- 1Department of Ocean and Earth Sciences, Old Dominion University, Norfolk, VA, United States
- 2Bermuda Institute of Ocean Sciences, St. George's, Bermuda
Optical surveys of aquatic particles and their particle size spectra have become important tools in studies of light propagation in water, classification of water masses, and the dynamics of trophic interactions affecting particle aggregation and flux. Here, we demonstrate that typical settings used in image analysis vastly underestimate particle numbers due to the particle – gel continuum. Applying a wide range of threshold values to change the sensitivity of our detection system, we show that macrogels cannot be separated from more dense particles, and that a true particle number per volume cannot be ascertained; only relative numbers in relation to a defined threshold value can be reported. A quandary thus presents itself between choosing a detection threshold low enough to accurately record orders of magnitude more particles on one hand or selecting a higher threshold to yield better image quality of plankton on the other. By observing the dynamics of coagulation and dissolution steps unique to cation-bridged gels abundant in aquatic systems, we find naturally occurring gels, and microscopic particles attached to them, to cause the ill-defined particle numbers. In contrast, the slopes in particle number spectra remained largely unaffected by varying sensitivity settings of the image analysis. The inclusion of fainter particles that are not typically captured by imaging systems provides a window into the true microscale spatial heterogeneity at scales relevant to small plankton organisms and processes that are dependent on particle density such as surface-associated chemical reactions as well as particle coagulation and aggregation dynamics.
Introduction
Optical particle characterizations through imaging systems have become indispensable for aquatic research (Jackson et al., 1997; Sosik and Olson, 2007; Stemmann et al., 2008; Guidi et al., 2009; Iversen et al., 2010; Picheral et al., 2010; Forest et al., 2012; Boss et al., 2015; Omand et al., 2015; Lombard et al., 2019; Hatton et al., 2021; Picheral et al., 2022). However, what defines a particle in aquatic systems is not as straightforward as it seems. A definition can be borrowed from the classification of colloids (Gustafsson and Gschwend, 1997), which is that a particle is physically and chemically different from the outside, or in other words that there exists an interface. In contrast to a colloid, however, a particle does not have an upper size limit (Hatton et al., 2021).
Differences in physico-chemical characteristics between particles and the surrounding medium aid in their optical detection through light absorbance and scatter (Jonasz and Fournier, 2007). As a consequence, image analysis algorithms that convert image information to particle information are as important as the opto-mechanical configurations to separate what is considered a particle from the non-particle background (Giering et al., 2020). This requires the important decision about what constitutes a particle in terms of optical characteristics, yet thus far, no absolute cut-off or threshold values have been reported in the literature for any of the existing imaging systems. Threshold levels of camera - image analysis pairings are typically adjusted subjectively, often by relying on suitable visualizations of plankton organisms which lie at the far end of the range of signal intensity (Samson et al., 2001; Davis et al., 2005; Sosik and Olson, 2007; Cowen and Guigand, 2008; Picheral et al., 2010; Ohman et al., 2019; Gillard et al., 2022). By training cameras on optically dense particles alone, however, information on the much more numerous fainter particles is lost.
In this study, we used an optical arrangement that is sufficiently sensitive to capture a portion of the aquatic gels in situ and explored their abundance relative to the denser particle pool. While we demonstrate this problem in one optical configuration, considerations of particle definition apply universally to all optical and non-optical systems.
Materials and methods
In-situ imaging
The basic configuration of our system is the same as in previous shadowgraph cameras (Arnold and Nuttall-Smith, 1974; Cowen and Guigand, 2008; Ohman et al., 2019), except for the direct inline configuration without mirrors and smaller spatial scales of our system (image field: 15.36 mm x 11.52 mm, 1280 x 960 pixels, image volume: 5.3 ml). The light source was a red LED (625 nm, Cree XLamp) collimated by a 150 mm plano-convex lens. The light then passes in sequence, through a 25.4 mm sapphire window, 30 mm of seawater, and another 25.4 mm thick sapphire window, a 100 mm plano-convex lens, before being collected by a 1/3” monochrome CMOS chip with a global shutter (Imaging Source, LLC) and equipped with a 25 mm board camera lens (f/2.5, V-4325, Marshall Electronics). In this telecentric setup, blur at the far edges of the image path is symmetric, and the center of mass is retained, so that the edge of the particle is rendered relatively accurately, even if it is slightly out of focus (Watanabe and Nayar, 1997; Lange, 2022). Images were recorded by a mini-PC on a 1 TB micro-SD card. For the conductivity, temperature and depth (CTD) rosette casts in the Sargasso Sea, the optical setup and the electronics were enclosed in a stainless-steel housing rated to 6000 m. For the shallow deployments in the Gulf of Trieste, optics and electronics were enclosed in a lighter polyvinyl chloride (PVC) housing and still equipped with 25.4 mm sapphire windows to retain the same optical configuration as the deep-sea version. The lower practical particle size cut-off in this analysis was 43 μm, which is equivalent to approximately 4 pixels linear dimension.
For images from the Sargasso Sea, the camera was mounted on the lower ring of the CTD rosette deployed during the Oceanic Flux program (Conte et al., 2001). Images (n = 45,512) of the surface layer (0-100 m) were taken at 1 second intervals during 11 casts (both down- and upcasts) from April 14 to April 21 2021, at 63.0 - 64.3 N Latitude, and 31.0 - 32.5 W Longitude. Images in the Gulf of Trieste (n = 2,125; 45° 31.56’ N latitude, 13° 35.41 E Longitude) were recorded by a SCUBA diver on July 18, 2021, below the first thermocline at depths between 4 and 7 m for 36 minutes with a frame rate of 1 image per second. A stage micrometer (1 mm total, 0.01 mm increments) and stepped neutral density filters on a microscope slide (11 discrete density steps from OD = 0.04 to 1.0, design wavelengths 400 to 700 nm, Edmund Optics) were recorded in pure water for calibration.
Laboratory experiments
To test whether the shadowgraph imaging system is sensitive to a portion of aquatic gels, we designed an experiment that provides gels in their purest form in ultrapure water. This avoided any contamination by solids or coprecipitates that could have influenced the results. Alginates are representative precursors of transparent exopolymer particles (TEP) (Meng and Liu, 2016), and as they contain sugar acids (e.g., uronic acids) to which Alcian blue attaches, they are used as analytical standards in the definition of environmentally-occurring TEP (Alldredge et al., 1993; Passow, 2002). TEP are an important subset of aquatic gels that span a broad size spectrum from micro- to macrogels (Verdugo et al., 2004). The laboratory experiments with gel particles were recorded with the same optical configuration as the in-situ images (30 mm optical path) except for the use of a small (40 mL) glass tank. A magnetic stirrer kept solutions well mixed, and decreased the time of schlieren effects due to variable physical densities (Davidhazy, 2006). Images were recorded in 15 minute sequences each of ultrapure water (18.2 MΩ.cm resistivity), replaced with a sodium alginate solution in ultrapure water (0.2 g L-1), the same solution with the addition of 0.4 ml of 0.1 M CaCl2 solution (Meng and Liu, 2016), and the same solution followed by the addition of 0.4 ml of 0.5 mM Ethylenediaminetetraacetic acid (EDTA). Experiments were conducted at 25° C. Stage micrometer and neutral density filters were recorded as above. During each sequence, a 5 mL subsample was taken for the staining of transparent exopolymer particles (Alldredge et al., 1993). They were filtered onto 0.4 μm pore-size polycarbonate filters (Isopore, Millipore Sigma), stained with Alcian blue, rinsed with ultrapure water, mounted on Cyto-clear slides (Logan et al., 1994), and observed under a compound microscope with brightfield illumination (Alldredge et al., 1993).
Image processing
Raw images were corrected for unevenness in illumination using the flat field method (Wilkinson, 1994). In the laboratory, blank images taken with ultrapure water were subtracted from the experimental images. For analysis of in situ images, and to account for any changes in the overall light field, or changes in the performance of the LED or the camera chip, image pairs of consecutive images were subtracted from each other (Bochdansky et al., 2013). Particles are thus determined by difference, removing any impurities on lens or optical port surfaces, and as such represent conservative estimates of particle numbers. The volume of each image pair used in the analysis is therefore 10.6 mL (2 x 5.3 mL). Grayscale images were then binarized using global thresholds (5 to 70). Particles were detected by Canny edge detection (Ohman et al., 2019; Giering et al., 2020), and analyzed for size and other characteristics using the Matlab Imaging Toolbox. Particle number spectra were calculated using logarithmic bin sizes (Jackson et al., 1997; Ghasemi et al., 2018). Particle size is calculated as the equivalent spherical diameter of a sphere of the same area as the shadowgram (in pixels) of the original particle (Bochdansky et al., 2017).
For linear regression analysis of spectral slopes against threshold levels, the residuals failed the normality assumption of parametric tests according to the Kolmogorov-Smirnov test statistic. P-values were thus calculated using randomization tests based on 10,000 randomized data pairs (Manly, 2007).
