- 1Department of Marine Sciences, University of Georgia, Athens, GA, United States
- 2Department of Biology, Creighton University, Omaha, NE, United States
Salt marshes play a crucial role in coastal biogeochemical cycles and provide unique ecosystem services. Salt marsh biomass, which can strongly influence such services, varies over time in response to hydrologic conditions and other environmental drivers. We used gap-filled monthly observations of Spartina alterniflora aboveground biomass derived from Landsat 5 and Landsat 8 satellite imagery from 1984-2018 to analyze temporal patterns in biomass in comparison to air temperature, precipitation, river discharge, nutrient input, sea level, and drought index for a southeastern US salt marsh. Wavelet analysis and ensemble empirical mode decomposition identified month to multi-year periodicities in both plant biomass and environmental drivers. Wavelet coherence detected cross-correlations between annual biomass cycles and precipitation, temperature, river discharge, nutrient concentrations (NOx and PO43–) and sea level. At longer periods we detected coherence between biomass and all variables except precipitation. Through empirical dynamic modeling we showed that temperature, river discharge, drought, sea level, and river nutrient concentrations were causally connected to salt marsh biomass and exceeded the confounding effect of seasonality. This study demonstrated the insights into biomass dynamics and causal connections that can be gained through the analysis of long-term data.
1 Introduction
Salt marshes are the dominant intertidal habitat in the SE US and have important roles in carbon sequestration, the modulation of organic and inorganic nutrient supplies to the coastal ocean, and many additional ecosystem services (Mcleod et al., 2011; Mitsch and Gosselink, 2015). These systems are highly productive, but their primary productivity – and the strength of the associated ecosystem services - can vary substantially among years, as reflected in 2-3-fold interannual variations in observed Spartina aboveground biomass (Więski and Pennings, 2014). As prior long term ecological studies reported, coastal marshes are sensitive to climate change (Reed et al., 2022). Therefore, with a changing climate and increases in temperature, drought frequency and severity, and sea level, the connections between these drivers and plant production are even more critical to understand, particularly since plant biomass and productivity are tightly linked to CO2 exchange with the atmosphere (Abdul-Aziz et al., 2018).
Patterns of primary production in salt marshes can be correlated with climate and hydrologic variables (Odum, 1988). Using an extensive set of field observations, Więski & Pennings (2014) showed that over a 10-year period annual net primary production of Spartina alterniflora, the dominant plant species in salt marshes in the SE US, varied with nearby river discharge, precipitation, sea level, and air temperature. Changes in vegetation can also be captured using the spatially extensive observations from repeated satellite flyovers. O’Donnell and Schalles (2016) used Landsat 5 imagery and connected these to in situ measurements to successfully estimate salt marsh aboveground biomass. They found that peak fall biomass in Spartina marshes at and near Sapelo Island, Georgia estimated with Landsat 5 imagery captured between 1984 and 2011 was correlated with river discharge, drought index, precipitation, and mean sea level. However, these studies do not address longer-term periodicities or causation.
Identifying causal connections between abiotic conditions and ecosystem responses (Morris et al., 2002; Hanson et al., 2016; Crosby et al., 2017) can be challenging because of complex interactions and nonlinear or time-delayed relationships of climate variables and various ecosystem properties such as aboveground biomass (Feher et al., 2017). However, techniques such as convergent cross mapping can extract embedded causal connections from time series measurements that then may prompt further investigation in replicated experimental efforts. Along with that, wavelets and empirical mode decomposition can be used to identify periodicities in these complex systems. Moreover, subtracting shorter-term periodicities can reveal underlying long-term patterns. Although wavelets have been applied to investigate connections between tidal salt marshes and CO2 and CH4 fluxes (Li et al., 2018; Wei et al., 2020; Chu et al., 2021), this suite of techniques has not been used previously to evaluate decadal-scale patterns in salt marsh biomass and detect causal connections.
In this study, we examined the temporal patterns in marsh plant biomass in a southeastern US salt marsh and its potential environmental drivers over 35 years (1984-2018). We first produced a continuous time series of aboveground biomass estimates, which involved comparing several methods of gap-filling. Second, we characterized the intrinsic temporal patterns of both biomass and potential environmental drivers with wavelets and empirical mode decomposition. As part of this, we were able to remove shorter periodicities and identify underlying long-term patterns. Third, we explored similarities in the variations of biomass and environmental parameters, as well as lags between them, using wavelet coherence. Lastly, going beyond the identification of correlations and coherence of patterns, we identified potential causal connections between salt marsh biomass and environmental variables through empirical dynamic modeling, illustrating that the analysis of ecological timeseries can discover known (and potentially new) ecological interactions in complex natural systems such as coastal marshes.
2 Methods
2.1 Site description
The Altamaha River is one of the largest freshwater sources to the Atlantic Ocean within the United States. It has a significant impact on the Georgia coast and forms a complex system of distributary channels, creeks, and intertidal areas (Di Iorio and Castelao, 2013). The Altamaha estuary experiences semidiurnal tides with an average amplitude of ~2 m; intrusion of saltwater is limited to approximately the lower 20 km of the river (Alber and Sheldon, 1999). The polyhaline portion of the estuary has extensive salt marshes dominated by Spartina alterniflora (Więski and Pennings, 2014).
Our study encompassed S. alterniflora marshes located within the lower Altamaha tidal watershed (Figure 1; light blue), as delineated by the US Geological Survey HUC boundary (USGS HUC 03070106; see https://water.usgs.gov/GIS/huc.html) and an overlay of S. alterniflora communities (green shading) along the central Georgia coast. Recent digital mapping of Georgia’s coastal saltmarsh and brackish marsh at 2 m resolution (Alexander and Hladik, 2015) was resized using ENVI 5.3 (Harris L3Geospatial) to 30 m resolution, in order to match the 30 m pixel resolution of the Landsat 5 imagery used in this study (Figure 1). Within the Altamaha tidal watershed, there are 43,847 pixels of S. alterniflora, 30 m in size, covering ~ 39.46 million m2 (3,946 ha).
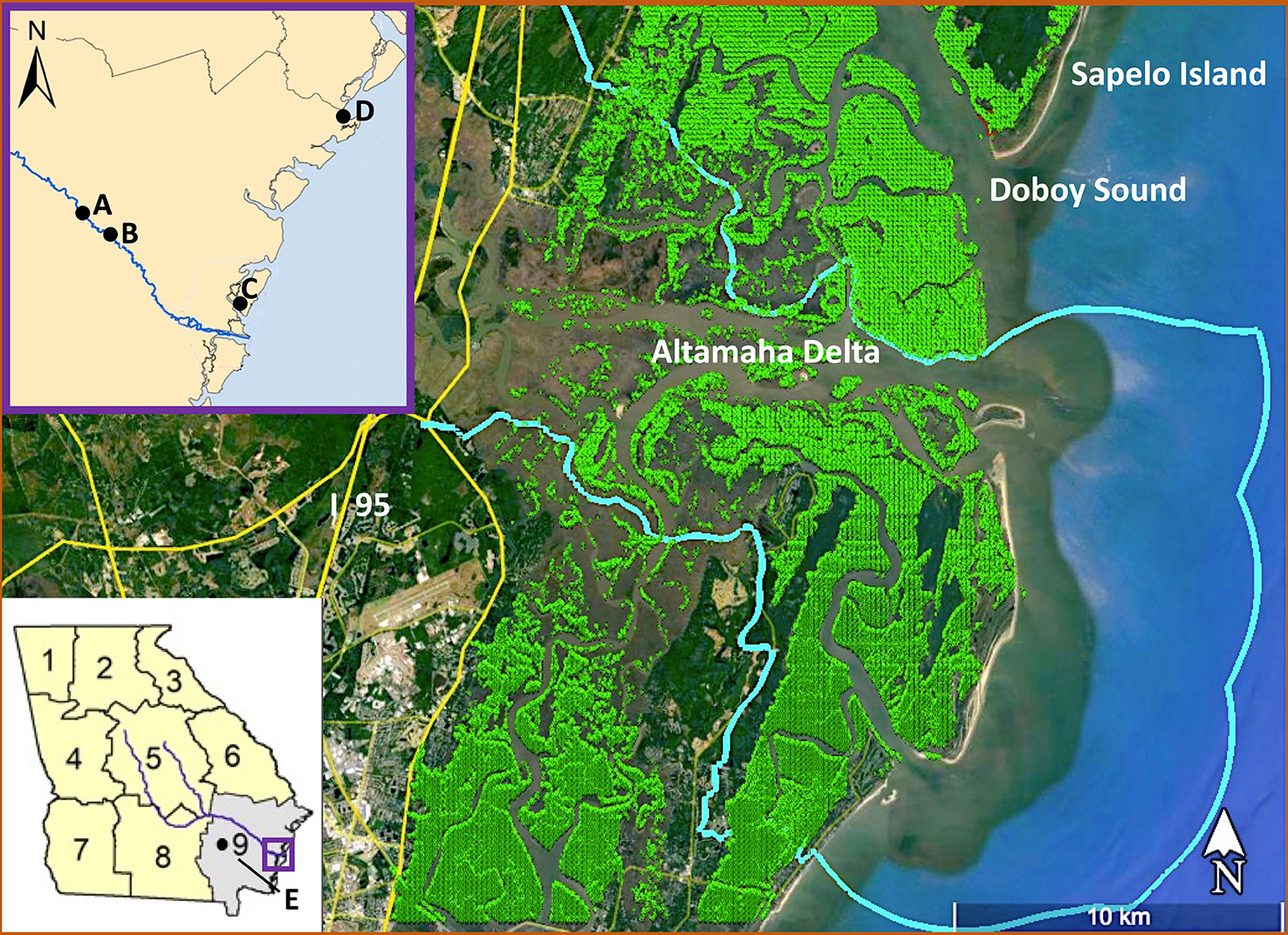
Figure 1 Study site and environmental data sources. A - River Discharge (USGS Doctortown, Gauge 02226000); B - River Nutrients (USGS near Gardi), C - Air Temp & Precipitation (NOAA Sapelo Island), D - Sea Level & Tidal Data (NOAA Fort Pulaski, Gauge 8670870), E - PDSI (NOAA NCDC). Light blue is the contour of the HUC in which the biomass was quantified, the light green pixels are those identified as Spartina alterniflora.
