- 1Laboratory for Marine Fisheries Science and Food Production Processes, Pilot National Laboratory for Marine Science and Technology (Qingdao), Key Laboratory of Maricultural Organism Disease Control, Ministry of Agriculture and Rural Affairs, Yellow Sea Fisheries Research Institute, Chinese Academy of Fishery Sciences, Qingdao, China
- 2Harbin University of Science and Technology, Weihai, China
- 3Key Laboratory of Tropical Aquatic Germplasm of Hainan Province, Sanya Oceanology Institute, Ocean University of China, Sanya, China
Malformation disease (MD) is one of the major constraints in the mariculture of the commercially important seaweed Saccharina japonica, which can cause severe losses of the kelp seedlings in the production process. However, the pathogenesis of MD remains largely undetermined. In this study, using cultivation experiments under laboratory conditions, MD severity was estimated for the young sporophytes of S. japonica, which were separately cultivated under different treatment conditions, including light intensity, duration of light exposure, and maturity level of the parent kelp. The associations between MD severity and epibacterial community divergence and assembly patterns were characterized. Higher MD severity significantly correlated with longer light exposure, and with both unmatured and overmatured parent kelp. The bacterial classes γ-Proteobacteria and α-Proteobacteria dominated all samples, but different MD severities were associated with different epibacterial communities. Comparisons of predicted functions for epibacterial communities revealed that longer light exposure led to a depletion in development and regeneration, while overmatured parent kelp resulted in a depletion in glycan biosynthesis and metabolism. Functional comparisons of the epibacterial communities between normal and unmatured parent kelp-generated seedlings revealed significantly different categories related to metabolism, environmental information processing, cellular processes, drug resistance, and bacterial infection. The significantly different pathways between groups, notably those related to bacterial infection and cellular processes, were partly consistent with the differences in bacterial compositions. The assembly of epiphytic bacterial communities was predominately governed by deterministic processes, and less impact was determined when there was significantly higher MD severity except when using overmatured parent kelp. Co-occurrence networks of the epibacterial communities associated with higher MD severity contained fewer nodes and exhibited lower modularity, but had higher graph density and degrees compared with those of seedlings with lower MD severity, indicating more complicated interactions. Nesterenkonia, Glycocaulis, Halomonas, Pseudoalteromonas, Pseudomonas, Loktanella, and Cobetia were frequently determined keystone taxa in communities associated with higher MD severity. The present study enhances our understanding of the factors significantly associated with MD severity and the potential roles of epimicrobiome in determining the disease severity, which will be useful for disease management in the future.
Introduction
Seaweeds are a phylogenetically diverse group of macroscopic marine algae in marine ecosystems, which include brown, red, and green algae. These multicellular photosynthetic eukaryotes are well-equipped to function as ecosystem engineers by contributing to primary productivity, and by providing food and shelter for aquatic life (Watt and Scrosati, 2014; Hasselström et al., 2018). The cultivation of commercially important seaweed species has become a significant and growing industry owing to their significant value in food, medicines, and cosmetics (Lomartire et al., 2021). However, like other living organisms, seaweeds are susceptible to diseases, leading to significant losses in the natural population and economic losses in commercial species. For example, the annual losses caused by oomycete pathogens can reach approximately 15–20% and 25–30% for the cultivated laver in Korea and China respectively, with up to a 64% loss rate reported in Japan (Gachon et al., 2010).
The fitness and ecological traits of seaweeds are influenced by mutual interactions with the associated microbial community, which can be referred to as the “holobiont” (Wahl et al., 2012; Dittami et al., 2021). The microbial community associated with seaweeds consists of potentially beneficial species along with pathogenic species; thus, bacterial-host interactions have the potential to influence seaweed health and development in two different ways. On the one hand, these interactions can be beneficial by supplying nutrients or assisting with nutrient acquisition (Singh and Reddy, 2015; Dogs et al., 2017), providing chemicals for morphogenesis and development (Goecke et al., 2010; Alsufyani et al., 2020), and conferring resistance to stressful environments (Dittami et al., 2016; Morrissey et al., 2021) and pathogen infections (Saha and Weinberger, 2019; Li et al., 2022). On the other hand, these interactions can be detrimental if opportunistic pathogens colonize and proliferate, which notably occur under unfavorable environments when the host’s immunity is undermined (Egan and Gardiner, 2016; Ward et al., 2021; Behera et al., 2022). It is now recognized that seaweed diseases are tightly associated with interactions that occur among macroalgae, bacteria, and the environment. Emerging evidence demonstrates that disruption of the host’s natural microbiota under suboptimal conditions is correlated with disease development (Zozaya-Valdés et al., 2015; Ghaderiardakani et al., 2020; Yan et al., 2022). For an infection disease, it is typically characterized by the increase in potentially pathogenic bacteria and/or virulence-related functional genes. For example, under bleaching conditions, the red alga Delisea pulchra is significantly enriched in certain members of the bacterial families Rhodobacteraceae and Flavobacteriaceae, in addition to functional genes related to chemotaxis, motility, oxidative stress response, vitamin biosynthesis, and nutrient acquisition (Zozaya-Valdés et al., 2015; Zozaya-Valdés et al., 2017). Collectively, the investigation on seaweed microbiome requires a broader perspective by combining their interactions with host and environments.
Currently, it is difficult to disentangle the causal relationship between the changes in epibacterial community and the occurrences of seaweed disease due to the existence of multiple disease stages and the complexity of potential opportunistic pathogens (Zozaya-Valdés et al., 2015). Unraveling the mechanisms that govern community assembly is crucial to predicting the incidence of disease, and can also guide the establishment of new strategies against community disruption and/or pathogenic infections (De Schryver et al., 2012; Pearson et al., 2018; Li et al., 2022). Recently, two principles have been proposed. The “lottery hypothesis,” combining both selective and neutral aspects, suggests that the community assembly depends more on functional level of genes (Burke et al., 2011a). Another hypothesis argues that communities are structured by both untargeted recruitment and targeted deterrence processes from the host (Saha and Weinberger, 2019). These principles combined with other studies suggest that the assembly of microorganisms on seaweeds is controlled by both host- and non-host-related factors, such as the already present colonizers (Longford et al., 2019), host health status (Yan et al., 2022), growth stage of the host (Lemay et al., 2018), and environmental conditions (Florez et al., 2019), which can be categorized into two different ecological processes: deterministic (niche-based) and stochastic (neutral) processes. A deterministic process is expected to drive microbial niche structures that favor one species over the other through host selection and species-to-species interactions (Zhou and Ning, 2017). Alternatively, stochastic processes assume species to be ecologically equivalent, emphasizing the importance of chance colonization, random extinction, and ecological drift (Chase and Myers, 2011; Zhou and Ning, 2017). However, scarce data are available to quantify the relative importance of deterministic and stochastic processes in bacterial assembly on the surfaces of seaweeds, notably when they are plagued by disease.
Increasing evidence demonstrates that responses of a community to disease depend on species-to-species interactions (Zhu et al., 2016; Dai et al., 2020). The alterations can be indicative by the presence of certain taxa within the microbial communities that have greater biotic connectivity to the resident members, irrespective of their abundance; these can be referred to as “keystone taxa” (Herren and McMahon, 2018). Previous studies have identified keystone taxa (also denoted as “gatekeepers”) from the gut microbiota of shrimp, demonstrating their disproportional roles in sustaining stability, dynamics, and function. Such keystone taxa are identified as candidate pathogens in diseased shrimps, further validated by their enrichment in terms of relative abundance and encoded virulence genes (Dai et al., 2018). Moreover, keystone taxa can function as potential probiotics under certain contexts (Xiong, 2018; Dai et al., 2019). Therefore, identifying keystone taxa can provide clues for the identification of causal agents of a disease, and thus offer strategies for the management of maintaining a “healthy” microbiota in disease prevention. Network analysis has emerged as a powerful tool for the integrated understanding of microbe-to-microbe interactions, and the network scores can statistically identify the potential importance of specific microorganisms to the network, such as keystone taxa (Berry and Widder, 2014; Banerjee et al., 2018). For example, taxa with high degree, high closeness centrality, and low betweenness centrality scores in the network are considered as the keystone taxa in the community (Berry and Widder, 2014). Thus, network analysis has been widely applied in the analyses of interactions occurring in multiple ecosystems, including aquaculture systems (Dai et al., 2020; Lin et al., 2021).
The brown seaweed Saccharina japonica (Areschoug) C. E. Lane, C. Mayes, Druehl & G. W. Saunders, 2006 has been cultivated in China since the 1920s as a commercial marine algal species (Tseng, 2001). S. japonica has an alternate life history comprising generations of micro-gametophyte and macro-sporophyte, and its culture process consists of indoor cultivation of seedlings (i.e., sporelings or young sporophytes) and outdoor cultivation of mature sporophytes (Duan et al., 2015). The artificial seedling-rearing techniques developed in the 1950s substantially contributed to the rapid development of the kelp aquaculture industry in China (Tseng, 2001). Recently, S. japonica has been increasingly threatened by various diseases at both the seedling-rearing and open-sea cultivation stages, notably diseases that target young sporophytes, leading to complete failure of seedling production (Peng and Li, 2013; Wang et al., 2014; Li et al., 2020). Malformation disease (MD) is one of the disastrous and frequent diseases in seaweed mariculture, which is becoming an increasingly serious bottleneck in kelp cultivation by causing massive losses of seedlings (Qian et al., 2016; Sheng et al., 2016). According to our field investigations during 2018–2021, the malformation rates in certain tanks were > 50% in kelp genotypes collected in northern China. MD frequently occurs in the early stage of young sporophytes, notably when reaching the stage of 4–8 columns of cells (Wu et al., 1979; Qian et al., 2016). MD was observed to cause abnormal division in some or all of the cells, characterized by expanded cell size and/or irregular division. Eventually, these malformed seedlings disintegrate, leaving no seedlings remaining on the cultivation curtains. The pathogenesis for MD remains largely unknown, although associated bacteria and/or potential factors (i.e. duration of light exposure, maturity level of the parent kelp) have been proposed to play a role (Wu et al., 1979; Qian et al., 2016; Sheng et al., 2016).
In the present study, the MD severity of young sporophytes of S. japonica was estimated under laboratory conditions. Sporophytes were separately cultivated under different treatment conditions, including variation in light intensity, duration of light exposure, and maturity level of the parent kelp, respectively. With these experiments, we aimed to reveal the following: (1) factors significantly correlated with MD severity, (2) divergence of the epiphytic bacterial community associated with different MD severities, and (3) alterations in the bacterial assembly patterns associated with different MD severities. These findings are of great significance for the production of kelp seedlings in the field of disease management.