Results
Particle numbers vary over orders of magnitude while spectral slopes change little
We analyzed the shadow images of particles in surface waters of two very different oceanic environments: the Sargasso Sea (0 – 100 m depth) and the Gulf of Trieste (Northern Adriatic Sea, 4 – 7 meter depth). The Sargasso Sea is an oligotrophic open ocean gyre system, while the Gulf of Trieste is a eutrophic coastal environment with high abundances algal aggregates and discarded appendicularian houses that form the initial support matrices of marine snow. In both environments, images were analyzed using a wide variety of threshold levels. The resulting number spectra show the typical decrease of numbers with increasing particle size (Figure 1). However, particle numbers also greatly changed with the applied threshold levels in both environments (Figures 1A–D). The relative change in particle numbers with applied lower threshold levels was greater in the Sargasso Sea (Figure 1A) than in the Gulf of Trieste (Figure 1C) indicating that proportionately more faint particles were detected in the Sargasso Sea.
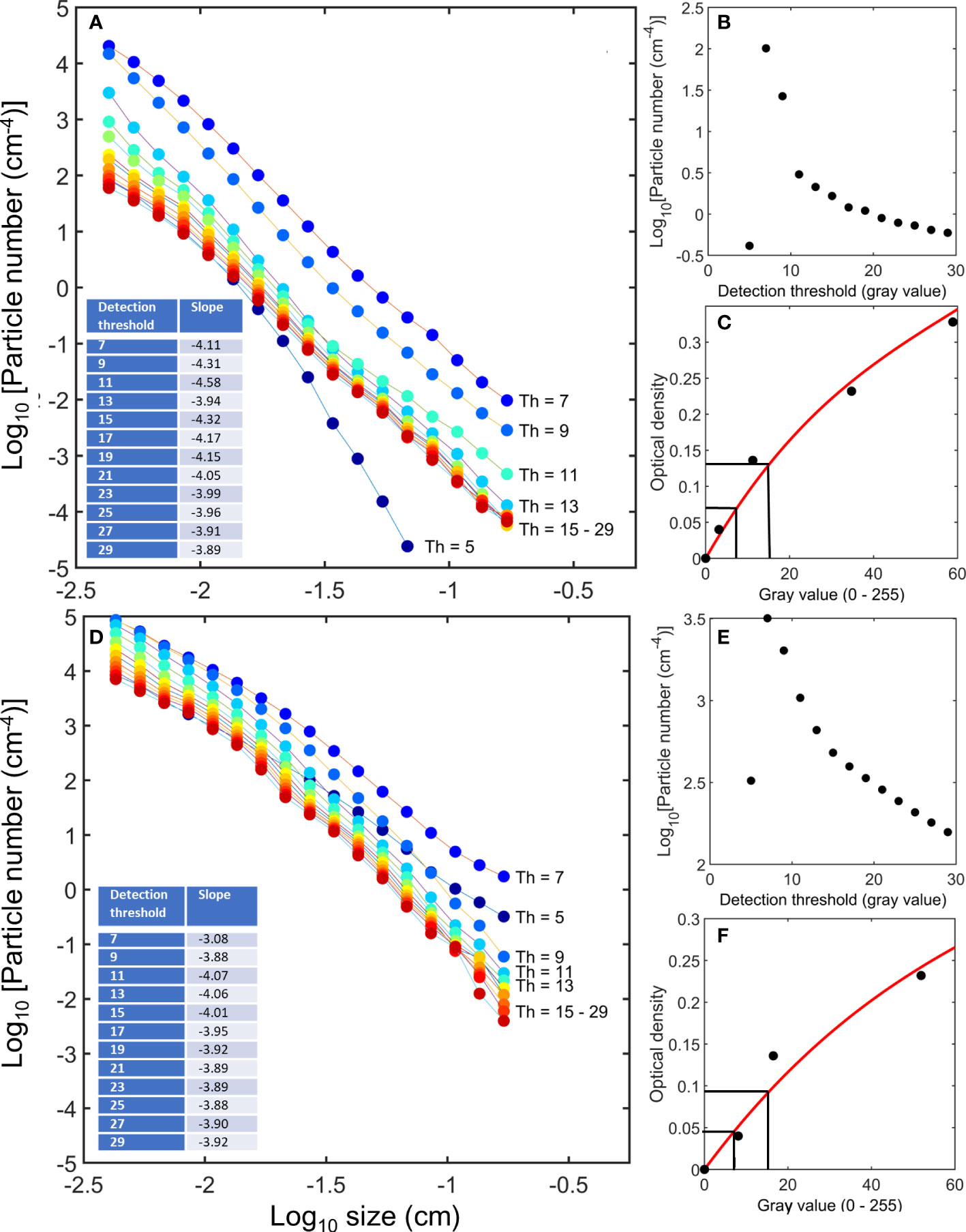
Figure 1 Particle number spectra of particles in the size range of 43 μm to 1.7 mm in the Sargasso Sea (A) and the Gulf of Trieste (D). Warmer colors (red to yellow) represent particle numbers at thresholds typically used in imaging systems. Blue colors indicate lower thresholds at which gels with associated microbial communities and faint detritus become visible. Applying lower detection thresholds, yielded vastly different particle numbers. Particle numbers increased continuously without shoulder or cutoff with decreasing thresholds except for a sudden drop due to image “whiteout” at threshold = 5 (particle size bin = 170 μm only, (B, E). This analysis demonstrates that faint particles (blue shades) outnumber particles that are typically detected (warm colors) by orders of magnitude. Using neutral density filters, threshold values can be translated into absolute attenuation values regardless of the instrument in use (C, F). Black lines in C and F correspond to thresholds 7 and 15.
Below a defined threshold value (7 in our analytical setup), the particle numbers appear to decrease as a result of overlapping particles in the 2-dimensional projection. At even lower thresholds of 5 and below, particles began to blend into each other and appear as fewer and larger particles, essentially creating a “whiteout”.
To calibrate the gray values in terms of absorbance, neutral density filters printed on a microscope slide were used to transform gray values into optical densities (Figures 1C, F). For instance, a gray value of a threshold of 7 translated to an attenuation value of 0.066 (Figure 1C) or 0.045 (Figure 1F), depending on the camera. This meant that for a pixel to be detected as part of a particle, at least 13% or 10% of the light needed to be blocked (attenuated), respectively. A threshold value of 15 in turn translated to an attenuation of 0.13 (26% attenuated, Figure 1C) or 0.091 (19% attenuated, Figure 1F), respectively.
Despite changes in the elevation of the regression in the number to size relationship at various threshold levels, the slopes remained relatively unchanged once the relationship emerged from the “whiteout” at thresholds > 5 (Figures 1A, D). The relative independence of the spectral slope from threshold levels indicates that faint particles are not restricted to the smallest size classes but occur proportionally to denser particles over the entire size range from 43 μm to 1.7 mm length. This may explain why the particle spectral slopes of many different particle analysis methods are similar despite the very different techniques in use.
At lower thresholds, shadowgraph images reveal a portion of the gel fraction
In laboratory experiments, we created a sequence of chemical additions that selectively changed the abundance of cation-bridged gel particles. Using alginates pre-hydrated in ultrapure water, we added in sequence 1) Ca2+ ions to promote gel formation by cation cross-linking, and 2) EDTA to chelate calcium ions and to redissolve gels (Figure 2). The response of particle abundance to calcium ions and EDTA, the coagulation and dissolution, respectively, is specific to gel particles (Passow, 2002; Meng and Liu, 2016), and does not occur with other more solid particles. Using the same image analysis techniques as in the field, we observed clear changes in particle numbers at a low threshold (Th = 7), while most of these particles remained undetectable at a higher threshold (Th = 15) (Figures 2A, B). Alcian Blue staining (Alldredge et al., 1993) of water from the experiments as they were conducted confirmed the coagulation and dissolution sequence of gels during the experiment (Figure 2C). The calcium addition caused a thick layer of gels on the filter (Figure 2C), while before the calcium, and after the EDTA addition, the gel particle numbers were much lower. There was a difference in total mass of gels detected in the binarized camera images (Figure 2B) and the Alcian blue staining (Figure 2C). However, this difference was simply the result of a higher retention of gel particles on the polycarbonate filters (pore size = 0.4 μm) in contrast to the size detection limit of particles in our optical setup (pixel resolution = 12.4 μm).
