- 1Institut de Ciències del Mar, Spanish National Research Council (CSIC), Barcelona, Spain
- 2Seafood Technologies, The New Zealand Institute for Plant and Food Research, Nelson, New Zealand
The distribution of age classes is a key demographic parameter of populations and thus proper age estimation is crucial for fisheries management and for conservation biology. Age estimation in fishes has traditionally relied on the analysis of growth marks in hard structures such as otoliths. However, besides being lethal this method is time-consuming, can have low accuracy in some species and cannot be applied in others. Thus, there is a need for the development of new methods. DNA methylation is an epigenetic modification consisting in the addition of a methyl group in cytosine-guanine loci. Aging is associated with changes in DNA methylation. Among a background of global and weak genome hypomethylation, there are some loci in which age-associated DNA methylation changes are of a “clock-like” nature and thus predictable. Chronological age estimators built from DNA methylation are termed ‘epigenetic clocks’. Epigenetic clocks have been developed in the last ten years for many species, notably vertebrates, including already several fish species. Here, we review the piscine epigenetic clocks built so far and outline the major considerations to be taken into account for the development of new epigenetic clocks for additional species, which include the number of samples to be collected and tissues to be targeted. The steps on how to develop such a clock and the techniques available to do so are also discussed. Next, we focus on the features of epigenetic clocks as measuring devices, considering aspects such as accuracy, precision and reproducibility. Finally, we discuss the possibility of developing a multi-species piscine epigenetic clock and how processing automation can greatly reduce the cost per sample. One important knowledge gap is to determine how environmental changes, especially temperature and food availability, may affect the tick rate of piscine epigenetic clocks. Improved age prediction through the use of piscine epigenetic clocks can contribute to better fisheries management practices in a context of overexploited fish stocks worldwide, and in the estimation of age classes in endangered species.
1. Introduction
1.1. Rationale for age estimation in fish
Age is one of the most influential of biological variables. Age-class distribution is considered a key population parameter, with important influences on biomass distribution, intra-specific interactions and reproductive potential, among others (Campana, 2001). Effective and accurate age estimation in fishes is crucial to know the demographic structure of populations, which needs to be assessed in a representative, informative and robust way. This assessment is of paramount importance in species of commercial interest for fisheries management (Pardo et al., 2013). Also, it is valuable for the estimation of age classes in populations of endangered species in order to design proper conservation strategies (Heydenrych et al., 2021). More specifically, and according to New Zealand’s National Institute of Water and Atmospheric Research (NIWA), age estimation for the monitoring and management of fishery resources enables the following (Sutton et al., 2023):
1) Calculation of growth rates. By ageing many fish over the whole size-range in a population, it is possible to generate a growth curve (i.e., average length at each age) for that population. This method allows to assess how productive species are and to estimate the variability in their growth rates over time.
2) Calculation of mortality rates. By ageing a random sample of individuals from a population, and evaluating how the frequency of fish in each year class (all fish born in the same year) diminishes with age, it is possible to estimate: a) the rate of natural mortality given the sample size is very large, or, b) in a heavily fished population, the combined rate of mortality due to natural causes and fishing.
3) Assessment of population age-class structures. The aged random sample described above also estimates the distribution of age classes in a population if the sample is from a particular fishery, allowing to infer what group of age classes are particularly targeted by fisheries.
4) Estimation of annual spawning success. After accounting for mortality over time, an aged random sample can show the relative strengths of individual year classes and illustrate how successful spawning was in each year. The more estimates that can be derived for the strength of a particular year class (e.g., from consistent sampling in consecutive years), the greater the confidence in the estimate for that year class. These estimates of year class strength can explain variations in total stock abundance and are used to predict future recruitment to the fishery.
5) Investigations of stock structure. In the same geographic region, there may be more than one stock of a given species, for example, if distinct populations of the same species with little or no genetic or physical mixing are present. Differences in growth rates or population age structures between areas can be suggestive of multiple stocks (Sutton et al., 2023).
To illustrate the importance of age estimation in fish, it was estimated that about two million fish were aged worldwide in 1999 (Campana and Thorrold, 2001). Despite some efforts we have not been able to obtain a more current figure. However, Campana (2001) considered that fish aging efforts dwarf those routinely applied to non-fish species, and highlighted the importance attributed to age-structured information in fisheries science.
1.2. Shortcomings of current methods for age estimation and limitations
Age estimation in fishes has traditionally relied on the analysis of growth marks in hard structures such as vertebra, scales and otoliths (fish ear stones; Figure 1), in an analogous manner that rings are used to estimate the age of trees (Campana, 2001). Traditional methods require well-trained personnel, are time-consuming, often lethal depending upon choice of material (vertebra and otoliths), and can have low accuracy or are impossible to apply in some species. Otolith-based methods can nowadays be aided by deep neural network procedures of machine learning. However, despite recent technical improvements, traditional methods still have many shortcomings, including the fact that knowledge about the internal structure of the otolith is needed to improve the results for the youngest age groups, and that the contour shape and size attributes are sometimes not good enough for the younger age groups (Ordoñez et al., 2020).
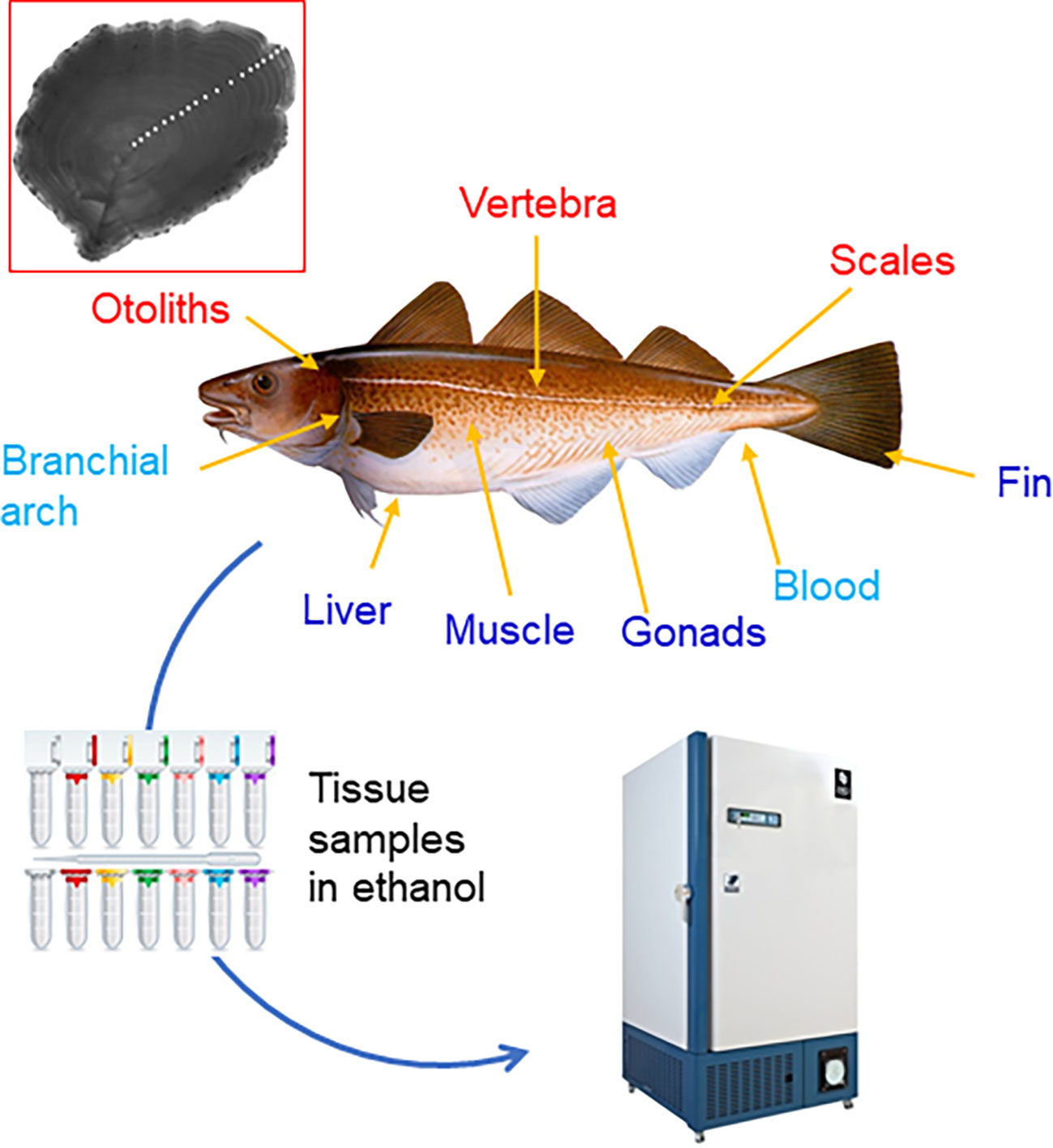
Figure 1 Hard structures traditionally used to estimate age in fish (names above the fish figure and in red), particularly otoliths (insert); and tissues either already used (names below the fish figure in dark blue) or with potential use (light blue) to develop epigenetic clocks. Fin clips are the preferred choice because they are easy to access and collect, and represent a non-invasive and non-lethal technique. Insert: the otolith image corresponds to yellowfin flounder, aged 19 years. The annual increments are marked by white dots. Source: Wikimedia Commons.
Error in fish age estimation has two major sources: 1) Error associated with what is being measured, i.e., the structure being examined. Thus, some of the bony structures may not exhibit a complete growth sequence throughout lifetime, and this type of error can result in under- or over-age estimation. 2) Error due to sample preparation and interpretation of growth marks in calcified structures, which can vary markedly among readers and laboratories (Campana, 2001). This type of error can be biased or random. Together, both types of errors can result in estimates that can differ up to a factor of three among investigators (Campana, 2001).
There are some fish species of worldwide economic importance for which age estimation based on these traditional methods is very difficult or impossible. For example, the monkfish (Lophius americanus), supports an important commercial fishery in the Northeastern United States. Despite healthy stock status, annual catch limits are typically low. This is in part due to uncertainty in the stock assessment since age estimation in monkfish traditionally suffers from lack of effective validation due to irregular growth of the otolith. In consequence, this hampers its use as a reliable age structure assessment method (Bank, 2016). As a second option, annual growth rings are counted on the vertebrae and are assumed to follow a seasonal pattern but, again, they failed to accurately determine the age of monkfish (Bank, 2016). A similar situation occurs in hake, Merluccius merluccius (Morales-Nin et al., 1998). Thus, there is a need for the development of new, widely applicable methods.
Taking into account all the above and the rapid development of molecular methods to interrogate different aspects of the living organisms, exploring the possibility of applying some of these methods for age estimation in fish is warranted.
2. Epigenetic clocks
2.1. The definition and main mechanisms of epigenetics
Epigenetics can be defined as “the study of phenomena and mechanisms that cause chromosome-bound, heritable changes to gene expression that are not dependent on changes to DNA sequence.” (Deans and Maggert, 2015). The term “heritable” has two different implications because these changes can be passed from one cell to their daughter cells during mitotic cell division, but also during the formation of gametes through meiotic cell division, and thus can be transmitted from parents to offspring. The main epigenetic mechanisms for the regulation of gene expression are: DNA methylation, modification of histones and histone variants, and the presence of non-coding RNAs (ncRNAs) (Brock and Fisher, 2005). The epigenetic clocks built to date are based on DNA methylation analysis. Therefore, from now on this review will discuss only DNA methylation with more detail, while the other epigenetic mechanisms will not be further considered.
2.2. DNA methylation
DNA methylation is a chemical modification of the DNA chain itself, whereby the 5’ carbon of cytosine is modified by the addition of a methyl (CH3) group (“methylated”), becoming 5’-methylcytosine (5mC). In vertebrates, DNA methylation essentially occurs in a CpG context, i.e., when a cytosine is followed by a guanine.
