- 1Laboratory of Marine Geophysics and Georesources of Hainan Province, Institute of Deep-sea Science and Engineering, Chinese Academy of Sciences, Sanya, China
- 2Southern Marine Science and Engineering Guangdong Laboratory (Zhuhai), Zhuhai, China
- 3Yangtze University, Wuhan, China
- 4University of Chinese Academy of Sciences, Beijing, China
Classifying natural gas hydrate reservoirs effectively and carrying out reservoir classification modelling is crucial, but to date, research on building artificial intelligence-assisted logging curve reservoir classification models is not abundant. As exploration and development have progressed, an increasing number of fine-grained reservoirs are being discovered, and their strong heterogeneity makes correct reservoir classification even more important. Two wells used for detecting hydrates in the Qiongdongnan (QDN) Basin are used to explore the relationship between logging response parameters and reservoir quality, as well as the method of building a logging-based reservoir classification model. Through K-means clustering and Adaboost methods, the K-means method is considered to be able to correspond to the hydrate enrichment degree, while the random forest method can establish an effective reservoir classification model (the recognition accuracy is 95%). In the different categories of reservoirs, the physical properties of the reservoirs are obviously poor, and the corresponding hydrate saturation is also low, which indicates that heterogeneity has indeed affected the enrichment of hydrates in fine-grained reservoirs. This reservoir classification research method can effectively recognize reservoirs.
1 Introduction
As a type of unconventional fossil energy, methane hydrates are solids composed of methane molecules trapped in a water cage, which remain stable at low temperatures and high pressures (Collett, 2002; Cai et al., 2022a; Li et al., 2022a; Zhu et al., 2023b). These hydrates are found in the oceans and frozen soil, and the largest natural gas hydrate resources are located in the oceans. Studying gas hydrates is important for various fields, such as energy, marine environments, and carbon cycles (Boswell and Collett, 2011; Berndt et al., 2014; Ketzer et al., 2020; Wan et al., 2020; Song et al., 2021).
Sandy sediments with simple structures and sufficient space create hydrates that are found in various locations, such as Mexico’s deep-water bays and Japan’s Nankai Trough (Konno et al., 2015; Cook and Portnov, 2019; Johnson et al., 2022). These reservoirs have good physical properties, high hydrate levels, and even hydrate distribution. Coarse-grained reservoirs have high hydrate saturation, but it is difficult to produce a nonhomogenous hydrate distribution (Winters et al., 2011; Liu et al., 2020; Singh et al., 2022). In contrast, fine-grained reservoirs, such as those in the Hikurangi subduction margin of New Zealand and the northern South China Sea (Wei et al., 2018; Cook et al., 2020; Dutilleul et al., 2020; Greve et al., 2020; Su et al., 2021; Wei et al., 2021; Behboudi et al., 2022), have complex pore structures resulting in massive visible hydrate forms (such as fractured, massive, thinly layered, vein-like, thickly layered and pore-like, which have been found and have shown strong heterogeneity). These reservoirs consist mainly of silt and clay, have low hydrate saturation levels and poor physical properties, and require further research for classification and evaluation using logging. Therefore, identifying optimal hydrate-rich reservoirs before drilling is challenging.
Classifying fine-grained reservoirs is crucial due to their high heterogeneity in hydrate distribution (Ye et al., 2019; Lai et al., 2021; Zhang et al., 2022a). Zhu et al. (2022b) confirmed that fine-grained hydrate-bearing reservoirs with different physical properties should be modelled with different saturation models. Bai et al. (2022) found that fine sorting, high calcareous ultramicrofossil abundance, and a high clay mineral content have a significant impact on hydrate generation. Riedel et al. (2013) attempted to identify seismic facies using principal component analysis and achieved good results. Wang D. et al. (2021) found that microfractures and fine-grained sediments both influence hydrate generation. Lubo-Robles et al. (2023) conducted stratigraphic classification using principal component analysis and self-organizing maps. Despite recent advances in reservoir classification, particularly in the area of logging-based classification, there is still much room for improvement. The Guangzhou Marine Geological Survey conducted the GMGS5 cruise, drilling multiple times in strata with obvious continuous seabed-like bottom-simulating reflectors (BSRs), finding that hydrate-bearing layer thicknesses in different wells range from 4 to 165 metres, presenting significant differences. Due to ongoing research on saturation calculations based on resistivity or acoustic logging, it is difficult to determine the enrichment of hydrates in geological formations (Pan et al., 2020 Li et al., 2022; Wan et al., 2022; Zhu et al., 2022b; Zhu et al., 2023a). This study is based on K-means clustering and the Adaboost method and provides reliable reservoir classification results.