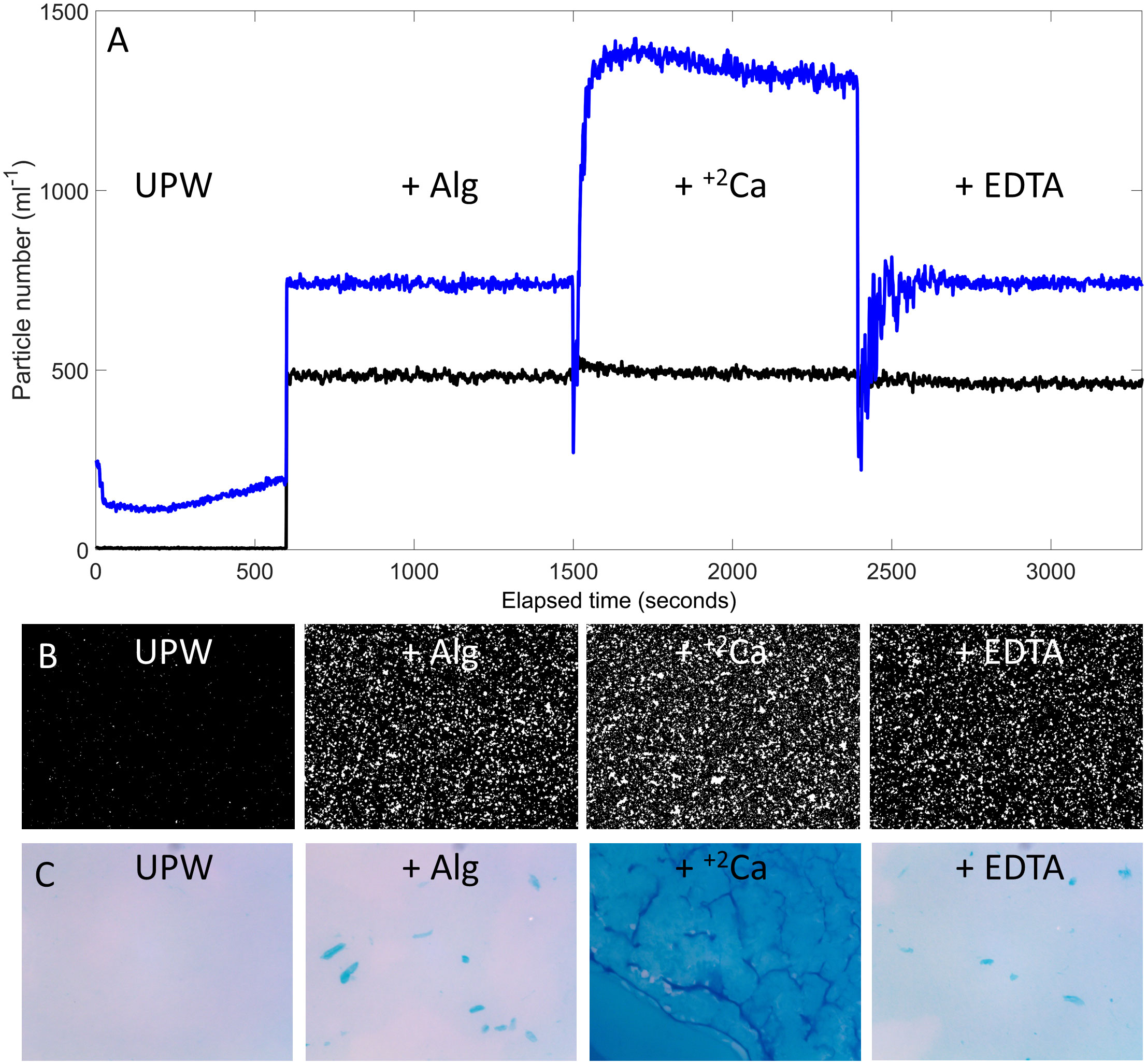
Figure 2 Laboratory experiments with alginates as models of aquatic gels. UPW = ultra-pure water (18.2 MΩ.cm), + Alg = solution of sodium alginates in UPW. Images are stacked up vertically in the time series. (A) Coagulation and dissolution dynamics of the gels due to the addition of calcium ions (+ +2Ca) and EDTA (+ EDTA), respectively, were only visible at a lower threshold (Th = 7, blue) and not at higher threshold (Th = 15, black). The short-lived decreases in particle numbers immediately after the chemical additions were due to temporary obfuscation of particles by schlieren effects caused by the different osmolarities of the liquids (Davidhazy, 2006) (B) Binarized example images during the course of the experiment (Th = 7 only). (C) During this sequence, Alcian blue staining of subsamples of water during the experiment shows the buildup of a thick gel layer on the filter due to cation bridges (due to the calcium addition), and dissolution after the addition of EDTA.
Lower thresholds reveal some but not all structural gels in nature
To confirm that particles as faint as gels can be detected with the same settings in situ, we performed the analysis on three types of natural particles known to contain gel-like matrices: hydromedusae, appendicularian houses, and algal exudates-based marine snow. In all three particle types, lowering thresholds increased the detail of the gel structures until the limit of the dynamic range of the camera was reached (Figure 3). The food collection tube and parts of the tail chamber appeared in the appendicularian house (Flood et al., 1990) (Figure 3A). Portions of the non-cellular mesoglea (Tiemann et al., 2002) appeared between the manubrium and the umbrella in the hydromedusa (Figure 3B). In marine snow, the matrix that connected larger pieces of the particle began to appear at lower thresholds (Figure 3C). However, even at the lowest thresholds, structures that we know to exist remained invisible (e.g., a missing portion of a tentacle, the large cushion chambers of the appendicularian house (Flood et al., 1990), or thin mucus threads that hold the marine snow particle together in the upper portion of this example).
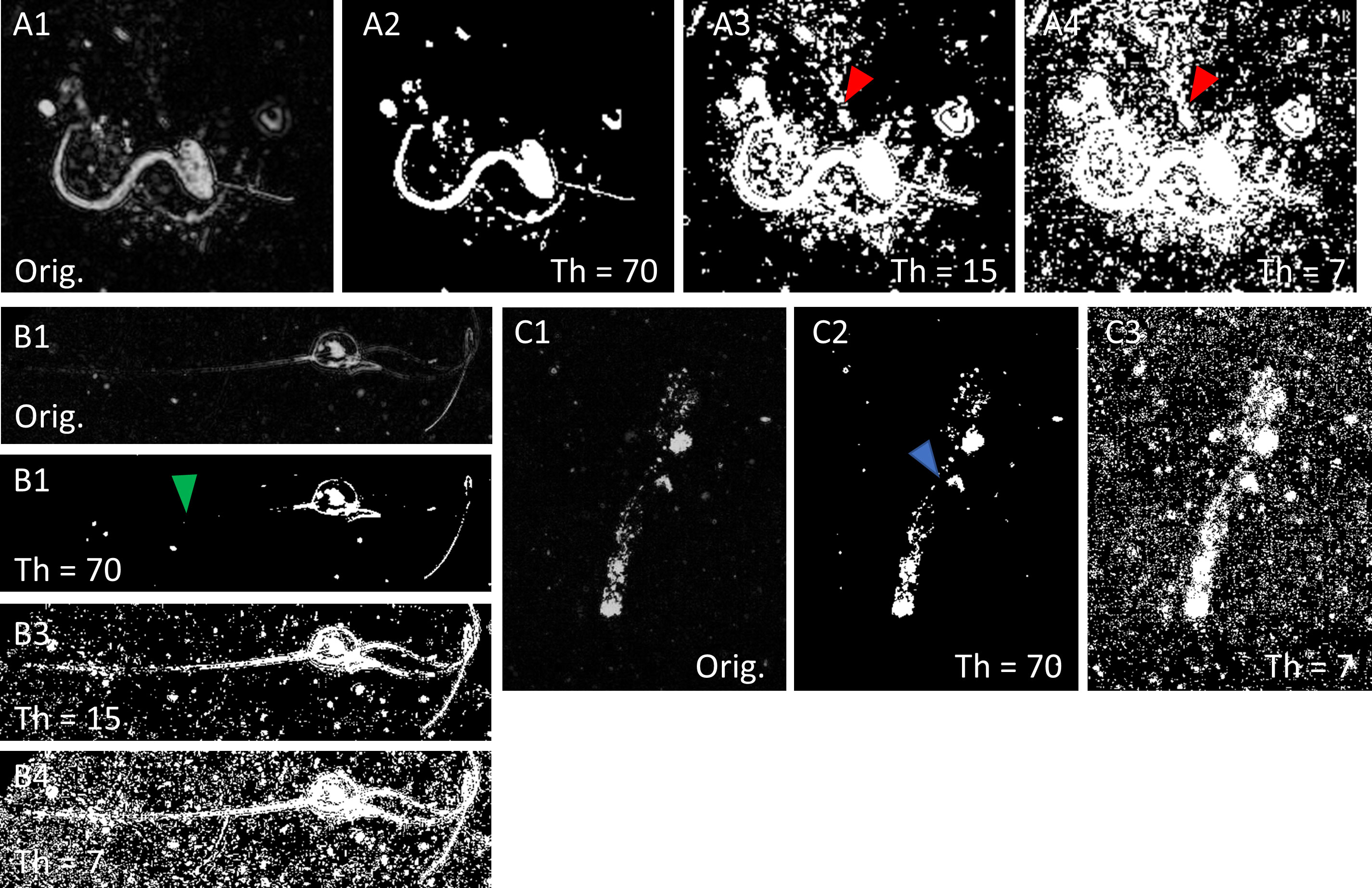
Figure 3 Images of plankton and particles in the Gulf of Trieste using shadowgraphy (note that images are inverted for better contrast of smaller objects). (A) Appendicularian (Oikopleura sp.) actively filtering in its house, (B) a hydromedusa, (C) a marine snow particle. The original gray scale images (A1, B1, C1) were binarized at various threshold levels (Th) (A2, 3, 4, B2, 3, 4, C2, 3). A high threshold level (Th = 70) isolates plankton and denser particles from the background while a low threshold makes faint particles and gel structures appear. Even at the lowest practical threshold level shown here (A4, B4, C3), particles are not exhaustively detected. Red arrows: food tube of the appendicularian house. Green arrow: missing tentacle in the binarization. Blue arrow: mucus strands connect lower and upper portions of the marine snow particle. By optimizing image algorithms to perfectly render the shapes of zooplankton for taxonomic identification, the vast abundance of faint particles (A4, B4, C3) remains unaccounted.