Material and methods
Experimental design and sample collection
The parent kelp used in the present study was collected from Ailun Bay (122°33’32” E, 37°9’14”) in July, 2021, and a cultivar that has long been cultivated in this area was used for zoospore collection. Approximately 6 individuals (2 replicate individuals × 3 maturity levels) of the cultivar were collected and then washed, incubated, and stimulated to release zoospores in the laboratory according to standard methods (DB37/T 1190—2009). After reaching a density of approximately 3–5 zoospores per microscopic field (100×) with completed attachment on microscopic slides, the zoospores were incubated under various experimental conditions for seedling incubation (Table 1). Factors previously found to be associated with MD in our investigations and other published reports (Qian et al., 2016; Sheng et al., 2016) were selected to further investigate their associations with MD severity, including light intensity, light duration, and maturity level of the parent kelp (estimated based on standard methods SC/T 2024-2006). For each factor, three treatment groups were established with varying levels of the given factor (referred to as a “set”; Table 1). For the set of light intensity, seedlings generated by normally-matured parent kelp were cultivated at 8°C under different light intensities (500 lx, 1500 lx, and 3000 lx) with a photoperiod of 10h: 14h (light: dark). These seedlings were separately grouped and denoted as L500, L1500, and L3000, respectively (Table 1). For the set of light duration, seedlings generated by normally-matured parent kelp were cultivated at 8°C under the light intensity 1500 lx with different photoperiods (5h: 19h, 10h: 14h, and 15h: 9h), and they were grouped and denoted as T5, T10, and T15, respectively (Table 1). For the set of maturity level, seedlings were generated by different maturity levels of the parent kelp, including unmatured, normally-matured, and overmatured, which were grouped and denoted as UM, NM, and OM, respectively (Table 1). These seedlings were cultivated under standard environmental conditions, including 8°C, 1500 lx, 10-h light exposure (DB37/T 1190—2009). Within these groups, the seedlings of the groups L1500, T10, and NM represented the standard cultivation conditions (normally-matured parent kelp and standard environmental conditions), which can function as the control group for each set.
Assays for each set were performed in separate laboratories. Nitrogen and phosphorus nutrients, which are essential for seedling growth, were supplied in sterile natural seawater within the culture Petri dishes according to the standard protocols (DB37/T 1190—2009), and the seawater was exchanged every 3 days. Twelve replicate slides were established as biological replicates for each treatment group and one slide per Petri dish was incubated. After approximately 20 days of incubation when sporophytes entered the stage of 4–8 columns of cells, the malformation severity was estimated based on microscopic observation. For each slide, 10 microscopic fields were selected to count the total number of malformed seedlings. MD severity was then calculated as (total observed malformed seedlings/total observed individuals) × 100%. It has been reported that certain gametophytes cannot convert to sporophytes at a normal pace due to slow growth or death under inappropriate conditions (e.g., lower or higher light intensity, shorter or longer light exposure) (Duan et al., 2015). Therefore, an unconverted proportion was also calculated for each replicate as (total observed individuals not converted to sporophytes/total observed individuals) ×100%. This is useful to evaluate seedling growth and development status so that we can select groups at approximately the same status for the comparisons of MD severity and epibacterial community. Finally, six randomly selected replicates were used to scrape seedlings on cotton swabs for analysis of the epiphytic bacterial communities.
DNA extraction, PCR amplification, and illumina sequencing
For detection and quantification of epiphytic bacteria, DNA was isolated from each replicate using the EZNA Soil DNA Kit (OMEGA Bio-Tek, Norcross, GA, USA). To avoid any loss of the firmly attached bacteria, both cotton swab and the collected seedlings on it were used for DNA extraction. The extracted DNA was assessed by gel electrophoresis, and the qualified DNA was then stored at −20°C until used for amplification. To preclude amplification of plastid sequences, the primers 799F and 1193R were used to amplify the V5–V7 hypervariable regions of the 16S rRNA gene which has been used in the study of plants and algae microbiota (Zhang et al., 2019; Aires et al., 2021). The amplicon libraries were constructed using TruSeq Nano DNA LT Library Prep Kit (Illumina, San Diego, CA, USA), following the manufacturer’s recommendations, and index codes were added. Subsequently, the amplicon libraries were sequenced on a NovaSeq 6000 sequencer (Illumina), and 250-bp paired-end reads were generated. The raw sequence data have been deposited in the Genome Sequence Archive (Chen et al., 2021) in National Genomics Data Center (Xue et al., 2022), China National Center for Bioinformation/Beijing Institute of Genomics, Chinese Academy of Sciences (GSA: CRA008545) that are publicly accessible at https://ngdc.cncb.ac.cn/gsa.
Sequence processing
The paired-end reads were joined using the command “fastq_mergepairs” in VSEARCH (version 2.15.1) (Rognes et al., 2016), and then truncated (fastq_stripleft 19 and fastq_stripright 18) and quality-filtered (fastq_maxee 1.0) using the command “fastx_filter” in VSEARCH. High-quality reads were clustered into zero-radius operational taxonomic units (ZOTUs) using the unoise3 algorithm in USEARCH (version 10.0.240) (Edgar, 2010). Taxonomy assignment for representative ZOTU sequences was also performed in USEARCH using the sintax algorithm against the RDP 16S training set 18 database (cutoff = 0.8). Sequences assigned as plastids and mitochondria, as well as those not belonging to the domain Bacteria, were discarded. Taxonomic information of ZOTUs in the following analyses, e.g., community comparisons and keystone taxa, were also checked using Basic Local Alignment Search Tool (BLAST). A rarefied ZOTU table at the same depth was used to calculate α- and β-diversities in R using the “vegan” package (version 2.5-6) (Oksanen et al., 2019). Relative codes for taxa filtering, taxa circular visualization plots, and diversity calculation were obtained from a direct application documented in Zhang et al. (2019).
Functional prediction of the epibacterial community
The ZOTU table was normalized by dividing the abundance of each ZOTU by its predicted 16S rRNA gene copy number to produce the Kyoto Encyclopedia of Genes and Genomes (KEGG) orthology (KO) functional categories using PICRUSt2 (Douglas et al., 2020). The script “summarizeAbundance.py” was used to calculate pathway count abundance at different levels, which is documented in EasyMicrobiome (version 1.16)1.
Null model analyses of epiphytic bacterial communities
To disentangle the relative contributions of stochastic and deterministic processes to microbial community assembly, the NST value was used to quantify ecological stochasticity in communities (Ning et al., 2019). This index measures the relative importance of stochasticity versus determinism considering both the situations where deterministic factors drive the communities to be more similar or dissimilar than random patterns (Ning et al., 2019); NST < 0.5 indicates a more deterministic community and NST > 0.5 indicates a more stochastic community assembly. NST was calculated based on Jaccard and Bray–Curtis similarity metrics using the null model algorithm PF (with fixed data richness and proportional taxa occurrence frequency). NST analysis was performed using the “NST” R package (Ning et al., 2019).
Construction of the co-occurrence network
The networks were individually constructed to infer associations between ZOTUs for each treatment group. To construct the co-occurrence network, compositionality-robust correlations from the median of 20 iterations and 100 bootstrap samples were used to infer pseudo p values (Kurtz et al., 2015). The inferred correlations were restricted to those having correlation coefficients > 0.8 or < −0.8 (p < 0.05, two-sided). Visualization was performed with the Gephi 0.9.3 platform, using a Fruchterman Reingold layout (Bastian et al., 2009). To describe the topology of the networks, the following metrics were calculated: average path length, network diameter, average degree, clustering coefficient, and graph density. Average path length refers to the average network distance between all pairs of nodes, network diameter refers to the greatest distance between the nodes that exist in the network, average degree refers to the average connections of each node with another unique node in the network, clustering coefficient represents the degree to which the nodes tend to cluster together, and graph density refers to the intensity of connections among nodes (Newman, 2002; Newman, 2006). A higher average degree, clustering coefficient, and graph density suggest a more connected network. In addition, lower average path lengths and diameters indicate closer associations in the network (Barberán et al., 2012; Ma et al., 2016). The node-level topological features, including degree, betweenness, and closeness centrality, were also calculated for each node. The ZOTUs with the highest degree, highest closeness centrality, and lowest betweenness centrality scores were considered as the keystone taxa (Berry and Widder, 2014).
Statistical analysis
One-way analysis of variance (ANOVA) was used to test the significance in differences of MD severity, α-diversity indices, and normalized stochasticity ratio (NST) values among groups within each set (Chambers et al., 1992). Constrained principal co-ordinates analysis (CPCoA) was performed based on the Bray–Curtis distance matrix and visualized using the web server ImageGP (Chen et al., 2022). A random forest (RF) algorithm was used to screen significantly differentiated bacteria ZOTUs among treatment groups using the “randomForest” R package (Breiman, 2001). The significance of differentially abundant ZOTUs was also tested in STAMP (version 2.1.3) based on ANOVA with a Tukey post-hoc test (p < 0.05) (Parks et al., 2014). STAMP (version 2.1.3) was also used to determine bacterial ZOTUs that are significantly different between groups with significantly different MD severities, and Welch’s t-test was used to test the pair-wise significance (p < 0.05). All the aforementioned analyses performed in STAMP were also applied to determine significantly different functions based on their relative abundance, and categories related to humans were excluded from the statistical analysis.
Results
Malformation severity for different treatments
The presence and severity of MD were observed when the experiments were terminated. As shown in Figure 1, several types of individuals were observed in the present study, including normal, unconverted, and malformed individuals. Given unsuccessful cultivation for zoospores within certain replicates, the MD severity was estimated using the remaining replicates for each treatment condition (Table 2). For the treatments with different light intensities, the MD severity was not significantly different among groups. Lower light intensity also prevented more gametophytes from transforming into sporophytes, resulting in a significantly higher unconversion rate (Table 2). Longer light exposure generated a significantly higher MD severity than found in the seedlings cultivated with standard light conditions (1500 lx, L1500). Although shorter light exposure (5 h, T5) generated significantly lower MD severity, more gametophytes could not properly convert into sporophytes under this condition, with an unconversion rate reaching over 66% (Table 2). In particular, the normally-matured parent kelp (NM) generated significantly lower MD severity of the seedlings than that derived from both unmatured (UM) and overmatured (OM) parent kelp (Table 2). These results demonstrated that groups of T10 and T15, NM and UM, and NM and OM warrant further comparisons concerning epibacterial communities due to their significantly different MD severity and similar growth status (i.e., unconverted proportion).
