- 1Faculty of Fisheries, Ha Long University, Nam Khe, Uong Bi, Quang Ninh, Vietnam
- 2Faculty of Biology, VNU University of Science, Hanoi, Vietnam
- 3Research Institute for Aquaculture Number 1, Tu Son, Bac Ninh, Vietnam
- 4Institute for Marine and Antarctic Studies, College of Science and Engineering, University of Tasmania, Hobart, TAS, Australia
- 5Centre for Marine Socioecology, University of Tasmania, Hobart, TAS, Australia
- 6Bioinnovation Centre, University of Sunshine Coast, Maroochydore, QLD, Australia
- 7Statistical and ASReml Consultant, Orange, NSW, Australia
- 8NSW Department of Primary Industries, Port Stephens Fisheries Institute, Taylors Beach, NSW, Australia
- 9Biosecurity Unit, Viet Uc Seafood Corporation, Ho Chi Minh City, Vietnam
- 10Aquatic Systems Biology Unit, TUM School of Life Sciences, Technical University of Munich, Freising, Germany
- 11Faculty of Fisheries, Vietnam National University of Agriculture, Trau Quy, Gia Lam, Hanoi, Vietnam
- 12Department of Animal Science, College of Agriculture and Natural Resources, Michigan State University, East Lansing, MI, United States
- 13Institute of Genome Research, Vietnam Academy of Science and Technology, Hanoi, Vietnam
- 14English Department, University of Languages and International Studies, Vietnam National University, Hanoi, Vietnam
Aquaculture mollusc production is predominantly from Asia, with more than 80% of the total biomass produced in China. Vietnam’s annual mollusc production is growing rapidly but is comparatively small given its coastal resources. A significant challenge for future mollusc production, and oysters in particular, is the supply of high-quality spat. Most mollusc spat in Vietnam comes from local wild sources or is imported from China. Particularly in the case of oysters, where wild collection is low and importation of oyster spat into Vietnam from other jurisdictions is not controlled, supply is unreliable and presents a biosecurity risk to the industry. Controlling the life cycle in hatcheries can increase the sustainability and reliability of spat supply with the advantages of genetic enhancement in the long term. Beyond well-established selection methods based on phenotypic data for pedigreed families, the rapid development of genomic technology has enabled innovation in hatchery production based on genetic programs. This technology allows greater insight into oyster genetics in intensive aquaculture production systems. Recent reviews of selection programs demonstrate that inbreeding control via mate selection is an effective strategy for oyster species. Genetic response through combinations of individual and family-based selection can enhance morphometric traits by 10% per generation and disease resistance by 15% per generation in many aquaculture species. Genomic techniques provide information for selecting candidates at an earlier stage and improve prediction accuracy. In this paper, we review the literature on popular genomic tools and breeding techniques used for molluscs, focusing on the Portuguese oyster, Crassostrea angulata. This is to better comprehend how modern quantitative and molecular genetic technologies are being applied in mollusc breeding programs. It considers opportunities for and the feasibility of using genomic-based selection as well as the challenges that are faced in breeding programs transitioning to these new methods.
1 Introduction
The global production of marine bivalves for human consumption is more than 15 million tonnes per year, and represents about 14% of the total marine production in the world (Wijsman et al., 2018). Before 2008, mollusc production in Vietnam was dominated by clams (Meretrix meretrix and M. lyrata) with seed collected from the wild, and small numbers of oyster spat were collected from the wild because of the unavailability of seed production technology. Other mollusc species, including oysters, only accounted for a small proportion of total production (RIA1 annual report, 2012). Over the last decade, there has been remarkable growth in oyster production in Vietnam (Ugalde et al., 2023), despite many difficulties related to limited skill, experience and technical knowledge of hatchery production and grow out of stock, poor natural spat quality, extreme weather such as typhoons, hatchery production failures, disease outbreaks, food poisoning, and water pollution. The historic singular focus on clams and lack of diversification has limited market opportunities and predisposed the mollusc industry to the vulnerabilities that can arise from such dependence. Disruptors such as poor natural spat fall (settlement of larvae onto a suitable substrate), typhoons, hatchery production failures or shortfalls, the accidental introduction or outbreak of significant disease, food poisoning or pollutant scares could cause or already have caused considerable industry and social hardship.
In Asia, there is a wide range of oyster species being cultured, including the Pacific oyster (Crassostrea gigas), the Portuguese oyster (Crassostrea angulata), the Suminoe oyster (C. ariakensis), the Zhe oyster (C. plicatula), and the Iwagaki oyster (C. nippona). Among oyster species being cultured around the world, C. gigas and C. angulata are the most common. These two oyster species are difficult to distinguish using morphological traits (In et al., 2017). They can hybridize and produce fertile progeny (Huvet et al., 2002). Today, China produces 80% of the total production worldwide and about 88% of value in 2016 (Botta et al., 2020), showing a major shift since 1950 when Asia had only contributed 22% of world oyster production (Botta et al., 2020). In Vietnam, the oyster industry is growing rapidly and production of C. angulata had reached 50,000 t in 2019). Now one of the most important oysters being cultured in Asia, C. angulata, has become common to the Pacific coast of Asia (Boudry et al., 1998; Foighil et al., 1998). The species is a popular choice for aquaculture due to fast growth and high survival (Batista, 2007), and was introduced into France in 1868, where production reached 100,000 t (Boudry et al., 1998). However, it almost disappeared in Europe in the early 1970s due to iridovirus outbreaks, which are commonly associated with gill necrosis disease (Boudry et al., 1998; Lapegue et al., 2004). C. angulata production in Europe has fallen to less than 10 t (FAO, 2007). Production of this species in China is estimated to be 21 million t, which is about 36% of the global oyster production (Botta et al., 2020). In Taiwan, C. angulata was the major aquaculture oyster species (Boudry et al., 1998), with production exceeding 20,750 t in 2004 (FAO, 2007). Oyster production in Vietnam has taken 15 years to establish reliable hatchery production and grow out techniques, being the culmination of international partnerships and investment. The industry now needs to focus on improving oyster quality and growout through the production cycle.
In 2002, the Research Institute for Aquaculture No.1 (RIA1) of Vietnam started to develop hatchery production techniques for C. gigas, however, the spat produced did not survive beyond 5 months at growout sites (RIA1 annual report, 2009). To address survival, RIA1 imported broodstock from Taiwan and China and had further hatchery production attempts for C. gigas in 2007 (RIA1 annual report, 2009), but hatchery production was poor due to sub-optimal algal diet, rearing techniques and hatchery equipment. Some spat was deployed to a growout site at Bai Tu Long Bay (Figure 1), but there also, survival to a commercial size was very low (RIA1 annual report, 2009). These attempts were followed by a project that reviewed and improved hatchery methods and transferred hatchery skills and knowledge which led to the successful and reliable production of spat to establish the oyster industry in Vietnam in 2008 (Pierce and O’Connor, 2014). The first commercial batches of 10 million oyster spat were produced in 2008 and more than 22 million spat were generated in the following years at Cat Ba National Broodstock Center (Figure 1), then being delivered to farmers for grow-out. Following this, further investment in oyster research projects by the Vietnamese Government funded a research project aiming to establish hatchery production and growout techniques targeting export (Giang, 2011). Portuguese oyster aquaculture in Vietnam has since grown to a production level of approximately 50,000 tonnes in 2019 and is now the dominant mollusc species farmed in northern Vietnam (In et al., 2017; Vu et al., 2020a; Vu et al., 2021b) where it is well-suited to warm sub-tropical environments of this region.
