- 1Departamento de Zoología, Genética y Antropología Física, Facultad de Veterinaria, Universidade de Santiago de Compostela, Lugo, Spain
- 2Departamento de Pesca e Aquicultura, Universidade Federal Rural de Pernambuco, Recife, Brazil
- 3Centro de Investigacións Mariñas (CIMA), Consellería do Mar da Xunta de Galicia, Vilanova de Arousa, Spain
- 4Departamento de Ciencias de la Vida, Universidad de Alcalá, Alcalá de Henares, Spain
- 5Research Centre for Experimental Marine Biology and Biotechnology (PIE), University of the Basque Country (UPV/EHU), Plentzia, Spain
The common cockle (Cerastoderma edule) plays an important role in marine ecosystems and represents a valuable socioeconomic resource for coastal communities. In 2012, the cockle beds from Rı́a de Arousa (Galicia, NW Spain) were seriously decimated by the protozoan Marteilia cochillia responsible for marteiliosis. We aimed to identify single nucleotide polymorphisms (SNP) markers potentially associated with resilience to marteiliosis to be used in marker-assisted selection programs for restoring affected cockle beds and recovering their production. For this, we carried out a population genomics approach using 2b-RADseq, where 38 naive samples (before the first detection of M. cochillia in 2012) from two beds of Rı́a de Arousa were compared with 39 affected samples collected in 2018/2019 (after several years of marteiliosis occurring in the area), collected either before (15 non-exposed samples) or during (24 exposed samples) the marteiliosis outbreak. Additionally, 767 differentially expressed genes (DEG) from a previous transcriptomic study addressed during the aforementioned 2018/19 marteiliosis outbreak, were evaluated to identify SNPs showing signals of selection. Using 2b-RADseq, 9,154 SNPs were genotyped and among them, 110 consistent outliers for divergent selection were identified. This set of SNPs was able to discriminate the samples according to their marteiliosis status (naive vs affected; exposed vs non-exposed), while another 123 SNPs were identified linked to DEGs associated with the level of infection across a temporal series. Finally, combining the population genomics and transcriptomics information, we selected the 60 most reliable SNPs associated with marteiliosis resilience. These SNPs were close to or within DEGs, and many of them were related to immune response (phagocytosis and cell adhesion), defence, such as apoptosis, stress, and cellular cycle, among other functions. This set of SNPs will eventually be validated to develop a cost-effective genotyping tool for their application for obtaining cockle-resilient strains for marteiliosis.
1 Introduction
The common cockle (Cerastoderma edule) is a marine bivalve species buried just below the surface of the sand or mud in intertidal and shallow subtidal areas of estuarine and marine coastal waters. It is widely distributed in the Northeast Atlantic, from the west coast of Africa in Senegal to the Barents Sea in Norway (Hayward and Ryland, 1995; Tyler-Walters, 2007). C. edule lives on average 2-4 years but can reach up to 10 years (Ponsero et al., 2009). It is a dioecious species that reaches sexual maturity at about one year of age (Maia et al., 2021). Like most bivalves, the common cockle releases gametes into the water, where external fertilization takes place (Moreira Sanmartín et al., 2016), and larvae remain in the water column for about 30 days (Creek, 1960), which allows their dispersal by marine currents (more than 100 km; Coscia et al., 2020). The spawning season runs from March to October, reaching the peak of activity between July and September when the water temperature is higher (~20°C; Maia et al., 2021).
Cockles are a highly valued shellfish species due to the range of ecosystem services that provide, e.g., the ability to reshape the seabed and alter the sediment properties (Ciutat et al., 2006; Neumeier et al., 2006; Andersen et al., 2010) depending on the substrate type (Rakotomalala et al., 2015; Eriksson et al., 2017). Like other bivalves, cockles contribute to improve water quality as filter feeders (Carmichael et al., 2012; van der Schatte Olivier et al., 2020) and aid to maintain biodiversity either indirectly, increasing the production of microphytobenthos (Swanberg, 1991) or as a direct source of food for different species (Sutherland, 1982; Beukema and Dekker, 2005). Furthermore, cockles are largely appreciated as a feeding resource for humans; Europe reported captures of 24,237 tonnes of C. edule in 2019, with Denmark, UK, Spain, and Portugal being the main producers (Food and Agriculture Organization of the United Nations [FAO], 2021). However, inter-annual production is unstable depending on different biotic and abiotic factors such as bacterial, viral, and parasitic infections (Lauckner, 1983; Bower et al., 1994), predation (Sutherland, 1982; Mascaró and Seed, 2000; Beukema and Dekker, 2005), food limitation (Bos et al., 2006), over-exploitation (Ferns et al., 2000), and environmental changes such as water temperature, salinity, pollution and in recent years climate change (Møhlenberg and Kiørboe, 1983; Ducrotoy et al., 1991; Beukema and Dekker, 2005; Parada and Molares, 2008; Burdon et al., 2014; Rowley et al., 2014).
Galicia (NW Spain) holds the most productive shellfisheries in Spain (Subsecretaría Subdirección General de Análisis Coordinacion y Estadística, 2020). In 2012, massive cockle losses were recorded in the Ría de Arousa, which houses one of the most productive shellfisheries on the Galician coast, associated with the protozoan parasite Marteilia cochillia (Villalba et al., 2014). In the following years, marteiliosis outbreaks spread to the southern Rías of Pontevedra and Vigo, almost depleting cockle production in the area. An annual pattern outbreak was recorded since the first 2012 outbreak; cases of infection are detected in newly recruited individuals in summer/early fall, followed by a progressive increase of prevalence and mortality until reaching almost 100% of cumulative mortality in the next months (Iglesias et al., 2023).
The parasite M. cochillia is a protistan parasite that colonizes the epithelium of the digestive gland of cockles destroying digestive diverticula and causing death due to starvation (Montaudouin et al., 2021). The complete life cycle of M. cochillia has not been yet disclosed. Darriba et al. (2020) observed parasitic forms (sporangia) being released through faeces into the environment. Intermediary hosts are hypothesized for its transmission, similarly to what is suspected to occur in the flat oyster (Ostrea edulis) infected by M. refrigens, where copepods of the genus Paracartia, i.e., P. grani, are involved (Audemard et al., 2002; Carrasco et al., 2015; Carballal et al., 2019). In this natural scenario, generating effective preventive measures against parasite infection is complex. Increasing resilience against M. cochillia through breeding programmes is an appealing approach to diminish the impact of the parasite in cockle beds, as has been demonstrated before in other bivalves (Ford and Haskin, 1987; Ragone Calvo et al., 2003; Kube et al., 2011; Proestou et al., 2016; Smits et al., 2020). This approach has been tested in natural environments, e.g. the breeding program for Saccostrea glomerata to obtain strains resistant to winter mortality and Qx disease caused by Bonamia roughleyi and Marteilia sydneyi, respectively (Nell et al., 2000), and in controlled conditions, such as the increased survival to ostreid herpesvirus 1 (OsHV-1) in Crassostrea gigas (up to 61.8%) after four generations of selection (Dégremont et al., 2015). On average, response to disease resistance selection in molluscs was higher than any other traits, such as growth (15% vs 10% per generation; see review of Hollenbeck and Johnston, 2018).
Genomic strategies are essential to understand the genetic basis of host-parasite interaction, for controlling marteiliosis and, eventually, for its application in breeding programs. For example, in Crassostrea gigas genomic prediction of OsHV-1 resistance was more accurate (around 19%) than family-based prediction (Gutierrez et al., 2020). Genomic resources of common cockle have recently increased in the framework of the COCKLES Interreg (EAPA_458/2016) and the Scuba Cancers (ERC-2016-STG) projects, which ensured a robust genetic baseline for that purpose. A population genomics approach using 2b-RADseq along with the chromosome-level genome assembly of the species (Bruzos et al., 2022) was applied to disentangle the demographic and environmental factors underlying the common cockle structure in the Northeast Atlantic (Vera et al., 2022). Furthermore, RNAseq was applied to identify differentially expressed genes (DEG) in the digestive gland across the different infection stages. In this study, 767 DEGs, among the ~ 9000 annotated in the cockle’s transcriptome, were identified when comparing samples of different infection levels across the outbreak 2018/19, many of which related to key immune pathways (Pardo et al., 2022).
The main goal of our study was to identify SNPs (Single Nucleotide Polymorphism), associated with genomic regions related to marteiliosis resilience in common cockle from Ría de Arousa for their eventual application in breeding programs and management of cockle beds. For this purpose, we followed two complementary approaches: i) identification of SNPs associated with divergent selection using groups of samples subjected to differential parasite pressure, and ii) detection of SNPs linked to the DEGs detected in response to M. cochillia outbreak by Pardo et al. (2022) showing significant genetic differentiation across groups with different level of infection. We used 2b-RAD and RNAseq data for genotyping anonymous and gene-linked SNPs, respectively, and further, we explored their involvement in the immune response that could explain the resilience to the parasite. A set of the most consistent SNPs were included thinking on its future validation for their potential application in breeding programs and management of common cockle beds.
2 Materials and methods
2.1 Population genomics approach
2.1.1 Sampling and DNA extraction
The sampling sites analysed in this study were selected according to relevant information on cockle marteiliosis epidemics. Marteiliosis was first detected in cockles from Galicia (NW Spain), namely from Ría de Arousa, in 2012 (Villalba et al., 2014); since then, outbreaks were recorded in this ría starting every summer/early fall, affecting each newly recruited annual cohort, and causing mass mortality (Iglesias et al., 2023). According to this information and the goals of the study, a total of 79 individuals were collected from two shellfish beds of Ría de Arousa (Table 1; Figure 1): i) 40 individuals in January 2012 before the first detection of M. cochillia (naive samples: NS) from Lombos do Ulla (SLO12) and O Sarrido (SSA12); and ii) 39 individuals from Lombos do Ulla in the 2018/19 period, after several generations of marteiliosis pressure (“affected”- samples, AS); among these, 15 juveniles were collected in spring 2018, before the annual marteiliosis outbreak (SLO18; non-exposed samples: NES), and 24 cockles mostly from a single cohort in spring 2019 (only four samples from September 2018) during the 2018/19 marteiliosis outbreak (SLO19; exposed samples: ES). A small portion of the gills was extracted from each individual and stored in 100% ethanol at 4°C. Genomic DNA was extracted from gills using the e.Z.N.A. E-96 mollusc DNA kit (OMEGA Bio-tech), following the manufacturer’s recommendations. The quality and quantity of DNA were assessed with NanoDrop® ND-1000 (Nanodrop Technologies).