The enzymes responsible for DNA methylation are called DNA methyltransferases (DNMTs) (Goll and Bestor, 2005). DNMTs are classified according to their target. DNMT3 methylates previously unmethylated CpGs, and is responsible for de novo DNA methylation. DNMT1 methylates the unmethylated opposing pair of a hemimethylated site (Goll and Bestor, 2005). DNMT1 is called maintenance DNMT, and is responsible for copying the existing methylation profile during cell division. Thus, DNMT1 participates in the transmission of epigenetic marks and contributes to the epigenetic inheritance mechanism (Hermann et al., 2004). Methylated DNA can be demethylated in either an active or passive manner. Active demethylation occurs mainly through the action of ten-eleven translocation (TET) deoxygenases, which can remove methyl groups independently of replication (Kohli and Zhang, 2013). Passive demethylation occurs when newly synthesized DNA strands are not methylated by DNMT1 either because DNMT1 activity is lost or because DNMT1 concentration is diluted during several replication rounds (Rasmussen and Helin, 2016). The same effect, i.e., inhibition of DNMT1 activity, can be achieved by the use of demethylating agents such as 5-azacytidine (Ribas et al., 2017). In fishes, because of several genome duplications, there can be several isoforms of each type of DNMT and TET enzymes (Chen et al., 2017) although with no new unique functions known so far as a result of these genome duplications. In vertebrate genomes, CpGs are usually methylated and evenly distributed, except in regions where there is an elevated content of CpGs (Illingworth and Bird, 2009; Moore et al., 2013). These regions are called CpG islands (CGIs), and they are normally associated with promoter or regulatory regions (Illingworth and Bird, 2009; Moore et al., 2013). Changes in methylation levels in these CGIs are associated with gene expression regulation (Illingworth and Bird, 2009; Moore et al., 2013). When comparing groups, a difference in the methylation level of a given CpG loci is referred to as differentially methylated cytosine (DMC), and many of them as DMCs. Likewise, genomic regions with different DNA methylation are called differentially methylated regions (DMRs).
2.3. Epigenetic changes with age
Aging is associated with changes in DNA methylation. Variation in DNA methylation is influenced by both intrinsic and extrinsic factors, plus stochastic events (Jung and Pfeifer, 2015). In general, there is an age-dependent change in DNA methylation that may be summarized in global genomic hypomethylation (Heyn et al., 2012), accompanied by hypermethylation of specific CpGs. However, aside from this “epigenetic drift”, there are changes that are of a “clock-like” nature and thus are predictable (De Paoli-Iseppi et al., 2019) (Figure 2A). Chronological age (referred to as cAge in some publications) predictors built with information on DNA methylation at several CpG loci are termed “epigenetic clocks” (Guevara and Lawler, 2018; Zhang et al., 2019). Epigenetic clocks integrate age-associated (hyper- and hypomethylation) changes from a group of carefully selected loci across the genome that provide what is termed estimated or predicted age and, in some cases, epigenetic age (eAge). High correlation between eAge against cAge underlies the use of epigenetic clocks and their ability of predicting cAge with high accuracy.
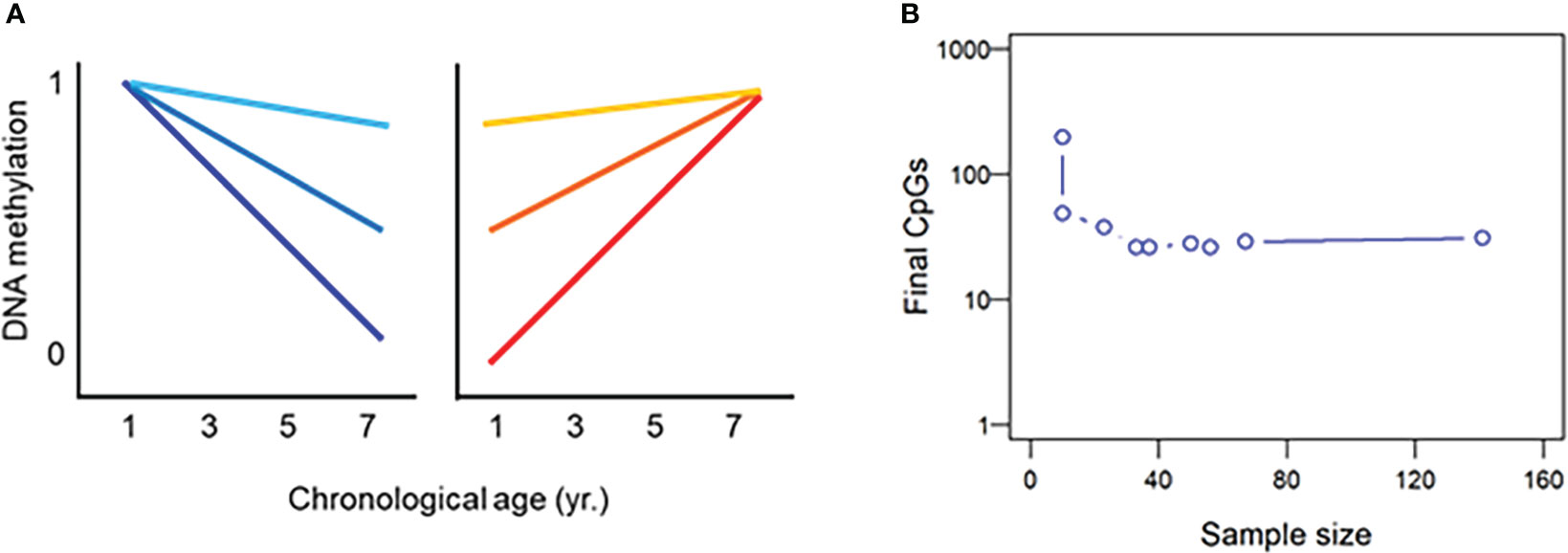
Figure 2 Principles behind the development of epigenetic clocks. (A) There are some CpG loci the methylation of which is of a clock nature becoming progressively hypo- (blue) or hypermethylated (red) in different degrees. (B) Relationship between sample size and number of informative loci in the piscine epigenetic clocks developed so far.
There are still many unknown aspects regarding what epigenetic clocks actually measure (Bell et al., 2019). It has been argued that they do actually measure aspects of age-related epigenetic drift but the fact that eAge can be measured in both proliferating and non-proliferating tissues suggest that passive demethylation unlikely underpins epigenetic clocks (Simpson and Chandra, 2021). Thus, the nature of eAge is still unknown but does not preclude the use of epigenetic clocks to estimate age in different taxa, mostly vertebrates. Therefore, in this review we will use the term “age estimation” rather than eAge. It has also been argued that epigenetic clocks can provide both chronological and biological age information (i.e., as measured now by variables such as creatinine, fasting glucose, telomere length, etc.) in different relative proportions, which depend on the CpG loci used to build the clock (Bell et al., 2019).
Although DNA methylation changes with age provides a strong foundation for the development of epigenetic clocks, consideration could perhaps be paid to other epigenetic mechanisms or modifications such as histone modifications and, specially, non-coding RNAs (ncRNAs), including micro-RNAs (miRNAs) (van Gelderen et al., 2022). In particular, quantifying levels of miRNAs is relatively easy (Lee and Shin, 2012). However, although fish miRNAs can change along developmental stages, e.g., during flatfish metamorphosis (Campos et al., 2014), to the best of our knowledge, variations of a clock type have not been described yet.
2.4. Vertebrate epigenetic clocks
A tissue-independent epigenetic clock was first developed in humans in a landmark study (Horvath, 2013) and was made of 353 CpGs. These CpGs can be divided into two sets according to their correlation with age. Thus, the 193 positively and 160 negatively correlated CpGs become hypermethylated and hypomethylated with age, respectively. Subsequently, additional epigenetic clocks have been developed for several vertebrates (De Paoli-Iseppi et al., 2019), mostly mammals, but also birds, a marine turtle (Mayne et al., 2022) and fishes (Table 1). More recently, epigenetic clocks have also been developed in an invertebrate, the European lobster (Fairfield et al., 2021), and in a tree, the loblolly pine (Gardner et al., 2022), suggesting that age-dependent DNA methylation changes may be a universal phenomenon in eukaryotes. Of note, recently, a multi-species epigenetic clock was built to age eight species of toothed whales and dolphins (odontocetes) (Robeck et al., 2021). Further still, a single universal clock was built based on 185 mammalian species that is capable of predicting accurately (average correlation > 0.91) the age across species and tissues, indicating that aging is an evolutionarily conserved developmental process (Lu et al., 2021).
Epigenetic clocks are characterized by very high accuracy when compared to more traditional methods of age estimation in mammals such as assessment of telomere length. Since epigenetics bridges genomic and environmental information many epigenetic changes are sensitive to environmental influences (O’Dea et al., 2016). Thus, building an epigenetic clock is based on finding CpGs the methylation of which is dependent only on age, not external influences.
2.5. DNA methylation changes with age in fishes
In accordance with the general pattern that seems to be conserved in vertebrates, and shortly after the publication of Horvath’s human clock in 2013, it was found that zebrafish (Danio rerio) exhibited a general hypomethylation of CpGs with age (Shimoda et al., 2014). Subsequently, changes in DNA methylation were identified and were found to be gene-, tissue- and age-dependent in Chinook salmon (Oncorhynchus tshawytscha) (Venney et al., 2016). These results indicated that epigenetic clocks could be developed in fishes.
2.6. Piscine epigenetic clocks
The first piscine epigenetic clock (and the first one in poikilothermic vertebrates) was developed in the European sea bass (Dicentrarchus labrax), a modern teleost distributed in the NE Atlantic, Mediterranean and Black Sea and important for both fisheries and aquaculture (Anastasiadi and Piferrer, 2020). Although it is possible to estimate age based on otolith readings in this species (Aguilera et al., 2009), we took advantage of fish of different ages in different research facilities. In this way, chronological age was known with absolute precision and thus, in principle, there was one less possible interfering source of error. We faced two difficulties compared to epigenetic clocks developed until that time. First, in contrast to birds and mammals, fish are poikilothermic vertebrates and thus we did not know how environmental factors such as temperature could affect the results. Second, in contrast to mammals, which exhibit determinate growth, many fish exhibit indeterminate growth, particularly long-lived species of temperate and cool regions (Dutta, 1994). We used epaxial muscle samples and a candidate gene approach, i.e., we started with a set of CpGs distributed in four genes analyzed in another experiment (Anastasiadi et al., 2018). A total of 48 CpGs were tested of which finally 28 CpGs were retained for clock construction. In a parallel test of clock building, 299 CpGs (including the 48 initial) in 12 samples of muscle were used for age prediction and across tissues for testing accuracy. Additional proof that the retained CpGs are reliable age predictors comes from the fact that overall methylation of the 299 CpGs showed no significant differences between the extreme age classes (1.28 vs. 10.5 years) in the muscle, testis or ovary, indicating that differences in the selected CpGs were specific and not due to an overall age-related trend. The performance of the fish epigenetic clock in terms of accuracy and precision (Table 1) compared favorably with clocks developed for other vertebrates (De Paoli-Iseppi et al., 2019). In juvenile European sea bass, accelerated growth due to elevated temperatures had no effect in age prediction, indicating that the clock is able to predict cAge independently of at least some major environmentally-driven perturbations. This study is important not only because it was the first epigenetic clock in a poikilothermic animal and with indeterminate growth, but also because different tissues were assayed and the effects of temperature tested. Nevertheless, environmental influences, especially the early environment, should be assessed since in mammals environmental stress is associated with epigenetic age acceleration (Parrott and Bertucci, 2019). Specifically, glucocorticoid-induced epigenetic changes have been shown to be the mechanisms linking chronic stress with accelerated aging and higher disease risk in mammals (Zannas et al., 2015). In the last years, other piscine epigenetic clocks have been built (Table 1), and are discussed below. Most likely many more are currently being built.
