- 1Centre national de la Recherche Scientifique (CNRS) and Sorbonne Université, Laboratoire d’Océanographie de Villefranche (LOV), Villefranche-sur-Mer, France
- 2Centre national de la Recherche Scientifique (CNRS) and Sorbonne Université, Observatoire des Sciences de l’Univers (OSU) Ecce Terra, Paris, France
- 3Observatoire des Sciences de l’Univers (OSU) and Sorbonne Université, Institut de la Mer de Villefranche (IMEV), Villefranche-sur-Mer, France
In-situ fluorescence is a widely used method to estimate the chlorophyll-a (Chla) concentration, a proxy of the phytoplankton biomass. With the emergence of autonomous platforms such as BioGeoChemical-Argo (BGC-Argo) profiling floats, its use has expanded to global scale observations. However, the relationship between in-situ fluorescence and Chla may vary significantly, leading to major discrepancies between oceanic regions. This study aims to investigate the main sources of the natural variability in the in-situ fluorescence signal in the global open ocean, specifically the influence of the phytoplankton community composition. In this view, we analyzed a combination of three datasets comprising concomitant measurements of in-situ fluorescence, pigment concentrations and phytoplankton absorption spectra. Two datasets cover several contrasted bioregions of the global ocean whereas the third one consists of a regional time series in the northwestern Mediterranean Sea, which allows to examine the effect of phytoplankton community composition on the fluorescence signal on the global, seasonal and vertical scales. We studied the variability of the two major drivers of the natural variability of the fluorescence process, i.e. the light absorption and the fluorescence quantum yield of phytoplankton, in regards of the variability of the pigment composition of the communities. The community composition correlates substantially with the Chla-to-fluorescence ratio, with high fluorescence values associated with phytoplankton communities dominated by large cells. This trend may be explained by the combined effects of the community composition on the phytoplankton absorption coefficient and the fluorescence quantum yield, and is consistently observed globally, seasonally and vertically. Non-photosynthetic pigments also appear to play a critical role in oligotrophic surface waters, leading to a reduction of the quantum yield of fluorescence. The results indicate that the phytoplankton community composition plays a key role in the relationship between the in-situ fluorescence signal and Chla concentration. Therefore, we suggest that taking into account the composition of phytoplankton communities in the retrieval of the Chla concentration from current in-situ fluorometers, those mounted on BGC-Argo floats in particular, would lead to a better estimation of the phytoplankton biomass on a wide range of spatial and temporal scales.
1 Introduction
The ongoing global changes result in significant changes of oceanic biogeochemical cycles (Bindoff et al., 2019). The drivers of these modifications have to be better characterized and understood, a prerequisite for the predictive modeling of the evolution of the ocean biogeochemistry and its feedback to climate. As phytoplankton play a critical role in oceanic biogeochemical cycles (Falkowski, 1994), mapping their biomass at appropriate spatial and temporal scales appears essential. However, the wide spatial distribution and variable cell sizes (Chisholm, 1992; Roy et al., 2013) of phytoplankton communities make a global assessment of their biomass challenging. Several techniques are used that cover distinct ranges of the size spectrum of phytoplankton organisms, from flow cytometry analysis (Dubelaar and Jonker, 2000) to microscopic observation (Booth, 1993; Karlson et al., 2010), or High Performance Liquid Chromatography (HPLC) pigment analysis (Jeffrey et al., 1997). Nevertheless, these techniques rely on discrete sampling, which restricts the spatial and temporal coverages of the observations. Satellite-based methods permit to estimate phytoplankton biomass on a global scale but are limited to the ocean surface layer (i.e. the light first penetration depth) and hence do not encompass the vertical distribution of phytoplankton within the whole water column.