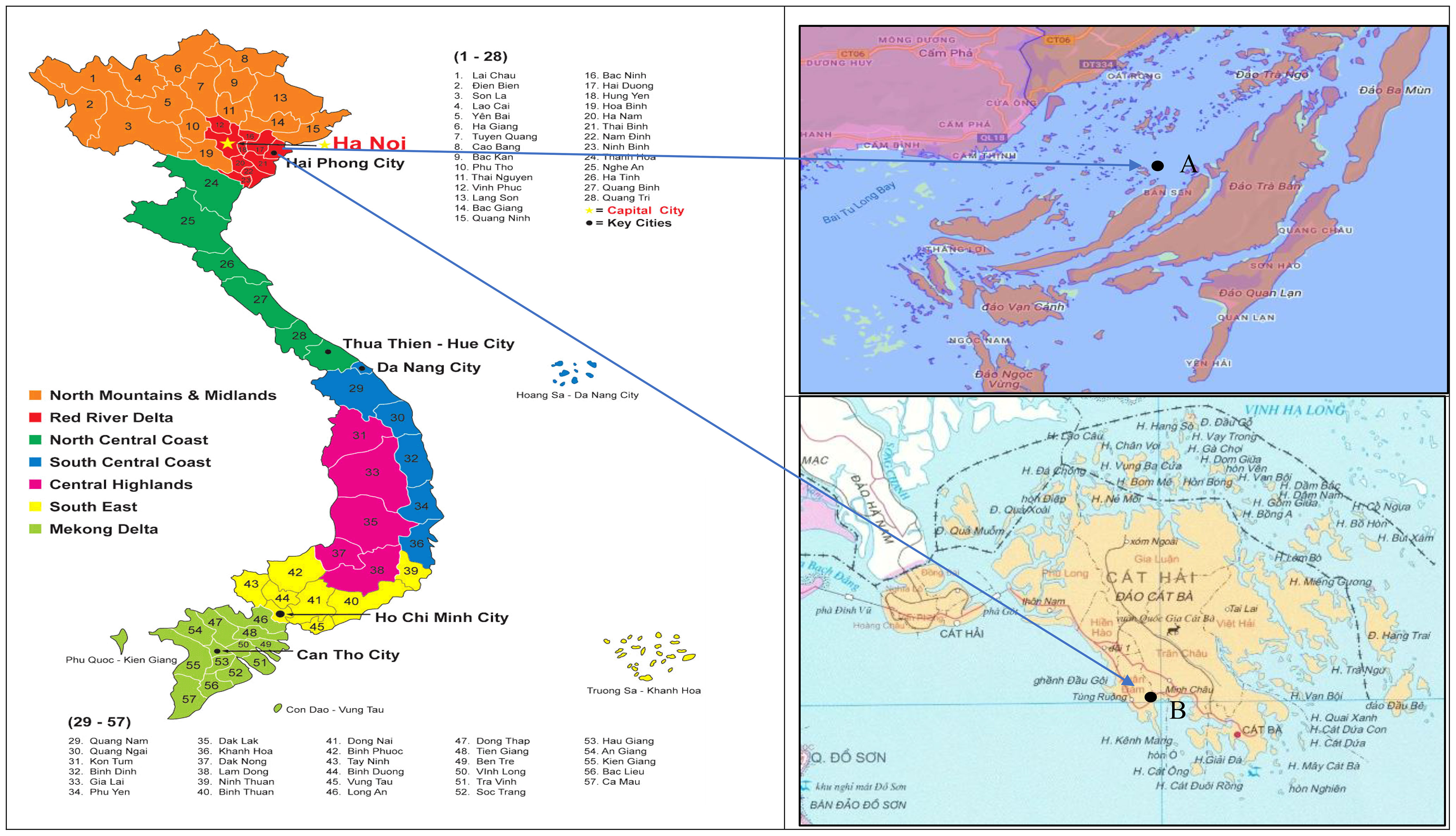
Figure 1 Selective breeding program of the Portuguese oyster (Crassostrea angulata) performed at the Research institute for Aquaculture No.1 (A) Location for grow-out culture of different families of Portuguese oyster at Ban Sen Island-Van Don-Quang Ninh Province. Latitude and longitude (20.958115, 107.454345). (B) Conducting selective breeding program of the Portuguese oyster at the Northern National Broodstock Center for Mariculture (Xuan Dam-Cat Hai-Hai Phong city). Latitude and longitude (20.746211, 106.990529).
The C. angulata industry in Vietnam continues to grow rapidly, but production challenges are emerging. These are mainly related to oyster quality and survival; however, these issues can be addressed through breeding. The breeding techniques chosen need to be cost-effective, at the same time addressing industry and market requirements. This growing industry has enabled farmer diversification creating more jobs, more assets, more protein sources, and improved quality of life for farmers and their families. However, these socio-economic benefits come with challenges (Pierce and O’Connor, 2014).
Most current oyster production relies on cultch set spat from Chinese and Vietnamese hatcheries. Chinese spat is imported because there is insufficient locally produced spat in the peak culturing season from March to June. The essential demands for a successful and sustainable mollusc aquaculture industry are to gain complete control over the entire life cycles of more species, enhancing rearing technology in the hatcheries and improving genetics to provide better quality spat in sufficient numbers for industry. Selective breeding is a feasible option to address many challenges facing mollusc production, particularly around improving survival and oyster quality traits. C. angulata are an important aquaculture species in Vietnam and experience high losses through the growout period. Additionally, many oysters when harvested are of poor quality due to fouling organisms and infestations. Addressing oyster survival and quality will dramatically increase production levels and the overall value of this oyster industry. Focusing on reliable and high-quality spat production within Vietnam will secure and safeguard this industry into the future and reduce biosecurity risks that threaten this industry.
The development of genotyping technology has opened an opportunity to use powerful genetic tools in hatchery-based breeding programs. In recent years, a significant number of full genome sequences have been obtained and annotated in molluscs. However, use of whole genome sequencing is usually expensive and beyond the financial capacity of individual breeders in Vietnam and many other countries where oysters are produced. Cheaper alternatives include reduced-representation genome sequencing techniques such as genotyping-by-sequencing (GBS) (Elshire et al., 2011), RAD sequencing (RAD-sq) (Baird et al., 2008), and DArTseq™ sequencing technology representing a combination of DArT complexity reduction methods and next generation sequencing platforms (Kilian et al., 2012; Courtois et al., 2013).
The principal aim of this review is to provide an overview of molecular and quantitative genetic methods being adopted by oyster industries in different regions of the world. The current state of mollusc genetic programs worldwide is reported to identify lessons for Vietnam to support and continue the recent rapid growth of its oyster industry. In addition, the application of genomic selection will also be reviewed as well as the use of “omic” information in oyster genetic improvement programs in Vietnam.
2 Reproductive biology and genetic diversity in oysters
High fecundity together with a high genetic load results in large variation in productive success among breeders (Hedgecock and Pudovkin, 2011), a situation found in mollusc hatcheries (Lallias et al., 2010). The unequal contribution of broodstock can rapidly decrease the effective population size of breeding populations. This may result in a decrease of genetic diversity due to genetic drift and an increased level of inbreeding (Hollenbeck and Johnston, 2018), as has been observed in other bivalve species with high numbers of offspring such as the freshwater pearl mussel (Geist et al., 2021). Consequently, the response to selection can decrease in the long-term (Meuwissen and Woolliams, 1994). Genetic diversity of broodstock for breeding can be restricted because of selection of just a few outstanding families, or simply due to random sampling of a few families as the species is highly fecund, and only a few breeders may contribute to the majority of the next generation (Taris et al., 2006). Oysters may be particularly vulnerable to loss of genetic diversity and consequent inbreeding when no pedigree is maintained as a single oyster female can release millions of eggs in one spawning event (O’Connor and Dove, 2009). There are several selection methods to enhance traits of interests such as mass selection or family selection (Vu et al., 2020b). Mass selection is able to improve traits of interest quickly. Although effective, mass selection may quickly result in inbreeding if genetic diversity is not properly monitored (Jourdan et al., 2023a). The selection of individuals on the basis of best performance of certain traits without regarding to pedigree information can lead to inbreeding and loss of potentially valuable alleles and additive genetic variation. Bivalve aquaculture is facing inbreeding concerns since fitness is typically reduced at increasing inbreeding coefficients (Charlesworth and Willis, 2009). Inbreeding depression leads to a reduction of growth and an increase in mortality early in life as reported in the European flat oyster (Naciri-Graven et al., 2000), Pacific oyster (Evans et al., 2004), and Sydney rock oyster (In et al., 2016; Vu, 2017). Methods to control inbreeding while securing the rate of genetic gain include optimal selection of contribution (Meuwissen, 1997) and mate selection (Kinghorn, 2011). Mate selection is the avoidance of mating closely related individuals. Poor management of inbreeding in hatcheries is a common cause of the decrease in body traits and fitness (survival rate) of cultured animals. Last but not least, other solutions can be considered in actual selective programs such as importation of wild populations from overseas or using molecular genetics to track the pedigree to mitigate the inbreeding.
3 Current state of selective breeding programs
The objective of breeding programs is to enhance one or more traits of economic importance, like growth rate, survival, shape, yield, shell or mantle colour, temperature tolerance, and disease resistance. A common important commercial trait in mollusc species is growth, especially for the initial phase of a breeding program because the development of fast-growing strains can significantly lower production costs by decreasing the culturing time (Saillant et al., 2009). Any trait can be improved through genetic selection if heritable variation exists for it (Boudry et al., 1997). Beside production traits, survival is considered an essential trait for inclusion in selective breeding programs as survival rates directly increase economic return for aquaculture enterprises (Van Khang et al., 2018). Several studies have shown additive genetic variance components for survival in molluscs (Dégremont et al., 2005; de Melo et al., 2016). Though survival strongly depends on species, weather events and production systems, all these studies demonstrate that a main factor of economic loss across the aquaculture sector is mortality. Mollusc grow-out is almost always conducted in a natural environment that is highly variable being exposed to extreme weather events and disease outbreaks. The variability of stressors (pathogens) makes selective breeding for survival and disease resistance very difficult as new pathogens appear as fast as breeding programs are developed to combat them (Dégremont et al., 2019). Nonetheless, programs have been successfully developed for disease resistance. In the Sydney Rock Oyster (S. glomerata) industry, winter mortality syndrome and QX disease (Marteilia sydneyi) have caused an over 40% decline in production (Heasman et al., 2000). However, the mortality from both diseases has been reduced by selective breeding (O’Connor and Dove, 2009). Since survival in bivalves is largely determined by the genetic adaptability of a stock to local environmental conditions (Denic et al., 2015), morphometric traits and survival should generally be traits included in a selective breeding program. In addition to growth, disease resistance and survival, other potentially important breeding objectives include body condition or meat quality, soft tissue mass and shell shape. Meat processing traits and body composition such as condition index, and soft tissue mass are also important components of commercial profitability as they impact the yield of the commercial product, its quality, and its acceptance by the consumers (price) (Kause et al., 2002; Neira et al., 2004). The lipid composition of the flesh also impacts meat quality, including texture (Andersen et al., 1997), storage characteristics (Lie, 2001), and eventually acceptance of the product by the consumer. The meat condition is also one of the most important criteria to assess the intrinsic response of molluscs to various environments (Sasikumar and Krishnakumar, 2011). Therefore, a new approach should be considered to the conditioning of oysters prior to sale in an environment such as direct transportation of oysters to the consumers/whole salers/retailers. Research could examine and explore the genetic correlation between composition quality scores in different environmental conditions for grow-out to determine if this trait can be reliably improved. The ratio of dry weight: wet weight reflects the percentage of dry matter in the soft tissue. The percentage of water can indicate the energy balance of a tissue (Lucas and Beninger, 1985). The main trait of interest for genetic programs has been growth rate. The other traits such as disease resistance, shape and uniformity, condition index, and soft tissue will increasingly become important (Appleyard and Ward, 2006; Kube et al., 2013). In addition to traits of interest, the estimates of genetic parameters such as heritability, genetic correlation, selection response are the key aspects of breeding programs.