2.2 Spartina alterniflora biomass data
Spartina alterniflora aboveground biomass estimates were obtained from satellite images as described in O’Donnell and Schalles (2016). Briefly, the Landsat Ecosystem Disturbance Adaptive Processing System (LEDAPS) protocol for atmospheric correction of Landsat 5 and Landsat 8 images was used to estimate Spartina biomass in salt marshes within the study site. The nominal return rate for Landsat imagery is 16 days; however, two overlapping Landsat Path/Rows (16/38 and 17/38) cover the study area (O’Donnell and Schalles, 2016), providing two prospective scenes every 16 days. Many satellite images were discarded because of cloud coverage and tidal inundation, which obscured the canopy reflectance signals of coastal marshes. As described in O’Donnell and Schalles (2016), no images coinciding with a tidal inundation recording above 0.65 m relative to NAVD88 at the Fort Pulaski gauge (NOAA station 8670870, the closest to the study site) (offset by +25 minutes) were used.
2.3 Gap-filling
The analyses used in this study require equally spaced timeseries. Therefore, from the available satellite imagery, monthly means of Spartina alterniflora aboveground biomass (g/m2) were calculated from the beginning of 1984 to the end of 2018. However, due to cloud coverage, inundation, and lack of Landsat data from late 2011 to early 2013, the availability of suitable images varied widely. Over the 35-year observational period, there were a total of 227 missing monthly biomass estimates out of 420 months (193 existing data points), with data gaps ranging from 1 to 17 months.
To establish a continuous time series of biomass at monthly resolution, we tested several gap-filling approaches including autoregressive integrated moving average (ARIMA) models, kriging, and inpainting. ARIMA models utilize autoregressive and moving average components of time series data to predict (Box et al., 2015) or gap-fill (e.g., Afrifa-Yamoah et al., 2020; Dorich et al., 2020) time series. We used the R package imputeTS (v3.2), which seasonally decomposes time series, fits an ARIMA model to the time series, uses Kalman smoothing to fill the gaps, and then adds the seasonal component again (Moritz and Bartz-Beielstein, 2017). Kriging is a geostatistical approach commonly used for spatial interpolation (Li and Heap, 2008). As suggested by Knotters and Heuvelink (2010) and Lepot et al. (2017), this approach can be adapted to temporal interpolation such as predicting missing values in air temperature time series (Shtiliyanova et al., 2017). In this study, ordinary kriging was performed. Due to the strong seasonality in marsh aboveground biomass, the data were recast in two dimensions, with the time of year (i.e., month) as one axis and the calendar year of an observation as the second axis, similar to the approach of Walter et al. (2013). This 2D kriging method was implemented using the R package gstat (v2.0-9) (Pebesma, 2004). From the available variogram models the exponential model was selected as it produced the best fit for our biomass data. Since satellite data coverage was more complete during winter than summer months due to reduced cloud cover, the seasonal axis was set to start in January, ensuring that the regions of data gaps were well embedded into measured data. For the inpainting approach, we again separated the seasonal pattern from interannual long-term trends. Missing biomass values in the 2D month vs. year coordinates were in-filled by solving a boundary value problem for an elliptic partial differential equation. This was implemented using the MATLAB script inpaint_nans (their spring-metaphor method) by D'Errico (2022).
The performance of gap-filling methods was assessed both quantitatively and qualitatively. The former was measured by the accuracy of predicting existing data points with bootstrapping using 100 Monte Carlo realizations and computing prediction uncertainties by comparing modeled and observed data. The qualitative assessment focused on periods with long data gaps (such as the 17-month period between the last data from Landsat 5 in November 2011 and the first data from Landsat 8 in March 2013) where the lack of data constraints can lead to poor gap-fill performance. Reproducing seasonal patterns was also critical since late Summer/Fall season peaks in biomass are important metrics of salt marsh productivity (Visser et al., 2006; Kirwan et al., 2009).
2.4 Environmental data
Monthly records of air temperature, precipitation, sea level and Palmer Drought Severity Index (PDSI: high = wet; low = dry) for 1984-2018 were obtained from the NOAA National Climatic Data Center (NCDC). Monthly mean air temperature and total precipitation data were acquired from the NOAA Sapelo station (Figure 1, Lawrimore et al., 2016). PDSI data were retrieved as a monthly time series for the Southeast Climatic Division (NOAA, 2022). In contrast to the biomass data, these datasets have minimal gaps (~10%), which were gap-filled with kriging, as this was the method selected for gap-filling the biomass data (see Results). River discharge data for the Altamaha River were obtained from the USGS Doctortown station because it is the most downstream river gage that covers the time period of our study and provides an accurate measure of all of the water that enters the estuary (Figure 1, U.S. Geological Survey, 2016). Nutrient concentrations (NOx and PO43–) were obtained from the USGS Altamaha River Near Gardi station which is approximately 30 miles upstream from the Altamaha Estuary (Figure 1, U.S. Geological Survey, 2016b). We used nutrient concentrations as a proxy for the composition of the water flooding the marsh to which the plants are exposed. The small number of missing values (<10%) in the nutrient dataset were also gap-filled by kriging. Monthly mean sea level data and tidal projections were obtained from the NOAA Fort Pulaski station (Figure 1; NOAA 2022a; NOAA 2022b).
2.5 Wavelet analysis and wavelet coherence
The continuous wavelet transform (CWT) was used to analyze the time series in time/frequency space. We selected Morlet wavelets for their balance of time and frequency localization and facilitation of detection of time-dependent amplitude and phase (Lau and Weng, 1995; Grinsted et al., 2004). To investigate local correlations between CWTs of environmental variables and biomass, coherence patterns in time/frequency space were computed and phase shifts were quantified. Statistical significance of the results was estimated using Monte Carlo randomizations. Both wavelet and wavelet coherence analyses were implemented using the biwavelet (v0.20.21) R package (Grinsted et al., 2004).
2.6 Empirical mode decomposition
Ensemble empirical mode decomposition (EEMD) was used to decompose the time series into intrinsic mode functions (IMF) (Wu & Huang, 2009). This data-driven method analyzes nonlinear and nonstationary processes by breaking down complex time series into components that are based on the observed minima and maxima in the signal. By sequentially subtracting these modes from the original signal, the time series is effectively decomposed into signals of increasingly lower frequencies (modes). To address the issue of the occurrence of similar frequency signals in multiple modes (mode mixing), IMFs are computed as the ensemble average of multiple stochastic realizations of empirical mode decomposition (Huang et al., 1998), after addition of white noise to the original data. Noise was set to 20% of the standard deviation as suggested by Wu and Huang (2009), and the MATLAB implementation of Yang et al. (2018) was used with Z-score normalization.
2.7 Convergent cross mapping
To explore univariate causal connections between environmental variables and salt marsh biomass, we used convergent cross mapping (CCM; Sugihara et al., 2012). This empirical dynamic modeling approach builds on Takens’ theorem (Takens, 1981), creating state-space reconstructions from lagged time series. The dimensionality of the state space and prediction time lags between biomass and other variables were determined as the ones that maximize prediction skill (Ye et al., 2015). A causal connection was then inferred from the information embedded in the response variable (i.e., biomass) that leads to higher prediction skills with an increasing length of the time series (library size used in the reconstruction of the manifold). Prediction skills produced by causal variables were expected to be higher than regular cross correlation between the two variables (Bonotto et al., 2022). This method was implemented using the R package rEDM (v1.9.3).
To avoid potential false causal implication resulting from seasonality in two variables, we compared our analysis of the observed data with outcomes using randomized seasonal time series (Deyle et al., 2016). One thousand surrogate time series were generated with a seasonal pattern based on the multi-year monthly averages and adding randomly shuffled residuals to each point. These surrogates were tested against biomass in CCM, and the resulting prediction skills were obtained. By comparing those with the prediction skill computed for the real data, the confounding effect of seasonality was quantified.
3 Results
3.1 Satellite imagery-derived biomass estimates and gap filling
A strong seasonal pattern was apparent in our biomass data (Figure S1, top panel). Notably, imagery (and derived biomass estimates) was most limited during summer months because of frequent cloud coverage during months with high humidity, precipitation, and temperature (bottom panel in Figure S1; see also O’Donnell and Schalles, 2016), which led to larger uncertainties in the biomass estimates during peak season. Additionally, interannual differences in biomass led to larger variability during periods of substantial growth or decay (spring green-up and fall senescence), contributing to larger uncertainties during those parts of the year.
The different gap-filling methods for the monthly biomass data showed similar performance. ARIMA/Kalman performed best when data gaps were small, but yielded the lowest accuracy when the number of missing data points was high (i.e. > 50%) (Figure S2). The two non-traditional temporal interpolation methods, inpainting and kriging, with years and time of year as major axes, performed comparably to or better than the ARIMA/Kalman approach, depending on the amount of missing data. Both yielded similar results and produced realistic seasonal peaks. Given the similar gap-filling performance, datasets filled by kriging were used in this study because of its statistical basis and more extensive literature support than inpainting (Shtiliyanova et al., 2017).
Monthly averaged biomass ranged from 254 g/m2 to 2650 g/m2, and gap-filling could recover missing peaks in biomass data (Figure 2). The largest gap between the end of 2011 and Spring 2013 was caused by the interval between the end of Landsat 5 and the start of Landsat 8 operations.
