- 1Computational Science, The Jackson Laboratory, Farmington, CT, United States
- 2Department of Earth System Sciences and Environmental Technologies, National Research Council of Italy (CNR), Rome, Italy
Anthropogenic and natural factors impacting health and well-being in coastal waters, regional seas, and the global ocean have long been recognized by the marine scientists, however not as much by the medical and public health community. Although establishing causal effects that directly or indirectly affect human health-related conditions is problematic and depends on the complex marine ecosystem, significant influences are present at both local and global levels, i.e., specific to coastal areas but also associated with sea activities referred to the ‘ocean health’ status. This offers a good rationale for an assessment of the human-marine environment interaction, evolution and complexity landscape. The health ecosystem as a whole (humans and environment, especially marine in our interests) is a complex bio-entity whose dynamics are largely unknown due to the presence of biodiversity and heterogeneity. In parallel, this complexity translates into various new processes that the stakeholders face to establish possible interventions and preserve the sustainability. A major checkpoint in our discussion refers to how to leverage the consolidated and indeed pervasive role of digital information across multiple fields and disciplines, supported by developments in artificial intelligence, machine learning and network science. This is an urgency, as the scientific marine community and the public health policy makers are struggling to gather big data from multiple sources and/or devices that help reveal the marine environmental status. Improvements in the ability of analyzing efficiently and effectively data are needed, and we suggest to profitably look at knowledge transfer strategies. In particular, considering and valuing how the scientific biomedical community has made use of network inference approaches to better understand complex biosystems in both structural and functional terms, we believe that the existing knowledge base can be further generalized to deal with the marine environmental ecosystem context.
Human and marine ecosystems
The global ocean is the largest connected ecosystem on Earth and influences our climate, weather, global food production and international trade. Of interest here is to examine the link between human and ocean health (Fleming et al., 2019; Franke et al., 2020) and discuss how to approach their inherent systems complexity.
To start, seas and oceans constitute overall a complex environment whose dynamics are governed by a) Interconnected physical and biological variables, and b) A variety of human activities determining the marine ecosystem’s state, and in turn directly or indirectly affecting the human health factors. Examples of how the ocean can exert positive or negative effects are linked to food (say, the chemical pollution on fish that enters the food chain, wellness (the access to coastal areas facilitating physical exercise and reducing cardiac diseases), climate (the mitigation of hot waves, the increase of hurricanes and harmful algal blooms (McMichael and WHO, 2003; Borja et al., 2020), etc.). As a note, more than 250 million clinical cases of gastroenteritis and respiratory disease are linked worldwide annually to swimming in contaminated seas (Inniss and Simcock, 2016; Fleming et al., 2019; Landrigan et al., 2020).
The United Nations has announced the Decade of Ocean Science for Sustainable Development from 2021 to 2030 (see here at: forum.oceandecade.org) with the focus on transforming the way of interacting with our seas and ultimately build a fruitful collaboration between citizens, policy-makers, entrepreneurs and researchers to enable a sustainable socio-economic use of the marine resources and space. Expanding this horizon, there is also a scientific challenge, that of a critical understanding of the complex relations between people and the environment in which they live, and this is the main argument of the ecological anthropology in the Anthropocene (Liu et al., 2021). Then, given the multidisciplinary context, promoting the synergy between marine and health sciences research communities would be reasonable.
Recently, some descriptions have appeared of the different marine exposure environments in relation with human health outcomes (‘Seas, Oceans & Public Health in Europe’, https://sophie2020.eu/). There are problems with this type of data. One is sparsity, another is the lack of validation and thus the need of providing through more models a better understanding of the processes that link marine environments with human health (Short et al., 2021). A good starting point is that human and marine health are an example of ‘coupled ecosystem’ in which systems properties can emerge due to interaction effects. Also, health and well-being at one side and good environmental marine status at the other side offer a general perception of representing ideal conditions to guarantee sustainability. However, a deeper analysis involving extra-environmental factors useful to maintain functionality may determine key system states.
Furthermore, while the biomedical and health communities have systematically prioritized the investigation of systems complexity with emphasis on data integration, explainable models etc., to develop new domains like personalized, precision and translational medicine, the marine community is struggling with the problems of collecting data efficiently and providing effective analyses. Among the guidelines for managing in a cost-effective way resources and infrastructures, a central role is played by ‘soft’ activities like data collection, performance analyses, support to decisions etc. Recently, a first attempt to implement an innovative approach for assessing the marine environmental status has been framed within a joint action launched by the European Initiative “Healthy and Productive Seas and Oceans” (Sprovieri et al., 2021b).
Our work here is based on the delineation of a few directions that would benefit from a better use of data to face current knowledge gaps. They are centered on: A) Approaches for assessing marine environmental status; B) Feasible policies enabling knowledge transfer from other fields; C) Actions from the marine scientific community toward sustainability (i.e., challenges and interventions with reference to people-environment interaction).
Context of reference
Background
From an anthropological point of view, a good status of an environment like the oceans would guarantee healthy conditions for those species populating for millennia our planet and successfully selected based on their evolutionary fitness (see for instance: ‘Understanding theories of human-environment relationship’, by Hemlata O, https://egyankosh.ac.in/bitstream/123456789/71432/1/Unit-9.pdf). In consideration of evolving environmental conditions and thus changes, it is also worth noting that humans look at places with most suitable living conditions; however, when these changes are fast or massive with multiple dimensions involved, a modification of health status could be reasonably expected.
A theoretical and practical foundation for research on Coupled Human and Natural Systems (CHANS) has been provided (Liu et al., 2021) and can constitute the framework for understanding the increased complexity of the Anthropocene. In general, the main challenge in understanding the human-environmental relationship has roots in the environmental determinism and possibilism, then evolves in the cultural ecology and the recent concept of ecosystem as the “complex of living organisms, their physical environment and all their interrelationships in a particular unit of space”. This last definition has definitely introduced the need to consider the system’s complexity at the cost of reducing the attention on the causes of the changes driven by humans and the strategies essential to an ecosystem approach maintained in equilibrium or in homeostatic conditions.
CHANS concepts have been also applied to get insights into restoration and governance of ocean ecosystems (Lubchenco and Petes, 2010). When dealing with marine ecosystems, a good status depends on the use of them. In fact, a marine area enables economic exploitation, for instance, and the human activities can impact, and even transform, the environmental status and conditions. These effects can be direct or indirect, and visible in the short or long term (cumulatively). The anthropogenic activities (i.e., maritime shipping, fishing, aquaculture, renewable energy production, oil and gas exploration and exploitation, extraction of raw materials, ecosystem and biodiversity conservation, tourism, and underwater cultural heritage) may generate conflict due to their demand of maritime space and in relation to productivity, but mostly require a healthy marine environment.
