- 1Liaoning Key Laboratory of Marine Animal Immunology and Disease Control, Dalian Ocean University, Dalian, China
- 2Liaoning Key Laboratory of Marine Animal Immunology, Dalian Ocean University, Dalian, China
- 3Laboratory of Marine Fisheries Science and Food Production Process, Qingdao National Laboratory for Marine Science and Technology, Qingdao, China
- 4Dalian Key Laboratory of Aquatic Animal Disease Prevention and Control, Dalian Ocean University, Dalian, China
Bacteria in coastal waters drive global biogeochemical cycling and are strongly related to coastal environmental safety. The bacterial community in offshore shellfish farming waters of North China has its own characteristics and particularities, while the knowledge is limited. In this study, the bacterial community characteristics, the particularities of bacterial community in the waters with surface cold patches (SCPs) and the variation of pathogenic bacteria were investigated in the offshore shellfish farming waters in the North Yellow Sea (NYS) from 2017 to 2019. For all studied samples, Desulfobacterales acted as the keystone species taxon in microbial co-occurrence networks, and the proportional abundance of Actinobacteriota was found to be as low as 1.3%. The abundance of Marinobacter and Synechococcus was remarkably prominent in 13 genera with nitrogen-transforming function. The top two different bacterial functions in the spatial analysis (between the waters with SCPs and the ambient waters) were xenobiotics biodegradation and metabolism and metabolism of cofactors and vitamins, which were same with that in the seasonal analysis (between spring and summer). The abundance differences of most pathogenic bacteria analyzed in this study (11 out of 12 genera) also had the same variation dynamics between the spatial analysis and the seasonal analysis. An ANN predictive model for Vibrio abundance was constructed for Vibrio forecasting, with acceptable predictive accuracy. According to the above results, the bacterial community in the shellfish aquaculture waters in this study was characterized by the enhancing ability of nitrogen removal. Temperature was concluded as the predominant environmental factor to drive the variation of bacterial community function and pathogenic bacteria patterns in the offshore shellfish farming waters with SCPs. The results of this study will further our understanding of the bacterial community characteristics in offshore shellfish farming waters, and help for Vibrio forecasting and coastal environmental safety in aquaculture seawater.
Introduction
Bacteria are important components in the marine ecosystem, driving global biogeochemical cycling (Falkowski et al., 2008). They play important roles in maintenance and sustainability of microbial food webs and contribute to the rapid adjustment towards marine environmental changes and deterioration (Dash et al., 2013). The marine planktonic bacteria, especially those distributed near the coast, are of importance in people’s life due to their close relationships to human and animal’s health, industry and tourism, as about 60% of the world’s population residing within 100 km of the coast (Vitousek et al., 1997).
Shellfish have been considered as “keystone species” and “ecosystem engineers” in coastal environment (Gutiérrez et al., 2003; Newell, 2004). The shellfish aquaculture industry, which is always located near the coast, has greatly developed in recent years to meet the growing global demand for protein (eg. 17.7 million tons in 2018) and contribute to world economic development (FAO, 2020). Shellfish are known to modify microbial assemblages by filtering water and transferring nutrients in the water system. On one hand, shellfish aquaculture can reduce water turbidity and improve nutrient recycling in anthropogenic impacted coastal environment, thus acting as an excellent nutrient bioextraction system in eutrophic areas. On the other hand, the suspension filtering and deposition through shellfish aquaculture are always involved in nutrient flux regulation and plankton speciation, which sometimes stimulates the growth of red tide species of plankton (Dumbauld et al., 2009; He et al., 2017). The increasing farming areas and stocking density have raised considerable concern, due to their influence on the marine environment (Yuan et al., 2010; Han et al., 2013). Even though there were some reports about the microbiome in the coastal environment, the bacterial community dynamics concluded from different studies are not always in strong agreement, because of different biogeographic and environmental features (Hartwick et al., 2019). The effect of shellfish aquaculture on the microbial community is quite complex, and the understanding of bacterial community characteristics in offshore shellfish farming waters is very limited.
The North Yellow Sea (NYS) is closed to a continental margin of major economic importance in China and Korea with unique oceanographic characteristics. Shellfish aquaculture is an important industry in the NYS and plays critical roles in the development of the marine economy. It is located near the coastal areas, especially around the islands off the coast, rearing Yesso scallop (Patinopecten yessoensis), Pacific oyster (Crassostrea gigas) and bay scallop (Argopecten irradians irradians), etc. In recent years, aquaculture and industrial wastes run-off and atmospheric deposition also affected the nutrient concentrations in the NYS, further leading to the change of microbial community and dynamics and even hypoxic dead zones (Lin et al., 2005; Tang, 2009). The NYS is always considered as one of the most complicated continental sea areas in the world, due to the seasonal variation of strong currents, wind stress and nutrient-enriched freshwater outflows in the shallow sea area (Hur et al., 1999). The Yellow Sea is also well known because of the Yellow Sea Cold Water Mass, which is a basin-scale water mass of relatively low temperature lying under the seasonal thermocline. Surface cold patches (SCPs) could be observed scattering around the Yellow Sea Cold Water Mass in boreal summer, in contrast to the ambient waters with relative higher sea surface temperature (Xia and Guo, 1983; Zou et al., 2001). The environmental particularity of SCPs was speculated to result in different bacterial communities in shellfish farming waters, while the principal environmental parameter involved in this was not clear. The dynamics of bacterial community variation in the NYS or even in its offshore shellfish farming waters are attracting increasing interest in recent years.
Pathogenic bacteria in coastal waters are strongly related to the health of human and animals. The environmental problems caused by microbiome change and the infections caused by pathogenic bacteria have been reported frequently in shellfish farming waters (Azandégbé et al., 2012; He et al., 2017). Among various potential pathogenic bacteria, the prevalence patterns of Vibrio spp. in marine environment are attracting widespread concern, since they include some pathogenic species that could infect to human and other organisms, such as V. parahaemolyticus and V. vulnificus. Pathogenic species of Vibrio spp. in seawater can cause seafood-borne illnesses through consumption of contaminated seafood and cause wound infections by exposure of an open wound to seawater, probably resulting in diarrhea, septicemia and even death (Hsieh et al., 2008). The forecasting of Vibrio abundance and breakout would help to prevent human illness by identifying and reporting the forecasting information and making it publicly available (Froelich et al., 2013; Izumiya et al., 2017).
In short, investigating the characteristics and dynamics of bacterial community variation and potentially pathogenic bacteria in the offshore shellfish farming waters in the NYS would contribute to coastal environmental safety. To achieve this, in the present study, seawater samples were collected from two offshore shellfish farming areas (the waters with SCPs and the ambient waters) in the NYS from every March to August in 2017-2019 with the aims to (1) illuminate the characteristics of bacterial community in the offshore shellfish farming waters and its seasonal dynamics; (2) reveal the particularities of bacterial community in the waters with SCPs; (3) analyze the variation patterns of pathogenic bacteria abundance and develop a predictive model for Vibrio abundance level.
Material and methods
Sample collection
Two sampling regions (location X and location Z) were set for the offshore Yesso scallop farming waters in the NYS (Figure S1). The geographic coordinates of location X and Z are N39°17’44”–E122°40’38” and N39°1’7”–E122°42’2”, respectively. According to Lü et al. (2010), the location X could be considered as within SCPs and the location Z was among the ambient region with relative higher sea surface temperature, because our three-year data also showed that the surface water temperature in summer in location X was generally 2-3°C lower than that in location Z. There were three sampling sites included in each region as three parallels. The sampling experiments were carried out from March to August in 2017-2019, as March to August was the main period of scallop farming. In this study, spring was defined from March to May, and summer from June to August. Seawater samples were collected at 3 m of depth where scallops were suspension-cultured and were then stored at 4°C within 1 h before further processing as previous description (Yu et al., 2019a; Yu et al., 2019b).
Determination of water parameters
Water temperature (T), salinity (Sal), pH and dissolved oxygen (DO) were monitored using an YSI Professional Plus meter (YSI, Yellow Springs, Ohio, USA) in situ. According to the procedures of the National Specification for Marine Monitoring (SOA of China, 2007), the concentration of TAN, NO2–N, NO3–N, PO4-P and SiO4-Si of the water were analyzed using indophenol blue spectrophotometric method, N-(1-naphthyl)-ethylenediamine dihydrochloride spectrophotometric method, Zn-Cd reduction method, Phosphomolybdenum blue spectrophotometric method and Silicon molybdenum yellow Spectrophotometry method, respectively. The concentration of Chl-a was measured with spectrophotometry after extraction with acetone (Lorenzen, 1967).
DNA extraction and high−throughput sequencing
One liter of seawater was filtered using 0.22 μm pore size membranes (Sagon, Shanghai, China) to enrich the microbial cells for each sample, which was then used for genomic DNA extraction using the Water DNA Kit (Omega, GA, USA) following the manufacturer’s instruction. DNA quality and quantity were analyzed by 1% agarose gel electrophoresis and NanoDrop spectrophotometer (Thermo Fisher Scientific, DE, USA). The high-throughput sequencing of the V3–V4 hypervariable region of 16S rDNA genes was performed using the Illumina HiSeq platform by Novogene (Beijing, China) with low-quality reads filtered.