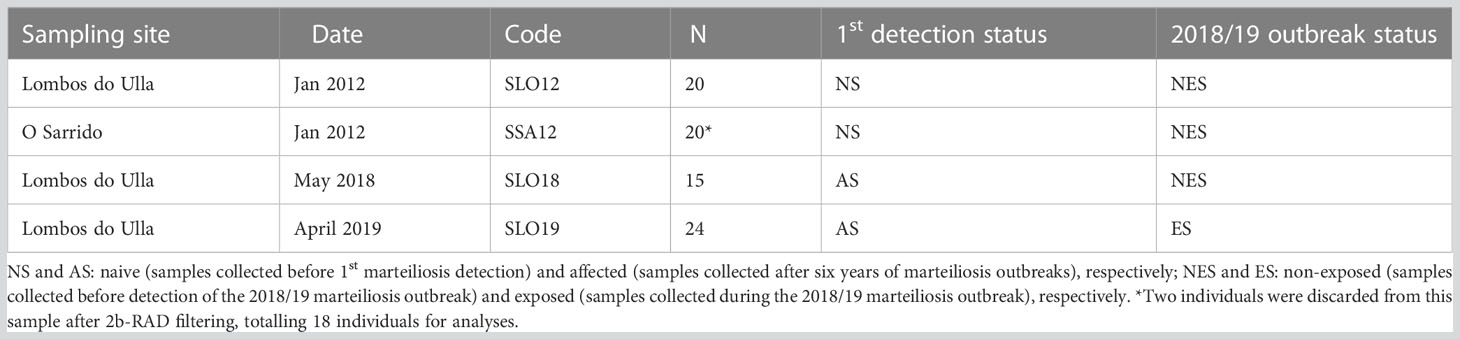
Table 1 Cerastoderma edule samples analysed from Ría de Arousa for the population genomics approach.
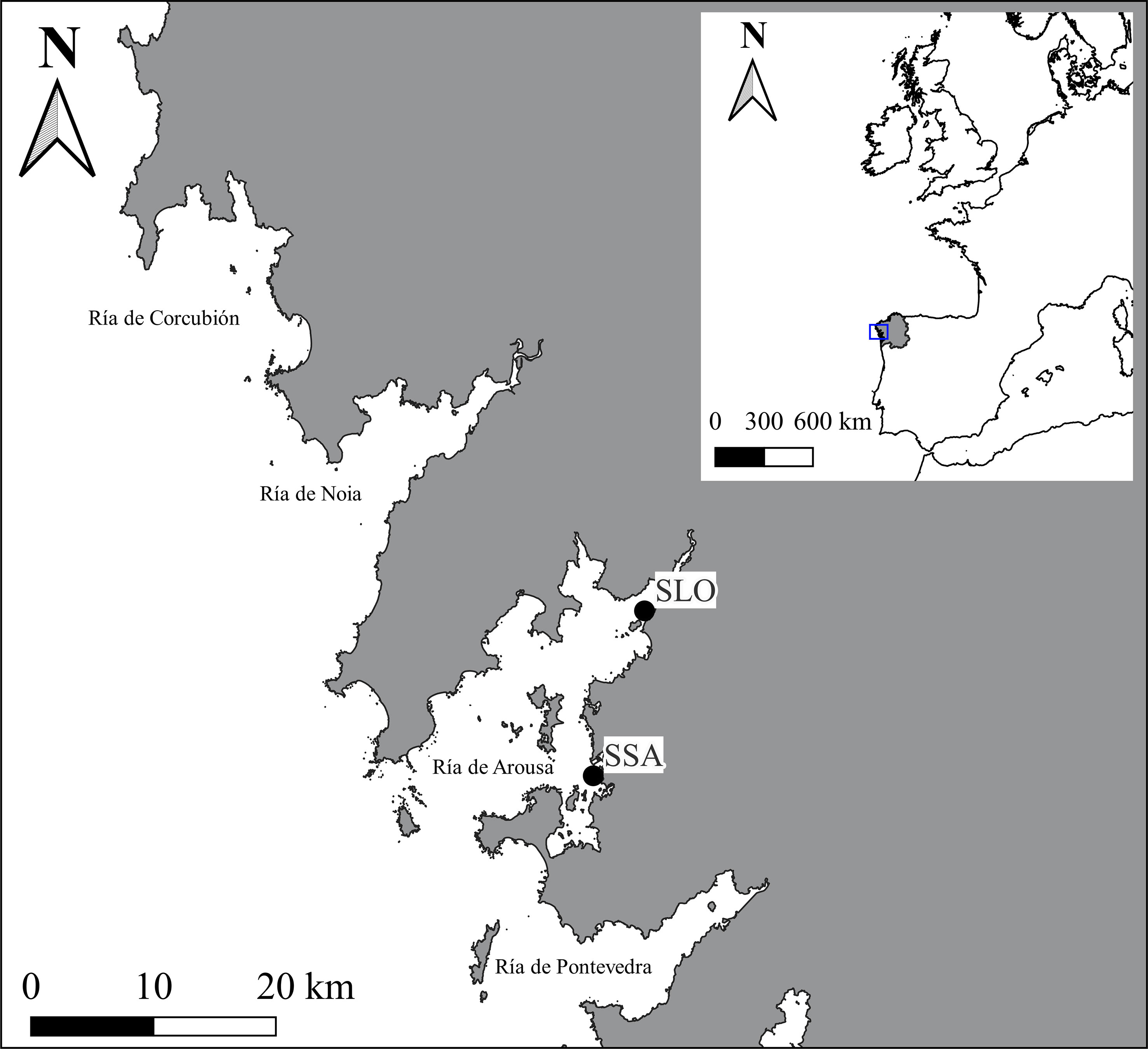
Figure 1 Study area showing the two sampled Cerastoderma edule beds in the Ría de Arousa (Galicia, NW Spain). Geographic Coordinate System – EPSG:25829 − SLO: Lombos do Ulla (518449 - 4719641); SSA: O Sarrido (514263 – 4706171).
2.1.2 SNP calling and genotyping
Genotyping was performed following the 2b-RAD genotyping-by-sequencing (GBS) protocol (Wang et al., 2012). In brief, we obtained millions of 36 bp fragments in each sample produced by the digestion of genomic DNA with the AlfI IIb restriction enzyme (RE) (Thermo Fisher), which cut DNA at both sides of the RE site. 2b-RAD libraries were constructed at the Genomics Platform of Universidad de Santiago de Compostela (USC) and delivered to the FISABIO Platform (Valencia, Spain) for sequencing in a NextSeq 500 sequencer (Illumina). Then, reads from each individual were aligned to the common cockle genome (Bruzos et al., 2022) using Bowtie 1.1.2 (Langmead et al., 2009), and SNP calling was performed with Stacks 2.0 (Catchen et al., 2013; Rochette et al., 2019), following the parameters described by Vera et al. (2022). The RAD-tag SNP panel reported by Vera et al. (2022) mapped in the common cockle genome (Bruzos et al., 2022) was used as reference for genotyping to make feasible comparison with previous studies. Finally, some SNPs/RAD-tags or individuals were removed from the data using Plink 1.9 (Purcell et al., 2007) according to the following criteria: i) SNPs deviated from Hardy-Weinberg proportions (p < 0.05) in at least two sampling sites; ii) RAD-tags with more than 3 SNPs; iii) SNPs with missing data in > 50% individuals, and iv) individuals with < 30% of the SNP panel genotyped in any of the sampling sites.
2.1.3 Genetic diversity
The different subsets of SNPs used for analyses were extracted in Genepop format using the R package GENEPOPEDIT 1.0 package (Stanley et al., 2017). Allelic richness (Ar), observed (Ho) and expected (He) heterozygosity, and intrapopulation fixation index (FIS) were calculated for each sample site to assess genetic diversity with the R package DiveRsity 1.9 (Keenan et al., 2013) (function “divBasic”) with 1000 bootstraps. Departure from Hardy-Weinberg equilibrium (HWE) was estimated with exact tests using the enumeration method with GENEPOP 4.7.5 (Rousset, 2008).
2.1.4 Divergent selection for marteiliosis: Outlier detection
Two statistical approaches were performed to detect consistent outlier loci related to divergent selection against the neutral genomic background: i) the Bayesian FST-based method implemented in BAYESCAN v2.01 (Foll & Gaggiotti, 2008) was run using default parameters (i.e., 20 pilot runs; prior odds value of 10; burn-in of 50,000), 100,000 iterations and a sample size of 5,000; ii) the FDIST FST method implemented in ARLEQUIN v3.5 (Excoffier and Lischer, 2010) which uses a maximum likelihood approach (Beaumont & Nichols, 1996) was applied to incorporate a priori information regarding population structure with 100,000 simulations and 100 demes. For this purpose, we considered two scenarios where significant changes at specific genomic regions could hypothetically occur as a consequence of the differential selective marteiliosis pressure regarding the neutral background (Table 1): i) a temporal criterion (2012 vs 2018/19 outbreak), where naive samples (NS: SLO12, SSA12) constituted one group and affected samples (AS: SLO2018, SLO19) another; and ii) an exposure criterion, where samples from non-exposed cockles (NES: SLO12, SSA12 and SLO18) were grouped and compared to exposed samples (ES: SLO19). We used more strict parameters for ARLEQUIN (three technical replicates at p < 0.01), since this approach is more prone to false positives and a standard p < 0.05 for BAYESCAN, since it follows a more conservative approach (Narum and Hess, 2011).
All outliers detected were mapped on the C. edule genome (Bruzos et al., 2022), and those close to DEGs or another outlier (± 250 kb) were considered the most consistent ones (Population Genomic Candidates - PGCAND). Minor allele frequency (MAF) obtained with R package “adegenet” (Jombart, 2008) using “minorAllele” and “tab” functions respectively were calculated for further filtering steps.
2.1.5 Population structure
Global and pairwise relative coefficients of genetic differentiation (FST) were calculated between cockle sampling sites with GENEPOP 4.7.5 and R package StAMPP 1.6.2 with the ‘stamppFst’ function (Pembleton et al., 2013) using 10,000 bootstraps to calculate 95% confidence interval to test the null hypothesis (FST = 0). Genetic structure was additionally investigated through STRUCTURE 2.3.4 (Pritchard et al., 2000) using the R package ParallelStructure 1.0 (Besnier and Glover, 2013) to identify the most likely number of population units (K) in the samples. This program uses a Bayesian clustering approach to explore the population genetic units (clusters) using genotyping data. The program assigns the proportion of the genome that belongs to each of the clusters identified in each individual. Tests were performed without a priori information regarding the origin of samples, using an admixture model with correlated allele frequencies and burn-in of 100,000 iterations and 200,000 Markov Chain Monte Carlo steps. The number of K tested ranged from 1 to 5 (the number of sampling sites +1). For each K, ten replicates were performed to increase statistical confidence. The optimal number of K was estimated using the website program StructureSelector (Li and Liu, 2018) using different approaches: deltaK (Evanno et al., 2005), Mean LnP (K) (Pritchard et al., 2000) and those published by Puechmaille (2016). Graphical outputs were obtained with CLUMPAK (Kopelman et al., 2015). Further, a Discriminant Analysis Principal Component Analysis (DAPC) was performed with the R package adegenet to complement the STRUCTURE analysis. First, “find.cluster” function was used to assess the number of clusters in the population determining the optimal number of subpopulations with the Bayesian Information Criterion (BIC). Then, a cross-validation function was performed to detect the best number of principal components (PCs) given by the smallest mean square error (RMSE).