3.1 Heritability
Heritabilities vary with genetic variability, stage of life, and environmental conditions (Gjedrem and Thodesen, 2005; Symonds et al., 2019). Heritability estimates for morphometric, shape, soft tissue, and colour traits have been published in various oyster species including S. glomerata (Dove et al., 2020), C. gigas (Evans et al., 2009; de Melo et al., 2016; Wan et al., 2020), C. virginica (McCarty et al., 2020; Peterson et al., 2020; Allen et al., 2021), O. edulis (Naciri-Graven et al., 1998), Chilean oysters, Ostrea chilensis (Toro and Newkirk, 1991), and C. angulata (Vu et al., 2020a; Vu et al., 2020b). For C. gigas, heritabilities were 0.49; 0.36, 0.45; 0.35 at 12 months of age for shell height, shell length, shell width, wet weight, respectively (Kong et al., 2015); and were 0.41, 0.51, 0.89, 0.40, 0.87, and 0.65 at 16 months of age for glycogen content, total protein content, total fat content, zinc content, and selenium content, respectively (Wan et al., 2020). From the above studies, additive genetic variance for production traits can be a useful indicator for starting a genetic program.
In addition, genetic markers are being developed as an efficient tool to improve predicted accuracy of selection via utilising family variance components, because parentage assignment using genetic markers allows families to be cultured in a common environment, eradicating a potentially confounding source of environmental variation (Vandeputte et al., 2004; Vu et al., 2021a). Genetic markers such as microsatellites or single nucleotide polymorphisms (SNPs) are widely used in determining pedigree relationships among animals from families reared together. Eight to fifteen microsatellites have been used to assign parents with an accuracy of over 99% in fish (Vandeputte and Haffray, 2014). The rate of high null alleles in bivalves adds significantly to the cost of parentage assignment (Hedgecock et al., 2004). In population studies, null alleles may complicate the interpretation of deviations from Hardy-Weinberg equilibrium, and multiple nulls could confuse analyses of population structure (Reece et al., 2004). Null alleles can be treated as recessive alleles for mapping purposes. Another approach uses amplicon sequencing for a moderate number of SNPs with very low amounts of missing data. For instance, 10 microsatellites were enough to give consistent PCR amplification across 74 full-sib families in Australian blue mussel, which were validated from screening 135 microsatellites. Only 62.5% of 2,536 offspring tested were assigned to their true parents. There are pros and cons between a traditional family breeding program where families are kept separated, and molecular marker assisted approaches. Use of parentage assignment requires less infrastructure and labour to culture large numbers of families separately. A disadvantage includes the potential loss of many families due to differential mortality at early life stages (especially in oysters where mortality can be high). Next-generation sequencing (NGS) and genotyping-by-sequencing (GBS) tools have been developed for various oyster species (McCarty et al., 2022a). Nevertheless, they do not illustrate easy transferability of the same set of markers from one population to another (e.g. between training population and breeding population) and are dependent on DNA quality, which limits their potential to develop repeatable genomic analyses. Alternatively, SNP arrays have been developed for the silver-lip pearl oyster, P. maxima or in the Pacific oyster (Qi et al., 2017) or the medium species (Gutierrez et al., 2017a). New techniques using molecular markers could be a significant cost saver for oyster programs, especially in Vietnam, if a SNP chip can be developed that can predict and select candidate oysters to become parents for the subsequent generations. However, an economic analysis and comparison between traditional and genomic selection needs to be made before genomic approaches can be widely used in Vietnam oyster industry. Economic aspects need to be considered for the short- and long- term effects of each selection method.
3.2 Genetic correlations
High genetic correlations result in selection for one trait impacting correlated traits. Knowledge of such associations can be used to enhance traits that are hard to measure or require sacrificing individuals to be measured (Falconer and Mackay, 1996). Selection for improved harvest whole weight in C. angulata can result in improved soft tissue weight; while having little effect on other traits such as shell shape, condition index, shell and mantle colour or disease resistance (Vu et al., 2020a; Vu et al., 2020b). In addition, genetic correlations between yield and both survival (0.38 ± 0.04) and individual weight (0.90 ± 0.01) as well as between survival and individual weight (0.25 ± 0.04) were all positive and medium-to-high, suggesting that indirect gain in yield can be achieved by selection for higher growth and/or survival (de Melo et al., 2016). Other studies in C. gigas indicated that genetic correlations were positive and moderate-to-high among trait groups and shell dimensions (de Melo et al., 2021), ranging from 0.95 to 0.99 between individual average weight and individual weight (de Melo et al., 2019). Favourable genetic correlations were found between growth and hard to measure traits like soft tissue condition in C. gigas (de Melo et al., 2016). However, there are challenges in multiple trait selective programs when selection for the main trait, results in a significantly negative and unfavourable changes in correlated traits. In this circumstance, an index should be developed to combine all advantages from commercial traits of oysters.
3.3 Genotype by environment
The interactions of genotype by environment (G x E) are among the most important problems affecting cultured animal and plant species because they can result in highly different production outcomes for the same strains in different environments (Falconer and Mackay, 1996), and could necessitate separate breeding programs for each production environment (Lynch and Walsh, 1998; Geffroy et al., 2021). Two forms of G × E interaction are scaling and re-ranking (Sheridan, 1997; Nguyen, 2016). Firstly, the scaling effect refers to the differentiation in variance of a trait across environments. This effect is not a serious issue in genetic programs as the correlation between environments will be high. It can be handled by using analytical methods to accommodate the heterogeneous variance and/or data transformation. The second form of interaction is when the genetic correlation is low and re-ranking of the performance of the families occurs over environments (Falconer and Mackay, 1996). The re-ranking effect is more important than the scaling because it may require separate breeding programs for each trait across environments, and that will increase the cost of genetic improvement. Knowledge of how G × E influences potential selection response and estimates of genetic parameters will support the design and optimisation of breeding programs (Mulder et al., 2006; Sae-Lim et al., 2016). Unfortunately, G × E interactions have not been reported for most cultured molluscs. Culture systems for oysters are diverse, for example, bamboo rafts, net strings, and at different locations these may cause G x E effects. Studies of the C. gigas have reported weak or no G × E interactions for growth (Evans and Langdon, 2006) and survival rate (Dégremont et al., 2007), across comparatively limited regions. Similarly, G × E interactions between two testing locations in Vietnam were low for whole weight at harvest and its uniformity in C. angulata (Vu et al., 2021b). By contrast, considerable G × E interactions for both yield and cumulative mortality were found in the Eastern oyster, C. virginica (Proestou et al., 2016). Furthermore, a study was carried out to evaluate G x E interactions at three sites for harvest traits in C. gigas in China, showing that the G x E interactions were moderate for growth traits (0.45 – 0.87), meat yield traits (0.40 – 0.73) and shell shape (0.22 – 0.69); and G x E interactions were found to be weak for harvest survival (0.80 - 0.86) (Chi et al., 2023). In addition, genotype by environment can be used as a tool to mitigate the immediate effects of future environmental changes by altering gene expression and physiology (Sirovy et al., 2021).
3.4 Selection response
The heritability of production traits in molluscs can be higher than in finfish because molluscs have higher fecundity that enables a greater selection intensity (Gjedrem and Robinson, 2014). If a traditional selection approach is used, then the outcome for the generation of families and selection intensity would be the same for fish and bivalves except that it can be easier to generate large numbers of families simultaneously for bivalves since brooders can be easily conditioned in very large numbers due to their small size and limited requirements compared to fish. In bivalves, many studies have measured realised selection response by comparing selected and unselected lines. Most of these studies have reported genetic gain after a mass selection generation. A few studies have encompassed multi-generational mass selection such as in S. glomerata (Nell et al., 1999; O’Connor and Dove, 2009; In et al., 2016), in C. gigas (Langdon et al., 2003; Degremont et al., 2015) or family-based selection in S. glomerata (Rye, 2012; Dove et al., 2020). Other studies combined individual and family-based selection in C. angulata (Vu et al., 2020a; Vu et al., 2021b) and C. gigas (de Melo et al., 2016; de Melo et al., 2018). From the literature, the average selection response per generation for growth has varied from 6.8% for mussels to 12.1% in oysters; meanwhile selection response has been higher for disease resistance (Hollenbeck and Johnston, 2018). More specifically, selection response was above 6.0% per generation in C. angulata after three selection generations in Vietnam. The summary of selection responses in oysters is shown in Table 1.