2 Geological settings
The QDN area, which is adjacent to the Xisha trough in the east and Hainan Island to the north, is located in the western section of the northern continental slope of the South China Sea (Zhang et al., 2018; Zhao et al., 2018; Xie et al., 2019). With an area larger than 80,000 km2 and most of it being in deep water, the QDN area exhibits promising potential for hydrate exploration, given the large presence of gas chimneys and BSRs and the favourable conditions of seafloor water temperatures (2-3°C) and the average geothermal gradient (40°C/km) (Liang et al., 2019; Ye et al., 2019; Zhang et al., 2020). During the GMGS5 expedition by the Guangzhou Marine Geological Survey in 2018, hydrate exploration drilling was carried out in the Songnan low uplift in the QDN Basin, with considerable hydrate discoveries in wells, such as W8 and W9, in water depths of 1600-1800 m. In recent years, numerous publications have provided detailed descriptions of the location and characteristics of the area, and therefore, we do not repeat them here (Cheng et al., 2021; Deng et al., 2021; Lai et al., 2021; Ren et al., 2022).
The hydrates in the Qiongdongnan area consist primarily of seepage-type and type-II hydrates, with thinly layered, thickly layered, and massive distributions (Wei et al., 2019; Meng et al., 2021). Gas chimneys act as important vertical migration channels for the formation and accumulation of natural gas hydrates (Snyder et al., 2020; Callow et al., 2021), and whether their cracks extend to the Quaternary system determines the quality of gas sources for hydrate enrichment.
3 Materials and methods
3.1 Logging while drilling and coring
During the GMGS5 expedition, the operating mode for hydrate exploration activities in the South China Sea was followed, with pilot hole drilling and logging-while-drilling (LWD) measurements using Schlumberger measurement tools. The LWD tools provided conventional logging curves, including UCAV, GR, RD, AC, CNL, DEN, NMR, FMI, and other special logging curves that could help identify hydrate layers based on curve responses (Kang et al., 2020). However, special logging data were not collected from wells W8 and W9, which were selected for this study. While only UCAV, GR, RD, AC, CNL, and DEN logging curves were obtained from well W8, well W9 had only UCAV, GR, RD, AC, and DEN logging curves. Nevertheless, the conventional logging curves enabled the evaluation of some macroscopic reservoir parameters. Multiple boreholes are typically drilled at each site with close distances between them to ensure that logging curves obtained from some wells can be correlated with hydrate-bearing cores obtained from other wells. Conventional coring and pressure coring are effective in determining parameters such as particle size, gas composition, and mineral composition. In both the W8 and W9 wells, hydrates were found and valid core samples were obtained.
3.2 Reservoir classification methods and modelling processes
Hydrate saturation was used as a comparison parameter to evaluate the effectiveness of the classification method. Parameters such as the clay content, porosity, and grain size, which are commonly used in previous literature to reflect rock and physical properties, were used as input parameters for classification using the K-means clustering algorithm (Yu et al., 2021). These data were sourced from previous articles (Deng et al., 2021; Lai et al., 2021; Wei et al., 2021). The hydrate saturation was calculated through pore water analysis or obtained by decomposing hydrates after pressure coring. The grain size data of the core were obtained through a laser particle size analyser. The clay content data of the core were obtained through X-ray diffraction experiments. Subsequently, an Adaboost model was established, with the class as the output and the logging curve response as the input, to achieve the continuous evaluation of reservoirs (Al-Mudhafar et al., 2022). Figure 1 shows the distribution of data for the clay content, average grain size and hydrate saturation.
3.2.1 K-means clustering
K-means is a highly classical and effective clustering method (Macqueen, 1967). Unlike regression, discrimination, and generative machine learning methods, clustering methods aim to automatically give potential categories based on the intrinsic features of data. K-means is used to group data points with similar features and provide categories. It is an unsupervised learning method that groups data points based on their similarity or distance. The K-means clustering method can partition data points into K clusters, which can be manually set. The algorithm continuously iterates and gradually assigns data points to ensure that the similarity between the data points in each cluster is as high as possible. Formula (1) gives the similarity calculation formula (in a one-dimensional situation):
Formula (1) is a similarity calculation formula based on Euclidean distance theory and is also the most commonly used method for measuring similarity. Advantages of K-means include ease of implementation, efficiency, and ease of interpretation. Note that some outliers are deleted before use.
3.2.2 Adaboost method
Adaboost (adaptive boosting) is an ensemble learning method that evolves weak classifiers into strong classifiers and improves classifier accuracy (Schapire, 1990). Like the K-means algorithm, it is also an iterative algorithm. In each iteration, Adaboost biases the data distribution towards misclassified data by assigning weights to the data. When building a new classifier, Adaboost then biases the new classifier towards correcting the errors of the previous classifier, building a weaker classifier that is easier to predict accurately. Finally, the prediction results of each classifier are assigned a weight based on their accuracy, and all classifier results are combined to form a strong classifier and obtain the final classification result. Adaboost has many advantages, such as high accuracy, resistance to overfitting, and insensitivity to noisy data, and obtains the best model in a concatenated way.