Amplicon sequence analysis
The raw reads of the 16S rDNA sequence were processed using Quantitative Insights into Microbial Ecology (QIIME 2) pipeline (Bolyen et al., 2019). Paired-end reads were imported, then trimmed and denoised using DADA2 to remove chimeras and obtain amplicon sequence variants (ASVs). The abundance of nitrogen-transforming bacteria was calculated as log10 (relative abundance proportion * 10000 + 1). Taxonomy was assigned at the single nucleotide level to the ASVs using a feature classifier against a trained SILVA 138 SSU database. Alpha diversity and beta diversity were evaluated using the Shannon diversity index and unweighted UniFrac distances, respectively.
Statistical analysis
MicrobiomeAnalyst (http://www.microbiomeanalyst.ca) was used to compare the abundance and diversity of the bacterial community and to generate visual exploration, with ASV data and the metadata files (Chong et al., 2020). The functional profiles and metabolic pathways of the bacterial communities were predicted using Phylogenetic Investigation of Communities by Reconstruction of Unobserved States (PICRUSt) 2 software (Douglas et al., 2020). KEGG database was used for the annotation of predicted genes. STAMP software was used for differential analysis of functional profiles (Parks et al., 2014). The co-occurrence ecological network was analyzed using the online Molecular Ecological Network Analysis (MENA) pipeline (http://ieg2.ou.edu/MENA) (Deng et al., 2012), and the network construction was performed using Cytoscape software (Shannon et al., 2003). Redundancy analysis (RDA) was conducted to reveal the effect of environmental factors on the abundance and diversity of bacterial communities using CANOCO software (version 5) (Šmilauer and Lepš, 2014). Correlations between the Vibrio abundance and environmental factors were investigated through Spearman’s correlation analysis and Pearson’s correlation analysis using SPSS 26 (SPSS Inc. Chicago, IL) statistical software package. P-value<0.05 was considered statistically significant. P-value<0.01 was considered extremely significant.
Construction of the predictive model
A predictive model was constructed for the level of Vibrio abundance in this study. First, the co-occurrence relationships between the Vibrio abundance and the bacterial abundance at order level were investigated using MENA pipeline to get the order taxa (VAO) that were directly associated with the Vibrio variation. Second, all tested environmental factors and the VAO abundance were analyzed for their correlation with the Vibrio abundance in the next month using Spearman’s correlation analysis and Pearson’s correlation analysis, to get the factors (VAF, including environmental factors and order taxa) that had significant associations with Vibrio variation in the next month in both results of correlation analysis. Third, three levels of Vibrio relative abundance were set as High Level (proportional abundance ≥ 2%), Moderate Level (2% > proportional abundance ≥ 0.5%), and Low Level (proportional abundance< 0.5%). The multi-layer feed-forward artificial neural network (ANN) modeling was used to construct and test the neural networking by SPSS 26, using the VAFs as input variables and the Vibrio level in the next month as output variable. The feasible ANN model was constructed by trial and error. The ANN was classified into three layers, including input, hidden and output layers. The three layers processed signals and searches to obtain the best linear and nonlinear relationships between the input and output data. In this model, about 70% of the input data was used for training, with the remaining 30% used for testing. Levenberg–Marquardt algorithm was applied for modeling and calculating the weights among the input, hidden and output layers through modifying the learning rate and the number of hidden layers and neurons.
Results
Composition, function and co-occurrence of bacterial community
High-throughput sequencing of 16S rDNA was performed to obtain 12,988,676 sequences, which were clustered into 16,747 ASVs. A total of 415 orders across 56 phyla in all samples were detected through direct taxonomical classification and annotation. Proteobacteria (58.6%), Bacteroidetes (18.6%), Cyanobacteria (12.3%), Firmicutes (3.4%) and Verrucomicrobiota (2.0%) were dominant phyla in seawater accounting for 95.0% of the total abundance (Figure 1A). The average proportional abundance of Actinobacteriota was 1.3%. The abundance of nitrogen-transforming bacteria was investigated, and 13 genera were found in our samples (Figure 2). Among them, Marinobacter and Synechococcus were consistently present in all samples, and their abundance was markedly higher than that of the other nitrogen-transforming bacteria.
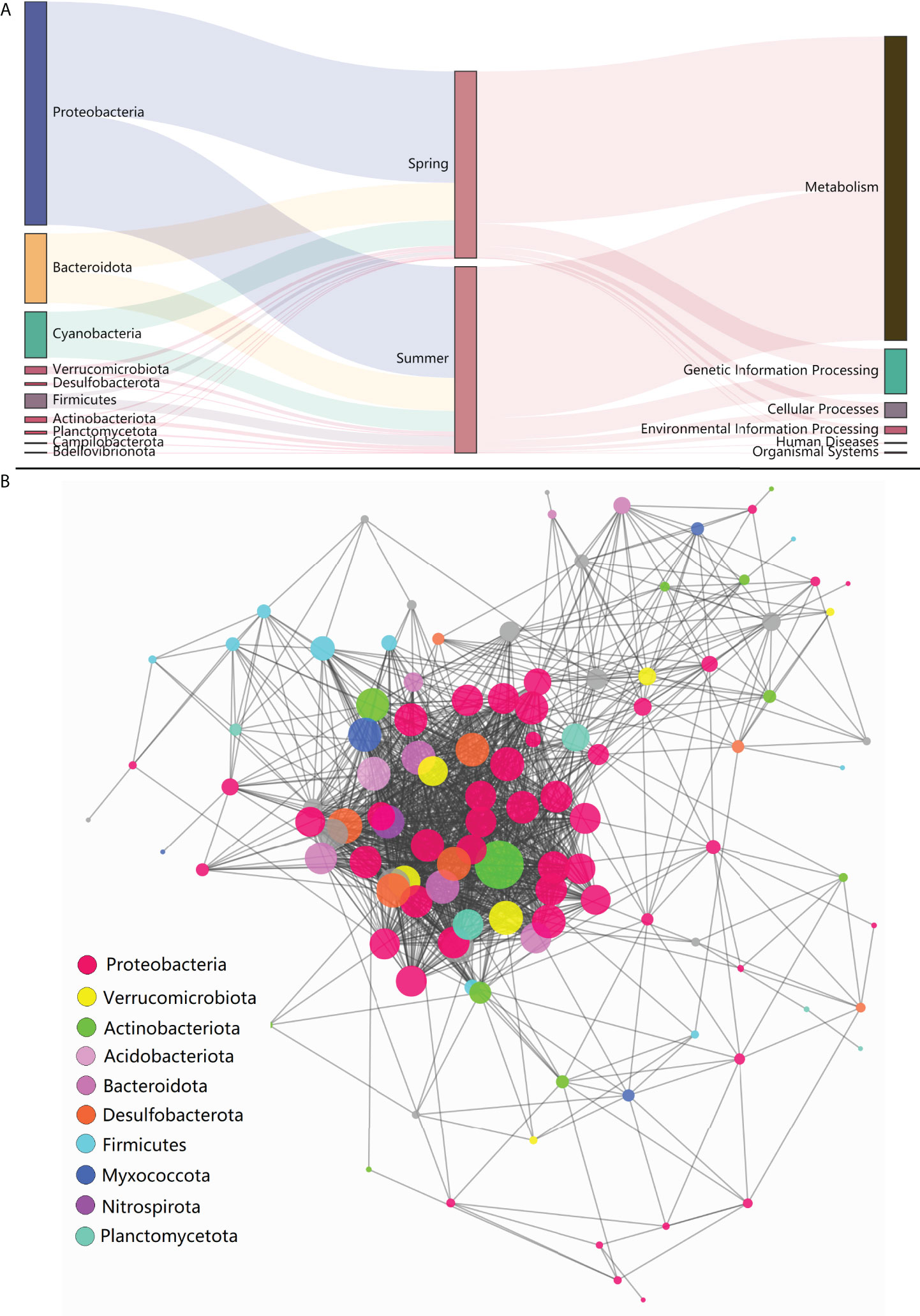
Figure 1 Composition, function and co-occurrence of bacterial community. (A) Distribution profiles of phylum taxa and functional pathways of the bacterial community; (B) Microbial co-occurrence networks at phylum-order level.