Another opportunity for genetic gain is to exploit non-additive genetic variance in survival and other traits. Crossbreeding is the optimal way to exploit heterosis for inbred lines rather than selection, which has been considered as the standard method of genetic enhancement for the majority of finfishes (Gjedrem and Rye, 2018). A trait with more non-additive genetic variation has greater potential for exploiting heterosis (Hollenbeck and Johnston, 2018). According to quantitative genetic theory, heterosis is only expressed when there is some level of overdominance in a trait. Crossing inbred strains is then able to capture a gain without inbreeding (Falconer and Mackay, 1996). Heterosis has been directly observed in some mollusc species (Hedgecock and Davis, 2007; Deng et al., 2010); while other studies have found little or no evidence for heterosis across multiple strains and production systems, as in C. angulata (In et al., 2017). Overall there is little evidence for overdominance of relevant traits. One important practical issue is the difficulty of producing and maintaining separate inbred lines of molluscs for crossbreeding. If inbred lines show inbreeding depression, outbred diverse populations need to be maintained to assess whether crossing simply resolves inbreeding or actually leads to real gain above the value of the outbred lines. Oysters in particular, or molluscs in general are as easily grown and maintained as other animals or plant species (Hallauer, 2008). While inter-specific hybridisation has shown some success, such as in abalone (Lafarga de la Cruz and Gallardo-Escárate, 2011), a technical barrier for crossbreeding in most oyster species is seen in the practical difficulties in maintaining and testing enough inbred lines for effective genetic gain. In general, the principal strategy for genetic improvement is to enhance production traits and control inbreeding. For sustainable development, an oyster breeding program, which delivers genetic gain for production traits, yet limits inbreeding, is required. Maintaining a wide range of genotypes could give a hatchery population more flexibility to adapt to different environments (Boudry, 2008), while increasing the resilience of the population.
4 Application of genomic information in genetic improvement programs
4.1 Opportunities for genomic selection
Together with conventional selective breeding based on phenotypes and pedigrees, there is the potential to use molecular and genomic information to boost selection response (Martinez, 2007), particularly for traits that are difficult or expensive to measure or have low heritabilities; this is especially the case for markers that are linked to large effect Quantitative Trait Loci (Robledo et al., 2017). For example, genomic selection is a good option for difficult to measure traits such as soft tissue mass at different ages. Whole genome sequencing costs are typically still too high to routinely genotype all animals in a pedigree, especially for non-model species. However, relatively inexpensive partial genome information can now be acquired by “genotyping by sequencing” (GBS). GBS provides not only a cost of low genotyping but also a significant amount of high-quality genetic marker information which can be suitable for genomic selection. However, this approach may result in missing data which is a problem for genomic selection. Data can be imputed to infer the rest of missing marker genotypes. In C. angulata, there is a significant amount of missing data obtained by GBS. Therefore, the other genotyping approaches such as whole genome sequencing with few missing data should be preferred for genomic selection.
Among the Restricted-site Associated DNA sequencing (RAD-seq) methods (i.e. GBS methods), Diversity Array Technology (DArTseq) sequencing provides a mixture of a DArT complexity reduction methods and next generation sequencing platforms (Kilian et al., 2012). DArTseq is similar to DArT methods which rely on array hybridisations. The technology is optimised for each organism and application by selecting the most suitable complexity reduction method (both the size of the representation and the fraction of a genome selected for assays). The development of recent technology such as genomic selection (GS), which is the extension of marker assisted selection (MAS) to a genome-wide scale, provides additional opportunities. Rather than aiming to discover particular QTLs, genomic selection assumes that genetic markers are sequenced at sufficient density such that all QTL are in linkage disequilibrium with at least one marker (Hayes and Goddard, 2001). Practically, a reference population with the records of genotypes and phenotypes is used to estimate the effects of each marker on the phenotype of interest, and these effects are used to estimate breeding values for relatives of the reference population that have been genotyped but have no phenotypes (Hayes and Goddard, 2001). MAS is beneficial for big effect loci, while genomic selection is well-suited for enhancement of traits that are controlled by many loci with small effect (Robledo et al., 2017).
Until now, GS has yet to be widely used in genetic programs for bivalves in developing countries, even though some other aquaculture species have applied them to increase the accuracy of breeding values for various traits (Boudry et al., 2021; Vu et al., 2021a; Vu et al., 2021c) and disease resistance (Bangera et al., 2017; Robledo et al., 2018). In bivalves, there has been remarkable investment in identifying QTLs, and large effect loci. QTL mapping studies involving RAD-seq have been reported for oysters (Wang et al., 2016). An important candidate QTL gene named PROP1, which regulates hormone production of growth in vertebrates, was identified from the growth-related QTL region (Jiao et al., 2013). In addition, Wang et al. (2016) reported twenty-seven quantitative trait loci (QTL) associated with five growth-related traits. Unfortunately, none of the above QTLs were validated in other populations or by PCR techniques. Although SNPs have been widely used in terrestrial animals, there has been limited use of SNPs for aquatic animal species. One of the main reasons for this limitation is that suitable SNP chips are not currently commercially available for molluscs and their development costs are very high. With DArTseq technology, the genome sequence data can be produced at relatively low cost, which provides an opportunity to estimate genomic breeding values for complex traits that are difficult or expensive to measure, and to identify underlying genes associated with traits of interest. A few studies have used genotyping sequencing at low cost and shown that it can satisfy the developmental demand of the oyster industry with a high accuracy for commercial traits. Recently, DArTseq technology has been used to calculate genomic relationship matrices in C. angulata, and to estimate breeding values which are required for genomic selection (Vu et al., 2021a). Estimated genomic heritabilities were higher in whole weight, shell length, shell depth, shell width, condition index, moisture, mantle and shell colour, taste, tenderness than those estimated by pedigree records in C. angulata (Vu et al., 2021a; Vu et al., 2021c), with similar results found in C. gigas (Boudry et al., 2021; Yang et al., 2022), and C. virginica (McCarty et al., 2022a).
4.2 Machine learning methods for optimisation of genomic selection in oysters
Machine learning (ML) algorithms have been revolutionizing the methods of data analysis and have been implemented in almost every science and engineering field of research. ML models have been routinely used in genomic selection for crop improvement and animal breeding programs (Statnikov et al., 2008; Wang et al., 2022). Recently, ML methods have been used in oyster breeding and genomics in three different ways. First, ML-based deep learning models and convolutional neural networks were used in conjuncture with machine vision systems to accurately predict oyster growth and pearl quality traits such as colour, size, lustre, and completion (Zenger et al., 2017; Zenger et al., 2019) and this forms the basis of next-generation phenotyping in oyster breeding programmes. These methods extract and analyse quantitative information from digital images of oysters, which are not visible to the human eye, and improve the accuracy of predicting the phenotypes in an automated way (Zenger et al., 2017; Jourdan et al., 2023a). Second, the Random Forest algorithm, a popular ML method, is used to predict the missing genotypes of the eastern Pacific oyster (Bernatchez et al., 2017). This reduces the cost of genotyping and provides a similar level of genetic information that could be obtained from high-density sequencing. Third, a typical ML method divides the genetic data into two parts consisting of training and testing (or validation) datasets. The ML algorithms learn the details using the training dataset, and its prediction efficiency is evaluated using the validation dataset. A derivation of this method called fivefold cross-validation analysis is now increasingly used in genomic prediction analyses of Portuguese oysters (Vu et al., 2021c), European flat oysters (Peñaloza et al., 2022), and Pacific oysters (Kriaridou et al., 2023). Typically, the genotype data is divided into 80% training and 20% validation datasets. The breeding values of the validation dataset were predicted based on the information from the training dataset. This process was repeated by randomly selecting samples into training and testing categories, which improves the precision of prediction. Therefore, the overall accuracy of prediction is higher compared to conventional genomic prediction methods.
5 Options for Vietnam to enhance oyster spat quality
Despite some initial achievements in the C. angulata selection program in Vietnam (including a new set of genetic parameters and identifying the effects of the G×E interactions for morphometric, soft tissue, and disease traits), implementation of the genetic program for C. angulata is facing challenges. One challenge is that the sex ratio is commonly unequal with a much larger percentage of males (over 95%) than females (5.0%) for several key production months (April to August 2014 to 2022) in northern Vietnam. The rate of C. angulata whose sex was indeterminate was around 10.7%. It may be feasible to use hormones to induce changing of the sex from male to female. However, the cost of hormone production must be compared to the profits of spat production. Such an economic analysis, along with an analysis of acceptance of this approach for targeted export countries, is required to justify the use of hormones. Oyster sex is also thought to be affected by the productivity of the culture areas. Therefore, further studies need to be realised to properly understand the effect of food concentration on the sex ratio of oysters. In Vietnam, there are algal concentration fluctuations in the seawater environment where oysters are grown. For example, from April to July, when the algal concentration is low, the male oyster ratio is high and vice-versa. However, there has been no research to investigate this problem and to find suitable solutions for sustainable development of oyster industry.