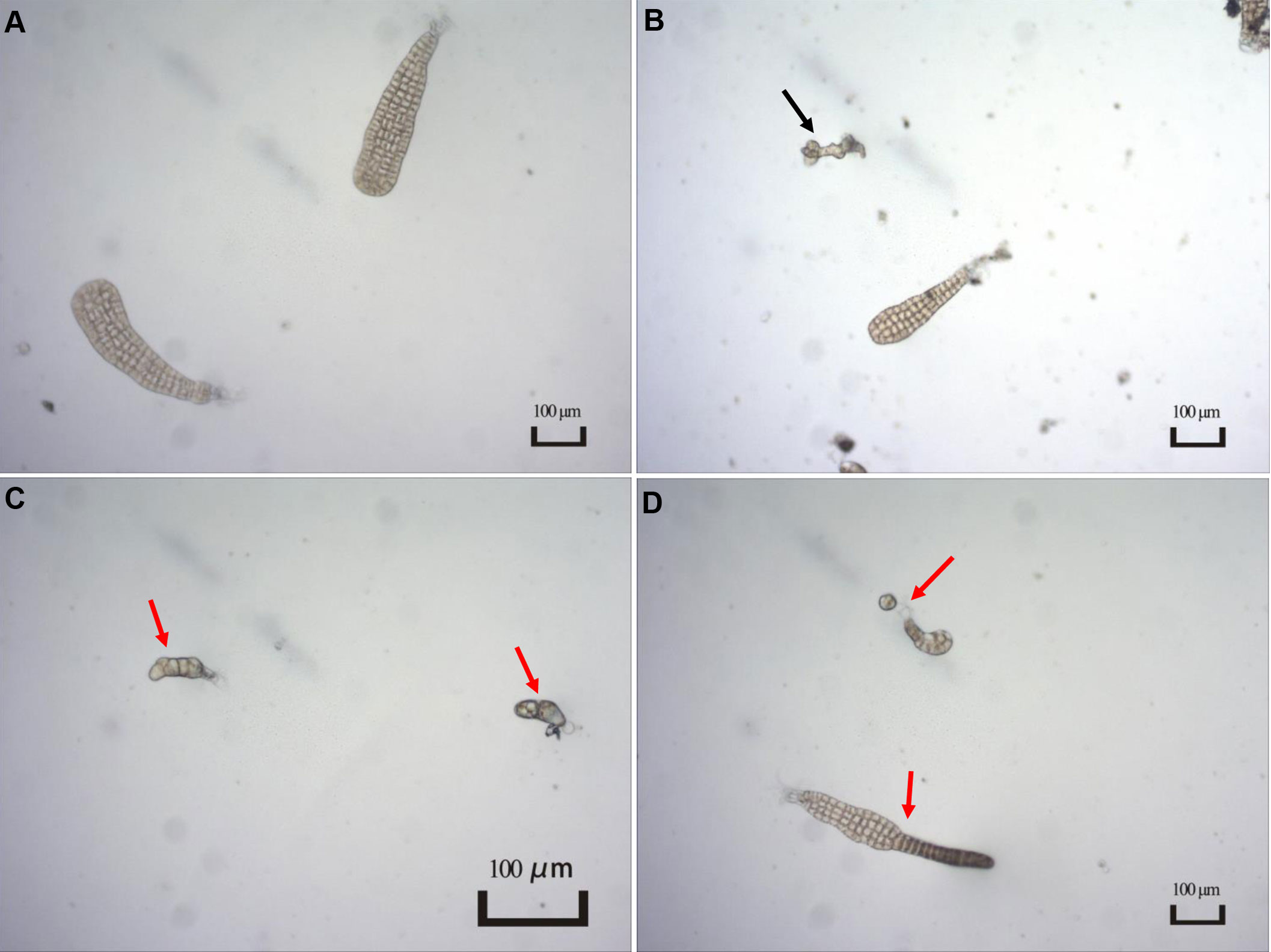
Figure 1 Typical characteristics of different types of seedlings observed in the present study. (A) Normal seedlings. Image from T15 group; (B) Individual not converted to sporophyte as indicated by a black arrow. Image from T10 group; (C, D) Malformed seedlings indicated by red arrows. Image from T15 group.
Epiphytic bacterial community composition and diversity
All samples were dominated by the bacterial classes γ-Proteobacteria and α-Proteobacteria, which accounted for 92.85 ± 6.59% (mean ± standard deviation) of the total sequences (Figure 2). The relative abundance of γ-Proteobacteria declined as the light intensity increased (Figure 2A). In contrast, the relative abundance of α-Proteobacteria increased as the light intensity increased (Figure 2A). Similar patterns were also observed for the groups cultivated under different light durations (Figure 2B). The relative abundance of γ-Proteobacteria was the highest in the group cultivated with normally matured parent kelp (NM), but was the lowest in the UM group, and did not tend to decline progressively with maturity level (Figure 2C). Conversely, the changes in the relative abundance of α-Proteobacteria showed the opposite trend with respect to the maturity level of parent kelp (Figure 2C).
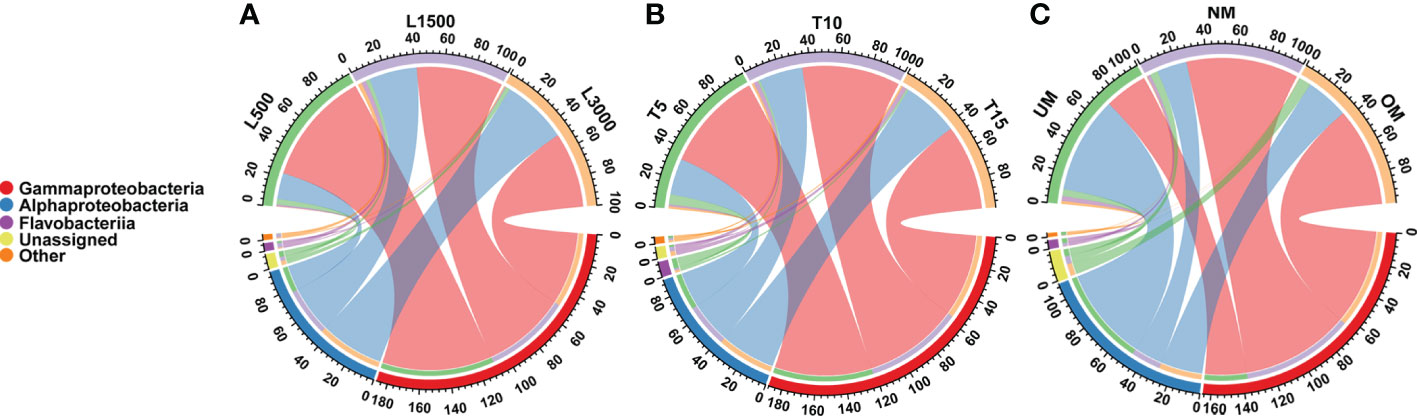
Figure 2 Distribution of major bacterial taxa in different treatment groups at the class level. (A) Groups treated with different light intensities; (B) Groups treated with different durations of light exposure; (C) Groups derived from different maturity levels of parent kelps.
Observed species and Shannon index was used to estimate the α-diversity for all treatment groups. The light intensity did not have a significant effect on α-diversity, although the diversity of the L1500 group (representing the standard culture condition) was higher than that of the other two groups (Figure 3A). The T5 group showed a significantly lower Shannon index compared with that of the groups exposed to light for 10 h (T10) and 15 h (T15), respectively (Figure 3A). Although the highest α-diversity was found for the T10 group, there was no significant difference from that of the T10 group (Figure 3A). With respect to the maturity of the parent kelp, NM showed a higher species richness value and a relatively lower Shannon index than the other two groups, although no significant difference was observed (Figure 3A). CPCoA plots revealed that the bacterial communities were separately clustered according to the different treatments (Figure 3B). Further, perMANOVA confirmed significant associations between treatments and the community differences (p < 0.05), with light intensity, light duration, and maturity level explaining 16.6%, 14.8%, and 16.9% of the community variations, respectively (Figure 3B).
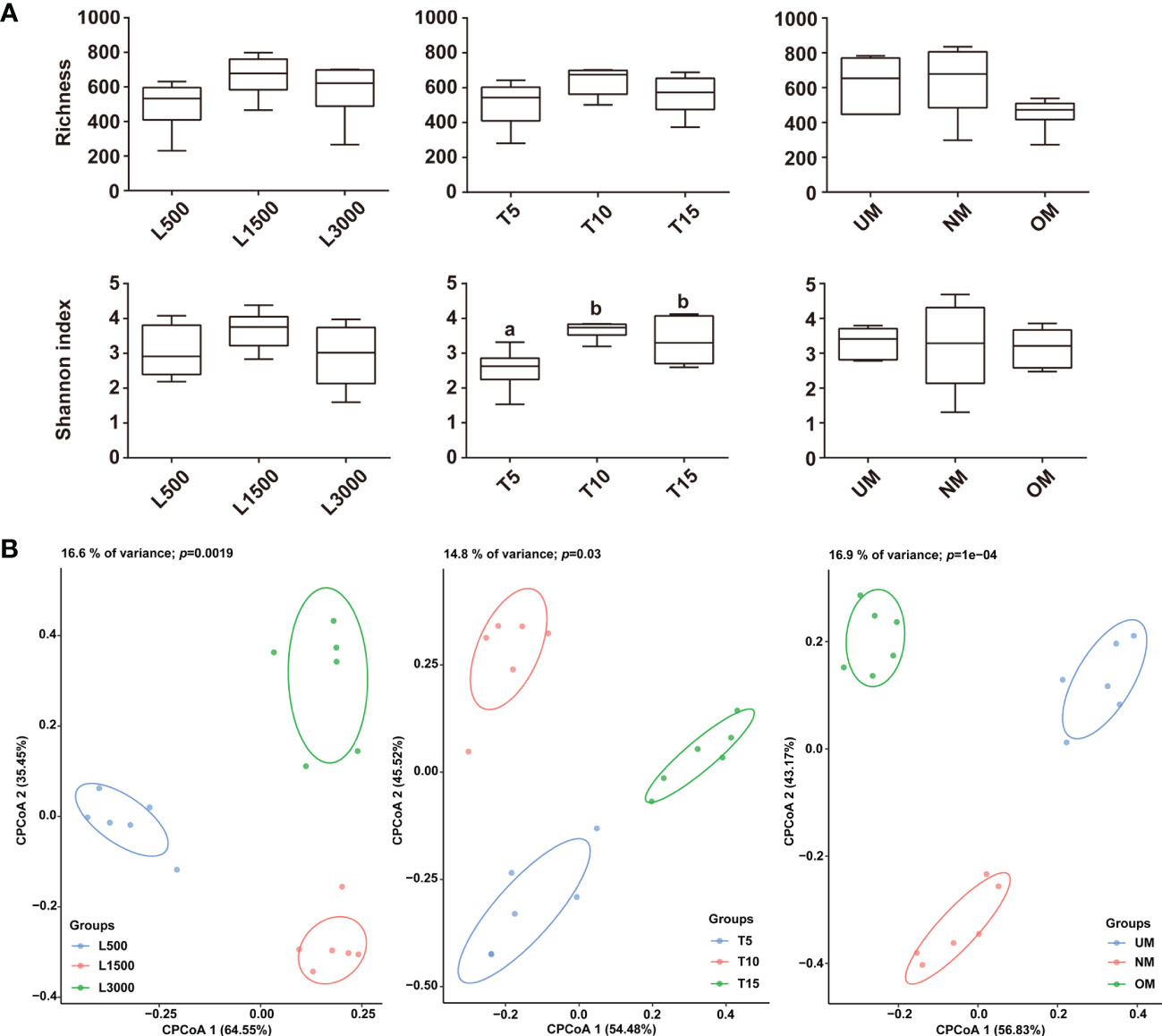
Figure 3 Comparisons of α (A) and β (B) diversities among the epiphytic bacterial communities associated with different treatment groups. The α-diversity was estimated using Observed species and Shannon index, and letters on the boxes indicate significant difference (p < 0.05, ANOVA, Tukey post-hoc test) among groups. Plots for the β-diversity were performed based on the Bray–Curtis dissimilarity.