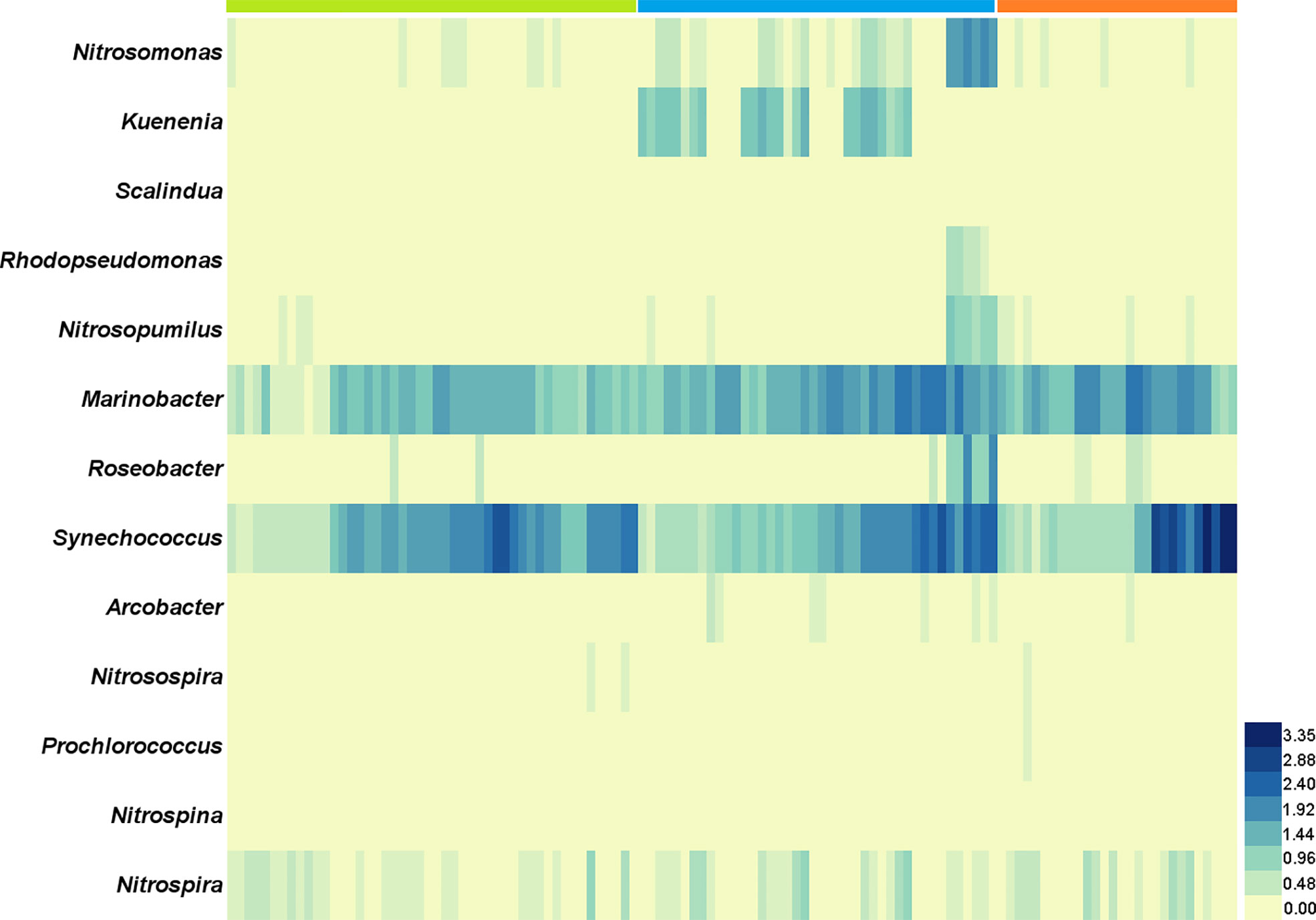
Figure 2 The abundance variation of 13 genera of nitrogen-transforming bacteria in the offshore shellfish farming waters. The x-axis is samples in chronological order. Green: 2017; blue: 2018; orange: 2019.
The KEGG pathways that the bacterial community involved were predicted to explore the potential biosynthetic and ecological functions. By searching against the KEGG database using PICRUSt2, a total of 188 third-level pathways were identified, including Valine, leucine and isoleucine biosynthesis, C5-Branched dibasic acid metabolism and Biosynthesis of ansamycins, etc. After grouping into second-level pathways, 36 pathways were determined, including carbohydrate metabolism, infectious disease and replication and repair, etc. For the further grouped first-level pathways, six types were identified (Figure 1A). The co-occurrence analysis was performed to investigate the interaction relationships among specific taxa and to construct the microbial co-occurrence networks. A total of 114 orders (belong to 22 phyla) were included in the network construction (Figure 1B). In general, complex co-occurrence relationships were showed for the whole bacterial community in this result. Among all orders listed in this work, the orders in Proteobacteria processed dominate positions in co-occurrence relationship, which occupied 48.1% of the co-occurrence degree in the whole microbiota. Desulfobacterales was suggested to be the keystone species taxon, as it was most directly associated among all orders in this network.
For the composition variation of bacterial community between spring and summer, some apparent patterns could be observed among different years, indicating that bacterial compositions differed in interannual patterns (Figure 3A). In the PCA analysis of bacterial beta diversity, the bacterial community differed obviously between spring and summer (Figure 3B). This difference could be investigated more clearly in the analysis among different months. Progressive changes could be outlined in bacterial community patterns from March to August (Figure 3B). Microbiota comparisons were conducted between spring and summer by LEfSe analysis. Firmicutes and Bacteroidota were identified as discriminative features at phylum level (Figure 3C). Seven genus taxa were identified as discriminative features at genus level, in which Vibrio genus was found to be significantly abundant in summer (Figure 3C). The variation of bacterial functional patterns was pronounced at seasonal scales, and the seasonal variation could be discriminated from PCA analysis (Figure 3D). The differently predicted pathways between spring and summer were mainly related to metabolism and cell functional pathways, in which the top two different pathways were xenobiotics biodegradation and metabolism and metabolism of cofactors and vitamins (Figure 3E). The pathways related to infectious disease were also found to be differently enriched between spring and summer.
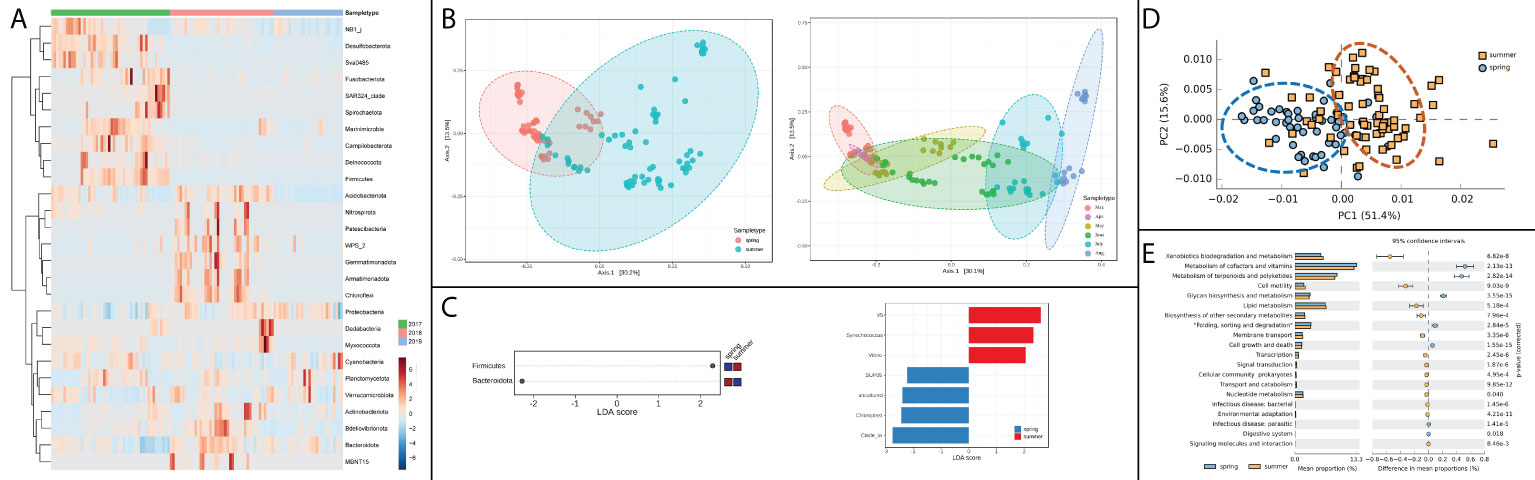
Figure 3 Temporal variation of bacterial community and function in shellfish farming areas. (A) Annual abundance heatmap at phylum level; (B) Seasonal (left) and monthly (right) variation of alpha diversity; (C) Discriminative features of bacterial community at phylum level (left) and genus level (right); (D) Principal components analysis (PCA) of bacterial functional characterization; (E) The functional pathways in significantly temporal variation patterns.
The composition and function characteristics of bacterial community in the waters with SCPs
The difference in alpha diversity was non-significant between the two locations (Figure 4A). Differences could be found in some of the most dominant phyla between locations, that the samples from location X had a higher relative abundance of Bacteroidota and Actinobacteriota, and lower relative abundance of Firmicutes (Figure 4B). The differences became even more pronounced in specific seasons or months. For instance, in summer, the variation of six phyla, such as Actinobacteriota, Planctomycetota and Patescibacteria, etc., had significantly different patterns between the two locations (Figure 4C). When further focused on the situation of August, Actinobacteriota was identified as the only discriminative feature at phylum level between the two locations by LEfSe analysis. The functional patterns of the bacterial community did not differ markedly between locations, but still showing a few differences that could be observed in PCA analysis on PC1 axis, which explained 51.4% of the total variation (Figure 4D). The differently predicted pathways between locations were mainly enriched in metabolism pathways, in which the top two different pathways were xenobiotics biodegradation and metabolism and metabolism of cofactors and vitamins (Figure 4E).