An incomplete rendering of objects due to an invisible gel matrix is a major problem for accurately measuring the sizes and shapes of marine snow particles. After binarization even at a relatively low threshold of Th=15, the aggregate in this example registers as many smaller ones (Figure 4). In this or similar cases, a potential - albeit computationally expensive - solution is the application of algorithms such as density-based clustering (e.g., the dbscan function using the Euclidean distance metric in Matlab) and then adding bounding regions around these clusters (e.g., the boundary function in Matlab, Figure 4B).
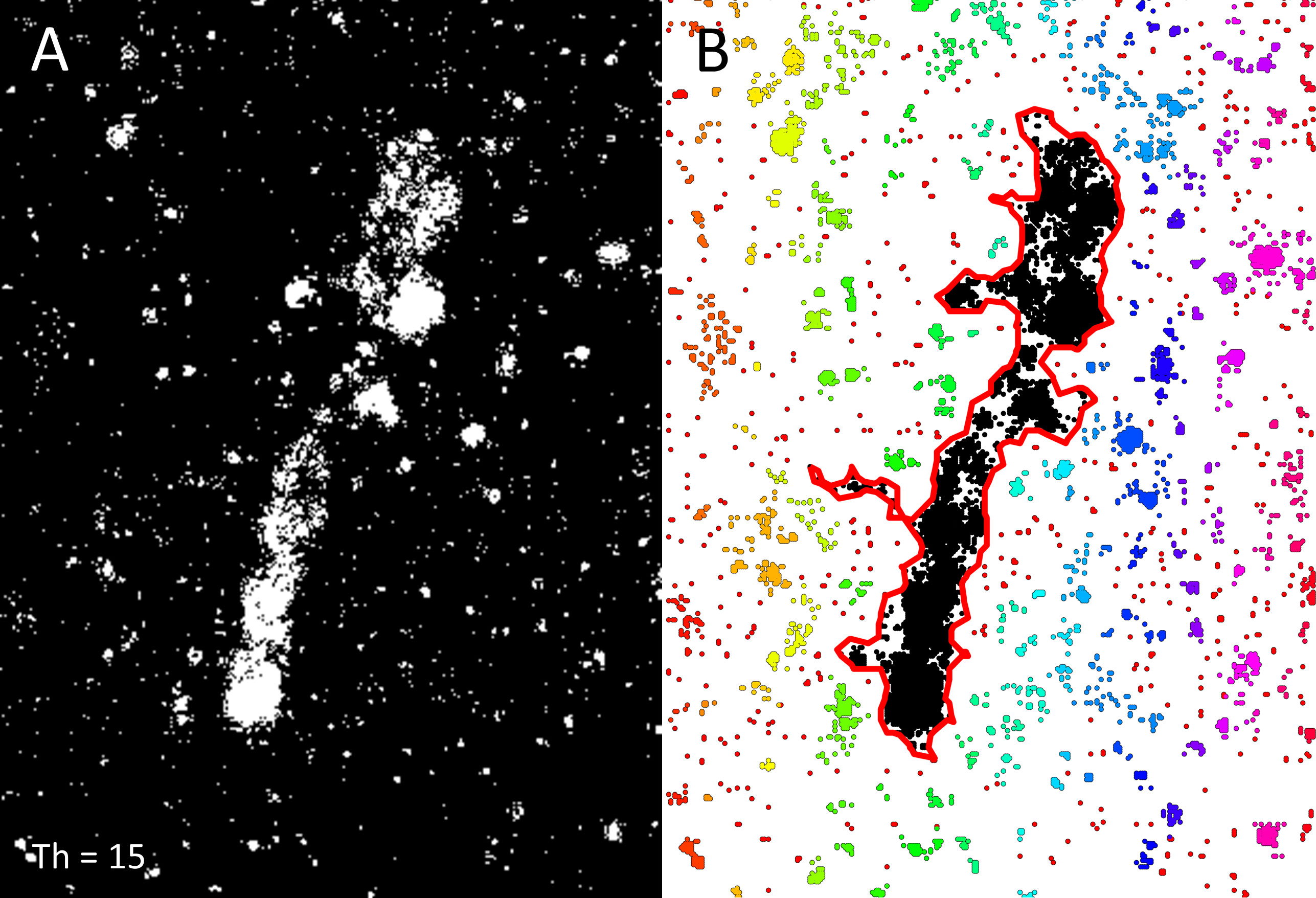
Figure 4 (A) Binarization of a shadowgraph of the amorphous marine aggregate (Figure 3) at threshold = 15. Parts of the mucous matrix are not detected at this threshold. (B) Density-based spatial clustering (Ester et al., 1996) assigns the same index value to components of the particle (black) so that a boundary function (red line) can envelope the entire marine snow particle. In such fashion, a more accurate size and shape determination can be performed on fragmented structures. Colors other than black represent more loosely defined particle associations.
As is apparent in Figure 3, large numbers of particles surrounding the main objects also became visible at lower thresholds (Figures 3A4, B4, C3). To distinguish whether these features represent actual particles or noise-generated patterns, we randomly selected ten binarized images each at threshold 7 from the Sargasso Sea and the Gulf of Trieste and resampled a 900 x 900 pixel region from each image at five window sizes (3x3, 9x9, 15x15, 30x30, 300x300 pixels) to calculate the Morisita Index of Dispersion (IM) (Morisita, 1962; Hurlbert, 1990). The IM is used widely in ecology to determine the degree of clustering in the spatial distribution of species (Krebs, 1999). A random distribution of objects results in an IM of 1. If the IM is less than 1, the population is underdispersed (i.e., more evenly distributed than random), and if the IM is significantly larger than 1, the population is overdispersed (i.e., clustered or patchy). We used this principle to determine if image pixels after binarization are clustered (i.e., part of aggregates). We resampled the images at the five different scales because the IM can be sensitive to the size and number of the counting quadrats (Amaral et al., 2015; Hayes and Castillo, 2017). The IM ranged from an average of 3.77 (SD = 0.677) for 3x3 pixel windows to 1.29 (SD = 0.551) for 300x300 pixel windows for the Sargasso Sea, and from 2.24 (SD = 0.277) for 3x3 windows to 1.10 (SD = 0.018) for 300x300 windows for the Gulf of Trieste. In all cases, the index of dispersion was significantly different from a random distribution (i.e., IM = 1) using the chi-square test statistic (p<0.0001) (Hurlbert, 1990). Electronic noise that contributes to the signal at lower thresholds generally produces a random pixel signal. Having a significantly higher IM than expected from a random signal distribution demonstrates that aggregation of particles was observed through the noise. These aggregates or clusters are also clearly visible in the image portions surrounding the main objects in Figure 3.
Discussion
Decreasing the threshold of detection results in higher particle abundances, but even when the limits of the dynamic range of the optical setup is reached, the true particle number is under-estimated. This problem of threshold settings on particle detection is well-recognized, and is especially important in the context of rendering accurate size distributions for the smallest size range detected by the instrument and for the calculation of equivalent spherical diameters of non-spherical particles (Costello et al., 1994). Given different instrument’s operational differences in particle detection as well as image analysis algorithms (Giering et al., 2020; Markussen et al., 2020), it is thus not surprising that reported particle numbers vary widely in the literature even in the same environment. Standardization among platforms will make results more comparable (Picheral et al., 2010; Lombard et al., 2019; Giering et al., 2020; Neeley et al., 2021), but the problem goes beyond that of mere standardization and intercalibration of various optical systems. It is the high abundance of interconnected three-dimensional exopolymer fibers in aqueous systems that make them inherently difficult, if not - as our analysis suggests - impossible to enumerate accurately (Leppard et al., 1996; Verdugo et al., 2004; Wurl et al., 2011; Zamanillo et al., 2019). Transparent exopolymer particles, exopolymer substances, gels, larger organic aggregates and flocs are not restricted to oceanic environments but are also abundant in freshwater systems (Grossart et al., 1997; Bar-Zeev et al., 2015; Shi et al., 2021; Walch et al., 2022). We thus expect the conundrum of particle detection thresholds in imaging systems to fully apply in freshwater as well. In contrast, particle numbers may not be as ambiguous when accounting for aerosols, a suspension of particles in a gaseous medium (Li et al., 2016).