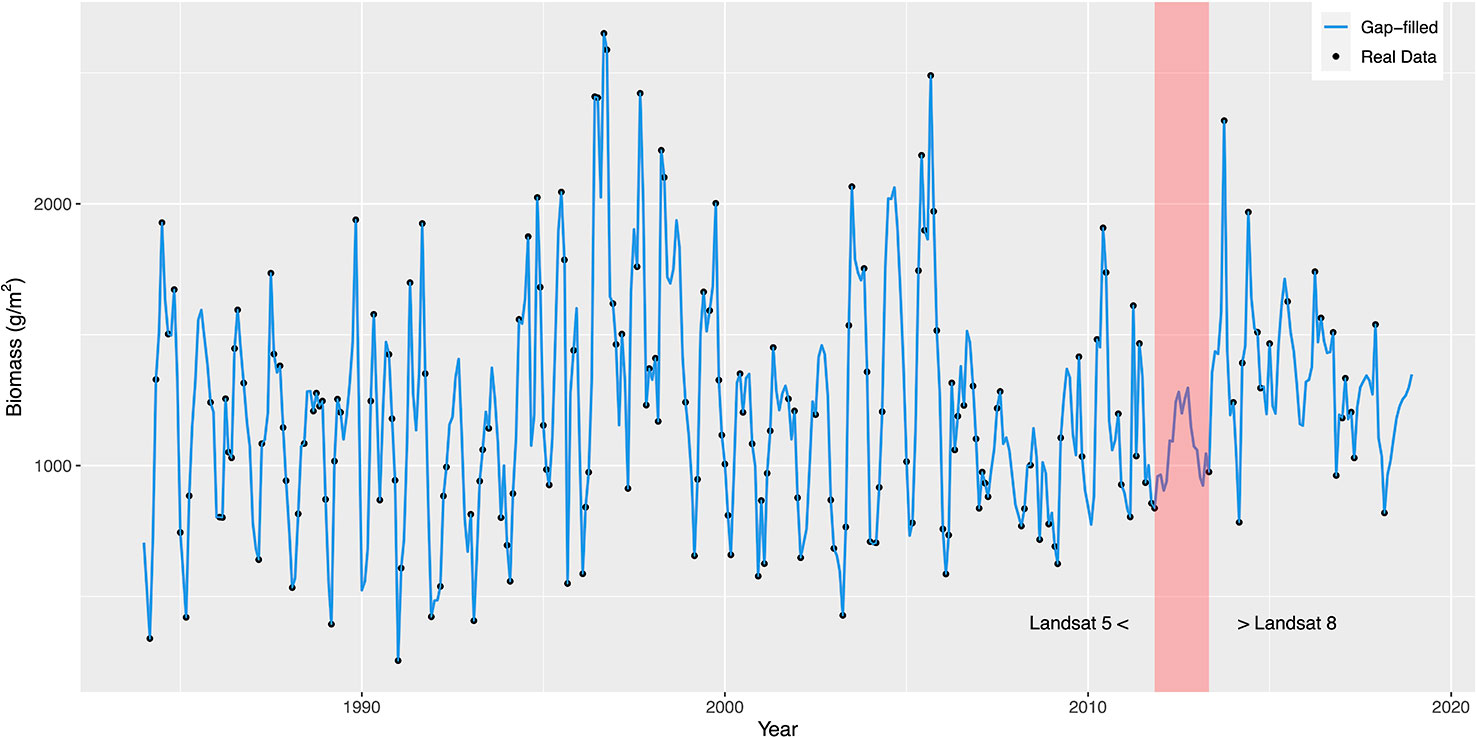
Figure 2 Monthly biomass estimates (black dots) and gap-filled data set using kriging (blue line). The red shaded area shows the gap between the operation of Landsat 5 and 8.
3.2 Observed temporal patterns
We used both wavelet analysis and EEMD to identify periodicities in the data. As described below, these yielded similar results.
Wavelet analysis of biomass identified significant periodicity at the episodic (0-4 mo), multi-month (4-8 mo), annual (8-16 mo), and multi-year (64-128 mo) scales (Figure 3A). At the sub-annual scale (0-8 mo), intermittent signals of short duration were observed between the late 1980s and late 1990s. There was a strong annual band between 1984 and 2009, after which the annual periodicity became weaker for the remainder of the time series (Figure 3A). Multi-year signals were apparent for the period of 1995-2006 (the only years not influenced by edge effects at these long periods). Similar patterns were observed using EEMD, with sub-annual cycles captured in IMF 1, the dominant annual cycle captured in IMFs 2 and 3, and the multi-year signal in IMF 5, with the rest of the IMFs contributing less to the signal (Figure 4, left). The EEMD residuals (original signal with all the modes subtracted) showed an overall increase of biomass over time, in particular prior to 2000 (Figure 4, top right).
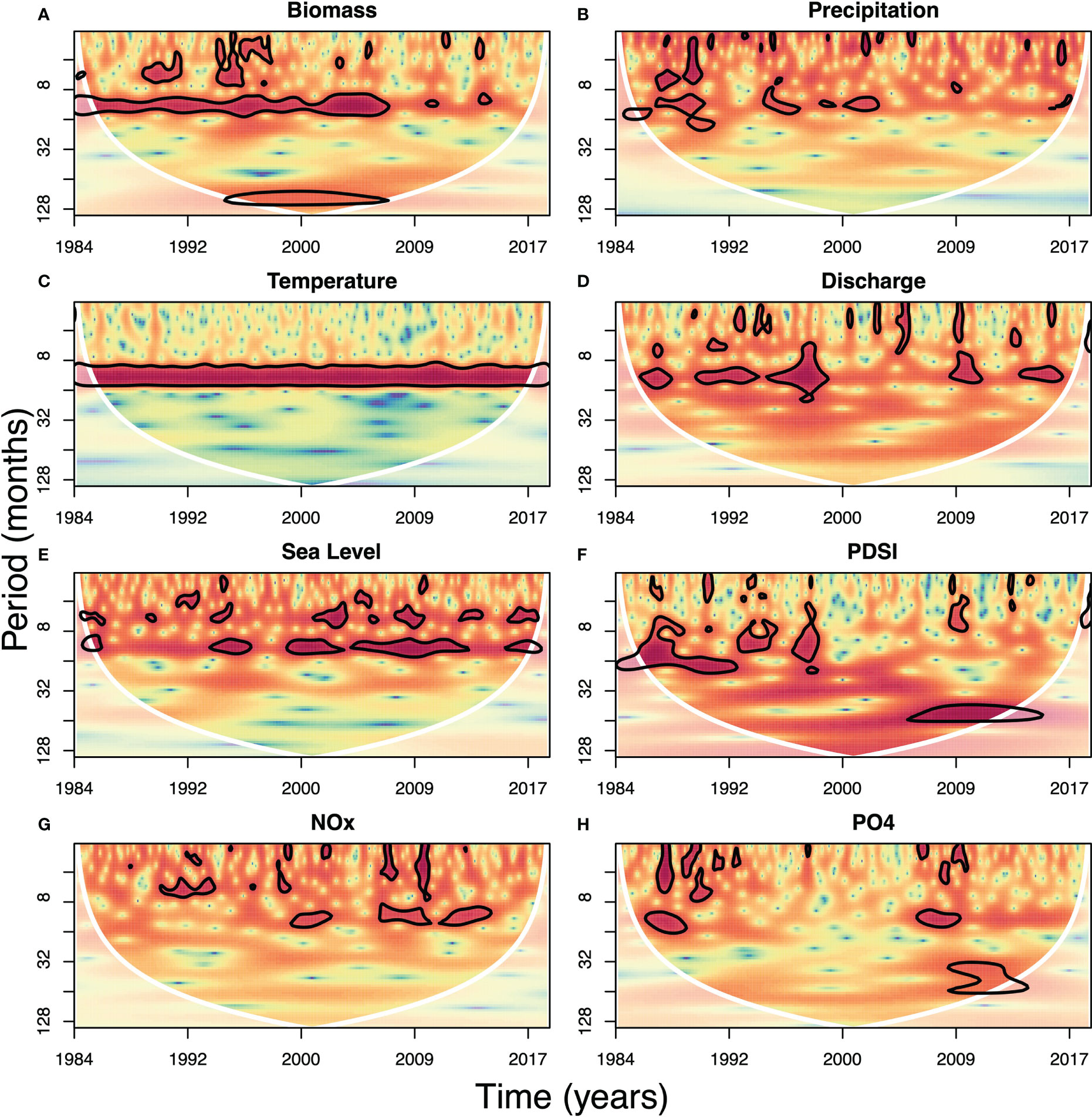
Figure 3 CWT of biomass (A), precipitation (B), temperature (C), river discharge (D), sea level (E), drought index (F), NOx (G) and PO43– (H). Coloring indicates the power of the spectra, with warmer colors indicating greater power of the signal at that time and frequency. Thick black lines indicate time-frequency regions that show significance (95% confidence level). White lines indicate the cone of influence; values in the shaded areas outside the line are prone to edge effects (Grinsted et al., 2004).