Using the zebrafish (Danio rerio) model and Reduced Representation Bisulfite Sequencing (RRBS), a genome-wide technique to assess DNA methylation (see section 3.4.2. below), and starting with more than half a million CpG loci (Mayne et al., 2020) built a epigenetic clock from fin clips, of high accuracy and consisting of 29 final informative CpG loci. Further, using 26 of these CpGs they successfully implemented a PCR-based method followed by sequencing, retaining clock performance (r = 0.97). However, methylation-sensitive PCR did not work as well (r = 0.62) to predict age with sufficient accuracy. This study demonstrated that age can be epigenetically determined in fish with high accuracy at low cost (Mayne et al., 2020). The same authors then used a PCR-based approach and the CpGs of the zebrafish clock to determine age in three endangered species, the Australian lungfish (Neoceratodus forsteri), the Mary river cod (Maccullochella mariensis) and the Murray cod (Maccullochella peelii) using also fin clips. Remarkably, accuracy was high (r > 0.9 in the three species). This study is important because demonstrates that an epigenetic clock developed in one species can be still used with good performance to determine age in phylogenetically distant species and in a non-lethal manner (Mayne et al., 2021b).
Using medaka (Oryzias latipes) between 2 and 12 months of age and RRBS (Bertucci et al., 2021) built an epigenetic clock using liver samples. Furthermore, they showed that exposure to ionizing radiation during early development caused epigenetic age acceleration. This study is also important because it sets the basis to further explore aging-by-environment interactions (Bertucci et al., 2021). More recently, Weber et al. (2022) developed epigenetic clocks for two species present in the Gulf of Mexico, the Northern red snapper (Lutjanus campechanus) and the Red grouper (Epinephelus morio). The low sample size used (10 fish in each case) prevented the application of sample splitting and rigorous testing to prevent model overfitting, probably explaining the high accuracy obtained (r = 0.98) but lack of proper precision estimation. Nevertheless, the study is important for at least two aspects. First, in contrast to previous studies, this was the first time that an epigenetic clock was developed using wild-caught fish where age had to be also determined by otolith readings. Second, because also for the first time bisulfite RAD-sequencing (bis-RAD-seq) is used. This technique has the advantage that it starts with a still considerable number of CpGs but fewer than with WGBS or RRBS (see section 3.4.2. below) and hence the cost per sample is cheaper.
Regardless of the target species, there are a series of important aspects common to all epigenetic clocks: the age range available to build the clock, the number of samples used, the tissue biopsied to obtain DNA and the method used to analyze its methylation level, the number of CpG loci available for clock construction, the type of penalized (or regularized) regression used, how samples are split between training and test samples, the method of result validation, and then the performance of the clock itself, mainly in terms of accuracy and precision. These characteristics roughly correspond to columns in Table 1 and because of their importance they are further discussed below. But first we want to highlight four aspects worth mentioning.
First, penalized regressions are used for epigenetic clock building because they consist of a type of regression that is “penalized” for having a number of variables (methylation at each CpG loci in epigenetic clocks) much, much higher than the number of samples typically available. Penalization is achieved by adding a constraint in the equation that reduces the coefficients of the less contributing variables towards zero (Fu, 1998). The three most common penalized regression types are: Ridge, LASSO and elastic net, discussed in detail in the review on the bioinformatics aspects of epigenetic clocks (Anastasiadi and Piferrer, 2023). Second, when looking at the informative CpGs used in piscine epigenetic clocks, it seems that the number of CpGs that become hypomethylated with age is higher than the number that become hypermethylated (Weber et al., 2022), but whether this is actually related to the global hypomethylation of vertebrate genomes with age remains to be established. Third, the importance that environmental factors may have on the performance of piscine epigenetic clocks. In this regard, the information available so far seems contradictory. On one hand, as explained above, temperature changes during the thermosensitive period, i.e., the period in which temperature changes are able to elicit important physiological responses such as to affect the sex ratio (Navarro-Martín et al., 2009), did not seem to affect the European sea bass epigenetic clock (Anastasiadi and Piferrer, 2020). However, in the medaka exposure to ionizing radiation affected epigenetic aging by changing the rate of DNA methylation in age-associated CpGs (Bertucci et al., 2021). Environmental effects on ageing in poikilotherms certainly deserve more research to obtain robust conclusions. Fourth, it is worth to mention that age-associated CpG loci in zebrafish were used to build two epigenetic clocks for three threatened freshwater fish species: one for the Australian lungfish and another for the Murray cod and Mary River cod, showing that even phylogenetically divergent species share the same CpG sites that change methylation with age (Mayne et al., 2021b). This significant observation suggests that it may be possible to develop a pan-fish epigenetic clock like the pan-mammalian one (Lu et al., 2021).
3. Outlook and important considerations
Epigenetic clocks constitute a new age estimation tool that is less than 10 years old taking into account that the human epigenetic clock, the first one, was published in December of 2013 (Horvath, 2013). Further, in contrast to other molecular approaches that can be applied to fisheries management such as environmental DNA (eDNA) or Close-kin mark-recapture (CKMR), reviewed elsewhere in this volume (Ramírez-Amaro et al., 2022), no literature reviews exist on the subject of epigenetic clocks to estimate age in fishes, although genomic predictors of lifespan do exist for vertebrates (Mayne et al., 2020). Also, to the best of our knowledge, there are only epigenetic clocks for eight fish species so far (Table 1). Therefore, some considerations relevant in the development of new epigenetic clocks for additional species, with the aim to aid in age estimation in fisheries management and in conservation biology will be discussed.
3.1. General outline for the development of a new piscine epigenetic clock
The steps towards the development of piscine epigenetic clocks for new species can be summarized in the following points below (Figure 3), which do not significantly differ from the ones that should be used for any other vertebrate where age estimation is sought for management or conservation purposes:
1) Species selection
2) Sampling scheme
3) Molecular procedures
4) Bioinformatics and clock-building machine learning methods
5) Validation and implementation: ready-to-use kit
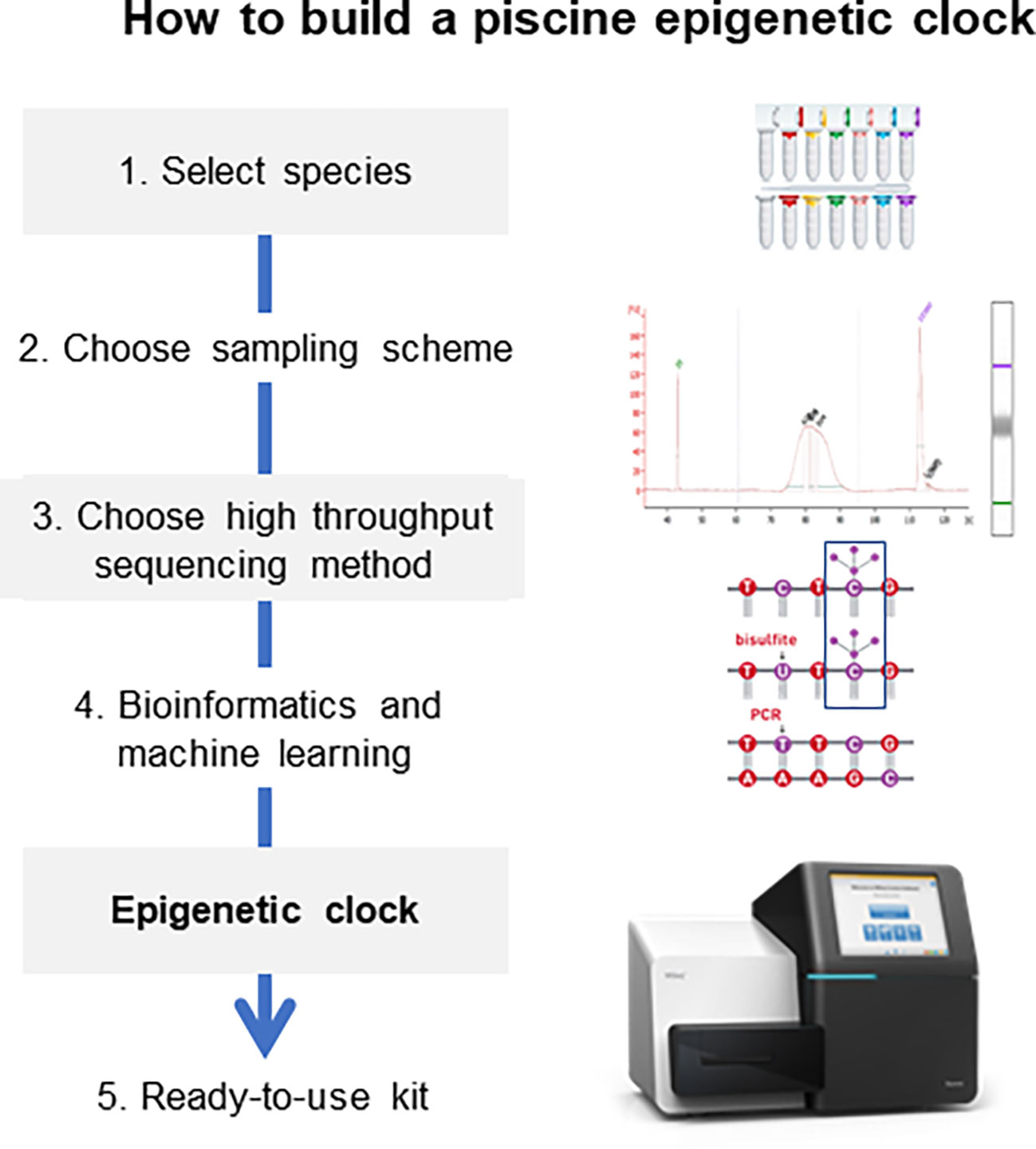
Figure 3 General procedure for the development of epigenetic clocks. Notice that in the bioinformatics pipeline not all samples are used in the same manner; some are used for the train set while others are used for the test set (Anastasiadi and Piferrer, 2023).
Points above are dealt in detail in the following sections below, with the exception of point 4, which are dealt in an ad hoc review purely focused on the bioinformatics aspects of epigenetic clock building (Anastasiadi and Piferrer, 2023).
3.2. Species selection
Since changes in DNA methylation with age is a conserved feature across vertebrates (Jung and Pfeifer, 2015), and since several epigenetic clocks have been already built in fishes (Table 1) an epigenetic clock should be possible to build, in principle, for any fish species, not to mention the possibility to build an all-fish epigenetic clock, as advanced above. However, the actual species to be targeted should have commercial relevance for fisheries or for conservation programs.
One interesting aspect is that fisheries target species of both short (ca. 1 yr.) and long lifespan, e.g., > 10 years. Thus, it should be proved whether the clock performs equally well in both types of species, although a priori there is no reason to suspect otherwise due to the conserved nature of DNA methylation changes. In a comparison involving six mammalian taxa, epigenetic aging seemed faster in species with shorter lifespans, suggesting that the underlying mechanisms of epigenetic aging might contribute to our understanding of interspecific lifespan diversity (Parrott and Bertucci, 2019). Similarly, to study DNA methylation changes associated with age in fishes, species selected should include those with different morphologies (i.e., large and small species in terms of attained maximum length) and also those with differences in life-history traits (i.e., semelparous vs. iteroparous species), to determine whether epigenetic clocks perform well in all of these cases. Lastly, the species considered should come from different geographic locations. Epigenetic modifications have a strong underlying genetic basis and, in addition, can be influenced by changes in the environment such as by temperature changes (Anastasiadi et al., 2018), diet composition (Dhanasiri et al., 2020) and stress (Krick et al., 2021). Therefore, it is possible to find differences among different populations of the same species. If that were the case, clock construction should take into account these differences by selecting loci that are not influenced by genetic variation such as single nucleotide polymorphism (SNPs).