Because particle number is a function of threshold levels without a defined cutoff, particle number can only be determined in relative, not in absolute terms. Oceanic particles – even in the oligotrophic Sargasso Sea – overlap in the image before the absolute number of particles is reached (Figures 1B, C). This occurs even at the relatively modest optical path of 3 cm which is small by comparison to other in-situ instruments (Cowen and Guigand, 2008; Ohman et al., 2019). Decreasing the thickness of the optical slice does not eliminate the problem; it only shifts the peak particle abundance to lower thresholds. In this study, the relative change in particle numbers with the decreasing threshold levels was greater in the Sargasso Sea than in the Gulf of Trieste. Whether this skewed distribution towards fainter particles in the Sargasso Sea was a result of decreased background turbidity at which fainter particles were able to stand out more, or of a proportionally larger pool of faint particles cannot be ascertained from our data.
The faint gel-like particles observed with our instrument are only the tip of the iceberg of a much larger undetected pool of particles forming a continuum from the particulate to the dissolved phase (Verdugo et al., 2004). That only a fraction of the gels could be captured by our camera was reflected in the results of the laboratory experiments where alginates created a thick layer on the 0.4 μm pore-size filter but only added a modicum of larger particles to the images (Figure 2). Macrogels and some microgels are collected by 0.2 and 0.4 μm pore-size polycarbonate filters and even by GF/F glass fiber filters with effective pore sizes ranging from 0.2 μm to 0.7 μm depending on the temperature of combustion (Nayar and Chou, 2003; Verdugo et al., 2004; Chaves et al., 2021). As combusted glass fiber filters are used in the very definition of particulate organic carbon and nitrogen, gels should not be excluded from optical analysis, otherwise too much carbon will be erroneously associated with optically denser particles. The inclusion of fainter particles in the optical analysis as we suggest here (i.e., the use of lower thresholds) will thus reduce one important source error in any attempt to translate particle images into carbon values.
While imaging systems other than shadowgraphy may not have the same dynamic range or sensitivity (e.g., Markussen et al., 2020), the problem of an arbitrary threshold setting remains the same. In all cases, it is the sensitivity of the camera that ultimately sets the threshold, not the image analysis algorithm. In back- or side-scatter configurations (Davis et al., 2005; Picheral et al., 2010) the relevant metric in particle detection is scatter, and gels also have poorer backscatter characteristics than, for instance, pigmented particles or ones that contain calcite (Collister et al., 2020). The small-angle forward scattering properties are sensitive to the reflectance and transparency of particles, as well as on their geometry (Boss et al., 2009). Consequently, particle analyses based on laser scattering (e.g., laser in-situ scattering and transmissometry (LISST), Boss et al., 2018) suffer similar problems of poor detection of particles with a high content of exopolymers and refractive indices close to water. Thus, instrument calibrations based upon highly scattering reference materials such as latex or PVC spheres may not accurately reflect the numbers of less optically dense and non-spherical plankton and gels. Non-optical systems such as impedance measurements are also affected by threshold settings. For instance, Coulter Counters do not detect gels and are best suited to counting phytoplankton cells, especially those with hard frustules such as diatoms or coccolithophorids, which make LISST and Coulter Counter data agree well (Reynolds et al., 2010).
At lower thresholds of detection, shadowgraphy is very sensitive to schlieren effects caused by inhomogeneities of the refractive index due to density changes (Davidhazy, 2006). This problem was identified previously in transmissometry and LISST data resulting in “particle peaks” associated with pycnoclines as a result of increased light scattering (Bogucki et al., 1998; Mikkelsen and Pejrup, 2001; Styles, 2006; Karageorgis et al., 2015). In our analysis of field data, we avoided depths with schlieren patterns, which were only detectable in strong pycnoclines. In the laboratory, schlieren formation occurred briefly after gels or ions were added (in this case the effect was obfuscation of the more numerous smaller particles by the larger schlieren effects, Figure 2) but dispersed quickly due to continuous use of the magnetic stirrer. If analysis is performed using lower thresholds to detect fainter gel particles, care must be taken to either exclude images that contain schlieren from image analysis or develop deep-learning algorithms that identify and exclude schlieren artifacts within individual images.
Most settings of the camera-image analysis pairings are adjusted so that sufficient detail can be recovered in plankton organisms for taxonomic identification (Davis et al., 2005; Cowen and Guigand, 2008; Picheral et al., 2010; Lombard et al., 2019; Ohman et al., 2019; Gillard et al., 2022; Picheral et al., 2022), however, these organisms are at the high end in the range of optical densities and scattering values. By adjusting threshold levels to optimize visualization of plankton, the optical system becomes blind to the much more numerous fainter particles. A quandary exists because while exceedingly low thresholds detect more faint particles, there is a loss of image detail that contains important information for taxonomic identification (Figure 3). To solve this dichotomy, at least two passes are required for each image: one that captures plankton to produce high quality segments at a higher threshold, and a second one at the lowest practical threshold that more accurately enumerates faint particles.
The co-occurrence of gels with more solid particles would be no impediment to accurate particle enumeration if the separation between the two could be made clearly. However, as our study shows, there is no clear cutoff or shoulder in the relationship between particle numbers and thresholds, or any other reliable indicator of a clear division between gels and denser particles. Importantly, pure gels simply do not exist in aquatic environments; gels are heavily colonized by microorganisms (e.g., Busch et al., 2017), and particles more optically dense than gels (from clays and phytoplankton to fecal pellets) stick to the gel polymers. This invariably changes the overall optical characteristics in a continuum, making the definition of an objective cutoff intractable. An asymptote may exist towards the higher threshold ranges (Figures 1B, E) but we strongly discourage the use of such a non-inclusive metric as its application only enumerates rare particles with the highest light attenuation.
Marine snow (large amorphous aggregates >500 μm) dominate particle mass in many ocean provinces such as upwelling systems and regional seas (Alldredge and Silver, 1988; Rinaldi et al., 1995; Jackson et al., 1997; Trudnowska et al., 2021), and may be more abundant than plankton organisms of the same size (Parsons and Strickland, 1962; Bochdansky et al., 2016). Pumps, filters, plankton nets and large pore size screens destroy fragile marine snow aggregates, which means that marine snow is greatly under sampled with most physical collection methods (Trent et al., 1978; Alldredge and Silver, 1988; Bochdansky and Herndl, 1992; González-Quirós and Checkley, 2006). As we show here, marine snow is also “optically fragile” as fragmentation during segmentation during image analysis occurs (Thuy et al., 2017). This problem is exacerbated at high threshold settings. In both physical and optical methods, large marine snow particles are thus misclassified as many smaller particles (Figure 4A). Image analysis algorithms that fill in the object after edge detection (Giering et al., 2020) typically fail in rendering these amorphous aggregates because, unlike plankton, they do not have well-defined outlines. If fragmentation occurs evenly across the particle size spectrum, and fragments of larger particles are sequestered into the bins of the much more numerous smaller particles, the overall slope in the particle number spectrum will hardly be affected. However, there are many examples of specific “bumps” in the spectrum with disproportionally higher numbers in some size fractions due to strong blooms of specific plankton organisms, and especially due to the occurrence of marine snow aggregates both at the surface and in the deep ocean (Rodrı́guez et al., 2002; Iversen et al., 2010; Bochdansky et al., 2016; Runyan et al., 2020). These particles peaks may become less pronounced with automated image analysis if optical fragmentation is allowed to occur. A solution to recapturing the shapes and sizes of these larger particles may lie in statistical approaches that use clustering algorithms to detect and better describe marine snow particles. Density-based spatial clustering (Ester et al., 1996), demonstrated on the marine snow example in Figure 4B, indicates that once clustered, bounding polygons can better delineate the spatial extent of a particle that is inherently fractal in nature (Kilps et al., 1994).
The concept of fractal dimension is relevant in two aspects: First, fractal geometry changes with porosity and density of the particle (Logan and Wilkinson, 1990; Azetsu-Scott and Johnson, 1992; Khelifa and Hill, 2006) and has been observed to change as the floc size increases, leading to a lower effective density of flocs (Khelifa and Hill, 2006). Second, fractals are also relevant in analogy to the problem of scale. As in the well-known coastline paradox, where the coastline does not have a well-defined length (i.e., the length of the coastline is directly related to the length of the “measuring stick”, Mandelbrot, 1967), we find ourselves confronted with a similar problem here: particle numbers per volume are a direct function of the threshold settings of the camera (i.e., the scale in this case), and the dependence between particle number and threshold levels spans orders of magnitude (Figure 1).