Divergent bacterial communities among different treatments
The associated bacterial communities were then compared at the ZOTU level to reveal the community divergence according to treatment condition. Based on the RF algorithm and ANOVA, approximately 6, 16, and 3 ZOTUs were determined to differentiate treatment groups for the sets of light intensity, light duration, and maturity level, respectively (Figure 4A). This indicated that these bacterial ZOTUs, though distributed in various genera (Table S1), can potentially serve as indicators of host status. Bacterial communities associated with seedlings with different MD severities were further compared based on Welch’s t-test. Approximately 12, 17, and 18 ZOTUs were determined to be significantly different between groups in the comparisons of light duration (T10 vs. T15), NM vs. UM, and NM vs. OM, respectively (Figure 4B). Based on BLAST and phylogenetic analysis, these ZOTUs were phylogenetically diverse notably those affiliated with Rhodobacteraceae (Figure S1 and Table S2). Specifically, seven of the 12 significantly different ZOTUs between T10 and T15 were enriched in T10, which were distributed in seven genera. By contrast, the relative abundances of five ZOTUs affiliated with Sulfitobacter, Fluviibacterium (two ZOTUs), Psychroserpens, and Pacificibacter were higher in T15 than in T10 (Figure 4B). Ten of the 17 significantly different ZOTUs between NM and UM were more significantly abundant in NM, including those affiliated with Colwellia (two ZOTUs), Pseudoalteromonas (four ZOTUs), Pseudomonas (two ZOTUs), and Cobetia (two ZOTUs) (Figure 4B). Compared with those in OM, seven ZOTUs were significantly enriched in NM, distributed in Colwellia (two ZOTUs), Cobetia (two ZOTUs), Pseudoalteromonas, Yoonia, and Pseudomonas, respectively. Whereas, eleven ZOTUs were significantly depleted in NM, including those assigned to Pseudomonas (four ZOTUs), Parasedimentitalea (two ZOTUs), Halopseudomonas (two ZOTUs), Paracoccus (two ZOTUs), and Lentibacter (Figure 4B).
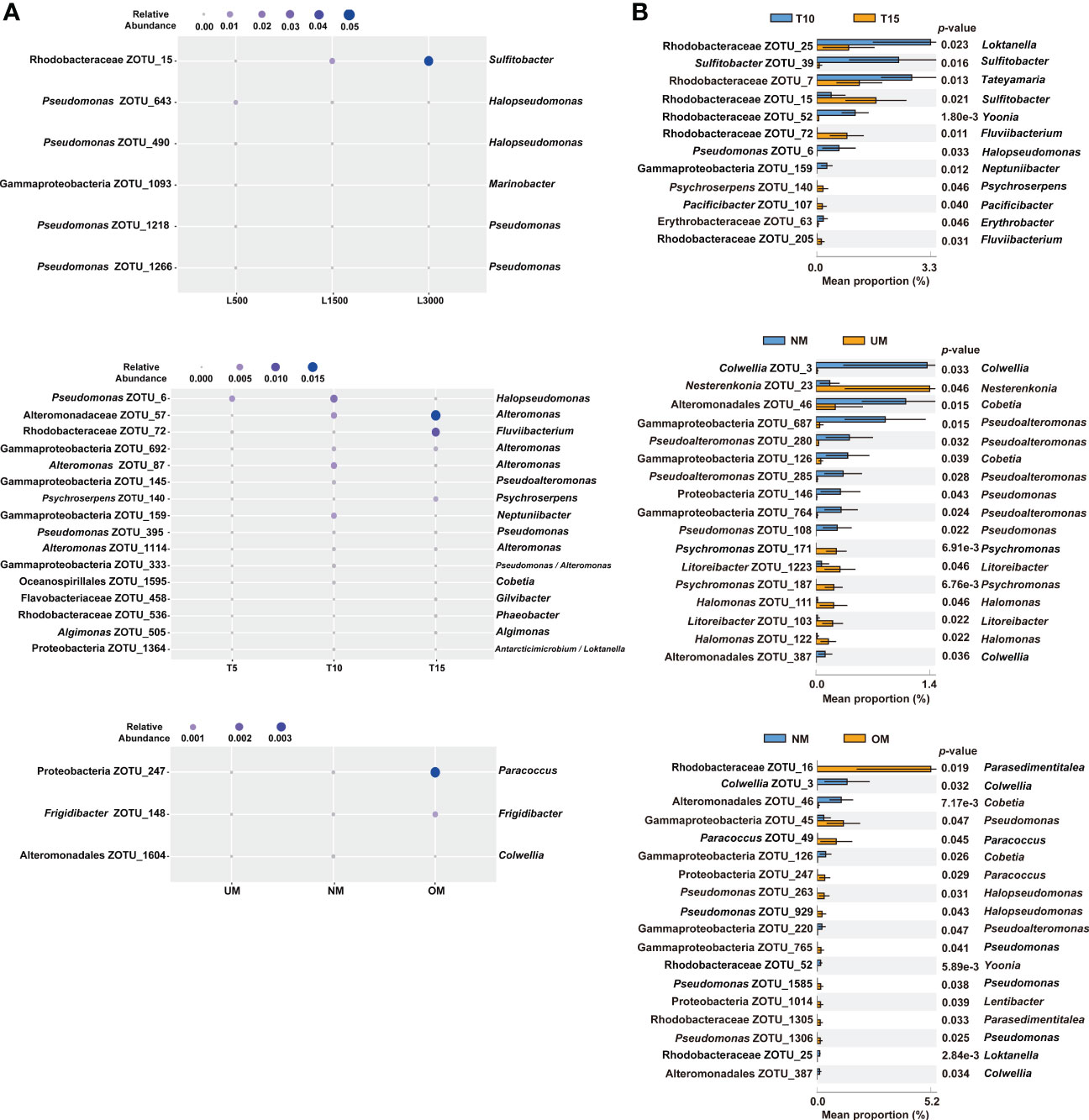
Figure 4 Significantly different epiphytic bacterial ZOTUs among treatment groups (A) and between groups with different MD severity (B). For (A), significant differences were determined using RF and ANOVA (p < 0.05, Tukey post-hoc test); for (B), significant differences were determined using Welch’s t-test (effect size >0.1, p < 0.05). Taxonomic information on the right of all the plots is determined using BLAST.
Divergent potential functional groups among different treatments
From the comparisons of PICRUSt2 predictions, no significant functional difference was determined among the groups treated with different light durations. However, significant differences among groups treated with different light intensities were detected, which were mainly derived from pathways related to amino acid metabolism, carbohydrate metabolism, and metabolism-related protein families (Figure 5A). For groups derived from parent kelp with different maturity levels, significant differences were mainly distributed in pathways related to metabolism, including energy metabolism, metabolism of other amino acids, and metabolism of terpenoids and polyketides, in addition to the aforementioned three categories that were divergent according to light intensity (Figure 5A). Moreover, pathways related to environmental information processing (signal transduction) and cellular processes (cell motility) were also significantly differentially distributed among groups (Figure 5A).
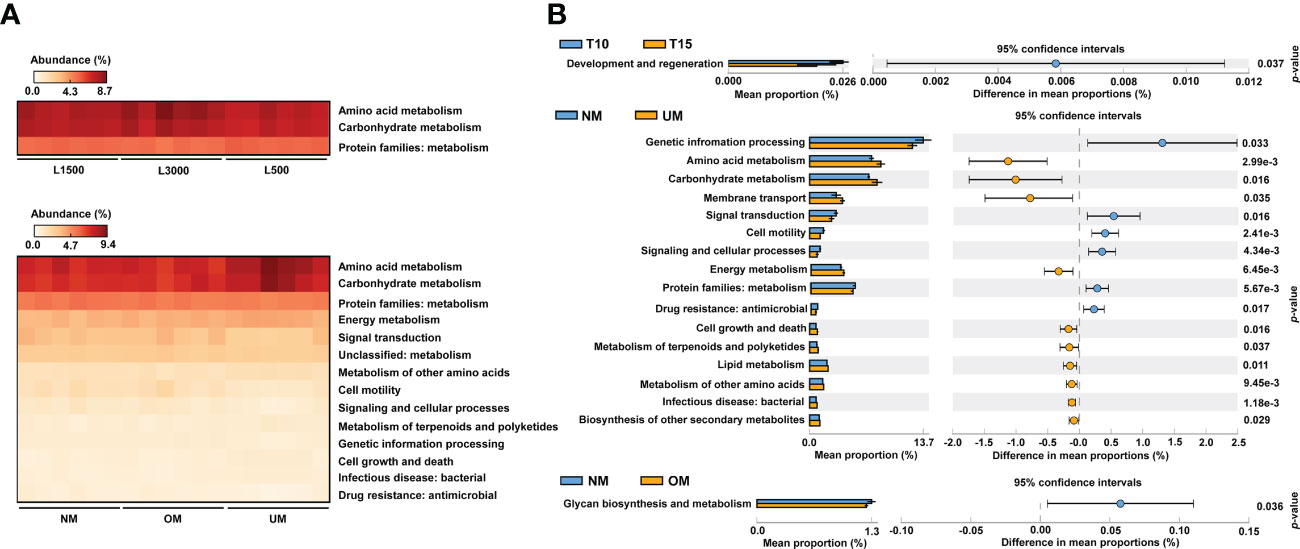
Figure 5 Comparisons of predicted functional profiles among treatment groups (A) and between groups with significantly different MD severity (B). Significant differences were determined based on relative abundance using ANOVA (p < 0.05, Tukey post-hoc test) and Welch’s t-test (p < 0.05), respectively.