In Europe, the Maritime Spatial Planning Directive (MSPD) is the legislative tool used to address the above issues and aims to maintain the value of marine resources and enable a sustainable use of the economic potential of the marine space (Ehler and Douvere, 2009). Within the same European legislative package addressing marine and maritime aspects, the Marine Strategy Framework Directive (MSFD, 2008/56/EC) constitutes an overarching framework that sets the environmental objectives by integrating all the activities that affect marine ecosystems and by ensuring sustainability.
The MSPD and MSFD are indirectly cross-linked, both based on the adoption of an ecosystem approach and both with the aim to ensure that the collective anthropogenic pressures are compatible with the achievement of Good Environmental Status (GES). MSPD and MSFD represent therefore different perspectives on the marine environment: MSPD asks the maritime sectors to be balanced, while MSFD focuses on the ecosystem conservation. Therefore, at a political level, the assessment of the status of marine ecosystems is considered fundamental to inform management decisions (Eborja et al., 2016).
Moving forward
The marine policies, whether driven by economic or environmental priorities, need GES evaluation based on the best available ecosystem’s knowledge, including the dynamics. Policy makers and managers require scientists to support their decisions. The main challenge is that marine scientists are still pursuing consensus about a definition of GES. The evaluation of the impacts of collective pressures aimed to keep them within levels compatible with a sustainable environment, implies an understanding of the processes guiding the system and an assessment of the status. To accomplish such tasks, comprehensive ecosystem models should provide robust predictions based on collecting data and analyzing salient features. In addition, marine observations are very expensive and public funding agencies are reluctant to increase their investments (Edgar et al., 2016). A comprehensive review of methods and criteria adopted to evaluate the cost-effectiveness of marine monitoring suggests that this kind of assessments lacks scientific rigor and focus. This results from the difficult association between monetary costs and use of data: cost information is generally difficult to obtain and data usefulness is difficult to discern (Hyvärinen et al., 2021).
Viable solution: linear approach
Due to the need of providing marine data to support governance and management tasks, a first step requires that an ocean observing system framework must be conceived to coordinate and integrate the acquisition of physical, biogeochemical, and biological essential ocean variables (EOVs). As a note, for more than two decades the Global Ocean Observing System (GOOS) has requested to governments priority actions for creating a focal initiative advising on physics, climate and biogeochemistry observations (Lindstrom, 2018). The proliferation of proposed monitoring frameworks designed to identify “essential variables” under different contexts and political drivers has generated confusion and delays rather than effective actions. Consequently, despite an increased number of GOOS physical and biogeochemical EOVs (i.e., microbial diversity, biomass) supported by essential climate variables (ECVs) (Bauer et al., 2015; O’Brien et al., 2017), two issues have remained unsolved: 1. The understanding of the contextual drivers of the ecosystem’s stress (Sun et al., 2012); 2. The interconnections between the different agents (natural and anthropogenic).
In practice, EOVs should address fundamental characteristics of the marine ecosystems that can be combined into indicators to describe the real-world complexity, to monitor temporal and spatial dynamics in the environmental states, and to deliver information that support interventions (Hayes et al., 2015). In this scenario, EOVs represent necessary measurements when assessing the state and change of marine ecosystems. Apart from a few cases of EOVs capturing the dynamics of specific ecosystems, for instance the macroalgal canopy (Strain et al., 2014), the complexity of ecosystems comes with nonlinear dynamics, and the study of some independent variables or indicators is not sufficient. In principle, EOVs or any indicator obtained from combined data must be relevant, feasible and cost-effective.
Figure 1 shows a framework in use to address the state of the marine environment. While dealing with many variables and approaches, the acquired data often captures specific environmental aspects that are then analyzed by very different models (a comprehensive list of marine data and algorithms (with software) is available at https://www.seadatanet.org). In 2018, a survey on biological ocean observation completed by more than 100 observing program leaders/managers reported that two thirds of the observing programs provided the abundance, diversity, distribution and coverage as indicators of the marine ecosystem (Miloslavich et al., 2018).
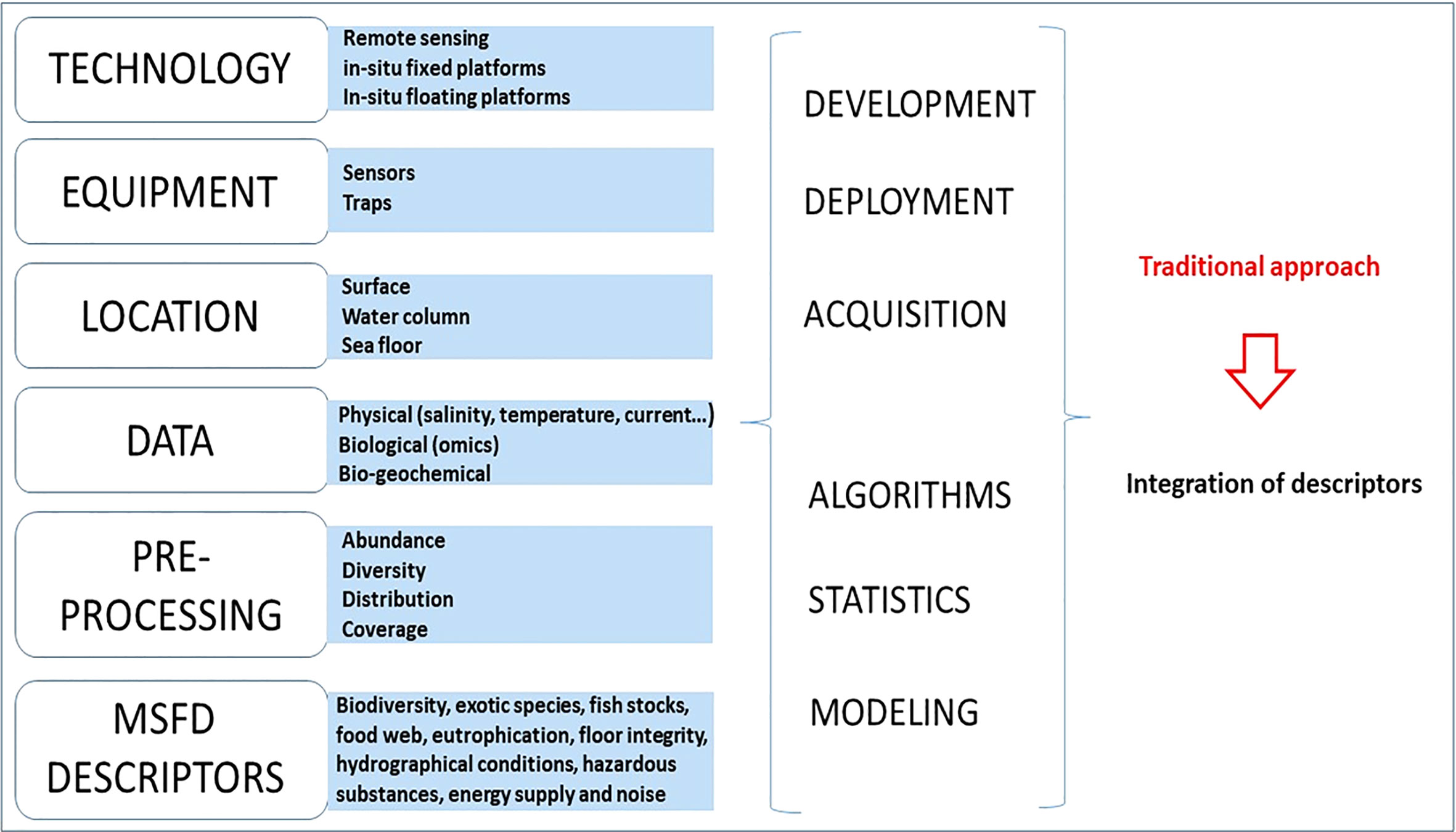
Figure 1 Sketch of the marine environment traditional MSFD learning approach towards the assessment of the environmental status, based on descriptors of different marine agents.