2.2 Transcriptomics approach
Pardo et al. (2022) identified 767 differentially expressed genes (DEG) in cockles collected before (July 2018) and at three different times (November 2018, April 2019 and July 2019) during a natural outbreak of M. cochillia in 2018/2019 in Lombos do Ulla. Samples were classified histologically according to the level of infection as non-infected, mild, moderate and heavily infected. Then, DEGs were detected across a temporal series and according to the level of infection during the 2018/19 outbreak in Ría de Arousa. RNAseq data from these 767 differentially expressed genes (DEG) was used to call associated SNPs and estimate allele frequencies in each sample using SAMtools 1.9 (Li et al., 2009) with the following parameters: –skip-indels, –adjust-MQ 0, –max-depth 250. Then, SNPs were investigated for their association with the level of infection in exposed samples taken at three different times during a parasite outbreak (T1, T2 and T3). Samples were classified and pooled according to their level of infection across time using histopathology (Iglesias et al., 2023): I0: non-infected; I1: early infection; I2: moderate infection; I3: heavy infection; I4: final infection stage (Table 2). Allele frequency, missing data, expected heterozygosity and minimum allele frequency (MAF) were estimated per locus from the VCF file using all exposed samples (N = 50). SNPs that fitted the cut-off criteria of MAF > 0.05 and missing data < 30% were selected and mapped into the cockle’s genome (Bruzos et al., 2022). Finally, the highest polymorphic SNP with the lowest missing data per DEG was chosen among those available.
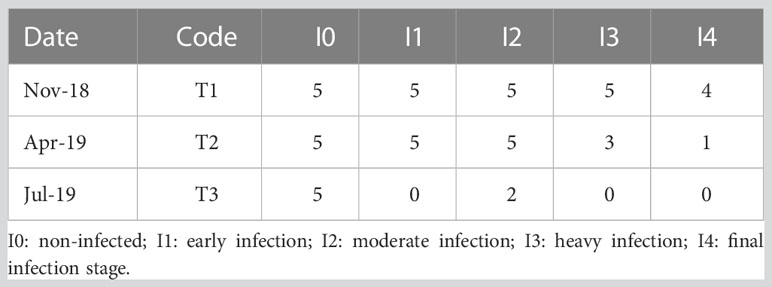
Table 2 Cerastoderma edule samples collected in Lombos do Ulla used for the transcriptomics approach classified by infection level.
Assuming the presence of genetic variation at DEGs related to marteiliosis response in Lombos do Ulla samples, we hypothesized that if divergent selection was occurring due to selective pressure, associated SNPs would show genetic differentiation between samples according to their level of infection, to say, on average exposed but non-infected samples would carry allelic variants related to resilience at a higher frequency, while heavily infected ones, would do for susceptibility variants. To increase statistical power, exposed samples were grouped into three sets according to their infection level: i) non-infected (15 individuals); ii) early/moderately infected (22 individuals), and iii) heavily infected/final stage of infection (13 individuals). Global FST was estimated for all selected SNPs using those three groups and their significance was estimated with exact tests (p < 0.05) using Genepop 4.7.5. When possible, the two most polymorphic SNPs were retrieved per DEG. Candidate SNPs were finally selected from the transcriptome approach (TCAND) from those showing the highest genetic differentiation (p < 0.05).
2.3 Final selection SNP panel
Once candidates from the population genomics approach (PGCAND) and transcriptomics (TCAND) were identified, a final set of SNPs was selected to design a cost-effective molecular tool including ~60 SNPs to be eventually used for obtaining resilient strains to marteiliosis. Significant SNPs from both approaches were first filtered by missing data < 30% and MAF > 0.05 and then by technical issues related to primer design and multiplexing. This preselected SNP set was next sorted according to the statistical confidence to be under divergent selection (p-value) and further prioritize for consistency using the following criteria: i) signals of selection in more than one approach (i.e., temporal and exposition among PGCAND); ii) more than one SNP detected in less than 250 kb; and iii) higher genetic diversity and lower missing data. Finally, when more than one outlier was found in the same gene or region (± 250 kb), only one of the markers was selected.
3 Results
3.1 Population genomics approach
Genetic diversity and structure were investigated with different sets of SNPs on several groups of samples related to the strategies followed to identify the most reliable set of outlier markers associated with divergent selection against marteiliosis (PGCAND outliers). Analyses were performed with i) the whole polymorphic SNP dataset; ii) the divergent outlier dataset; and iii) the neutral dataset, defined after removing outliers from the whole data.
3.1.1 Outlier detection and mapping
After filtering, 9,154 SNPs were retained (Table S1) from the SNP panel reported by Vera et al. (2022), and among them, 6,252 SNPs (68.3%) were polymorphic in our collection. The detection of outlier markers was performed using the 6,252 polymorphic SNPs, representing 1.6 SNPs/Mb according to the common cockle genome size (794 Mb; Bruzos et al., 2022), under the null hypothesis of neutrality across the whole genome. Thus, outliers with FST significantly above the neutral background were considered under divergent selection, while those with FST below the neutral background were considered under stabilizing selection. The two statistical methods implemented in BAYESCAN and ARLEQUIN programs, respectively, were applied and tested in the temporal (naive vs affected) and exposure (non-exposed vs exposed) scenarios (see Materials and Methods). BAYESCAN, the most conservative and sensitive to sampling error method, only detected one outlier under divergent selection in the temporal scenario, while ARLEQUIN detected a total of 213 consistent outliers (p < 0.01 in three technical replicates), 74 in the temporal (t) and 156 in the exposure (E) scenarios, respectively, 17 of them shared in both scenarios, including the one detected by BAYESCAN (Table S2). No outliers under stabilizing selection were detected with any of both methods. All the 213 consistent divergent outliers were mapped in the C. edule genome and additionally checked for their proximity to other outliers (< 250 kb) (Table 3) or to any of the 767 DEGs reported by Pardo et al. (2022) (Table S3). A total of 110 SNPs (10 shared between both scenarios) met the criteria and were selected as the most reliable set of SNPs from the population genomics approach (PGCAND).
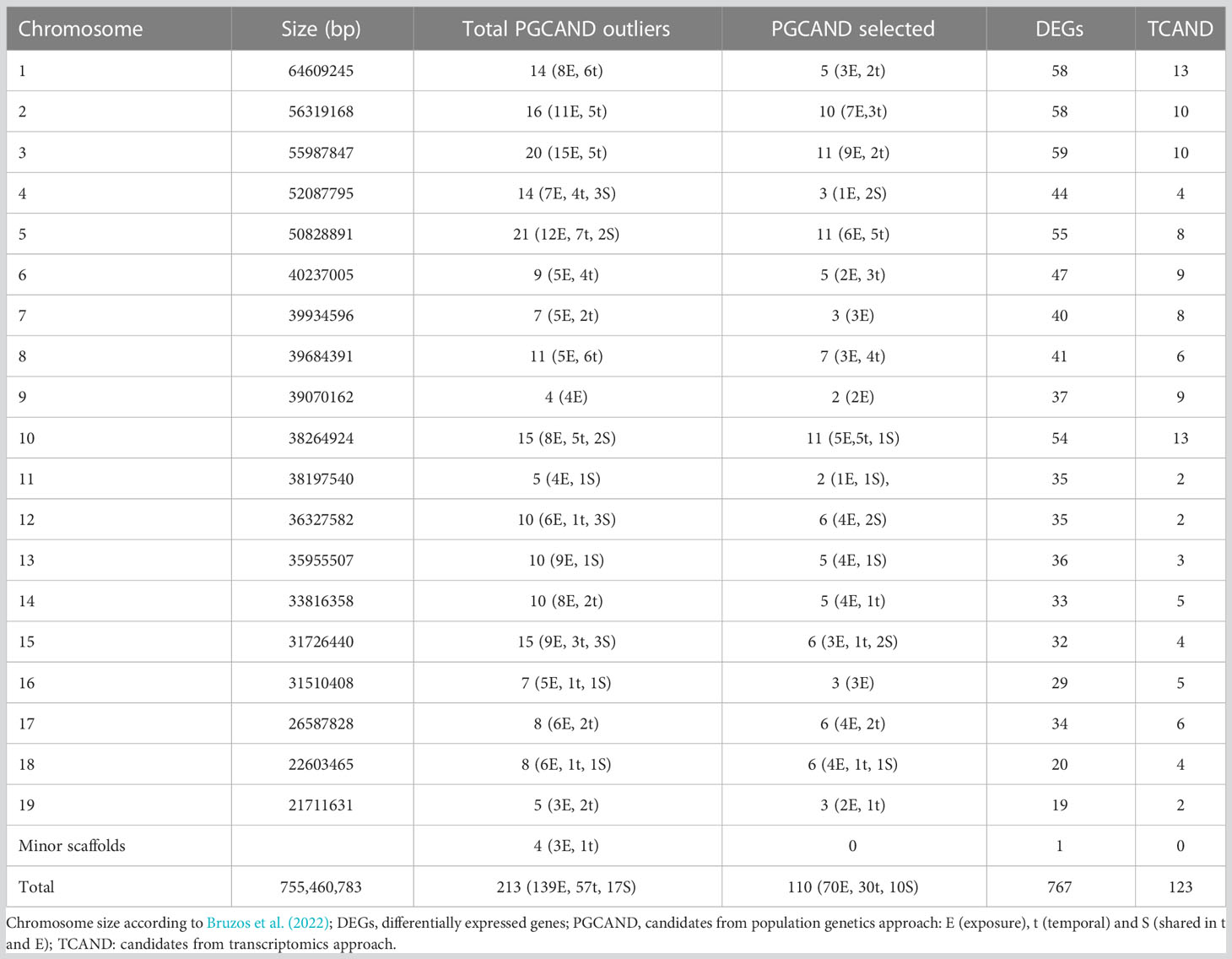
Table 3 Genomic location of consistent SNPs detected in Cerastoderma edule following population genomics and transcriptomics approaches along with the differentially expressed genes reported by Pardo et al. (2022).
3.1.2 Genetic diversity
Genetic diversity using the whole 9,154 SNP dataset was slightly but significantly higher (p < 0.05) in samples from 2012 as compared to those from the 2018-19 period both for allelic richness (Ar: 1.362 vs 1.321) and expected heterozygosity (He: 0.085 vs 0.077). None of the samples showed global deviation from HWE (p > 0.05), although there was a significant deficit of heterozygotes in most samples using the confidence interval approach (Table 4A). Results were very similar when considering only the neutral SNPs (data not shown). However, when using the 110 PGCAND outlier panel (Table 4B), genetic diversity was lower in non-exposed vs exposed samples (NES vs ES: Ar: 1.330 vs 1.672, He: 0.100 vs 0.169, respectively; p < 0.05, Mann-Whitney tests) and further, a highly significant departure from HWE involving a remarkable heterozygote deficit was detected in the exposed sample (SLO19 FIS = 0.364; p < 0.001). Interestingly, the high value detected in the exposed sample was mainly due to the 156 outliers of the exposure scenario (FIS = 0.456; p < 0.001), while it remained significant but very similar across the four populations when the 74 outliers of the temporal scenario were compared (FIS SLO12: 0.293; SSA12: 0.307; SLO18: 0.287 and SLO19: 0.290).