To further reveal functional differences between groups, functional profiles between T10 and T15, NM and UM, and NM and OM were respectively compared. T10 was significantly enriched in categories related to development and regeneration compared with T15 (Figure 5B). Compared with UM, approximately six functional categories were significantly enriched in NM, including certain protein families (metabolism, genetic information processing, and signaling and cellular processes), environmental information processing (signal transduction), cellular process (cell motility), and drug resistance (Figure 5B). In addition, 10 pathways were found to be significantly depleted in NM compared with those of UM, which mainly included metabolism-related pathways (e.g., amino acid, carbohydrate, energy, lipid, and biosynthesis of other secondary metabolites), environmental information processing (membrane transport), bacterial infection, and cellular process (cell growth and death) (Figure 5B). For the comparison between the NM and OM groups, glycan biosynthesis and metabolism was the only category that showed a significant difference, which was more enriched in the NM group (Figure 5B).
Assembly process of epiphytic bacterial community
NST values based on both the Bray–Curtis distance and Jaccard dissimilarity revealed consistent trends among different treatment groups (Figure 6). Specifically, the NST values for all groups ranged from 16.79% to 35.06% and from 19.23% to 42.99% based on the Bray–Curtis distance (Figure 6A) and Jaccard dissimilarly (Figure 6B), respectively, indicating that the assembly of epiphytic bacterial communities on the seedlings was predominately governed by deterministic processes. For seedlings treated with different light intensities, the epiphytic bacterial community on seedlings cultivated under standard conditions (L1500) had a lower NST value (16.79% and 19.35% for the Bray–Curtis distance and Jaccard similarity, respectively) compared with those of the other two groups (L500: 35.06% and 38.30%; L3000: 31.41% and 42.99%, respectively), although no statistically significant difference was determined. Similar patterns were also observed for the groups treated with different light durations; notably, the T10 group had a significantly lower value (17.06%) than the T15 group (29.60%) based on the Bray–Curtis distance. For seedlings cultivated using different maturity level of parent kelp, NST values showed a slightly decreasing trend in accordance with the maturity level.
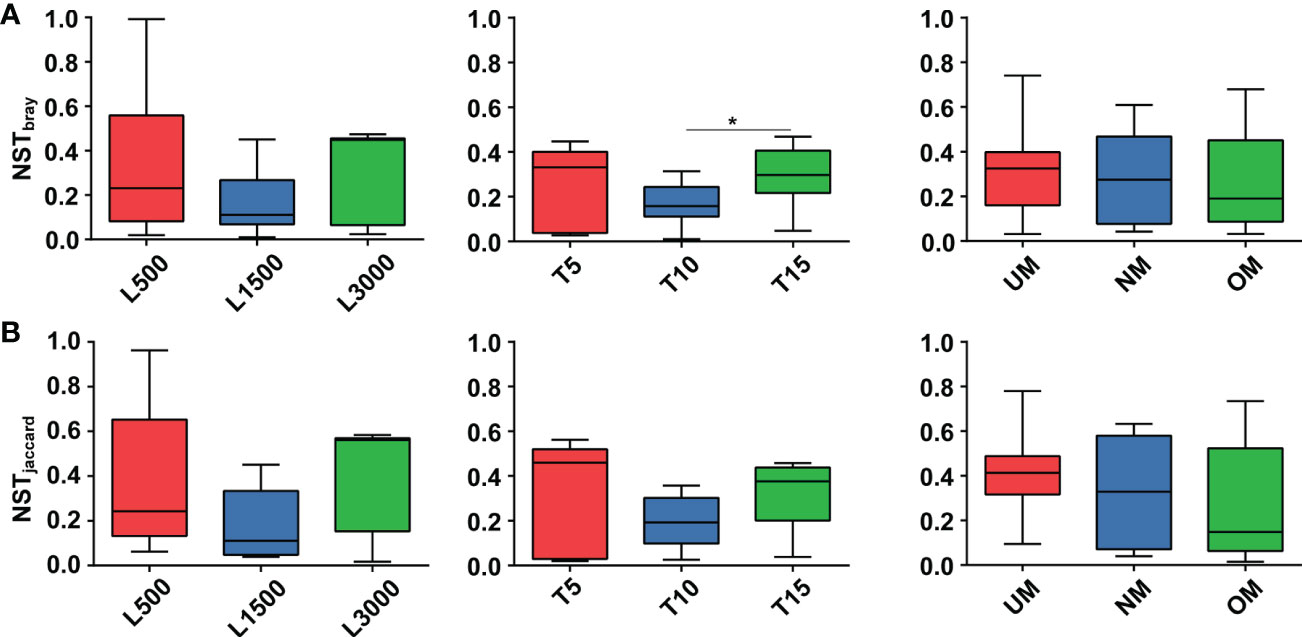
Figure 6 Comparisons of NST values among different treatment groups based on Bray-Curtis (A) and Jaccard (B) dissimilarity. Asterisk on the boxes indicate significant difference (*p < 0.05 Tukey post-hoc test) among groups.
Correlation networks
Networks constructed for the groups under stressful conditions, notably those with significant MD severity, tended to harbor fewer nodes but a relatively higher average degree and graph density compared with those constructed under the standard cultivation conditions in each set (Table 3 and Figure S2). For example, the T15 network had 99 nodes, which was less than the number of nodes in the T10 network. The average degree and density of the T15 network were 2.222 and 0.023, respectively, which were also both higher than those of the T10 network. Similar trends were also determined for the comparisons between the NM and UM networks, and between the NM and OM networks, respectively (Table 3). The modularity indices for all networks were greater than 0.4, indicating that all networks harbored a modularized structure (Newman, 2006). Networks for groups with significantly higher MD severities had modularity indices lower than those of the groups cultivated under standard conditions (Table 3). Specifically, the modularity of the T15 network was 0.791, which was lower than the network of the T10 group (0.856) that also had a lower MD severity than the T15 group. Modularity indices of the UM (0.641) and OM (0.806) networks were also both lower than that of the NM group (0.818).
For all networks, ZOTUs with degree ≥ 5, closeness centrality > 0.15, and betweenness centrality < 200 were selected as the keystone taxa. Consequently, different profiles of keystone taxa were determined for all groups. The taxonomic information of these keystone taxa and their relative abundances are listed in Table S3. All keystone taxa belonged to the predominant groups identified in all networks: γ-Proteobacteria, α-Proteobacteria, and Actinobacteria. The keystone taxa of the T15 groups were determined to be Halomonas (ZOTU_81) and Pseudoalteromonas (ZOTU_1 and ZOTU_220), whereas those of the T10 group were identified as Phaeobacter (ZOTU_245), Sulfitobacter (ZOTU_462), and Pseudomonas (ZOTU_2). The two groups also shared three keystone taxa: Nesterenkonia (ZOTU_23), Glycocaulis (ZOTU_34), and Aliihoeflea (ZOTU_38). Both the UM and OM groups were associated with more complicated profiles of keystone taxa since only two genera (i.e., Pseudomonas and Halopseudomonas) were determined as keystone taxa in the NM group. ZOTUs belonging to Nesterenkonia, Glycocaulis, Halomonas, Pseudoalteromonas, Pseudomonas, Loktanella, and Cobetia were frequently determined keystone taxa in groups with significantly higher MD severity.
Discussion
In the present study, different MD severities of kelp seedlings were determined under various laboratory cultivation conditions. The epiphytic bacterial communities of the groups varying in MD severity were then analyzed and compared with respect to their structural and predicted functional profiles, in addition to the bacterial assemblage patterns.
Overall, the results demonstrated that the maturity level of parent kelp has a significant association with MD severity. Longer light exposure also showed a significant correlation with MD severity. These findings are consistent with previous reports proposing that either unfavorable light exposure or the maturity level of parent kelp is highly associated with the occurrence of MD (Qian et al., 2016; Sheng et al., 2016). However, scarce field investigations or experimental data have ever been provided to confirm such speculation. Exceeding the limits of environmental adaptation and tolerance is known to have negative impacts on the host state and defenses (Harvell et al., 2002; Moenne et al., 2016), while parent kelp of poor quality can also produce gametophytes and young sporophytes of poor quality (Qian et al., 2016; Sheng et al., 2016). The overall undermined physiology and performance of a host makes it potentially more susceptible to disruption of the associated microbiota, which can further facilitate pathogen infection, thereby providing good opportunities for disease occurrence. Multiple lines of evidence have demonstrated that bacteria are significant participants in macroalgal diseases, including rotting (Wang et al., 2008; Yan et al., 2022), bleaching (Zozaya-Valdés et al., 2017; Ward et al., 2021), gall formation (Ashen and Goff, 1996), and others. However, in many cases, a specific causal bacterial pathogen of the disease has not yet been conclusively identified, because diverse bacterial genera may be involved in the same disease or even induce similar symptoms as found in field observations. In such a context, it is necessary to investigate the pathogenesis at the level of the bacterial community. In the present study, significant community divergence was determined among different treatment groups, as confirmed by the CPCoA plots and perMANOVA for these groups. This indicates that seedlings of different health status had different bacterial communities.
To further reveal the nature of the observed divergence, the bacterial communities were compared between seedling groups with different MD severities. The predicted pathway changes (i.e., those involved in development and regeneration) enriched in T10 might indicate that the microbiota disruption caused by longer light exposure in T15 led to a lack of bacteria responsible for the normal development of seedlings. It has been demonstrated that disruption of associated microbiomes under stressful environments could cause abnormal phenotypes in macroalgae, e.g., malformations (Wichard, 2015; Ghaderiardakani et al., 2020). This is also a plausible mechanism related to MD given the characteristic of the disease in displaying abnormal morphology in seedling development. The enrichment of ZOTUs affiliated with the genus Loktanella (ZOTU_25) and Sulfitobacter (ZOTU_39) on the T10 seedlings is consistent with this observation, because both taxa have been reported to include essential members that stimulate the growth and/or morphology of macroalgal species (Spoerner et al., 2012; Dogs et al., 2017). As such, the differences detected between NM and UM further suggest the significant role played by bacteria in MD severity, including enriched signal transduction/signaling and cellular processes in NM. Signal-mediated quorum sensing allows bacteria to assemble as biofilms on seaweeds, and some bacteria (e.g., Pseudoalteromonas) are known to regulate zoospore settlement through cross-kingdom signaling (Marshall et al., 2006; Joint et al., 2007). Some bacteria are also well-equipped to have a positive impact on the morphology and growth of algae (Marshall et al., 2006), which is somewhat in line with our structural comparison at the ZOTU level. We found that ZOTUs belonging to Pseudoalteromonas (ZOTU_687, ZOTU_280, ZOTU_285, and ZOTU_764) and Pseudomonas (ZOTU_146 and ZOTU_108) were significantly enriched in NM. A significant proportion of the Pseudomonas members have been reported to induce morphological changes in green algal species (Nakanishi et al., 1996).