Viable solution: complexity approach
Recently, in an attempt to deal formally with complexity within the marine environment, network science attracted more attention and started to integrate within traditional marine domains. Apart from the use of indices describing the ecosystem’s state, an open question is how to link the ecosystem’s responses to both structural and functional aspects. This might be a crucial step for quantifying ensemble properties and investigating dynamic aspects related to resistance or resilience.
Trophic interactions represented through food webs are examples of how species are organized in a marine ecosystem. Their quantification and characterization by ecological network analysis (ENA) indices have rapidly increased (Saint-Béat et al., 2015; Fath et al., 2019). ENA is defined as “a systems-oriented methodology to analyze within system interactions used to identify holistic properties that are otherwise not evident from the direct observations”. Different approaches, from binary (presence or absence of link between species) to flow-weighted networks, can be considered (Zhang and Wu, 2013; Niquil et al., 2014; Corso et al., 2015).
However, the approach of leveraging a single parameter which is measurable at network scale (e.g., diversity, connectance, interaction strength) does not allow to draw conclusions on system’s behavior, and especially stability. To address such issues, the ENA indices were developed to provide a more integrated framework and many theories have been proposed for linking ecosystem stability to some ENA indices (e.g., omnivory, cycling and ascendency). Nevertheless, ENA remains incomplete for describing real conservation cases that combine local and/or global stability and is not widely used in the marine contexts (Landi et al., 2018). Thermodynamics represents the reference physical theoretical framework for describing the properties of the ecosystem, in particular entropy (note that the link between thermodynamics and ecology has a long history (Nielsen et al., 2020)).
Challenges
Multiple tools exist to derive various indicators of both structure and functioning of ecosystems (Rombouts et al., 2013; de la Vega et al., 2018). The ENA indices are considered useful tools to evaluate ecosystem states and to differentiate ecosystem structures under assumed boundary conditions. Other indices are focused on biodiversity. Unfortunately, a generalization of the connection between emergent ecosystem properties and functioning to the specific system’s components of the system is lacking or at least not adopted by the marine community. Some valuable approaches have been proposed for the description of the ecosystem resilience and the identification of tipping points (see Petri nets or Boolean networks or different models based on discrete events and qualitative systems (Gaucherel et al., 2017; Gaucherel and Pommereau, 2019; Gaucherel et al., 2020)). The underlying assumption is that it is possible to exhaustively evaluate qualitatively behavioral aspects from the ecosystem interactions. Such models, also used in ecology (Ewing et al., 2002; Cordier et al., 2014; Baldan et al., 2018), lack in general quantitative metrics to infer system properties (Koch et al., 2011).
One problem is that with partial and short-term observations the models remain phenomenological, focusing on short-term dynamics and patterns (Sole and Bascompte, 2006). Ecologists analyzing a snapshot of the ecosystem’s patterns instead of the long-term dynamics, end up restricting the analysis to isolated states rather than the interconnected trajectories, and supporting decisions based on myopic statistics (Badii and Politi, 1999). Physics models have addressed phase states and indicators of complex systems dynamics (Biggs et al., 2021). Despite enabling confident predictions of future system states based on established research tools (Staver et al., 2011), the applications often address selected sub-systems (Accatino et al., 2010; Kininmonth et al., 2022) only partially representing the diversity and complexity of the environmental-human interaction landscapes that theoretical models can hardly validate with accuracy given the limited availability of data.
Innovation from connected systems
From simple to heterotypic networks
One promising approach was recently proposed to assess a community structure (‘The Global Ocean Plankton Interactome’), where 20,810 nodes represent operational taxonomic units (OTU) and 86,026 edges represent potential biotic interactions, with organismal interactions driven by environmental drivers (Chaffron et al., 2021). It is an example of use of Tara Oceans data focused on plankton diversity to elucidate interconnected network dynamics, and with the goal of modeling the complex community structure of ecological associations (Fuhrman, 2009; Faust and Raes, 2012; Banerjee et al., 2018) and assessing the vulnerability to environmental change. A critical factor common to most interactome analyses is the consideration of structure versus dynamics, and the properties inferred from the global scale sampling of plankton summarize well the community structure, leaving open the question of what between taxonomy and function is the real model predictions driver.
A complex biological system may display spatiotemporal interaction dynamics of partially or totally unknown nature. Nevertheless, there are identifiable conditions from which emergent and self-organizing behavior can be observed, which allow to infer how complex biosystems evolve over time by changing internal structure and dynamics in response to both their endogenous governing laws and various exogenous (environmentally caused) adaptations. In general, the study of network dynamics and topology cannot be kept separate: the relationships between bio-entities subject to nonlinear/stochastic influences may justify the observed structural associations, and in turn provide inference hypotheses to be tested at systems level (Turnbull et al., 2018).
In marine ecosystems two main challenges are: a) Tackling large-sized problems with incomplete information, and b) Obtaining efficient solutions. More specifically, network properties such as stability, robustness, fragility, controllability, synchronization that are relevant to the study of dynamics, may be involving unknown nonlinear functions that can only be approximated but not analytically solved. Marine ecosystems can be naturally represented by heterotypic networks with a mix of structured and unstructured information, which potentially calls for new deep learning (DL) solutions with none or minimal need of supervision.
How to deal with dynamics?
Bio-ecosystems can be investigated with respect to the reconstruction of the phase space of the generating dynamical process. Here, the dynamics assumed to perform a trajectory in a state-space measure at each time point all the measurable quantities and concatenate them over time. By considering a trajectory of observed states, the steady dynamics that may occur are the result of reconciling the transient components observed under non-equilibrium conditions. The complexity due to indeterminacy, inaccuracy of measurements, uncertainty, etc., which are also typical of marine ecosystems, calls for new integrative inference approaches. Networks can allow causative associations to be investigated through regulatory dynamics as a main source of inference. As they may be limited in spatiotemporal resolution and use averages over conditions or time points, their inherent potential to discover causal relationships may be reduced. Still, networks allow the monitoring of information transmission mechanisms underlying observed phenomena (i.e., phenotypic alterations and signaling processes in biosystems, or altered functionality of human-environment interaction in marine ecosystems).