A second problem has been that the genetic diversity of the current population is declining due to the unavailability of other resource populations (Vu et al., unpublished). It is essential to increase the genetic diversity of the current populations possibly by importing parents from overseas (China or Taiwan) to boost the genetic diversity and hence the ability to adapt to changing conditions. However, this needs to be done in a structured and rigorous way where the new genetic material is properly assessed for the value it brings to the breeding program. Any new source of genetic material should be assessed in terms of genetic diversity and biosecurity to secure the current oyster populations in Vietnam. The ability to source oysters from other existing industries also raises the possibility of collaboration on the development of breeding programs with other countries, which offers the opportunity to share costs and potentially performance data for mutual benefit. An additional challenge is the occurrence of hybridization in the current oyster population where hybrid offspring of C. angulata and C. gigas produced in commercial farms in Vietnam degrades the genetic diversity of the Vietnamese oyster population. Therefore, the genetic tools should be used to differentiate C. angulata and C. gigas.
The proportion of diseased oysters is increasing due to declining water quality in production areas in Van Don, Quang Ninh, Vietnam. This is mainly due to anthropogenic impacts from increasing population pressure. In particular, the recent development of industrial zones near oyster production areas has caused a decrease in water quality. Microplastics and the uptake of pollutants from the ocean are problematic for the oyster industry in Vietnam. There is a need to look for locations to hold key oyster families where the impacts of pollutants and diseases might be reduced. A comprehensive study should be carried out to identify the pathogens causing diseases in oysters. From that, a full solution can be developed to treat these diseases in oysters. Last but not least, relationships between disease and water environmental conditions in oyster culturing areas need to be investigated to identify causes of disease.
In addition to securing appropriate water quality and production conditions, a genetic selection program which can jointly select for whole weight, survival, and disease resistance traits is necessary to develop the oyster industry further, especially for an oyster industry with a production level of 50,000 t/annum. The selective breeding program of C. angulata has completed the eighth generation from the base population established from parents sourced from three geographic regions. The importation of new genetic resources is essential to securing the genetic diversity of the current oyster breeding population. It is also time in Vietnam to improve the efficiency of genetic programs by improving the accuracy of breeding value prediction by applying genomic technologies. However, this requires a large investment for research to further discover the actual benefits to oyster industry in Vietnam. Due to the high initial cost for genomic selection, it would be helpful to organise a workshop or conference to disseminate knowledge to farmers and young researchers on how to utilise this technology for the oyster industry in Vietnam. The formation of a national genetic program which collaborates with industry would be a wise action to improve the spat quality of oysters in a sustainable way. The selective breeding nucleus populations are currently held by RIA1, the big oyster companies are multiplicators, and Universities such as Vietnam National University could assist with genetic analysis and genetic program design.
Climate change poses one of the biggest threats to the oyster industry in Vietnam, in particular through the increase in water temperature that is anticipated. Water temperature affects spawning, rearing and grow-out of oysters. Selective breeding can mitigate the effects of climate change through improvement of traits such as resilience to higher temperatures and salinity (Parker et al., 2015; Parker et al., 2021). The best solution is for the big companies to collaborate to solve problems on genotyping cost and develop SNP chips that are affordable to farmers. To incorporate genomic technology into current genetic programs, it is best to genotype all candidates to become parents for subsequent generations and their progenies. This would remove the need to rear oyster families separately during the early stages and eliminate this common environment confounding effect. Moreover, genomic selection can occur at younger ages to decrease the operating and labour costs of oyster farmers in Vietnam. The immediate need for research is to develop SNP chips for C. angulata to reduce the genotyping cost. Once SNP chips are available, the sequencing cost for one oyster is currently $2 which is affordable for oyster farmers. Use of SNP chips would allow farmers/researchers to select oysters easily using markers associated with traits of interest. Currently, facilities/machines for molecular genetics do exist in Vietnam at universities such as Vietnam National University, that could provide genetic testing, prediction of estimated breeding values, genetic program design and SNP chip development.
Author contributions
TL: Writing – original draft, Writing – review & editing. SV: Conceptualization, Methodology, Writing – original draft, Writing – review & editing. SU: Funding acquisition, Methodology, Writing – review & editing. SS: Writing – review & editing. AG: Writing – review & editing. MD: Writing – review & editing. IV: Writing – review & editing. JG: Writing – review & editing. TT: Writing – review & editing. CG: Writing – review & editing. GC: Writing – review & editing. TL: Writing – review & editing. TN: Writing – review & editing. TN: Writing – review & editing. TV: Writing – review & editing. PH: Writing – review & editing. WK: Writing – review & editing. WO: Conceptualization, Methodology, Writing – review & editing.
Funding
The publication fee for this paper was funded by 1) Australian Centre for International Agricultural Research (ACIAR) via the project: "Blue economy: Valuing the carbon sequestration potential in oyster aquaculture” (FIS/2020/175) 2) Ha Long University, Quang Ninh, Vietnam 3) Professor Cedric Gondro, Michigan State University, USA.
Acknowledgments
Dr Nguyen Thi Binh (National Institute of Medicinal Materials, Vietnam) and Ms Nguyen Thi Tham (Ha Long University, Vietnam) for formatting this manuscript before submission.
Conflict of interest
Author AG was employed by Statistical and ASReml Consultant. Author IV was employed by company Viet Uc Seafood Corporation.
The remaining authors declare that the research was conducted in the absence of any commercial or financial relationships that could be construed as a potential conflict of interest.
Publisher’s note
All claims expressed in this article are solely those of the authors and do not necessarily represent those of their affiliated organizations, or those of the publisher, the editors and the reviewers. Any product that may be evaluated in this article, or claim that may be made by its manufacturer, is not guaranteed or endorsed by the publisher.
References
Allen S. K. Jr., Small J. M., Kube P. D. (2021). Genetic parameters for Crassostrea virginica and their application to family-based breeding in the mid-Atlantic, USA. Aquaculture 538, 736578. doi: 10.1016/j.aquaculture.2021.736578
Andersen U. B., Thomassen M. S., Rørå A. M. B. (1997). Texture properties of farmed rainbow trout (Oncorhynchus mykiss): effects of diet, muscle fat content and time of storage on ice. J. Sci. Food Agric. 74 (3), 347–353. doi: 10.1002/(SICI)1097-0010(199707)74:3<347::AID-JSFA802>3.0.CO;2-F
Appleyard S. A., Ward R. D. (2006). Genetic diversity and effective population size in mass selection lines of Pacific oyster (Crassostrea gigas). Aquaculture 254, 148–159. doi: 10.1016/j.aquaculture.2005.10.017
Baird N. A., Etter P. D., Atwood T. S., Currey M. C., Shiver A. L., Lewis Z. A., et al. (2008). Rapid SNP discovery and genetic mapping using sequenced RAD markers. PloS One 3, e3376. doi: 10.1371/journal.pone.0003376
Bangera R., Correa K., Lhorente J. P., Figueroa R., Yáñez J. M. (2017). Genomic predictions can accelerate selection for resistance against Piscirickettsia salmonis in Atlantic salmon (Salmo salar). BMC Genomics 18, 121. doi: 10.1186/s12864-017-3487-y
Batista F. M. M. (2007). Assessment of the aquacultural potential of the Portuguese oyster. Crassostrea angulata.
Bernatchez L., Wellenreuther M., Araneda C., Ashton D. T., Barth J. M. I., Beacham T. D., et al. (2017). Harnessing the power of genomics to secure the future of seafood. Trends Ecol. Evol. 32 (9), 665–680. doi: 10.1016/j.tree.2017.06.010
Botta R., Asche F., Borsum J. S., Camp E. V. (2020). A review of global oyster aquaculture production and consumption. Mar. Policy 117, 103952. doi: 10.1016/j.marpol.2020.103952
Boudry P. (2008). Review on breeding and reproduction of European aquaculture species. Aqua Breed. 2008.
Boudry P., Allal F., Aslam M. L., Bargelloni L., Bean T. P., Brard-Fudulea S., et al. (2021). Current status and potential of genomic selection to improve selective breeding in the main aquaculture species of International Council for the Exploration of the Sea (ICES) member countries. Aquaculture Rep. 20, 100700. doi: 10.1016/j.aqrep.2021.100700
Boudry P., Barre M., Gerard A. (1997). Genetic improvement and selection in shellfish: A review based on oyster research and production. Seminar Genet. Breed. Mediterr. Aquaculture Species CIHEAM-FAO,. 1997.
Boudry P., Heurtebise S., Collet B., Cornette F., Gérard A. (1998). Differentiation between populations of the Portuguese oyster, Crassostrea angulata (Lamark) and the Pacific oyster, Crassostrea gigas (Thunberg), revealed by mtDNA RFLP analysis. J. Exp. Mar. Biol. Ecol. 226 (2), 279–291. doi: 10.1016/S0022-0981(97)00250-5
Charlesworth D., Willis J. H. (2009). The genetics of inbreeding depression. Nat. Rev. Genet. 10, 783–796. doi: 10.1038/nrg2664
Chi Y., Li Q., Xu C. (2023). Genetic parameters and genotype by environment interactions for harvest traits in the Pacific oyster (Crassostrea gigas). Aquaculture Int. 1–21. doi: 10.1007/S10499-023-01159-8/METRICS
Courtois B., Audebert A., Dardou A., Roques S., Ghneim-Herrera T., Droc G., et al. (2013). Genome-wide association mapping of root traits in a japonica rice panel. PloS One 8, e78037. doi: 10.1371/journal.pone.0078037
Dégremont L., Bédier E., Boudry P. (2010). Summer mortality of hatchery-produced Pacific oyster spat (Crassostrea gigas). II. Response to selection for survival and its influence on growth and yield. Aquaculture 299 (1-4), 21–29.