In the comparison between NM and OM, it is noted that the growth and/or morphology-related ZOTUs were significantly enriched in OM, such as ZOTU_45, ZOTU_765, ZOTU_1585, and ZOTU_1306 assigned to Pseudomonas, although only one predicted pathway (i.e., glycan biosynthesis and metabolism) was significantly enriched in NM. In addition, the OM-enriched taxon Paracoccus (ZOTU_49 and ZOTU_247) has also been reported to promote growth and morphogenesis in Ulva species (Ghaderiardakani et al., 2017). Collectively, these results suggest that the overgrowth of parent kelp might also be associated with an outcome of serious MD, potentially by influencing bacteria that impact host growth and morphology. Based on these observations, we cannot preclude the possibility that certain bacterial members are capable of infecting seedlings as opportunistic pathogens, hence manipulating host cellular processes to cause malformation. The production of molecular mimics of algal hormones in marine bacteria have been proposed to facilitate the bypass of host defenses, thus facilitating invasion of the macroalgal tissue to interfere with host cell growth (Egan et al., 2014). We also found that certain morphology and/or growth-related ZOTUs were significantly enriched in seedlings with significantly higher MD severity, such as ZOTU_15 assigned as Sulfitobacter (Dogs et al., 2017) in T15, ZOTU_111 and ZOTU_122 assigned as Halomonas (Spoerner et al., 2012) in UM, and the aforementioned four ZOTUs assigned as Pseudomonas (Nakanishi et al., 1996) in OM. All of these taxa have been determined to constitute a significant proportion of the epiphytic bacterial community on diseased S. japonica (Wang et al., 2008; Zhang et al., 2020). Their capabilities of degrading algal polysaccharides (e.g., structural elements for cell walls and storage materials) and manipulating cellular processes by inducing development and growth have been well documented (Wang et al., 2008; Spoerner et al., 2012; Tapia et al., 2016; Dogs et al., 2017), which are conceived as significant virulence traits to be potential opportunistic pathogens for seaweeds (Egan et al., 2014). Additionally, the pathways related to bacterial infection and cell growth and death were significantly enriched in UM, in contrast to downregulation in the pathways of signaling and cellular processes. This indicates a functional transition from mild to more severe MD by disrupting the host’s cell signaling and development. Taken together, our results suggest that disruption of the epiphytic microbiota caused by suboptimal growth and development conditions might provide a good opportunity for the invasion of opportunistic pathogens to interfere with the host’s cellular processes, hence leading to more severe MD in the seedlings. However, more efforts are required to investigate how the epiphytic microbiome may interact with the host across stages of disease progression, which can provide further insight into MD pathogenesis.
In the present study, the assembly of all epiphytic bacterial communities was predominantly governed by deterministic processes. There is evidence that algal-associated bacterial communities differ from planktonic communities, and that seaweeds harbor species-specific and temporally adapted epiphytic bacterial communities on their surfaces (Lachnit et al., 2009; Lachnit et al., 2011; Burke et al., 2011b). Such differences between seaweed-associated communities imply selective mechanisms of assembly of the bacteria, further supporting a significant role of deterministic processes. We found variations in the assembly of bacterial communities on seedlings with significantly higher MD severity, particularly a decrease in determinism for the T15 and UM groups. This indicates that the relative contribution of the ecological processes that govern the establishment of epiphytic bacterial communities is linked to MD severity. Similar cases have also been reported for assembly of the gut microbiota in aquaculture animals during disease occurrence (Zhu et al., 2016; Xiong et al., 2017; Yao et al., 2018). Specifically, previous studies have suggested that the emergence of host disease reduces the relative importance of deterministic processes. One possible explanation is that the host’s selective capability on planktonic bacteria is reduced under environmental stresses (e.g., longer light exposure in T15). Conversely, it is reported that both environmental stress and poor-quality parent kelp can lead to a decline in the health state of seedlings (Sheng et al., 2016), which may in turn lead to disruptions of the proper functions of the epiphytic bacterial community. Therefore, it is also possible that changes in the epiphytic bacterial assembly provide an open niche (a consequent lack of competition) for ambient bacterial species. Health-undermined seedlings are subject to stochastic colonization by dispersed microorganisms from the ambient water, including bacteria capable of causing infection, which is partly supported by the significant enrichment of the bacterial infection pathway in the UM group (Figure 5). Hence, this might support the possibility of opportunistic pathogen infection from the angle of community assembly. From a practical point, it is necessary to reduce such process variations to resist disease occurrence. Notably, the deterministic process became more important in OM compared with NM. This demonstrates that the ability of selection on microbiota increased as the hosts matured (Burns et al., 2016), further indicating an increase in the relative importance of deterministic processes. However, more detailed investigations are required to determine if overmatured parent kelp will have a direct influence on the assembly of the epiphytic bacterial community in their seedlings.
Bacterial interactions were also determined and their co-occurrence patterns were then compared between treatment groups with different MD severity. Groups with significantly higher MD severity (T15, UM, and OM) tended to contain fewer nodes but had higher graph density and degree, indicating more connected and complicated networks. Lower modularity was also determined for these networks, indicating less modularized structures. A module in the network is generally recognized as a group of taxa that are highly connected, which may represent similar niche preferences for these taxa (Ma et al., 2020). In such a context, lower modularization for the severe MD networks may indicate a relatively wide range of niche preferences for the colonized bacteria, thus reflecting disruptions of the epiphytic bacterial communities and increased complexity from the perspective of species–species interactions. Similar cases have also been reported for both shrimp and kelp diseases (Dai et al., 2020; Ling et al., 2022). In particular, the diseased shrimp also harbored a more highly connected and more complex bacterial network of gut microbiota (Dai et al., 2020). In addition, more diverse keystone taxa were determined for diseased shrimp compared with those identified in the healthy cohorts (Dai et al., 2020). This previous finding is to a large extent consistent with the more complicated profiles of keystone taxa detected in the present study for seedlings with significantly higher MD severity. Because keystone taxa play indispensable roles in the microbiome, and their removal could cause devastating changes in microbial composition and function (Banerjee et al., 2016; Banerjee et al., 2018; McMahon, 2018). This suggests that more diverse keystone taxa sustain and drive the changes in the epiphytic microbiota of seedlings with more severe MD. In this regard, multiple keystone taxa identified in groups with more severe MD, including Halomonas, Pseudoalteromonas, Pseudomonas, Loktanella, and Cobetia, have been reported to be capable of causing rotting, spotting, or degrading polysaccharides in macroalgae (Wang et al., 2008; Martin et al., 2015; Han, 2015), in addition to the aforementioned capabilities of inducing morphology and/or growth and development. Therefore, these taxa could be significant contributors to disease severity. Additionally, ZOTUs related to these taxa were also determined to be significantly different in relative abundance between mild and more severe groups. A recent study indicated that the functions of a certain bacterial taxon, i.e., beneficial or harmful to the algal host, depend on the species or specific strains to a large extent (Li et al., 2022). Therefore, the significant roles of these keystone taxa in severe MD samples, notably specific bacterial strains, warrants deeper investigation in future studies to gain further insights into the mechanisms of disease occurrence.
Conclusions
MD severity was estimated for young S. japonica sporophytes under laboratory conditions. The findings illustrated that light exposure and the maturity level of parent kelp are significantly associated with MD severity. The divergence of the epiphytic bacterial communities and assembly patterns were also determined to be closely linked to MD severity. The present study enhances our understanding of the factors that significantly correlate with MD severity, and provides insights into the potential roles that epiphytic bacteria may play in the mechanism driving this association. Thus, it is proposed that external factors such as longer light exposure and parent kelp may be sufficient to cause a poor health status for young sporophytes, which then induces negative effects on their subsequent growth and development. Under such a stressed context, the structures and functions of the epimicrobiome were significantly disrupted compared with those of seedlings cultivated under standard conditions, which in turn significantly increased the MD severity through modifying host–bacteria interactions, such as lacking sufficient functions to fulfill host growth and development and/or inducing potential pathogens to manipulate cellular processes. However, more data (e.g., metagenomic and metatranscriptomic data) are still required to explore how the influencing factors, host cellular processes, and epiphytic microbiota interact with disease severity to determine the specific underlying causes of the disease. Besides, the determined keystone taxa, e.g., Halomonas, Pseudoalteromonas, Pseudomonas, Loktanella, and Cobetia, also require these fine-scale data in addition to their co-incubation with seedlings to provide deeper insights into their significant roles for the disease.
Data availability statement
The datasets presented in this study can be found in online repositories. The names of the repository/repositories and accession number(s) can be found in the article/Supplementary Material.
Author contributions
YY, ZM, and JL designed the experiments. SW, KL, and HY conducted experiments. YY performed the microbiome analysis. YY and JL prepared the manuscript. XR was involved in designing the experiments. All authors contributed to the article and approved the submitted version.
Funding
This work was financially supported by the China Agriculture Research System (CARS-50).
Acknowledgments
We would like to thank Weihai Changqing Ocean Science and Technology Co., Ltd. for their many help in the investigation of malformation disease, including providing samples for surveys and laboratories for the incubation in the present study.
Conflict of interest
The authors declare that the research was conducted in the absence of any commercial or financial relationships that could be construed as a potential conflict of interest.
Publisher’s note
All claims expressed in this article are solely those of the authors and do not necessarily represent those of their affiliated organizations, or those of the publisher, the editors and the reviewers. Any product that may be evaluated in this article, or claim that may be made by its manufacturer, is not guaranteed or endorsed by the publisher.
Supplementary material
The Supplementary Material for this article can be found online at: https://www.frontiersin.org/articles/10.3389/fmars.2023.1089349/full#supplementary-material
References
Aires T., Stuij T. M., Muyzer G., Serrão E. A., Engelen A. H. (2021). Characterization and comparison of bacterial communities of an invasive and two native Caribbean seagrass species sheds light on the possible influence of the microbiome on invasive mechanisms. Front. Microbiol. 12, 653998. doi: 10.3389/fmicb.2021.653998
Alsufyani T., Califano G., Deicke M., Grueneberg J., Weiss A., Engelen A. H., et al. (2020). Macroalgal-bacterial interactions: identification and role of thallusin in morphogenesis of the seaweed Ulva (Chlorophyta). J. Exp. Bot. 71, 3340–3349. doi: 10.1093/jxb/eraa066
Ashen J. B., Goff L. J. (1996). Molecular identification of a bacterium associated with gall formation in the marine red alga Prionitis lanceolata. J. Phycol. 32, 286–297. doi: 10.1111/j.0022-3646.1996.00286.x
Banerjee S., Kirkby C. A., Schmutter D., Bissett A., Kirkegaard J. A., Richardson A. E. (2016). Network analysis reveals functional redundancy and keystone taxa amongst bacterial and fungal communities during organic matter decomposition in an arable soil. Soil Biol. Biochem. 97, 188–198. doi: 10.1016/j.soilbio.2016.03.017
Banerjee S., Schlaeppi K., van der Heijden M. G. A. (2018). Keystone taxa as drivers of microbiome structure and functioning. Nat. Rev. Microbiol. 16, 567–576. doi: 10.1038/s41579-018-0024-1
Barberán A., Bates S. T., Casamayor E. O., Fierer N. (2012). Using network analysis to explore co-occurrence patterns in soil microbial communities. ISME J. 6, 343–351. doi: 10.1038/ismej.2011.119
Bastian M., Heymann S., Jacomy M. (2009) Gephi: an open source software for exploring and manipulating networks. Available at: http://gephi.org.
