- 1Zhejiang Marine Fisheries Research Institute, Scientific Observing and Experimental Station of Fishery Resources for Key Fishing Grounds, Ministry of Agriculture, Key Laboratory of Sustainable Utilization of Technology Research for Fishery Resources of Zhejiang Province, Zhoushan, China
- 2Marine and Fishery Institute, Zhejiang Ocean University, Zhoushan, China
Coastal waters provide an important spawning and nursery ground for offshore marine organisms. To understand the suitable habitat and distribution of Harpadon nehereus, a key nearshore species, this study assessed the survey data of fishery resources and environment in Zhejiang’s nearshore fishery in Spring of 2017 to 2020. We used the generalized additive model (GAM) and random forests model (RF) to analyze the environmental factors affecting the selection of spawning habitats by H. nehereus and analyzed the suitable habitat characteristics of H. nehereus in nearshore fisheries. Our results indicate that H. nehereus is widely distributed in Zhejiang’s nearshore and that its density is higher in waters near islands and reefs. Among the relative importance scores of predictors calculated based on RF, seawater salinity is an important environmental factor affecting the distribution of H. nehereus in April (surface seawater salinity was 38.67% and bottom seawater salinity was 34.5%), followed by depth (34.19%), whereas the change in water temperature had no obvious effect on H. nehereus distribution. The suitable habitat characteristics of H. nehereus mainly include high salinity near a water depth of 40 m and a water area with sea bottom dissolved oxygen levels < 6 mg/L. The prediction results of the model indicate that the suitable habitat of H. nehereus is mainly located in the region near Zhoushan Islands in the north of the fishing grounds. Overall, these results may serve as a basis for determining the protection strategies of key nearshore species and enhancing fishery management units.
1 Introduction
Marine fishery resources provide a strong guarantee for human survival (Botsford et al., 1997), and their optimal utilization and a healthy ecosystem are prerequisites for sustainable fisheries production (Rice, 2005). The choice of the location is important for marine fish to increase opportunities for feeding and escaping predation and to fulfill the necessary requirements of fish for survival from the egg to the adult stage and subsequently dispersing across different habitats (Ciannelli et al., 2015). Individuals usually choose their aquatic habitats based on factors such as salinity and temperature within their physiological tolerance range as habitat (Gordó-Vilaseca et al., 2021).
Coastal waters provide important habitats for a wide range of fish species. These waters sustain terrestrial and marine-origin nutrient supplies and spatiotemporal changes in the nearshore physicochemical environment (e.g., water depth, temperature and salinity) due to flood and ebb tides (Kasai et al., 2010; Wang et al., 2019; Kume et al., 2021). Furthermore, they create different types of fluctuating habitats, which provide a highly productive, ever-changing ecosystem for fish communities (Seitz et al., 2014; Potter et al., 2015). Environmental factors (e. g., water temperature, salinity, and their fluctuations) are thought to be some of the main contributors to large-scale patterns of coastal fish communities (Florin et al., 2009; Pasquaud et al., 2015; Kume et al., 2021). For example, adult Perca fluviatilis prefer shallow areas with suitable temperature development as their spawning habitat during spring (Snickars et al., 2010). The depth and habitat use affect seasonal up and downslope movements of Gadus morhua in the Western Baltic Sea (Funk et al., 2020). Coastal waters are, therefore, recognized to play an important ecosystemic role at the land–sea interface in order to generate and sustain high levels of production in the fisheries. However, these areas are also highly susceptible to human activities, including fishing and urban construction, which can lead to changes in coastal fish habitats (Ciannelli et al., 2015).
Harpadon nehereus is a marine species dependent on coastal habitats, especially in early developmental stages. Compared to other economic species (e.g., Larimichthys crocea, Trichiurus haumela), H. nehereus had long been considered as a low-valued species for a long time and was not the main target species for fisheries. However, due to its muti-stage of spawning, high survival rates and fast growth, H. nehereus comprised the majority of fishery composition in Zhejiang coastal areas (Chen and Zhang, 2015; Wang et al., 2021, Jiang et al., 2019). The suitability of the habitat, as well as the availability of feed preferred by fish may play important roles in the thriving. Recent surveys of fisheries community resources indicate that H. nehereus has become a dominant species in some areas off the coast of China, such as the Yueqing Bay and the Yangtze River Estuary (Sun et al., 2015; Yuan et al., 2017). Among the fish species found in the southern Zhejiang coastal waters (Wang et al., 2022), H. nehereus is the second most important species after Trichiurus lepturus, which plays a decisive role in the stability of fish community structure and the transmission of interspecies information in coastal waters. Changes in the patterns of activities of different species can change the food web structure at the regional scale, affect the relationship between feeding and competition, and alter ecosystem functions (Long and Seitz, 2008). The habitat preferences of fish determine their food sources in waters of different depths. H. nehereus is an omnivorous fish with a wide range of feeding habits, and large individuals demonstrate cannibalistic behavior (Allen et al., 2014); its increasing dominance has widespread implications for existing food webs and community structures. Wang et al. (2021) pointed out that with climate change, H. nehereus is moving northward along the coast of China, which may compress the ecological niches of other coastal species and affect marine ecosystems.
The relationship between resources and the marine environment is complex. Generally, the movement, activity, and connectivity patterns of fish are influenced by dynamic environmental conditions (Perry et al., 2018). Ambient salinity determines a fish’s capability to adapt to environmental variations. For instance, when fish such as Trachinotus marginatus and Sparus aurata are exposed to low salinity gradients, fish oxygen consumption increases and fish mortality increases, and as salinity increases, fish become more tolerant of toxic compounds, such as ammonia (Costa et al., 2008; Kir and Sunar, 2018; Kir et al., 2019). Annual mean benthic water temperature is reportedly the most important variable in determining the distribution of H. nehereus (Wang et al., 2021). Moreover, water temperature has a strong influence on the distribution patterns of coastal fish such as Gadus morhua (Staveley et al., 2019) and Homarus americanus (Jury and Watson, 2013), that seek suitable habitats by migrating seasonally in relation to water temperature (Freitas et al., 2021).
Habitat protection of coastal fishery resources is a hotspot of relevant research. At present, research on the characteristics of fish habitats mainly focuses on particular species (e.g., Conger myriaster, Albula vulpes) with high economic and ecological value (Li et al., 2017; Brownscombe et al., 2019). According to pertinent research in recent years, H. nehereus has gradually become an important dominant species in the coastal waters of China, occupying a dominant position in some sea areas, but few studies have assessed its habitat needs. In the present study, we chose H. nehereus as an example to evaluate the modelling approach for the key species affecting coastal ecosystems. The objectives of this study were to: (1) assess the driving environmental variables for determining the distribution of H. nehereus; and (2) The distribution pattern of suitable habitats of H. nehereus in Zhejiang’s coastal waters. This study could help strengthen environmental impact assessments and support decision-making in ocean planning.
2 Material and methods
2.1 Sampling method and stations
The study area is located in the mid-west of the East China Sea (Figure 1) and is affected by different water masses and scouring waters; the salinity gradient changes greatly, and food is plentiful. The coastal waters of Zhejiang are located in the western East China Sea, which is an important site for fish spawning, feeding, breeding, and migration (Wang and Fan, 2004; Su and Yuan, 2005). The area has many islands, and the coastline is tortuous. Water circulation and water mass interactions make this area a suitable hydrological environment rich in basic nutrients that provide a variety of habitats for various fish species (Wang and Fan, 2004; Zhang et al., 2020).
Bottom trawl surveys were carried out in the spring (April) of 2017–2020 off the coast of Zhejiang (27°00′N−31°00′N, 120°30′E−121°30′E). The isobath of the surveyed sea area is parallel to the shoreline. Therefore, we set up a total of 123 stations according to the distance from the shore and adopted the fixed-point survey method for sampling in different years. The type of fishing boat used was a single-bottom trawler, with a main engine power of 220 kw. The surveys were conducted at 3 knots for about 30 minutes on average. The mesh size of the capsule net was 25 mm, and the perimeter of the net port was 50 m. A catch was landed at each survey station, and the weight of the catch was converted into the catch per unit net with a drag speed of 2.0 kn and a trawl time of 1.0 h as the resource abundance index.
2.2 Environmental variables
In addition to obtaining the resource data of the dominant fish at various stations, we also used multifunctional water quality meters to synchronously obtain environmental data. Organisms have a tolerance range for environmental variables and environmental conditions suitable for their habitats (Shelford, 1911). The spawning period of H. nehereus is mainly from May to September. In April, H. nehereus migrates from offshore to coastal waters for spawning. H. nehereus is benthopelagic, living in brackish water and seawater, preferring to inhabit shallow depths (0–150 m). H. nehereus lays floating eggs, which are greatly affected by the sea surface environment. Considering the biological characteristics, and the availability and reliability of data, the chosen environmental variables mainly included depth, surface, and bottom environment variables (sea temperature, salinity, chlorophyll, and dissolved oxygen). The environmental variables (temperature, salinity, chlorophyll, and dissolved oxygen) were sampled simultaneously. The details are included in Table 1.
2.3 Data analysis
This study mainly used models to analyze trends in environmental variables that affect species distribution.