Initially introduced to oceanography in 1966 (Lorenzen, 1966), in-situ fluorescence relies on the fluorescence property of the chlorophyll-a (Chla) molecule. A fluorometer emits blue light exciting the chlorophyll-a (Chla) molecule and detects the red shifted light that is subsequently reemitted in the environment; the increase in the fluorescence signal is then interpreted as an increase in Chla concentration. Thanks to the possibility to equip with fluorometers autonomous in-situ platforms (profiling floats, gliders), in-situ fluorescence has become the most widely used technique to assess the phytoplankton biomass at large space and time scales with a fine vertical resolution. In particular, the BioGeoChemical-Argo (BGC-Argo) program aims to monitor and understand key biogeochemical processes on the global scale by developing a network of profiling Argo floats equipped with a suite of biogeochemical sensors (Roemmich et al., 2019; Claustre et al., 2020). BGC-Argo floats are all equipped with an ECO-series Chla fluorometer (SeaBird Electronics), providing time series of vertical profiles of Chla concentration for a broad range of oceanic regimes, resulting in a powerful dataset for investigating the distribution and dynamics of the phytoplankton biomass. Nevertheless, a recent study by Roesler et al. (2017) pointed to the large regional (natural) variability in the relationship between fluorescence and reference Chla concentration measurements, in addition to a global overestimation bias of the Chla concentration by fluorometers of the ECO-series. These results stress out the necessity to better understand the sources of variability in the fluorescence-to-Chla concentration relationship, with an aim to improve the calibration of fluorescence into Chla equivalent for all types of in-situ platforms, BGC-Argo profiling floats in particular.
The variability in the ratio between the fluorescence signal and the Chla concentration has been shown to depend not only on the physiological state of phytoplankton cells (Behrenfeld et al., 2009; Escoffier et al., 2015; Schuback et al., 2021; Gorbunov and Falkowski, 2022), but also on the accessory pigment composition that varies with the phytoplankton taxonomic composition (Johnsen and Sakshaug, 2007; Proctor and Roesler, 2010; Roy et al., 2011). Two main factors influence the fluorescence process; the amount of (blue) light absorbed by cells which depends on both the intensity of the light emitted by the sensor and the absorption capacity of the cells (Bricaud et al., 1995; Bricaud et al., 2004; Roy et al., 2013), and the efficiency of the cells in reemitting part of the absorbed blue light as red light, an efficiency known as the fluorescence quantum yield (Alpine and Cloern, 1985; Olaizola and Yamamoto, 1994; Falkowski and Kolber, 1995).
Despite a significant variability in phytoplankton communities and environmental conditions encountered by the BGC-Argo floats and their attached fluorometers, an identical standard calibration equation is used to convert the electric signal associated to red light emission into Chla concentration (Bittig et al., 2019).
While phytoplankton communities and, hence, pigment composition (e.g. Claustre, 1994; Mackey et al., 1996; Jeffrey et al., 1997; Zapata et al., 2004; Ras et al., 2008) vary tremendously at the global scale, the effect of such variations on the estimation of Chla concentration based on in-situ fluorescence measurements is still poorly understood and quantified. This knowledge is however crucial to better assess phytoplankton biomass in the world ocean and, thus, to improve our understanding of biogeochemical cycles.
In this study, we examine the role of phytoplankton community structure in driving the fluorescence signal through its effects on the phytoplankton absorption coefficient and fluorescence quantum yield. To this end, we analyze data of BGC-Argo in-situ chlorophyll fluorescence, HPLC pigment concentrations, and phytoplankton absorption spectra, from various regions of the global open ocean. To investigate further the effect of seasonal succession and vertical distribution of phytoplankton communities on the fluorescence signal, we also consider a time series of in-situ fluorescence, HPLC pigments and phytoplankton absorption spectra acquired in the North Western Mediterranean (Ligurian) Sea. This region is characterized by a strong seasonality and contrasted oceanographic and biogeochemical conditions (Marty et al., 2002; Lavigne et al., 2015; Mayot et al., 2017) which will possibly permit to generalize the regional results to other temperate areas. Ultimately, we discuss possibilities to improve the calibration of the in-situ fluorescence signal to Chla concentrations by taking into account information on the phytoplankton community composition.