4 Results
4.1 Intelligent classification of hydrate-bearing sedimentary reservoirs
Here, to verify the reliability of the method, we use the data from well W8 to build a model and predict the reservoir types of both well W8 and well W9. Previous studies have found that in hydrate-bearing sedimentary formations in the Qiongdongnan Basin, the enrichment of hydrates is mainly related to porosity, clay content, grain size, and pore structure (Deng et al., 2021; Wu et al., 2021; Wang et al., 2022). When the porosity of the formation is larger, the pore structure tends to be simpler, and natural gas is more easily trapped in the pores to form hydrates. An increase in the clay content can increase the capillary pressure in the pore space, preventing natural gas from further entering the pores and reducing the formation of natural gas hydrates (Wang et al., 2022). In addition, an increase in the clay content can reduce the growth space of hydrates by filling the pores, further increasing the difficulty of hydrate formation (Li et al., 2021a; Li et al., 2022b). Moreover, when the reservoir is dominated by fine-grained sediments, the complexity of the pore structure, especially the reduction in the pore size, can also increase the difficulty of hydrate formation (Li et al., 2021b; Wang S. et al., 2021).
For well W8, we collected core data for porosity (determined by porosity logging curves, although it may be affected by reservoir heterogeneity), but because porosity has not undergone significant diagenetic alterations, the accuracy of porosity logging calculations can still be guaranteed (Tavakoli and Jamalian, 2019; Nazemi et al., 2021; Tavakoli et al., 2022), as well as the clay content and grain size. Therefore, by using the K-means clustering method in combination with the above core data, the reservoir can be classified. We used the commercial software MATLAB R2020b to implement the K-means clustering method. In the collected data, there were a total of 43 samples, which were divided into 3 categories after normalization and clustering. The classification results after clustering are shown in Table 1, and the category results after clustering are shown in Figure 2.
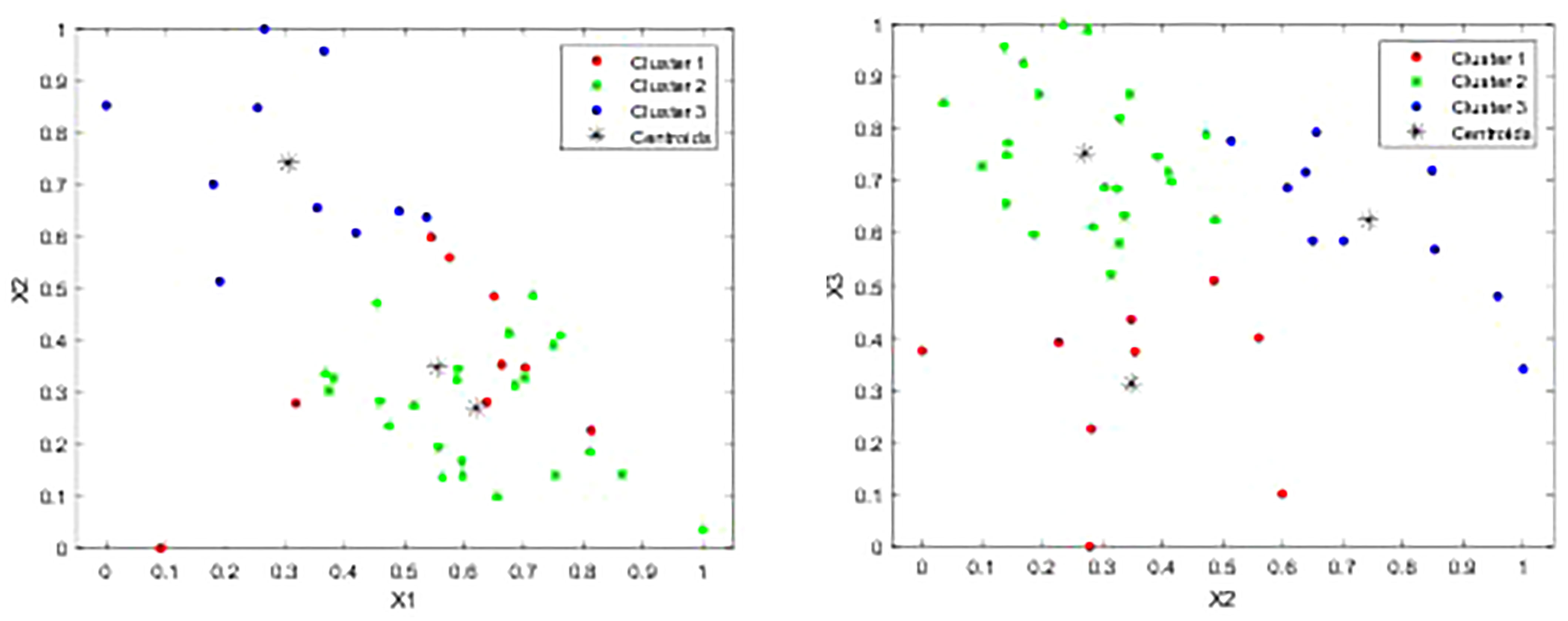
Figure 2 Shows the clustering results. X1 represents the normalized clay content, X2 represents the normalized porosity, and X3 represents the normalized average grain size. From the clustering results, the relationship between the clusters and X2 and X3 is stronger.