2.3.1 Generalized additive models
Generalized additive models (GAM) (Hastie and Tibshirani, 1990) are universal and convenient statistical algorithms in traditional regression methods and are often used for standardizing catch data. GAMs are an extension of generalized linear models (GLMs), and both are based on the statistical distribution of response variables for data analysis (Li et al., 2017). GLMs assume that the predictive function between the response variable and the explanatory variable is a linear relationship, whereas GAMs consider a non-linear relationship (Wang et al., 2019). Data analysis is based on the additive predictive function, which ensures that the model has some non-parametric characteristics. The formula of GAM is as follows:
where g is the differentiable and monotonic link function, y is the response variable, α is the function intercept, fi is a smooth function, xiis the predictor variable, and ϵ is a random error term.
Owing to the unique geographical location of the marine environment, some environmental variables may have strong correlations. To reduce the influence of predictor variables with multicollinearity on the model fitting results, we first performed a correlation analysis on the predictor variables and conducted preliminary screening of the predictor variables added to the model. Thus, we only considered removing the predictor variables with strong collinearity in the process of constructing the GAM.
2.3.2 Random forests
Random forest (RF) (Breiman, 2001) is an improved method for bagged trees, and has the advantage of combining many randomly constructed decision trees to reduce the influence of outliers and redundant data on the prediction results. RF can quantify complex nonlinear relationships without obvious overfitting. According to the RF algorithm summarized by Liaw et al. (Liaw and Wiener, 2002), the operation of the model is mainly controlled by two model parameters, ntree and mtry; ntree determines the number of decision trees and mtry determines the number of random features. The ntree value is generally determined by the relationship between the result and error, and mtry defaults to 1/3 of the number of predictors. In this study, we determined that ntree was 1000 according to the stable trend, and that mtry was 4.
We can also sort the relative importance of predictors using RF and the relative importance score calculation formula is as follows:
where Virepresents the explanatory rate of the predictor variable Xi to the model, SXi represents the set of nodes split by Xi in the random forest of ntree trees, and G (Xi, v) represents the Gini information gain of Xi at the split node v, which is used to select the explanatory variable of the maximum information gain.
2.4 Model evaluation
To ensure that the model has high accuracy and to add fewer predictor variables, we usually use the Akaike information criterion (AIC) as an index to filter the model (Akaike, 1998). However, owing to algorithm differences, this may not be applicable to tree-based regression (Li et al., 2015). This study combined AIC and mean absolute deviation (MAD) to screen the predictor variables added to the GAM, whereas the predictor screening of the RF model was completely based on MAD. Smaller values of the AIC and MAD are considered to indicate a higher accuracy of the model. In the fitting process, a backward selection regression method was used to substitute the predictor variables into the model, and the optimal model was selected based on AIC and MAD, as described previously (Li et al., 2015).
The AIC can be computed as follows:
where k is the number of parameters, L is the likelihood function.
MAD can be computed as follows:
where N is the number of samples, is the estimated value (the predicted value) from the model for the th observation, and is the actual value.
K-fold cross-validation is a common method for comparing different types of models and their prediction performance (Arlot and Celisse, 2010; Li et al., 2015). Therefore, we used it to evaluate the prediction performance of the RF and GAM. We used 80% of the dataset as the training set and the remaining 20% as the test set. Further, to facilitate the subset method for replacing a single model, we performed independent repeated sampling on the subset. The entire process was repeated 100 times. To quantitatively evaluate the prediction performance of the model, performance was mainly based on the root mean square error (RMSE) of the predicted value of the model (Hyndman and Koehler, 2006), the degree of linear regression between the predicted value, and the actual value in the test set.
To understand the distribution of suitable habitats for H. nehereus in different years using the two models, we divided the research water area into a grid size of 0.1° × 0.1° and obtained the coordinates of the center point of each grid. According to the environmental survey data of each site from 2017 to 2020, the conventional Kriging interpolation method was used to calculate the environmental data of the center point for each grid. The relative resource density of each center point was obtained based on the GAM and RF model, and the habitat suitability index (HSI) was calculated as follows:
here HSIi,t is the habitat suitability index of the ith grid point in year t, is the predicted value of the ith grid point in year t predicted by the model. and are the highest and lowest values for the predicted value of year t, respectively.
3 Results
3.1 Distribution of harpadon nehereus
There were more high-value biomass stations in 2018 than in the previous years (Figure 2). By comparing the number of high-density stations in the surveyed sea area, H. nehereus biomass in the sea area near 30°N was found to be significantly higher than that at 28°N (t test p<0.05), and the center of gravity of H. nehereus resources in different years was basically the same.
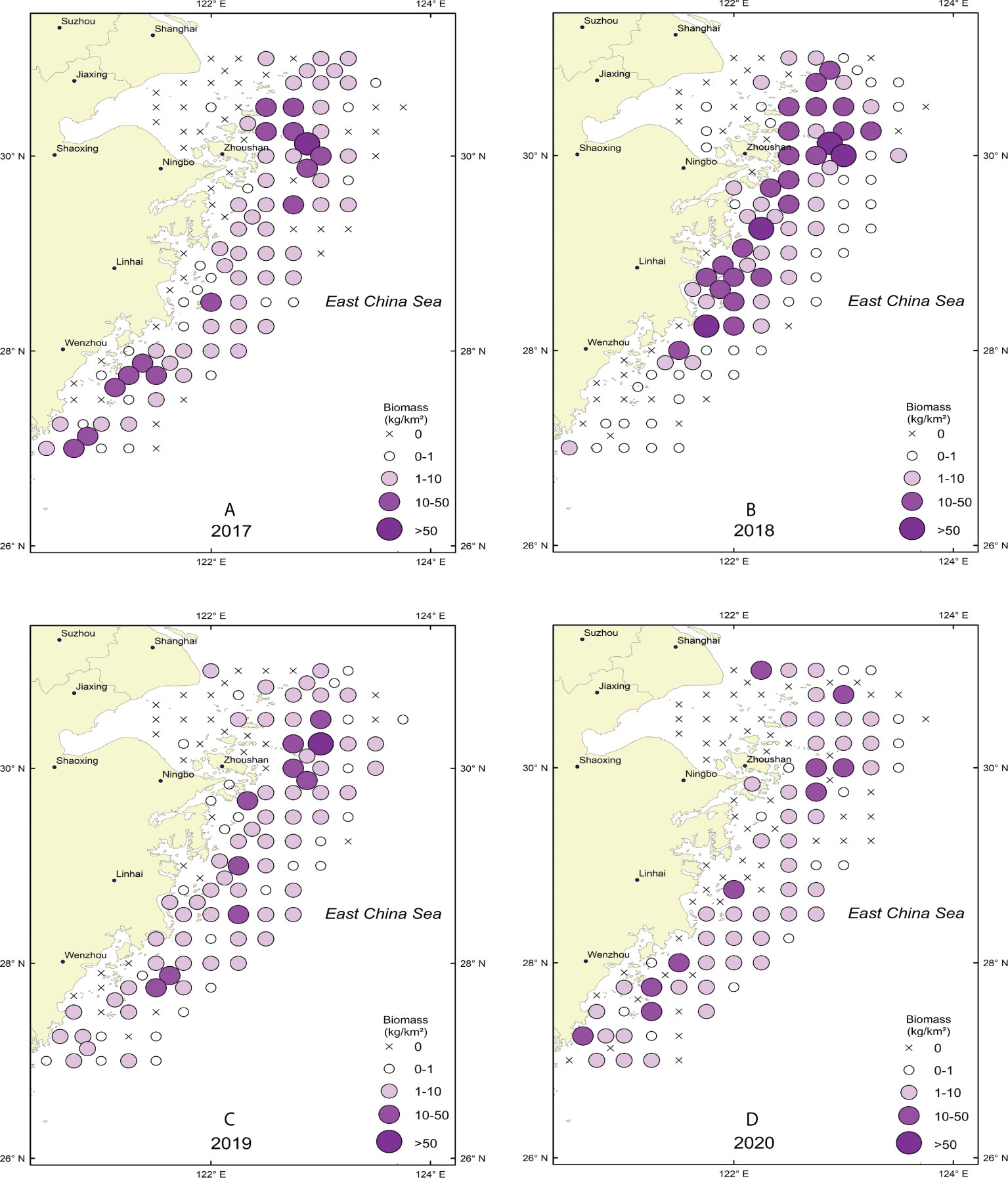
Figure 2 Spatial distribution of H. nehereus from 2017 to 2020. (A: 2017. B: 2018. C: 2019. D:2020).
3.2 Analysis and screening of the influence of factors
Habitat is an important factor that affects species distribution. By constructing a Spearman correlation matrix, we preliminarily understood the relationship between H. nehereus population resources and different non-biological variables and analyzed the correlation strength between two different predictor variables (Figure 3). Pearson’s R was used as the correlation coefficient between various predictor variables. The larger the color block, the greater was the correlation. The color of the block represents the size of the coefficient. Mantel’s R and Mantel’s P respectively represent the correlation coefficient and the significance of the degree of correlation between the resource density of H. nehereus and predictor variables. The results show that the amount of H. nehereus resources is significantly correlated with depth, sea surface temperature (SST), and Sea bottom temperature (BST) (P < 0.01), and that the correlation coefficient with SST was the highest among them. Further, the resources were also significantly correlated with the Longitude, Latitude, and sea surface salinity (SSS) (P < 0.05).