Moving forward: bottlenecks and directions to explore
A dynamic network approach leverages the study of coordinated functional activation depending on conditions. Differential network biology (Ideker and Krogan, 2012) and analysis (Xie et al., 2020) have been pervasively explored (in brain (Basha et al., 2020), in cancer (Gill et al., 2014), etc.) to compare topologies and modular structures in health versus disease states or between two different disease states, supported by expression, genetic, and clinical information. Here, the expected variation may reflect the distinctiveness in the molecular signatures of gene expression. The focus is on aggregate rather than individual target dynamics and on monitoring transient and permanent changes at various scales by pinpointing significant associations or dissociations (break down) of modular structures. Intuitively, in marine ecosystems one would like to assess first the degree of participation of the identified constituent modules with a significant role in network dynamics, and then how the differential conditions alter topologically the network depending on the participation of key individual entities to the significant modules studied under various conditions.
A few criticalities emerge from a number of factors:
-Quantifying the system’s robustness. Robust solutions beyond single nodal scale require that a minimal number of dominant, influential or controllable nodes be found (Sharma et al., 2017).
- Identifying vulnerabilities of network measures. Entropy is not a measure independent of a particular network representation and cannot capture the properties of the underlying generative process. The popular Shannon entropy is only partially useful in dealing with complexity, information content, causation and temporal information. Other entropy-based measures require a focus on particular properties (adjacency matrix, degree sequence, etc.).
- Dealing with loss of information. To understand interactions, the observed dynamics must be aligned with the context, the type of data and the level of uncertainty in the system (that is, conditions and stressors).
Some of the relevant properties emerging from networks, are summarized below (Bertolero et al., 2017):
- High connectivity in the network has effects on structure. The high-degree central nodes tend to coordinate the global network communication. An example is provided by the brain regions in which such nodes tend to be pathological. If removed, the global efficiency of the network decreases. Therefore, the rich club is a stable core of brain regions coordinating the transmission of information.
- High-participation nodes have effects on functions. These nodes exhibit diverse connectivity degree and are more consistent with the development of integration functions. For instance, in the brain these nodes belong to different communities, and some are physically proximal. Also, they control functionally connected regions (those more predictive of changes). Finally, their activity increases in complex tasks requiring specialization and more communities involved needing integration. Therefore, removing these nodes would likely damage the overall functionality as they govern modularly the local processing of information.
- Controllability. Highly non-linear systems typically present conditions of non-equilibrium and manifest the effects of critical transitions between unstable and stable points. A system is controllable when it can be driven from any initial state to any final state within finite time. The typical control strategy involves efficient guidance of a system’s behavior towards a desired state through only a few input variables. Finding these few points is challenging and refers to a key question: what is necessary to control in a network? In general, knowledge from two domains is needed: system’s architecture, i.e., network structure/configuration, and dynamical rules that are observed through time-dependent interactions. Controllability is computationally prohibitive even for moderately sized networks due to highly complex functions. Therefore, since many nodes may be regulators of dynamics in biomolecular networks, the full network state space can be the real target of complete controllability at the price of computationally efficiency.
- Transittability. This useful property (Wu et al., 2014) in biosystems allows to steer the regulatory network, which thus transits from abnormal to healthy phenotype (phenotype transition). Usually, the cellular phenotypes can be studied by the network states collectively, i.e., considering all molecular expressions. Also, phenotypic changes work as dynamic transitions between states of the network cellular reprogramming. A steering node is where an input control signal is directly acted and is a minimal set of steering nodes network transiting from one state to another (much less than minimum set of driver nodes). Therefore, transittability is less requiring than complete controllability for cellular phenotype transitions.
Discussion
Recently, an experts’ consensus (Danovaro et al., 2020) about deep-sea essential ecological variables has classified them into five priority groups aimed at a more effective global scale management: biodiversity, ecosystem functions; impacts and risk assessment; climate change, adaptation and evolution; and ecosystem conservation. These are all aspects that would benefit from new research directions in marine environmental studies. We have recalled lessons learned in other similarly complex systems, motivated by a largely unexplored potential in the cross-disciplinary evaluation of GES and the impacts on human health (see a summary in Figure 2).
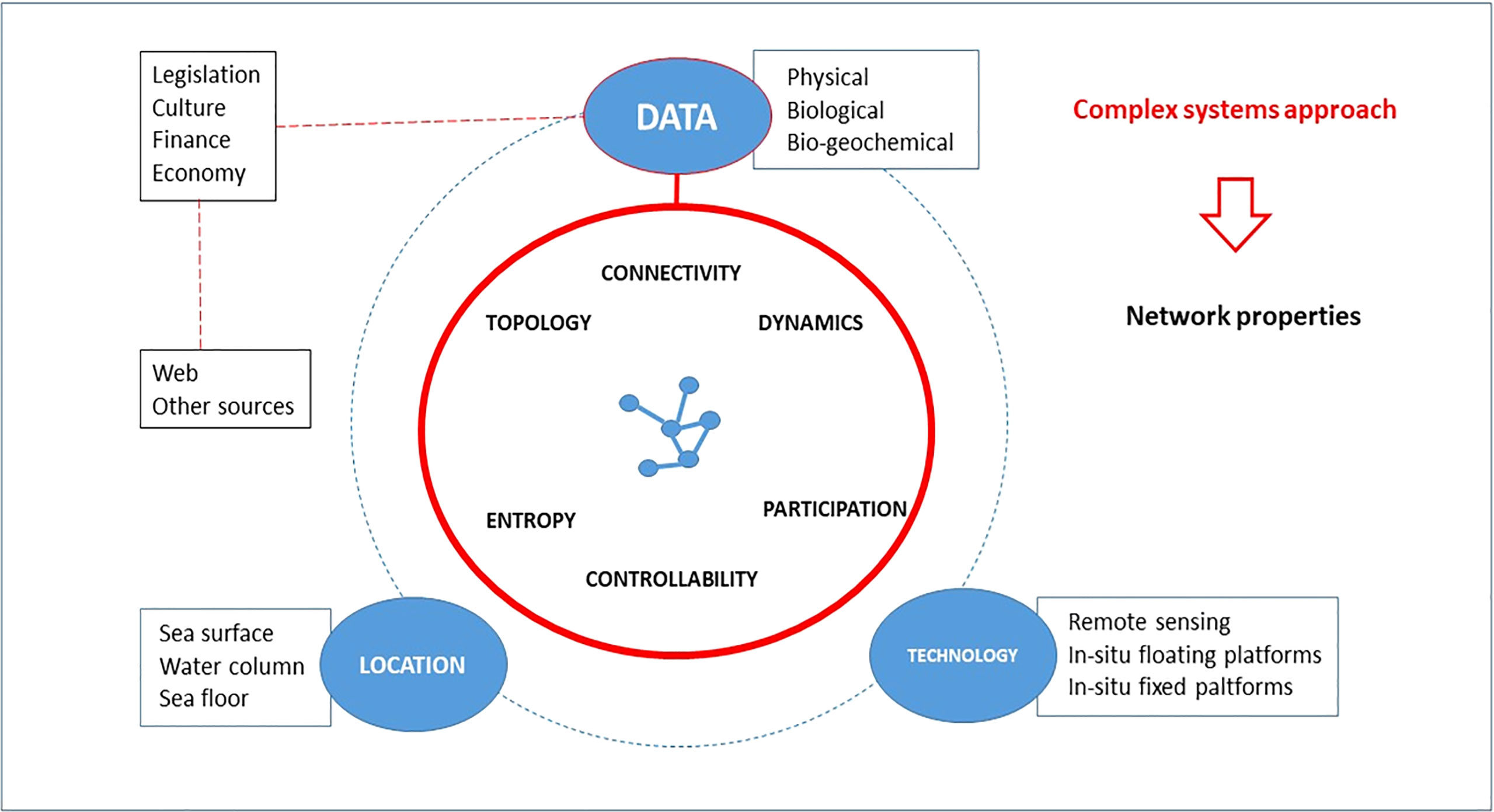
Figure 2 A proposal for a learning process approach to the marine ecosystem, mainly focused on the properties of the coupled natural and anthropogenic system constituted by the network of agents.