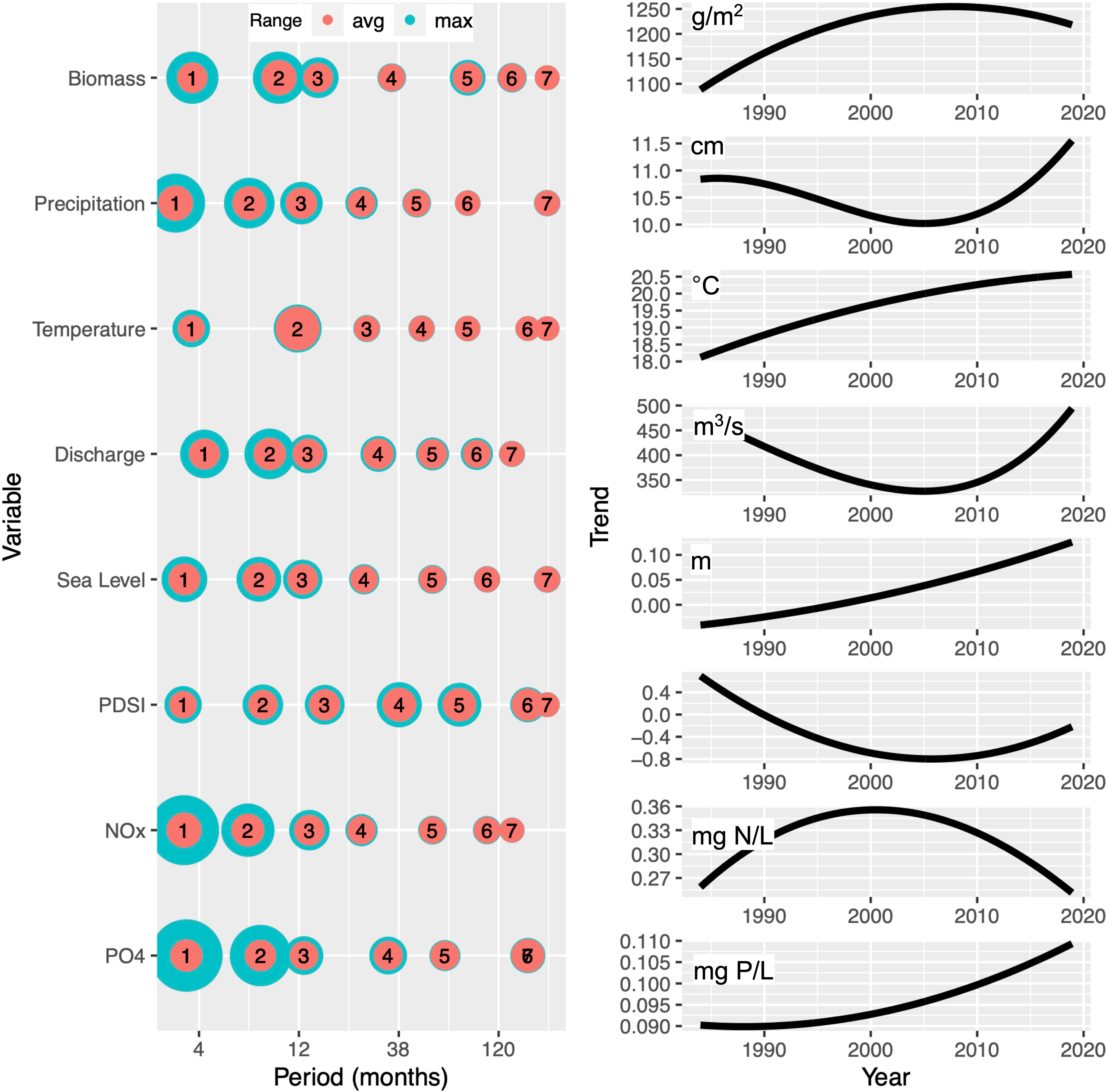
Figure 4 Relative amplitude and characteristic timescales of empirical modes derived from time series data for biomass, total monthly precipitation, temperature, river discharge, sea level, drought index and nutrients. Left column: frequency and amplitude of empirical modes. The radius of the circles reflects the average (red) and maximum (green) amplitude in each of the modes. Thus, large differences in the size of red and green circles indicate larger temporal variability of the signal strength in each mode, while for modes that exhibit a persistent signal over time, red and green circles are of similar size. The approximate signal frequency that defines the horizontal position of each mode is estimated by counting the number of maxima per observed period, which do not necessarily need to be equally spaced. Trends (right column) represent the residuals remaining after the subtraction of all IMFs from the original signal.
Monthly precipitation on Sapelo Island showed annual patterns, but the signal was discontinuous throughout the time series (Figure 3B). It also showed multiple intermittent episodic (i.e., <4 months) signals. EEMD of precipitation also showed these high frequency signals, with the largest signal contributions by intrinsic modes 1 and 2 (Figure 4, left). In line with the absence of low frequency signals in the wavelet analysis, intrinsic modes representing variations over longer timescales made a small contribution to the observed precipitation signal (Figures 4, S5 left).
Air temperature showed the expected strong and consistent annual pattern (Figure 3C). Additionally, signals with longer periodicities (i.e., >1 year) were weaker than the average signal intensities observed in other variables. This pure seasonality was also evident in the EEMD with a dominant IMF2 that exhibited a minimal difference between maximum and average range, and the remaining IMFs contributing considerably less to the observed temperature signal (Figures 4, S4 right). The EEMD residual showed an increasing trend of approximately 2°C over 35 years (Figure 4, right).
River discharge showed time-frequency patterns similar to precipitation, but with a stronger annual signal and some discontinuous sub-annual signals (Figure 3D); this pattern was also apparent in the larger amplitudes in IMFs 1, 2 and 3 (Figure 4, left). Discharge also showed higher power at lower frequencies (Figure 3D) compared to precipitation.
Annual and sub-annual periodicities in sea level were identified by both CWT and EEMD (Figures 3E, 4); however, these periodicities were intermittent, and most pronounced in 2000 - 2013. The residual in the EEMD analysis revealed the rising trend in sea level during the study period of approximately 0.15 m over the last 3 decades (Figure 4, right). Additionally, CWT for NOAA tidal projections showed high seasonality (Figure S3).
The drought index showed periodicities on the order of 8-20 months in the wavelet analysis from the 1980s until around 2000 (Figure 3F). In the second half of the time series a shift to a longer period (~5 yr) was observed. EEMD also demonstrated the slower variations of the drought index. It revealed a shift to longer period signals in the second half of the data set with decreased amplitude in IMF2 and increased contributions of IMFs 4 and 5 (Figure 3, S6 right). Additionally, the long-term trend showed intensifying drought conditions until the mid-2000s, which aligned with the decrease in precipitation and continuous increase in temperature as underlying variables (Figure 4, right). This alignment between environmental variables might have a synergistic effect on biomass; however, since our methods do not consider combined impact of variables on biomass, we could not quantify such effects.
Both riverine NOx and PO43– concentrations showed intermittent seasonality and sub-annual signals (Figures 3G, H), which resulted in larger differences between maximum and average ranges of modes 1 and 2 (Figures 4, S7).
3.3 Coherence of biomass with environmental variables
To compare the patterns in hydrological, climatic and nutrient variables with those in the biomass, we analyzed the coherence of their respective wavelets.
The strongest coherence between biomass and precipitation occurred in the annual band, reflecting the seasonality of both biomass and precipitation. Coherence was not persistent over time, and the two variables were mostly in phase (Figure 5A).
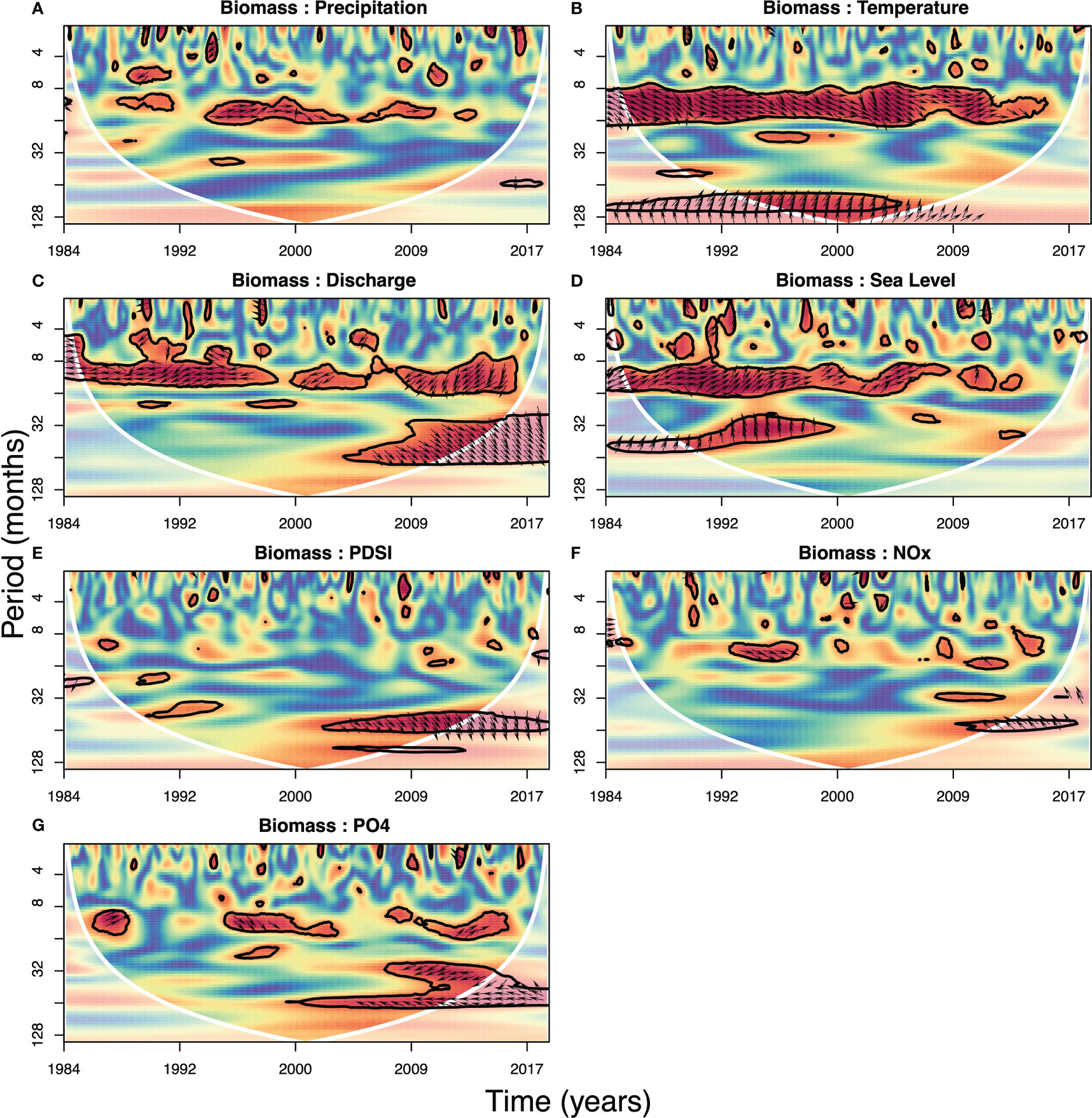
Figure 5 Wavelet coherence for biomass with other variables: precipitation (A), temperature (B), discharge (C), sea level (D), PDSI (E), NOx (F) and PO43– (G). Colors indicate the normalized wavelet coherence between two signals ranging from 1 (red, high correlation) to 0 (blue, low correlation) (Grinsted et al., 2004). Phase differences are indicated by arrows and increase from arrows pointing right (in phase, no phase difference) to arrows pointing left (anti-phase, completely out of phase). Arrows pointing down (up) mean that the environmental variable is leading biomass with one fourth (three fourth) of the period (i.e., 3 months or 9 months in the annual band). Black lines outline time-frequency couples that show significance (95% confidence level) and white lines indicate the cone of influence where values outside the line are prone to edge effects.
