol. 59, 313–320. doi: 10.1007/s001289900481
Hall J. L.W., Anderson R. D. (1999). A deterministic ecological risk assessment for copper in European saltwater environments. Mar. pollut. Bull. 38 (3), 207–218. doi: 10.1016/S0025-326X(98)00164-7
Halpern B. S., Walbridge S., Selkoe K. A., Kappel C. V., Micheli F., d'Agrosa C., et al. (2008). A global map of human impact on marine ecosystems. Science 319 (5865), 948–952. doi:10.1126/science.1149345
Halpern B. S., Frazier M., Potapenko J., Casey K. S., Koenig K., Longo C., et al. (2015). Spatial and temporal changes in cumulative human impacts on the world’s ocean. Nat. Commun. 6 (1), 1–7. doi: 10.1038/ncomms8615
Harley C. D., Randall Hughes A., Hultgren K. M., Miner B. G., Sorte C. J., Thornber C. S., et al. (2006). The impacts of climate change in coastal marine systems. Ecol. Lett. 9 (2), 228–241. doi: 10.1111/j.1461-0248.2005.00871.x
Hastie T., Tibshirani R. (1990). Exploring the nature of covariate effects in the proportional hazards model. Biometrics 46 (4), 1005–1016. doi: 10.2307/2532444
Holmstrup M., Bindesbøl A. M., Oostingh G. J., Duschl A., Scheil V., Köhler H. R., et al. (2010). Interactions between effects of environmental chemicals and natural stressors: a review. Sci. Total Environ. 408 (18), 3746–3762. doi: 10.1016/j.scitotenv.2009.10.067
Huang X., Cui H., Duan W. (2020). Ecotoxicity of chlorpyrifos to aquatic organisms: A review. Ecotoxicol. Environ. Saf. 200, 110731. doi: 10.1016/j.ecoenv.2020.110731
Hughes T. P., Connell J. H. (1999). Multiple stressors on coral reefs: A long-term perspective. Limnol. Oceanogr. 44 (3part2), 932–940. doi: 10.4319/lo.1999.44.3_part_2.0932
Inouye B. D. (2001). Response surface experimental designs for investigating interspecific competition. Ecology 82 (10), 2696–2706. doi: 10.1890/0012-9658(2001)082[2696:RSEDFI]2.0.CO;2
Inouye B. D. (2005). The importance of the variance around the mean effect size of ecological processes: comment. Ecology 86 (1), 262–265. doi: 10.1890/03-3180
Jackson M. C., Pawar S., Woodward G. (2021). The temporal dynamics of multiple stressor effects: from individuals to ecosystems. Trends Ecol. Evol. 36 (5), 402–410. doi: 10.1016/j.tree.2021.01.005
Johnston E. L., Keough M. J. (2000). Field assessment of effects of timing and frequency of copper pulses on settlement of sessile marine invertebrates. Mar. Biol. 137, 1017–1029. doi: 10.1007/s002270000420
Kendrick G. A., Nowicki R. J., Olsen Y. S., Strydom S., Fraser M. W., Sinclair E. A., et al. (2019). A systematic review of how multiple stressors from an extreme event drove ecosystem-wide loss of resilience in an iconic seagrass community. Front. Mar. Sci. 6, 455. doi: 10.3389/fmars.2019.00455
Kiaune L., Singhasemanon N. (2011). Pesticidal copper (I) oxide: environmental fate and aquatic toxicity. Rev. Environ. Contam. Toxicol. 213, 1–26. doi: 10.1007/978-1-4419-9860-6_1
Kim W. S., Yoon S. J., Yang D. B. (2004). Effects of chlorpyrifos on the endogenous rhythm of the manila clam, ruditapes philippinarum (Bivalvia: Veneridae). Mar. Pollut. Bull. 48 (1-2), 182–187. doi: 10.1016/j.marpolbul.2003.09.005
Kramer K. J., Jenner H. A., de Zwart D. (1989). The valve movement response of mussels: a tool in biological monitoring. Hydrobiologia 188 (1), 433–443. doi: 10.1007/BF00027811
Kramer V. J., Etterson M. A., Hecker M., Murphy C. A., Roesijadi G., Spade D. J., et al. (2011). Adverse outcome pathways and ecological risk assessment: Bridging to population-level effects. Environ. Toxicol. Chem. 30 (1), 64–76. doi: 10.1002/etc.375