3.3. Sampling scheme
3.3.1. Sample size
In the epigenetic clocks developed so far in fishes, the number of individuals used has been usually lower than 100 (mean: 49; range 10-141). The important aspect of the required sample size to build a reliable epigenetic clock has been addressed by Mayne and colleagues (Mayne et al., 2021a). These authors determined the performance of epigenetic clocks by mean Pearson correlation (r) and maximum absolute error (MAE) in simulations using different sample sizes ranging from 15 to 394 for humans and 15 to 96 for zebrafish with a step size of one. The minimum sample size was determined when the correlation approached the true correlation, defined as the correlation achieved that does not increase more with increasing sample size. These Monte-Carlo simulations included determination of the point of stability (POS), defined when the correlation is within a pre-defined range or width (w) around the true correlation with a certain degree of confidence. Mayne et al. (2021a) showed that the minimum sample size required to develop an epigenetic clock with appropriate accuracy and precision was 70 (w = 0.20 and 80% level of confidence interval) but that for better performance it should approach 134 (w = 0.10 and 95% confidence interval). Of note, the approach used by (Mayne et al., 2021a) provides a robust framework to determine whether future epigenetic clocks have the required statistical power.
There is not a particular number of age-classes that should be sampled per se, as far as the number of samples are evenly distributed and adequately cover the age range for which the clock is meant to be used to estimate age. However, DNA methylation in a given CpG will have a certain level of variation among individuals. If the samples available for clock construction are divided into discrete age classes, then their number per age class might become too low if the total number is reduced. Therefore, the number of age classes should be calculated based on the number of replicates per age class and considering the ideal total estimated by simulations. For example, if the minimum sample size is, say, 72, then not less than four age classes with 18 replicates each and ideally six to eight age classes with 12 and 9 replicates each, respectively, should be used. The availability of enough fish per age class becomes less relevant as far as specimens covering a wide spectrum of sizes are sampled. In any case, it is important to include individuals representative of the top and bottom 10% of known ages (Mayne et al., 2021b). Attention should also be paid to the relationship between time points and age classes as determined by other age-estimation methods such as otolith analysis.
Studies carried out using human blood and saliva samples as tissue sources to develop epigenetic clocks showed that almost 100% of the variation in chronological age could be effectively captured by the DNA methylation probes on the arrays used. Development of epigenetic clocks implies the use of machine learning procedures (see Anastasiadi and Beemelmanns, 2023, for review). This means that the accuracy of a chronological age predictor should increase with a very large training set (Zhang et al., 2019). In practice, actual sample size will determine aspects of machine learning model building, i.e., how samples will be split into training and test and how the models will be evaluated.
3.3.2. Data to be collected from the same individuals
In order to develop piscine epigenetic clocks, it is important to gather information on other variables. Usually, fish age is determined by a combination of size-age relationship, i.e., year classes or the range of length for individuals of a given species at a given age class, and/or the assessment of the hard structures mentioned above (Morales-Nin et al., 1998). Weight usually has a higher coefficient of variation than length because at a given length fish can exhibit quite different weights depending upon food abundance and other environmental factors. In order to relate length and weight the condition factor (K, typically defined as K = 100* W/L3, where W is the weight and L is the length) is used (Karuppiah et al., 2022). There are three ways to determine length: a) standard length (SL), the length measured from the tip of the snout to the posterior end of the last vertebra, i.e., the length without the caudal tail, b) fork length (FL), the length measured from the tip of the snout to the end of the middle caudal fin rays, used in fishes in which it is difficult to tell where the last vertebra ends, and c) total length (TL), the length measured from the tip of the snout to the tip of the longer lobe of the caudal fin (Froese, 2006). It does not matter which one is used for epigenetic age estimation as far it is clearly stated. However, the measure that allows comparison with historical series of data should be favored.
Sex and maturity status are routinely determined during fish sampling and these are important because there are many species in which after a certain age/length there is sexual growth dimorphism (SGD), i.e., one sex grows more than the other (Parker, 1992). Thus, in order to account for the influence of SGD, sex and, if possible, maturity status (e.g., immature, maturing, mature, or spent gonads, after visual inspection) should be included. In the epigenetic clocks developed so far in fish sex has not been properly taken into account, although in the European sea bass muscle epigenetic clock was tested in ovaries and testes (Anastasiadi and Piferrer, 2020). Thus, it is interesting to know about the possible existence of sex-related differences in the tick rate of piscine epigenetic clocks. In fact, for many species of commercial importance such as the Atlantic cod, Gadus morhua (Armstrong et al., 2004), the European hake, Merluccius merluccius (de Pontual et al., 2006) and the Pacific halibut, Hippoglossus stenolepis (Drinan et al., 2018), just to name a few, there are large differences in growth rate and maximum size depending on the sex. Development of epigenetic clocks in these species will therefore need to take into account these differences and to seek whether a sex-independent clock can be built that is also highly accurate.
Geographic location (latitude and longitude) of wild caught samples should also be recorded. This may be helpful in species with large geographical variation, where polymorphisms at certain loci may exist due to the genetic structure of populations. Taking into account the possible effect of genetics may help in excluding polymorphic loci. Also, location can help to know whether there are differences in the magnitude of certain environmental variables that would need to be taken into account as having possible influences on DNA methylation at certain environmentally responsive loci (ERL), loci the methylation of which depends on the magnitude of an environmental factor (Garg et al., 2018).
Fishes are poikilothermic animals and as such their body temperature depends on that of the water. There are some exceptions, such as tunas, which do generate a certain amount of body heat and therefore their body temperature, although still dependent on the water temperature, can be a few degrees above (Barrett and Hester, 1964). Temperature impacts biological processes at different levels of organization by changing the rates of chemical reactions and physiological processes, or by changing the three-dimensional shapes of biomolecules (Schulte et al., 2011). Growth, therefore, depends on temperature and seasonal cycles of growth in fish are particularly apparent in, for example, species living in temperate latitudes or cold latitudes with a short growing season (Boltaña et al., 2017).
Thus, in order to properly construct an epigenetic clock in fish, when sampling the following information should be collected from each fish: 1) Length 2) Weight 3) Condition factor (K), based on the combination of #1 and #2; 4) Sex; 5) Maturity status; 6) Location; and 7) Environmental information, mainly water temperature. Having this data collected will allow to correlate variation in these variables with age or vice versa and, importantly, to have them as potential covariates for optimal clock development.
3.3.3. Selection of tissues from which DNA samples should be obtained
The two major criteria for selection of tissues to be sampled would be: 1) easy access to the tissue and, if possible, that its collection is not lethal to the animal, and 2), that their inclusion should not represent a significant increase in labor in the context of routine samplings during periodic surveys.
In the piscine epigenetic clocks built so far (Table 1), basically three tissues have been used: fin, liver and muscle (Figure 1). Fin clips are very easy to obtain, only a pair of scissors and tweezers are required, and are commonly preferred for genotyping, so they are already routinely taken. Fin clips, in addition, do not damage the aspect of the fish and therefore their commercial value should be retained for sales, and sampling them is not lethal. The liver is a large organ very easy to distinguish once the internal organs have been exposed. During routine sampling cruises, the body cavity of fish is typically opened up since their sex and maturity status is usually assessed. Taking advantage of this to also collect a fragment of the liver would require minimal extra work, while the aspect of fish is not further damaged either, especially if destined for filleting. Other tissues potentially useful would be a muscle fragment or the branchial arch. If the muscle is chosen, care should be taken not to include skin and scales. Blood can be also considered a good candidate since, in fact, has been the tissue of choice in the development of epigenetic clocks in mammals (Bell et al., 2019; De Paoli-Iseppi et al., 2019). Mayne et al. (2020) suggested that, despite its diverse cellular heterogeneity, blood would provide a more homogeneous cell population than fin clips and therefore require less CpGs to build an epigenetic clock but fin clips have already provided very good results (Table 1). The size of samples does not have to be large since DNA is the only thing is needed, so a cube of a few millimeters in volume is sufficient. Dissected tissues should be placed in 95% ethanol (1:3 volume ratio at least) with no methanol content, avoiding prolonged exposure to sunlight, flash frozen with liquid nitrogen or dry ice, if available on board, and in any case stored at 4°C, -20°C or -80°C depending on the possibilities until further analysis.
The tissue should be also selected based on having low propensity to epigenetic age acceleration, i.e., the difference between eAge vs. cAge. This is referred to as the tick rate of the epigenetic clock, usually higher in younger individuals and diminishing in older ones (Horvath, 2013). This means that the relationship between eAge and cAge can be non-linear over the lifespan as shown, for example, in teenager humans (Bell et al., 2019) although the underlying cause and possible external influences are not well understood. With the data available so far it is still soon to know whether age acceleration is also present in fish. Until this aspect becomes clearer, some precautions seem appropriate. Thus, the pronephros or anterior kidney of fish is an analogue of the bone marrow of higher vertebrates and functions as the primary hematopoietic tissue and lymphoid organ in bony fishes (Kondera, 2019). Therefore, the pronephros should probably be avoided when developing an epigenetic clock in fish in order to avoid possible age-related changes in hematopoiesis. Likewise, the brain should also probably be avoided, the reason being that 5-hydoxymethyl-cytosine (5hmC) is an epigenetic modification particularly prevalent in the brain and indistinguishable from 5mC after bisulfite conversion. It is possible that this characteristic of the brain could cause errors in age prediction (Simpson and Chandra, 2021). In humans, in order to aim for higher accuracy for a particular cell-type or specific application, several epigenetic clocks have been developed for single tissues, since cell heterogeneity in tissue composition can compromise the accuracy of epigenetic clocks (Simpson and Chandra, 2021). However, a multi-tissue epigenetic clock like the one developed for humans (Horvath, 2013) has not been developed in fish.
Finally, an aspect worth considering is that the use of fin clips and thus non-lethal sampling theoretically allows repeated measures in the same individuals (e.g., if kept in tanks or through mark-recapture) and hence to expand the age of the models as well as to study DNA methylation changes as fish age (Mayne et al., 2021b). This offers an opportunity that is not possible or very difficult for most tissues, except blood, and hence allows the study of age-related methylation changes on an individual basis, something that perhaps could help to refine models.
3.4. Molecular procedures
3.4.1. DNA samples
DNA quality parameters should be in line with those typically used in molecular biology applications. Therefore, ideally at least 1 µg of clean DNA of high molecular weight (>10 kb) per sample at 20 ng/µl in at least 50 µl volume should be available for clock building. Samples should be checked for degradation. Bisulfite conversion of DNA samples is an essential step of the procedure, nowadays routinely done with commercial kits allowing for > 99.5% bisulfite conversion rates. Targeting specific genomic parts may be performed via enzyme digestion or PCRs or other methods and would typically be followed by sequencing to obtain information at single nucleotide resolution. Downstream procedures are explained in the Bioinformatics review published elsewhere (Anastasiadi and Piferrer, 2023).
3.4.2. High throughput sequencing methods
A whole-genome or genome-wide method should be employed to simultaneously interrogate the methylation of hundreds or thousands of candidate CpGs with the aim to find the most informative CpGs. In the common ancestor of teleosts there was an additional genome duplication (referred to as 3R or teleost-specific genome duplication, TGD) when compared to the rest of vertebrates Thus, teleost genomes tend to contain 10–15% more duplicated genes and pseudogenes than those of other vertebrates (Volff, 2005). Whole Genome Bisulfite Sequencing (WGBS), Reduced Representation Bisulfite Sequencing (RRBS) (Gu et al., 2011) or Bisulfite RAD-seq (bis-RAD-seq;Trucchi et al., 2016) are appropriate techniques for these steps since they provide single nucleotide resolution and wide genome coverage. Here it would suffice to mention that two of these three methods have been used to develop epigenetic clocks in fish and below we made recommendations as which one can be used to develop new clocks. It should be pointed out that by using WGBS one deals with all CpG loci present in the genome, whereas RRBS and bis-RAD-seq are genome-wide but not whole genome methods since they provide only small (< 5%) representation of the genome. Too many CpGs imply also a heavy workload for subsequent bioinformatics processing of the data. For this reason, it is best to use bis-RAD-seq or epiGBS or a similar approach as the first option and RRBS as the second option. Bis-RAD-seq can provide 10.000 to 50.000 CpGs per sample. Notice that this is in the range of initial CpGs used for the development of the human epigenetic clock, but much higher than targeted approaches such as multiplex PCR-based protocols and much lower than RRBS- or WGBS- based protocols (Table 1). Finally, long-read sequencing platforms such as the Oxford nanopore should be mentioned because a major advantage is that it skips the treatment of DNA with bisulfite. This, along the fact that there are portable devices to implement this technique makes it an approach to consider for future development and implementation to estimate age in fish. This review is not intended to discuss details of each method but the reader interested in further details can consult any of the excellent reviews available, e.g., Kurdyukov and Bullock (2016).