2 Data and methods
2.1 Fluorescence principle
The determination of the Chla concentration, [Chla] (mg m-3); using an in-situ fluorometer is based on the fluorescence principle expressed as follows:
where the fluorescence signal, F (mol quanta), depends on the spectral Chla-specific absorption coefficient of phytoplankton at a wavelength λ, a*(λ) (m2 (mg Chla)-1); the quantum yield of fluorescence, Φ (relative unit); and the light energy emitted by the fluorometer at the considered wavelength, E(λ) (mol quanta m-2) (Cosgrove and Borowitzka, 2010). While F is defined as a quantity of energy (i.e. mol quanta), the output of the fluorometer is expressed in digital counts or in relative fluorescence units (RFU).
BGC-Argo floats are equipped with a Seabird Electronics SBE (previously WET Labs) ECO-series fluorometer. This sensor emits exciting energy on a range of wavelength going from 454 to 480 nm with a peak at 470 nm (Schmechtig et al., 2014). We note that this wavelength does not coincide with the in vivo Chla absorption peak in the blue region of the spectrum (Bricaud et al., 2004), but rather excites accessory pigments that transfer the energy to the reaction centers (RC) of the photosynthetic apparatus. The energy reemitted by phytoplankton cells is measured at a nominal wavelength of 690 nm and expressed as counts that correspond to F. This signal is then converted into Chla concentration ([Chla]fluo) following Equation (2):
with “Dark signal” the number of fluorescence relative units in the dark and “Calibration slope” the factory determined coefficient that permits to convert the number of counts into [Chla]fluo (mg m-3 count-1). The Calibration slope is derived from a calibration performed by the manufacturer, based on a series of measurements of [Chla]fluo for a range of concentrations of a monospecific culture of the diatom Contricriba weissfglogii (also known as Thalassiosira weissflogii)in controlled conditions (SBE ECO Chlorophyll Fluorometer Characterization sheet).
The bias in the estimate of [Chla]fluo is quantified in reference to [Chla] determined from HPLC analysis ([Chla]HPLC), assuming that [Chla]HPLC is equal to [Chla] in Equation (1). As in Roesler et al. (2017), the bias introduced in the conversion of F into [Chla]fluo is assessed through the coefficient of a linear regression between concurrent measurements of [Chla]fluo and [Chla]HPLC, and is referred to as the “slope factor”. Combining and rearranging Equations (1) and (2), the slope factor (dimensionless) can be defined as follows:
with two constant values, E and Calibration slope, and two variable quantities, a*(λ) and Φ. The only two parameters that can explain the variability of the slope factor are therefore a*(λ) and Φ.
In order to grasp the variability of these important quantities, we analyzed different datasets that are presented in the next sections. The combination of this different datasets allows to investigate their spatial variability on the global scale as well as their seasonality and depth variability.
2.2 Global scale databases
In order to examine the sources of spatial variability of the fluorescence signal on the global scale, we analyze the “Glo-Argo” database. This database comprises concurrent measurements of [Chla]fluo, [Chla]HPLC, and concentrations of accessory pigments, along with indirect determinations of the a*(470), which is the phytoplankton absorption coefficient at 470 nm, the excitation wavelength of the ECO fluorometers mounted on the BGC-Argo floats. The [Chla]fluo data arise from BGC-Argo float fluorescence measurements, and the HPLC-determined [Chla]HPLC and accessory pigments concentrations from discrete seawater samples collected simultaneously to the BGC-Argo float deployments. The phytoplankton absorption coefficient was generally not measured at float deployment and was thus derived from [Chla]HPLC using the empirical relationship of Bricaud et al. (1995). This relationship is based on a global scale database of contrasted environments and has proven robust for application to large scale dataset and does not show substantial regional variability – see Figure 1 in Bricaud et al. (1995).