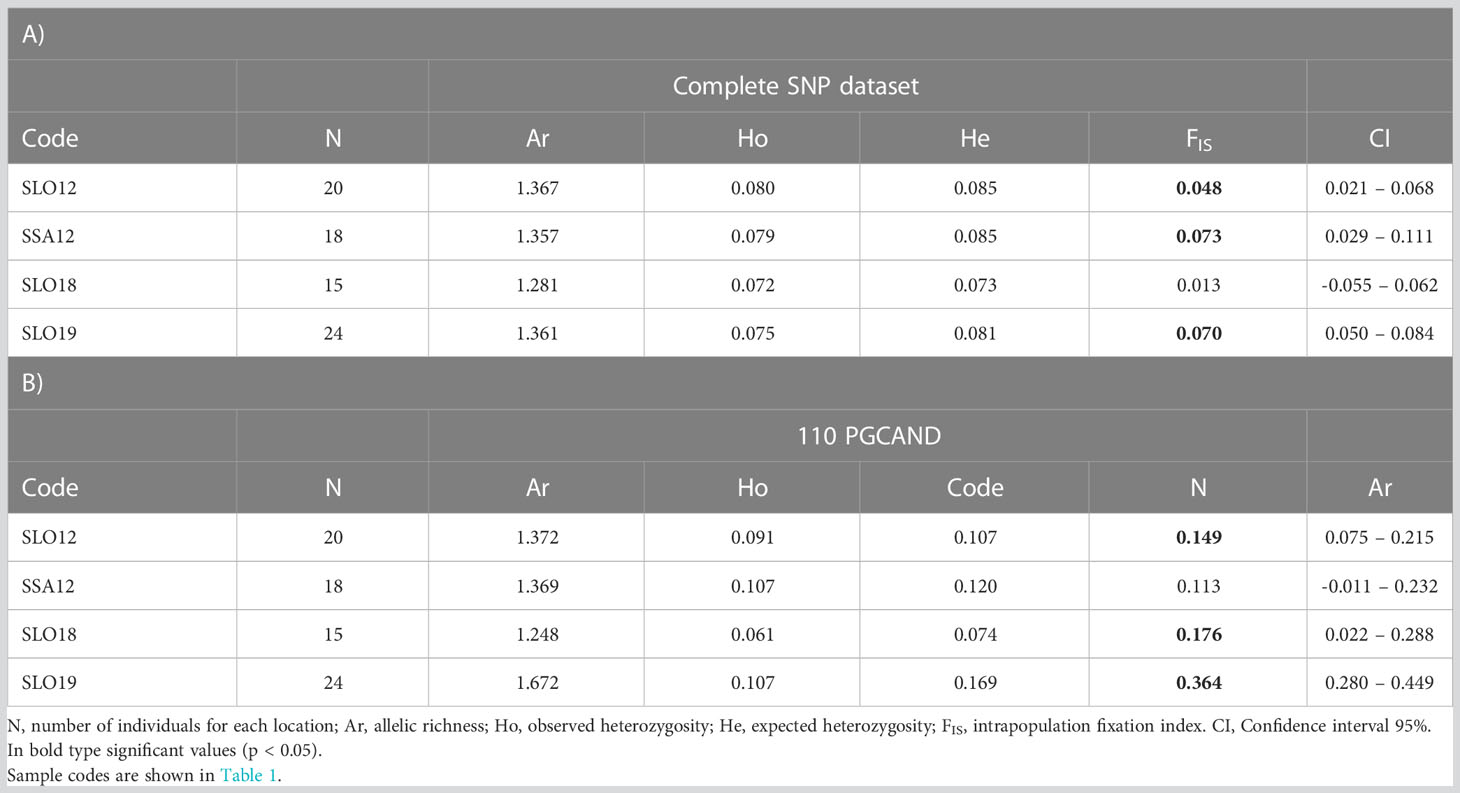
Table 4 Genetic diversity in Cerastoderma edule samples from Ría de Arousa with: A) Whole dataset (9154 SNPs); B)110 PGCAND dataset.
3.1.3 Genetic structure and differentiation
The global FST = 0.0032 showed low but significant (p < 0.01) genetic differentiation with the whole SNP dataset. Pairwise FST comparisons showed low and usually non-significant differentiation when using the neutral panel (6,006 SNPs), but highly significant differentiation when using the 110 most consistent outlier SNPs, especially when comparing naive (2012) and affected (2018/19) groups (average FST = 0.091), but also between non-exposed and exposed samples in the 2018/19 outbreak (FST = 0.033; Table 5).
The clustering method of STRUCTURE applied to the neutral dataset showed K = 1 as the optimal number of clusters according to all K estimators described by (Puechmaille, 2016) and K = 2 with ΔK and LnP (K) (Figure 2). Nevertheless, the second population unit would be spurious according to the criterion defined by Puechmaille (2016). Results with 110 PGCAND yielded different optimal Ks depending on the estimator used. According to LnP (K) (Pritchard et al., 2000), seven distinct groups were detected, while Puechamaille’s estimators and ΔK reported optimal K = 3 differentiating naive (NS: SLO12/SSA12), non-exposed (NES: SL18) and exposed (ES: SLO19) samples. DAPC analyses yielded the lowest Bayesian information criterion for K = 1 with the neutral panel, and K = 2 (BIC K = 2; 161.754) with the 110 PGCAND, but K = 3 (162.722) rendered a slightly lower value showing a sample differentiation similar to that by STRUCTURE (Figure 3). All in all, two or three groups were identified, respectively, in the exposure (2 groups) and temporal (3 groups) scenarios, the non-exposed group (SLO18) being in-between the two more differentiated naive (SLO12, SSA12) and the exposed (SLO19) groups (Figure 3B).
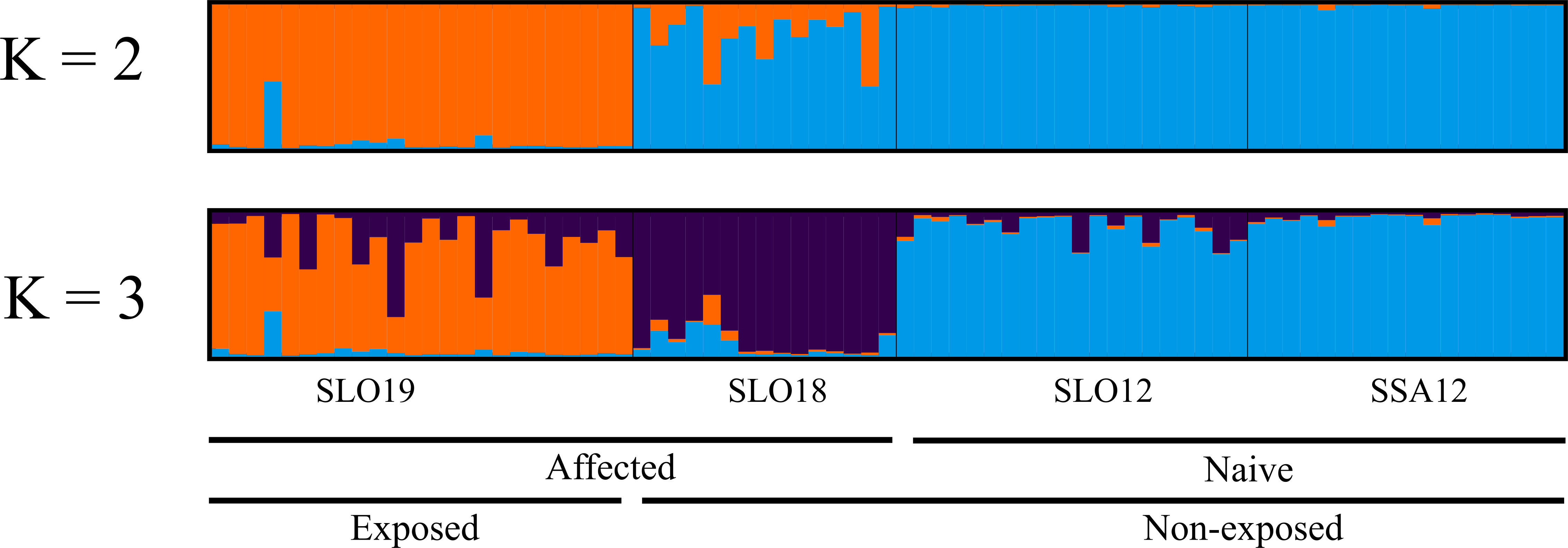
Figure 2 Population structure of Cerastoderma edule beds analysed with STRUCTURE with 110 PCAND dataset for K = 2 and K= 3). Each individual is represented by a vertical bar and its colour is proportional to the posterior probability assigned to each cluster.
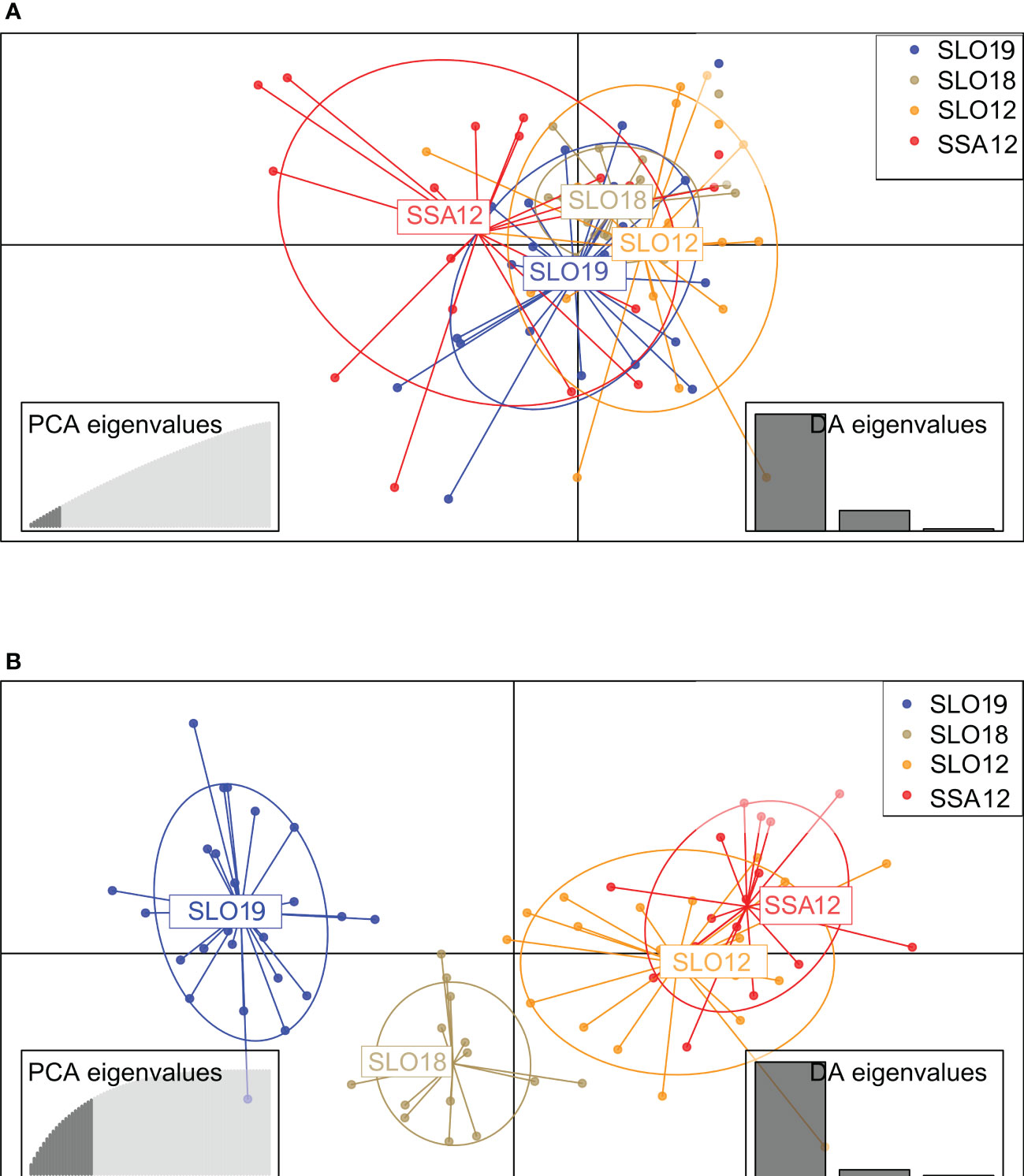
Figure 3 Discriminant Analysis of Principal Components (DAPC) plots of cockles Cerastoderma edule using: (A) neutral dataset (10 PCs; 18% of variance explained); (B) 110 PCAND dataset DAPC (20 PCs, 72% of explained variance). Codes are shown in Table 1. PCs retained according to the cross-validation method with the lowest RMSE are shown at the left bottom of each panel.