DMRs would theoretically be possible to use, i.e., a group of CpGs closely located in a given genomic region the methylation of which changes with age in the same manner. However, in epigenetic clocks DMRs may add noise instead of power since three good CpGs were sufficient in the mice to predict age (Han et al., 2019). During filtering to find informative CpGs, at step 1 low stringency is used and CpGs can be loosely ranked by P-values.
The use of genes or gene parts with as little as possible sequence variation across species, i.e., genes with high nucleotide sequence conservation, can be considered, so informative CpGs in one species could also be informative in other species. Evidence along these lines has recently been provided (Mayne et al. (2021b), where informative CpGs in zebrafish were used to build epigenetic clocks for three fish species phylogenetically quite apart. In the European lobster, the evolutionary conserved ribosomal DNA was targeted for constructing an epigenetic clock (Fairfield et al., 2021). Finally, if many loci are available for selection, one possibility is to target loci with function related to developmental genes, since the ageing process was shown to be evolutionary conserved and linked to developmental processes across mammalian species (Lu et al., 2021).
3.5. Bioinformatics and clock-building machine learning methods
A typical epigenetic biomarker discovery procedure should be followed in general for epigenetic clock construction (Anastasiadi and Beemelmanns, 2023), although some steps can be skipped or adapted depending on resources and phylogenetic information. The aim is to build a model that addresses the question of which epigenetic features (CpGs) show a higher association (correlation) with the outcome quantitative variable (age). Detailed explanations and examples on bioinformatics and clock-building machine learning methods can be found elsewhere in this volume (Anastasiadi and Piferrer, 2023), while expanded details on epigenetic biomarker bioinformatics and statistics can be found elsewhere (Anastasiadi and Beemelmanns, 2023). Briefly, the outcome variable will be predicted based on machine learning methods. Penalized regressions have been used for almost all epigenetic clocks developed so far because they fit well the structure of data and the final objective. These result in the best model or epigenetic clock based on a set of few CpGs with highest accuracy and precision. The number of final informative CpGs in epigenetic clocks developed so far ranges between 3 (mice) to 353 (human) with an average around 50. Among the final, informative, age-correlated CpGs, there tends to be a higher frequency of them that are hypomethylated than hypermethylated (Weber et al., 2022).
3.6. Validation and implementation: Ready-to-use kit
The CpGs that best correlate with age are selected to develop a targeted assay. Methods that can interrogate a few number of loci in a high number of samples are adequate, e.g. multiplex bisulfite sequencing, MBS (Anastasiadi et al., 2018) and MeDIP-qPCR (Jacinto et al., 2008). Other promising methods for application of epigenetic piscine clock for age prediction in thousands of samples include high-throughput multiplex PCR similar to Genotyping-in-Thousands by sequencing (GT-seq) (Kelsey et al., 2017), Tagmentation-based Indexing for Methylation Sequencing (TIMEseq) (Griffin et al., 2021) and cross-species methylation arrays based on conserved CpGs (Arneson et al., 2022). Finally, the practical utility of the approach should be assessed using independent samples.
With the data available from the piscine epigenetic clocks built so far, there seems to be no relationship between the number of samples used to build the clock and the final number of informative CpGs that predict age. Likewise, there seems to be no relationship between the initial number of available CpG sites and the number of informative sites (Figure 2B). However, it is interesting to note that many of the piscine epigenetic clocks built so far end up consisting of around 30 informative CpGs (Table 1). Thus, 30 seems to be an appropriate number of informative CpG able to estimate age in fish with high accuracy. Undoubtedly, more data is needed to determine whether the above affirmations hold as epigenetic clocks are developed for additional species. In this regard, it has been suggested that increasing sample size may capture more biological variation and thus require more CpG sites to accurately predict age (Mayne et al., 2020).
Any epigenetic clock should be, at the end, downscaled to a simple test so cost per sample could be drastically reduced. In this regard, microarrays could provide a good solution. Arrays are available that enable quantitative interrogation of selected methylation sites across the genome, offering high-throughput capabilities that minimize the cost per sample. Thus, Illumina’s Infinium Methylation EPIC BeadChip Kit covers ~850,000 CpGs of the human genome and comes in different formats to analyze 16 (8x2), 32 (8x4) or 96 (8x12) samples arranged in groups of eight. The cost per sample is around 245 € because of the large amount of CpGs covered. However, this cost can be drastically reduced to ~10 € or even less in custom arrays with a significantly lower coverage of CpG sites (Smith, 2022). For example, a 9K Illumina BeadChip for polar bears (Ursus maritimus) was designed from RAD and transcriptome sequencing (Malenfant et al., 2015). Also, a 21K Illumina custom-microarray containing ~80% of the protein coding genes was developed in the European sea bass (Ribas et al., 2019). Therefore, it is possible to envisage a multi-species array for age estimation in fish that could provide reliable results at low cost and able to process a large amount of samples coming from fisheries survey cruises. For example, a five-species array with an average of 50 CpG sites per species done in triplicate plus hybridization controls would require less than one thousand probes. Another alternative would be the construction of high-throughput multiplex PCR assays to determine the methylation level of several age-correlated CpG sites. In this regard, multiplex PCR has been used to estimate the methylation levels of conserved CpGs exhibiting age-related changes of a clock type to build an epigenetic clock for three endangered species (Mayne et al., 2021b).
4. Features of piscine epigenetic clocks as a measuring device
An epigenetic clock to estimate age is, after all, a measuring device based on molecular methods. As such, in this section some characteristics common to all measuring devices are discussed and adapted to the specifics of epigenetic age estimation and, in particular, in fish. These characteristics are: accuracy, precision, repeatability, reproducibility, resolution, sensitivity, and range. The definitions used below are taken from, and in accordance with, accepted international standards (ISO, 1994).
4.1. Accuracy
Accuracy refers to how close is the measured age to the actual “true” age. In epigenetic clocks, accuracy is measured by the degree of correlation between cAge and estimated age. Thus, estimates of model performance are used to report accuracy as Pearson correlation (r), the most popular metric to evaluate regressions. A useful piscine epigenetic clock should be able to correctly classify different age classes. According to Horvath and Raj (2018), an age estimator can be called an “epigenetic clock” only if the correlation between chronological age and estimated age is > 0.80 in a large independent validation data set with a broad range of chronological ages. The piscine epigenetic clocks developed so far have mean accuracy of 0.93 but values of 0.98 are not unusual (Table 1 and Figure 4). Thus, they are able to tell chronological age with high accuracy and nowadays represent the best way to estimate age in fish, much better than what the traditional methods can achieve (Luo et al., 2016). For comparison, in the grey mullet, Mugil cephalus, otolith image analysis coupled with deep learning produced accuracy values of 0.64 at best (Politikos et al., 2021).
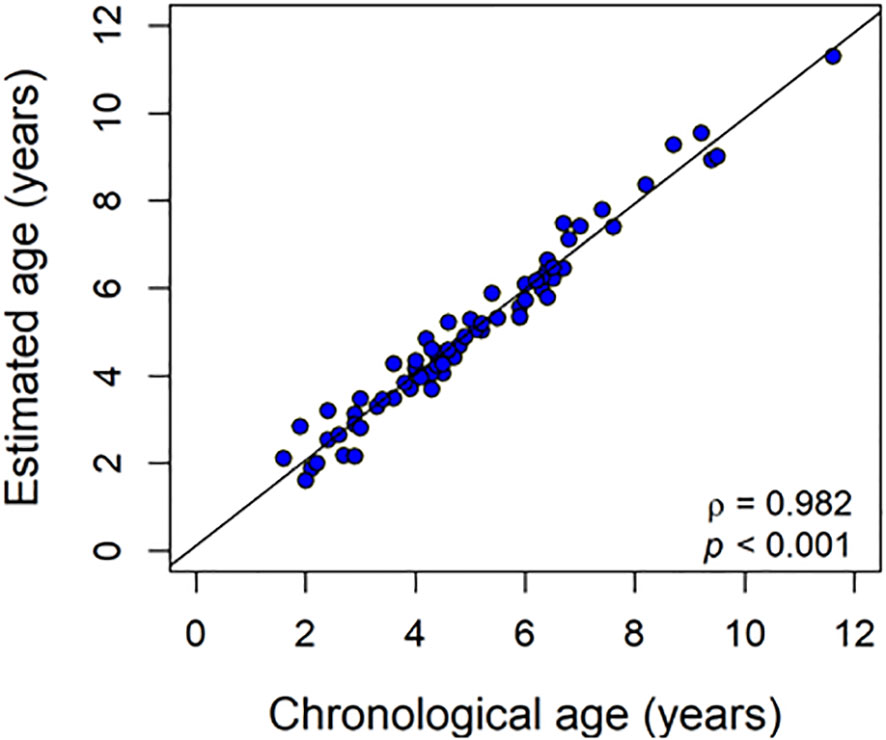
Figure 4 Typical epigenetic clock in fish. Estimated age is regressed against chronological age. In this hypothetical example, the sample size is 70, a number capable of producing good results while accuracy, represented by the correlation coefficient, is 0.95 or higher, common in the latest developed piscine epigenetic clocks.
An inherent aspect of accuracy is bias. Bias is constituted by non-random effects (systematic error) on measured values, and its magnitude should be known and be as low as possible (ISO, 1994). Differences between estimated and true values can be corrected by calibration, e.g., by using a different method of measure. In species where age estimation by otoliths is not possible, this would not be possible either, unless another independent method of age estimation is used (e.g., rearing artificially the species, if feasible). Thus, the selection of the calibration method could include: 1) Length/weight data for independent length-age estimations across a range of environmental conditions, 2) Comparison with other age estimation methods such as otolith or scale readings, and 3) The use of different aquaculture species to fine calibrate representative species, although this possibility can be risky unless age-growth curves among the two species to be compared are well known and are as similar as possible.
The accuracy of epigenetic clocks can be reduced under certain circumstances. For example, in humans cancer is known to change the tick rate of the epigenetic clock (Horvath, 2013). This results in age acceleration, i.e., estimated age is higher than actual chronological age and leads to wrong age estimations. At present, it is not clear whether age acceleration can occur in fishes and, if it does, be of such magnitude as to impose the need for correction measures. For example, one possibility would be that overall changes in temperature or high levels of contamination could affect the tick rate of epigenetic clocks. Addressing such a possibility should be part of the priorities in the development of few piscine epigenetic clocks since we know that coastal or offshore waters, from different regions, will be differentially impacted by contaminants, and clearly, it is vital to be able to exclude such possible impacts from wild-caught fish.
Other potential sources of error can be bias in the sampling, but this is not related to the epigenetic approach per se and, if it is detected, e.g., by observing unusual age-size distributions or that some age classes are under- or over-represented, this is something that should be corrected by improving sampling procedures or by using bootstrapping techniques (Hoyle and Cameron, 2003).
4.2. Precision
Precision is defined as how close to each other are repeated measures of the same variable (ISO, 1994). Thus, given a set of measurements, they are said to be precise if the values are close to each other (e.g., different fish of the same age class in our case). Notice that precision has nothing to do with accuracy, since a series of measurements can be both accurate and precise, accurate but not precise, precise but not accurate or neither.