Behera D. P., Ingle K. N., Mathew D. E., Dhimmar A., Sahastrabudhe H., Sahu S. K., et al. (2022). Epiphytism, diseases and grazing in seaweed aquaculture: a comprehensive review. Rev. Aquacult. 14, 1345–1370. doi: 10.1111/raq.12653
Berry D., Widder S. (2014). Deciphering microbial interactions and detecting keystone species with co-occurrence networks. Front. Microbiol. 5, 219. doi: 10.3389/fmicb.2014.00219
Burke C., Steinberg P., Rusch D., Kjelleberg S., Thomas T. (2011a). Bacterial community assembly based on functional genes rather than species. Proc. Natl. Acad. Sci. U.S.A. 108, 14288–14293. doi: 10.1073/pnas.1101591108
Burke C., Thomas T., Lewis M., Steinberg P., Kjelleberg S. (2011b). Composition, uniqueness and variability of the epiphytic bacterial community of the green alga Ulva australis. ISME J. 5, 590–600. doi: 10.1038/ismej.2010.164
Burns A. R., Stephens W. Z., Stagaman K., Wong S., Rawls J. F., Guillemin K., et al. (2016). Contribution of neutral processes to the assembly of gut microbial communities in the zebrafish over host development. ISME J. 10, 655–664. doi: 10.1038/ismej.2015.142
Chambers J. M., Freeny A., Heiberger R. M. (1992). “Analysis of variance: designed experiments,” in Statistical models in s. Eds. Chambers J. M., Hastie T. J. (Pacific Grove, CA: Wadsworth & Brooks/Cole).
Chase J. M., Myers J. A. (2011). Disentangling the importance of ecological niches from stochastic processes across scales. Philos. Trans. R. Soc B Biol. Sci. 366, 2351–2363. doi: 10.1098/rstb.2011.0063
Chen T., Chen X., Zhang S., Zhu J., Tang B., Wang A., et al. (2021). The genome sequence archive family: Toward explosive data growth and diverse data types. Genom. Proteom. Bioinf. 19, 578–583. doi: 10.1016/j.gpb.2021.08.001
Chen T., Liu Y., Huang L. (2022). ImageGP: an easy-to-use data visualization web server for scientific researchers. iMeta 1, e5. doi: 10.1002/imt2.5
Dai W., Chen J., Xiong J. (2019). Concept of microbial gatekeepers: positive guys? Appl. Microbiol. Biotechnol. 103, 633–641. doi: 10.1007/s00253-018-9522-3
Dai W., Sheng Z., Chen J., Xiong J. (2020). Shrimp disease progression increases the gut bacterial network complexity and abundances of keystone taxa. Aquaculture 517, 734802. doi: 10.1016/j.aquaculture.2019.734802
Dai W., Yu W., Xuan L., Tao Z., Xiong J. (2018). Integrating molecular and ecological approaches to identify potential polymicrobial pathogens over a shrimp disease progression. Appl. Microbiol. Biotechnol. 102, 3755–3764. doi: 10.1007/s00253-018-8891-y
De Schryver P., Defoirdt T., Boon N., Verstraete W., Bossier P. (2012). “Managing the microbiota in aquaculture systems for disease prevention and control,” in Infectious disease in aquaculture: Prevention and control. Ed. Austin B. (Sawston: Woodhead Publishing), 394–418. doi: 10.1533/9780857095732.3.394
Dittami S. M., Arboleda E., Auguet J.-C., Bigalke A., Briand E., Cárdenas P., et al. (2021). A community perspective on the concept of marine holobionts: current status, challenges, and future directions. PeerJ 9, e10911. doi: 10.7717/peerj.10911
Dittami S. M., Duboscq-Bidot L., Perennou M., Gobet A., Corre E., Boyen C., et al. (2016). Host-microbe interactions as a driver of acclimation to salinity gradients in brown algal cultures. ISME J. 10, 51–63. doi: 10.1038/ismej.2015.104
Dogs M., Wemheuer B., Wolter L., Bergen N., Daniel R., Simon M., et al. (2017). Rhodobacteraceae on the marine brown alga Fucus spiralis are abundant and show physiological adaptation to an epiphytic lifestyle. Syst. Appl. Microbiol. 40, 370–382. doi: 10.1016/j.syapm.2017.05.006
Douglas G. M., Maffei V. J., Zaneveld J. R., Yurgel S. N., Brown J. R., Taylor C. M., et al. (2020). PICRUSt2 for prediction of metagenome functions. Nat. Biotechnol. 38, 685–688. doi: 10.1038/s41587-020-0548-6
Duan D., Miao G., Wang X. (2015). Aquacultural biology of saccharina japonica (Beijing: Science Press).
Edgar R. C. (2010). Search and clustering orders of magnitude faster than BLAST. Bioinformatics 26, 2460–2461. doi: 10.1093/bioinformatics/btq461
Egan S., Fernandes N. D., Kumar V., Gardiner M., Thomas T. (2014). Bacterial pathogens, virulence mechanism and host defence in marine macroalgae. Environ. Microbiol. 16, 925–938. doi: 10.1111/1462-2920.12288
Egan S., Gardiner M. (2016). Microbial dysbiosis: rethinking disease in marine ecosystems. Front. Microbiol. 7, 991. doi: 10.3389/fmicb.2016.00991
Florez J. Z., Camus C., Hengst M. B., Marchant F., Buschmann A. H. (2019). Structure of the epiphytic bacterial communities of Macrocystis pyrifera in localities with contrasting nitrogen concentrations and temperature. Algal Res. 44, 101706. doi: 10.1016/j.algal.2019.101706
Gachon C. M. M., Sime-Ngando T., Strittmatter M., Chambouvet A., Kim G. H. (2010). Algal diseases: spotlight on a black box. Trends Plant Sci. 15, 633–640. doi: 10.1016/j.tplants.2010.08.005
Ghaderiardakani F., Coates J. C., Wichard T. (2017). Bacteria-induced morphogenesis of Ulva intestinalis and Ulva mutabilis (Chlorophyta): a contribution to the lottery theory. FEMS Microbiol. Ecol. 93, fix094. doi: 10.1093/femsec/fix094
Ghaderiardakani F., Quartino M. L., Wichard T. (2020). Microbiome-dependent adaptation of seaweeds under environmental stresses: A perspective. Front. Mar. Sci. 7, 575228. doi: 10.3389/fmars.2020.575228
Goecke F., Labes A., Wiese J., Imhoff J. F. (2010). Chemical interactions between marine macroalgae and bacteria. Mar. Ecol. Prog. Ser. 409, 267–299. doi: 10.3354/meps08607
Han X. (2015). Identification and complete genome sequencing of a cobetia sp. causing green rot disease in porphyra haitanensis (Qingdao: Ocean University of China).
Harvell C. D., Mitchell C. E., Ward J. R., Altizer S., Dobson A. P., Ostfeld R. S., et al. (2002). Climate warming and disease risks for terrestrial and marine biota. Science 296, 2158–2162. doi: 10.1126/science.1063699
Hasselström L., Visch W., Gröndahl F., Nylund G. M., Pavia H. (2018). The impact of seaweed cultivation on ecosystem services - a case study from the west coast of Sweden. Mar. pollut. Bull. 133, 53–64. doi: 10.1016/j.marpolbul.2018.05.005
Herren C. M., McMahon K. D. (2018). Keystone taxa predict compositional change in microbial communities. Environ. Microbiol. 20, 2207–2217. doi: 10.1111/1462-2920.14257
Joint I., Tait K., Wheeler G. (2007). Cross-kingdom signalling: exploitation of bacterial quorum sensing molecules by the green seaweed Ulva. Philos. Trans. R. Soc B Biol. Sci. 362, 1223–1233. doi: 10.1098/rstb.2007.2047
Kurtz Z. D., Müller C. L., Miraldi E. R., Littman D. R., Blaser M. J., Bonneau R. A. (2015). Sparse and compositionally robust inference of microbial ecological networks. PloS Comput. Biol. 11, e1004226. doi: 10.1371/journal.pcbi.1004226
Lachnit T., Blümel M., Imhoff J. F., Wahl M. (2009). Specific epibacterial communities on macroalgae: phylogeny matters more than habitat. Aquat. Biol. 5, 181–186. doi: 10.3354/ab00149
Lachnit T., Meske D., Wahl M., Harder T., Schmitz R. (2011). Epibacterial community patterns on marine macroalgae are host-specific but temporally variable. Environ. Microbiol. 13, 655–665. doi: 10.1111/j.1462-2920.2010.02371.x
Lemay M. A., Martone P. T., Hind K. R., Lindstrom S. C., Wegener Parfrey L. (2018). Alternate life history phases of a common seaweed have distinct microbial surface communities. Mol. Ecol. 27, 3555–3568. doi: 10.1111/mec.14815
Li J., Majzoub M. E., Marzinelli E. M., Dai Z., Thomas T., Egan S. (2022). Bacterial controlled mitigation of dysbiosis in a seaweed disease. ISME J. 16, 378–387. doi: 10.1038/s41396-021-01070-1
Ling F., Egan S., Zhuang Y., Chang L., Xiao L., Yang Q., et al. (2022). Epimicrobiome shifts with bleaching disease progression in the brown seaweed Saccharina japonica. Front. Mar. Sci. 9, 865224. doi: 10.3389/fmars.2022.865224
Lin W., Lu J., Yao H., Lu Z., He Y., Mu C., et al. (2021). Elevated pCO2 alters the interaction patterns and functional potentials of rearing seawater microbiota. Environ. pollut. 287, 117615. doi: 10.1016/j.envpol.2021.117615
Li J., Pang S., Shan T., Su L. (2020). Changes of microbial community structures associated with seedlings of Saccharina japonica at early stage of outbreak of green rotten disease. J. Appl. Phycol. 62, 191. doi: 10.1007/s10811-019-01975-7
Lomartire S., Marques J. C., Gonçalves A. M. M. (2021). An overview to the health benefits of seaweeds consumption. Mar. Drugs 19, 341. doi: 10.3390/md19060341
Longford S. R., Campbell A. H., Nielsen S., Case R. J., Kjelleberg S., Steinberg P. D. (2019). Interactions within the microbiome alter microbial interactions with host chemical defences and affect disease in a marine holobiont. Sci. Rep. 9, 1363. doi: 10.1038/s41598-018-37062-z
Marshall K., Joint I., Callow M. E., Callow J. A. (2006). Effect of marine bacterial isolates on the growth and morphology of axenic plantlets of the green alga Ulva linza. Microb. Ecol. 52, 302–310. doi: 10.1007/s00248-006-9060-x
Martin M., Barbeyron T., Martin R., Portetelle D., Michel G., Vandenbol M. (2015). The cultivable surface microbiota of the brown alga Ascophyllum nodosum is enriched in macroalgal-polysaccharide-degrading bacteria. Front. Microbiol. 6, 1487. doi: 10.3389/fmicb.2015.01487
Ma B., Wang H., Dsouza M., Lou J., He Y., Dai Z., et al. (2016). Geographic patterns of co-occurrence network topological features for soil microbiota at continental scale in eastern China. ISME J. 10, 1891–1901. doi: 10.1038/ismej.2015.261
Ma B., Wang Y., Ye S., Liu S., Stirling E., Gilbert J. A., et al. (2020). Earth microbial co-occurrence network reveals interconnection pattern across microbiomes. Microbiome 8, 82. doi: 10.1186/s40168-020-00857-2
Moenne A., González A., Sáez C. A. (2016). Mechanisms of metal tolerance in marine macroalgae, with emphasis on copper tolerance in chlorophyta and rhodophyta. Aquat. Toxicol. 176, 30–37. doi: 10.1016/j.aquatox.2016.04.015
Morrissey K. L., Iveša L., Delva S., D’Hondt S., Willems A., De Clerck O. (2021). Impacts of environmental stress on resistance and resilience of algal-associated bacterial communities. Ecol. Evol. 11, 15004–15019. doi: 10.1002/ece3.8184
Nakanishi K., Nishijima M., Nishimura M., Kuwano K., Saga N. (1996). Bacteria that induce morphogenesis in Ulva pertusa (Chlorophyta) grown under axenic conditions. J. Phycol. 32, 479–482. doi: 10.1111/j.0022-3646.1996.00479.x
Newman M. E. J. (2002). The structure and function of networks. Comput. Phys. Commun. 147, 40–45. doi: 10.1016/S0010-4655(02)00201-1
Newman M. E. J. (2006). Modularity and community structure in networks. Proc. Natl. Acad. Sci. U.S.A. 103, 8577–8582. doi: 10.1073/pnas.0601602103
Ning D., Deng Y., Tiedje J. M., Zhou J. (2019). A general framework for quantitatively assessing ecological stochasticity. Proc. Natl. Acad. Sci. U.S.A. 116, 16892–16898. doi: 10.1073/pnas.1904623116
Oksanen J., Simpson G., Blanchet F. G., Kindt R., Legendre P., Minchin P., et al. (2019) Vegan: community ecology package. package version 2.5-6. Available at: https://cran.r-project.org/package=vegan.