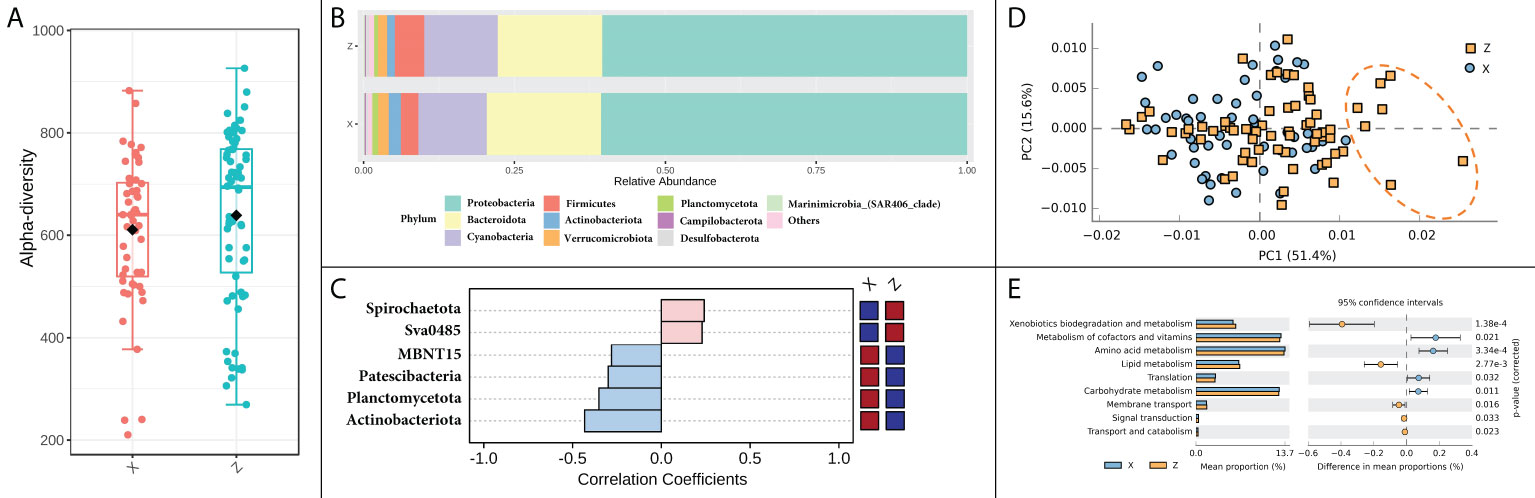
Figure 4 Spatial variation of bacterial community and function in shellfish farming areas. (A) Bacterial alpha diversity; (B) Bacterial relative abundance in phylum level; (C) The phyla in significantly spatial variation patterns. (D) Principal components analysis (PCA) of bacterial functional characterization; (E) The functional pathways in significantly spatial variation patterns.
Variation patterns of pathogenic bacteria abundance
During the sampling period of different months, the Vibrio abundance generally increased with warming temperature (Figure 5). In order to fully investigate the seasonal variation of potential pathogenic bacteria, the abundance of 12 genera of common pathogenic bacteria were analyzed (Figure 6). For the comparison between spring and summer, the average abundance of five genera were higher in spring than in summer (fold of (log10 abundance): 1.02-2.70), including Shewanella, Pseudomonas, Streptococcus, Campylobacter and Escherichia. The average abundance of the other seven genera were lower in spring than in summer (fold of (log10 abundance): 0.34-0.97), including Vibrio, Aeromonas, Photobacterium, Francisella, Staphylococcus, Clostridium and Legionella. For the comparison between the two locations, the average abundance of four genera were higher in location X than in location Z (fold of (log10 abundance): 1.02-1.41), including Shewanella, Streptococcus, Campylobacter and Escherichia. The average abundance of eight genera were lower in location X than in location Z (fold of (log10 abundance): 0.51-0.99), including Vibrio, Pseudomonas, Aeromonas, Photobacterium, Francisella, Staphylococcus, Clostridium and Legionella.
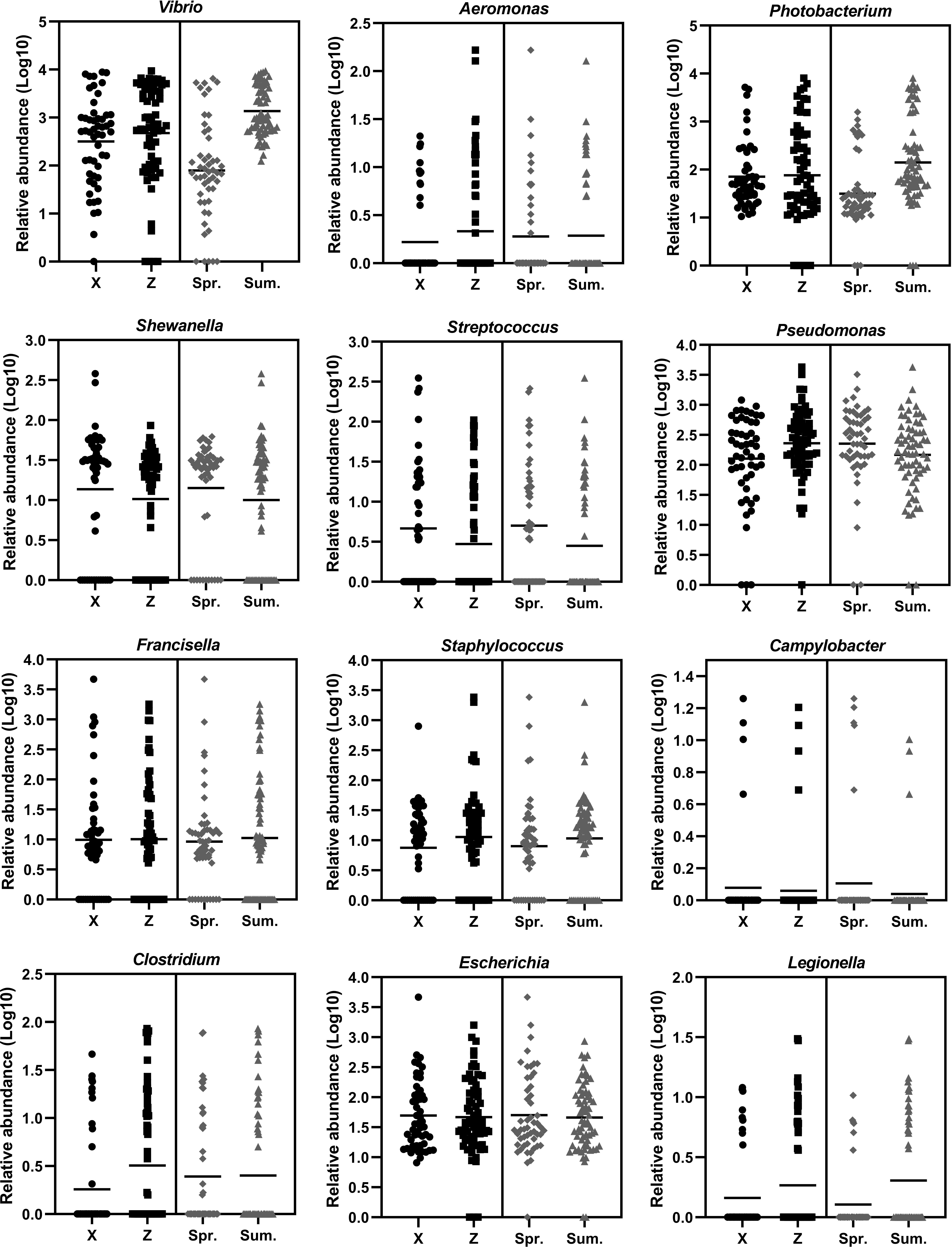
Figure 6 The temporal and spatial variation of the abundance of 12 genera of potential pathogenic bacteria. X indicates location X. Z indicates location Z. Spr. indicates spring. Sum. indicates summer.
Relationships between environmental factors and bacterial community
The relationships between the environmental factors and bacterial community were analyzed and were presented in the RDA biplot (Figure 7A). The first and second RDA axes explained 45.88% and 12.14% of the total variation, respectively. The top three significant environmental factors that constrained the bacterial community were DO, T and Chl-a, with the explaining rate of 33.6%, 33.4% and 28.3%, respectively. T and Chl-a had a strong negative influence on the abundance of most phyla taxa located in the upper right quadrant and the lower right quadrant, while DO had a strong positive influence instead. WPS-2 generally had an opposite relationship with environmental factors compared to some other phyla that had a positive relationship with DO and a negative relationship with T and Chl-a. T and DO almost had no influence on the abundance of SAR324. For specific pairs of environmental factors, T and DO had opposite influences on the bacterial community, while Chl-a and SiO4 had similar influences on the bacterial community. Through Spearman’s correlation analysis and Pearson’s correlation analysis, the environmental factors that were significantly associated with the Vibrio abundance in both analyses were identified, including T, DO, Chl-a, SiO4, PO4 and Si/N (Figure 7B). The environmental factors that had correlation coefficients above 0.5 in both analyses were T and SiO4.