Dégremont L., Bédier E., Soletchnik P., Ropert M., Huvet A., Moal J., et al. (2005). Relative importance of family, site, and field placement timing on survival, growth, and yield of hatchery-produced Pacific oyster spat (Crassostrea gigas). Aquaculture 249, 213–229. doi: 10.1016/j.aquaculture.2005.03.046
Dégremont L., Ernande B., Bédier E., Boudry P. (2007). Summer mortality of hatchery-produced Pacific oyster spat (Crassostrea gigas). I. Estimation of genetic parameters for survival and growth. Aquaculture 262, 41–53. doi: 10.1016/j.aquaculture.2006.10.025
Dégremont L., Maurouard E., Ledu C., Benabdelmouna A. (2019). Synthesis of the PLAN DE SAUVEGARDE using selected all-triploid oysters to reduce the shortage of spat in France due to OsHV-1–associated mortality in Crassostrea gigas. Aquaculture 505, 462–472. doi: 10.1016/j.aquaculture.2019.03.014
Degremont L., Nourry M., Maurouard E. (2015). Mass selection for survival and resistance to OsHV-1 infection in Crassostrea gigas spat in field conditions: response to selection after four generations. Aquaculture 446, 111–121. doi: 10.1016/j.aquaculture.2015.04.029
de Melo C. M. R., Divilov K., Durland E., Schoolfield B., Davis J., Carnegie R. B., et al. (2021). Introduction and evaluation on the US West Coast of a new strain (Midori) of Pacific oyster (Crassostrea gigas) collected from the Ariake Sea, southern Japan. Aquaculture 531, 735970.
de Melo C. M. R., Divilov K., Schoolfield B., Langdon C. (2019). Selection of group and individual traits of Pacific oysters (Crassostrea gigas) on the West Coast, US. Aquaculture 512, 734389.
de Melo C. M. R., Durland E., Langdon C. (2016). Improvements in desirable traits of the Pacific oyster, Crassostrea gigas, as a result of five generations of selection on the West Coast, USA. Aquaculture 460, 105–115. doi: 10.1016/j.aquaculture.2016.04.017
de Melo C. M. R., Morvezen R., Durland E., Langdon C. (2018). Genetic by environment interactions for harvest traits of the Pacific oyster Crassostrea gigas (Thunberg) across different environments on the West Coast, USA. J. Shellfish Res. 37, 49–61. doi: 10.2983/035.037.0104
Deng Y., Du X., Wang Q. (2009). Selection for fast growth in the Chinese pearl oyster, Pinctada martensii: response of the first-generation line. J. World Aquaculture Soc. 40 (6), 843–847. doi: 10.1111/j.1749-7345.2009.00307.x
Deng Y., Liu X., Zhang G., Wu F. (2010). Heterosis and combining ability: a diallel cross of three geographically isolated populations of Pacific abalone Haliotis discus hannai Ino. Chin. J. Oceanology Limnology 28, 1195–1199. doi: 10.1007/s00343-010-9903-7
Denic M., Taeubert J. E., Lange M., Thielen F., Scheder C., Gumpinger C., et al. (2015). Influence of stock origin and environmental conditions on the survival and growth of juvenile freshwater pearl mussels (Margaritifera margaritifera) in a cross-exposure experiment. Limnologica 50, 67–74. doi: 10.1016/J.LIMNO.2014.07.005
Dove M., Kube P., Lind C., Cumbo V., Raftos D., O'Connor W. (2020). Accelerated sydney rock oyster (SRO) breeding research, department of planning, industry and environment. Final Rep. to FRDC. 33 pp.
Dove M. C., Nell J. A., O'Connor W. A. (2013). Evaluation of the progeny of the fourth-generation Sydney rock oyster Saccostrea glomerata (Gould 1850) breeding lines for resistance to QX disease (Marteilia sydneyi) and winter mortality (Bonamia roughleyi). Aquaculture Res. 44, 1791–1800.
Elshire R. J., Glaubitz J. C., Sun Q., Poland J. A., Kawamoto K., Buckler E. S., et al. (2011). A robust, simple genotyping-by-sequencing (GBS) approach for high diversity species. PloS One 6, e19379. doi: 10.1371/journal.pone.0019379
Evans S., Camara M. D., Langdon C. J. (2009). Heritability of shell pigmentation in the Pacific oyster, Crassostrea gigas. Aquaculture 286, 211–216. doi: 10.1016/j.aquaculture.2008.09.022
Evans S., Langdon C. (2006). Effects of genotype× environment interactions on the selection of broadly adapted Pacific oysters (Crassostrea gigas). Aquaculture 261, 522–534. doi: 10.1016/j.aquaculture.2006.07.022
Evans F., Matson S., Brake J., Langdon C. (2004). The effects of inbreeding on performance traits of adult Pacific oysters (Crassostrea gigas). Aquaculture 230, 89–98. doi: 10.1016/j.aquaculture.2003.09.023
Falconer D. S., Mackay T. F. C. (1996). Introduction to Quantitative Genetics. 4th ed (Harlow: Longman).
Foighil D.Ó., Gaffney P. M., Wilbur A. E., Hilbish T. J. (1998). Mitochondrial cytochrome oxidase I gene sequences support an Asian origin for the Portuguese oyster Crassostrea angulata. Mar. Biol. 131 (3), 497–503. doi: 10.1007/S002270050341/METRICS
Geffroy B., Besson M., Sánchez-Baizán N., Clota F., Goikoetxea A., Sadoul B., et al. (2021). Unraveling the genotype by environment interaction in a thermosensitive fish with a polygenic sex determination system. Proc. Natl. Acad. Sci. 118, e2112660118.
Geist J., Bayerl H., Stoeckle B. C., Kuehn R. (2021). Securing genetic integrity in freshwater pearl mussel propagation and captive breeding. Sci. Rep. 11 (1), 1–11. doi: 10.1038/s41598-021-95614-2
Giang C. T. (2011). Establishing hatchery production and growout culture techniques of Pacific Oyster for export. Final Rep. Natl. Project.
Gjedrem T., Robinson N. (2014). Advances by selective breeding for aquatic species: a review. Agric. Sci. 5, 1152.
Gjedrem T., Rye M. (2018). Selection response in fish and shellfish: a review. Rev. Aquaculture 10, 168–179. doi: 10.1111/raq.12154
Gjedrem T., Thodesen J. (2005). Selection in Selection and Breeding Programs in Aquaculture. Ed. Gjedrem T. (Dordrecht: Springer), 89–110.
Gutierrez A. P., Turner F., Gharbi K., Talbot R., Lowe N. R., Peñaloza C., et al. (2017a). Development of a Medium Density Combined-Species SNP Array for Pacific and European Oysters (Crassostrea gigas and Ostrea edulis). G3 (Bethesda Md.) 7 (7), 2209–2218. doi: 10.1534/G3.117.041780
Hayes B., Goddard M. (2001). Prediction of total genetic value using genome-wide dense marker maps. Genetics 157, 1819–1829. doi: 10.1093/genetics/157.4.1819
Heasman M. P., Goard L., Diemar J., R.B C. (2000). Improved early survivals of mollusks: Sydney Rock Oyster, NSW Fishereis Report, NSW (Australia: Port Stephens Fisheries Centre), 2003.
Hedgecock D., Davis J. P. (2007). Heterosis for yield and crossbreeding of the Pacific oyster Crassostrea gigas. Aquaculture 272, S17–S29. doi: 10.1016/j.aquaculture.2007.07.226
Hedgecock D., Li G., Hubert S., Bucklin K., Ribes V. (2004). Widespread null alleles and poor cross-species amplification of microsatellite DNA loci cloned from the Pacific oyster, Crassostrea gigas. J. Shellfish Res. 23, 379–386.
Hedgecock D., Pudovkin A. I. (2011). Sweepstakes reproductive success in highly fecund marine fish and shellfish: a review and commentary. Bull. Mar. Sci. 87, 971–1002.
Hollenbeck C. M., Johnston I. A. (2018). Genomic tools and selective breeding in molluscs. Front. Genet. 9, 253.
Huvet A., Gérard A., Ledu C., Phélipot P., Heurtebise S., Boudry P. (2002). Is fertility of hybrids enough to conclude that the two oysters Crassostrea gigas and Crassostrea angulata are the same species? Aquat. Living Resour. 15, 45–52.
In V.-V., O'Connor W., Dove M., Knibb W. (2016). Can genetic diversity be maintained across multiple mass selection lines of Sydney rock oyster, Saccostrea glomerata despite loss within each? Aquaculture 454, 210–216.
In V.V., Sang V.V., O’Connor W., Van P. T., Dove M., Knibb W., et al. (2017). Are strain genetic effect and heterosis expression altered with culture system and rearing environment in the Portuguese oyster (Crassostrea angulata)? Aquaculture Res. 48 (8), 4058–4069. doi: 10.1111/are.13227
Jiao W., Fu X., Dou J., Li H., Su H., Mao J., et al. (2013). High-resolution linkage and quantitative trait locus mapping aided by genome survey sequencing: building up an integrative genomic framework for a bivalve mollusc. DNA Res. 21, 85–101.