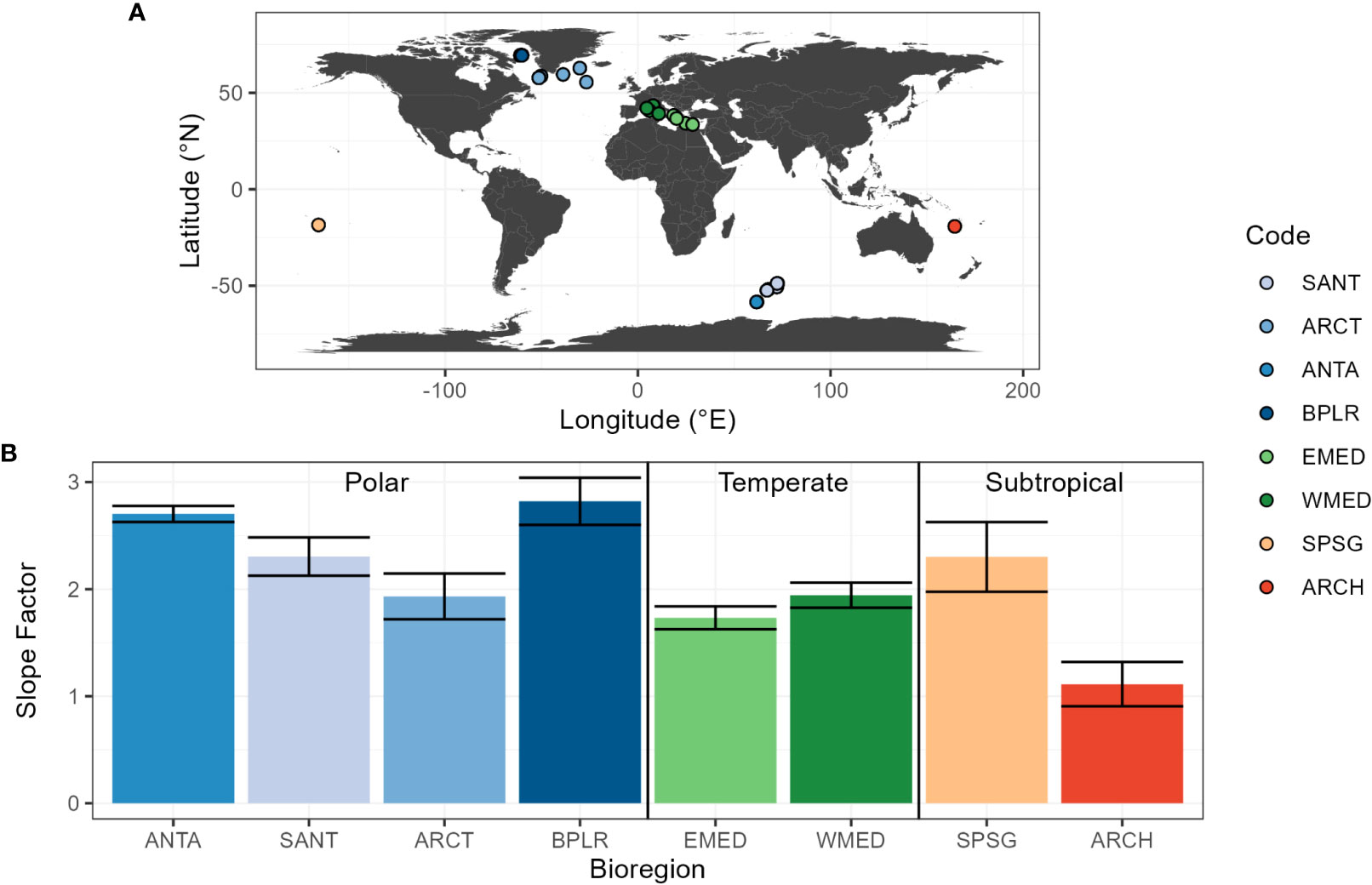
Figure 1 (A) Geographic location of the sampling stations in the Glo-Argo database and (B) mean value of the slope factor, defined as the coefficient of the regression between [Chla]Fluo and [Chla]HPLC, for each bioregion of the Glo-Argo database (ANTA, Antarctic Province; ARCH, Archipelagic Deep Basin Province; ARCT, Atlantic arctic Province; BPLR, Boreal Polar Province; EMED, Eastern Mediterranean Sea; SANT, Subantarctic Province; SPSG, South Pacific Subtropical Gyre Province; WMED, Western Mediterranean Sea). The error bars indicate 95% confidence limits.