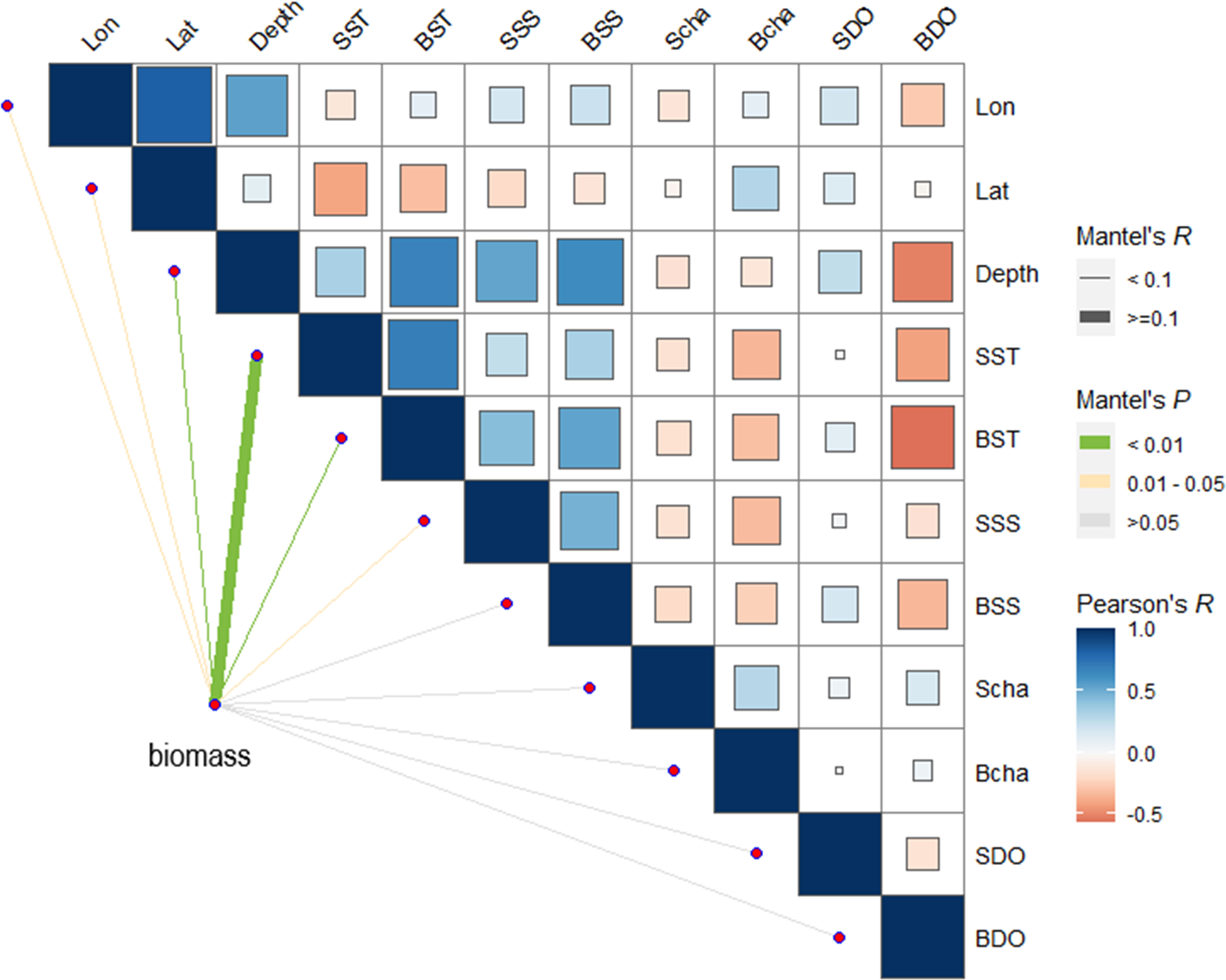
Figure 3 Correlation matrix for the relative biomass of H. nehereus and predictor variables. Pearson’s R was used as the correlation coefficient between various predictor variables. The larger the color block, the greater was the correlation. The color of the block represents the size of the coefficient. Mantel’s R and Mantel’s P respectively represent the correlation coefficient and the significance of the degree of correlation between the resource density of H. nehereus and predictor variables.
Among the predictor variables, there was a strong correlation between Depth, Longitude, BST, and sea bottom salinity (BSS); the correlation coefficient between longitude and latitude was the largest, and that between SST and BST was higher than that of other variables. To reduce the influence of collinearity on the fitting effect of the GAM, we performed a multicollinearity test on the predictor variables based on the variance inflation factor (VIF); as Longitude and other variables (Longitude VIF value > 4) have the dramback of multicollinearity, Longitude was not considered a predictor variable when constructing the GAM. Simultaneously, one of the predictor variables with strong correlation was selected as an explanatory variable and added to the GAM.
3.3 Model fitting results
In the model fitting process, we logarithmized the response variables and biomass data of H. nehereus at each station to reduce the influence of some maximum values on the model fitting effect. Table 2 shows the best model results for the GAM and RF after stepwise screening. The explained deviation between the two models is small, and several predictor variables have a significant effect on the response variable. We calculated the relative importance scores of predictors based on the RF model (Figure 4); SSS, BSS, and depth are the three most important predictor variables for the distribution of H. nehereus (relative importance scores > 30%).
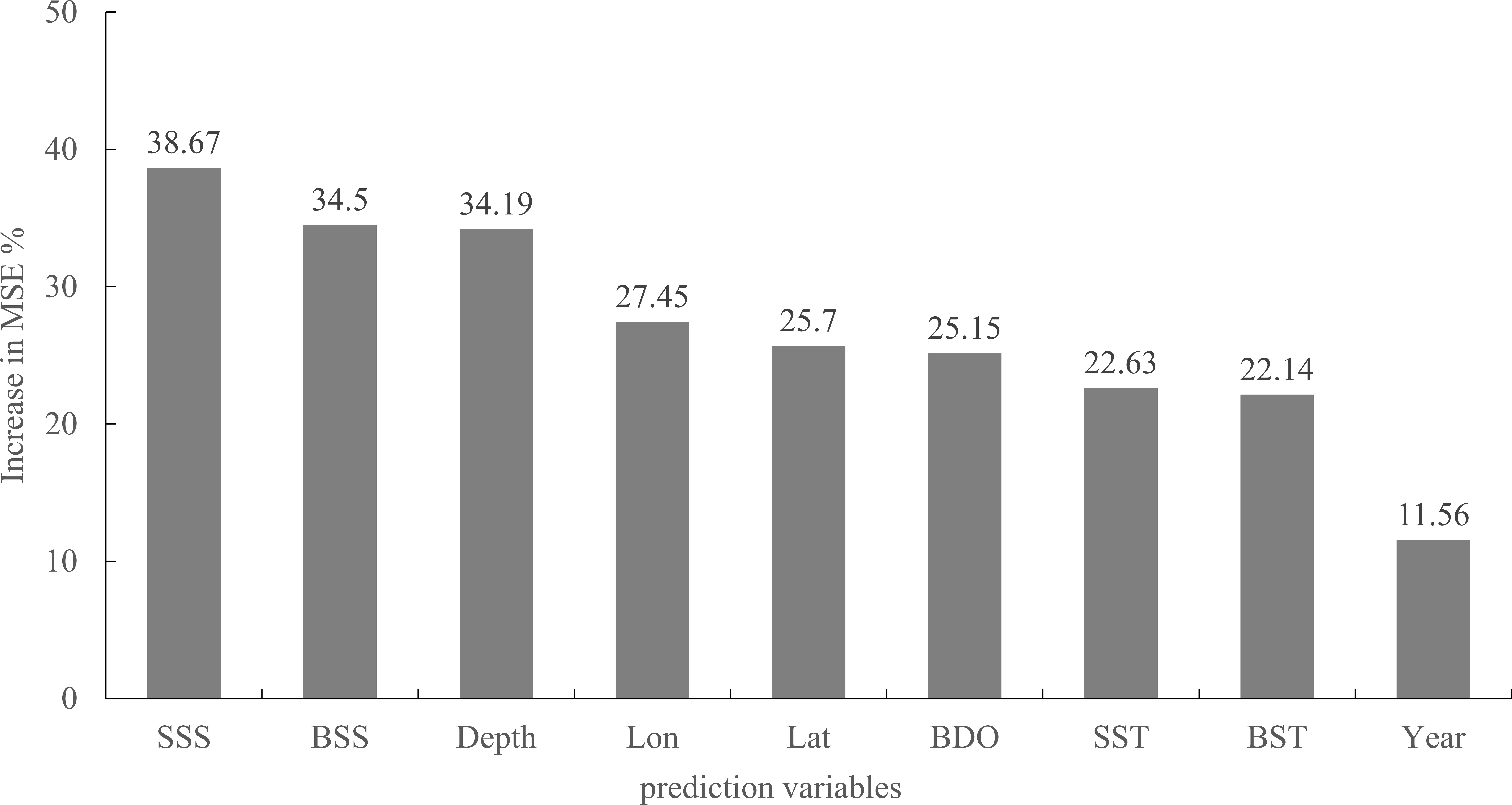
Figure 4 Relative importance of predictor variables for determining the distribution of H. nehereus. The relative importance score of the predictor variables was calculated based on RF; the higher the score, the more important the predictor variables are in explaining the distribution of H. nehereus.
3.4 The influence of different factors on the resource density of H. nehereus
In the best GAM and RF models (Figures 5, 6), the influence of different predictors on H. nehereus resource fluctuations indicated that, the year had little effect on the changes in the resources of H. nehereus (Figures 5A, 6A). In the fitting results of the two models, different predictors showed a nonlinear relationship with the density of H. nehereus. Under the same predictor, the trends of changes in the resources of H. nehereus fitted with the two models were similar (for example, in the RF model fitting results, the trend of relative biomass with SSS was similar to the lower limit of the 95% confidence interval in the GAM).