In the same way that bias was associated with accuracy, there are also aspects associated with precision. In this regard, error is defined as random effects on measured value, and it should be also estimated. Measurement of errors should be reported in combination with accuracy to properly evaluate any regression model. Precision of an epigenetic clock is usually reported as mean absolute error (MAE), which indicates how well the model (the epigenetic clock) fits the actual data, and can usually expressed in time units without conversion, most commonly years. Other typical error measurements for regression models, such as Root Squared Mean Error (RMSE) would require conversion of their units and are thus less intuitive (Hodson, 2022). Error as proportion of the total species lifespan is another intuitive way of reporting, but estimation of MAE would still precede it.
Error can be introduced in many different points of the procedure including during sampling, sample preparation, sequencing and bisulfite conversion. Error can be associated with the specific technique used to assess DNA methylation. Error due to bisulfite treatment should be low, since conversion rates >99% are usually required nowadays (Leontiou et al., 2015). In any case, technologies for measuring DNA methylation are constantly improving and deeper sequencing or other more accurate methodologies are expected to further improve the precision of the epigenetic clocks (Polanowski et al., 2014). Aside from the European sea bass epigenetic clock, built as a proof-of-concept piscine epigenetic clock (Anastasiadi and Piferrer, 2020), current epigenetic clocks have MAE typically of less than 1 year and sometimes even of less than 6 months (Table 1). Minimizing error is very important in age estimation for fisheries management because otherwise poor decisions may be made. The best hard structure-based methods currently available, i.e., Fourier transform near-infrared spectroscopy (FT-NIRS) that can derive age estimates from the vertebral centre provide error values of about 1.5 years (Helser et al., 2019).
4.3. Repeatability and reproducibility
Repeatability is the precision obtained by making the same measure on the same set of samples with the same method. In addition, these measures should be carried out in the same laboratory by the same operator using the same equipment within short intervals of time (Pryseley et al., 2010). This leads to the intra-assay error, expressed as the coefficient of variation (the ratio of the standard deviation to the mean). On the other hand, reproducibility is the precision obtained by making the same measure on the same set of samples in different laboratories with different operators using different equipment and at different times (Pryseley et al., 2010). In addition, measures can include testing complimentary but different methods of measure. This leads to the estimation of inter-assay error. These are inherent parts of precision and should also be considered. In particular, clocks can provide good repeatability but loose reproducibility (e.g., if the tick rate changes), and this is something known to occur in biological clocks as well (Horvath, 2013). Thus, these are aspects worth considering because it is conceivable that samples obtained in a sampling cruise could be processed in different labs. However, repeatability and reproducibility have not been properly reported in the piscine epigenetic clocks developed so far.
4.4. Resolution
The resolution is the minimum difference of age (in months/years) that can be detected and is related to the precision of the clock. A desirable property of a piscine epigenetic clock would be the possibility to identify differences of less than a year, for example, to distinguish 1 from 1+, 1+ from 2, 2 from 2+, etc. age classes. This would allow to gather fine-scale information that would represent an advantage for fisheries management. Assessing differences of less than one year is possible in some species using otoliths (Luo et al., 2016) but is not the norm. However, this requires an exhaustive analysis of the daily growth rings, if feasible, using scanning electron microscopy and it is done only for research purposes, but not for routine age estimation. It should be noted that the tick rate of vertebrate epigenetic clocks is higher with younger individuals, as assessed both in different human cell lines (Horvath, 2013) and so it seems to be in sea bass (Anastasiadi and Piferrer, 2020). This may imply higher variability in DNA methylation at a given series of CpGs and thus it may require including more samples of these early age classes when developing epigenetic clocks for new species.
4.5. Sensitivity
The sensitivity is the minimum value (age) that can be detected. This is a challenge because in the epigenetic clocks developed in vertebrates age estimation around one year seems difficult. From a fisheries/conservation point of view, ability to distinguish 0, 0+ and 1 age classes would be considered an important aspect because these are crucial stages for the recruitment towards future exploited populations, especially when it comes to highly dynamic populations or very short-lived species. Clocks developed specifically for younger individuals might provide a solution to this. Having precise information of these age classes is an important priority for fisheries management. Not counting the model species like zebrafish and medaka, which are short-lived, and based in what has been reported for the Australian lungfish and Mary river cod (Mayne et al., 2021b), discrimination among the 0, 0+ and 1 ages classes seems feasible.
4.6. Range
The range, or the difference between maximum and minimum age predicted, should also be defined for a piscine epigenetic clock. This is a very important aspect because otolith measurement accuracy ceases to work once fish reach a certain age/length due to the peculiarities of the growth curve (Campana and Thorrold, 2001).
This limitation of current methods may be circumvented with epigenetic clocks since DNA methylation changes still take place even when growth slows down with age. In this regard, the vertebrate epigenetic clocks developed so far have been used with wide age ranges: 0.1-77 years in fish (Table 1); 0-21 years in a sea bird (De Paoli-Iseppi et al., 2019); 0-30 years in whales (Polanowski et al., 2014); 0-14 years in bats (Wright et al., 2018), 2-39 years in chimpanzees (IIto et al., 2018), 0-23 years in naked mole-rats (Lowe et al., 2020). Therefore, epigenetic clocks in fishes should allow age estimation during the whole lifespan and thus including the age classes commonly targeted by fisheries. An interesting aspect of the DNA methylation-based clocks is that the differences in known and estimated age show little heteroscedasticity, meaning that variance in the differences is similar throughout the range of ages assayed (Polanowski et al., 2014).
5. How to address the integration of age estimation using DNA methylation-based biomarkers in fisheries management?
In the sections above, some of the drawbacks and limitations of the methods commonly used to estimate age in fish for fisheries management have been explained. It has been shown that DNA methylation changes occur as animals age and that some of these changes are of a clock-like type. Consequently, identifying loci that exhibit DNA methylation changes highly correlated with time is the basis for the development of epigenetic clocks. Their development in humans (Horvath, 2013) and other vertebrates (De Paoli-Iseppi et al., 2019) suggested that epigenetic clocks should be possible to develop in fish. The major doubt about the success of such enterprise was the fact that all previous clocks in vertebrates were generated in several mammalian and a bird species, i.e., warm-blooded species with determinate growth, while fish are poikilothermic and have indeterminate growth. The first epigenetic clock in fish was developed in the European sea bass, taking advantage of the fact that age could be known with accuracy because fish were of hatchery origin (Anastasiadi and Piferrer, 2020). This helped to pave the way for the development of similar clocks in other fish species of commercial importance (Table 1). The current state of the art constitutes, in our opinion, a very promising framework upon which additional applications can be developed, especially now that we know that epigenetic clocks across many species can be developed (Lu et al., 2021; Robeck et al., 2021).
To summarize, in our opinion the major advantages of the new DNA methylation-based epigenetic clocks for age estimation in fish over traditional methods such as otolith analysis are:
1. High accuracy
2. High precision
3. Non-lethal
4. Does not damage the commercial value of the specimens
5. Potential transferability to other species since informative loci developed in one species can be used in a different species (with adjustments) reducing cost of development for the new species.
6. Higher consistency between labs/investigators, since standardized measurements rely on computed values with clear protocols, and thus do not rely on interpretation like otolith readings.
7. Age can be estimated independently of growth patterns by the identification of CpGs with clock-like methylation changes with age.
Points of caution and strategies for mitigation include:
1. Personnel needs specific knowledge but training in general molecular biology/sequencing techniques and general bioinformatics should be enough.
2. The process of sampling tissue, extracting DNA, assessing DNA methylation, analyzing, predicting age can be time-consuming at the beginning but the whole process is prone to be automated and eventually will allow the processing of hundreds or thousands of samples together.
3. A ready-to-use kit for commercial application has not been developed yet. Cost per sample can be expensive until such type of kit is ready.
4. The influence of environmental and other cues needs to be better studied. Information is still too fragmentary to make strong conclusions about the independence of such external influences.
Some aspects that are important to take into account towards the development of piscine epigenetic clocks for their application in fisheries management have also been presented. These included the number of samples to be collected, tissues to be targeted, handling DNA sample preservation and the type of epigenetic modifications to be measured, clearly favoring DNA methylation. The steps on how to develop such a clock and the techniques available to do so have also been explained. Finally, a section has been devoted on the different characteristics such as accuracy, precision, etc., that as any measuring device the piscine epigenetic clock should have. Here it should be emphasized that most likely a set of < 100 carefully selected loci would be enough to predict age with both accuracy and precision. Expertise in epigenetics, machine learning methods and fisheries management are essential to bring this endeavor to success.
No major technical difficulties are envisaged, perhaps the most important aspect is, once developed, the actual technique can then be used in large sample sizes at a very low cost. Nowadays age estimation involves otolith extraction, processing and analysis and therefore constitutes a significant part of the cost of stock assessment (Helser et al., 2019). The cost benefit of implementing epigenetic clocks is dealt with in great detail in another review of this special volume that also includes other molecular-based techniques for fisheries management (Rodríguez-Rodríguez et al., 2022).
Importantly, improved age prediction would contribute to better fisheries management in a context of overexploited fish stocks worldwide. It can be envisaged that one day regional, national and supranational organizations involved in fisheries management will use epigenetic clocks for age estimation in commercial fisheries. Further, advances in techniques aimed at measuring DNA methylation will make it possible to estimate age in large amounts of fish at a very low cost.
Challenges to be resolved include to experimentally verify that, as expected, there will be specific CpG loci with age-related methylation changes that are conserved across fish species. If so, this would facilitate the development of multi-species epigenetic clocks. A particularly important aspect is to determine how environmental changes, especially temperature and food availability may affect the tick rate of piscine epigenetic clocks. Recall that the evidence gathered so far does not allow firm conclusions since temperature did not affect the performance of the European sea bass clock (Anastasiadi and Piferrer, 2020) but ionizing radiation affected the medaka epigenetic clock (Bertucci et al., 2021). Further, it may be difficult to obtain individual samples from the whole age range, especially towards the maximum lifespan, for some fish species, or if age estimation by otoliths or other current method is problematic, calibration of the epigenetic clock may be challenging.
Fish are the most diverse group of vertebrates in many aspects, including life-history traits, morphology, habitat occupancy, reproduction, etc. This diversity may be regarded as a challenge, but in fact piscine epigenetic clocks have been built from both short-lived species such as zebrafish or medaka and very long-lived species such as the Australian lungfish, although the error of the clock of the Australian lungfish was lower in the oldest fish (Mayne et al., 2021b). Thus, despite their high degree of diversity in many aspects of their biology, including longevity, this does not seem an obstacle for the development of epigenetic clocks.
Last but not least, estimation of fish age would also be a very useful molecular resource for ecological studies and, in particular, for those aimed at conservation purposes. Thus, epigenetic clocks could be of practical use in the estimation of age classes in endangered species, as well as for fish biology in general. Epigenetic age estimation as well as estimation of other essential parameters such as species population structure will likely improve stock assessment and fisheries management in a significant manner in the years to come.
Author contributions
FP conceived and wrote the manuscript. DA conceived and wrote the manuscript. All authors contributed to the article and approved the submitted version.
Funding
The study was funded through EU Contract – EMFF/2018/015. Research at the F.P. laboratory was also supported by Spanish Ministry of Science Grant No. PID2019-108888RB-I00. Also with funding from the Spanish government through the ‘Severo Ochoa Centre of Excellence’ Accreditation (CEX2019-000928-S).
Acknowledgments
We would like to thank Drs. Gary Carvalho, Sissel Jentoft, Laszlo Orban and Allan Tucker and the two reviewers for constructive criticisms and suggestions for improvement.
Conflict of interest
The authors declare that the research was conducted in the absence of any commercial or financial relationships that could be construed as a potential conflict of interest.
Publisher’s note
All claims expressed in this article are solely those of the authors and do not necessarily represent those of their affiliated organizations, or those of the publisher, the editors and the reviewers. Any product that may be evaluated in this article, or claim that may be made by its manufacturer, is not guaranteed or endorsed by the publisher.