Temperature and biomass showed coherence in the annual band, which was significant for the period 1984-2014 and weaker afterwards (Figure 5B). Phase differences were around 0-3 months, with temperature leading biomass. This in-phase relationship reflected the strong seasonality in both signals, as increases and decreases were aligned. Additionally, longer term signals showed coherence around the 8-year period with a 6.5-year lag between temperature and biomass which was partially affected by the cone of influence.
The wavelet coherence of river discharge with biomass was high in the annual band, with most of the significant values between 1984-2016 (Figure 5C). The approximately 6-month lag between discharge and biomass is consistent with the observation that river discharge peaks in early spring and biomass peaks in early fall. After 2008, multi-year patterns (i.e., 3-6 years) of river discharge correlated with biomass, with discharge leading biomass by several months. Note though that the multi-year coherence is affected by the cone of influence and hence has limited support.
Coherence of sea level with biomass showed roughly in-phase annual patterns (Figure 5D). From 1984-2001, sea level showed coherence with biomass in longer period signals (~3 years). In these regions, sea level led biomass by approximately 2 years.
Drought index and biomass did not show an annual band in wavelet coherence (Figure 5E). The only significant patterns occurred during a 5-year period around 2009 in which PDSI led biomass by less than a year, suggesting that a wet (dry) fall period was followed by high (low) biomass the next year. The drought index was also strongly coherent and in-phase with river discharge over longer periodicities (not shown).
Nutrients generally showed intermittent coherence with biomass. NOx only had two interpretable short significant zones (annual and 5-year periods), only one of which was outside the cone of influence. This was around the mid-1990s in the annual band, where NOx led biomass by ~1.5 months. PO43– had three similar zones in the annual band, with a slight out-of-phase relationship with biomass. After the mid-2000s, PO43– showed out-of-phase coherence with biomass around 3- and 5-year periods.
3.4 Causal connections
To assess if the observed coherences between environmental factors and biomass are indicative of a causal connection, the time series observations were analyzed using convergent cross mapping (CCM). Results showed that for air temperature, river discharge, sea level, drought index and river nutrient concentrations, the prediction skill both increased with increasing library size and exceeded their linear cross correlation (Figure 6). Thus, these variables were considered causal to biomass. CCM for precipitation did not yield better prediction skill than maximum lagged cross correlation (with up to 12 months of lag) and the use of longer time series did not steadily improve the predictive power. This indicated the lack of a causal connection.
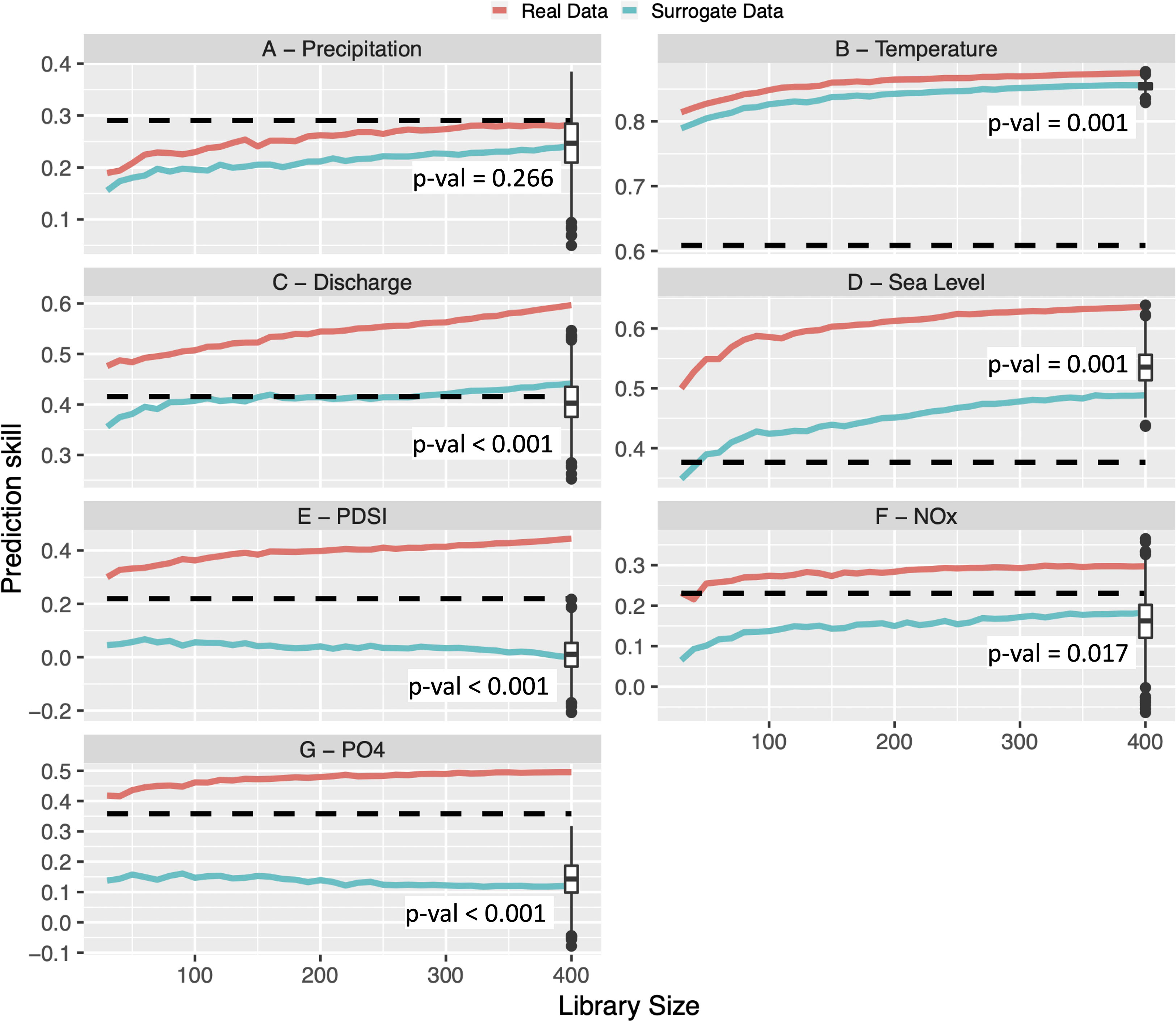
Figure 6 Convergent cross mapping results: real (red) and one instance of surrogate (blue) for each environmental variable (precipitation (A), temperature (B), discharge (C), sea level (D), PDSI (E), NOx (F) and PO43– (G)) vs biomass. Horizontal dashed black lines show the maximum cross correlation between the (time-shifted) environmental variable and biomass. Library size represents the length of the time series used in CCM. The prediction skill measures the strength of the connection between environmental variables and biomass. Zero or negative prediction skill indicates the lack of information about the dynamics of the environmental variable embedded in biomass (Sun et al., 2021). Boxes and whiskers show the range of prediction skill for surrogate data, interquartile range, median and outliers (significance tests comparing surrogate and observed data were run with full library size).
Tests with surrogate signals were performed to investigate if the apparent causal connections were a result of the inherent seasonal cycles in most of the variables. The results indicated that temperature, river discharge, and sea level data performed significantly better than their seasonal surrogates (p-value <0.05). Similarly, and unsurprising given their weak annual bands (Figures 5E–G), the causal connections identified from the drought index and nutrients (NOx and PO43–) to biomass were not due to seasonality. CCM for precipitation did not exhibit prediction skills significantly better than its surrogate, which further supported the lack of causal connection between precipitation and biomass.
4 Discussion
Aboveground biomass data exhibited strong seasonality and a general increase over time (Figure 2). The decomposition of the timeseries using EEMD pointed to a long-term trend characterized by a substantial increase prior to 2007, with a plateau or slight decrease thereafter (Figure 4). Wavelet analysis also indicated intermittent sub-annual as well as multi-year periodicities in the above-ground biomass (Figure 3A).
4.1 Patterns in environmental variables and their coherence with biomass
To put these observations into context, the patterns in environmental factors potentially affecting plant growth are identified and discussed, and then analyzed regarding their correlation in time-frequency space with that of aboveground biomass using wavelet coherence.
Short-term precipitation signals observed in CWT and captured in the first intrinsic mode reflect the episodic nature of precipitation and fronts generated by storm events (Srock and Bosart, 2009). The wavelet analysis did not reveal significant patterns at longer timescales. However, previous studies have shown that southeastern US precipitation depends on large-scale climate patterns such as the North Atlantic Oscillation, Bermuda High Index, El Niño/Southern Oscillation, and the Pacific Decadal Oscillation (Sheldon and Burd, 2014). Among these, the Bermuda High Index is most influential, especially during drought events when it is negative, pointing to westward migrating high pressure areas that block storm impact on the east coast (Sheldon and Alber, 2013). However, important large-scale oscillations often vary intermittently over longer periods and variable periodicities (e.g., the North Atlantic Oscillation; Markovic and Koch, 2005) and hence may not appear as a consistent pattern in the wavelet scalograms (Figure 3B).
Our analyses indicate an alignment of annual peaks in precipitation and biomass (Figure 5A). This might reflect that precipitation can be beneficial for biomass as it freshens the marsh environment. Additionally, analysis of precipitation and CO2 fluxes in a salt marsh (Chu et al., 2021) showed high wavelet coherence in the annual band and revealed the importance of early growing season precipitation. However, precipitation can also act as a stressor. For example, Hanson et al. (2016) have demonstrated the effect of precipitation patterns by showing that S. alterniflora biomass under ambient daily precipitation exceeded that subjected to biweekly storms (with similar amount of total rain) or drought conditions. This indicates that the nature of precipitation events is more important than total amount of precipitation and therefore extreme events might weaken the seasonal precipitation/biomass coherence.
The temperature signal was strongly and consistently seasonal (Figure 3C) and EEMD showed an increase of approximately 2°C over 35 years (Figure 4, right). This is consistent with long-term warming in the state of Georgia of approximately 3°F since the late 1970s and an overall trend of the Georgia coast warming faster than the state as a whole (Frankson et al., 2022; U.S. EPA, 2022).