Overall, the Glo-Argo dataset includes in-situ measurements associated with 46 BGC-Argo floats deployed in 8 oceanic provinces defined by Longhurst (2006), also called “bioregions”. We will further refer to these bioregions by the following abbreviations (codes): ANTA, Antarctic Province; ARCH, Archipelagic Deep Basin Province; ARCT, Atlantic arctic Province; BPLR, Boreal Polar Province; EMED, Eastern Mediterranean Sea; SANT, Subantarctic Province; SPSG, South Pacific Subtropical Gyre Province; and WMED, Western Mediterranean Sea. They range from polar to subtropical biomes and are thus representative of most of the environmental conditions observed in the global ocean (Table 1). From the entire BGC-Argo fleet, we selected the floats that have been deployed with concomitant high-resolution vertical sampling for HPLC analysis. The float data were extracted from the official Coriolis database (ftp://ftp.ifremer.fr/ifremer/argo). The first measurement profile of each float has been matched with its associated HPLC profile at deployment. A maximum delay of 24h between the float fluorescence profile and the seawater sampling was set as a condition for the final matchups have a time lag of 9 h ± 6 h (mean ± standard deviation).
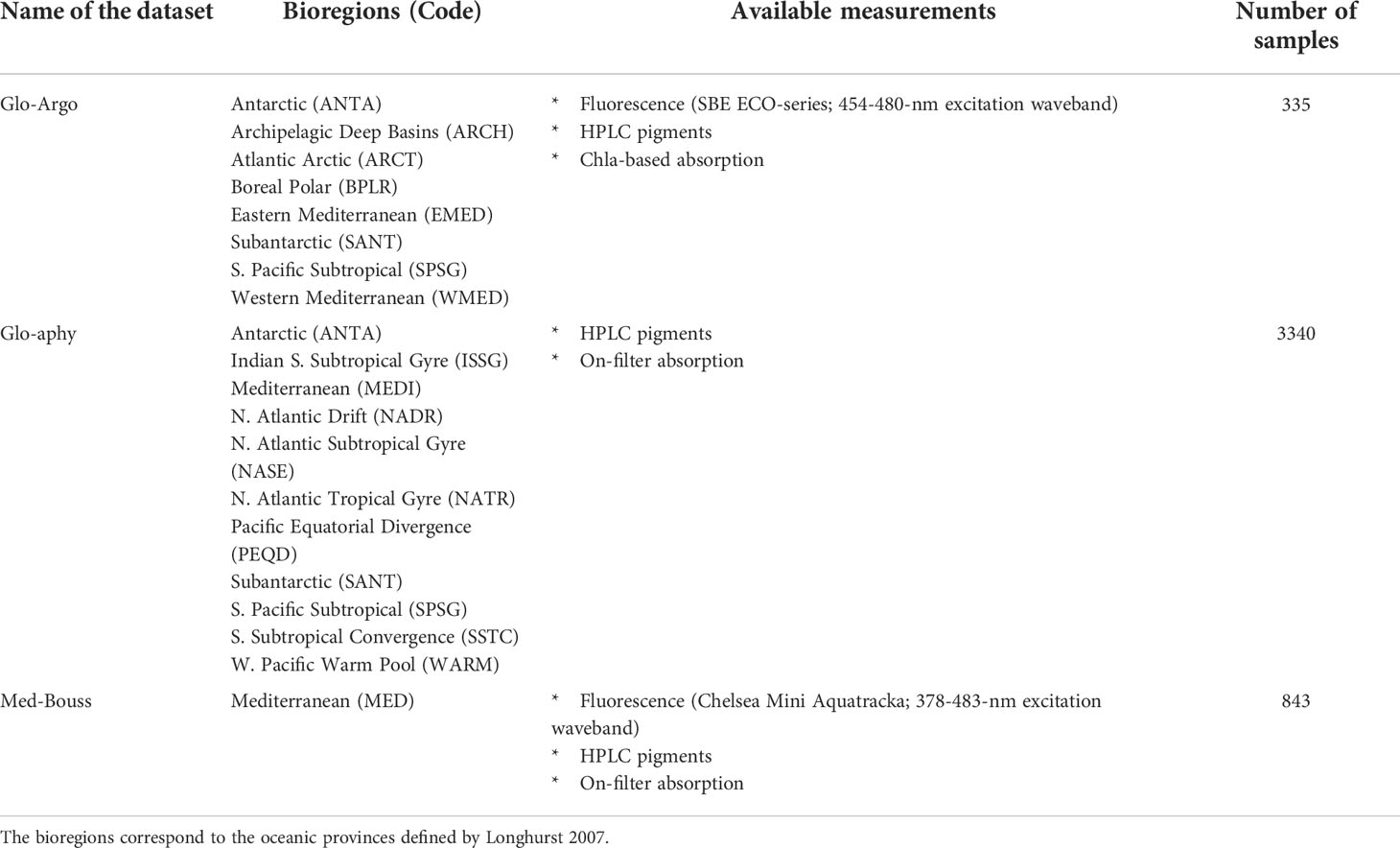
Table 1 Summary of the three different datasets used in the present study, the name of the sampled bioregions with the correspond abbreviations, the available measurements and the number of samples in each dataset.
All of the BGC-Argo floats are equipped with identical SBE ECO Puck Triplet fluorometers, to which the same standard calibration procedure is applied (Schmechtig et al., 2014). Thisensures that the observed variability in the fluorescence signal can be attributed to natural variability rather than to inter-sensor variability. The [Chla]fluo is calculated from F, expressed as counts, a relative unit, following the manufacturer calibration coefficient (cf. Equation 2). The data are corrected for non-photochemical quenching (NPQ) following