Ill-defined particle numbers have consequences for a variety of predictions of coagulation theory (Jackson and Burd, 1998; Burd, 2013). Coagulation is highly dependent on the number of particles per volume, which greatly influences overall particle mass and collision rate through nearest-neighbor distances. Transparent exopolymer particles (a subgroup of extracellular polymeric substances) are key to aggregation phenomena (Mari et al., 2017) but are typically not accounted for with conventional optical systems unless they are stained with specific dyes [e.g., Alcian blue (Alldredge et al., 1993), Coomassie brilliant blue (Long and Azam, 1996)]. It is exactly those particles, however, that form the glue that binds larger particles together. This has important consequences for the vertical carbon flux because aggregation is an important factor in creating particles of larger sizes that according to Stokes’ law, and all else being equal, sink faster than smaller particles.
Although absolute particle numbers in a volume of water remain elusive, the slope of the number spectrum is relatively conserved across many different thresholds. There was a very slight but significant shallowing of the negative slopes with increasing threshold levels (from 7 to 29) for the Sargasso Sea (linear regressions, n= 12, F = 8.10, p=0.0157) but not in the Gulf of Trieste (linear regression, n= 12, F = 1.26, p=0.344). Particle number slopes typically range from -2 to -5, with most eutrophic and coastal regions converging on slopes of -3 to -3.5, and oligotrophic open ocean systems on slopes closer to -4.5 (Guidi et al., 2009). Consistent with this concept, our Sargasso Sea data had an average slope of -4.12 (SD = 0.206, n =12), characteristic of these open ocean systems. The Gulf of Trieste data, in contrast, displayed a lower average slope of -3.87 (SD = 0.258 n = 12). The independence of the particle number slope on the sensitivity level of the analysis is reassuring and validates the use of these slopes as an intrinsic water mass characteristic (Stemmann et al., 2008; Guidi et al., 2009; Runyan et al., 2020; Chaikalis et al., 2021).
Our study demonstrates that all literature reports of particle numbers per unit volume in aqueous systems need to be treated with great skepticism, as they do not represent “true” particle numbers regardless of the methods employed. For data on particle numbers per volume to be useful, they must be accompanied by information on a precise threshold defined by a specific optical property such as optical density, scatter or reflectance of representative calibration objects. While most laboratories optimize their analyses towards the detection and classification of plankton (warm colors in Figure 1), analysis at lower thresholds (blue shades in Figure 1) is better suited for understanding the oceanic particle landscape and its heterogeneity at spatial scales that are most relevant to organisms that navigate them (Azam and Long, 2001; Stocker, 2012; Seymour et al., 2017).
Data availability statement
The datasets presented in this study can be found in online repositories. The names of the repository/repositories and accession number(s) can be found below: Biological & Chemical Oceanography Data Management Office # OCE-2128438.
Author contributions
AB designed, built and deployed the camera system, analyzed data, produced the figures and wrote the manuscript. HH performed image analysis, analyzed data, and conducted the laboratory experiments. MC led the Sargasso Sea research expedition, facilitated deployment of the camera, provided oceanographic context data, and co-wrote the manuscript. All authors contributed to the article and approved the submitted version.
Funding
This study was funded by the U.S. National Science Foundation Chemical Oceanography program award OCE 1829885 supporting the Oceanic FluxProgram time-series (MC), and the U. S. National Science Foundation Biological and Chemical Oceanography Program awards OCE 1851368 (AB) and OCE 2128438 (AB).
Acknowledgments
We thank the crew of the RV Atlantic Explorer for assistance during the deployment of the camera system, Charles Sukenik, Department of Physics, Old Dominion University, for use of their optical bench and equipment, and Karoline Bochdansky for assistance during dive operations.
Conflict of interest
The authors declare that the research was conducted in the absence of any commercial or financial relationships that could be construed as a potential conflict of interest.
Publisher’s note
All claims expressed in this article are solely those of the authors and do not necessarily represent those of their affiliated organizations, or those of the publisher, the editors and the reviewers. Any product that may be evaluated in this article, or claim that may be made by its manufacturer, is not guaranteed or endorsed by the publisher.
References
Alldredge A. L., Passow U., Logan B. E. (1993). The abundance and significance of a class of large, transparent organic particles in the ocean. Deep Sea Res. Part Oceanogr. Res. Pap. 40, 1131–1140. doi: 10.1016/0967-0637(93)90129-Q
Alldredge A. L., Silver M. W. (1988). Characteristics, dynamics and significance of marine snow. Prog. Oceanogr. 20, 41–82. doi: 10.1016/0079-6611(88)90053-5
Amaral M. K., Pellico Netto S., Lingnau C., Figueiredo Filho A. (2015). Evaluation of the morisita index for determination of the spatial distribution of species in a fragment of araucaria forest. Appl. Ecol. Environ. Res. 13, 361–372. doi: 10.15666/aeer/1302_361372
Arnold G. P., Nuttall-Smith P. B. N. (1974). Shadow cinematography of fish larvae. Mar. Biol. 28, 51–53. doi: 10.1007/BF00389116
Azetsu-Scott K., Johnson B. D. (1992). Measuring physical characteristics of particles: a new method of simultaneous measurement for size, settling velocity and density of constituent matter. Deep Sea Res. Part Oceanogr. Res. Pap. 39, 1057–1066. doi: 10.1016/0198-0149(92)90039-V
Bar-Zeev E., Passow U., Romero-Vargas Castrillón S., Elimelech M. (2015). Transparent exopolymer particles: From aquatic environments and engineered systems to membrane biofouling. Environ. Sci. Technol. 49, 691–707. doi: 10.1021/es5041738
Bochdansky A. B., Clouse M. A., Hansell D. A. (2017). Mesoscale and high-frequency variability of macroscopic particles (> 100 μm) in the Ross Sea and its relevance for late-season particulate carbon export. J. Mar. Syst. 166, 120–131. doi: 10.1016/j.jmarsys.2016.08.010
Bochdansky A. B., Clouse M. A., Herndl G. J. (2016). Dragon kings of the deep sea: Marine particles deviate markedly from the common number-size spectrum. Sci. Rep. 6, 22633. doi: 10.1038/srep22633
Bochdansky A., Herndl G. (1992). Ecology of amorphous aggregations (marine snow) in the northern Adriatic sea. v. role of fecal pellets in marine snow. Mar. Ecol. Prog. Ser. 89, 297–303. doi: 10.3354/meps089297
Bochdansky A. B., Jericho M. H., Herndl G. J. (2013). Development and deployment of a point-source digital inline holographic microscope for the study of plankton and particles to a depth of 6000 m: Deep-sea holographic microscopy. Limnol. Oceanogr. Methods 11, 28–40. doi: 10.4319/lom.2013.11.28
Bogucki D. J., Domaradzki J. A., Stramski D., Zaneveld J. R. (1998). Comparison of near-forward light scattering on oceanic turbulence and particles. Appl. Opt. 37, 4669. doi: 10.1364/AO.37.004669
Boss E., Guidi L., Richardson M. J., Stemmann L., Gardner W., Bishop J. K. B., et al. (2015). Optical techniques for remote and in-situ characterization of particles pertinent to GEOTRACES. Prog. Oceanogr. 133, 43–54. doi: 10.1016/j.pocean.2014.09.007
Boss E., Haëntjens N., Westberry T. K., Karp-Boss L., Slade W. H. (2018). Validation of the particle size distribution obtained with the laser in-situ scattering and transmission (LISST) meter in flow-through mode. Opt. Express 26, 11125. doi: 10.1364/OE.26.011125
Boss E., Slade W. H., Behrenfeld M., Dall’Olmo G. (2009). Acceptance angle effects on the beam attenuation in the ocean. Opt. Express 17, 1535. doi: 10.1364/OE.17.001535
Burd A. B. (2013). Modeling particle aggregation using size class and size spectrum approaches: Modeling particle aggregation. J. Geophys. Res. Oceans 118, 3431–3443. doi: 10.1002/jgrc.20255
Busch K., Endres S., Iversen M. H., Michels J., Nöthig E.-M., Engel A. (2017). Bacterial colonization and vertical distribution of marine gel particles (TEP and CSP) in the Arctic fram strait. Front. Mar. Sci. 4. doi: 10.3389/fmars.2017.00166
Chaikalis S., Parinos C., Möbius J., Gogou A., Velaoras D., Hainbucher D., et al. (2021). Optical properties and biochemical indices of marine particles in the open Mediterranean Sea: The R/V maria s. merian cruise, march 2018. Front. Earth Sci. 9. doi: 10.3389/feart.2021.614703
Chaves J. E., Cetinić I., Dall’Olmo G., Estapa M., Goñi M., Graff J. R., et al. (2019). Particulate organic carbon sampling and measurement protocols: consensus towards future ocean color missions. International Ocean Colour Coordinating Group report. pp. 48. Available at: https://ioccg.org/what-we-do/ioccgpublications/.