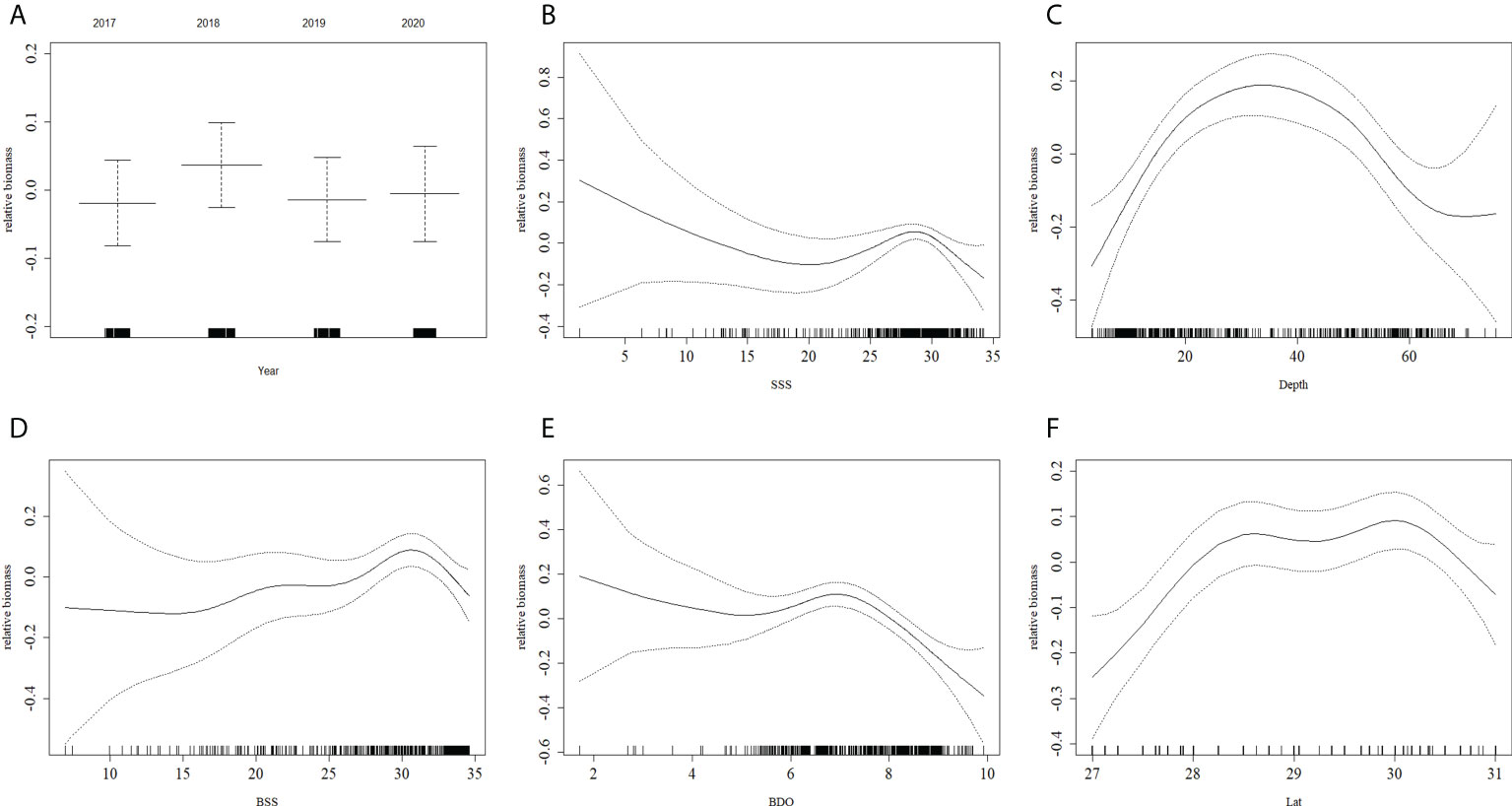
Figure 5 Effects of factors from GAM on the relative biomass of H. nehereus. (A: Year. B: SSS. C: Depth. D: BSS. E: BDO. F: Lat).
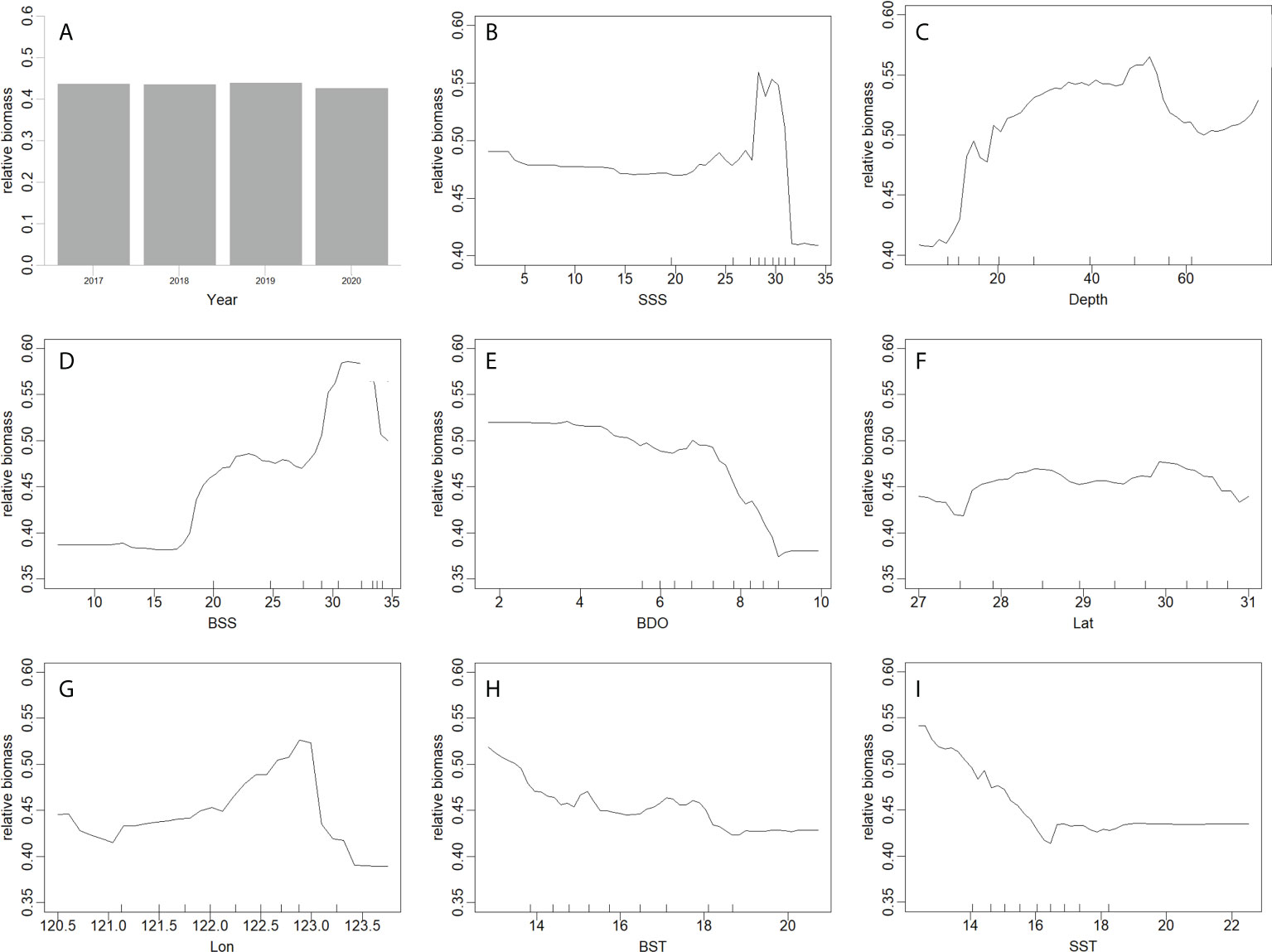
Figure 6 Effects of factors from RF on the relative biomass of H. nehereus. (A: Year. B: SSS. C: Depth. D:BSS. E: BDO. F: Lat. G: Lon. H: BST. I: SST).
Fluctuations in H. nehereus resources mainly occurred in high-salinity waters. When SSS > 25, there was an obvious trend of first increasing and then decreasing resource density, and the maximum resources appeared at SSS 30 (Figures 5B, 6B). At BSS > 15, the amount of H. nehereus resources increased with an increase in BSS (Figures 5D, 6D). The suitable SSS range was 25–32 and the suitable BSS range was 27–34. The resource density of H. nehereus presented a trend of first increasing and then decreasing with a change in water depth (Figures 5C, 6C). When the BDO concentration was less than 7 mg/L, the resource density of H. nehereus did not decrease significantly (Figure 5E, 6E). The areas with a high density of H. nehereus resources were mainly distributed between 28°N–30°N (Figures 5F, 6F) and 122°E–123°E (Figure 6G). An impact of water temperature on H. nehereus resources was mainly observed at SST < 16°C (Figure 6I), and the changing trend of resources with BST was not obvious (Figure 6H).
3.5 Model prediction performance evaluation and display
Each group of data in the table represents the mean and range of the statistical results (Figure 7). In the cross-validation results, the average RMSE of the RF model (0.10) was less than that of GAM (0.23). In the linear regression results of the true and predicted values, the statistical results of the slope and the intercept of the two models had relatively small differences. The average coefficient of determination for RF cross-validation was 0.73, which was higher than that of GAM at 0.30 (Table 3). Overall, RF was better than GAM in terms of the model prediction performance.