the method of Xing et al. (2012) In Brief, this method extrapolates the deep fluorescence value toward surface. It has been validated using data from contrasted conditions in the Southern Ocean and Mediterranean Sea and successfully used in various open oceans (Barbieux et al., 2018; Mignot et al., 2018; Taillandier et al., 2018). The [Chla]fluo values greater than 4 times the Cook distance of the regression between [Chla]fluo and [Chla]HPLC in each bioregion are identified as outliers and removed. Roesler et al. (2017) recommends a factor of 2 to be applied to the BGC-Argo [Chla]fluo data so as to account for the mean overestimation of [Chla] by the ECO-series fluorometers on the global scale. Nevertheless, the correction factor of 2 is not applied here as our goal is to compare regional values of the slope factor computed for the Glo-Argo database with those of Roesler et al. (2017). Finally, the euphotic depth (Zeu) was calculated from the [Chla]fluo vertical profile adjusted with [CHLA]HPLC following Morel and Maritorena (2001), which allows to compute Zeu for all vertical profiles, including the 13 night profiles; the data below Zeu were discarded. The final dataset comprises 335 samples distributed over 46 different profiles in 8 oceanic bioregions (Table 1).
Our global-scale investigation is completed by the analysis of the “Glo-aphy” dataset, which comprises concurrent HPLC pigment determinations and on-filter measurements of the phytoplankton absorption spectrum for seawater samples collected over 13 cruises from 1990 to 2016, most of them being part of the databases presented in Bricaud et al., 1995; Bricaud et al., 2004; Bricaud et al., 2010). This dataset includes 3340 in-situ data spanning 14 different bioregions that cover contrasted ocean environments from polar to subtropical biomes and, thus, may be considered as representative of the global open ocean. As for the Glo-Argo dataset, Zeu was computed following Morel and Maritorena (2001) and the data located below Zeu were discarded.