Kroeker K. J., Kordas R. L., Harley C. D. (2017). Embracing interactions in ocean acidification research: confronting multiple stressor scenarios and context dependence. Biol. Lett. 13 (3), 20160802. doi: 10.1098/rsbl.2016.0802
Lewis J. A. (1998). Marine biofouling and its prevention on underwater surfaces. Mater. Forum 22, 41–61. doi: 10.1051/978-2-7598-2942-2.c003
Lin D. K., Peterson J. J. (2006). Statistical inference for response surface optima. Response Surf. Method. Related Topics World Sci., 65–88. doi: 10.1142/9789812774736_0004
Lyons D. A., Benedetti-Cecchi L., Frid C., Vinebrooke R. (2015). Modifiers of impacts on marine ecosystems: disturbance regimes, multiple stressors and receiving environments. Mar. Ecosys.: Hum. Impacts Biodivers. Function. Serv., 73–110. doi: 10.1017/CBO9781139794763.004
MacDougall A. S., McCann K. S., Gellner G., Turkington R. (2013). Diversity loss with persistent human disturbance increases vulnerability to ecosystem collapse. Nature 494 (7435), 86–89. doi: 10.1038/nature11869
Makepeace D. K., Smith D. W., Stanley S. J. (1995). Urban stormwater quality: Summary of contaminant data. Crit. Rev. Environ. Sci. Technol. 25 (2), 93–139. doi: 10.1080/10643389509388476
Martínez-Crego B., Alcoverro T., Romero J. (2010). Biotic indices for assessing the status of coastal waters: a review of strengths and weaknesses. J. Environ. Monit. 12 (5), 1013–1028. doi: 10.1039/b920937a
Mayer-Pinto M., Underwood A. J., Tolhurst T., Coleman R. A. (2010). Effects of metals on aquatic assemblages: what do we really know? J. Exp. Mar. Biol. Ecol. 391 (1-2), 1–9. doi: 10.1016/j.jembe.2010.06.013
Mayer-Pinto M., Ledet J., Crowe T. P., Johnston E. L. (2020). Sublethal effects of contaminants on marine habitat-forming species: a review and meta-analysis. Biol. Rev. 95 (6), 1554–1573. doi: 10.1111/brv.12630
McKenzie L. A., Brooks R., Johnston E. L. (2011). Heritable pollution tolerance in a marine invader. Environ. Res. 111 (7), 926–932. doi: 10.1016/j.envres.2010.12.007
McKenzie L. A., Brooks R. C., Johnston E. L. (2012). A widespread contaminant enhances invasion success of a marine invader. J. Appl. Ecol. 49 (4), 767–773. doi: 10.1111/j.1365-2664.2012.02158.x
Meikle R. W., Youngson C. R. (1978). The hydrolysis rate of chlorpyrifos, OO-diethylO-(3, 5, 6-trichloro-2-pyridyl) phosphorothioate, and its dimethyl analog, chlorpyrifos-methyl, in dilute aqueous solution. Arch. Environ. Contam. Toxicol. 7 (1), 13–22. doi: 10.1007/BF02332034
Moreira F. T., Browne M. A., Coleman R. A. (2013). Effect of extraction-method, period of inCubation and tidal emersion on the viability of haemocytes from oysters. Mar. pollut. Bull. 74 (1), 208–212. doi: 10.1016/j.marpolbul.2013.06.056
Müller W. E., Koziol C., Dapper J., Kurelec B., Batel R., Rinkevich B. (1995). Combinatory effects of temperature stress and nonionic organic pollutants on stress protein (hsp70) gene expression in the freshwater sponge Ephydatia fluviatilis. Environ. Toxicol. Chem.: Int. J. 14 (7), 1203–1208. doi: 10.1002/etc.5620140712
Munns J. W.R. (2006). Assessing risks to wildlife populations from multiple stressors: overview of the problem and research needs. Ecol. Soc. 11 (1). doi: 10.5751/es-01695-110123
Newell R. I. (2004). Ecosystem influences of natural and cultivated populations of suspension-feeding bivalve molluscs: a review. J. Shellfish Res. 23 (1), 51–62.