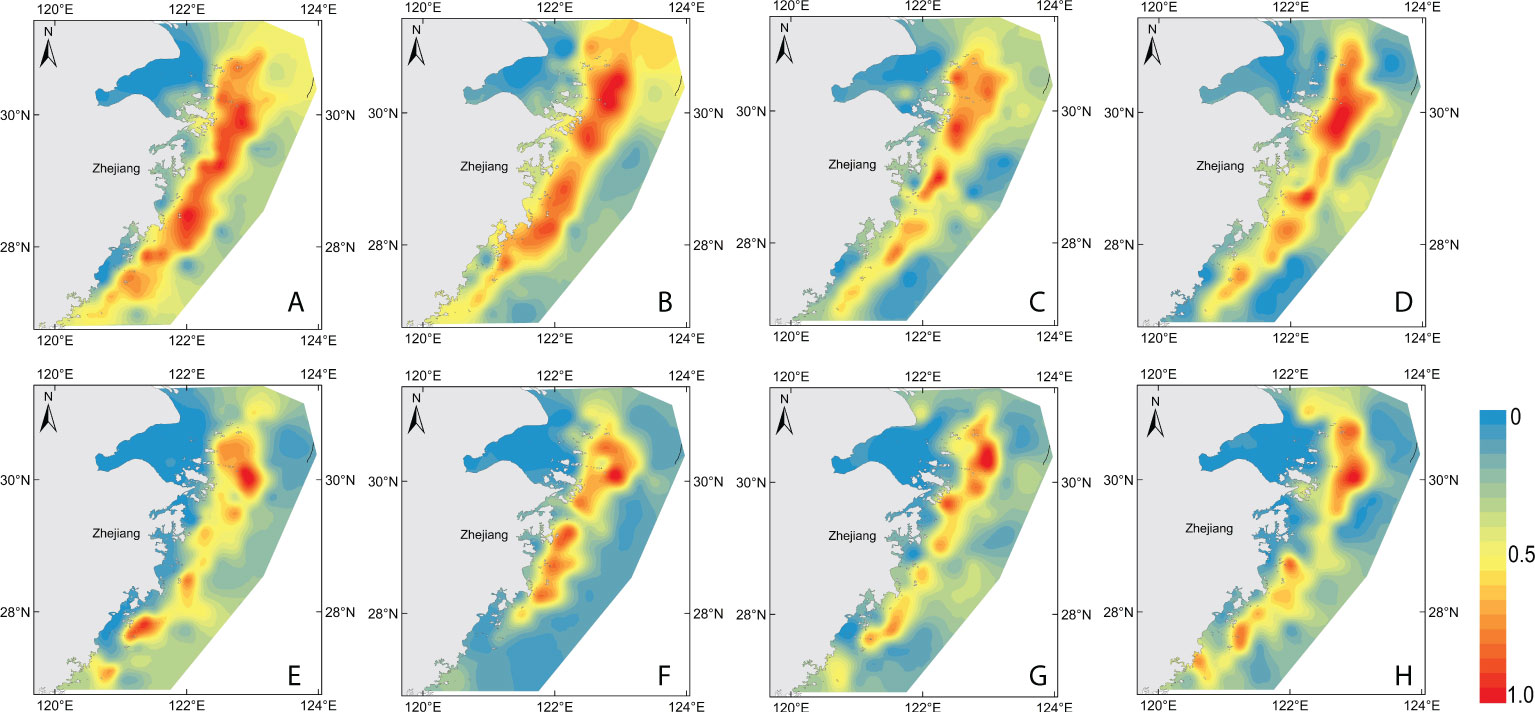
Figure 7 Maps of 2017–2020 model-based habitats suggested to be suitable for H. nehereus. (A–D): 2017–2020 GAM model-based habitats, (E–H): 2017–2020 RF model-based habitats.
The habitat suitability index (HSI) was used to predict the suitable habitat of H. nehereus based on the two models (Figure 7). A HSI with a numerical value from 0 to 1 represents a range from an unsuitable area (HSI value is 0) to the most suitable area (HSI value is 1). The highly suitable area for H. nehereus based on GAM fitting was wider than that obtained with the RF model. The central and northern waters of the study area were found to be highly suitable areas for H. nehereus. The eastern sea area of Zhoushan Islands was found to be the most suitable area for the distribution of H. nehereus. Further, the distribution areas of highly suitable habitats of H. nehereus also varied in different years.
4 Discussion
4.1 The influence of environmental variables
The oceanographic environment is a direct factor that stimulates fish to spawn and migrate. Consequently, factors such as water temperature and salinity affect the distribution of fish spawning grounds (Ciannelli et al., 2015). H. nehereus spawn over a prolonged time period ranging from summer to autumn, under the influence of coastal currents and the Kuroshio Current. The adult H. nehereus begin to swim from wintering waters (e.g., the East China Sea, the Yellow Sea) to nearshore waters in spring (Luo et al., 2012). Under the right conditions, the spawning brood stock of H. nehereus migrates to the offshore areas and estuaries to spawn (Taqwa et al., 2020; Wang et al., 2021). Salinity and depth were the most powerful predictors for H. nehereus distribution in spring. These patterns may be attributed to the seasonal migratory habits of H. nehereus, including spawning and overwintering migration. Furthermore, predation, starvation, or abnormal diffusion processes can limit the change in the habitat that fish experience from year to year (Jørgensen et al., 2008).
In this study, sea salinity had a significant influence on the distribution of H. nehereus in spring, which could be attributed to its selection of suitable habitats (Wang et al., 2019). The species was heavily concentrated in estuaries and offshore areas, indicating that it has a wide salinity tolerance range. Furthermore, H. nehereus has also been reported to be highly abundant in other coastal hypersaline habitats due to its ecological tolerance. (Nooralabettu, 2008; Liu et al., 2021; Wang et al., 2021) However, for coastal fish, the osmoregulatory mechanism in different life stages may differ. (Allen et al., 2014; Thomas et al., 2021) For example, Pomatoschistus microps is a widespread small-sized fish occurring in temperate estuaries, which has a relatively wide tolerance for salinity variation. However, juveniles display better physiological performances at low levels of salinity. (Souza et al., 2018) During the course of this study, 70% of H. nehereus samples captured in spring were adults with a body length greater than 160 mm. Compared with juveniles, adults have stronger swimming capability and can choose more suitable habitats for survival.
The salinity of the East China Sea was generally > 30‰ (Luo et al., 2012), and living in waters with similar salinity can ensure that H. nehereus will not experience a strong acute stress response (Ciannelli et al., 2015). Therefore, it would be able to meet osmoregulation and ionoregulation needs with lower energy expenditure. Additionally, the higher biomass of H. nehereus in high salinity waters may be related to the distribution of prey organisms. Benthosema pterotum and Apogon lineantus are the main prey organisms for H. nehereus in spring, and the optimum seawater salinities for the two species are 29.7-34‰ and 31.4-32‰, respectively (Liu et al., 2021; Tang et al., 2021). H. nehereus swim to the area where the prey organisms are located to forage, despite the increased energetic costs of living at higher salinities. Consequently, the distribution of the prey organisms may be one of the influencing factors of sea salinity on the distribution of H. nehereus.
Shallow waters have long been assumed to afford small fish protection from piscivorous fish; large piscivores enjoy a metabolic benefit by occupying deeper waters. In the Tsitsikamma National Park marine protected area, South Africa, the shallow reef (water depth of 11–25 m) assemblages were dominated by juveniles and low trophic level species, while deep reef (water depth of 45–75 m) assemblages were characterized by large, sexually mature, and predatory fish. (Heyns et al., 2016). In relevant research in this field, bottom water temperature and depth are considered important environmental variables affecting the habitat and distribution of H. nehereus (Wang et al., 2021).Changes in the water depth gradient can directly affect the bottom water environment, thereby altering the distribution of fish populations (McLean et al., 2016; Whitfield, 2017). Some bottom environmental variables (sea temperature, salinity) in this study showed a strong positive correlation with water depth. With the increase in depth, the resources of H. nehereus first increased and then decreased, which could explain the migration behavior of H. nehereus as a result of the bottom water environment change in this season. For example, cod (Gadus morhua) is a cold-water species, and their optimum water temperature for growth ranges between 9 and 15°C; sea surface temperatures that are too high or too low can prompt them to swim to deeper areas (Freitas et al., 2021). In addition, Funk et al. (2020) found that the inhabitation of different water depths by cods was associated with spawning behavior in the Baltic Sea. In the case of H. nehereus, April coincides with the pre-spawning time. At this time, H. nehereus uses deeper, more saline waters, to potentially maximize food availability, temperature preferences, and egg development. Besides, the seabed sediments at depths of 40–70 m consist of silty mud and fine argillaceous sand in the survey area, and adult H. nehereus prefers deep waters and sand-mud bottoms to resist high temperatures and light (Li et al., 2017).