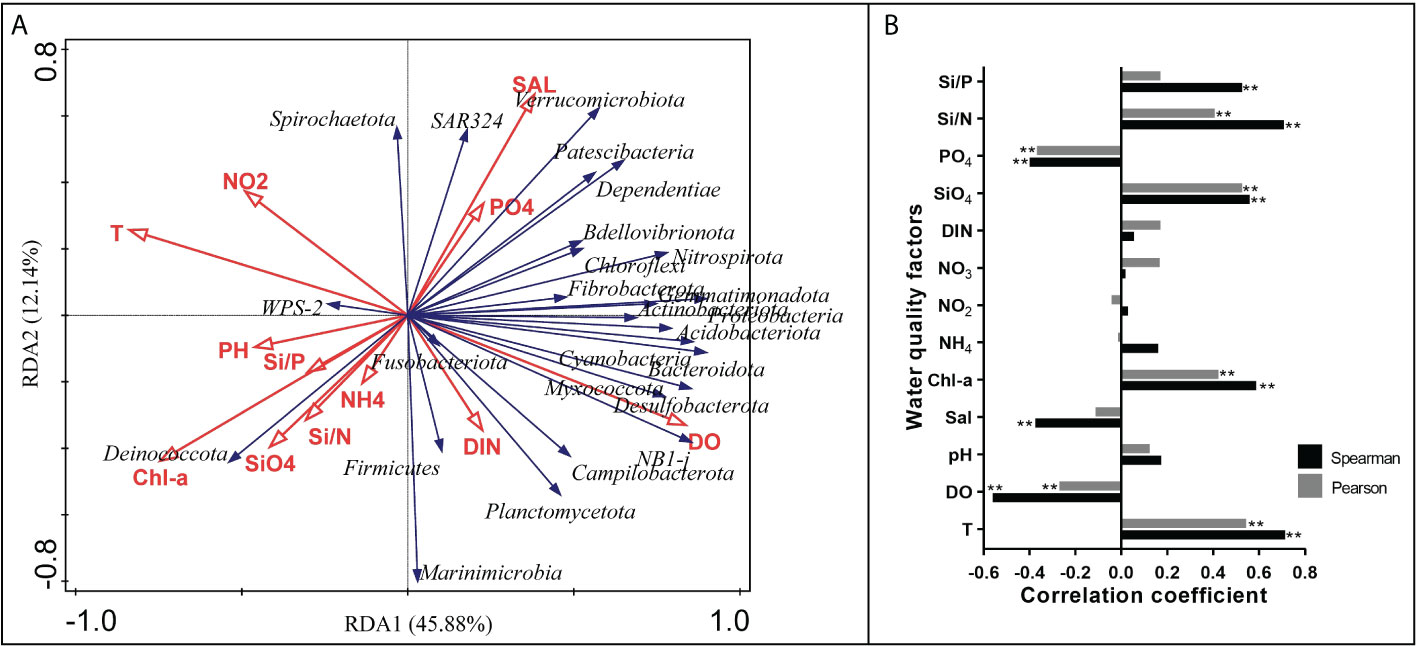
Figure 7 Relationships between environmental factors and bacterial community. (A) RDA ordination plot for the first two principal dimensions of the relationships between the phylum taxa abundance and environmental factors in shellfish farming areas. (B) The correlation between Vibrio abundance and environmental factors using Spearman’s correlation analysis and Pearson’s correlation analysis. “**” represent an extremely significant difference (P<0.01).
Prediction for Vibrio abundance level
Before the construction of ANN model, 41 VAOs were found to be directly associated with the Vibrio abundance. After the correlation analysis, 32 VAFs were found to be significantly associated with the Vibrio abundance in the next month. One hidden layer and 8 neurons in the hidden layer were finally used for the ANN model training, with structure exhibition in Figure 8A. The predictive accuracy was shown in Table S1. The mean predictive accuracy for training samples was 95.8%, with each sub-accuracy above 85%. The mean predictive accuracy for testing samples was 96.4%, with each sub-accuracy above 85%. The prediction probability can also be seen in Figure 8B, which showed acceptable performance of the ANN model for the Vibrio abundance level prediction.
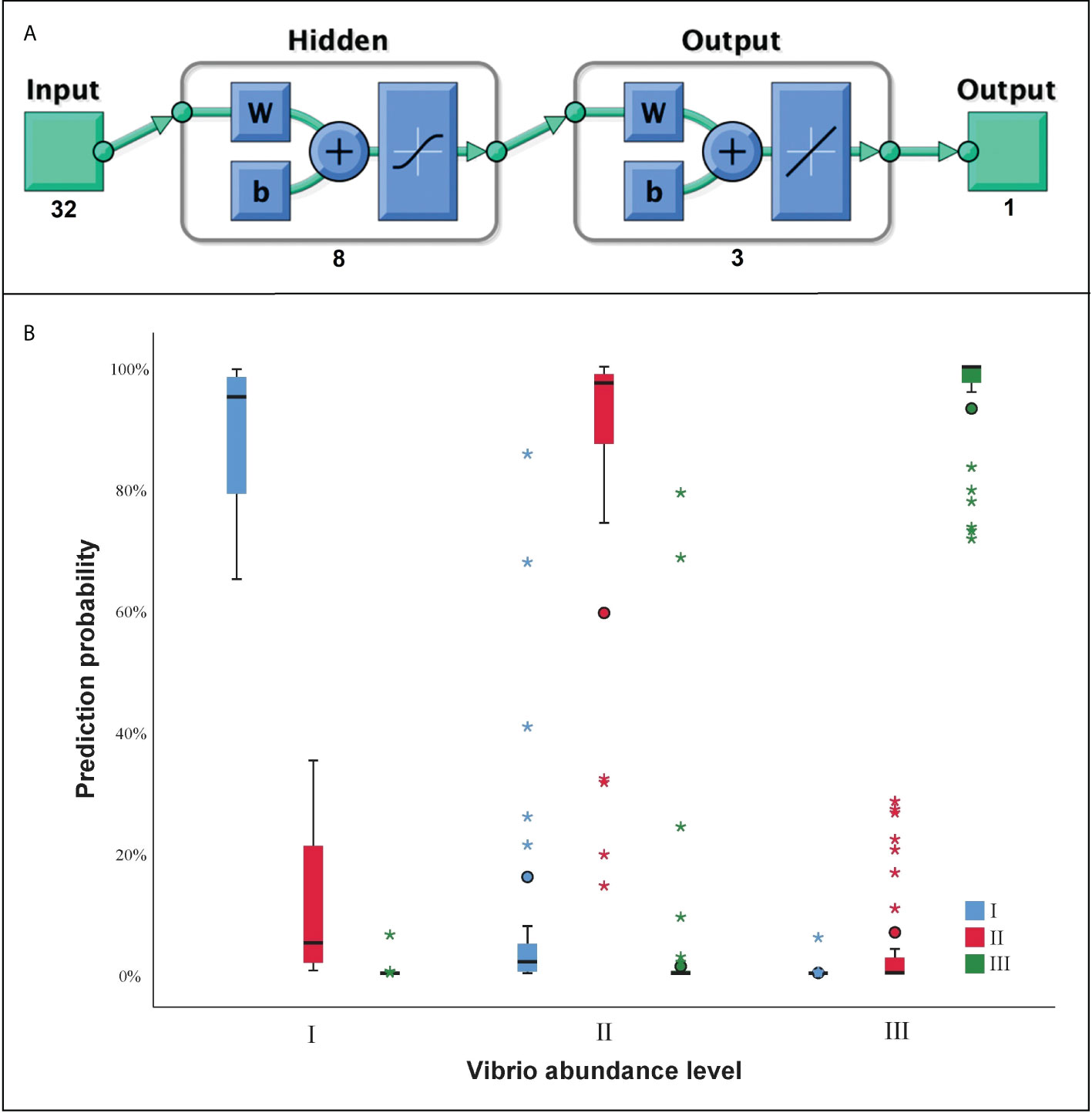
Figure 8 The artificial neural network (ANN) for Vibrio abundance prediction. (A) The structure of ANN layer; (B) The prediction probability of ANN model at three abundance level of Vibrio. “I, II, III” indicate the three levels of prediction.
Discussion
Characteristics of bacterial community in the offshore shellfish farming waters and its seasonal dynamics
The bacterial phyla dominant in our samples were investigated and were generally consistent with previous studies, in which Proteobacteria, Bacteroidetes, Cyanobacteria and Actinobacteriota, etc. were characterized as the top ubiquitous phyla in the Yellow Sea or other adjacent sea areas (Yu et al., 2018; Kim et al., 2019). However, the proportional abundance of Proteobacteria in this study (58.63%) is relatively higher than that in some other studies (generally below 50%) (He et al., 2017; Yu et al., 2018). The higher abundance of Proteobacteria is in agreement with their dominant positions in co-occurrence relationships of microbiota in this study. Proteobacteria is always the dominant bacterial taxon in seawater, and its further increase may preempt the space of other bacteria and reduce microbiota diversification.
The seasonal and monthly variation of the bacterial community was more pronounced than inter-annual variation, mainly due to the intra-annual environmental changes. This followed common sense and was in agreement with other reports. Kim et al. (2019) found that bacterial abundance closely fitted a chronological seasonal pattern in the bays of the Yellow Sea, and temporal patterns of microbial communities were also observed in the New Jersey coast and the Pearl River Estuary area (Nelson et al., 2008; Xie et al., 2018). The monthly progressive changes of bacterial community patterns were probably caused by one factor that was in continuously unidirectional changes, which was most likely the water temperature. Water temperature was always considered as the most important environmental factors that drove the bacterial community (Kim et al., 2019). We can also have this inference according to the results of RDA analysis. The relative high T and low DO in summer may play important roles in constraining and shaping the bacterial community. Except for T and DO, in the present study, Chl-a was another important environmental factor that was associated with bacterial community variation. Previous studies also reported that the Chl-a was the key predictor of microbiota, and some bacterial taxa were clearly correlated with Chl-a (Kim et al., 2019). It should be noted that shellfish aquaculture always has a great influence on the phytoplankton abundance and community, as phytoplankton is the main food source of shellfish. Thus, the bacterial community in the offshore shellfish farming waters would have specific structure patterns, resulted from the phytoplankton variation.