Collister B. L., Zimmerman R. C., Hill V. J., Sukenik C. I., Balch W. M. (2020). Polarized lidar and ocean particles: Insights from a mesoscale coccolithophore bloom. Appl. Opt. 59, 4650. doi: 10.1364/AO.389845
Conte M. H., Ralph N., Ross E. H. (2001). Seasonal and interannual variability in deep ocean particle fluxes at the oceanic flux program (OFP)/Bermuda Atlantic time series (BATS) site in the western Sargasso Sea near Bermuda. Deep Sea Res. Part II Top. Stud. Oceanogr. 48, 1471–1505. doi: 10.1016/S0967-0645(00)00150-8
Costello D. K., Hou W., Carder K. L. (1994). Some effects of the sensitivity threshold and spatial resolution of a particle imaging system on the shape of the measured particle size distribution. Ed. Jaffe J. S. (Bergen, Norway, SPIE Digital Library), 768–783. doi: 10.1117/12.190124
Cowen R. K., Guigand C. M. (2008). In situ ichthyoplankton imaging system ( I SIIS): System design and preliminary results. Limnol. Oceanogr. Methods 6, 126–132. doi: 10.4319/lom.2008.6.126
Davidhazy A. (2006) Introduction to shadowgraph and schlieren imaging. Available at: http://scholarworks.rit.edu/article/478.59.
Davis C. S., Thwaites F. T., Gallager S. M., Hu Q. (2005). A three-axis fast-tow digital video plankton recorder for rapid surveys of plankton taxa and hydrography: New video plankton recorder. Limnol. Oceanogr. Methods 3, 59–74. doi: 10.4319/lom.2005.3.59
Ester M., Kriegel H.-P., Xu X. (1996). A density-based algorithm for discovering clusters in Large spatial databases with noise, KDD-96 Proceedings in Proc. Second Int. Conf. Knowl. Discov. Databases Data Min. 226–231 (Portland OR: AAAI Press).
Flood P. R., Deibel D., Morris C. C. (1990). Visualization of the transparent, gelatinous house of the pelagic tunicate Oikopleura vanhoeffeni using Sepia ink. Biol. Bull. 178, 118–125. doi: 10.2307/1541970
Forest A., Stemmann L., Picheral M., Burdorf L., Robert D., Fortier L., et al. (2012). Size distribution of particles and zooplankton across the shelf-basin system in southeast Beaufort Sea: Combined results from an underwater vision profiler and vertical net tows. Biogeosciences 9, 1301–1320. doi: 10.5194/bg-9-1301-2012
Ghasemi M., Alexandridis P., Tsianou M. (2018). Conversion of particle size distribution data from mass to number-based and its application to biomass processing. Biosyst. Eng. 176, 73–87. doi: 10.1016/j.biosystemseng.2018.10.007
Giering S. L. C., Hosking B., Briggs N., Iversen M. H. (2020). The interpretation of particle size, shape, and carbon flux of marine particle images is strongly affected by the choice of particle detection algorithm. Front. Mar. Sci. 7. doi: 10.3389/fmars.2020.00564
Gillard B., Harbour R. P., Nowald N., Thomsen L., Iversen M. H. (2022). Vertical distribution of particulate matter in the clarion clipperton zone (German sector)–potential impacts from deep-Sea mining discharge in the water column. Front. Mar. Sci. 9. doi: 10.3389/fmars.2022.820947
González-Quirós R., Checkley D. M. Jr (2006). Occurrence of fragile particles inferred from optical plankton counters used in situ and to analyze net samples collected simultaneously. J. Geophys. Res. 111, C05S06. doi: 10.1029/2005JC003084
Grossart H.-P., Simon M., Logan B. E. (1997). Formation of macroscopic organic aggregates (lake snow) in a large lake: The significance of transparent exopolymer particles, phytoplankton, and zooplankton. Limnol. Oceanogr. 42, 1651–1659. doi: 10.4319/lo.1993.38.3.0532
Guidi L., Stemmann L., Jackson G. A., Ibanez F., Claustre H., Legendre L., et al. (2009). Effects of phytoplankton community on production, size, and export of large aggregates: A world-ocean analysis. Limnol. Oceanogr. 54, 1951–1963. doi: 10.4319/lo.2009.54.6.1951
Gustafsson C., Gschwend P. M. (1997). Aquatic colloids: Concepts, definitions, and current challenges. Limnol. Oceanogr. 42, 519–528. doi: 10.4319/lo.1997.42.3.0519
Hatton I. A., Heneghan R. F., Bar-On Y. M., Galbraith E. D. (2021). The global ocean size spectrum from bacteria to whales. Sci. Adv. 7, eabh3732. doi: 10.1126/sciadv.abh3732
Hayes J. J., Castillo O. (2017). A new approach for interpreting the morisita index of aggregation through quadrat size. ISPRS Int. J. Geo-Inf. 6, 296. doi: 10.3390/ijgi6100296
Hurlbert S. H. (1990). Spatial distribution of the montane unicorn. Oikos 58, 257. doi: 10.2307/3545216
Iversen M. H., Nowald N., Ploug H., Jackson G. A., Fischer G. (2010). High resolution profiles of vertical particulate organic matter export off cape blanc, Mauritania: Degradation processes and ballasting effects. Deep Sea Res. Part Oceanogr. Res. Pap. 57, 771–784. doi: 10.1016/j.dsr.2010.03.007
Jackson G. A., Burd A. B. (1998). Aggregation in the marine environment. Environ. Sci. Technol. 32, 2805–2814. doi: 10.1021/es980251w
Jackson G. A., Maffione R., Costello D. K., Alldredge A. L., Logan B. E., Dam H. G. (1997). Particle size spectra between 1 μm and 1 cm at Monterey bay determined using multiple instruments. Deep Sea Res. Part Oceanogr. Res. Pap. 44, 1739–1767. doi: 10.1016/S0967-0637(97)00029-0
Jonasz M., Fournier G. R. (2007). Light scattering by particles in water: theoretical and experimental foundations. 1st ed (London: Burlington, MA Academic Press).
Karageorgis A., Georgopoulos D., Gardner W., Mikkelsen O., Velaoras D. (2015). How schlieren affects beam transmissometers and LISST-deep: An example from the stratified Danube river delta, NW black Sea. Mediterr. Mar. Sci. 16, 366. doi: 10.12681/mms.1116
Khelifa A., Hill P. S. (2006). Models for effective density and settling velocity of flocs. J. Hydraul. Res. 44, 390–401. doi: 10.1080/00221686.2006.9521690
Kilps J. R., Logan B. E., Alldredge A. L. (1994). Fractal dimensions of marine snow determined from image analysis of in situ photographs. Deep Sea Res. Part Oceanogr. Res. Pap. 41, 1159–1169. doi: 10.1016/0967-0637(94)90038-8
Lange B. (2022). Fixed focal length or telecentric lens? the perspective decides – concept and advantages of telecentric lenses. PhotonicsViews 19, 41–43. doi: 10.1002/phvs.202200034
Leppard G. G., Heissenberger A., Herndl G. J. (1996). Ultrastructure of marine snow. i. transmission electron microscopy methodology. Mar. Ecol. Prog. Ser. 135, 289–298. doi: 10.3354/meps135289
Li W., Shao L., Zhang D., Ro C.-U., Hu M., Bi X., et al. (2016). A review of single aerosol particle studies in the atmosphere of East Asia: Morphology, mixing state, source, and heterogeneous reactions. J. Clean. Prod. 112, 1330–1349. doi: 10.1016/j.jclepro.2015.04.050
Logan B. E., Grossart H.-P., Simon M. (1994). Direct observation of phytoplankton, TEP and aggregates on polycarbonate filters using brightfield microscopy. J. Plankton Res. 16, 1811–1815. doi: 10.1093/plankt/16.12.1811
Logan B. E., Wilkinson D. B. (1990). Fractal geometry of marine snow and other biological aggregates. Limnol. Oceanogr. 35, 130–136. doi: 10.4319/lo.1990.35.1.0130
Lombard F., Boss E., Waite A. M., Vogt M., Uitz J., Stemmann L., et al. (2019). Globally consistent quantitative observations of planktonic ecosystems. Front. Mar. Sci. 6. doi: 10.3389/fmars.2019.00196
Long R. A., Azam F. (1996). Abundant protein-containing particles in the sea. Aquat. Microb. Ecol. 10, 213–221. doi: 10.3354/ame010213
Mandelbrot B. (1967). How long is the coast of Britain? Statistical self-similarity and fractional dimension. Science 156, 636–638. doi: 10.1126/science.156.3775.636
Manly B. F. J. (2007). Randomization, bootstrap, and Monte Carlo methods in biology. 3rd ed (Boca Raton, FL: Chapman & Hall/ CRC).