3.2 Transcriptomics approach
More than five million SNPs were initially identified from the RNAseq reads aligned against the common cockle reference genome, which were reduced to > 950,000 high-quality SNPs after filtering with SAMtools. Among them, ~ 45,000 SNPs were inside the 767 DEGs reported by Pardo et al. (2022). After filtered by MAF (> 0.05) and missing data (< 30%), a total of 12,753 SNPs were obtained. Two SNPs with the highest MAF and the lowest missing data were retained per DEG (when available), thus constituting a total of 1,418 SNPs more manageable dataset (Tables S3, S4). Among the three groups of samples classified according to the level of infection (I0: no infection, I1: early/moderate, I3: heavy/final), 123 SNPs showed significant genetic differentiation (p FST < 0.05) and constituted the set of candidate markers from the transcriptomics approach (TCAND) (Tables 5, S5). Among them, 41 SNPs showed a progressive increase (or decrease) in the frequency of the reference allele across the three infection level groups (Table S5). Chromosomes (C) C1 and C10 housed the highest number of candidates (13 SNPs) (Table 3).

Table 5 Pairwise FST matrix for Cerastoderma edule samples collected in 2012 (NS: naive) and in the 2018/19 period (AS: affected) in the Ría de Arousa, Galicia (NW Spain).
3.3 Final selection SNP panel
A final marker selection was made with the most suitable SNPs from the 110 PGCANDs and the 123 TCANDs to constitute a set of markers to be further validated by their technical and marteilioisis diagnosis usefulness (Table S6). PGCAND and TCAND were first preselected by MAF (> 0.05), missing data (< 30%) and technical criteria (± 100 bp flanking regions lacking additional polymorphisms), yielding a final set of 44 PGCAND and 38 TCAND. Then, they were ranked from the lowest to the highest genetic differentiation p-value for selection and additionally filtered to retain only one marker per genomic window (± 250 kb), and those with the highest FST and lowest missing data when more than one was available. A final panel of 60 SNPs was considered as a suitable set to define a cost-effective tool for validation (36 from PGCAND and 24 from TCAND approaches, respectively). These SNPs were placed in the cockle genome (Table 6). Chromosomes C5 and C10 housed the higher number of SNPs (5 and 8 SNPs respectively). Selected markers were related to catalytic functions and binding activities (17), such as the cathepsin family L, calmodulin, IgGFc-binding protein gene, Golgin subfamily A member, glutathione S-transferase sigma or proteasome related genes. Many of these genes have also been related to immune response (phagocytosis and cell adhesion), and defense, such as apoptosis, stress, and cellular cycle, among other functions (Table 6) (Niu et al., 2013; Nanut et al., 2014; Vigneron and Van den Eynde, 2014; Han et al., 2021).
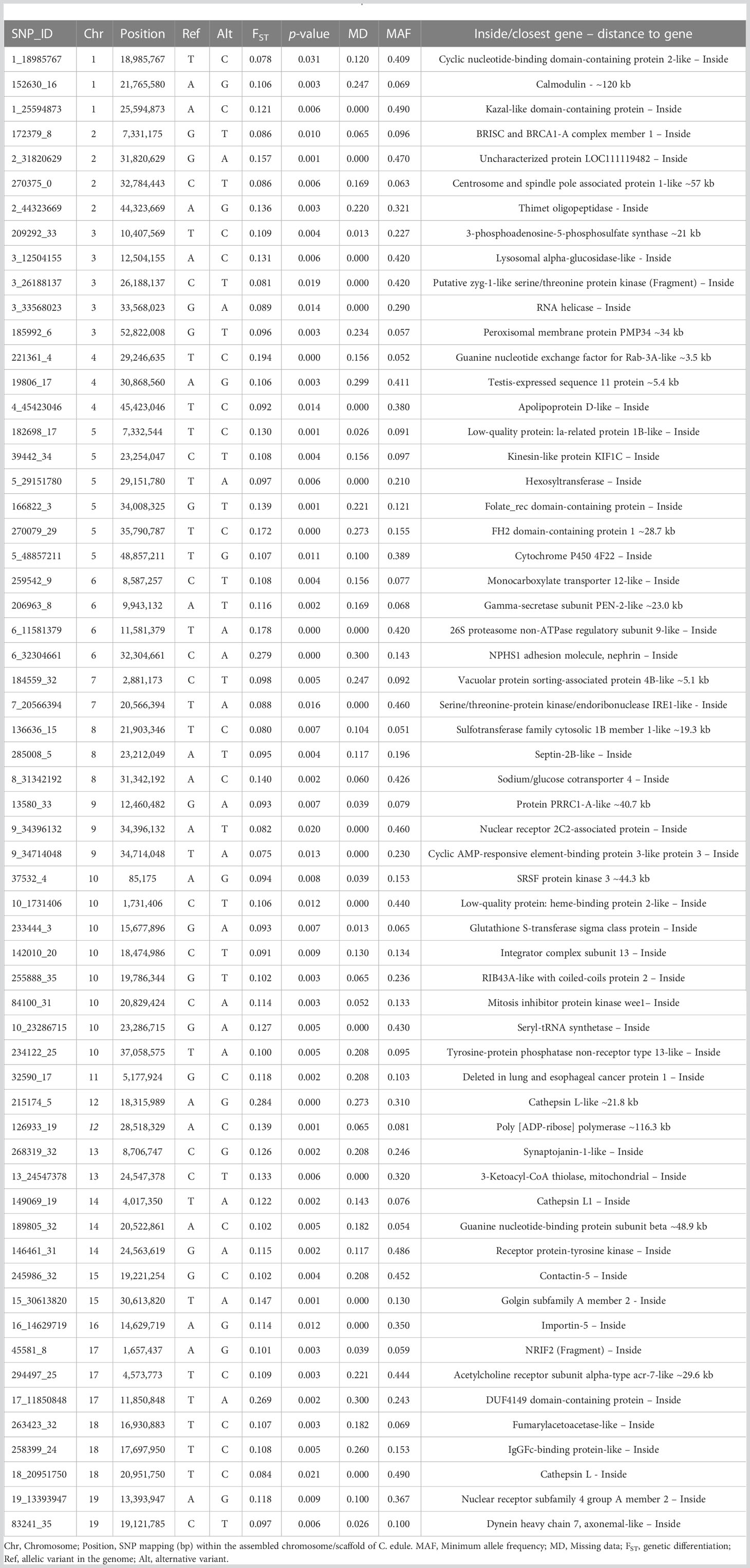
Table 6 List of the 60 SNPs selected from transcriptomic (TCAND) and population genomics (PGCAND) approaches positioned in the cockle Cerastoderma edule genome selected for resilience to Marteilia cochillia.
4 Discussion
Cockle beds in Ría de Arousa experience annual outbreaks since 2012 (Villalba et al., 2014; Iglesias et al. 2023) due to the parasite Marteilia cochillia, which has collapsed its shellfishery. Although the parasite has only been recorded in restricted areas, namely Rías de Arousa, Pontevedra and Vigo in Galicia (Northwest Atlantic coast of Spain), Fangar and Alfacs bay in Catalonia (Northeast Mediterranean coast of Spain); Huelva (Southwest Atlantic coast of Spain); and Ría de Aveiro and Formosa Ras (North and South of Portugal, respectively) (Carrasco et al., 2013; Navas et al., 2018; Montaudouin et al., 2021; Iglesias et al., 2023), its presence threatens cockle production regardless that larval dispersal and connectivity between beds (Vera et al., 2022) could be aiding to recover recruitment every year (Iglesias et al. 2023).
Prevalence of marteiliosis and mortality of cockles have decreased since 2017 in Lombos do Ulla (Iglesias et al., 2023) and furthermore, batches from the naive shellfish bed of Noia (Ría de Noia, just north of Ría de Arousa) transplanted into Lombos do Ulla in 2017 and 2018 experienced much higher marteiliosis prevalence and mortality than those recruited in Lombos do Ulla during the same season. These observations led to hypothesize that Lombos do Ulla cockles had acquired certain resiliency to the parasite due to natural selection (Iglesias et al., 2019) and suggested that candidate genes and associated markers were probably underlying marteiliosis resilience. Thus, searching for those genetic markers was considered a main goal for recovering cockle production and preventing its expansion to other areas through breeding programs or appropriate shellfishery management. Similar approaches have been tackled for the identification of molecular markers for disease resilience in other mollusc species (de la Ballina et al., 2018; Ronza et al., 2018; Farhat et al., 2020; Leprêtre et al., 2021).
The immune system of molluscs lacks adaptative immunity and depends mostly on innate immune response, constituted by cellular and humoral responses (see review Allam and Raftos, 2015). Pardo et al. (2022) reported a set of DEGs associated with innate immune function, such as signal transduction, response to stimulus, cytoskeletal organization, pathogen recognition receptor (PRR), serine protease inhibition and antimicrobial response when analysing the transcriptomic response of cockles collected from Ría de Arousa during the same marteiliosis outbreak analysed in our study. Understanding the genetic basis of differential immune response may help to identify mechanisms conferring resilience or susceptibility to a particular disease. Moreover, detection of genetic markers associated with those differences, either responsible or not, but in linkage disequilibrium with the responsible variants, would be decisive for obtaining cockle strains resilient to the parasite.