In the present study, Desulfobacterales acted as the keystone species taxon in co-occurrence relationships of microbiota and may function in nitrogen metabolism with further effect on other bacterial communities through nitrogen cycling. Desulfobacterales plays important roles in nitrogen cycling and removal, and could contribute about 12% of the genes in nitrogen pathways (Nie et al., 2021). Recently, dominant sulfate reducing microorganisms (SRM) within Desulfobacterales were also reported in the sediments of coastal oyster aquaculture ecosystems (Mara et al., 2021). The proportional abundance of Actinobacteriota (1.3%) in this study was obviously lower than that in other studies, in which it was reported up to 5%-20% (He et al., 2017; Yu et al., 2018). The abundance of Actinobacteriota has been proved to be strongly correlated with nitrogen cycling, and it can thrive under oligotrophic conditions and be suppressed with high organic matter and inorganic nutrient availability (Kulaš et al., 2021; Wang et al., 2021). The impact of shellfish farming on nitrogen cycling has been investigated in previous reports by analyses of water/sediment quality parameters (Erler et al., 2017; Jiang et al., 2020; Pan et al., 2021). The understanding of the bacterial community characteristics involved in nitrogen cycling in shellfish farming water is very limited. In order to further explore the details of nitrogen-transforming function of bacterial community, the commonly reported nitrogen-transforming bacteria (Kuypers et al., 2018) were investigated in our study. The presence of Marinobacter and Synechococcus was remarkably prominent in all 13 genera with nitrogen-transforming function. Marinobacter and Synechococcus were mainly responsible for the process and remove of organic nitrogen and NH4+ in seawater. The bacterial community characteristics involved in nitrogen cycling were rarely reported in the Yellow Sea (Bai et al., 2012; Yu et al., 2018; Jing et al., 2019; Yang et al., 2022). Together, the above results indicated that the bacterial community in the shellfish aquaculture waters in this study was characterized by the enhancing ability of nitrogen removal.
The particularities of bacterial community in the waters with SCPs
The bacterial community between the two locations had less variation compared to the seasonal variation. Greater temporal variation and less spatial variation of the bacterial community were also found in the Yellow Sea area and some aquaculture sea areas (He et al., 2017; Kim et al., 2019). Spatial differentiation may superficially mask the environmental effects only when the physicochemical factors are closely related to the spatial conditions. Otherwise, persistent environmental heterogeneity would cover the geographic difference and shape the microbial diversity directly at intermediate spatial scales (Crossland et al., 2005; Wang et al., 2015).
SCP is an important geographic feature of the Yellow Sea and some other seas. Its formation results from the upwelling, which carries the cold deep water up to the sea surface. The more water exchange in vertical direction may change the water environment and bring more nutrients and attachments from the bottom water layer to surface water layer (Lü et al., 2010; Huang et al., 2018; Lin et al., 2019). In our study, the bacterial community variation between the two locations had more significant differences in summer than that in spring. It was suggested that different geographic conditions may lead to the variation of the bacterial community, mainly through the environmental factor variation. Environmental factors always play key roles in the spatial variation of the bacterial community. For instance, the different concentration levels of Chl-a were reported to be in charge of the bacterial community diversity between two stations of the Yellow Sea (Kim et al., 2019).
Interestingly, consistency was found in two points between the spatial analysis (location X vs location Z) and the seasonal analysis (spring vs summer). First, the top two differently predicted pathways of microbial function in both spatial analysis and seasonal analysis were same, and they were xenobiotics biodegradation and metabolism and metabolism of cofactors and vitamins, which may indicate the occurrence of environmental stresses and resultant activation of stress responses (Huo et al., 2019; Ye et al., 2021). Second, 11 of the 12 common genera of pathogenic bacteria (except for Pseudomonas) had the same dynamic patterns in the spatial and seasonal analyses, in other words, if the abundance of one pathogenic bacterial genus was higher (or lower) in the waters with SCP than that in ambient waters, it was most likely that the abundance of the pathogenic bacterial genus was also higher (or lower) in spring than that in summer. Temperature was the predominant environmental factor that drove the seasonal dynamic of bacterial community variation between spring and summer. Temperature was also the most critical feature that distinguished the waters with SCP from ambient waters. As there are highly similar dynamic patterns of bacterial community variation between the seasonal and spatial analyses, it is reasonable to speculate that temperature is the predominant environmental factor to drive the variation of bacterial community function and pathogenic bacteria patterns in the offshore shellfish farming waters with SCPs.
Vibrio abundance variation and the predictive model construction
The Vibrio abundance generally increased with the elevated water temperature and showed clear seasonal patterns, consistent with some other reports (Hsieh et al., 2008; Vezzulli et al., 2009; Oberbeckmann et al., 2012; Froelich et al., 2015). The enrichment of the pathways related to infectious disease in summer suggested that the risk from pathogenic bacteria increased in summer and need more attention. Shellfish aquaculture was found to play important roles in shaping Vibrio characteristics (Joye and Anderson, 2008; Feinman et al., 2018), probably through disturbing the structure of phytoplankton and zooplankton, both of which served as determinants of Vibrio presence and abundance (Johnson, 2015). There were many reports about the Vibrio variation in water environment, in which the key environmental factors that affect Vibrio community were identified and the variation tendency of Vibrio was speculated based on environmental factors (Hsieh et al., 2008; Oberbeckmann et al., 2012; Johnson, 2015). In the present study, except for T, SiO4-Si was also found to be significantly associated with the Vibrio abundance. We speculate that: On one hand, SiO4-Si exists in the sea-water column as a sol state, which could provide attachment for the growth of Vibrio. On the other hand, phytoplankton and Chl-a are key drivers of the bacterial community. SiO4-Si is an important component of some phytoplankton taxa such as diatom (DeLuca et al., 2020) and could thus influence the Vibrio abundance. However, as shown in Figure 6, the Vibrio abundance in the same month of the different years differed apparently based on the three-year data, suggesting that it is infeasible to estimate the Vibrio abundance only according to environmental factors or historical values of Vibrio abundance.
In recent years, some studies of model establishment and Vibrio prediction have been reported. Hidemasa Izumiya et al. conducted a multi-coastal study to examine relationships between environmental factors and Vibrio and build a linear regression model for Vibrio prediction (Izumiya et al., 2017). Brett Froelich et al. conducted a five-parameter mechanistic model to predict Vibrio abundance in the river based on environmental processes (Froelich et al., 2013). According to the previous reports, the construction of Vibrio predictive model is better to be improved in two points. First, environmental factors were always included in the predictive model construction as the only type of input variables in most of the previous studies. Aside from the environmental factors, Vibrio are also likely to respond to, or be associated with, many other aquatic bacterial species (Johnson, 2015) in some ways, such as quorum sensing. The interactions between Vibrio and other bacterial communities should also be included in the studies of Vibrio forecasting. Second, among the environmental factors, one factor usually has weak or strong dependence on others. The exclusive effect of one environmental factor on Vibrio abundance is hard to assess. The relationship between Vibrio and input variables of the predictive model is not a direct correlation but rather the relation to which many variables contribute. Multiple regression analysis is not good at the modelling with too many input variables in the construction of Vibrio predictive model. Therefore, an integrated model that has environmental factors and bacterial species as input variables should be established to better understand the variation of Vibrio abundance.
Our study improved the Vibrio predictive modelling by (1) including the environmental factors as well as bacterial species as input variables for more accurate prediction of Vibrio abundance; (2) using ANN to solve the complex mapping relationships between dozens of input variables and Vibrio abundance and to construct the predictive model. The accuracy was acceptable, with 95.8% of accuracy for training samples and 96.4% for testing samples. The spatial scope of model application and the accuracy will be further improved in our future studies through the inclusion of more data for model training, as the Vibrio prediction is better to be processed according to regional differences, rather than using a “one-size-fits-all” approach. The results of this study will contribute to the monitoring and modelling efforts of Vibrio abundance in the NYS for area-specific Vibrio forecasting and public health risk prediction.
Conclusions
The main conclusions drawn from this study are as following (Figure S2):
● The bacterial community in the shellfish aquaculture waters in this study was characterized by the enhancing ability of nitrogen removal.
● Temperature was the predominant environmental factor to drive the variation of bacterial community function and pathogenic bacteria patterns in the offshore shellfish farming waters with SCPs.
● An ANN predictive model for Vibrio abundance was constructed in this study for Vibrio forecasting, with acceptable predictive accuracy.
Data availability statement
The data presented in the study are deposited in the GenBank repository, accession number PRJNA861897.
Author contributions
LG: Methodology, Formal analysis, Writing original draft; ZY: Investigation, Sample collection; CL and NK: Sample collection; LW and LS: Writing Review & Editing, Supervision, Funding acquisition. All authors contributed to the article and approved the submitted version.