Nielsen K. J. (2001). Bottom-up and top-down forces in tide pools: test of a food chain model in an intertidal community. Ecol. Monogr. 71, 187–217. doi: 10.1890/0012-9615(2001)071[0187:BUATDF]2.0.CO;2
Noël L. M.-L., Griffin J. N., Thompson R. C., Hawkins S. J., Burrows M. T., Crowe T. P., et al. (2010). Assessment of a field inCubation method estimating primary productivity in rockpool communities. Estuarine Coast. Shelf Sci. 88, 153–159. doi: 10.1016/j.ecss.2010.03.005
Norling P., Kautsky N. (2007). Structural and functional effects of Mytilus edulis on diversity of associated species and ecosystem functioning. Mar. Ecol. Prog. Ser. 351, 163–175. doi: 10.3354/meps07033
O’Connor N. E., Crowe T. P. (2007). Biodiversity among mussels: separating the influence of sizes of mussels from the ages of patches. J. Mar. Biol. Assoc. United Kingdom 87 (2), 551–557. doi: 10.1017/S0025315407050503
Oris J. T., Giesy J.P. Jr. (1985). The photoenhanced toxicity of anthracene to juvenile sunfish (Lepomis spp.). Aquat. Toxicol. 6 (2), 133–146. doi: 10.1016/0166-445X(85)90012-8
Orr J. A., Vinebrooke R. D., Jackson M. C., Kroeker K. J., Kordas R. L., Mantyka-Pringle C., et al. (2020). Towards a unified study of multiple stressors: divisions and common goals across research disciplines. Proc. R. Soc. B 287 (1926), 20200421. doi: 10.1098/rspb.2020.0421
Paine R. T. (1966). Food web complexity and species diversity. Am. Nat. 100 (910), 65–75. doi: 10.1086/282400
Paine R. T., Tegner M. J., Johnson E. A. (1998). Compounded perturbations yield ecological surprises. Ecosystems 1 (6), 535–545. doi: 10.1007/s100219900049
Pan K., Wang W.-X. (2009). Biodynamics to explain the difference of copper body concentrations in five marine bivalve species. Environ. Sci. Technol. 43 (6), 2137–2143. doi: 10.1021/es802888u
Piggott J. J., Townsend C. R., Matthaei C. D. (2015). Reconceptualizing synergism and antagonism among multiple stressors. Ecol. Evol. 5 (7), 1538–1547. doi: 10.1002/ece3.1465
Rainbow P. S. (1997). Trace metal accumulation in marine invertebrates: marine biology or marine chemistry? J. Mar. Biol. Assoc. United Kingdom 77 (1), 195–210. doi: 10.1017/S0025315400033877
Rao D. P., Khan M. (2000). Zebra mussels: enhancement of copper toxicity by high temperature and its relationship with respiration and metabolism. Water Environ. Res. 72 (2), 175–178. doi: 10.2175/106143000X137257
R Development Core Team (2014). R: A language and environment for statistical computing. (Vienna, Austria: R Foundation for Statistical Computing).
Rindi L., He J., Benedetti-Cecchi L. (2022). Spatial correlation reverses the compound effect of multiple stressors on rocky shore biofilm. Ecol. Evol. 12 (10), e9418. doi: 10.1002/ece3.9418
Roberts D. A., Poore A. G., Johnston. E. L. (2006). Ecological consequences of copper contamination in macroalgae: effects on epifauna and associated herbivores. Environ. Toxicol. Chem. 25, 2470–2479. doi: 10.1897/05-661R.1
Rosenblatt A. E., Schmitz O. J. (2016). Climate change, nutrition, and bottom-up and top-down food web processes. Trends Ecol. Evol. 31 (12), 965–975. doi: 10.1016/j.tree.2016.09.009
Russell B. D., Thompson J. A. I., Falkenberg L. J., Connell S. D.. (2009). Synergistic effects of climate change and local stressors: CO2 and nutrient-driven change in subtidal rocky habitats. Global Change Biol. 15 (9), 2153–2162. doi: 10.1111/j.1365-2486.2009.01886.x
Serrano R., Hernández F., López F. J., Pena J. B. (1997). Bioconcentration and depuration of chlorpyrifos in the marine mollusc mytilus edulis. Arch. Environ. Contam. Toxicol. 33, 47–52.