Figure 2 shows that the clustering algorithm gave reasonable results based on the parameter information of the core. Combined with previous research on the impact of the above geological parameters on hydrate enrichment, we believe that this reservoir classification method is indeed effective. The next step is to explore whether effective stratigraphic division can be achieved based on logging curve responses to deepen our understanding of hydrate enrichment.
4.2 Prediction of gas hydrate sediment reservoir types
As previously mentioned, Adaboost was chosen as the classifier, with multiple logging curve responses as inputs for prediction. For wells W8 and W9, only a few reliable conventional logging curves have been collected, including density, neutron porosity, natural gamma and resistivity logging curves, so we used these 4 curves for model training. It is worth noting that in Adaboost, we used decision trees as the base classifier model, so there is no need to normalize the logging curves. MaxNumSplits is a critical parameter in the Adaboost algorithm. A large MaxNumSplits increases the accuracy of the model in the training samples but also decreases its generalization ability to some extent. After testing, we found that when all decision trees were allowed to split only once, the accuracy of back-testing on the training samples was 79%, and when they were allowed to split twice, the accuracy was 95%. When allowed to split more than three times, the accuracy of back-testing can reach 100%. Considering the need for back-testing accuracy and generalization ability, we chose to allow a maximum of two splits. After building the model, we predicted the reservoir types of the W8 and W9 wells. The predicted results of the reservoir types for wells W8 and W9 are shown in Figures 3, 4, respectively.
Figures 3, 4 clearly demonstrate the results of reservoir classification. The yellow-highlighted intervals are potential high-quality gas hydrate reservoirs that were identified through a combination of intelligent reservoir classification and comprehensive subdivision. First, the results of reservoir classification should be acknowledged because the identified optimal reservoirs (Cluster 3) are often located in intervals with relatively high gas hydrate saturation (as the gas hydrate saturation measured from core samples can be considered relatively accurate). This means that the reservoir categories obtained through clustering based on the clay content, porosity, and average grain size can effectively guide reservoir classification based on well logs.
Furthermore, the correspondence between the reservoir classification results and the logging responses also indicates that the logging curves have successfully learned the rules of reservoir classification. For example, according to the clustering results, Cluster 3 should be the best reservoir type, which has a higher porosity, lower clay content, and coarser grain size. Corresponding changes can also be observed in the logging responses. For instance, the low natural gamma ray logging response value of Cluster 3 indicates a lower clay content and coarser grain size; the low-density logging response value reflects high-porosity characteristics; and the high resistivity logging response value is due to the high hydrate enrichment degree of the high-quality reservoirs, which affects the conductivity of the electric current. This consistency between reservoir classification and prediction can be explained. Moreover, the advantage of this study is that although we can roughly distinguish potential high-quality reservoirs from logging responses, we cannot clearly define effective reservoir classification boundaries without relying on core data. Combining the results of core-based reservoir classification solved this problem. Currently, logging-based reservoir classification and prediction technologies are not widely used in drilling for hydrate reservoirs globally, including the Qiongdongnan area in the South China Sea. Therefore, the research in this paper can support future exploration and trial production of hydrates.
5 Conclusions
Exploration and trial production of gas hydrates are progressing rapidly, but the corresponding detailed methods for understanding gas hydrate reservoirs using well logging data are still lacking. In this paper, we studied a method for reservoir classification and prediction based on both core and well logging data and obtained certain insights and good results, as follows.
The enrichment of hydrates in sedimentary reservoirs is related to the clay content, porosity, and average grain size. An increase in the porosity, a decrease in the clay content, and a finer reservoir grain size make hydrate formation more difficult. Based on the three parameters of clay content, porosity, and average grain size, rock data can be classified into different reservoir categories, which represent the quality of the reservoir.
The Adaboost algorithm can effectively predict the reservoir category, using the logging curve response as input and the rock core reservoir type as output. When the maximum number of splits is 2, the back-testing accuracy of the rock core data can reach 95%. Based on the established model, the reservoir categories of wells W8 and W9 were predicted. It is believed that logging curves can learn the difference information of reservoir categories, and the predicted Cluster 3 corresponds to the high-quality reservoir in the formation. The average water saturation of gas hydrates obtained from the high-quality reservoir is higher than that of other types of reservoirs. The logging-based reservoir classification and prediction method plays a key role in further understanding gas hydrate reservoirs.
Data availability statement
The original contributions presented in the study are included in the article/supplementary material. Further inquiries can be directed to the corresponding author.
Author contributions
LZ: Write, Review, Conceptualization. XZ: Write, Review. JS: Review. YL: Review. JW: Review. SW: Review, Funding. All authors contributed to the article and approved the submitted version.
Funding
This project was funded by the Hainan Provincial Natural Science Foundation of China (No. 422RC746); National Natural Science Foundation of China (No. 42106213); National key research and development program (No. 2021YFC3100601); China Postdoctoral Science Foundation (Nos. 2021M690161 and 2021T140691).