Funding
This research was supported by a grant (41961124009) from National Science Foundation of China, National key R&D Program of China (2018YFD0900501), China Agriculture Research System of MOF and MARA, the Fund for Outstanding Talents and Innovative Team of Agricultural Scientific Research in MARA, the Research Foundation for Climbing Scholar in Liaoning, and Distinguished Professor in Liaoning (XLYC1902012), Key R&D Program of Liaoning Province (2017203001, 2017203004), the Scientific Research Project of Liaoning Province Department of Education (No. QL201902), the Research Foundation for Talented Scholars in Dalian Ocean University (to LW), and the Science and Technology Innovation Fund of Dalian (2021JJ13SN73).
Conflict of interest
The authors declare that the research was conducted in the absence of any commercial or financial relationships that could be construed as a potential conflict of interest.
Publisher’s note
All claims expressed in this article are solely those of the authors and do not necessarily represent those of their affiliated organizations, or those of the publisher, the editors and the reviewers. Any product that may be evaluated in this article, or claim that may be made by its manufacturer, is not guaranteed or endorsed by the publisher.
Supplementary material
The Supplementary Material for this article can be found online at: https://www.frontiersin.org/articles/10.3389/fmars.2022.997817/full#supplementary-material
Supplementary Figure 1 | Location X and location Z were set as the two sampling regions for the offshore Yesso scallop farming waters in the NYS. Horizontal distribution of mean sea surface temperature in August of 2004–2008 was showed. This figure is cited from (Lü et al., 2010).
Supplementary Figure 2 | Graphical abstract of this study.
Supplementary Table 1 | The prediction accuracy of ANN model for Vibrio abundance for both training samples and testing samples.
References
Azandégbé A., Poly F., Andrieux Loyer F., Kérouel R., Philippon X., Nicolas J. L. (2012). Influence of oyster culture on biogeochemistry and bacterial community structure at the sediment–water interface. FEMS Microbiol. Ecol. 82 (1), 102–117. doi: 10.1111/j.1574-6941.2012.01410.x
Bai X., Wang M., Liang Y., Zhang Z., Wang F., Jiang X. (2012). Distribution of microbial populations and their relationship with environmental variables in the North Yellow Sea, china. J. Ocean U. China 11 (1), 75–85. doi: 10.1007/s11802-012-1799-8
Bolyen E., Rideout J. R., Dillon M. R., Bokulich N. A., Abnet C. C., Al Ghalith G. A., et al. (2019). Reproducible, interactive, scalable and extensible microbiome data science using QIIME 2. Nat. Biotechnol. 37 (8), 852–857. doi: 10.1038/s41587-019-0209-9
Chong J., Liu P., Zhou G., Xia J. (2020). Using MicrobiomeAnalyst for comprehensive statistical, functional, and meta-analysis of microbiome data. Nat. Protoc. 15 (3), 799–821. doi: 10.1038/s41596-019-0264-1
Crossland C. J., Kremer H. H., Lindeboom H., Crossland J. I. M., Le Tissier M. D. (2005). Coastal fluxes in the anthropocene: the land-ocean interactions in the coastal zone project of the international geosphere-biosphere programme (Berlin: Springer Science & Business Media).
Dash H. R., Mangwani N., Chakraborty J., Kumari S., Das S. (2013). Marine bacteria: potential candidates for enhanced bioremediation. Appl. Microbiol. Biot. 97 (2), 561–571. doi: 10.1007/s00253-012-4584-0
DeLuca N. M., Zaitchik B. F., Guikema S. D., Jacobs J. M., Davis B. J., Curriero F. C. (2020). Evaluation of remotely sensed prediction and forecast models for vibrio parahaemolyticus in the Chesapeake bay. Remote Sens. Environ. 250, 112016. doi: 10.1016/j.rse.2020.112016
Deng Y., Jiang Y. H., Yang Y., He Z., Luo F., Zhou J. (2012). Molecular ecological network analyses. BMC Bioinform. 13 (1), 1–20. doi: 10.1186/1471-2105-13-113
Douglas G. M., Maffei V. J., Zaneveld J. R., Yurgel S. N., Brown J. R., Taylor C. M., et al. (2020). PICRUSt2 for prediction of metagenome functions. Nat. Biotechnol. 38 (6), 685–688. doi: 10.1038/s41587-020-0548-6
Dumbauld B. R., Ruesink J. L., Rumrill S. S. (2009). The ecological role of bivalve shellfish aquaculture in the estuarine environment: A review with application to oyster and clam culture in West Coast (USA) estuaries. Aquaculture 290 (3-4), 196–223. doi: 10.1016/j.aquaculture.2009.02.033
Erler D. V., Welsh D. T., Bennet W. W., Meziane T., Hubas C., Nizzoli D., et al. (2017). The impact of suspended oyster farming on nitrogen cycling and nitrous oxide production in a sub-tropical Australian estuary. Estuar. Coast. Shelf Sci. 192, 117–127. doi: 10.1016/j.ecss.2017.05.007
Falkowski P. G., Fenchel T., Delong E. F. (2008). The microbial engines that drive earth’s biogeochemical cycles. Science 320 (5879), 1034–1039. doi: 10.1126/science.1153213
FAO (2020). The state of world fisheries and aquaculture 2020, sustainability in action (Rome: Food and Agriculture Organization of the United Nations).
Feinman S. G., Farah Y. R., Bauer J. M., Bowen J. L. (2018). The influence of oyster farming on sediment bacterial communities. Estuaries Coast. 41 (3), 800–814. doi: 10.1007/s12237-017-0301-7
Froelich B., Ayrapetyan M., Fowler P., Oliver J., Noble R. (2015). Development of a matrix tool for the prediction of vibrio species in oysters harvested from North Carolina. Appl. Environ. Microb. 81 (3), 1111–1119. doi: 10.1128/AEM.03206-14
Froelich B., Bowen J., Gonzalez R., Snedeker A., Noble R. (2013). Mechanistic and statistical models of total vibrio abundance in the neuse river estuary. Water Res. 47 (15), 5783–5793. doi: 10.1016/j.watres.2013.06.050
Gutiérrez J. L., Jones C. G., Strayer D. L., Iribarne O. O. (2003). Mollusks as ecosystem engineers: The role of shell production in aquatic habitats. Oikos 101 (1), 79–90. doi: 10.1034/j.1600-0706.2003.12322.x
Han Q., Wang Y., Zhang Y., Keesing J., Liu D. (2013). Effects of intensive scallop mariculture on macrobenthic assemblages in Sishili Bay, the Northern Yellow Sea of China. Hydrobiologia 718 (1), 1–15. doi: 10.1007/s10750-013-1590-x
Hartwick M. A., Urquhart E. A., Whistler C. A., Cooper V. S., Naumova E. N., Jones S. H. (2019). Forecasting seasonal vibrio parahaemolyticus concentrations in New England shellfish. Int. J. Environ. Res. Public Health 16 (22), 4341. doi: 10.3390/ijerph16224341
He Y., Sen B., Shang J., He Y., Xie N., Zhang Y., et al. (2017). Seasonal influence of scallop culture on nutrient flux, bacterial pathogens and bacterioplankton diversity across estuaries off the bohai Sea coast of Northern China. Mar. pollut. Bull. 124 (1), 411–420. doi: 10.1016/j.marpolbul.2017.07.062
Hsieh J. L., Fries J. S., Noble R. T. (2008). Dynamics and predictive modelling of vibrio spp. in the neuse river estuary, north Carolina, USA. Environ. Microbiol. 10 (1), 57–64. doi: 10.1111/j.1462-2920.2007.01429.x
Huang M., Liang X. S., Wu H., Wang Y. (2018). Different generating mechanisms for the summer surface cold patches in the Yellow Sea. Atmos. Ocean 56 (4), 199–211. doi: 10.1080/07055900.2017.1371580
Huo D., Sun L., Zhang L., Ru X., Liu S., Yang H. (2019). Metabolome responses of the sea cucumber apostichopus japonicus to multiple environmental stresses: Heat and hypoxia. Mar. Pollut. Bull. 138, 407–420. doi: 10.1016/j.marpolbul.2018.11.063
Hur H., Jacobs G., Teague W. (1999). Monthly variations of water masses in the Yellow and East China seas, November 6, 1998. J. Oceanogr. 55 (2), 171–184. doi: 10.1023/A:1007885828278
Izumiya H., Furukawa M., Ogata K., Isobe J., Watanabe S., Sasaki M., et al. (2017). A double-quadratic model for predicting vibrio species in water environments of Japan. Arch. Microbiol. 199 (9), 1293–1302. doi: 10.1007/s00203-017-1402-1
Jiang H., Lan W., Li T., Xu Z., Liu W., Pan K. (2020). Isotopic composition reveals the impact of oyster aquaculture on pelagic nitrogen cycling in a subtropical estuary. Water Res. 187, 116431. doi: 10.1016/j.watres.2020.116431
Jing X., Gou H., Gong Y., Ji Y., Su X., Zhang J., et al. (2019). Seasonal dynamics of the coastal bacterioplankton at intensive fish-farming areas of the yellow Sea, China revealed by high-throughput sequencing. Mar. Pollut. Bull. 139, 366–375. doi: 10.1016/j.marpolbul.2018.12.052
Johnson C. N. (2015). Influence of environmental factors on vibrio spp. in coastal ecosystems. Microbiol. Spectr. 3 (3), VE–0008-2014. doi: 10.1128/microbiolspec.VE-0008-2014
Joye S. B., Anderson I. C. (2008). Nitrogen in the marine environment (Burlington, MA: Academic Press).