Jourdan A., Morvezen R., Enez F., Haffray P., Lange A., Vétois E., et al. (2023a). Potential of genomic selection for growth, meat content and colour traits in mixed-family breeding designs for the Pacific oyster Crassostrea gigas. Aquaculture 576, 739878. doi: 10.1016/J.AQUACULTURE.2023.739878
Kause A., Ritola O., Paananen T., Mäntysaari E., Eskelinen U. (2002). Coupling body weight and its composition: a quantitative genetic analysis in rainbow trout. Aquaculture 211, 65–79.
Kilian A., Wenzl P., Huttner E., Carling J., Xia L., Blois H., et al. (2012). “Diversity arrays technology: a generic genome profiling technology on open platforms,” in Data production and analysis in population genomics (Springer), 67–89.
Kinghorn B. P. (2011). An algorithm for efficient constrained mate selection. Genet. Selection Evol. 43, 1–9.
Kong N., Li Q., Yu H., Kong L. F. (2015). Heritability estimates for growth-related traits in the Pacific oyster (Crassostrea gigas) using a molecular pedigree. Aquaculture Res. 46, 499–508.
Kriaridou C., Tsairidou S., Fraslin C., Gorjanc G., Looseley M. E., Johnston I. A., et al. (2023). Evaluation of low-density SNP panels and imputation for cost-effective genomic selection in four aquaculture species. Front. Genet. 14. doi: 10.3389/FGENE.2023.1194266/BIBTEX
Kube P., Hick P., Cunningham M., Kirkland P., Elliott N., O’Connor W., et al. (2013). “Current progress in genetic selection for resistance to Pacific Oyster mortality syndrome caused by OsHV-1 microvariant (μ-var) in Australia,” in "5th International Oyster Symposium (IOS5), World Oyster Society(Ho Chi Minh city).
Lafarga de la Cruz F., Gallardo-Escárate C. (2011). Intraspecies and interspecies hybrids in Haliotis: natural and experimental evidence and its impact on abalone aquaculture. Rev. Aquaculture 3, 74–99.
Lallias D., Taris N., Boudry P., Bonhomme F., Lapegue S. (2010). Variance in the reproductive success of flat oyster Ostrea edulis L. assessed by parentage analyses in natural and experimental conditions. Genet. Res. 92, 175–187.
Langdon C., Evans F., Jacobson D., Blouin M. (2003). Yields of cultured Pacific oysters Crassostrea gigas Thunberg improved after one generation of selection. Aquaculture 220, 227–244.
Lapegue S., Batista F., Heurtebise S., Yu Z., Boudry P. (2004). Evidence for the presence of the Portuguese oyster, Crassostrea angulata, in northern China. J. Shellfish Res. 23, 759–763.
Lie Ø. (2001). Flesh quality - the role of nutrition. Aquaculture Res. 32, 341–348. doi: 10.1046/j.1355-557x.2001.00026.x
Lucas A., Beninger P. G. (1985). The use of physiological condition indices in marine bivalve aquaculture. Aquaculture 44(3), 187–200.
Martinez V. (2007). Marker-assisted selection in fish and shellfish breeding schemes. Marker-Assisted selection-Current Status Future Perspect. crops livestock forestry fish, 329–362.
McCarty A. J., Allen S. K., Plough L. V. (2022a). Genome-wide analysis of acute low salinity tolerance in the eastern oyster Crassostrea virginica and potential of genomic selection for trait improvement. G3 Genes|Genomes|Genetics 12 (1). doi: 10.1093/G3JOURNAL/JKAB368
McCarty A. J., McFarland K., Small J., Allen S. Jr., Plough L. (2020). Heritability of acute low salinity survival in the Eastern oyster (Crassostrea virginica). Aquaculture 529, 735649. doi: 10.1016/j.aquaculture.2020.735649
Meuwissen T. (1997). Maximizing the response of selection with a predefined rate of inbreeding. J. Anim. Sci. 75, 934–940. doi: 10.2527/1997.754934x
Meuwissen T. H., Woolliams J. A. (1994). Effective sizes of livestock populations to prevent a decline in fitness. Theor. Appl. Genet. 89, 1019–1026. doi: 10.1007/BF00224533
Mulder H., Veerkamp R., Ducro B., Van Arendonk J., Bijma P. (2006). Optimisation of dairy cattle breeding programs for different environments with genotype by environment interaction. J. Dairy Sci. 89, 1740–1752. doi: 10.3168/jds.S0022-0302(06)72242-1
Naciri-Graven Y., Launey S., Lebayon N., Gerard A., Baud J.-P. (2000). Influence of parentage upon growth in Ostrea edulis: evidence for inbreeding depression. Genet. Res. 76, 159–168. doi: 10.1017/S0016672300004663
Naciri-Graven Y., Martin A.-G., Baud J.-P., Renault T., Gérard A. (1998). Selecting the flat oyster Ostrea edulis (L.) for survival when infected with the parasite Bonamia ostreae. J. Exp. Mar. Biol. Ecol. 224, 91–107. doi: 10.1016/S0022-0981(97)00171-8
Neira R., Lhorente J. P., Araneda C., Díaz N., Bustos E., Alert A. (2004). Studies on carcass quality traits in two populations of Coho salmon (Oncorhynchus kisutch): phenotypic and genetic parameters. Aquaculture 241, 117–131. doi: 10.1016/j.aquaculture.2004.08.009
Nell J. A., Smith I. R., McPhee C. C. (2000). The Sydney rock oyster Saccostrea glomerata (Gould 1850) breeding programme: progress and goals. Aquaculture Res. 31 (1), 45–49. doi: 10.1046/j.1365-2109.2000.00387.x
Nell J. A., Smith I. R., Sheridan A. (1999). Third generation evaluation of Sydney rock oyster Saccostrea commercialis (Iredale and Roughley) breeding lines. Aquaculture 170, 195–203. doi: 10.1016/S0044-8486(98)00408-6
Newkirk G. F., Haley L. E. (1982). Progress in selection for growth rate in the European oyster Ostrea edulis. Mar. Ecol. Prog. series. Oldendorf 10 (1), 77–79.
Nguyen N. H. (2016). Genetic improvement for important farmed aquaculture species with a reference to carp, tilapia and prawns in Asia: achievements, lessons and challenges. Fish Fisheries 17, 483–506.
O’Connor W. A., Dove M. C. (2009). The changing face of oyster culture in New South Wales, Australia. Journal of Shellfish Research 28, 4, 803–811. doi: 10.2983/035.028.0409
Parker L. M., O’Connor W. A., Raftos D. A., Pörtner H. O., Ross P. M. (2015). Persistence of Positive Carryover Effects in the Oyster, Saccostrea glomerata, following Transgenerational Exposure to Ocean Acidification. PloS One 10 (7), e0132276. doi: 10.1371/JOURNAL.PONE.0132276
Parker L. M., Scanes E., O’Connor W. A., Ross P. M. (2021). Transgenerational plasticity responses of oysters to ocean acidification differ with habitat. J. Exp. Biol. 224 (12). doi: 10.1242/JEB.239269/238066
Peñaloza C., Barria A., Papadopoulou A., Hooper C., Preston J., Green M., et al. (2022). Genome-wide association and genomic prediction of growth traits in the European flat oyster (Ostrea edulis). Front. Genet. 13. doi: 10.3389/FGENE.2022.926638/BIBTEX
Peterson B. C., Burr G. S., Pietrak M. R., Proestou D. A. (2020). Genetic improvement of North American Atlantic Salmon and the Eastern Oyster Crassostrea virginica at the US Department of Agriculture–Agricultural Research Service National Cold Water Marine Aquaculture Center. North Am. J. Aquaculture 82, 321–330. doi: 10.1002/naaq.10144
Pierce J., O’Connor W. (2014). “Impact of Oyster Farming on Rural Community Sustainability in North Vietnam,” in Linking Local and Global Sustainability. Eds. McKenzie S., Sandhu S., Harris H. (Springer Netherlands), 207–224.
Proestou D. A., Vinyard B. T., Corbett R. J., Piesz J., Allen S. K., Small J. M., et al. (2016). Performance of selectively-bred lines of eastern oyster, Crassostrea virginica, across eastern US estuaries. Aquaculture 464, 17–27. doi: 10.1016/j.aquaculture.2016.06.012
Qi H., Song K., Li C., Wang W., Li B., Li L., et al. (2017). Construction and evaluation of a high-density SNP array for the Pacific oyster (Crassostrea gigas). PloS One 12 (3). doi: 10.1371/JOURNAL.PONE.0174007
Reece K. S., Ribeiro W. L., Gaffney P. M., Carnegie R. B., Allen S. K. (2004). Microsatellite marker development and analysis in the eastern oyster (Crassostrea virginica): Confirmation of null alleles and non-mendelian segregation ratios. J. Heredity 95 (4), 346–352. doi: 10.1093/jhered/esh058
RIA1 annual report (2009). Building bivalve mollusc hatchery Production capacity project in Vietnam and Australia, FIS/2005/114.
Robledo D., Matika O., Hamilton A., Houston R. D. (2018). Genome-wide association and genomic selection for resistance to amoebic gill disease in atlantic salmon. G3: Genes Genomes Genet.