Srinivasan M., Swain G. W. (2007). Managing the use of copper-based antifouling paints. Environ. Manage. 39, 423–441. doi: 10.1007/s00267-005-0030-8
Strain E. M., Thomson R. J., Micheli F., Mancuso F. P., Airoldi L. (2014). Identifying the interacting roles of stressors in driving the global loss of canopy-forming to mat-forming algae in marine ecosystems. Global Change Biol. 20 (11), 3300–3312. doi: 10.1111/gcb.12619
Thompson P. L., MacLennan M. M., Vinebrooke R. D. (2018). An improved null model for assessing the net effects of multiple stressors on communities. Global Change Biol. 24 (1), 517–525. doi: 10.1111/gcb.13852
Tilman D., Clark M., Williams D. R., Kimmel K., Polasky S., Packer C. (2017). Future threats to biodiversity and pathways to their prevention. Nature 546 (7656), 73–81. doi: 10.1038/nature22900
Townsend C. R., Uhlmann S. S., Matthaei C. D. (2008). Individual and combined responses of stream ecosystems to multiple stressors. J. Appl. Ecol. 45 (6), 1810–1819. doi: 10.1111/j.1365-2664.2008.01548.x
Underwood A. J. (1980). The analysis of stress in natural populations. Biol. Jounral Linean Soc. 37 (1-2), 51–78. doi: 10.1111/j.1095-8312.1989.tb02005.x
Underwood A. J., Peterson C. P. (1988). Towards an ecological framework for investigating pollution. Mar. Ecol. Prog. Ser. 46 (1), 227–234. doi: 10.3354/meps046227
Valkirs A. O., Seligman P. F., Haslbeck E., Caso J. S. (2003). Measurement of copper release rates from antifouling paint under laboratory and in situ conditions: implications for loading estimation to marine water bodies. Mar. pollut. Bull. 46 (6), 763–779. doi: 10.1016/S0025-326X(03)00044-4
Varaksin A. N., Panov V. G., Katsnelson B. A., Minigalieva I. A. (2018). Using various nonlinear response surfaces for mathematical description of the type of combined toxicity. Dose Response 16 (4), 1559325818816596. doi: 10.1177/1559325818816596
Vinebrooke R. D. L., Cottingham K., Norberg Marten Scheffer I. J., Dodson S. C., Maberly S., Sommer U. (2004). Impacts of multiple stressors on biodiversity and ecosystem functioning: The role of species co-tolerance. Oikos 104 (3), 451–457. doi: 10.1111/j.0030-1299.2004.13255.x
Keywords: non-additive effects, antagonistic, synergistic, levels of organization, ecosystem, assemblage, mussels
Citation: Brooks PR, Browne MA, Benedetti-Cecchi L, Lyons DA and Crowe TP (2023) A response-surface approach into the interactive effects of multiple stressors reveals new insights into complex responses. Front. Mar. Sci. 10:1169677. doi: 10.3389/fmars.2023.1169677
Received: 19 February 2023; Accepted: 14 August 2023;
Published: 06 September 2023.
Edited by:
Nafsika Papageorgiou, National and Kapodistrian University of Athens, GreeceReviewed by:
Patrik Kraufvelin, Swedish University of Agricultural Sciences, SwedenJosé Lino Vieira De Oliveira Costa, University of Lisbon, Portugal
Awantha Dissanayake, University of Gilbraltar, Gibraltar
Copyright © 2023 Brooks, Browne, Benedetti-Cecchi, Lyons and Crowe. This is an open-access article distributed under the terms of the Creative Commons Attribution License (CC BY). The use, distribution or reproduction in other forums is permitted, provided the original author(s) and the copyright owner(s) are credited and that the original publication in this journal is cited, in accordance with accepted academic practice. No use, distribution or reproduction is permitted which does not comply with these terms.
*Correspondence: Paul R. Brooks, paul.brooks@ucd.ie