Mari X., Passow U., Migon C., Burd A. B., Legendre L. (2017). Transparent exopolymer particles: Effects on carbon cycling in the ocean. Prog. Oceanogr. 151, 13–37. doi: 10.1016/j.pocean.2016.11.002
Markussen T. N., Konrad C., Waldmann C., Becker M., Fischer G., Iversen M. H. (2020). Tracks in the snow – advantage of combining optical methods to characterize marine particles and aggregates. Front. Mar. Sci. 7. doi: 10.3389/fmars.2020.00476
Meng S., Liu Y. (2016). New insights into transparent exopolymer particles (TEP) formation from precursor materials at various Na+/Ca2+ ratios. Sci. Rep. 6, 19747. doi: 10.1038/srep19747
Mikkelsen O., Pejrup M. (2001). The use of a LISST-100 laser particle sizer for in-situ estimates of floc size, density and settling velocity. Geo-Mar. Lett. 20, 187–195. doi: 10.1007/s003670100064
Morisita M. (1962). Iσ -index, a measure of dispersion of individuals. Popul. Ecol. 4, 1–7. doi: 10.1007/BF02533903
Nayar S., Chou L. M. (2003). Relative efficiencies of different filters in retaining phytoplankton for pigment and productivity studies. Estuar. Coast. Shelf Sci. 58, 241–248. doi: 10.1016/S0272-7714(03)00075-1
Neeley A., Beaulieu S. E., Proctor C., Cetinić I., Futrelle J., Soto Ramos I., et al. (2021). Standards and practices for reporting plankton and other particle observations from images (Woods Hole Oceanographic Institution). doi: 10.1575/1912/27377
Ohman M. D., Davis R. E., Sherman J. T., Grindley K. R., Whitmore B. M., Nickels C. F., et al. (2019). Zooglider: an autonomous vehicle for optical and acoustic sensing of zooplankton: Autonomous Zooglider. Limnol. Oceanogr. Methods 17, 69–86. doi: 10.1002/lom3.10301
Omand M. M., D’Asaro E. A., Lee C. M., Perry M. J., Briggs N., Cetini I., et al. (2015). Eddy-driven subduction exports particulate organic carbon from the spring bloom. Science 348, 222–225. doi: 10.1126/science.1260062
Parsons T. R., Strickland J. D. H. (1962). Oceanic detritus. Science 136, 313–314. doi: 10.1126/science.136.3513.313
Passow U. (2002). Transparent exopolymer particles (TEP) in aquatic environments. Prog. Oceanogr. 55, 287–333. doi: 10.1016/S0079-6611(02)00138-6
Picheral M., Catalano C., Brousseau D., Claustre H., Coppola L., Leymarie E., et al. (2022). The underwater vision profiler 6: an imaging sensor of particle size spectra and plankton, for autonomous and cabled platforms. Limnol. Oceanogr. Methods 20, 115–129. doi: 10.1002/lom3.10475
Picheral M., Guidi L., Stemmann L., Karl D. M., Iddaoud G., Gorsky G. (2010). The underwater vision profiler 5: An advanced instrument for high spatial resolution studies of particle size spectra and zooplankton: Underwater vision profiler. Limnol. Oceanogr. Methods 8, 462–473. doi: 10.4319/lom.2010.8.462
Reynolds R. A., Stramski D., Wright V. M., Woźniak S. B. (2010). Measurements and characterization of particle size distributions in coastal waters. J. Geophys. Res. 115, C08024. doi: 10.1029/2009JC005930
Rinaldi A., Vollenweider R. A., Montanari G., Ferrari C. R., Ghetti A. (1995). Mucilages in Italian seas: The Adriatic and tyrrhenian seas 1988–1991. Sci. Total Environ. 165, 165–183. doi: 10.1016/0048-9697(95)04550-K
Rodrı́guez J., Jiménez-Gómez F., Blanco J. M., Figueroa F. L. (2002). Physical gradients and spatial variability of the size structure and composition of phytoplankton in the Gerlache Strait (Antarctica). Deep Sea Res. Part II Top. Stud. Oceanogr. 49, 693–706. doi: 10.1016/S0967-0645(01)00119-9
Runyan H., Reynolds R. A., Stramski D. (2020). Evaluation of particle size distribution metrics to estimate the relative contributions of different size fractions based on measurements in Arctic waters. J. Geophys. Res. Oceans 125. doi: 10.1029/2020JC016218
Samson S., Hopkins T., Remsen A., Langebrake L., Sutton T., Patten J. (2001). A system for high-resolution zooplankton imaging. IEEE J. Ocean. Eng. 26, 671–676. doi: 10.1109/48.972110
Seymour J. R., Amin S. A., Raina J.-B., Stocker R. (2017). Zooming in on the phycosphere: The ecological interface for phytoplankton–bacteria relationships. Nat. Microbiol. 2, 17065. doi: 10.1038/nmicrobiol.2017.65
Shi J., Yang Y., Yi Q., Zhang J., Wang L. (2021). Transparent exopolymer particles in drinking water treatment–a brief review. Int. J. Environ. Res. Public. Health 18, 12344. doi: 10.3390/ijerph182312344
Sosik H. M., Olson R. J. (2007). Automated taxonomic classification of phytoplankton sampled with imaging-in-flow cytometry: Phytoplankton image classification. Limnol. Oceanogr. Methods 5, 204–216. doi: 10.4319/lom.2007.5.204
Stemmann L., Eloire D., Sciandra A., Jackson G. A., Guidi L., Picheral M., et al. (2008). Volume distribution for particles between 3.5 to 2000 μm in the upper 200 m region of the south pacific gyre. Biogeosciences 5, 299–310. doi: 10.5194/bg-5-299-2008
Stocker R. (2012). Marine microbes see a Sea of gradients. Science 338, 628–633. doi: 10.1126/science.1208929
Styles R. (2006). Laboratory evaluation of the LISST in a stratified fluid. Mar. Geol. 227, 151–162. doi: 10.1016/j.margeo.2005.11.011
Thuy N. T., Huang C.-P., Lin J.-L. (2017). Visualization and quantification of transparent exopolymer particles (TEP) in freshwater using an auto-imaging approach. Environ. Sci. pollut. Res. 24, 17358–17372. doi: 10.1007/s11356-017-9292-y
Tiemann H., Sötje I., Jarms G., Paulmann C., Epple M., Hasse B. (2002). Calcium sulfate hemihydrate in statoliths of deep-sea medusae. J. Chem. Soc Dalton Trans., 2002(7), 1266–1268. doi: 10.1039/b111524c
Trent J. D., Shanks A. L., Silver M. W. (1978). In situ and laboratory measurements on macroscopic aggregates in Monterey bay, California 1: Macroscopic aggregates. Limnol. Oceanogr. 23, 626–635. doi: 10.4319/lo.1978.23.4.0626
Trudnowska E., Lacour L., Ardyna M., Rogge A., Irisson J. O., Waite A. M., et al. (2021). Marine snow morphology illuminates the evolution of phytoplankton blooms and determines their subsequent vertical export. Nat. Commun. 12, 2816. doi: 10.1038/s41467-021-22994-4
Verdugo P., Alldredge A. L., Azam F., Kirchman D. L., Passow U., Santschi P. H. (2004). The oceanic gel phase: A bridge in the DOM–POM continuum. Mar. Chem. 92, 67–85. doi: 10.1016/j.marchem.2004.06.017
Walch H., von der Kammer F., Hofmann T. (2022). Freshwater suspended particulate matter–key components and processes in floc formation and dynamics. Water Res. 220, 118655. doi: 10.1016/j.watres.2022.118655
Watanabe M., Nayar S. K. (1997). Telecentric optics for focus analysis. IEEE Trans. Pattern Anal. Mach. Intell. 19, 1360–1365. doi: 10.1109/34.643894
Wilkinson M. H. F. (1994). Shading correction and calibration in bacterial fluorescence measurement by image processing system. Comput. Methods Programs Biomed. 44, 61–67. doi: 10.1016/0169-2607(94)90086-8
Wurl O., Miller L., Vagle S. (2011). Production and fate of transparent exopolymer particles in the ocean. J. Geophys. Res. Oceans 116, 2011JC007342. doi: 10.1029/2011JC007342
Keywords: particles, marine snow, transparent exopolymer particles, aquatic optics, imaging, gels, optics, aggregates
Citation: Bochdansky AB, Huang H and Conte MH (2022) The aquatic particle number quandary. Front. Mar. Sci. 9:994515. doi: 10.3389/fmars.2022.994515
Received: 14 July 2022; Accepted: 03 October 2022;
Published: 07 November 2022.
Edited by:
Toshi Nagata, The University of Tokyo, JapanReviewed by:
Emmanuel Boss, University of Maine, United StatesJun Sun, China University of Geosciences Wuhan, China
Yosuke Yamada, Japan Agency for Marine-Earth Science and Technology (JAMSTEC), Japan
Copyright © 2022 Bochdansky, Huang and Conte. This is an open-access article distributed under the terms of the Creative Commons Attribution License (CC BY). The use, distribution or reproduction in other forums is permitted, provided the original author(s) and the copyright owner(s) are credited and that the original publication in this journal is cited, in accordance with accepted academic practice. No use, distribution or reproduction is permitted which does not comply with these terms.
*Correspondence: Alexander B. Bochdansky, abochdan@odu.edu