2.3 North Western Mediterranean Sea fluorescence database
In order to understand the seasonal and vertical variability of the fluorescence signal, we completed our analysis using the “Med-Bouss” database, a time series acquired at the long-term fixed station BOUSSOLE (BOUée pour l’acquiSition d’une Série Optique à Long termE) located in the Ligurian (Northwestern Mediterranean) Sea at 7°54′E, 43°22′N (Antoine et al., 2008). Seawater sampling was carried out at the BOUSSOLE station every month from 2013 to 2015, at seven discrete depths (5, 10, 20, 30, 40, 50 and 60 m) using a CTD-rosette system equipped with 12-L Niskin bottles (Golbol et al., 2020). A Chelsea Aquatracka III fluorometer was mounted on a CTD-rosette device and acquired fluorescence profiles concomitantly with seawater sampling. The Aquatracka fluorometer has an excitation waveband ranging from 378 to 483 nm with a peak at 430 nm, and an emission waveband ranging from 670 to 700 centered on 685 nm. The fluorometer calibration did not change over the whole time series. The fluorescence output is expressed as RFU. The total dataset comprises 843 samples for which are available concurrent data of [Chla]fluo, [Chla]HPLC, HPLC-determined accessory pigments, and on-filter phytoplankton absorption spectra.
2.4 Phytoplankton absorption
For both the Glo-aphy and Med-Bouss databases, the spectral phytoplankton absorption coefficient, aph(λ) (m-1), was measured following the same analytical protocol and using the same filters as those used afterwards for HPLC analyses (Bricaud et al., 1995; Bricaud et al., 2004; Bricaud et al., 2010). In brief, absorption measurements were performed with a Perkin Elmer 850 spectrophotometer, equipped with a 150 mm diameter integrating sphere, using a blank filter as reference. Spectra were shifted to zero in the near infrared by subtracting the average optical density between 750 and 800 nm (Röttgers and Gehnke, 2012). Optical densities were then corrected for the amplification effect (Bricaud and Stramski, 1990) and converted into particulate absorption (in m-1) (Allali et al., 1997). The contribution of phytoplankton to the particulate absorption was then determined following the numerical decomposition of Bricaud et al. (2010). In this study we focus on the specific absorption wavelength of 470 nm, which corresponds to the excitation wavelength of the SBE ECO-series fluorometer mounted on the BGC-Argo floats. We recall that phytoplankton absorption was not systematically available for the Glo-Argo dataset and that aph was derived from [Chla]HPLC following the general relationship of Bricaud et al. (1995) regardless of the bioregion.
2.5 Phytoplankton pigments and community composition
For the global (Glo-Argo and Glo-aphy) and Mediterranean (Med-Bouss) datasets, the Chla concentration and the composition of phytoplankton communities are estimated using HPLC pigment measurements. In brief, seawater from discrete sampling is filtered onto glass fiber filters (GF/F Whatman 25 mm), that are stored in liquid nitrogen during cruises then transferred at −80°C in the laboratory until further analysis at the SAPIGH HPLC analytical facility at the Institut de la Mer de Villefranche (IMEV). Phytoplankton pigments are extracted from the cells by sonication in 100% methanol, clarified by filtration (GF/F Whatman 0.7 µm), and finally separated and quantified by HPLC. More details about the HPLC analytical protocol may be found in Ras et al. (2008). The concentration of total chlorophyll-a (TChla) is defined as the sum of Chla, divinyl-chlorophyll-a and chlorophyllid-a concentrations. In this paper, we will refer to [Tchla] as [Chla]. We specifically investigate the distribution of seven diagnostic pigments (DP) identified, among the full suite of accessory pigments, as biomarkers of major phytoplankton taxa, further grouped into three size classes (Claustre, 1994; Vidussi et al., 2001). The seven DP are presented in Table 2 along with their abbreviations which, for the sake of simplicity, will be used hereafter.