Differences in dissolved oxygen (DO) in the water environment affect the classification and size of the zooplankton community, affecting the foraging and growth of fish and altering the spatial ecology and population dynamics of the water area. DePasquale et al. (2015) found that early life estuarine fish were more sensitive to low DO concentrations than to water acidification. Low DO has been reported to reduce the scope for activity, such as swimming and feeding, of Atlantic cod (Gadus morhua), resulting in reduced foraging success and increased predation risk (Chabot and Claireaux, 2008). However, potential lethality and avoidance has been observed in fish inhabiting areas with high DO concentrations. A fish kill in Lake Waubesa was thought to have been caused by supersaturation of DO, produced by a dense bloom of Chlamydomonas sp. (Nordlie, 2006). Low DO areas are believed to be unsuitable for the survival of benthic organisms (Gong et al., 2017), and hypoxia-tolerant organisms may use hypoxic waters as refuges to avoid predation (Roman et al., 2019). In this study, the amount of H. nehereus resources and bottom DO showed a non-linear negative correlation; the resources decreased with an increase in water temperature and bottom DO concentration in spring. When the bottom DO concentration was lower than 7 mg/l, the extent of resource fluctuation was small, and it showed a significantly reduced trend when the bottom DO was greater than 7 mg/L. This may be related to the distribution of regional population structure caused by changes in DO concentration. Breitburg et al. (2001) proposed that DO concentration has an important effect on the distribution of estuarine biological resources. Oxygen depletion can induce vertical or horizontal displacement of fish and this movement may render the individual more vulnerable to predation (Long and Seitz, 2008). Ocean deoxygenation is an important cause of increase in H. nehereus resources, as reported in a new study (Kang et al., 2021). High water content in the muscle and other tissues of H. nehereus can greatly reduce the demand for oxygen, giving H. nehereus an advantage when competing with other fish in the shallow sea. However, at higher DO concentrations, H. nehereus faces an increased risk of predation; therefore, the resources show a downward trend.
Water temperature was considered the most important factor affecting the geographical distribution pattern of offshore organisms (Snickars et al., 2010; Bučas et al., 2013; McLean et al., 2016). Wang et al. (2021) analyzed the impact of future climate change on the distribution of H. nehereus in the coastal waters of China and indicated that the annual average benthic water temperature was the most important environmental variable affecting H. nehereus distribution. In this study, water temperature had a negative impact on the H. nehereus distribution in spring. The thermal tolerance of fish that live in a highly variable environment for long durations is generally considered to be higher than that of fish living in a thermally stable environment. For individuals, there are significant differences in the width of thermal tolerance windows at different developmental stages (Pörtner and Peck, 2010). For example, in adult Solea, due to the need to provide oxygen for egg or sperm masses, the oxygen supply capacity gradually decreases relative to the demand, making larger individuals more heat sensitive and less tolerant to extreme temperatures (Rijnsdorp et al., 2009). The results of a study on glass eels Anguilla anguilla showed that lower water temperatures can significantly reduce eels’ migration behavior from higher salinity seawater to lower salinity freshwater to reduce migration-related energy expenditure, thereby increasing the success rate of spawning reproduction (Edeline et al., 2006).
The complex water environment provides diverse habitats for marine life. The study area has a tropical monsoon climate, and its unique ocean conditions are suitable for various marine organisms to thrive. The coastal waters of Zhejiang Province exhibit typical spatial heterogeneity (Jiang et al., 2019); there are spatial differences in the resource and environment utilization and in the similarity and adaptability among species (Zhang et al., 2020), causing suitable habitats to often be distributed in patches. The suitable habitat of H. nehereus is narrow, the central and northern parts of the study area are greatly affected by Taiwan’s warm, saline current, which maintains the salinity and water temperature in this sea area at a relatively high level. Simultaneously, the northern area is an estuary of the Yangtze River (Robinson et al., 2011; Sun et al., 2015; Gong et al., 2017), where the fresh water and abundant food provides a better prey environment for H. nehereus. Consequently, the density of H. nehereus resources was significantly higher in this area than in the southern seas. Owing to the strong adaptability of H. nehereus (Kang et al., 2021; Wang et al., 2021), its population has an advantage in the fierce competition for suitable habitats. Simultaneously, short-distance migration habits allow H. nehereus to be widely distributed in the surveyed seas (Lin, 2009). In recent years, the increasing fishing intensity has severely reduced the resources of fish, such as Trichiuridae, occupying higher trophic levels, thus reducing the number of small fish. (Whitfield, 2017; Liu et al., 2021). Furthermore, the peak breeding season of H. nehereus in the coastal waters of Zhejiang is mainly during the summer closing season mandated by the management policy in the East China Sea. Consequently, the recruitment cohort is less affected by anthropogenic disturbances; thus, the recruitment of H. nehereus can be effectively recovered during the breeding season.
4.2 Model performance evaluation
Compared with other traditional regression models such as GLM, the advantage of GAM is that it adopts an additive method to comprehensively analyze the influence of different predictor variables on response variables and can optimize the expression of nonlinear relationships between variables (Park et al., 2020). In fact, many predictive variables used to analyze marine environmental variables may have strong collinearity issues (such as water depth and other variables used in this study). To reduce the deviation of fitting results caused by collinearity, a traditional regression model is usually used to screen the predictor variables, which may cause important predictors to be ignored (Wood and Augustin, 2002). Unlike traditional regression methods, machine learning methods, such as RF, can reduce the influence of discrete values on regression results without considering the collinearity of predictors. Relying on the advantages of the model, the correlation and interaction between variables can be effectively resolved. Based on the results of this study, we conclude that GAM can provide a good fitting effect based on fewer predictors (Yu et al., 2013). Machine learning methods, such as RF, can use the advantages of model algorithms to solve the problem of strong collinearity between predictor variables, which can further improve the predictive performance of the model, and simultaneously reflect the influence of more predictive variables on response variables (Reiss et al., 2011; Rinde et al., 2014). However, one of the main problems of machine learning is that it cannot provide clear statistical principles.
Various methods, including machine learning, have been widely used in environmental assessment, species distribution prediction, and other fields. In recent years, these have been gradually used in marine fish ecology-related research (Bučas et al., 2013; Smoliński and Radtke, 2017). We used two models to analyze the distribution of H. nehereus spawning stock in the study area, to fully understand the environmental factors that affect the fluctuations in their resources (Li et al., 2015). In this study, the gap between GAM, which is based on traditional regression methods, and RF, which is based on machine learning methods, was smaller in the model interpretation rate. However, RF was performed better than GAM in the model prediction performance and provided details of the habitat distribution upon suitable habitat prediction. The suitable habitat area for H. nehereus predicted by the GAM was wider than that predicted by RF. This difference may be attributed to the different descriptions of the basic and actual niches of the species in different models (Liu et al., 2020).
Species’ distributions are affected by processes operating in both environmental and geographical space (Barry and Elith, 2006). The final prediction performance of the two models is not particularly high, which may be related to the small amount of data used in the experiment, key environmental variables may be undescribed. We suggested that when using different models to analyze suitable habitat characteristics of species to reduce the impact of data source constraints, the model should be matched with ecological knowledge, so that the information obtained through the model will be more abundant, and even raise new questions about ecology by elucidating subtle relationships in the model results.
5 Conclusion
Our results indicated that seawater salinity was an important environmental factor affecting the distribution of H. nehereus, followed by depth, whereas a change in water temperature had no obvious effect on H. nehereus distribution. In spring, H. nehereus mainly inhabited deep water areas with high salinity, which are characterized by lower dissolved oxygen and water temperature. The model-based prediction results indicated that the suitable habitat of H. nehereus mainly encompasses the region near Zhoushan Islands to the north of the fishing grounds, owing to the abundant nutrients and complex habitats brought and sustained by the Yangtze River. These results add to our understanding of the environmental factors affecting H. nehereus migration and spawning in the sea areas of interest and provide reference and theoretical basis for analyzing the habitat characteristics of other key nearshore species.
Data availability statement
The raw data supporting the conclusions of this article will be made available by the authors, without undue reservation.
Author contributions
RJ and HS contributed to methodology, writing–original draft, writing–review and editing. XL contributed to project administration, conceptualization, and methodology. YZ contributed to supervision, project administration, conceptualization, methodology, resources, and writing–review and editing. FC contributed to data curation and investigation. KX contributed to project administration. PL contributed to conceptualization. HZ contributed to data curation. All authors contributed to the article and approved the submitted version.
Funding
This study was supported financially by funds from the National Key R&D Program of China (2020YFD0900804, 2019YFD0901204, 2018YFD0900904) and the Key R&D Program of Zhejiang Province (2019C02056).
Acknowledgments
The authors express their gratitude to the staff of the Zhejiang Marine Fisheries Research Institute, Scientific Observing and Experimental Station of Fishery Resources for Key Fishing Grounds, Ministry of Agriculture, and Key Laboratory of Sustainable Utilization of Technology Research for Fishery Resources of Zhejiang Province for help with conducting the experiments.
Conflict of interest
The authors declare that the research was conducted in the absence of any commercial or financial relationships that could be construed as a potential conflict of interest.
Publisher’s note
All claims expressed in this article are solely those of the authors and do not necessarily represent those of their affiliated organizations, or those of the publisher, the editors and the reviewers. Any product that may be evaluated in this article, or claim that may be made by its manufacturer, is not guaranteed or endorsed by the publisher.