Acknowledgments
The authors would like to express their sincere gratitude to the editor for his enthusiasm, patience, and tireless efforts. The authors are grateful to the 2 reviewers for their constructive advice on how to improve the paper.
Conflict of interest
The authors declare that the research was conducted in the absence of any commercial or financial relationships that could be construed as a potential conflict of interest.
Publisher’s note
All claims expressed in this article are solely those of the authors and do not necessarily represent those of their affiliated organizations, or those of the publisher, the editors and the reviewers. Any product that may be evaluated in this article, or claim that may be made by its manufacturer, is not guaranteed or endorsed by the publisher.
References
Al-Mudhafar W. J., Abbas M. A., Wood D. A. (2022). Performance evaluation of boosting machine learning algorithms for lithofacies classification in heterogeneous carbonate reservoirs. Mar. Petroleum Geol. 145, 105886. doi: 10.1016/j.marpetgeo.2022.105886
Bai C., Su P., Su X., Cui H., Shang W., Han S., et al. (2022). Characterization of the sediments in a gas hydrate reservoir in the northern south China Sea: Implications for gas hydrate accumulation. Mar. Geol. 453, 106912. doi: 10.1016/j.margeo.2022.106912
Behboudi E., McNamara D. D., Lokmer I., Wallace L. M., Saffer D. (2022). Spatial variation of shallow stress orientation along the hikurangi subduction margin: Insights from in-situ borehole image logging. J. Geophys. Res.: Solid Earth 127, e2021JB023641. doi: 10.1029/2021JB023641
Berndt C., Feseker T., Treude T., Krastel S., Liebetrau V., Niemann H., et al. (2014). Temporal constraints on hydrate-controlled methane seepage off svalbard. Science 343 (6168), 284–287. doi: 10.1126/science.1246298
Boswell R., Collett T. (2011). Current perspectives on gas hydrate resources. Energy Environ. Sci. 4 (4), 1206–1215. doi: 10.1039/C0EE00203H
Cai J., Zhao L., Zhang F., Wei W. (2022a). Advances in multiscale rock physics for unconventional reservoirs. Adv. Geo-Energy Res. 6 (4), 271–275. doi: 10.46690/ager.2022.04.01
Callow B., Bull J. M., Provenzano G., Böttner C., Birinci H., Robinson A. H., et al. (2021). Seismic chimney characterisation in the north Sea – implications for pockmark formation and shallow gas migration. Mar. Petroleum Geol. 133, 105301. doi: 10.1016/j.marpetgeo.2021.105301
Cheng C., Jiang T., Kuang Z., Ren J., Liang J., Lai H., et al. (2021). Seismic characteristics and distributions of quaternary mass transport deposits in the qiongdongnan basin, northern south China Sea. Mar. Petroleum Geol. 129, 105118. doi: 10.1016/j.marpetgeo.2021.105118
Collett T. (2002). Energy resource potential of natural gas hydrates. AAPG Bull. 86 (11), 1971–1992. doi: 10.1016/S0031-0182(02)00486-8
Cook A., Paganioni M., Clennell M. B., McNamara D. D., Nole M., Wang X., et al. (2020). Physical properties and gas hydrate at a near-seafloor thrust fault, hikurangi margin, new Zealand. Geophys. Res. Lett. 47 (16), e2020GL088474. doi: 10.1029/2020GL088474
Cook A., Portnov A. (2019). Gas hydrates in coarse-grained reservoirs interpreted from velocity pull up: Mississippi fan, gulf of Mexico: comment. Geology 47 (3), e457. doi: 10.1130/G45609C.1
Deng W., Liang J., Zhang W., Kuang Z., Zhong T., He Y. (2021). Typical characteristics of fracture-filling hydrate-charged reservoirs caused by heterogeneous fluid flow in the qiongdongnan basin, northern south China sea. Mar. Petroleum Geol. 124, 104810. doi: 10.1016/j.marpetgeo.2020.104810
Dutilleul J., Bourlange S., Géraud Y., Stemmelen D. (2020). Porosity, pore structure, and fluid distribution in the sediments entering the northern hikurangi margin, new Zealand. J. Geophys. Res.: Solid Earth 125, e2020JB020330. doi: 10.1029/2020JB020330