Author disclaimer
The information and views set out in this publication are based on scientific data and information collected under Service Contract “Improving cost-efficiency of fisheries research surveys and fish stocks assessments using next-generation genetic sequencing methods [EMFF/2018/015]” signed with the European Climate, Infrastructure and Environment Executive Agency (CINEA) and funded by the European Union. The information and views set out in this publication are those of the author(s) and do not necessarily reflect the official opinion of CINEA or of the European Commission. Neither CINEA nor the European Commission can guarantee the accuracy of the scientific data/information collected under the above Specific Contract or the data/information included in this publication. Neither CINEA nor the European Commission or any person acting on their behalf may be held responsible for the use which may be made of the information contained therein.
References
Aguilera B., Catalán I. A., Palomera I., Olivar M. P. (2009). Crecimiento del otolito en larvas de lubina europea (Dicentrarchus labrax, l.) bajo régimen de alimentación constante o variable. Sci. Mar. 73, 183–197. doi: 10.3989/scimar.2009.73n1173
Anastasiadi D., Beemelmanns A. (2023). “Development of epigenetic biomarkers in aquatic organisms,” in Piferrer F., Wang H. P. Epigenetics in Aquaculture (Hoboken, NJ: John Wiley & Sons Ltd).
Anastasiadi D., Piferrer F. (2020). A clockwork fish: Age prediction using DNA methylation-based biomarkers in the European seabass. Mol. Ecol. Resour. 20, 387–397. doi: 10.1111/1755-0998.13111
Anastasiadi D., Piferrer F. (2023). Bioinformatic analysis for age prediction using epigenetic clocks: Application to fisheries management and conservation biology. Front. Mar. Sci. 10, 1096909. doi: 10.3389/fmars.2023.1096909
Anastasiadi D., Vandeputte M., Sánchez-Baizán N., Allal F., Piferrer F. (2018). Dynamic epimarks in sex-related genes predict gonad phenotype in the European sea bass, a fish with mixed genetic and environmental sex determination. Epigenetics 13, 988–1011. doi: 10.1080/15592294.2018.1529504
Armstrong M. J., Gerritsen H. D., Allen M., McCurdy W. J., Peel J. A. D. (2004). Variability in maturity and growth in a heavily exploited stock: cod (Gadus morhua l.) in the Irish Sea. ICES J. Mar. Sci. 61, 98–112. doi: 10.1016/j.icesjms.2003.10.005
Arneson A., Haghani A., Thompson M. J., Pellegrini M., Kwon S. B., Vu H., et al. (2022). A mammalian methylation array for profiling methylation levels at conserved sequences. Nat. Commun. 13, 783. doi: 10.1038/s41467-022-28355-z
Bank C. (2016). Validation of age determination methods for monkfish (Lophius americanus) M.Sc. thesis. (Dartmouth: University of Massachusetts) 104. doi: 10.13140/RG.2.2.36759.57761
Barrett I., Hester F. J. (1964). Body temperature of yellowfin and skipjack tunas in relation to sea surface temperature. Nature 203, 96–97. doi: 10.1038/203096b0
Bell C. G., Lowe R., Adams P. D., Baccarelli A. A., Beck S., Bell J. T., et al. (2019). DNA Methylation aging clocks: challenges and recommendations. Genome Biol. 20, 249. doi: 10.1186/s13059-019-1824-y
Bertucci E. M., Mason M. W., Rhodes O. E., Parrott B. B. (2021). Exposure to ionizing radiation disrupts normal epigenetic aging in Japanese medaka. Aging 13, 22752–22771. doi: 10.18632/aging.203624
Boltaña S., Sanhueza N., Aguilar A., Gallardo-Escarate C., Arriagada G., Valdes J. A., et al. (2017). Influences of thermal environment on fish growth. Ecol. Evol. 7, 6814–6825. doi: 10.1002/ece3.3239
Brock H. W., Fisher C. L. (2005). Maintenance of gene expression patterns. Dev. Dyn. 232, 633–655. doi: 10.1002/dvdy.20298
Campana S. E. (2001). Accuracy, precision and quality control in age determination, including a review of the use and abuse of age validation methods. J. Fish Biol. 59, 197–242. doi: 10.1111/j.1095-8649.2001.tb00127.x
Campana S. E., Thorrold S. R. (2001). Otoliths, increments, and elements: keys to a comprehensive understanding of fish populations? Can. J. Fish. Aquat. Sci. 58, 30–38. doi: 10.1139/f00-177
Campos C., Sundaram A. Y., Valente L. M., Conceição L. E., Engrola S., Fernandes J. M. (2014). Thermal plasticity of the miRNA transcriptome during Senegalese sole development. BMC Genomics 15, 525. doi: 10.1186/1471-2164-15-525
Chen X., Wang Z., Tang S., Zhao Y., Zhao J. (2017). Genome-wide mapping of DNA methylation in Nile tilapia. Hydrobiologia 791, 247–257. doi: 10.1007/s10750-016-2823-6
Deans C., Maggert K. A. (2015). What do you mean, “Epigenetic”? Genetics 199, 887–896. doi: 10.1534/genetics.114.173492
De Paoli-Iseppi R., Deagle B. E., Polanowski A. M., McMahon C. R., Dickinson J. L., Hindell M. A., et al. (2019). Age estimation in a long-lived seabird ( Ardenna tenuirostris ) using DNA methylation-based biomarkers. Mol. Ecol. Resour 19, 411–425. doi: 10.1111/1755-0998.12981
de Pontual H., Groison A. L., Piñeiro C., Bertignac M. (2006). Evidence of underestimation of European hake growth in the bay of Biscay, and its relationship with bias in the agreed method of age estimation. ICES J. Mar. Sci. 63, 1674–1681. doi: 10.1016/j.icesjms.2006.07.007
Dhanasiri A. K. S., Chen X., Dahle D., Siriyappagouder P., Fæste C. K., Fernandes J. M. O. (2020). Dietary inclusion of plant ingredients induces epigenetic changes in the intestine of zebrafish. Epigenetics 15, 1035–1051. doi: 10.1080/15592294.2020.1747777
Drinan D. P., Loher T., Hauser L. (2018). Identification of genomic regions associated with sex in pacific halibut. J. Heredity 109, 326–332. doi: 10.1093/jhered/esx102
Fairfield E. A., Richardson D. S., Daniels C. L., Butler C. L., Bell E., Taylor M. I. (2021). Ageing European lobsters ( Homarus gammarus ) using DNA methylation of evolutionarily conserved ribosomal DNA. Evol. Appl. 14, 2305–2318. doi: 10.1111/eva.13296
Froese R. (2006). Cube law, condition factor and weight-length relationships: History, meta-analysis and recommendations. J. Appl. Ichthyol 22, 241–253. doi: 10.1111/j.1439-0426.2006.00805.x
Fu W. J. (1998). Penalized regressions: The bridge versus the lasso. J. Comput. Graphical Stat 7, 397. doi: 10.2307/1390712
Gardner S. T., Bertucci E. M., Sutton R., Horcher A., Aubrey D., Parrott B. B. (2022). Development of DNA methylation-based epigenetic age predictors in loblolly pine ( Pinus taeda ). bioRxiv. doi: 10.1101/2022.01.27.477887
Garg P., Joshi R. S., Watson C., Sharp A. J. (2018). A survey of inter-individual variation in DNA methylation identifies environmentally responsive co-regulated networks of epigenetic variation in the human genome. PloS Genet. 14, e1007707. doi: 10.1371/journal.pgen.1007707
Goll M. G., Bestor T. H. (2005). Eukaryotic Cytosine Methyltransferases. Annu. Rev. Biochem. 74, 481–514. doi: 10.1146/annurev.biochem.74.010904.153721
Griffin P. T., Kane A. E., Trapp A., Li J., McNamara M. S., Meer M. V., et al. (2021). TIME-seq enables scalable and inexpensive epigenetic age predictions (preprint). bioRxiv. doi: 10.1101/2021.10.25.465725
Guevara E. E., Lawler R. R. (2018). Epigenetic clocks. Evol. Anthropol 27, 256–260. doi: 10.1002/evan.21745
Gu H., Smith Z. D., Bock C., Boyle P., Gnirke A., Meissner A. (2011). Preparation of reduced representation bisulfite sequencing libraries for genome-scale DNA methylation profiling. Nat. Protoc. 6, 468–481. doi: 10.1038/nprot.2010.190
Han Y., Franzen J., Stiehl T., Gobs M., Kuo C.-C., Nikolić M., et al. (2019). New targeted approaches for epigenetic age predictions. BMC Biol 18, 71. doi: 10.1186/s12915-020-00807-2
Helser T. E., Benson I. M., Barnett B. K. (2019). Proceedings of the research workshop on the rapid estimation of fish age using Fourier transform near infrared spectroscopy (FT-NIRS). AFSC Processed Rep. (Seattle WA: Alaska Fish. Sci. Cent., NOAA, Natl. Mar. Fish. Serv.,) 2019-06, 195. Available at: https://www.afsc.noaa.gov/Publications/ProcRpt/PR2019-06.pdf.
Hermann A., Gowher H., Jeltsch A. (2004). Biochemistry and biology of mammalian DNA methyltransferases. CMLS, cell. Mol. Life Sci. 61, 2571–2587. doi: 10.1007/s00018-004-4201-1
Heydenrych M. J., Saunders B. J., Bunce M., Jarman S. N. (2021). Epigenetic measurement of key vertebrate population biology parameters. Front. Ecol. Evol. 9. doi: 10.3389/fevo.2021.617376
Heyn H., Li N., Ferreira H. J., Moran S., Pisano D. G., Gomez A., et al. (2012). Distinct DNA methylomes of newborns and centenarians. Proc. Natl. Acad. Sci. U.S.A. 109, 10522–10527. doi: 10.1073/pnas.1120658109
Hodson T. O. (2022). Root-mean-square error (RMSE) or mean absolute error (MAE): when to use them or not. Geosci. Model. Dev. 15, 5481–5487. doi: 10.5194/gmd-15-5481-2022
Horvath S. (2013). DNA Methylation age of human tissues and cell types. Genome Biol. 14, R115. doi: 10.1186/gb-2013-14-10-r115
Horvath S., Raj K. (2018). DNA Methylation-based biomarkers and the epigenetic clock theory of ageing. Nat. Rev. Genet. 19, 371–384. doi: 10.1038/s41576-018-0004-3
Hoyle S. D., Cameron D. S. (2003). Confidence intervals on catch estimates from a recreational fishing survey: a comparison of bootstrap methods. Fisheries Manage. Ecol. 10, 97–108. doi: 10.1046/j.1365-2400.2003.00321.x
Illingworth R. S., Bird A. P. (2009). CpG islands - ‘A rough guide.’ FEBS letters 583, 1713–1720. doi: 10.1016/j.febslet.2009.04.012
ISO (1994). Accuracy (trueness and precision) of measurement methods and results — part 1: General principles and definitions (Vernier, Switzerland: International Standards Organization) Document no. 5725-1. Available at: https://www.iso.org/obp/ui/#iso:std:iso:5725:-1:ed-1:v1:en.