References
Akaike H. (1998). Information Theory and an Extension of the Maximum Likelihood Principle. Selected Papers of Hirotugu Akaike, 199–213. doi: 10.1007/978-1-4612-1694-0_15
Allen P. J., Mitchell Z. A., DeVries R. J., Aboagye D. L., Ciaramella M. A., Ramee S. W., et al. (2014). Salinity effects on Atlantic sturgeon (Acipenser oxyrinchus mitchil) growth and osmoregulation. J. Appl. Ichthyol. 30 (6), 1229–1236. doi: 10.1111/jai.12542
Arlot S., Celisse A. (2010). A survey of cross-validation procedures for model selection. Stat. Surv. 4 (0), 40–79. doi: 10.1214/09-ss054
Barry S., Elith J. (2006). Error and uncertainty in habitat models. J. Appl. Ecol. 43, 413–423. doi: 10.1111/j.1365-2664.2006.01136.x
Botsford L. W., Castilla J. C., Peterson C. H. (1997). The management of fisheries and marine ecosystems. Science 277, 509–515. doi: 10.1126/science.277.5325.509
Breitburg D. L., Pihl L., Kolesar S. E. (2001). Effects of low dissolved oxygen on the behavior, ecology and harvest of fishes: A comparison of the Chesapeake bay and Baltic-kattegat systems. Coast. Hypoxia: Consequences living Resour. Ecosyst. 58, 241–267. doi: 10.1029/CE058p0241
Brownscombe J. W., Griffin L. P., Gagne T. O., Haak C. R., Cooke S. J., Finn J. T., et al. (2019). Environmental drivers of habitat use by a marine fish on a heterogeneous and dynamic reef flat. Mar. Biol. 166 (2), 1–13. doi: 10.1007/s00227-018-3464-2
Bučas M., Bergström U., Downie A. L., Sundblad G., Gullström M., Von Numers M., et al. (2013). Empirical modelling of benthic species distribution, abundance, and diversity in the Baltic Sea: evaluating the scope for predictive mapping using different modelling approaches. ICES J. Mar. Sci. 70 (6), 1233–1243. doi: 10.1093/icesjms/fst036
Chabot D., Claireaux G. (2008). Environmental hypoxia as a metabolic constraint on fish: the case of Atlantic cod, gadus morhua. Mar. pollut. Bull. 57 (6-12), 287–294. doi: 10.1016/j.marpolbul.2008.04.001
Chen D. G., Zhang M. Z. (2015). Marine fishes of China (Qingdao, Shandong: China Ocean University Press), 416–418.
Ciannelli L., Bailey K., Olsen E. M. (2015). Evolutionary and ecological constraints of fish spawning habitats. ICES J. Mar. Sci. 72, 285–296. doi: 10.1093/icesjms/fsu145
Costa L. D. F., Miranda-Filho K. C., Severo M. P., Sampaio L. A. (2008). Tolerance of juvenile pompano trachinotus marginatus to acute ammonia and nitrite exposure at different salinity levels. Aquaculture 285 (1-4), 270–272. doi: 10.1016/j.aquaculture.2008.08.017
DePasquale E., Baumann H., Gobler C. J. (2015). Vulnerability of early life stage Northwest Atlantic forage fish to ocean acidification and low oxygen. Mar. Ecol. Prog. Ser. 523, 145–156. doi: 10.3354/meps11142
Edeline E., Lambert P., Rigaud C., Elie P. (2006). Effects of body condition and water temperature on Anguilla anguilla glass eel migratory behavior. J. Exp. Mar. Biol. Ecol. 331 (2), 217–225. doi: 10.1016/j.jembe.2005.10.011
Florin A. B., Sundblad G., Bergström U. (2009). Characterisation of juvenile flatfish habitats in the Baltic Sea. Estuar. Coast. Shelf Sci. 82 (2), 294–300. doi: 10.1016/j.ecss.2009.01.012
Freitas C., Villegas-Ríos D., Moland E., Olsen E. M. (2021). Sea Temperature effects on depth use and habitat selection in a marine fish community. J. Anim. Ecol. 90, 1787–1800. doi: 10.1111/1365-2656.13497
Funk S., Krumme U., Temming A., Möllmann C. (2020). Gillnet fishers’ knowledge reveals seasonality in depth and habitat use of cod (Gadus morhua) in the Western Baltic Sea. ICES J. Mar. Sci. 77 (5), 1816–1829. doi: 10.1093/icesjms/fsaa071
Gong S. B., Gao A. G., Ni G. T., Zhu X. X., Zhang Y. P., Hou Y. T. (2017). Progress in research of hypoxia in estuaries and coastal areas in China. Water Resour. Prot. 33 (4), 62–69. doi: 10.3880/jissn.1004-6933.2017.04.010
Gordó-Vilaseca C., Pennino M. G., Albo-Puigserver M., Wolff M., Coll M. (2021). Modelling the spatial distribution of sardina pilchardus and engraulis encrasicolus spawning habitat in the NW Mediterranean Sea. Mar. Environ. Res. 169, 105381. doi: 10.1016/j.marenvres.2021.105381
Hastie T., Tibshirani R. J. (1990). “Generalized additive models,” in In monographs on statistics and applied probability (London: Chapman and Hall), 335.
Heyns E. R., Bernard A. T. F., Richoux N. B., Parker D., Langlois T. J., Harvey E. S., et al. (2016). Depth and habitat determine assemblage structure of south africa’s warm-temperate reef fish. Mar. Biol. 163 (7), 1–17. doi: 10.1007/s00227-016-2933-8
Hyndman R. J., Koehler A. B. (2006). Another look at measures of forecast accuracy. Int. J. Forecast. 22 (4), 679–688. doi: 10.1016/j.ijforecast.2006.03.001
Jiang R., Zhang L., Xu K., Li P., Xiao Y., Fan Z, et al (2019). Characteristics and diversity of nekton functional groups in the coastal waters of south-central Zhejiang Province. Biodiv Sci. 27 (12), 1330–1338. doi: 10.17520/biods.2019281
Jørgensen C., Dunlop E. S., Opdal A. F., Fiksen Ø. (2008). The evolution of spawning migrations: state dependence and fishing-induced changes. Ecology 89, 3436–3448. doi: 10.1890/07-1469.1
Jury S. H., Watson W. H. (2013). Seasonal and sexual differences in the thermal preferences and movements of American lobsters. Can. J. Fish. Aquat. 70, 1650–1657. doi: 10.1139/cjfas-2013-0061
Kang B., Bakun A., Lin L., Pauly D. (2021). Increase of a hypoxia-tolerant fish, Harpadon nehereus (Synodontidae), as a result of ocean deoxygenation off southwestern China. Environ. Biol. Fish., 1–5. doi: 10.1007/s10641-021-01130-7
Kasai A., Kurikawa Y., Ueno M., Robert D., Yamashita Y. (2010). Salt-wedge intrusion of seawater and its implication for phytoplankton dynamics in the yura estuary, Japan. Estuar. Coast. Shelf Sci. 86 (3), 408–414. doi: 10.1016/j.ecss.2009.06.001
Kir M., Sunar M. (2018). Acute toxicity of ammonia and nitrite to Sea bream, sparus aurata (Linnaeu), in relation to salinity. J. World Aquacult. Soc 49, 516–522. doi: 10.1111/jwas.12448
Kir M., Sunar M., Gök M. (2019). Acute ammonia toxicity and the interactive effects of ammonia and salinity on the standard metabolism of European sea bass (Dicentrarchus labrax) aquaculture. Aquaculture 511, 734273. doi: 10.1016/j.aquaculture.2019.734273
Kume M., Lavergne E., Ahn H., Terashima Y., Kadowaki K., Ye F., et al. (2021). Factors structuring estuarine and coastal fish communities across Japan using environmental DNA metabarcoding. Ecol. Indic. 121, 107216.
Lin L. S. (2009). Spatial distribution and environmental characteristics of harpodon nehereus in the East China Sea region. J. Shanghai Univ. 18, 66–71.
Liu Z., Yang L., Yuan X., Jin Y., Yan L., Cheng J. (2020). Overwintering distribution and its environmental determinants of small yellow croaker based on ensemble habitat suitability modeling. Chinese Journal of Applied Ecology 31 (6), 2076–2086. doi: 10.13287/j.1001-9332.202006.034
Liu Z. H., Han D. Y., Gao C. X., Ye S. (2021). Feeding habits of Bombay ducks (Harpadon nehereus) in the offshore waters of southern zhejiang, based on predator CPUE weighting. J. Fish. Sci. China. 28 (04), 482–492. doi: 10.12264/JFSC2020-0249
Li Z., Ye Z., Wan R., Zhang C. (2015). Model selection between traditional and popular methods for standardizing catch rates of target species: a case study of Japanese Spanish mackerel in the gillnet fishery. Fish. Res. 161, 312–319. doi: 10.1016/j.fishres.2014.08.021
Li M., Zhang C., Xu B., Xue Y., Ren Y. (2017). Evaluating the approaches of habitat suitability modelling for whitespotted conger (Conger myriaster) fish. Res 195, 230–237. doi: 10.1016/j.fishres.2017.07.024
Long W. C., Seitz R. D. (2008). Trophic interactions under stress: hypoxia enhances foraging in an estuarine food web. Mar. Ecol. Prog. Ser. 362, 59–68. doi: 10.3354/meps07395
Luo H. Z., Zhang H. D., Li P. F., Zhou Y. D. (2012). Analysis of the current situation of fishery biology of Harpodon nehereus in the East China Sea. J. Zhejiang Ocean Univ. Nat. Sci. 31 (3), 202–205,233.