We hypothesized that M. cochillia decreased prevalence and mortality rates recorded since 2017 in the inner area of the Ría de Arousa (Iglesias et al., 2019; Iglesias et al., 2023), are associated with selection of specific immune gene variants, which could eventually be identified using population genomics and transcriptomic approaches. Accordingly, outlier loci for divergent selection might be identified against the neutral background if appropriate cockle samples related to different marteiliosis pressures were compared. On the other hand, SNPs within DEGs showing genetic differentiation associated with the level of infection would be another relevant source of information for detecting consistent candidate genes holding allelic variants associated with resilience to the parasite. A similar approach has been recently reported in flat oyster O. edulis, where a major QTL related to resilience to Bonamia ostreae was identified (Vera et al., 2019; Sambade et al., 2022). Other selection models, such as overdominance, that have been reported for specific immune genes (Penn, 2002; Kekäläinen et al., 2009), could be operating and therefore our results would only explain part of the increased resilience. Furthermore, epigenetic imprinting is increasingly being claimed to be involved in immune memory in molluscs (Pradeu and Du Pasquier, 2018; Yao et al., 2021) and have also been suggested for bonamiosis resilience in flat oyster (Sambade et al., 2022).
Accordingly, we compared, on one hand, DNA samples preserved since 2012, corresponding to cockles recruited before the parasite’s first detection (naive) vs those from the 2018/2019 period (affected), when the bed had been affected by marteiliosis for six years, the last ones including non-exposed (2018) and exposed (2019) samples to the parasite; and, on the other hand, RNAseq data from DEGs among samples with different levels of infection from the same outbreak (Pardo et al., 2022). The recent publication of the common cockle’s genome (Bruzos et al., 2022) enabled to integrate all that information to look for more consistent signals of selection associated with the response to the parasite.
Using a previously validated 2b-RAD panel by Vera et al. (2022), we observed that genetic diversity in Arousa samples (He: 0.073 to 0.085) was similar to that reported in other studies in northwest Europe (He = 0.077 – 0.088, Vera et al., 2022), although the number of polymorphic loci was lower (6,252 in Ría de Arousa), an expected outcome considering the small geographic area studied. We detected a lower genetic diversity in the affected samples (SLO18/SLO19) than the non-affected ones from 2012, also expected considering the heavy mortalities and strong bottlenecks affecting cockle beds after consecutive marteiliosis outbreaks in the Ría de Arousa (average He: 0.077 vs 0.085; p < 0.05). Besides, we detected a slight heterozygote deficit (FIS > 0) in all the samples with the whole and neutral SNP datasets, a usual observation in molluscs due to the presence of null alleles (see review Hollenbeck and Johnston, 2018). However, the heterozygote deficit was higher with the 110 outlier subset, and especially with the 156 exposure outliers dataset in the Marteilia-exposed sample (SLO19). Additionally, this sample showed significantly higher genetic diversity with outlier loci than non-exposed samples (0.169 vs 0.100; p < 0.05). We cannot rule out other types of selection operating on these markers/genes, i.e. diversifying selection, but currently there is not a straightforward explanation for these observations. New data coming from an ongoing common garden experiment carried out in Ría de Arousa with this set of markers should shed some light on the pattern of genetic diversity observed.
Low genetic structure was detected in Ría de Arousa using the whole SNP dataset, as previously reported for small geographic areas with microsatellites (Martínez et al., 2013) and SNPs (Coscia et al., 2020) in the common cockle, and in other mollusc species with microsatellites (Diz and Presa, 2009; Vera et al., 2016). This observation points towards the high dispersal capacity of the larvae while they remain in the water column. However, we identified 156 and 74 consistent outlier loci when comparing exposed and non-exposed samples and when considering the period of collection, respectively. Among them, a total of 110 outliers were close to DEG reported by Pardo et al. (2022) and were selected as the most consistent ones from this approach. These SNPs were able to discriminate between naive vs affected samples and even between exposed and non-exposed samples from the 2018/19 outbreak, although with less statistical support. On the other hand, we identified 123 SNPs linked to DEGs detected in the same marteiliosis outbreak by Pardo et al. (2022), many of them related to key immune functions, which showed significant genetic differentiation among samples with different levels of infection. We speculate that these SNPs could be associated with allelic variants responsible for the differential expression and consequently under selection to marteiliosis pressure. This approach has been followed to identify markers within DEGs associated with resistance to pathologies or other traits in aquaculture species (Robledo et al., 2017; Robledo et al., 2020; Moraleda et al., 2021).
Finally, taking advantage of the chromosome-level genome assembly, we selected a final panel of the most consistent 60 SNPs, to design a cost-effective molecular tool putatively useful for the selection of resistant strains and management of cockle beds for their recovery. Validation of the “in silico” genotyping information of this SNP set with a robust genotyping tool is being undertaken for its application in an ongoing common garden experiment in the Ría de Arousa, involving cockle stocks from naive and marteiliosis-affected shellfish beds to confirm their usefulness for discriminating resilient and susceptible cockles. This could be eventually used for the appropriate management and recovery of both cockle production and natural bed ecosystems in Galicia, which holds the most important shellfishery of this species in Spain.
Data availability statement
The transcriptomic data presented in this study are deposited in the NCBI repository (https://www.ncbi.nlm.nih.gov/bioproject/?term=PRJNA945848), accession number PRJNA945848. Genotype information from 2b-RADseq has been included as supplementary material in the text (Table S1).
Author contributions
PM performed the population genomics and transcriptomics analyses and selected the SNP panel in collaboration with AdC, VM and CR. FC carried out SNP calling and filtering from transcriptomics data. BA designed custom scripts and supervised the bioinformatic analysis. HM called and genotyped SNPs from 2b-RAD libraries. PB constructed 2b-RAD libraries. AsC and ID were involved in sampling in the field and histological evaluation of infection. VA and MP conceived the study, supervised the project and revised the manuscript. All authors collaborated in the manuscript and approved the final version.
Funding
This study was possible due to funds granted by the European Union through the project COCKLES within the INTERREG-AA programme (EAPA_458/2016) and the scholarship granted to M.R.M.Coimbra from CNPq (202015/2020-3). This research has been carried out under the framework of the Spain's Recovery and Resilience Plan, and more specifically under the investment line no.1 of its component number 17, where the complementary RTDI plan with the autonomous regions of Spain are foreseen, with one of those being the Complementary RTDI Plan for Marine Science which includes the Marine Science Programme for Galicia. This research in this paper corresponds to the Programme Work Package nº 6 and activity no. 6.3.A.2 about "Genetic architecture of marteiliosis resistance in common cockle" and has been funded by the Resilience and Recovery Funds).
Acknowledgments
We thank the bioinformatic support of the Centro de Supercomputación de Galicia (CESGA). M.J. Brianes Beiras, A.I. González Fontela, G. Martínez Verde, M.I. Meléndez Ramos, E. Penas Pampín, P. Rúa Santervas, P. Díaz Cedillo, J. Fernández González, I. López Maneiro, G. Pena Thomas, A. Pérez Caamaño and R. Viturro García provided technical assistance in field work and/or sample processing.
Conflict of interest
The authors declare that the research was conducted in the absence of any commercial or financial relationships that could be construed as a potential conflict of interest.
Publisher’s note
All claims expressed in this article are solely those of the authors and do not necessarily represent those of their affiliated organizations, or those of the publisher, the editors and the reviewers. Any product that may be evaluated in this article, or claim that may be made by its manufacturer, is not guaranteed or endorsed by the publisher.
Supplementary material
The Supplementary Material for this article can be found online at: https://www.frontiersin.org/articles/10.3389/fmars.2023.1057206/full#supplementary-material
References
Allam B., Raftos D. (2015). Immune responses to infectious diseases in bivalves. J. Invertebr. Pathol. 131, 121–136. doi: 10.1016/j.jip.2015.05.005
Andersen T. J., Lanuru M., van Bernem C., Pejrup M., Riethmueller R. (2010). Erodibility of a mixed mudflat dominated by microphytobenthos and Cerastoderma edule, East Frisian Wadden Sea, Germany. Estuar. Coast. Shelf Sci. 87, 197–206. doi: 10.1016/j.ecss.2009.10.014
Audemard C., Le Roux F., Barnaud A., Collins C., Sautour B., Sauriau P. G., et al. (2002). Needle in a haystack: Involvement of the copepod Paracartia grani in the life-cycle of the oyster pathogen Marteilia refringens. Parasitology 124, 315–323. doi: 10.1017/S0031182001001111
Beaumont M. A., Nichols R. A. (1996). Evaluating loci for use in the genetic analysis of population structure. Proc. R. Soc B Biol. Sci. 263, 1619–1626. doi: 10.1098/rspb.1996.0237
Besnier F., Glover K. A. (2013). ParallelStructure: A r package to distribute parallel runs of the population genetics program STRUCTURE on multi-core computers. PloS One 8, e70651. doi: 10.1371/journal.pone.0070651
Beukema J. J., Dekker R. (2005). Decline of recruitment success in cockles and other bivalves in the Wadden Sea: Possible role of climate change, predation on postlarvae and fisheries. Mar. Ecol. Ser. 287, 149–167. doi: 10.3354/meps287149
Bos O. G., Hendriks I. E., Strasser M., Dolmer P., Kamermans P. (2006). Estimation of food limitation of bivalve larvae in coastal waters of north-western Europe. J. Sea Res. 55, 191–206. doi: 10.1016/j.seares.2005.10.006
Bower S. M., McGladdery S. E., Price I. M. (1994). Synopsis of infectious diseases and parasites of commercially exploited shellfish. Annu. Rev. Fish Dis. 4, 1–199. doi: 10.1016/0959-8030(94)90028-0
Bruzos A. L., Santamarina M., García-souto D., Díaz S., Rocha S., Zamora J., et al. (2022). The evolution of two transmissible leukaemias colonizing the coasts of Europe. bioRixiv. doi: 10.1101/2022.08.06.503021
Burdon D., Callaway R., Elliott M., Smith T., Wither A. (2014). Mass mortalities in bivalve populations: A review of the edible cockle Cerastoderma edule (L.). Estuar. Coast. Shelf Sci. 150 (partB), 271–280. doi: 10.1016/j.ecss.2014.04.011
Carballal M. J., Iglesias D., Abollo E., Cao A., García L., Ramilo A., et al. (2019). “Looking for hosts of Marteilia cochillia in the zooplankton,” in 19th International Conference on Diseases of Fish and Shellfish Abstract Book, European Association of Fish Pathologists. (Porto - Portugal). 502.