Biomass peaks aligned with the temperature peaks, reflecting both of their seasonal cycles. Both biomass and temperature also exhibited an increase over the study period, which suggests a positive relationship between temperature and plant growth. However, the long-term trend in the biomass data indicated a maximum around 2008 while temperatures continue to rise (Figure 4). This points to a potential decoupling between these two variables, which can also be seen in the lower power of the wavelet coherence in the annual band after 2015 (Figure 5B). A positive relationship between temperature and plant aboveground biomass may not persist if temperatures increase to levels that decrease plant productivity (35°C, Giurgevich and Dunn, 1979). Such negative impacts are more likely to occur in more productive southern salt marshes studied here, which are closer to their optimum temperatures than northern Atlantic coast marshes (Kirwan et al., 2009).
Discharge showed both annual and longer-term patterns. The annual patterns reflected the historical spring maximum (Alber and Sheldon, 1999). Over longer time scales, there was a drop in discharge between 2000 and 2012 (Figure 4, right). This may reflect the potential combined impact of climatic and anthropogenic changes (Takagi et al., 2017). We observed higher power in lower frequency signals for river discharge compared to precipitation (Figures 3B–D). This is likely due to the fact that river discharge captures and integrates local effects across the entire watershed, whereas our precipitation data was from a single station on the coast of Georgia. Furthermore, seasonality in evapotranspiration rates may decouple precipitation and discharge on sub-annual timescales.
Coherence of biomass and river discharge shows that with some lag, an increase in discharge leads to an increase in biomass. This can be explained by the effect of discharge on estuarine mixing and the salinity and chemical composition of water that floods the intertidal marshes. The salinity of this water is mainly controlled by the Altamaha River which causes freshening of this estuarine system when discharge is increased (Di Iorio and Castelao, 2013). Consequently, it also affects the porewater salinity, which is an important driver of S. alterniflora production (Odum, 1988; Więski and Pennings, 2014; Miklesh and Meile, 2018). This aligns well with the observed stronger coherence of biomass with discharge than with precipitation.
Sea level showed annual and sub-annual signals as well as longer-term trends. Much of the annual and sub-annual signals in sea level can be attributed to tides (e.g., perigean spring tides), which can be seen by comparing the analyses of sea level with/without removal of the projected tides (Figures S3, 3E). However, discontinuities in significance levels in these bands indicate cases where discrete weather events overwhelmed tidal effects. For example, Andres et al. (2013) have shown that local forcings such as along-shelf wind stress caused interannual variability in sea level along the US east coast. These types of local forcings are highly variable; therefore, they manifest themselves as intermittent pulses and disturbances in the time series, but do not generate persistent signals in the scalograms. The long-term increase in sea level indicated by the EEMD residual was 0.15 m over the last 3 decades (Figure 4; i.e., 5 mm/year) which was comparable to the increase of 3.3 mm/year from 1983 to 2001 reported by Sweet et al. (2022) and showed acceleration in the last 2 decades.
Sea level had considerable seasonal, close to in-phase coherence with biomass, indicating that biomass increases with increased sea level. This positive impact of sea level was reported in previous studies (Więski and Pennings, 2014; O’Donnell and Schalles, 2016); however, this effect was shown to be sensitive to depth and duration of the flooding and can reverse in the long run with certain thresholds exceeded (Morris et al., 2013). This implication was also similar to the findings of Wei et al. (2020), who have shown lagged seasonal and multi-day coherence of tide height and CO2 fluxes in a salt marsh, and Souza et al. (2022) who documented both in and out of phase coherence between water level and CO2 fluxes on semi-diurnal (in phase) and multi-day (out of phase) timescales. Our analysis also revealed long period coherences of biomass with temperature (~ 8 years) and sea level (~3-4 years), with lags on the order of >6 years for temperature and approximately 2 years for sea level (Figure 5). These lags are long compared to the Spartina growth cycle and therefore may not reflect biological interactions with biomass.
Periodicities in the drought index shifted from shorter (8-20 months) to longer (5 yr) around 2000. This may reflect recurring extended drought periods, which decreased the impact of seasonality in the PDSI signal (Figure 3; Figure 4, IMFs 4,5). Long-term patterns in drought and river discharge were similar, highlighting their regional and integrative nature (Figure 3). The correlation between biomass and PDSI as reflected in their wavelet coherence points to the potential impact of droughts. Droughts are known to be associated with large-scale dieback (Alber et al., 2008), and there have been several such events on the GA coast since 2000. Such effects may not be immediate but propagate through feedbacks between above- and below-ground biomass which are tightly connected (Schubauer and Hopkinson, 1984; O’Connell et al., 2021). Note that size-class partitioning of central Georgia Spartina alterniflora (O’Donnell and Schalles, 2016; Zinnert et al., 2021) showed that high-marsh, short form Spartina (canopy < 50 cm) and mid-marsh medium form (canopy 50 – 100 cm) had less resistance to drought-induced biomass declines than low-marsh tall form (canopy > 100 cm) during the 5-yr severe drought event centered around 2000. The shorter forms of Spartina live in naturally stressed, higher pore water salinity areas of the elevation-graded marsh platforms and have lower average above- and below-ground biomass.
Nutrient concentrations in the Altamaha River are affected by increasing anthropogenic inputs resulting from agriculture, livestock, and changes in population density modulated by in-stream processes (i.e., biological/benthic uptake/release, ad-/de-sorption, precipitation/dissolution, dilution) (Schaefer and Alber, 2007; Takagi et al., 2017). Nutrient concentrations were not highly sensitive to discharge (NO3–: non-significant, DIP: slightly negative significant relationship between discharge and concentration) (Takagi et al., 2017). This could explain the weaker annual band in nutrients compared to discharge (Figure 3). Under drought conditions, short-term nutrient pulses can occur when storms/heavy rains cause flushing of retained nutrients from the terrestrial environment before it is diluted by a high flow event afterwards (Figure 4, left, IMFs 1 and 2; Whitehead et al., 2009).
Nutrients showed intermittent coherence patterns with biomass mostly similar to coherence patterns of PDSI and discharge. However, differences in phase lags point to differences in underlying processes (Figure 5).
4.2 Causality
The causal relationships identified in this study generally align well with observed coherences between environmental variables and saltmarsh biomass described above. Analyses showed that temperature, sea level, discharge, drought, and nutrients were causal to biomass while local precipitation was not.
Regression analyses from previous studies are consistent with our findings. Więski and Pennings (2014), working with annual data between 2000 and 2011, showed that river discharge and sea level best predict salt marsh productivity in salt marshes around the Altamaha River estuary. This is likely due to their impacts on porewater salinity and plant stress, which can be the potential mechanism for the causal connection that we observed in CCM for river discharge and sea level. In this analysis precipitation had only limited correlation with production. O’Donnell and Schalles (2016) came to similar conclusions based on an analysis of Landsat 5 data between 1984 and 2011. They found that river discharge, total precipitation, minimum temperature, and mean sea level were important predictors of aboveground Spartina alterniflora on the Central Georgia Coast. This generally agrees with our finding except for precipitation. Although we observed a temporary seasonal coherence with biomass, a causal relationship was not supported by our analysis. Additionally, PDSI was reported as a predictor in combination with other variables only during limited time periods; our results suggest that drought index is indeed causal to biomass. It has been suggested that the effect of drought on biomass can be through biogeochemical factors (salinity, pH, Alber et al., 2008) and can also intensify ecological controls (e.g., grazing pressure, Silliman et al., 2005). We note that the coherence between the biomass and drought is only strong during the second half of the observational period when drought conditions lasted longer, potentially suggesting that there is a minimum duration required to impact the marsh vegetation.
Spartina alterniflora has been shown to be sensitive to N supply (Morris et al., 2013). Although N is the main limiting nutrient in salt marsh ecosystems, P can also be limiting, as low PO43– availability can restrict nutrient replenishment by microbial organisms, in turn impacting carbon fixation in marsh plants (Sundareshwar et al., 2003; Rolando et al., 2022). Supporting these findings, our analysis showed that both nutrients are causal to salt marsh biomass. However, nutrient concentrations on the marsh will differ from those measured at the monitoring station in the freshwater reaches of the Altamaha due to mixing with oceanic water, in-stream processing of nutrients, and local sources and sinks such as those due to nitrogen fixation, nitrogen uptake and denitrification. Considering dilution of riverine nutrients resulted in different causal strength values but still shows the causal connections of nutrients to the marsh biomass (Figure S10). Additionally, the causality of the nutrients was sensitive to the gap-filling procedure for the biomass data (Figure S11). These findings emphasize the need for more detailed experimental studies of the causal relationship between nutrients and biomass beyond the analysis of time series.
5 Conclusion
Our work aimed to characterize complex time series from an estuarine environment where seasonal patterns and non linearity are key characteristics. It differed from autoregressive models and neural networks (e.g., Lim and Zohren, 2021) in that it focused on pattern identification and causal inferences; and from linear correlation and regression methods (e.g., Wu et al., 2015) in that our approach accounted for the nonlinear and state-dependent nature of causal relationships (Papagiannopoulou et al., 2017). We successfully used kriging to fill gaps in time series with seasonality.
Using time series analysis methods only, we showed dominant seasonal forcings and short- and long- frequency patterns embedded in salt marsh aboveground biomass and relevant abiotic variables; identified temporal lags between these patterns; and identified variables that are causal to salt marsh biomass. Longer-term trends revealed that increases in temperature and sea level were accompanied by an increase in aboveground biomass from 1984 through 2008.
Our results indicated that salt marsh biomass is coherent with seasonal patterns in temperature and sea level with small- or no-time lags but with longer lagged river discharge, reflecting the natural annual rhythm of plant dynamics and hydrology. Some environmental characteristics such as temperature and sea level also showed coherence with biomass at long periods with multi-year lags. Although such patterns could be spurious, they may well reflect complex interactions, such as those between marsh inundation, productivity, and biomass, with feedback between sea level rise, marsh production, accretion, and mineral and organic matter accumulation (e.g., Morris et al., 2013; Więski and Pennings, 2014).
The causal relationships identified in this study support previous findings and align with observed coherences between aboveground biomass and environmental variables (temperature, sea level, river discharge, drought, and nutrients). We showed that causal connections were not due to seasonality. Our work exemplifies the use of long time series to discover known and new ecological interactions in complex and highly seasonal natural systems.