McLean D. L., Langlois T. J., Newman S. J., Holmes T. H., Birt M. J., Bornt K. R., et al. (2016). Distribution, abundance, diversity and habitat associations of fishes across a bioregion experiencing rapid coastal development. Estuar. Coast. Shelf Sci. 178, 36–47. doi: 10.1016/j.ecss.2016.05.026
Nooralabettu K. P. (2008). Effect of sun drying and artificial drying of fresh, salted Bombay duck (Harpodon neherius) on the physical characteristics of the product. J. Aquat. Food Prod. Technol. 17 (2), 99–116. doi: 10.1080/10498850801936994
Nordlie F. G. (2006). Physicochemical environments and tolerances of cyprinodontoid fishes found in estuaries and salt marshes of eastern north America. Rev. Fish. Biol. Fish. 16, 51–106. doi: 10.1007/s11160-006-9003-0
Park S. Y., Sur C., Lee J. H., Kim J. S. (2020). Ecological drought monitoring through fish habitat-based flow assessment in the gam river basin of Korea. Ecol. Indic. 109, 105830. doi: 10.1016/j.ecolind.2019.105830
Pasquaud S., Vasconcelos R. P., França S., Henriques S., Costa M. J., Cabral H. (2015). Worldwide patterns of fish biodiversity in estuaries: Effect of global vs. local factors. Estuar. Coast. Shelf Sci. 154, 122–128. doi: 10.1016/j.ecss.2014.12.050
Perry D., Staveley T. A. B., Gullström M. (2018). Habitat connectivity of fish in temperate shallow-water seascapes. Front. Mar. Sci. 4. doi: 10.3389/fmars.2017.00440
Pörtner H. O., Peck M. A. (2010). Climate change effects on fishes and fisheries: towards a cause-and-effect understanding. J. Fish. Biol. 77, 1745–1779. doi: 10.1111/j.1095-8649.2010.02783.x
Potter I. C., Tweedley J. R., Elliott M., Whitfield A. K. (2015). The ways in which fish use estuaries: a refinement and expansion of the guild approach. Fish Fish. 16, 230–239. doi: 10.1111/faf.12050
Reiss H., Cunze S., König K., Neumann H., Kröncke I. (2011). Species distribution modelling of marine benthos: A north Sea case study. Mar. Ecol. Prog. Ser. 442, 71–86. doi: 10.3354/meps09391
Rice J. C. (2005). Understanding fish habitat ecology to achieve conservation. J. Fish Biol. 67, 1–22. doi: 10.1111/j.0022-1112.2005.00933.x
Rijnsdorp A., Peck M. A., Engelhard G. H., Möllmann C., Pinnegar J. K. (2009). Resolving the effect of climate change on fish populations. ICES J. Mar. Sci. 66, 1570–1583. doi: 10.1093/ICESJMS/FSP056
Rinde E., Christie H., Fagerli C. W., Bekkby T., Gundersen H., Norderhaug K. M., et al. (2014). The influence of physical factors on kelp and sea urchin distribution in previously and still grazed areas in the NE Atlantic. PLoS One 9 (6), e100222. doi: 10.1371/journal.pone.0100222
Robinson L. M., Elith J., Hobday A. J., Pearson R. G., Kendall B. E., Possingham H. P., et al. (2011). Pushing the limits in marine species distribution modelling: Lessons from the land present challenges and opportunities. Glob. Ecol. Biogeogr. 20, 789–802. doi: 10.1111/j.1466-8238.2010.00636.x
Roman M. R., Brandt S. B., Houde E. D., Pierson J. J. (2019). Interactive effects of hypoxia and temperature on coastal pelagic zooplankton and fish. Front. Mar. Sci. 6. doi: 10.3389/fmars.2019.00139
Seitz R. D., Wennhage H., Bergström U., Lipcius R. N., Ysebaert T. (2014). Ecological value of coastal habitats for commercially and ecologically important species. ICES J. Mar. Sci. 71 (3), 648–665. doi: 10.1093/icesjms/fst152
Shelford V. E. (1911). Physiological animal geography. J. Morphol. 22, 551–618. doi: 10.1002/jmor.1050220303
Smoliński S., Radtke K. (2017). Spatial prediction of demersal fish diversity in the Baltic Sea: comparison of machine learning and regression-based techniques. ICES J. Mar. Sci. 74 (1), 102–111. doi: 10.1093/icesjms/fsw136
Snickars M., Sundblad G., Sandström A., Ljunggren L., Bergström U., Johansson G., et al. (2010). Habitat selectivity of substrate-spawning fish: modelling requirements for the Eurasian perch perca fluviatilis. Mar. Ecol. Prog. Ser. 398, 235–243. doi: 10.3354/meps08313
Souza A. T., Ilarri M. I., Timóteo S., Marques J. C., Martins I. (2018). Assessing the effects of temperature and salinity oscillations on a key mesopredator fish from European coastal systems. Sci. Total Environ. 640, 1332–1345. doi: 10.1016/j.scitotenv.2018.05.348
Staveley T., Jacoby D., Perry D., Meijs F., Lagenfelt I., Gullström M. (2019). Sea Surface temperature dictates movement and habitat connectivity of Atlantic cod in a coastal fjord system. Ecol. Evol. 9, 9076–9086. doi: 10.1002/ece3.5453
Sun P. F., Dai F. Q., Chen Y. L., Shan X., Jin X. (2015). Seasonal variations in structure of fishery resource in the Yangtze river estuary and its adjacent waters. Prog. Fish. Sci. 36, 8–16. doi: 10.11758/yykxjz.20150602
Tang L., Zhang Y. L., Xu B. D., Zhang C. L., Ren Y. P., Xue Y. (2021). Habitat suitability analysis of Apogon lineatus during autumn in haizhou bay. Periodical Ocean Univ. China. 51 (S1), 154–160. doi: 10.16441/j.cnki.hdxb.20200268
Taqwa A., Burhanuddin A. I., Niartiningsih A., Nessa M. N. (2020). Nomei fish (Harpadon nehereus, ham. 1822) reproduction biology in tarakan waters. Conf. Ser.: Earth Environ. Sci. 473 (1), 12012. doi: 10.1088/1755-1315/473/1/012012
Thomas D., Rekha M. U., Angel J. R. J., Sreekanth G. B., Thiagarajan G., Subburaj R., et al. (2021). Effects of salinity amendments on the embryonic and larval development of a tropical brackishwater ornamental silver moony fish. Monodactylus argenteus (Linnaeus 1758) Aquaculture 544, 737073. doi: 10.1016/j.aquaculture.2021.737073
Wang G., Fan Z. (2004). Marine mammals and their distributions in coastal waters of zhejiang. Chin. J. @ Zool. 39 (1), , 60–, 63. doi: 10.13859/j.cjz.2004.01.012
Wang L., Wang X., Li C., Jia X. (2019). Seasonal distribution and habitat preferences of crimson seabream parargyrops edita: Implications for a marine protected area in beibu gulf, northern south China Sea. Mar. Coast. Fish. 11 (3), 258–270. doi: 10.1002/mcf2.10075
Wang S. C., Yang R., Gao C. X., Han D. Y., Ye S. (2022). Keystone species of fish community in the offshore waters of southern zhejiang: Insight from ecological network. J. Fish. Sci. China. 29 (01), 118–129. doi: 10.12264/JFSC2021-0140
Wang L., Zhang Z., Lin L., Peng X., Lin L., Kang B. (2021). Redistribution of the lizardfish Harpadon nehereus in coastal waters of China due to climate change. Hydrobiologia 848 (20), 4919–4932. doi: 10.1007/s10750-021-04682-y
Whitfield A. K. (2017). The role of seagrass meadows, mangrove forests, salt marshes and reed beds as nursery areas and food sources for fishes in estuaries. Rev. Fish Biol. Fish. 27, 75–110. doi: 10.1007/s11160-016-9454-x
Wood S. N., Augustin N. H. (2002). GAMs with integrated model selection using penalized regression splines and applications to environmental modelling. Eco. Model. 157 (2-3), 157–177. doi: 10.1016/s0304-3800(02)00193-x
Yuan H. R., Chen P. M., Qin C. X., Li X. G., Zhou Y. B., Feng X., et al. (2017). Seasonal variation of fish community structure in zhelin bay, the south China Sea. South China Fish. Sci. 13, 26–35. doi: 10.3969/j.issn.2095−0780.2017.02.004
Yu H., Jiao Y., Carstensen L. W. (2013). Performance comparison between spatial interpolation and GLM/GAM in estimating relative abundance indices through a simulation study. Fish. Res. 147, 186–195. doi: 10.1016/j.fishres.2013.06.002
Keywords: Harpadon nehereus, species distribution model, suitable habitat characteristics, environmental factors, coastal waters
Citation: Jiang R, Sun H, Li X, Zhou Y, Chen F, Xu K, Li P and Zhang H (2022) Habitat suitability evaluation of Harpadon nehereus in nearshore of Zhejiang province, China. Front. Mar. Sci. 9:961735. doi: 10.3389/fmars.2022.961735
Received: 05 June 2022; Accepted: 08 August 2022;
Published: 25 August 2022.
Edited by:
Jun Xu, Institute of Hydrobiology, (CAS), ChinaReviewed by:
Xiujuan Shan, Yellow Sea Fisheries Research Institute, (CAFS), ChinaXuefeng Wang, Guangdong Ocean University, China
Copyright © 2022 Jiang, Sun, Li, Zhou, Chen, Xu, Li and Zhang. This is an open-access article distributed under the terms of the Creative Commons Attribution License (CC BY). The use, distribution or reproduction in other forums is permitted, provided the original author(s) and the copyright owner(s) are credited and that the original publication in this journal is cited, in accordance with accepted academic practice. No use, distribution or reproduction is permitted which does not comply with these terms.
*Correspondence: Yongdong Zhou, enlkNTExQDEyNi5jb20=
†These authors have contributed equally to this work and share first authorship