Carmichael R. H., Walton W., Clark H. (2012). Bivalve-enhanced nitrogen removal from coastal estuaries. Can. J. Fish. Aquat. Sci. 69, 1131–1149. doi: 10.1139/F2012-057
Carrasco N., Green T., Itoh N. (2015). Marteilia spp. parasites in bivalves: A revision of recent studies. J. Invertebr. Pathol. 131, 43–57. doi: 10.1016/j.jip.2015.07.016
Carrasco N., Hine P. M., Durfort M., Andree K. B., Malchus N., Lacuesta B., et al. (2013). Marteilia cochillia sp. nov., a new Marteilia species affecting the edible cockle Cerastoderma edule in European waters. Aquaculture 412–413, 223–230. doi: 10.1016/j.aquaculture.2013.07.027
Catchen J., Hohenlohe P. A., Bassham S., Amores A., Cresko W. A. (2013). Stacks: an analysis tool set for population genomics. Mol. Ecol. 22, 3124–3140. doi: 10.1111/mec.12354
Ciutat A., Widdows J., Readman J. W. (2006). Influence of cockle Cerastoderma edule bioturbation and tidal-current cycles on resuspension of sediment and polycyclic aromatic hydrocarbons. Mar. Ecol. Prog. Ser. 328, 51–64. doi: 10.3354/meps328051
Coscia I., Wilmes S. B., Ironside J. E., Goward-Brown A., O’Dea E., Malham S. K., et al. (2020). Fine-scale seascape genomics of an exploited marine species, the common cockle Cerastoderma edule, using a multimodelling approach. Evol. Appl. 13, 1854–1867. doi: 10.1111/eva.12932
Creek G. A. (1960). The development of the lamellibranch Cardium edule l. Proc. Zool. Soc London 135, 243–260. doi: 10.1111/j.1469-7998.1960.tb05843.x
Darriba S., Iglesias D., Carballal M. J. (2020). Marteilia cochillia is released into seawater via cockle Cerastoderma edule faeces. J. Invertebr. Pathol. 172, 107364. doi: 10.1016/j.jip.2020.107364
Dégremont L., Nourry M., Maurouard E. (2015). Mass selection for survival and resistance to OsHV-1 infection in crassostrea gigas spat in field conditions: Response to selection after four generations. Aquaculture 446, 111–121. doi: 10.1016/j.aquaculture.2015.04.029
de la Ballina N. R., Villalba A., Cao A. (2018). Proteomic profile of the European flat oyster Ostrea edulis haemolymph in response to bonamiosis and identification of candidate proteins as markers of resistance. Dis. Aquat. Organ. 128, 127–145. doi: 10.3354/dao03220
Diz A. P., Presa P. (2009). The genetic diversity pattern of Mytilus galloprovincialis in Galician rías (NW Iberian estuaries). Aquaculture 287, 278–285. doi: 10.1016/j.aquaculture.2008.10.029
Ducrotoy J.-P., Rybarczyk H., Souprayen S., Bachelet G., Beukema J. J., Desprez M., et al. (1991). “A comparison of the population dynamics of the cockle (Cerastoderma edule, l.) in north-Western Europe,” In Elliot M., Ducrotoy J.-P. Eds. Estuaries and Coast: Spatial and Temporal Inrercomparisons. (Olsen and Olsen, pp. 173–183.
Eriksson B. K., Westra J., Van Gerwen I., Weerman E., Vander Zee E., Vander Heide T., et al. (2017). Facilitation by ecosystem engineers enhances nutrient effects in an intertidal system. Ecosphere 8, e02051. doi: 10.1002/ecs2.2051
Evanno G., Regnaut S., Goudet J. (2005). Detecting the number of clusters of individuals using the software STRUCTURE: A simulation study. Mol. Ecol. 14, 2611–2620. doi: 10.1111/j.1365-294X.2005.02553.x
Excoffier L., Lischer H. E. L. (2010). Arlequin suite ver 3.5: a new series of programs to perform population genetics analyses under Linux and windows. Mol. Ecol. Resour. 10, 564–567. doi: 10.1111/j.1755-0998.2010.02847.x
Farhat S., Tanguy A., Pales E., Guo X., Boutet I., Smolowitz R., et al. (2020). Identification of variants associated with hard clam, Mercenaria mercenaria, resistance to quahog parasite unknown disease. Genomics 112, 4887–4896. doi: 10.1016/j.ygeno.2020.08.036
Ferns P. N., Rostron D. M., Siman H. Y. (2000). Effects of mechanical cockle harvesting on intertidal communities. J. Appl. Ecol. 37, 464–474. doi: 10.1046/j.1365-2664.2000.00509.x
Foll M., Gaggiotti O. (2008). A genome-scan method to identify selected loci appropriate for both dominant and codominant markers: A Bayesian perspective. Genetics 993, 977–993. doi: 10.1534/genetics.108.092221
Food and Agriculture Organization of the United Nations [FAO] (2021) Fisheries and aquaculture. global capture production quantity, (1950 - 2020). Available at: https://www.fao.org/fishery/statistics-query/en/aquaculture/aquaculture_value (Accessed August 2, 2022).
Ford S. E., Haskin H. H. (1987). Infection and mortality patterns in strains of oysters Crassostrea virginica selected for resistance to the parasite Haplosporidium nelsoni (MSX). J. Parasitol. 73, 368–376. doi: 10.2307/3282092
Gutierrez A. P., Symonds J., King N., Steiner K., Bean T. P., Houston R. D. (2020). Potential of genomic selection for improvement of resistance to ostreid herpesvirus in pacific oyster (Crassostrea gigas). Anim. Genet. 51, 249–257. doi: 10.1111/age.12909
Han Y., Tang Y., Sun S., Kim T., Ju K., Ri S., et al. (2021). Modulatory function of calmodulin on phagocytosis and potential regulation mechanisms in the blood clam Tegillarca granosa. Dev. Comp. Immunol. 116, 103910. doi: 10.1016/j.dci.2020.103910
Hayward P. J., Ryland J. S. (1995). Handbook of the marine fauna of north-west europe. 2nd ed. Eds. Hayward P. J., Ryland J. S. (Oxford: Oxford University Press). doi: 10.1093/acprof:oso/9780199549443.001.0001
Hollenbeck C. M., Johnston I. A. (2018). Genomic tools and selective breeding in molluscs. Front. Genet. 9. doi: 10.3389/fgene.2018.00253
Iglesias D., Villalba A., Cao A., Carballal M. (2019). “Is natural selection enhancing resistance against marteiliosis in cockles recruited in the inner side of the ria of arousa?,” in 19th international conference on diseases of fish and shellfish, Oporto, Portugal (Porto, Portugal: European Association of Fish Pathologists), 184. Available at: https://eafp.org/wp-content/uploads/2020/01/2019-porto-abstract-book.pdf.
Iglesias D., Villalba A., Mariño C., No E., Carballal M. J. (2023). Long-Term Survey Discloses a Shift in the Dynamics Pattern of an Emerging Disease of Cockles Cerastoderma Edule, Marteiliosis, And Raises Hypothesis to Explain it. doi: 10.2139/ssrn.4381126
Jombart T. (2008). Adegenet: A r package for the multivariate analysis of genetic markers. Bioinformatics 24, 1403–1405. doi: 10.1093/bioinformatics/btn129
Keenan K., Mcginnity P., Cross T. F., Crozier W. W., Prodöhl P. A. (2013). DiveRsity: An r package for the estimation and exploration of population genetics parameters and their associated errors. Methods Ecol. Evol. 4, 782–788. doi: 10.1111/2041-210X.12067
Kekäläinen J., Vallunen J. A., Primmer C. R., Rättyä J., Taskinen J. (2009). Signals of major histocompatibility complex overdominance in a wild salmonid population. Proc. R. Soc B Biol. Sci. 276, 3133–3140. doi: 10.1098/rspb.2009.0727
Kopelman N. M., Mayzel J., Jakobsson M., Rosenberg N. A., Mayrose I. (2015). CLUMPAK: A program for identifying clustering modes and packaging population structure inferences across K. Mol. Ecol. Resour. 15, 1179–1191. doi: 10.1111/1755-0998.12387
Kube P. D., Cunningham M., Dominik S., Parkinson S., Henshall J., Finn B., et al. (2011). Enhancement of the pacific oyster selective breeding program. FRDC and SeafoodCRC Final Report Project No. 2006/227. (Hobart, TAS, Australia: CSIRO Marine and Atmospheric Research).
Langmead B., Trapnell C., Pop M., Salzberg S. L. (2009). Ultrafast and memory-efficient alignment of short DNA sequences to the human genome. Genome Biol. 10, R25. doi: 10.1186/gb-2009-10-3-r25
Lauckner G. (1983). Diseases of Mollusca: Bivalvia. In Ed. Kinne O. Diseases of Marine Animals (Hamburgh: Biologische Anstalt Helgoland) II (ed. Kinne O.) 805–817.
Leprêtre M., Faury N., Segarra A., Claverol S., Degremont L., Palos-ladeiro M., et al. (2021). Comparative proteomics of ostreid herpesvirus 1 and pacific oyster interactions with two families exhibiting contrasted susceptibility to viral infection. Front. Immunol. 11. doi: 10.3389/fimmu.2020.621994
Li H., Handsaker B., Wysoker A., Fennell T., Ruan J., Homer N., et al. (2009). The sequence alignment/map format and SAMtools. Bioinformatics 25, 2078–2079. doi: 10.1093/bioinformatics/btp352
Li Y., Liu J. (2018). StructureSelector: A web-based software to select and visualize the optimal number of clusters using multiple methods. Mol. Ecol. Resour. 18, 176–177. doi: 10.1111/1755-0998.12719
Møhlenberg F., Kiørboe T. (1983). Burrowing and avoidance behaviour in marine organisms exposed to pesticide-contaminated sediment. Mar. pollut. Bull. 14, 57–60. doi: 10.1016/0025-326X(83)90192-3
Maia F., Barroso C. M., Gaspar M. B. (2021). Biology of the common cockle Cerastoderma edule (Linnaeus 1758) in ria de aveiro (NW portugal): Implications for fisheries management. J. Sea Res. 171, 102024. doi: 10.1016/j.seares.2021.102024
Martínez L., Méndez J., Insua A., Arias-Pérez A., Freire R. (2013). Genetic diversity and population differentiation in the cockle Cerastoderma edule estimated by microsatellite markers. Helgol. Mar. Res. 67, 179–189. doi: 10.1007/s10152-012-0314-3
Mascaró M., Seed R. (2000). Foraging behavior of Carcinus maenas (L.): Comparisons of size-selective predation on four species of bivalve prey. J. Shellfish Res. 19, 283–291. doi: 10.1007/s002270100677
Montaudouin X., Arzul I., Cao A., Carballal M. J., Chollet B., Correia S., et al. (2021). Catalogue of parasites and diseases of the common cockle cerastoderma edule - COCKLES PROJECT (Aveiro: UA Editora – Universidade de Aveiro). doi: 10.34624/9a9c-9j21
Moraleda C. P., Robledo D., Gutiérrez A. P., Del-Pozo J., Yáñez J. M., Houston R. D. (2021). Investigating mechanisms underlying genetic resistance to salmon rickettsial syndrome in Atlantic salmon using RNA sequencing. BMC Genomics 22, 156. doi: 10.1186/s12864-021-07443-2
Moreira Sanmartín R., Roberts S., Figueras A. (2016). “Molluscs,” in Genomics in aquaculture. Eds. MacKenzie S., Jentoft S. (London: Elsevier), 223–245. doi: 10.1016/B978-0-12-801418-9.00009-3
Nanut M. P., Sabotič J., Jewett A., Kos J. (2014). Cysteine cathepsins as regulators of the cytotoxicity of NK and T cells. Front. Immunol. 5. doi: 10.3389/fimmu.2014.00616
Narum S. R., Hess J. E. (2011). Comparison of FST outlier tests for SNP loci under selection. Mol. Ecol. Resour. 11, 184–194. doi: 10.1111/j.1755-0998.2011.02987.x
Navas J. I., López-Sanmartín M., Perez-Miguel M., Drake P. (2018). “Detection of Martelia cochillia in Cerastoderma edule from Huelva coast (Andalusia Spain), Montpellier,” in AQUA 2018 - we r aquaculture, 463.