Kim J. G., Gwak J. H., Jung M. Y., An S. U., Hyun J. H., Kang S., et al. (2019). Distinct temporal dynamics of planktonic archaeal and bacterial assemblages in the bays of the yellow Sea. PloS One 14 (8), e0221408. doi: 10.1371/journal.pone.0221408
Kulaš A., Marković T., Žutinić P., Kajan K., Karlović I., Orlić S., et al. (2021). Succession of microbial community in a small water body within the alluvial aquifer of a large river. Water 13 (2), 115. doi: 10.3390/w13020115
Kuypers M. M., Marchant H. K., Kartal B. (2018). The microbial nitrogen-cycling network. Nat. Rev. Microbiol. 16 (5), 263–276. doi: 10.1038/nrmicro.2018.9
Lin L., Liu D., Luo C., Xie L. (2019). Double fronts in the yellow Sea in summertime identified using sea surface temperature data of multi-scale ultra-high resolution analysis. Cont. Shelf Res. 175, 76–86. doi: 10.1016/j.csr.2019.02.004
Lin C., Ning X., Su J., Lin Y., Xu B. (2005). Environmental changes and the responses of the ecosystems of the Yellow Sea during 1976–2000. J. Mar. Syst. 55 (3-4), 223–234. doi: 10.1016/j.jmarsys.2004.08.001
Lorenzen C. J. (1967). Determination of chlorophyll and pheo-pigments: spectrophotometric equations 1. Limnol. Oceanogr. 12 (2), 343–346. doi: 10.4319/lo.1967.12.2.0343
Lü X., Qiao F., Xia C., Wang G., Yuan Y. (2010). Upwelling and surface cold patches in the Yellow Sea in summer: Effects of tidal mixing on the vertical circulation. Cont. Shelf Res. 30 (6), 620–632. doi: 10.1016/j.csr.2009.09.002
Mara P., Edgcomb V. P., Sehein T. R., Beaudoin D., Martinsen C., Lovely C., et al. (2021). Comparison of oyster aquaculture methods and their potential to enhance microbial nitrogen removal from coastal ecosystems. Front. Mar. Sci. 8, 174. doi: 10.3389/fmars.2021.633314
Nelson J. D., Boehme S. E., Reimers C. E., Sherrell R. M., Kerkhof L. J. (2008). Temporal patterns of microbial community structure in the mid-Atlantic bight. FEMS Microbiol. Ecol. 65 (3), 484–493. doi: 10.1111/j.1574-6941.2008.00553.x
Newell R. I. (2004). Ecosystem influences of natural and cultivated populations of suspension-feeding bivalve molluscs: A review. J. Shellfish Res. 23 (1), 51–62.
Nie S., Zhang Z., Mo S., Li J., He S., Kashif M., et al. (2021). Desulfobacterales stimulates nitrate reduction in the mangrove ecosystem of a subtropical gulf. Sci. Total Environ. 769, 144562. doi: 10.1016/j.scitotenv.2020.144562
Oberbeckmann S., Fuchs B. M., Meiners M., Wichels A., Wiltshire K. H., Gerdts G. (2012). Seasonal dynamics and modeling of a vibrio community in coastal waters of the north Sea. Microb. Ecol. 63 (3), 543–551. doi: 10.1007/s00248-011-9990-9
Pan K., Lan W., Li T., Hong M., Peng X., Xu Z., et al. (2021). Control of phytoplankton by oysters and the consequent impact on nitrogen cycling in a subtropical bay. Sci. Total Environ. 796, 149007. doi: 10.1016/j.scitotenv.2021.149007
Parks D. H., Tyson G. W., Hugenholtz P., Beiko R. G. (2014). STAMP: statistical analysis of taxonomic and functional profiles. Bioinformatics 30 (21), 3123–3124. doi: 10.1093/bioinformatics/btu494
Shannon P., Markiel A., Ozier O., Baliga N. S., Wang J. T., Ramage D., et al. (2003). Cytoscape: A software environment for integrated models of biomolecular interaction networks. Genome Res. 13 (11), 2498–2504. doi: 10.1101/gr.1239303
Šmilauer P., Lepš J. (2014). Multivariate analysis of ecological data using CANOCO 5 (Cambridge: Cambridge University Press).
SOA of China (2007). Specification for marine monitoring (China: Standards Press of China, Beijing, China).
Tang Q. (2009). Changing states of the yellow Sea Large marine ecosystem: anthropogenic forcing and climate impacts. sustaining the world’s large marine ecosystems (Amsterdam: Elsevier Science Press).
Vezzulli L., Pezzati E., Moreno M., Fabiano M., Pane L., Pruzzo C., et al. (2009). Benthic ecology of vibrio spp. and pathogenic vibrio species in a coastal Mediterranean environment (La spezia gulf, Italy). Microb. Ecol. 58 (4), 808–818. doi: 10.1007/s00248-009-9542-8
Vitousek P. M., Mooney H. A., Lubchenco J., Melillo J. M. (1997). Human domination of earth’s ecosystems. Science 277 (5325), 494–499. doi: 10.1126/science.277.5325.494
Wang S., Li X., Zhang M., Jiang H., Wang R., Qian Y., et al. (2021). Ammonia stress disrupts intestinal microbial community and amino acid metabolism of juvenile yellow catfish (Pelteobagrus fulvidraco). Ecotox. Environ. Safe 227, 112932. doi: 10.1016/j.ecoenv.2021.112932
Wang K., Ye X., Chen H., Zhao Q., Hu C., He J., et al. (2015). Bacterial biogeography in the coastal waters of northern zhejiang, East China Sea is highly controlled by spatially structured environmental gradients. Environ. Microbiol. 17 (10), 3898–3913. doi: 10.1111/1462-2920.12884
Xia Z., Guo B. (1983). The cold water and upwelling in the tip areas of Shandong peninsula and liaodong peninsula. J. Oceanography Huanghai Bohai Seas 1 (1), 13–19.
Xie W., Luo H., Murugapiran S. K., Dodsworth J. A., Chen S., Sun Y., et al. (2018). Localized high abundance of marine group II archaea in the subtropical pearl river estuary: Implications for their niche adaptation. Environ. Microbiol. 20 (2), 734–754. doi: 10.1111/1462-2920.14004
Yang B., Gao X., Zhao J., Xie L., Liu Y., Lv X., et al. (2022). The impacts of intensive scallop farming on dissolved organic matter in the coastal waters adjacent to the yangma island, Northern Yellow Sea. Sci. Total Environ. 807, 150989. doi: 10.1016/j.scitotenv.2021.150989
Ye G., Zhang X., Yan C., Lin Y., Huang Q. (2021). Polystyrene microplastics induce microbial dysbiosis and dysfunction in surrounding seawater. Environ. Int. 156, 106724. doi: 10.1016/j.envint.2021.106724
Yuan X., Zhang M., Liang Y., Liu D., Guan D. (2010). Self-pollutant loading from a suspension aquaculture system of Japanese scallop (Patinopecten yessoensis) in the changhai sea area, Northern Yellow Sea of China. Aquaculture 304 (1-4), 79–87. doi: 10.1016/j.aquaculture.2010.03.026
Yu Z., Liu C., Fu Q., Lu G., Han S., Wang L., et al. (2019a). The differences of bacterial communities in the tissues between healthy and diseased yesso scallop (Patinopecten yessoensis). AMB Express 9 (1), 1–13. doi: 10.1186/s13568-019-0870-x
Yu Z., Liu C., Wang F., Xue Z., Zhang A., Lu G., et al. (2019b). Diversity and annual variation of phytoplankton community in yesso scallop (Patinopecten yessoensis) farming waters of Northern Yellow Sea of China. Aquaculture 511, 734266. doi: 10.1016/j.aquaculture.2019.734266
Yu S., Pang Y., Wang Y., Li J., Qin S. (2018). Distribution of bacterial communities along the spatial and environmental gradients from bohai Sea to Northern Yellow Sea. PeerJ 6, e4272. doi: 10.7717/peerj.4272
Keywords: shellfish aquaculture, bacterial community, Vibrio, surface cold patches, predictive model
Citation: Gao L, Yu Z, Liu C, Kong N, Wang L and Song L (2022) Characteristics and particularities of bacterial community variation in the offshore shellfish farming waters of the North Yellow Sea. Front. Mar. Sci. 9:997817. doi: 10.3389/fmars.2022.997817
Received: 19 July 2022; Accepted: 03 August 2022;
Published: 24 August 2022.
Edited by:
Ce Shi, Ningbo University, ChinaReviewed by:
Meng Li, Department of Environmental Biotechnology, (HZ), GermanyWansheng Jiang, Jishou University, China
Donghong Niu, Shanghai Ocean University, China
Copyright © 2022 Gao, Yu, Liu, Kong, Wang and Song. This is an open-access article distributed under the terms of the Creative Commons Attribution License (CC BY). The use, distribution or reproduction in other forums is permitted, provided the original author(s) and the copyright owner(s) are credited and that the original publication in this journal is cited, in accordance with accepted academic practice. No use, distribution or reproduction is permitted which does not comply with these terms.
*Correspondence: Lingling Wang, d2FuZ2xpbmdsaW5nQGRsb3UuZWR1LmNu; Linsheng Song, bHNoc29uZ0BkbG91LmVkdS5jbg==