Data availability statement
The datasets presented in this study can be found in online repositories. The names of the repository/repositories and accession number(s) can be found below: Data can be found in GCE-LTER data base with this link: http://dx.doi.org/10.6073/pasta/113d13d7192e24e6b67b11fc851705dd. Scripts used for analyses can be found here: https://bitbucket.org/MeileLab/kadir_altamaha/src/master/.
Author contributions
CM and KB conceived the original work. JS provided the original biomass data and study area map. KB carried out the data analyses and drafted the article with input from CM. JS, JES, MA and CM all critically revised the manuscript and contributed to the discussion of results. All authors reviewed and approved the final version.
Funding
This work was supported by the US National Science Foundation under grant OCE-1832178. This is UGAMI publication # 1114.
Acknowledgments
We thank Dr. E. Deyle for an introduction to the convergent cross mapping method.
Conflict of interest
The authors declare that the research was conducted in the absence of any commercial or financial relationships that could be construed as a potential conflict of interest.
Publisher’s note
All claims expressed in this article are solely those of the authors and do not necessarily represent those of their affiliated organizations, or those of the publisher, the editors and the reviewers. Any product that may be evaluated in this article, or claim that may be made by its manufacturer, is not guaranteed or endorsed by the publisher.
Supplementary material
The Supplementary Material for this article can be found online at: https://www.frontiersin.org/articles/10.3389/fmars.2023.1130958/full#supplementary-material
References
Abdul-Aziz O. I., Ishtiaq K. S., Tang J., Moseman-Valtierra S., Kroeger K. D., Gonneea M. E., et al. (2018). Environmental controls, emergent scaling, and predictions of greenhouse gas (GHG) fluxes in coastal salt marshes. J. Geophys Research: Biogeosciences 123 (7), 2234–2256. doi: 10.1029/2018JG004556
Afrifa-Yamoah E., Mueller U. A., Taylor S. M., Fisher A. J. (2020). Missing data imputation of high-resolution temporal climate time series data. Meteorological Appl. 27 (1), e1873. doi: 10.1002/met.1873
Alber M., Sheldon J. E. (1999). Use of a date-specific method to examine variability in the flushing times of Georgia estuaries. Estuarine Coast. Shelf Sci. 49 (4), 469–482. doi: 10.1006/ecss.1999.0515
Alber M., Swenson E. M., Adamowicz S. C., Mendelssohn I. A. (2008). Salt marsh dieback: an overview of recent events in the US. Estuarine Coast. Shelf Sci. 80 (1), 1–11. doi: 10.1016/j.ecss.2008.08.009
Alexander C., Hladik C. M. (2015). High-resolution mapping of vegetation, elevation, salinity and bathymetry to advance coastal habitat management in Georgia. Final Rep. submitted to Georgia Department Natural Resources Georgia Coast. Manage. Program 63 p.
Andres M., Gawarkiewicz G. G., Toole J. M. (2013). Interannual sea level variability in the western north Atlantic: Regional forcing and remote response. Geophys Res. Lett. 40 (22), 5915–5919. doi: 10.1002/2013GL058013
Bonotto G., Peterson T. J., Fowler K., Western A. W. (2022). Identifying causal interactions between groundwater and streamflow using convergent cross-mapping. Water Resour. Res. 58 (8), e2021WR030231. doi: 10.1029/2021WR030231
Box G. E., Jenkins G. M., Reinsel G. C., Ljung G. M. (2015). Time series analysis: forecasting and control (Hoboken, NJ: John Wiley & Sons), 784p.
Chu X., Han G., Wei S., Xing Q., He W., Sun B., et al. (2021). Seasonal not annual precipitation drives 8-year variability of interannual net CO2 exchange in a salt marsh. Agric. For. Meteorol 308, 108557. doi: 10.1016/j.agrformet.2021.108557
Crosby S. C., Angermeyer A., Adler J. M., Bertness M. D., Deegan L. A., Sibinga N., et al. (2017). Spartina alterniflora biomass allocation and temperature: implications for salt marsh persistence with sea-level rise. Estuaries Coasts 40 (1), 213–223. doi: 10.1007/s12237-016-0142-9
D'Errico J. (2022) MATLAB central file exchange. Available at: https://www.mathworks.com/matlabcentral/fileexchange/4551-inpaint_nans.
Deyle E. R., Maher M. C., Hernandez R. D., Basu S., Sugihara G. (2016). Global environmental drivers of influenza. Proc. Natl. Acad. Sci. 113 (46), 13081–13086. doi: 10.1073/pnas.1607747113
Di Iorio D., Castelao R. M. (2013). The dynamical response of salinity to freshwater discharge and wind forcing in adjacent estuaries on the Georgia coast. Oceanography 26 (3), 44–51. doi: 10.5670/oceanog.2013.44
Dorich C. D., De Rosa D., Barton L., Grace P., Rowlings D., Migliorati M. D. A., et al. (2020). Global research alliance N2O chamber methodology guidelines: Guidelines for gap-filling missing measurements. J. Environ. Qual. 49 (5), 1186–1202. doi: 10.1002/jeq2.20138
Feher L. C., Osland M. J., Griffith K. T., Grace J. B., Howard R. J., Stagg C. L., et al. (2017). Linear and nonlinear effects of temperature and precipitation on ecosystem properties in tidal saline wetlands. Ecosphere 8 (10), e01956. doi: 10.1002/ecs2.1956
Frankson R., Kunkel K. E., Stevens L. E., Stewart B. C., Sweet W., Murphey B., et al. (2022). Georgia State climate summary 2022. NOAA technical report NESDIS 150-GA (NOAA/NESDIS, Silver Spring, MD), 5 pp.
Giurgevich J. R., Dunn E. L. (1979). Seasonal patterns of CO2 and water vapor exchange of the tall and short height forms of Spartina alterniflora loisel in a Georgia salt marsh. Oecologia 43 (2), 139–156. doi: 10.1007/BF00344767
Grinsted A., Moore J. C., Jevrejeva S. (2004). Application of the cross wavelet transform and wavelet coherence to geophysical time series. Nonlinear Processes Geophysics 11 (5/6), 561–566. doi: 10.5194/npg-11-561-2004
Hanson A., Johnson R., Wigand C., Oczkowski A., Davey E., Markham E. (2016). Responses of Spartina alterniflora to multiple stressors: changing precipitation patterns, accelerated sea level rise, and nutrient enrichment. Estuaries Coasts 39 (5), 1376–1385. doi: 10.1007/s12237-016-0090-4
Huang N. E., Shen Z., Long S. R., Wu M. C., Shih H. H., Zheng Q., et al. (1998). The empirical mode decomposition and the Hilbert spectrum for nonlinear and non-stationary time series analysis. Proc. R. Soc. London. Ser. A: Mathematical Phys. Eng. Sci. 454 (1971), 903–995. doi: 10.1098/rspa.1998.0193
Kirwan M. L., Guntenspergen G. R., Morris J. T. (2009). Latitudinal trends in Spartina alterniflora productivity and the response of coastal marshes to global change. Global Change Biol. 15 (8), 1982–1989. doi: 10.1111/j.1365-2486.2008.01834.x
Knotters M., Heuvelink G. B. M. (2010). A disposition of interpolation techniques (No. 190). Wettelijke Onderzoekstaken Natuur & Milieu.
Lau K. M., Weng H. (1995). Climate signal detection using wavelet transform: How to make a time series sing. Bull. Am. Meteorological Soc. 76 (12), 2391–2402. doi: 10.1175/1520-0477(1995)076<2391:CSDUWT>2.0.CO;2
Lawrimore J. H., Ray R., Applequist S., Korzeniewski B., Menne M. J. (2016) Global summary of the month (GSOM), version 1. SAPELO ISLAND, GA US USC00097808 (NOAA National Centers for Environmental Information). 10.7289/V5QV3JJ5 (Accessed 31, 2022).
Lepot M., Aubin J. B., Clemens F. H. (2017). Interpolation in time series: An introductive overview of existing methods, their performance criteria and uncertainty assessment. Water 9 (10), 796. doi: 10.3390/w9100796
Li H., Dai S., Ouyang Z., Xie X., Guo H., Gu C., et al. (2018). Multi-scale temporal variation of methane flux and its controls in a subtropical tidal salt marsh in eastern China. Biogeochemistry 137 (1), 163–179. doi: 10.1007/s10533-017-0413-y
Li J., Heap A. D. (2008). A review of spatial interpolation methods for environmental scientists. Geosci. Aust. 2008/23, 137.
Lim B., Zohren S. (2021). Time-series forecasting with deep learning: a survey. Philos. Trans. R. Soc. A 379 (2194), 20200209. doi: 10.1098/rsta.2020.0209
Markovic D., Koch M. (2005). Wavelet and scaling analysis of monthly precipitation extremes in Germany in the 20th century: Interannual to interdecadal oscillations and the North Atlantic Oscillation influence. Water Resour. Res. 41 (9), W09420. doi: 10.1029/2004WR003843
Mcleod E., Chmura G. L., Bouillon S., Salm R., Björk M., Duarte C. M., et al. (2011). A blueprint for blue carbon: toward an improved understanding of the role of vegetated coastal habitats in sequestering CO2. Front. Ecol. Environ. 9 (10), 552–560. doi: 10.1890/110004
Miklesh D., Meile C. (2018). Porewater salinity in a southeastern united states salt marsh: controls and interannual variation. PeerJ 6, e5911. doi: 10.7717/peerj.5911
Moritz S., Bartz-Beielstein T. (2017). imputeTS: time series missing value imputation in r. R J 9, 1, 207. doi: 10.32614/RJ-2017-009
Morris J. T., Sundareshwar P. V., Nietch C. T., Kjerfve B., Cahoon D. R. (2002). Responses of coastal wetlands to rising sea level. Ecology 83 (10), 2869–2877. doi: 10.1890/0012-9658(2002)083[2869:ROCWTR]2.0.CO;2
Morris J. T., Sundberg K., Hopkinson C. S. (2013). Salt marsh primary production and its responses to relative sea level and nutrients in estuaries at plum island, Massachusetts, and north inlet, south Carolina, USA. Oceanography 26 (3), 78–84. doi: 10.5670/oceanog.2013.48
NOAA National Centers for Environmental information (2022) Climate at a glance: Divisional time series. Available at: https://www.ncdc.noaa.gov/cag/.