Parks D. H., Tyson G. W., Hugenholtz P., Beiko R. G. (2014). STAMP: statistical analysis of taxonomic and functional profiles. Bioinformatics 30, 3123–3124. doi: 10.1093/bioinformatics/btu494
Pearson D. E., Ortega Y. K., Eren Ö., Hierro J. L. (2018). Community assembly theory as a framework for biological invasions. Trends Ecol. Evol. 33, 313–325. doi: 10.1016/j.tree.2018.03.002
Peng Y., Li W. (2013). A bacterial pathogen infecting gametophytes of saccharina japonica (Laminariales, phaeophyceae). Chin. J. Oceanol. Limnol. 31, 366–373. doi: 10.1007/s00343-013-2136-9
Qian R., Zhang Z., Li X., Pan J., Sheng B., Jiang Y. (2016). Disease occurrence and controlling methods in nursery-cultivated Saccharina japonica. China Fish. 6, 96–99.
Rognes T., Flouri T., Nichols B., Quince C., Mahé F. (2016). VSEARCH: a versatile open source tool for metagenomics. PeerJ 4, e2584. doi: 10.7717/peerj.2584
Saha M., Weinberger F. (2019). Microbial “gardening” by a seaweed holobiont: surface metabolites attract protective and deter pathogenic epibacterial settlement. J. Ecol. 107, 2255–2265. doi: 10.1111/1365-2745.13193
Sheng B., Wang Q., Zhang Z., Pan J., Qu S. (2016). Prevention and treatment of sporeling malformation disease in the production of Saccharina japonica. Hebei Fish. 10, 45–46. doi: 10.3969/j.issn.1004−6755.2016.10.014
Singh R. P., Reddy C. R. K. (2015). Unraveling the functions of the macroalgal microbiome. Front. Microbiol. 6, 1488. doi: 10.3389/fmicb.2015.01488
Spoerner M., Wichard T., Bachhuber T., Stratmann J., Oertel W. (2012). Growth and thallus morphogenesis of Ulva mutabilis (Chlorophyta) depends on a combination of two bacterial species excreting regulatory factors. J. Phycol. 48, 1433–1447. doi: 10.1111/j.1529-8817.2012.01231.x
Tapia J. E., González B., Goulitquer S., Potin P., Correa J. A. (2016). Microbiota influences morphology and reproduction of the brown alga Ectocarpus sp. Front. Microbiol. 7, 197. doi: 10.3389/fmicb.2016.00197
Tseng C. K. (2001). Algal biotechnology industries and research activities in China. J. Appl. Phycol. 13, 375–380. doi: 10.1023/A:1017972812576
Wahl M., Goecke F., Labes A., Dobretsov S., Weinberger F. (2012). The second skin: ecological role of epibiotic biofilms on marine organisms. Front. Microbiol. 3, 292. doi: 10.3389/fmicb.2012.00292
Wang G., Lu B., Shuai L., Li D., Zhang R. (2014). Microbial diseases of nursery and field-cultivated Saccharina japonica (Phaeophyta) in China. Algol. Stud. 145, 39–51. doi: 10.1127/1864-1318/2014/0167
Wang G., Shuai L., Li Y., Lin W., Zhao X., Duan D. (2008). Phylogenetic analysis of epiphytic marine bacteria on hole-rotten diseased sporophytes of Laminaria japonica. J. Appl. Phycol. 20, 403–409. doi: 10.1007/s10811-007-9274-4
Ward G. M., Kambey C. S. B., Faisan J. P., Tan P., Daumich C. C., Matoju I., et al. (2021). Ice-ice disease: an environmentally and microbiologically driven syndrome in tropical seaweed aquaculture. Rev. Aquacult. 47, 361. doi: 10.1111/raq.12606
Watt C. A., Scrosati R. A. (2014). Seaweeds as ecosystem engineers. Bull. Ecol. Soc Am. 95, 250–251. doi: 10.1890/0012-9623-95.3.250
Wichard T. (2015). Exploring bacteria-induced growth and morphogenesis in the green macroalga order ulvales (Chlorophyta). Front. Plant Sci. 6, 86. doi: 10.3389/fpls.2015.00086
Wu C., Gao N., Che D., Zhou B., Cai P., Dong S., et al. (1979). On the malformation disease of Laminaria sporelings. Oceanol. Et Limnol. Sin. 10, 238–247.
Xiong J. (2018). Progress in the gut microbiota in exploring shrimp disease pathogenesis and incidence. Appl. Microbiol. Biotechnol. 102, 7343–7350. doi: 10.1007/s00253-018-9199-7
Xiong J., Zhu J., Dai W., Dong C., Qiu Q., Li C. (2017). Integrating gut microbiota immaturity and disease-discriminatory taxa to diagnose the initiation and severity of shrimp disease. Environ. Microbiol. 19, 1490–1501. doi: 10.1111/1462-2920.13701
Xue Y., Bao Y., Zhang Z., Zhao W., Xiao J., He S., et al. (2022). Database resources of the national genomics data center, China national center for bioinformation in 2022. Nucleic Acids Res. 50, D27–D38. doi: 10.1093/nar/gkab951
Yan Y., Wang S., Li J., Liu F., Mo Z. (2022). Alterations in epiphytic bacterial communities during the occurrence of green rot disease in Saccharina japonica seedlings. J. Mar. Sci. Eng. 10, 730. doi: 10.3390/jmse10060730
Yao Z., Yang K., Huang L., Huang X., Qiuqian L., Wang K., et al. (2018). Disease outbreak accompanies the dispersive structure of shrimp gut bacterial community with a simple core microbiota. AMB Express 8, 120. doi: 10.1186/s13568-018-0644-x
Zhang R., Chang L., Xiao L., Zhang X., Han Q., Li N., et al. (2020). Diversity of the epiphytic bacterial communities associated with commercially cultivated healthy and diseased Saccharina japonica during the harvest season. J. Appl. Phycol. 32, 2071–2080. doi: 10.1007/s10811-019-02025-y
Zhang J., Liu Y.-X., Zhang N., Hu B., Jin T., Xu H., et al. (2019). NRT1.1B is associated with root microbiota composition and nitrogen use in field-grown rice. Nat. Biotechnol. 37, 676–684. doi: 10.1038/s41587-019-0104-4
Zhou J., Ning D. (2017). Stochastic community assembly: does it matter in microbial ecology? Microbiol. Mol. Biol. Rev. 81, e00002–e00017. doi: 10.1128/MMBR.00002-17
Zhu J., Dai W., Qiu Q., Dong C., Zhang J., Xiong J. (2016). Contrasting ecological processes and functional compositions between intestinal bacterial community in healthy and diseased shrimp. Microbiol. Ecol. 72, 975–985. doi: 10.1007/s00248-016-0831-8
Zozaya-Valdés E., Egan S., Thomas T. (2015). A comprehensive analysis of the microbial communities of healthy and diseased marine macroalgae and the detection of known and potential bacterial pathogens. Front. Microbiol. 6, 146. doi: 10.3389/fmicb.2015.00146
Keywords: disease severity, epiphytic bacterial community, light exposure, malformation disease, maturity level, Saccharina japonica
Citation: Yan Y, Wang S, Liu K, Mo Z, Yang H, Rong X and Li J (2023) Divergence of epibacterial community assemblage correlates with malformation disease severity in Saccharina japonica seedlings. Front. Mar. Sci. 10:1089349. doi: 10.3389/fmars.2023.1089349
Received: 04 November 2022; Accepted: 05 January 2023;
Published: 26 January 2023.
Edited by:
Guangyi Fan, Beijing Genomics Institute (BGI), ChinaReviewed by:
Shijie Bai, Institute of Deep-Sea Science and Engineering, Chinese Academy of Sciences (CAS), ChinaDu Hong, Shantou University, China
Meng Yan, City University of Hong Kong, Hong Kong, Hong Kong SAR, China
Copyright © 2023 Yan, Wang, Liu, Mo, Yang, Rong and Li. This is an open-access article distributed under the terms of the Creative Commons Attribution License (CC BY). The use, distribution or reproduction in other forums is permitted, provided the original author(s) and the copyright owner(s) are credited and that the original publication in this journal is cited, in accordance with accepted academic practice. No use, distribution or reproduction is permitted which does not comply with these terms.
*Correspondence: Jie Li, lijie@ysfri.ac.cn