In general, for any possible knowledge transfer to exert measurable impacts on marine management policies, the first step that is required is an assessment of the general marine status. Consequently, data collection and method development offer the concrete tools to implement this first step.
Table 1 presents a group of topics for which methods have been proposed and a group of topics for which further method development is needed to better face the complexity of ecosystems. We stressed the role of complex biosystems considering that the influence of biological mechanisms is devoted to maximize the diversity of the interaction landscape. There are other properties of an exploratory nature but still highly desirable. An example is susceptibility (Manik et al., 2017), which quantifies the change of the systems’ state in response to a change in an external field. This property induces collective dynamics, depending on the topological configuration of connected systems and the mapping of perturbation dynamics (local properties). However, it is unclear how to define susceptibilities in systems and their relations with collective dynamics.
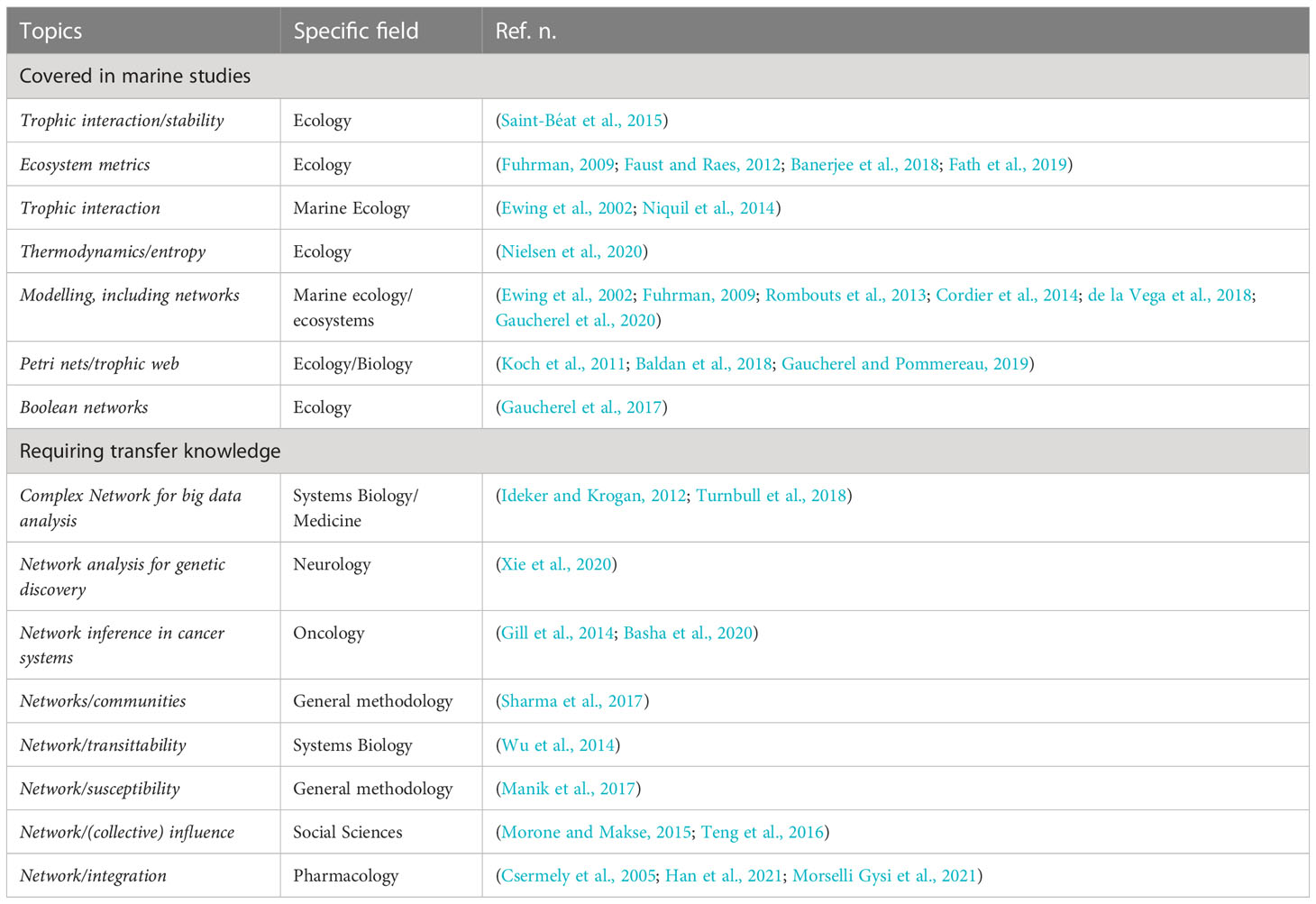
Table 1 a non-comprehensive list of topics and corresponding fields of study (with references) to address the complexity of ecosystems.
A first helpful distinction is between influence and flow. Network components are ranked by their dynamic impact, e.g., seeking the most influential nodes (capturing the magnitude of the systems’ response to perturbations). In genomics, a perturbed gene is a key source of information, but other genes propagate the signal and support the flow as mediators (Morone and Makse, 2015). Therefore, finding optimal/minimal sets of influencer nodes is key and weakly connected nodes tend to emerge as optimal influencers. Collective Influence (CI) methods performing influence maximization are therefore needed (Teng et al., 2016).
Conclusions
A useful description of the complexity of the marine environment is the first step toward a better understanding of the impacts of anthropogenic pressures relatively to the overall system’s state. In order to address the relationship between ocean and human health, a better knowledge of the functioning of the ecosystems is needed. Essentially, their complexity cannot be linearly decomposed and core information must be extracted to make any policy advice relevant and cost-effective.