Greve A., Kars M., Zerbst L., Stipp M., Hashimoto Y. (2020). Strain partitioning across a subduction thrust fault near the deformation front of the hikurangi subduction margin, new Zealand: A magnetic fabric study on IODP expedition 375 site U1518. Earth Planet. Sci. Lett. 542, 116322. doi: 10.1016/j.epsl.2020.116322
Johnson J. E., MacLeod D. R., Phillips S. C., Phillips M. P., Divins D. L. (2022). Primary deposition and early diagenetic effects on the high saturation accumulation of gas hydrate in a silt dominated reservoir in the gulf of Mexico. Mar. Geol. 444, 106718. doi: 10.1016/j.margeo.2021.106718
Kang D., Lu J., Zhang Z., Liang J., Kuang Z., Lu C., et al. (2020). Fine-grained gas hydrate reservoir properties estimated from well logs and lab measurements at the shenhu gas hydrate production test site, the northern slope of the south China sea. Mar. Petroleum Geol. 122, 104676. doi: 10.1016/j.marpetgeo.2020.104676
Ketzer M., Praeg D., Rodrigues L. F., Augustin A., Pivel M. A. G., Abkenar M. R., et al. (2020). Gas hydrate dissociation linked to contemporary ocean warming in the southern hemisphere. Nat. Commun. 11 (1), 3788. doi: 10.1038/s41467-020-17289-z
Konno Y., Yoneda J., Egawa K., Ito T., Jin Y., Kida M., et al. (2015). Permeability of sediment cores from methane hydrate deposit in the Eastern nankai trough. Mar. petroleum geol. 66, 487–495. doi: 10.1016/j.marpetgeo.2015.02.020
Lai H., Fang Y., Kuang Z., Ren J., Liang J., Lu J., et al. (2021). Geochemistry, origin and accumulation of natural gas hydrates in the qiongdongnan basin, south China Sea: Implications from site GMGS5-W08. Mar. Petroleum Geol. 123, 104774. doi: 10.1016/j.marpetgeo.2020.104774
Li M., Jian Z., Hassanpouryouzband A., Zhang L. (2022a). Understanding hysteresis and gas trapping in dissociating hydrate-bearing sediments using pore network modeling and three-dimensional imaging. Energy Fuels 36 (5), 10572–10582. doi: 10.1021/acs.energyfuels.2c01306
Li Y., Liu L., Jin Y., Wu N. (2021b). Characterization and development of natural gas hydrate in marine clayey-silt reservoirs: A review and discussion. Adv. Geo-Energy Res. 5 (1), 75–86. doi: 10.46690/ager.2021.01.08
Li N., Sun W., Li X., Feng Z., Wu H., Wang K. (2022). Gas hydrate saturation model and equation for logging interpretation. Petroleum Explor. Dev. 49 (6), 1243–1250. doi: 10.1016/S1876-3804(23)60346-5
Li M., Wu P., Zhou S., Zhang L., Yang L., Li Y., et al. (2021a). Permeability analysis of hydrate-bearing sediments during the hydrate formation process. Energy Fuels 35 (23), 19606–19613. doi: 10.1021/acs.energyfuels.1c02913
Li M., Zhou S., Wu P., Zhang L., Yang L., Li Y., et al. (2022b). Permeability analysis of hydrate-bearing sediments considering the effect of phase transition during the hydrate dissociation process. J. Natural Gas Sci. Eng. 97, 104337. doi: 10.1016/j.jngse.2021.104337
Liang J., Zhang W., Lu J., Wei J., Kuang Z., He Y., et al. (2019). Geological occurrence and accumulation mechanism of natural gas hydrates in the eastern qiongdongnan basin of the south China Sea: Insights from site GMGS5-W9-2018. Mar. Geol. 418, 106042. doi: 10.1016/j.margeo.2019.106042
Liu L., Zhang Z., Li C., Ning F., Liu C., Wu N., et al. (2020). Hydrate growth in quartzitic sands and implication of pore fractal characteristics to hydraulic, mechanical, and electrical properties of hydrate-bearing sediments. J. Natural Gas Sci. Eng. 75, 103109. doi: 10.1016/j.jngse.2019.103109
Lubo-Robles D., Bedle H., Marfurt K. J., Pranter M. J. (2023). Evaluation of principal component analysis for seismic attribute selection and self-organizing maps for seismic facies discrimination in the presence of gas hydrates. Mar. Petroleum Geol. 150, 106097. doi: 10.1016/j.marpetgeo.2023.106097
Macqueen J. (1967). Classification and analysis of multivariate observations. In 5th Berkeley Symp. Math. Statist. Probability (Los Angeles LA USA: University of California), pp. 281–297.