NOAA Tides and Currents (2022a) 8670870 fort pulaski, GA. observed water levels. Available at: https://tidesandcurrents.noaa.gov/waterlevels.html?id=8670870 (Accessed 31, 2022).
NOAA Tides and Currents (2022b) 8670870 fort pulaski, GA. tide predictions. Available at: https://tidesandcurrents.noaa.gov/noaatidepredictions.html?id=8670870 (Accessed 31, 2022).
O’Connell J. L., Mishra D. R., Alber M., Byrd K. B. (2021). BERM: a belowground ecosystem resiliency model for estimating Spartina alterniflora belowground biomass. New Phytol. 232 (1), 425–439. doi: 10.1111/nph.17607
O’Donnell J. P., Schalles J. F. (2016). Examination of abiotic drivers and their influence on Spartina alterniflora biomass over a twenty-eight year period using landsat 5 TM satellite imagery of the central Georgia coast. Remote Sens. 8 (6), 477. doi: 10.3390/rs8060477
Odum W. E. (1988). Comparative ecology of tidal freshwater and salt marshes. Annu. Rev. Ecol. systematics 19 (1), 147–176. doi: 10.1146/annurev.es.19.110188.001051
Papagiannopoulou C., Miralles D. G., Decubber S., Demuzere M., Verhoest N. E., Dorigo W. A., et al. (2017). A non-linear Granger-causality framework to investigate climate–vegetation dynamics. Geoscientific Model. Dev. 10 (5), 1945–1960. doi: 10.5194/gmd-10-1945-2017
Pebesma E. J. (2004). Multivariable geostatistics in s: the gstat package. Comput. Geosciences 30 (7), 683–691. doi: 10.1016/j.cageo.2004.03.012
Reed D. C., Schmitt R. J., Burd A. B., Burkepile D. E., Kominoski J. S., McGlathery K. J., et al. (2022). Responses of coastal ecosystems to climate change: Insights from long-term ecological research. BioScience 72 (9), 871–888. doi: 10.1093/biosci/biac006
Rolando J. L., Kolton M., Song T., Kostka J. E. (2022). The core root microbiome of Spartina alterniflora is predominated by sulfur-oxidizing and sulfate-reducing bacteria in Georgia salt marshes, USA. Microbiome 10 (1), 1–17. doi: 10.1186/s40168-021-01187-7
Schaefer S. C., Alber M. (2007). Temporal and spatial trends in nitrogen and phosphorus inputs to the watershed of the altamaha river, Georgia, USA. Biogeochemistry 86 (3), 231–249. doi: 10.1007/s10533-007-9155-6
Schubauer J. P., Hopkinson C. S. (1984). Above-and belowground emergent macrophyte production and turnover in a coastal marsh ecosystem, Georgia 1. Limnol Oceanogr 29 (5), 1052–1065. doi: 10.4319/lo.1984.29.5.1052
Sheldon J. E., Alber M. (2013). Effects of climate signals on river discharge to ossabaw, st. Andrew, and Cumberland sounds (Athens, GA: Georgia Coastal Research Council, Prepared for GA DNR – Coastal Resources Division), 1–14.
Sheldon J. E., Burd A. B. (2014). Alternating effects of climate drivers on altamaha river discharge to coastal Georgia, USA. Estuaries Coasts 37 (3), 772–788. doi: 10.1007/s12237-013-9715-z
Shtiliyanova A., Bellocchi G., Borras D., Eza U., Martin R., Carrère P. (2017). Kriging-based approach to predict missing air temperature data. Comput. Electron. Agric. 142, 440–449. doi: 10.1016/j.compag.2017.09.033
Silliman B. R., Van De Koppel J., Bertness M. D., Stanton L. E., Mendelssohn I. A. (2005). Drought, snails, and large-scale die-off of southern US salt marshes. Science 310 (5755), 1803–1806. doi: 10.1126/science.1118229
Souza R. B., Copertino M. S., Fisch G., Santini M. F., Pinaya W. H., Furlan F. M., et al. (2022). Salt marsh-atmosphere CO2 exchanges in patos lagoon estuary, southern Brazil. Front. Mar. Sci. 9, 892857. doi: 10.3389/fmars.2022.892857
Srock A. F., Bosart L. F. (2009). Heavy precipitation associated with southern Appalachian cold-air damming and Carolina coastal frontogenesis in advance of weak landfalling tropical storm Marco, (1990). Monthly Weather Rev. 137 (8), 2448–2470. doi: 10.1175/2009MWR2819.1
Sugihara G., May R., Ye H., Hsieh C. H., Deyle E., Fogarty M., et al. (2012). Detecting causality in complex ecosystems. science 338 (6106), 496–500. doi: 10.1126/science.1227079
Sun X., Fang W., Gao X., An S., Liu S., Wu T. (2021). Time-varying causality inference of different nickel markets based on the convergent cross mapping method. Resour. Policy 74, 102385. doi: 10.1016/j.resourpol.2021.102385
Sundareshwar P. V., Morris J. T., Koepfler E. K., Fornwalt B. (2003). Phosphorus limitation of coastal ecosystem processes. Science 299 (5606), 563–565. doi: 10.1126/science.1079100
Sweet W. V., Hamlington B. D., Kopp R. E., Weaver C. P., Barnard P. L., Bekaert D., et al. (2022). “Global and regional Sea level rise scenarios for the united states: Updated mean projections and extreme water level probabilities along U.S. coastlines,” in NOAA Technical report NOS 01 (National Oceanic and Atmospheric Administration, National Ocean Service, Silver Spring, MD), 111. Available at: https://oceanservice.noaa.gov/hazards/sealevelrise/noaa-nos-techrpt01-global-regional-SLR-scenarios-US.pdf.
Takagi K. K., Hunter K. S., Cai W. J., Joye S. B. (2017). Agents of change and temporal nutrient dynamics in the altamaha river watershed. Ecosphere 8 (1), e01519. doi: 10.1002/ecs2.1519
Takens F. (1981). “Detecting strange attractors in turbulence,” in Dynamical systems and turbulence, Warwick 1980 (Berlin, Heidelberg: Springer), 366–381.
U.S. Environmental Protection Agency (2022). “Climate change indicators in the united states,” in Rate of temperature change in the united states, 1901–2021. Available at: https://www.epa.gov/climate-indicators/climate-change-indicators-us-and-global-temperature.
U.S. Geological Survey (2016) National water information system data available on the world wide web (USGS water data for the nation). Available at: https://waterdata.usgs.gov/nwis/uv/?site_no=02226000 (Accessed 31, 2022).
U.S. Geological Survey (2016b) National water information system data available on the world wide web (USGS water data for the nation). Available at: https://nwis.waterdata.usgs.gov/usa/nwis/qwdata/?site_no=02226010 (Accessed 31, 2022).
Visser J. M., Sasser C. E., Cade B. S. (2006). The effect of multiple stressors on salt marsh end-of-season biomass. Estuaries Coasts 29 (2), 328–339. doi: 10.1007/BF02782001
Walter O. Y., Kihoro J. M., Athiany K. H. O., Kibunja H. W. (2013). Imputation of incomplete non-stationary seasonal time series data. Mathematical theory and modeling 3 (12), 142–154.
Wei S., Han G., Jia X., Song W., Chu X., He W., et al. (2020). Tidal effects on ecosystem CO2 exchange at multiple timescales in a salt marsh in the yellow river delta. Estuarine Coast. Shelf Sci. 238, 106727. doi: 10.1016/j.ecss.2020.106727
Whitehead P. G., Wilby R. L., Battarbee R. W., Kernan M., Wade A. J. (2009). A review of the potential impacts of climate change on surface water quality. Hydrol Sci. J. 54 (1), 101–123. doi: 10.1623/hysj.54.1.101
Więski K., Pennings S. C. (2014). Climate drivers of Spartina alterniflora saltmarsh production in Georgia, USA. Ecosystems 17 (3), 473–484. doi: 10.1007/s10021-013-9732-6
Wu Z., Huang N. E. (2009). Ensemble empirical mode decomposition: a noise-assisted data analysis method. Adv. adaptive Data Anal. 1 (01), 1–41. doi: 10.1142/S1793536909000047
Wu D., Zhao X., Liang S., Zhou T., Huang K., Tang B., et al. (2015). Time-lag effects of global vegetation responses to climate change. Global Change Biol. 21 (9), 3520–3531. doi: 10.1111/gcb.12945
Yang A. C., Peng C. K., Huang N. E. (2018). Causal decomposition in the mutual causation system. Nat. Commun. 9 (1), 1–10. doi: 10.1038/s41467-018-05845-7
Ye H., Deyle E. R., Gilarranz L. J., Sugihara G. (2015). Distinguishing time-delayed causal interactions using convergent cross mapping. Sci. Rep. 5 (1), 1–9. doi: 10.1038/srep14750
Keywords: salt marsh, biomass, time series, wavelet, causality
Citation: Biçe K, Schalles J, Sheldon JE, Alber M and Meile C (2023) Temporal patterns and causal drivers of aboveground plant biomass in a coastal wetland: Insights from time-series analyses. Front. Mar. Sci. 10:1130958. doi: 10.3389/fmars.2023.1130958
Received: 24 December 2022; Accepted: 22 March 2023;
Published: 06 April 2023.
Edited by:
Alberto Basset, University of Salento, ItalyReviewed by:
David Burdick, University of New Hampshire, United StatesSerena Moseman-Valtierra, University of Rhode Island, United States
Copyright © 2023 Biçe, Schalles, Sheldon, Alber and Meile. This is an open-access article distributed under the terms of the Creative Commons Attribution License (CC BY). The use, distribution or reproduction in other forums is permitted, provided the original author(s) and the copyright owner(s) are credited and that the original publication in this journal is cited, in accordance with accepted academic practice. No use, distribution or reproduction is permitted which does not comply with these terms.
*Correspondence: Kadir Biçe, bicekadir@gmail.com; Christof Meile, cmeile@uga.edu
†ORCID: Kadir Biçe, orcid.org/0000-0003-4714-0644
Joan E Sheldon, orcid.org/0000-0002-6409-9500
Merryl Alber, orcid.org/0000-0002-9467-4449
Christof Meile, orcid.org/0000-0002-0825-4596