However, the diversity of environmental contexts often does not allow to adopt a common and comprehensive model. We need therefore to identify an analytical framework capable to describe the dynamics of a network of inhomogeneous agents, preferably not limiting the ecosystem to the physical or biological variables of the marine environment (Miloslavich et al., 2018; Affatati and Moretti, 2021). Examples come from sectors such as soil (Masciandaro et al., 2013) or freshwater (Van Rossum et al., 2018) indicate the possibility of simplifying the evaluation of the state of the system by reducing the number/volume of data to be acquired, thus making more feasible the system’s state monitoring and management.
The challenges of understanding the status of the marine environment and managing the impacts of human activities depend largely on expensive “learning by doing” frameworks in which different models and data analysis are mostly adopted according to linear approaches and focused on environmental aspects. Very recently, a European initiative has been launched to revise this traditional framework based on the definition and evaluation of the good environmental status of seas and oceans. A joint action between many countries has promoted a path complementary to the one developed within the MFSD in order to overcome the limits of the adoption of indicators treated independently when addressing complex systems and motivate innovative observing strategies and models to describe the state of the marine environment (Sprovieri et al., 2021a).
We consider knowledge transfer a crucial factor for a better inclusion and understanding of network science-driven processes already well consolidated in other domains and relevant for the marine ecosystem too. In turn, this calls for a major integration of disciplines within ecology, in particular marine science. The many success examples provided in bio- and health-systems studies represent a proof of the effectiveness of cooperative approaches, which may help evaluate the status of complex coupled bio-ecosystems. By studying their network properties and their multilevel organization there will be more chances of identifying testable drivers of marine environment dysfunctionality and ultimately enable interventions.
Despite being aware of the complexity of the coupled human-environment system, in the marine community more than two thirds of the programmes and analysis do not involve an integrated approach yet. We therefore stressed the need of developing next generation methodological approaches bridging the traditional linear thinking in describing abundances/diversity/coverage/distribution of selected variables with the complex systems strategies based on interconnected networks where to investigate properties such as transittability, connectivity, controllability, participation etc., all relevant to ecosystems.
In conclusion, a shift from traditional solutions is needed to face the challenges of monitoring data-driven complex ecosystem dynamics and establishing their public health impact. The new solutions would in general address a collective action toward more collaborative environmental governance (Bodin, 2017; Dalton et al., 2020). As a start, a concrete solution in the marine environment is to assign a more central role to automated algorithmic solutions for integrating data-driven activities (including collection, processing, identification, classification, and prediction) and decisions nowadays widely provided and/or supported by network approaches.
Data availability statement
The original contributions presented in the study are included in the article/supplementary material. Further inquiries can be directed to the corresponding authors.
Author contributions
PM conceived the study. EC described the methods. PM and EC wrote the manuscript. All authors contributed to the article and approved the submitted version.
Acknowledgments
The authors acknowledge CNR’s support. The authors want to thank two reviewers for their work and constructive suggestions.
Conflict of interest
The authors declare that the research was conducted in the absence of any commercial or financial relationships that could be construed as a potential conflict of interest.
Publisher’s note
All claims expressed in this article are solely those of the authors and do not necessarily represent those of their affiliated organizations, or those of the publisher, the editors and the reviewers. Any product that may be evaluated in this article, or claim that may be made by its manufacturer, is not guaranteed or endorsed by the publisher.
References
Accatino F., De Michele C., Vezzoli R., Donzelli D., Scholes R. J. (2010). Tree-grass co-existence in savanna: interactions of rain and fire. J. Theor. Biol. 267 (2), 235–242. doi: 10.1016/j.jtbi.2010.08.012
Affatati A., Moretti P. F. (2021). “Sounding the sound in the ocean: back-casting foresight to identify observing strategies to understand the ecosystem,” in Tuning communication for functioning: sounding in/the fluids. Eds. Moretti P. F., Andreta E., Casacchia R. (Rome IT: Proc. Foresight Workshop), 5–12. Available at: http://www.foresight.cnr.it/pubblications/issn.html.
Badii R., Politi A. (1999). Complexity: Hierarchical Structures and Scaling in Physics (Cambridge, UK: Cambr Univ Press).
Baldan P., Bocci M., Brigolin D., Cocco N., Simeoni M. (2018). Petri netckis for modelling and analysing trophic networks. Fundamenta. Informat. 160, 27–52. doi: 10.3233/FI-2012-0000
Banerjee S., Schlaeppi K., van der Heijden M. G. A. (2018). Keystone taxa as drivers of microbiome structure and functioning. Nat. Rev. Microbiol. 16, 567–576. doi: 10.1038/s41579-018-0024-1
Basha O., Argov C. M., Artzy R., Zoabi Y., Hekselman I., Alfandari L., et al. (2020). Differential network analysis of multiple human tissue interactomes highlights tissue-selective processes and genetic disorder genes. Bioinformatics 36 (9), 2821–2828. doi: 10.1093/bioinformatics/btaa034
Bauer P., Thorpe A., Brunet G. (2015). The quiet revolution of numerical weather prediction. Nature 525, 47–55.394. doi: 10.1038/nature14956.395
Bertolero M. A., Yeo B. T. T., D’Esposito M. (2017). The diverse club. Nat. Commun. 8, 1277. doi: 10.1038/s41467-017-01189-w
Biggs R., de Vos A., Preiser R., Clements H., Maciejewski K., Schlüter M., et al (Ed.) (2021). The Routledge Handbook of Research Methods for Social-Ecological Systems. 1st Edition (London UK: Taylor & Francis Group), 526. doi: 10.4324/9781003021339
Bodin Ö. (2017). Collaborative environmental governance: Achieving collective action in social-ecological systems. Science 357,6352, eaan1114. doi: 10.1126/science.aan1114
Borja A., Mathew P. W., Berdalet E., Bock N., Eatock C., Kristensen P., et al. (2020). Moving toward an agenda on ocean health and human health in Europe. Front. Mar. Sci. 7. doi: 10.3389/fmars.2020.00037
Chaffron S., Delage. E., Budinich M., Vintache D., Henry N., Nef C., et al. (2021). Environmental vulnerability of the global ocean epipelagic plankton community interactome. Sci. Adv. 7 (35), eabg1921. doi: 10.1126/sciadv.abg1921
Cordier M. O., Largouët C., Zhao Y. (2014). Model-checking an ecosystem model fo decision-aid. In: IEEE 26th international conference on tools with artificial intelligence. IEEE Comp. Soc, 539–543. doi: 10.1109/ICTAI.2014.87
Corso G., Torres Cruz C. P., Plaza Pinto M., Monteiro de Almeida A., Lewinsohn T. M. (2015). Binary versus weighted interaction networks. Ecol. Complexity. 23, 68–72. doi: 10.1016/j.ecocom.2015.04.003. ISSN 1476-945X.