Meng M., Liang J., Lu J., Zhang W., Kuang Z., Fang Y., et al. (2021). Quaternary deep-water sedimentary characteristics and their relationship with the gas hydrate accumulations in the qiongdongnan basin, Northwest south China Sea. Deep sea Res. Part I: Oceanogr. Res. papers 177, 103628. doi: 10.1016/j.dsr.2021.103628
Nazemi M., Tavakoli V., Rahimpour-Bonab H., Sharifi-Yazdi M. (2021). Integrating petrophysical attributes with saturation data in a geological framework, permian–Triassic reservoirs of the central Persian gulf. J. Afr. Earth Sci. 179, 104203. doi: 10.1016/j.jafrearsci.2021.104203
Pan H., Li H. M., Chen J., Zhang Y., Cai S., Huang Y., et al. (2020). A unified contact cementation theory for gas hydrate morphology detection and saturation estimation from elastic-wave velocities. Mar. Petroleum Geol. 113, 104146. doi: 10.1016/j.marpetgeo.2019.104146
Ren J., Cheng C., Xiong P., Kuang Z., Liang J., Lai H., et al. (2022). Sand-rich gas hydrate and shallow gas systems in the qiongdongnan basin, northern south China Sea. J. Petroleum Sci. Eng. 215, 110630. doi: 10.1016/j.petrol.2022.110630
Riedel M., Collett T. S., Kim H. S., Bahk J. J., Kim J. H., Ryu B. J., et al. (2013). Large-Scale depositional characteristics of the ulleung basin and its impact on electrical resistivity and Archie-parameters for gas hydrate saturation estimates. Mar. Petroleum Sci. 47, 222–235. doi: 10.1016/j.marpetgeo.2013.03.014
Schapire R. E. (1990). The strength of weak learnability. Mach. Learn. 5 (2), 197–227. doi: 10.1007/BF00116037
Singh R. P., Lall D., Vishal V. (2022). Prospects and challenges in unlocking natural-gas-hydrate energy in India: Recent advancements. Mar. Petroleum Sci. 135, 105397. doi: 10.1016/j.marpetgeo.2021.105397
Snyder G. T., Sano Y., Takahata N., Matsumoto R., Kakizaki Y., Tomaru H. (2020). Magmatic fluids play a role in the development of active gas chimneys and massive gas hydrates in the Japan Sea. Chem. Geol. 535 (5), 119462. doi: 10.1016/j.chemgeo.2020.119462
Song Y., Wang S., Cheng Z., Huang M., Zhang Y., Zheng J., et al. (2021). Dependence of the hydrate-based CO2 storage process on the hydrate reservoir environment in high-efficiency storage methods. Chem. Eng. J. 415, 128937. doi: 10.1016/j.cej.2021.128937
Su M., Luo K., Fang Y., Kuang Z., Yang C., Liang J., et al. (2021). Grain-size characteristics of fine-grained sediments and association with gas hydrate saturation in shenhu area, northern south China Sea. Ore Geol. Rev. 129, 103889. doi: 10.1016/j.oregeorev.2020.103889
Tavakoli V., Hassani D., Rahimpour-Bonab H., Mondak A. (2022). How petrophysical heterogeneity controls the saturation calculations in carbonates, the barremian–aptian of the central Persian gulf. J. Petroleum Sci. Eng. 208, 109568. doi: 10.1016/j.petrol.2021.109568
Tavakoli V., Jamalian A. (2019). Porosity evolution in dolomitized permian–Triassic strata of the Persian gulf, insights into the porosity origin of dolomite reservoirs. J. Petroleum Sci. Eng. 181, 106191. doi: 10.1016/j.petrol.2019.106191
Wan Z., Chen C., Liang J., Zhang W., Huang W., Su P. (2020). Hydrochemical characteristics and evolution mode of cold seeps in the qiongdongnan basin, south China sea. Geofluids 2020, 4578967. doi: 10.1155/2020/4578967
Wan X., Zhou X., Liang J., Wu S., Lu J., Wei C., et al. (2022). Well-logging constraints on gas hydrate saturation in unconsolidated fine-grained reservoirs in the northern south China Sea. Energies 15 (23), 9215. doi: 10.3390/en15239215
Wang D., Ning F., Lu J., Lu H., Kang D., Xie Y., et al. (2021). Reservoir characteristics and critical influencing factors on gas hydrate accumulations in the shenhu area, south China Sea. Mar. Petroleum Geol. 133, 105238. doi: 10.1016/j.marpetgeo.2021.105238
Wang S., Cheng Z., Zhang Y., Jiang L., Liu Y., Song Y. (2021). Unstable density-driven convection of CO2 in homogeneous and heterogeneous porous media with implications for deep saline aquifers. Water Resour. Res. 57 (3), e2020WR028132. doi: 10.1029/2020WR028132
Wang X., Feng J., Zhang L., Yuan Y., Xu C., Liang J., et al. (2022). Mineralogy and pore characteristics of marine gas hydrate-bearing sediments in the northern south China Sea. Mar. Petroleum Geol. 141, 105711. doi: 10.1016/j.marpetgeo.2022.105711
Wei J., Fang Y., Lu H., Lu H., Lu J., Liang J., et al. (2018). Distribution and characteristics of natural gas hydrates in the shenhu Sea area, south China Sea. Mar. Petroleum Geol. 98, 622–628. doi: 10.1016/j.marpetgeo.2018.07.028