Nell J. A., Smith I. R., McPhee C. C. (2000). The Sydney rock oyster Saccostrea glomerata (Gould 1850) breeding programme: progress and goals. Aquac. Res. 31, 45–49. doi: 10.1016/S0044-8486(03)00133-9
Neumeier U., Lucas C. H., Collins M. (2006). Erodibility and erosion patterns of mudflat sediments investigated using an annular flume. Aquat. Ecol. 40, 543–554. doi: 10.1007/s10452-004-0189-8
Niu D., Jin K., Wang L., Feng B., Li J. (2013). Molecular characterization and expression analysis of four cathepsin l genes in the razor clam, Sinonovacula constricta. Fish Shellfish Immunol. 35, 581–588. doi: 10.1016/j.fsi.2013.06.001
Parada J. M., Molares J. (2008). Natural mortality of the cockle Cerastoderma edule (L.) from the Ría of Arousa (NW Spain) intertidal zone. Rev. Biol. Mar. Oceanogr. 43, 501–511. doi: 10.4067/S0718-19572008000300009
Pardo B. G., Fernández C., Pampín M., Blanco A., Iglesias D., Cao A., et al. (2022). Transcriptome characterization of the common cockle (Cerastoderma edule) after exposure to a Marteilia cochillia outbreak. bioRixiv. doi: 10.1101/2022.10.18.512677
Pembleton L. W., Cogan N. O. I., Forster J. W. (2013). StAMPP: An r package for calculation of genetic differentiation and structure of mixed-ploidy level populations. Mol. Ecol. Resour. 13, 946–952. doi: 10.1111/1755-0998.12129
Penn D. J. (2002). The scent of genetic compatibility: Sexual selection and the major histocompatibility complex. Ethology 108, 1–21. doi: 10.1046/j.1439-0310.2002.00768.x
Ponsero A., Dabouineau L., Allain J. (2009). Modelling of common European cockle Cerastoderma edule fishing grounds aimed at sustainable management of traditional harvesting. Fish. Sci. 75, 839–850. doi: 10.1007/s12562-009-0110-4
Pradeu T., Du Pasquier L. (2018). Immunological memory: What’s in a name? Immunol. Rev. 283, 7–20. doi: 10.1111/imr.12652
Pritchard J., Stephens M., Donnelly P. (2000). Inference of population structure using multilocus genotype data. Genetics 155, 945–959. doi: 10.1093/genetics/155.2.945
Proestou D. A., Vinyard B. T., Corbett R. J., Piesz J., Allen S. K., Small J. M., et al. (2016). Performance of selectively-bred lines of eastern oyster, crassostrea virginica, across eastern US estuaries. Aquaculture 464, 17–27. doi: 10.1016/j.aquaculture.2016.06.012
Puechmaille S. J. (2016). The program structure does not reliably recover the correct population structure when sampling is uneven: Subsampling and new estimators alleviate the problem. Mol. Ecol. Resour. 16, 608–627. doi: 10.1111/1755-0998.12512
Purcell S., Neale B., Todd-Brown K., Thomas L., Ferreira M. A. R., Bender D., et al. (2007). PLINK: A tool set for whole-genome association and population-based linkage analyses. Am. J. Hum. Genet. 81, 559–575. doi: 10.1086/519795
Ragone Calvo L. M., Calvo G. W., Burreson E. M. (2003). Dual disease resistance in a selectively bred eastern oyster, Crassostrea virginica, strain tested in Chesapeake bay. Aquaculture 220, 69–87. doi: 10.1016/S0044-8486(02)00399-X
Rakotomalala C., Grangeré K., Ubertini M., Forêt M., Orvain F. (2015). Modelling the effect of Cerastoderma edule bioturbation on microphytobenthos resuspension towards the planktonic food web of estuarine ecosystem. Ecol. Modell. 316, 155–167. doi: 10.1016/j.ecolmodel.2015.08.010
Robledo D., Hamilton A., Gutiérrez A. P., Bron J. E., Houston R. D. (2020). Characterising the mechanisms underlying genetic resistance to amoebic gill disease in Atlantic salmon using RNA sequencing. BMC Genomics 21, 271. doi: 10.1186/s12864-020-6694-x
Robledo D., Rubiolo J. A., Cabaleiro S., Martínez P., Bouza C. (2017). Differential gene expression and SNP association between fast- and slow-growing turbot (Scophthalmus maximus). Sci. Rep. 7, 12105. doi: 10.1038/s41598-017-12459-4
Rochette N. C., Rivera-Colón A. G., Catchen J. M. (2019). Stacks 2: Analytical methods for paired-end sequencing improve RADseq-based population genomics. Mol. Ecol. 28, 4737–4754. doi: 10.1111/mec.15253
Ronza P., Cao A., Robledo D., Gómez-Tato A., Álvarez-Dios J. A., Hasanuzzaman A. F. M., et al. (2018). Long-term affected flat oyster (Ostrea edulis) haemocytes show differential gene expression profiles from naïve oysters in response to Bonamia ostreae. Genomics 110, 390–398. doi: 10.1016/j.ygeno.2018.04.002
Rousset F. (2008). GENEPOP’007: a complete re-implementation of the GENEPOP software for windows and Linux. Mol. Ecol. Resour. 8, 103–106. doi: 10.1111/j.1471-8286.2007.01931.x
Rowley A. F., Cross M. E., Culloty S. C., Lynch S. A., Mackenzie C. L., Morgan E., et al. (2014). The potential impact of climate change on the infectious diseases of commercially important shellfish populations in the Irish Sea–a review. ICES J. Mar. Sci. 71, 741–759. doi: 10.1093/icesjms/fst234
Sambade I. M., Casanova A., Blanco A., Gundappa M. K., Bean T. P., Macqueen D. J., et al. (2022). A single genomic region involving a putative chromosome rearrangement in flat oyster (Ostrea edulis) is associated with differential host resilience to the parasite Bonamia ostreae. Evol. Appl. 00, 1–15. doi: 10.1111/eva.13446
Smits M., Enez F., Ferraresso S., Dalla Rovere G., Vetois E., Auvray J. F., et al. (2020). Potential for genetic improvement of resistance to Perkinsus olseni in the Manila clam, Ruditapes philippinarum, using DNA parentage assignment and mass spawning. Front. Vet. Sci. 7. doi: 10.3389/fvets.2020.579840
Stanley R. R. E. E., Jeffery N. W., Wringe B. F., DiBacco C., Bradbury I. R. (2017). GENEPOPEDIT: a simple and flexible tool for manipulating multilocus molecular data in r. Mol. Ecol. Resour. 17, 12–18. doi: 10.1111/1755-0998.12569
Subsecretaría Subdirección General de Análisis Coordinacion y Estadística (2020) Estadísticas pesqueras noviembre 2020 [Fishery statistics November 2020] (Ministerio de Agricultura Pesca y Alimentación). Available at: https://www.mapa.gob.es/es/estadistica/temas/estadisticas-pesqueras/ (Accessed June 15, 2022).
Sutherland W. J. (1982). Do oystercatchers select the most profitable cockles? Anim. Behav. 30, 853. doi: 10.1016/S0003-3472(82)80159-0
Swanberg I. (1991). The influence of the filter-feeding bivalve Cerastoderma edule l. on microphytobenthos: a laboratory study. J. Exp. Mar. Bio. Ecol. 151, 93–111. doi: 10.1016/0022-0981(91)90018-R
Tyler-Walters H. (2007). “Cerastoderma edule (Common cockle),” in Mar. life inf. netw. biol. sensit. key inf. rev. Eds. Tyler-Walters H., Hiscock K. (United Kingdom: Plymouth Mar. Biol. Assoc), 1–23. doi: 10.17031/marlinsp.1384.1
van der Schatte Olivier A., Jones L., Vay L., Christie M., Wilson J., Malham S. K. (2020). A global review of the ecosystem services provided by bivalve aquaculture. Rev. Aquac. 12, 3–25. doi: 10.1111/raq.12301
Vera M., Carlsson J., Carlsson J., Cross T., Lynch S., Kamermans P., et al. (2016). Current genetic status, temporal stability and structure of the remnant wild European flat oyster populations: conservation and restoring implications. Mar. Biol. 163, 239. doi: 10.1007/s00227-016-3012-x
Vera M., Maroso F., Wilmes S. B., Hermida M., Blanco A., Fernández C., et al. (2022). Genomic survey of edible cockle (Cerastoderma edule) in the northeast Atlantic: a baseline for sustainable management of its wild resources. Evol. Appl. 15, 262–285. doi: 10.1111/eva.13340
Vera M., Pardo B. G., Cao A., Vilas R., Fernández C., Blanco A., et al. (2019). Signatures of selection for bonamiosis resistance in European flat oyster (Ostrea edulis): New genomic tools for breeding programs and management of natural resources. Evol. Appl. 12, 1781–1796. doi: 10.1111/eva.12832
Vigneron N., Van den Eynde B. J. (2014). Proteasome subtypes and regulators in the processing of antigenic peptides presented by class I molecules of the major histocompatibility complex. Biomolecules 4, 994–1025. doi: 10.3390/biom4040994
Villalba A., Iglesias D., Ramilo A., Darriba S., Parada J. M., No E., et al. (2014). Cockle Cerastoderma edule fishery collapse in the Ría de Arousa (Galicia, NW Spain) associated with the protistan parasite marteilia cochillia. Dis. Aquat. Organ. 109, 55–80. doi: 10.3354/dao02723
Wang S., Meyer E., Mckay J. K., Matz M. V. (2012). 2b-RAD: a simple and flexible method for genome-wide genotyping. Nat. Methods 9, 808–810. doi: 10.1038/nmeth.2023
Keywords: SNP, bivalve, cockles, transcriptomics, population genomics, Marteilia cochillia, resilience
Citation: Pampín M, Casanova A, Fernández C, Blanco A, Hermida M, Vera M, Pardo BG, Coimbra RM, Cao A, Iglesias D, Carballal MJ, Villalba A and Martínez P (2023) Genetic markers associated with divergent selection against the parasite Marteilia cochillia in common cockle (Cerastoderma edule) using transcriptomics and population genomics data. Front. Mar. Sci. 10:1057206. doi: 10.3389/fmars.2023.1057206
Received: 29 September 2022; Accepted: 21 March 2023;
Published: 04 April 2023.
Edited by:
Fran Saborido-Rey, Spanish National Research Council (CSIC), SpainReviewed by:
Tim Regan, University of Edinburgh, United KingdomTereza Manousaki, Hellenic Centre for Marine Research (HCMR), Greece
Copyright © 2023 Pampín, Casanova, Fernández, Blanco, Hermida, Vera, Pardo, Coimbra, Cao, Iglesias, Carballal, Villalba and Martínez. This is an open-access article distributed under the terms of the Creative Commons Attribution License (CC BY). The use, distribution or reproduction in other forums is permitted, provided the original author(s) and the copyright owner(s) are credited and that the original publication in this journal is cited, in accordance with accepted academic practice. No use, distribution or reproduction is permitted which does not comply with these terms.
*Correspondence: P. Martínez, cGF1bGluby5tYXJ0aW5lekB1c2MuZXM=