Csermely P., Agoston V., Pongor S. (2005). The efficiency of multi-target drugs: the network approach might help drug design. Trends Pharmacol. Sci. 26 (4), 178–182. doi: 10.1016/j.tips.2005.02.007
Dalton K., Skrobe M., Bell H., Kantner B., Berndtson D., Gerhardinger L. C., et al. (2020). Marine-related learning networks: shifting the paradigm toward collaborative ocean governance. Front. Mar. Sci. 7. doi: 10.3389/fmars.2020.595054
Danovaro R., Fanelli E., Aguzzi J., Billett D., Carugati L., Corinaldesi C., et al. (2020). Ecological variables for developing a global deep-ocean monitoring and conservation strategy. Nat. Ecol. Evol. 4, 181–192. doi: 10.1038/s41559-019-1091-z
de la Vega C., Schückel U., Horn S., Kröncke I., Asmus R., Asmus H. (2018). How to include ecological network analysis results in management? A case study of th.ree tidal basins of the Wadden Sea, south-eastern North Sea. Ocean. Coast. Manag. 163, 401–416. doi: 10.1016/j.ocecoaman.2018.07.019
Eborja A., Elliott M., Andersen J. H., Eberg T. E. D., Carstensen J., Halpern B. S., et al. (2016). Overview of integrative assessment 387 of marine systems: the ecosystem approach in practice. Front. Mar. Sci. 3. doi: 10.3389/fmars.2016.00020
Edgar G. J., Bates A. E., Bird T. J., Jones A. H., Kininmonth S., Stuart-Smith R. D., et al. (2016). New approaches to marine conservation through the scaling up of ecological data. Ann. Rev. Mar. Sci. 81, 435–461. doi: 10.1146/annurev-marine-122414-033921
Ehler C., Douvere F. (2009). Marine spatial planning: A step-by-step approach toward ecosystem-based management. 385. Paris.: UNESCO. IOC. Manual. Guides., 99. doi: 10.25607/OBP-43
Ewing B., Yandell B., Barbieri J., Luck R., Forster L. (2002). Event-driven competing risks. Ecol. Model. 158, 35–50. doi: 10.1016/S0304-3800(02)00218-1
Fath B. D., Asmus H., Asmus R., Baird D., Borrett S., Jonge V. N., et al. (2019). Ecological network analysis metrics: The need for an entire ecosystem approach in management and policy. Ocean. Coast. Manage. 174, 1–14. doi: 10.1016/j.ocecoaman.2019.03.007
Faust K., Raes J. (2012). Microbial interactions: from networks to models. Nat. Rev. Microbiol. 10, 538–550. doi: 10.1038/nrmicro2832
Fleming L. E., Maycock B., White M. P., Depledge M. H. (2019). Fostering human health through ocean sustainability in the 21st century. People Nat. 1 (3), 276–283. doi: 10.1002/pan3.10038
Franke A., Blenckner T., Duarte C. M., Ott K., Fleming L. E., Antia A., et al. (2020). Operationalizing ocean health: towards integrated ocean health and recovery research to achieve ocean sustainability. One Earth 2 (6), 557–565. doi: 10.1016/j.oneear.2020.05.013
Fuhrman J. A. (2009). Microbial community structure and its functional implications. Nature 459, 193–199. doi: 10.1038/nature08058
Gaucherel C., Pommereau F. (2019). Using discrete systems to exhaustively characterize the dynamics of an integrated ecosystem. Meth. Ecol. Evol. 10 (9), 1–13. doi: 10.1111/2041-210X.13242
Gaucherel C., Pommereau F., Hely C. (2020). Understanding Ecosystem Complexity via Application of a Process-Based State Space rather than a Potential Surface. Complexity, 7163920. doi: 10.1155/2020/7163920
Gaucherel C., Thero H., Puiseux A., Bonhomme V. (2017). Understand ecosystem regime shifts by modelling ecosystem development using boolean networks. Ecol. Complexity. 31, 104–114. doi: 10.1016/j.ecocom.2017.06.001
Gill R., Datta S., Datta S. (2014). Differential network analysis in human cancer research. Curr. Pharm. Des. 20 (1), 4–10. doi: 10.2174/138161282001140113122316
Han Y., Wang C., Klinger K., Rajpal D. K., Zhu C. (2021). An integrative network-based approach for drug target indication expansion. PLoS One 16 (7), e0253614. doi: 10.1371/journal.pone.0253614
Hayes K. M., Dambacher J. M., Hosack G., Bax N., Dunstan P., Fulton E., et al. (2015). Identifying indicators and essential 400 variables for marine ecosystems. Ecol. Indicat. 57, 409–419. doi: 10.1016/j.ecolind.2015.05.006
Hyvärinen H., Skyttä A., Jernberg S., Meissner K., Kuosa H., Uusitalo L. (2021). Cost-efficiency assessments of marine 389 monitoring methods lack rigor—a systematic mapping of literature and an end-user view on optimal cost-efficiency 390 analysis. Environ. Monit. Assess. 193, 400. doi: 10.1007/s10661-021-09159-y
Ideker T., Krogan N. J. (2012). Differential network biology. Mol. Syst. Biol. 8, 565. doi: 10.1038/msb.2011.99
Inniss L., Simcock A. (Eds.) (2016). The First Global Integrated Marine Assessment World Ocean Assessment (New York: United Nations).
Kininmonth S., Blenckner T., NiIranen S., Watson J., Orio A., Casini M., et al. (2022). Is diversity the missing link in coastal fisheries management? Diversity 14 (2), 90. doi: 10.3390/d14020090
Koch I., Reisig W., Schreiber F. (2011). Modeling in systems biology - The Petri net approach (London: Springer-Verlag).
Landi P., Minoarivelo H. O., Brännström Å, Hui C., Dieckmann U. (2018). Complexity and stability of ecological networks: a review of the theory. Popul. Ecol. 60, 319–345. doi: 10.1007/s10144-018-0628-3
Landrigan P. J., Stegeman J. J., Fleming L. E., Allemand D., Anderson D. M., Backer L. C., et al. (2020). Human health and ocean pollution. Ann. Glob. Health 86 (1), 151. doi: 10.5334/aogh.2831
Lindstrom E. (2018). On the relationship between the Global Ocean Observing System and the Ocean Observatories 392 Initiative. Oceanography 31 (1), 38–41. doi: 10.5670/oceanog.2018.107.393
Liu J., Dietz T., Carpenter S. R., Taylor W. W., Alberti M., Deadman P., et al. (2021). Coupled human and natural systems: The evolution and applications of an integrated framework. Ambio 50, 1778–1783. doi: 10.1007/s13280-020-01488-5
Lubchenco J., Petes L. E. (2010). The interconnected biosphere: Science at the ocean’s tipping. Oceanography 23 (2), 115–129. doi: 10.5670/oceanog.2010.55
Manik D., Rohden M., Ronellenfitsch H., Zhang X., Hallerberg S., Witthaut D., et al. (2017). Network susceptibilities: Theory and applications. Phys. Rev. E. 95 (1), 12319. doi: 10.1103/PhysRevE.95.012319
Masciandaro G., Macci C., Peruzzi E., Ceccanti B., Doni S. (2013). Organic matter–microorganism–plant in soil bioremediation: a synergic approach. Rev. Environ. Sci. Biotechnol. 12, 399–419. doi: 10.1007/s11157-013-9313-3
McMichael A. J., WHO (2003). Climate change and human health. Risks. And. Responses. 322. Available at: https://apps.who.int/iris/handle/10665/42742.