Ito H., Udono T., Hirata S., Inoue-Murayama M. (2018). Estimation of chimpanzee age based on DNA methylation. Sci. Rep. 8, 9998. doi: 10.1038/s41598-018-28318-9
Jacinto F. V., Ballestar E., Esteller M. (2008). Methyl-DNA immunoprecipitation (MeDIP): Hunting down the DNA methylome. BioTechniques 44, 35–43. doi: 10.2144/000112708
Jung M., Pfeifer G. P. (2015). Aging and DNA methylation. BMC Biol. 13, 7. doi: 10.1186/s12915-015-0118-4
Karuppiah K., Ethiraj K., Sekar S., Rajendran K., Krishnamoorthy M., Dharmaraj D. (2022). Weight–length relationships and fulton’s condition factors of ten commercially important scombridae fish species in southeast coast of India, bay of Bengal. Thalassas 38, 709–713. doi: 10.1007/s41208-021-00390-5
Kelsey G., Stegle O., Reik W. (2017). Single-cell epigenomics: Recording the past and predicting the future. Science 358, 69–75. doi: 10.1126/science.aan6826
Kohli R. M., Zhang Y. (2013). TET enzymes, TDG and the dynamics of DNA demethylation. Nature 502, 472–479. doi: 10.1038/nature12750
Kondera E. (2019). Haematopoiesis and haematopoietic organs in fish. Roczniki Naukowe Polskiego Towarzystwa Zootechnicznego 15, 9–16. doi: 10.5604/01.3001.0013.4535
Krick M. V., Desmarais E., Samaras A., Guéret E., Dimitroglou A., Pavlidis M., et al. (2021). Family-effects in the epigenomic response of red blood cells to a challenge test in the European sea bass (Dicentrarchus labrax, l.). BMC Genomics 22, 111. doi: 10.1186/s12864-021-07420-9
Kurdyukov S., Bullock M. (2016). DNA Methylation analysis: Choosing the right method. Biology 5, 3. doi: 10.3390/biology5010003
Lee D., Shin C. (2012). MicroRNA–target interactions: New insights from genome-wide approaches. Ann. New York Acad. Sci. 1271, 118–128. doi: 10.1111/j.1749-6632.2012.06745.x
Leontiou C. A., Hadjidaniel M. D., Mina P., Antoniou P., Ioannides M., Patsalis P. C. (2015). Bisulfite conversion of DNA: Performance comparison of different kits and methylation quantitation of epigenetic biomarkers that have the potential to be used in non-invasive prenatal testing. PloS One 10, e0135058. doi: 10.1371/journal.pone.0135058
Lowe R., Danson A. F., Rakyan V. K., Yildizoglu S., Saldmann F., Viltard M., et al. (2020). DNA Methylation clocks as a predictor for ageing and age estimation in naked mole-rats, Heterocephalus glaber. Aging 12, 4394–4406. doi: 10.18632/aging.102892
Lu A. T., Fei Z., Haghani A., Robeck T. R., Zoller J. A., Li C. Z., et al. (2021). Universal DNA methylation age across mammalian tissues (preprint). bioRxiv. doi: 10.1101/2021.01.18.426733
Luo W.-W., Liu C.-S., Cao X.-J., Huang L.-F., Huang S.-Q. (2016). Precision of age estimations from scales, otoliths, vertebrae, opercular bones and cleithra of two loaches, Misgurnus anguillicaudatus and Paramisgurnus dabryanus. Folia Zoologica 65, 183–188 . doi: 10.25225/fozo.v65.i3.a2.2016
Malenfant R. M., Coltman D. W., Davis C. S. (2015). Design of a 9K illumina BeadChip for polar bears ( Ursus maritimus ) from RAD and transcriptome sequencing. Mol. Ecol. Resour 15, 587–600. doi: 10.1111/1755-0998.12327
Mayne B., Berry O., Jarman S. (2021a). Optimal sample size for calibrating DNA methylation age estimators. Mol. Ecol. Resour. 21, 2316–2323. doi: 10.1111/1755-0998.13437
Mayne B., Espinoza T., Roberts D., Butler G. L., Brooks S., Korbie D., et al. (2021b). Nonlethal age estimation of three threatened fish species using DNA methylation: Australian lungfish, Murray cod and Mary river cod. Mol. Ecol. Resour. 21, 2324–2332. doi: 10.1111/1755-0998.13440
Mayne B., Korbie D., Kenchington L., Ezzy B., Berry O., Jarman S. (2020). A DNA methylation age predictor for zebrafish. Aging 12, 24817–24835. doi: 10.18632/aging.202400
Mayne B., Mustin W., Baboolal V., Casella F., Ballorain K., Barret M., et al. (2022). Age prediction of green turtles with an epigenetic clock. Mol. Ecol. Resour. 22, 2275–2284. doi: 10.1111/1755-0998.13621
Moore L. D., Le T., Fan G. (2013). DNA Methylation and its basic function. Neuropsychopharmacol 38, 23–38. doi: 10.1038/npp.2012.112
Morales-Nin B., Torres G. J., Lombarte A., Recasens L. (1998). Otolith growth and age estimation in European hake. J. Fish Biol. 53, 1155–1168. doi: 10.1111/j.1095-8649.1998.tb00239.x
Navarro-Martín L., Blázquez M., Viñas J., Joly S., Piferrer F. (2009). Balancing the effects of rearing at low temperature during early development on sex ratios, growth and maturation in the European sea bass (Dicentrarchus labrax). Aquaculture 296, 347–358. doi: 10.1016/j.aquaculture.2009.07.022
O’Dea R. E., Noble D. W. A., Johnson S. L., Hesselson D., Nakagawa S. (2016). The role of non-genetic inheritance in evolutionary rescue: Epigenetic buffering, heritable bet hedging and epigenetic traps. Environ. Epigenet 2, dvv014. doi: 10.1093/eep/dvv014
Ordoñez A., Eikvil L., Salberg A.-B., Harbitz A., Murray S. M., Kampffmeyer M. C. (2020). Explaining decisions of deep neural networks used for fish age prediction. PloS One 15, e0235013. doi: 10.1371/journal.pone.0235013
Pardo S. A., Cooper A. B., Dulvy N. K. (2013). Avoiding fishy growth curves. Methods Ecol. Evol. 4, 353–360. doi: 10.1111/2041-210x.12020
Parker G. A. (1992). The evolution of sexual size dimorphism in fish*. J. Fish Biol. 41, 1–20. doi: 10.1111/j.1095-8649.1992.tb03864.x
Parrott B. B., Bertucci E. M. (2019). Epigenetic aging clocks in ecology and evolution. Trends Ecol. Evol. 34, 767–770. doi: 10.1016/j.tree.2019.06.008
Polanowski A. M., Robbins J., Chandler D., Jarman S. N. (2014). Epigenetic estimation of age in humpback whales. Mol. Ecol. Resour 14, 976–987. doi: 10.1111/1755-0998.12247
Politikos D. V., Petasis G., Chatzispyrou A., Mytilineou C., Anastasopoulou A. (2021). Automating fish age estimation combining otolith images and deep learning: The role of multitask learning. Fisheries Res. 242, 106033. doi: 10.1016/j.fishres.2021.106033
Pryseley A., Mintiens K., Knapen K., van der Stede Y., Molenberghs G. (2010). Estimating precision, repeatability, and reproducibility from Gaussian and non- Gaussian data: a mixed models approach. J. Appl. Stat 37, 1729–1747. doi: 10.1080/02664760903150706
Ramírez-Amaro S., Bassitta M., Picornell A., Ramon C., Terrasa B. (2022). Environmental DNA: State-of-the-art of its application for fisheries assessment in marine environments. Front. Mar. Sci. 9. doi: 10.3389/fmars.2022.1004674
Rasmussen K. D., Helin K. (2016). Role of TET enzymes in DNA methylation, development, and cancer. Genes Dev. 30, 733–750. doi: 10.1101/gad.276568.115
Ribas L., Crespo B., Sánchez-Baizán N., Xavier D., Kuhl H., Rodríguez J. M., et al. (2019). Characterization of the European sea bass (Dicentrarchus labrax) gonadal transcriptome during sexual development. Mar. Biotechnol. 21, 359–373. doi: 10.1007/s10126-019-09886-x
Ribas L., Vanezis K., Imués M. A., Piferrer F. (2017). Treatment with a DNA methyltransferase inhibitor feminizes zebrafish and induces long-term expression changes in the gonads. Epigenet. Chromatin 10, 59. doi: 10.1186/s13072-017-0168-7
Robeck T. R., Fei Z., Lu A. T., Haghani A., Jourdain E., Zoller J. A., et al. (2021). Multi-species and multi-tissue methylation clocks for age estimation in toothed whales and dolphins. Commun. Biol. 4, 642. doi: 10.1038/s42003-021-02179-x
Rodríguez-Rodríguez G., Ballesteros H. M., Sánchez-Llamas E., Bande R., Otero R. F. (2022). The state of the art in cost-benefit of HTS methods for stock assessment: An overview. Front. Mar. Sci. 9. doi: 10.3389/fmars.2022.1005534
Schulte P. M., Healy T. M., Fangue N. A. (2011). Thermal performance curves, phenotypic plasticity, and the time scales of temperature exposure. Integr. Comp. Biol. 51, 691–702. doi: 10.1093/icb/icr097
Shimoda N, Izawa T, Yoshizawa A, Yokoi H, Kikuchi Y, Hashimoto N., et al (2014). Decrease in cytosine methylation at CpG island shores and increase in DNA fragmentation during zebrafish aging. Age (Dodr) 36 (1), 103–15. doi: 10.1007/s11357-013-9548-5
Smith C. (2022). DNA Microarrays: A trusted tool keeps evolving. Biocompare. The buyer’s guide for Life scientists. Available at: https://www.biocompare.com/Editorial-Articles/172195-DNA-Microarrays-A-Trusted-Tool-Keeps-Evolving/.
Sutton C., Maolagáin C.Ó., McKenzie J. (2023). Determining the age of fish. National Institute of Water and Atmospheric Research (NIWA). Available at: https://niwa.co.nz/fisheries/research-projects/determining-the-age-of-fish.
Trucchi E., Mazzarella A. B., Gilfillan G. D., Lorenzo M. T., Schönswetter P., Paun O. (2016). Bs RAD seq: screening DNA methylation in natural populations of non-model species. Mol. Ecol. 25, 1697–1713. doi: 10.1111/mec.13550
van Gelderen T. A., Montfort J., Alvarez-Dios J. A., Thermes V., Piferrer F., Bobe J., et al. (2022). Deciphering sex-specific miRNAs as heat-recorders in zebrafish. Sci. Rep. 12, 18722. doi: 10.1038/s41598-022-21864-3
Venney C. J., Johansson M. L., Heath D. D. (2016). Inbreeding effects on gene-specific DNA methylation among tissues of Chinook salmon. Mol Ecol. 25(18), 4521–33. doi: 10.1111/mec.13777
Volff J.-N. (2005). Genome evolution and biodiversity in teleost fish. Heredity 94, 280–294. doi: 10.1038/sj.hdy.6800635
Weber D. N., Fields A. T., Patterson W. F., Barnett B. K., Hollenbeck C. M., Portnoy D. S. (2022). Novel epigenetic age estimation in wild-caught gulf of Mexico reef fishes. Can. J. Fish. Aquat. Sci. 79, 1–5. doi: 10.1139/cjfas-2021-0240
Wright P. G. R., Mathews F., Schofield H., Morris C., Burrage J., Smith A., et al. (2018). Application of a novel molecular method to age free-living wild bechstein’s bats. Mol. Ecol. Resour 18, 1374–1380. doi: 10.1111/1755-0998.12925
Zannas A. S., Arloth J., Carrillo-Roa T., Iurato S., Röh S., Ressler K. J., et al. (2015). Lifetime stress accelerates epigenetic aging in an urban, African American cohort: relevance of glucocorticoid signaling. Genome Biol. 16, 266. doi: 10.1186/s13059-015-0828-5
Keywords: age estimation, age predictor, epigenetic clocks, fisheries management, conservation biology, DNA methylation, machine learning, penalized regressions
Citation: Piferrer F and Anastasiadi D (2023) Age estimation in fishes using epigenetic clocks: Applications to fisheries management and conservation biology. Front. Mar. Sci. 10:1062151. doi: 10.3389/fmars.2023.1062151
Received: 05 October 2022; Accepted: 13 January 2023;
Published: 30 January 2023.
Edited by:
Rodrigo Vidal, University of Santiago, ChileReviewed by:
Holly Shiels, The University of Manchester, United KingdomFrédéric Silvestre, University of Namur, Belgium
Copyright © 2023 Piferrer and Anastasiadi. This is an open-access article distributed under the terms of the Creative Commons Attribution License (CC BY). The use, distribution or reproduction in other forums is permitted, provided the original author(s) and the copyright owner(s) are credited and that the original publication in this journal is cited, in accordance with accepted academic practice. No use, distribution or reproduction is permitted which does not comply with these terms.
*Correspondence: Francesc Piferrer, piferrer@icm.csic.es