Wei J., Liang J., Lu J., Zhang W., He Y. (2019). Characteristics and dynamics of gas hydrate systems in the northwestern south China Sea— results of the fifth gas hydrate drilling expedition. Mar. Petroleum Geol. 110, 287–298. doi: 10.1016/j.marpetgeo.2019.07.028
Wei J., Wu T., Zhu L., Fang Y., Liang J., Lu H., et al. (2021). Mixed gas sources induced co-existence of sI and sII gas hydrates in the qiongdongnan basin, south China Sea. Mar. Petroleum Geol. 128, 105024. doi: 10.1016/j.marpetgeo.2021.105024
Winters W., Walker M., Hunter R., Collett T., Boswell R., Rose K., et al. (2011). Physical properties of sediment from the mount Elbert gas hydrate stratigraphic test well, Alaska north slope. Mar. Petroleum Geol. 28, 361–380. doi: 10.1016/j.marpetgeo.2010.01.008
Wu P., Yang S., Song X., Sun X., Li Y. (2021). Influence of average grain size distribution on the physical characteristics of cementing hydrate-bearing sediment. Energy Rep. 7, 8187–8197. doi: 10.1016/j.egyr.2021.07.014
Xie Y., Zhang G., Sun Z., Zeng Q., Zhao Z., Guo S. (2019). Reservoir forming conditions and key exploration technologies of lingshui 17-2 giant gas field in deepwater area of qiongdongnan basin. Petroleum Sci. 4 (1), 1–18. doi: 10.1016/j.ptlrs.2019.01.004
Ye J., Wei J., Liang J., Lu J., Lu H., Zhang W., et al. (2019). Complex gas hydrate system in a gas chimney, south China Sea. Mar. Petroleum Geol. 104, 29–39. doi: 10.1016/j.marpetgeo.2019.03.023
Yu J., Zhu L., Qin R., Zhang Z., Li L., Huang Y. (2021). Combining K-means clustering and random forest to evaluate the gas content of coalbed bed methane reservoirs. Geofluids 2021, 9321565. doi: 10.1155/2021/9321565
Zhang W., Liang J., Qiu H., Deng W., Meng M., He Y., et al. (2022a). Double bottom simulating reflectors and tentative interpretation with implications for the dynamic accumulation of gas hydrates in the northern slope of the qiongdongnan basin, south China Sea. J. Asian Earth Sci. 229, 105151. doi: 10.1016/j.jseaes.2022.105151
Zhang W., Liang J., Su P., Wei J., Gong Y., Lin L., et al. (2018). Distribution and characteristics of mud diapirs, gas chimneys, and bottom simulating reflectors associated with hydrocarbon migration and gas hydrate accumulation in the qiongdongnan basin, northern slope of the south China Sea. Geol. J. 54, 3556–3573. doi: 10.1002/gj.3351
Zhang W., Liang J., Wei J., Lu J., Su P., Lin L., et al. (2020). Geological and geophysical features of and controls on occurrence and accumulation of gas hydrates in the first offshore gas-hydrate production test region in the shenhu area, northern south China Sea. Mar. Petroleum Geol. 114, 104191. doi: 10.1016/j.marpetgeo.2019.104191
Zhao Z., Sun Z., Liu J., Gussinyé M. P., Zhuo H. (2018). The continental extension discrepancy and anomalous subsidence pattern in the western qiongdongnan basin, south China Sea. Earth Planet. Sci. Lett. 501, 180–191. doi: 10.1016/j.epsl.2018.08.048
Zhu L., Ma Y., Cai J., Zhang C., Wu S., Zhou X. (2022a). Key factors of marine shale conductivity in southern China–part II: The influence of pore system and the development direction of shale gas saturation models. J. Petroleum Sci. Eng. 209, 109516. doi: 10.1016/j.petrol.2021.109516
Zhu L., Sun J., Zhou X., Li Q., Fan Q., Wu S., et al. (2022b). Well logging evaluation of fine-grained hydrate-bearing sediment reservoirs: Considering the effect of clay content. Petroleum Sci. doi: 10.1016/j.petsci.2022.09.018
Zhang C., Misra S., Zhou X., Cai J. (2023a). Characterization of pore electrical conductivity in porous media by weakly conductive and nonconductive pores. Surv. Geophys. doi: 10.1007/s10712-022-09761-w
Keywords: gas hydrate, reservoir classification, K-means (KM) clustering, Adaboost,, Qiongdongnan area
Citation: Zhu L, Zhou X, Sun J, Liu Y, Wang J and Wu S (2023) Reservoir classification and log prediction of gas hydrate occurrence in the Qiongdongnan Basin, South China Sea. Front. Mar. Sci. 10:1055843. doi: 10.3389/fmars.2023.1055843
Received: 26 October 2022; Accepted: 23 March 2023;
Published: 23 May 2023.
Edited by:
Hideyuki Kanematsu, Suzuka College, JapanReviewed by:
Vahid Tavakoli, University of Tehran, IranPibo Su, Guangzhou Marine Geological Survey, China
Copyright © 2023 Zhu, Zhou, Sun, Liu, Wang and Wu. This is an open-access article distributed under the terms of the Creative Commons Attribution License (CC BY). The use, distribution or reproduction in other forums is permitted, provided the original author(s) and the copyright owner(s) are credited and that the original publication in this journal is cited, in accordance with accepted academic practice. No use, distribution or reproduction is permitted which does not comply with these terms.
*Correspondence: Shiguo Wu, swu@idsse.ac.cn