Miloslavich P., Bax N. J., Simmons S. E., Klein E. (2018). Appeltans W. Aburto-Oropeza O. et al. Essential ocean variables for global sustained observations of biodiversity and ecosystem 405 changes. Glob. Ch. Biol. 24, 2416–2433. doi: 10.1111/gcb.14108
Morone F., Makse H. (2015). Influence maximization in complex networks through optimal percolation. Nature 524, 65–68. doi: 10.1038/nature14604
Morselli Gysi D., do Valle I., Zitnik M., Ameli A., Gan X., Varol O., et al. (2021). Network medicine framework for identifying drug-repurposing opportunities for COVID-19. PNAS 118 (19), e2025581118. doi: 10.1073/pnas.2025581118
Nielsen S. N., Muller F., Marques J. C., Bastianoni S., Jorgensen S. E. (2020). Thermodynamics in ecology – An introductionary review. Entropy 22, 820. doi: 10.3390/e22080820
Niquil N., Baeta A., Marques J., Chaalali A., Lobry J., Patrício J. (2014). Reaction of an estuarine 850 food web to disturbance: Lindeman’s perspective. Mar. Ecol. Progr. Ser. 512 (851), 141–154. doi: 10.3354/meps10885
O’Brien T., Lorenzoni L., Isensee K., Valdés L. (2017). What Are Marine Ecological Time Series Telling Us about the Ocean? 396 A Status Report Vol. 297 (Paris: IOC Techn. Ser), 397.
Rombouts I., Beaugrand G., Artigas L. F., Dauvin J. C., Gevaert F., Goberville E., et al. (2013). Evaluating marine ecosystem health: Case studies of indicators using direct observations and modelling methods. Ecol. Indic. 24, 353–365. doi: 10.1016/j.ecolind.2012.07.001
Saint-Béat B., Baird D., Asmus H., Asmus R., Bacher C., Pacella S. R., et al. (2015). Trophic networks: How do theories link ecosystem structure and functioning to stability properties? A review. Ecol. Indic. 52, 458–471. doi: 10.1016/j.ecolind.2014.12.017
Sharma A., Cinti C., Capobianco E. (2017). Multitype network-guided target controllability in phenotypically characterized osteosarcoma: role of tumor microenvironment. Front. Immunol. 8, 918. doi: 10.3389/fimmu.2017.00918
Short R. E., Cox D. T. C., Tan Y. L., Bethel A., Eales J. F., Garside R. (2021). Review of the evidence for oceans and human health relationships in Europe: A systematic map. Environ. Intern. 146, 106275j. doi: 10.1016/j.envint.2020.106275
Sole R. V., Bascompte J. (2006). Self-Organization in Complex Ecosystems (Princeton: University Press).
Sprovieri M., Ribera d'Alcalà M., Roose P., Drago A., De Cauwer K., Falcini F., et al. (2021a). Science for good environmental status: A European joint action to support marine policy. Sustainability 13 (15), 8664. doi: 10.3390/su13158664
Sprovieri M., Ribera d’Alcalà M., Roose P., Drago A., De Cauwer K., Falcini F. (2021b). Science for good environmental status: A European joint action to support 381 marine policy. Sustainability 13, 8664. doi: 10.3390/su13158664.382
Staver A. C., Archibald S., Levin S. (2011). Tree cover in sub-Saharan Africa: rainfall and fire constrain forest and savanna as alternative stable states. Ecology 92 (5), 1063–1072. doi: 10.1890/10-1684.1
Strain E. M. A., Thomson R. J., Micheli F., Mancuso F., Airoldi L. (2014). Identifying the interacting roles of stressors in driving 402 the global loss of canopy-forming to mat-forming algae in marine ecosystems. Glob. Ch. Biol. 1 20 (11), 3300–3312.403. doi: 10.1111/gcb.12619
Sun M. Y., Dafforn K. A., Brown M. V., Johnston E. L. (2012). Bacterial communities are sensitive indicators of contaminant 398 stress. Mar. pollut. Bull. 64 (5), 1029–1038. doi: 10.1016/j.marpolbul.2012.01.035.399
Teng X., Pei S., Morone F., Makse H. E. (2016). Collective influence of multiple spreaders evaluated by tracing real information flow in large-scale social networks. Sci. Rep. 6, 36043. doi: 10.1038/srep36043
Turnbull L., Hütt M. T., Ioannides A. A., Kininmonth S., Poeppl R., Tockner K., et al. (2018). Connectivity and complex systems: learning from a multi-disciplinary perspective. Appl. Netw. Sci. 3, 11. doi: 10.1007/s41109-018-0067-2
Van Rossum T., Uyaguari-Diaz M. I., Vlok M., Peabody M. A., Tian A., Cronin K. I., et al. (2018). Spatiotemporal dynamics of river viruses, bacteria and microeukaryotes. bioRxiv. doi: 10.1101/259861
Wu F. X., Wu L., Wang J., Liu J., Chen L. (2014). Transittability of complex networks and its applications to regulatory biomolecular networks. Sci. Rep. 4, 4819. doi: 10.1038/srep04819
Xie J., Yang F., Wang J., Karikomi M., Yin Y., Sun J., et al. (2020). DNF: A differential network flow method to identify rewiring drivers for gene regulatory networks. Neurocomputing 410, 202–210. doi: 10.1016/j.neucom.2020.05.028
Keywords: marine ecosystem, sustainability, complex networks, knowledge transfer, human health
Citation: Capobianco E and Moretti PF (2023) Complexity of the marine ecosystem in view of the human health factors: role of network science. Front. Mar. Sci. 10:1076258. doi: 10.3389/fmars.2023.1076258
Received: 12 December 2022; Accepted: 18 July 2023;
Published: 30 August 2023.
Edited by:
Panagiota Katikou, Greek Ministry of Rural Development and Food, GreeceReviewed by:
Stuart James Kininmonth, The University of Queensland, AustraliaZdenka Willis, Veraison Consulting LLC, Virginia, United States
Copyright © 2023 Capobianco and Moretti. This is an open-access article distributed under the terms of the Creative Commons Attribution License (CC BY). The use, distribution or reproduction in other forums is permitted, provided the original author(s) and the copyright owner(s) are credited and that the original publication in this journal is cited, in accordance with accepted academic practice. No use, distribution or reproduction is permitted which does not comply with these terms.
*Correspondence: Pier Francesco Moretti, pierfrancesco.moretti@cnr.it