Robledo D., Palaiokostas C., Bargelloni L., Martínez P., Houston R. (2017). Applications of genotyping by sequencing in aquaculture breeding and genetics. Rev. Aquac.
Rye M. (2012). Review of Australian breeding programs for Pacific Oysters, Sydney Rock Oyster, Barramundi and Prawns. Akvaforsk Genetics Centre A/S Norway. Confidential Rep. Seafood CRC.
Sae-Lim P., Gjerde B., Nielsen H. M., Mulder H., Kause A. (2016). A review of genotype-by-environment interaction and micro-environmental sensitivity in aquaculture species. Rev. Aquaculture 8, 369–393.
Saillant E., Dupont-Nivet M., Sabourault M., Haffray P., Laureau S., Vidal M.-O., et al. (2009). Genetic variation for carcass quality traits in cultured sea bass (Dicentrarchus labrax). Aquat. Living Resour. 22, 105–112.
Sasikumar G., Krishnakumar P. (2011). Aquaculture planning for suspended bivalve farming systems: The integration of physiological response of green mussel with environmental variability in site selection. Ecol. Indic. 11, 734–740.
Sirovy K. A., Johnson K. M., Casas S. M., La Peyre J. F., Kelly M. W. (2021). Lack of genotype-by-environment interaction suggests limited potential for evolutionary changes in plasticity in the eastern oyster. Crassostrea virginica. Mol. Ecol. 30 (22), 5721–5734. doi: 10.1111/MEC.16156
Statnikov A., Wang L., Aliferis C. F. (2008). A comprehensive comparison of random forests and support vector machines for microarray-based cancer classification. BMC Bioinf. 9 (1), 1–10. doi: 10.1186/1471-2105-9-319/TABLES/4
Symonds J. E., Clarke S. M., King N., Walker S. P., Blanchard B., Sutherland D., et al. (2019). Developing successful breeding programs for New Zealand aquaculture: a perspective on progress and future genomic opportunities. Front. Genet. 10, 27. doi: 10.3389/fgene.2019.00027
Taris N., Ernande B., McCombie H., Boudry P. (2006). Phenotypic and genetic consequences of size selection at the larval stage in the Pacific oyster (Crassostrea gigas). J. Exp. Mar. Biol. Ecol. 333 (1), 147–158. doi: 10.1016/J.JEMBE.2005.12.007
Toro J. E., Aguila P., Vergara A. M. (1996). Spatial variation in response to selection for live weight and shell length from data on individually tagged Chilean native oysters (Ostrea Chilensis Philippi 1845). Aquaculture 146 (1-2), 27–36. doi: 10.1016/S0044-8486(96)01368-3
Toro J. E., Newkirk G. F. (1991). Response to artificial selection and realised heritability estimate for shell height in the Chilean oyster Ostrea Chilensis. Aquat. Living Resour. 4, 101–108. doi: 10.1051/alr:1991009
Ugalde S. C., Vu S. V., Giang C. T., Ngoc N. T. H., Tran T. K. A., Mullen J. D., et al. (2023). Status, supply chain, challenges, and opportunities to advance oyster aquaculture in northern Vietnam. Aquaculture 572. doi: 10.1016/j.aquaculture.2023.739548
Vandeputte M., Haffray P. (2014). Parentage assignment with genomic markers: a major advance for understanding and exploiting genetic variation of quantitative traits in farmed aquatic animals. Front. Genet. 5, 432. doi: 10.3389/fgene.2014.00432
Vandeputte M., Kocour M., Mauger S., Dupont-Nivet M., De Guerry D., Rodina M., et al. (2004). Heritability estimates for growth-related traits using microsatellite parentage assignment in juvenile common carp (Cyprinus carpio L.). Aquaculture 235, 223–236. doi: 10.1016/j.aquaculture.2003.12.019
Van Khang P., Phuong T. H., Dat N. K., Knibb W., Nguyen N. H. (2018). An 8-Year breeding program for Asian seabass Lates calcarifer: Genetic evaluation, experiences, and challenges. Front. Genet. doi: 10.3389/fgene.2018.00191
Vu V. I. (2017). Genetic and physiological parameters associated with oyster reproduction (University of the Sunshine Coast).
Vu S. V., Gilmour A. R., Nguyen N. T., Dove M., Van Vu I., Le T. S., et al. (2021b). Does genetic correlation change across environments for harvest whole weight and its uniformity in the Portuguese oyster (Crassostrea angulata). Aquaculture 536, 736444. doi: 10.1016/j.aquaculture.2021.736444
Vu S. V., Gondro C., Nguyen N. T. H., Gilmour A. R., Tearle R., Knibb W., et al. (2021a). Prediction accuracies of genomic selection for nine commercially important traits in the portuguese oyster (Crassostrea angulata) using DArT-seq technology. Genes 12 (2), 210. doi: 10.3390/GENES12020210
Vu S., Knibb W., Gondro C., Subramanian S., Nguyen N. T., Alam M., et al. (2021a). Genomic prediction for whole weight, body shape, meat yield, and colour traits in the Portuguese oyster Crassostrea angulata. Front. Genet. 12, 1140. doi: 10.3389/fgene.2021.661276
Vu S. V., Knibb W., Nguyen N. T., Vu I. V., O'Connor W., Dove M., et al. (2020a). First breeding program of the Portuguese oyster Crassostrea angulata demonstrated significant selection response in traits of economic importance. Aquaculture 518, 734664. doi: 10.1016/j.aquaculture.2019.734664
Vu S. V., Knibb W., O’Connor W., Nguyen N. T. H., Van In V., Dove M., et al. (2020b). Genetic parameters for traits affecting consumer preferences for the Portuguese oyster, Crassostrea angulata. Aquaculture 526, 735391. doi: 10.1016/J.AQUACULTURE.2020.735391
Wan S., Li Q., Yu H., Liu S., Kong L. (2020). Estimating heritability for meat composition traits in the golden shell strain of Pacific oyster (Crassostrea gigas). Aquaculture 516, 734532. doi: 10.1016/j.aquaculture.2019.734532
Wang Q., Li Q., Kong L., Yu R. (2012). Response to selection for fast growth in the second generation of Pacific oyster (Crassostrea gigas). J. Ocean Univ. China 11, 413–418. doi: 10.1007/s11802-012-1909-7
Wang J., Li L., Zhang G. (2016). A High-Density SNP Genetic Linkage Map and QTL Analysis of Growth-Related Traits in a Hybrid Family of Oysters (Crassostrea gigas× C. angulata) Using Genotyping-by-Sequencing. G3: Genes Genomes Genet.
Wang X., Shi S., Wang G., Luo W., Wei X., Qiu A., et al. (2022). Using machine learning to improve the accuracy of genomic prediction of reproduction traits in pigs. J. Anim. Sci. Biotechnol. 13 (1). doi: 10.1186/S40104-022-00708-0
Wijsman J. W. M., Troost K., Fang J., Roncarati A. (2018). Global production of marine bivalves. Trends and challenges. Goods Serv. Mar. Bivalves, 7–26. doi: 10.1007/978-3-319-96776-9_2/FIGURES/5
Yang B., Zhai S., Zhang F., Wang H., Ren L., Li Y., et al. (2022). Genome-wide association study toward efficient selection breeding of resistance to Vibrio alginolyticus in Pacific oyster, Crassostrea gigas. Aquaculture 548, 737592. doi: 10.1016/J.AQUACULTURE.2021.737592
Zenger K. R., Khatkar M. S., Jerry D. R., Raadsma H. W. (2017). The next wave in selective breeding: implementing genomic selection in aquaculture. Proc. Assoc. Advmt. Anim. Breed. Genet. 22, 105–112.
Keywords: genomics, molluscs, selective breeding, Portuguese oyster, Crassostrea angulata
Citation: Le TNP, Vu SV, Ugalde SC, Subramanian S, Gilmour A, Dove M, Vu IV, Geist J, Tran TNT, Gondro C, Cao GT, Le TT, Nguyen TM, Ngo TKN, Vu TTH, H. K. A. P, Knibb W and O’Connor W (2023) The genetics and breeding of the Portuguese oyster, Crassostrea angulata: lessons, experiences, and challenges in Vietnam. Front. Mar. Sci. 10:1161009. doi: 10.3389/fmars.2023.1161009
Received: 07 February 2023; Accepted: 06 October 2023;
Published: 17 November 2023.
Edited by:
Julian Ransangan, University Malaysia Sabah, MalaysiaReviewed by:
Domitilia Coutinha Matias, Instituto Português do Mar e Atmosfera, PortugalPierre Boudry, Institut français de recherche pour l’exploitation de la mer (IFREMER), France
Copyright © 2023 Le, Vu, Ugalde, Subramanian, Gilmour, Dove, Vu, Geist, Tran, Gondro, Cao, Le, Nguyen, Ngo, Vu, H. K. A., Knibb and O’Connor. This is an open-access article distributed under the terms of the Creative Commons Attribution License (CC BY). The use, distribution or reproduction in other forums is permitted, provided the original author(s) and the copyright owner(s) are credited and that the original publication in this journal is cited, in accordance with accepted academic practice. No use, distribution or reproduction is permitted which does not comply with these terms.
*Correspondence: Sang Van Vu, sangvv@vnu.edu.vn
†These authors have contributed equally to this work