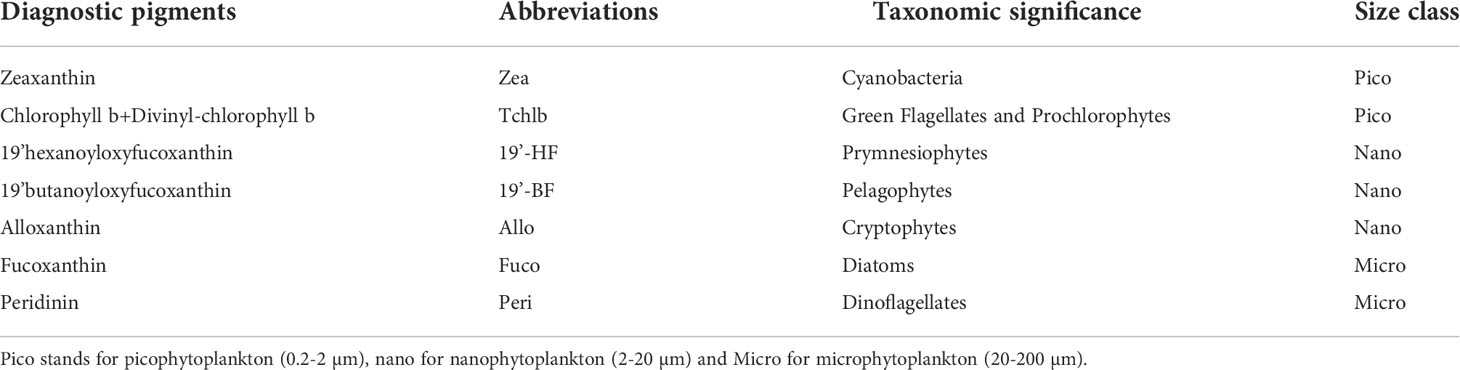
Table 2 Major biomarker pigments used in this study with their abbreviation, taxonomic significance and associated size class (Vidussi et al., 2001).
The DP-based method allows the estimation of the relative contribution to the [Chla] of three phytoplankton size classes: micro- (>20 μm), nano- (2-20 μm) and pico-phytoplankton (<2 μm) following equations given in Uitz et al. (2006). We note that, because it relies on biomarker pigment concentrations, this approach yields an average, synthetic estimate of both the taxonomic and size composition of the phytoplankton communities. Although we recognize that it has limits because some phytoplankton taxa may occasionally span several size classes and some DP may be found in several taxa, this approach has been shown to provide reliable, quantitative information for use on large spatial and temporal scales (e.g. Bricaud et al., 2004; Uitz et al., 2006; Brewin et al., 2014).
2.6 Data analysis
We determined the three variables of interest, i.e. slope factor, Chla-specific absorption at 470 nm (a*(470)) and quantum yield of fluorescence (Ф), using a type I linear regression model applied at the regional scale for the Glo-Argo and Glo-aphy datasets and at the seasonal and vertical scale for the Med-Bouss dataset.
The slope factor is computed as a regression of type I between [Chla]fluo and [Chla]HPLC. A type I regression is selected in regard of the robustness of the HPLC estimation of [Chla], considered as the reference (Claustre et al., 2004). The intercept is set to 0 because, after subtraction of the dark signal, an absence of Chla in the environment should be associated with a null fluorescence signal. Thus, the intercept corresponds the dark calibration, which improves the robustness of the statistics.
The Chla specific absorption coefficient of phytoplankton at 470 nm, a*(470), is calculated as the coefficient of the regression between the phytoplanktonic absorption at 470 nm, aph(470) (m-1), and [Chla]HPLC (mg m-3). The retrieved a*(470) coefficient is then expressed in m-2 mg Chla-1.
The fluorescence quantum yield, Ф, is calculated as the coefficient of the regression between F and aph(λ) (m-1), λ being the wavelength of the excitation peak of the considered fluorometer. For the Glo-Argo dataset, F is expressed as counts and λ equals 470 nm; and for Med-Bouss dataset, F is expressed as RFU and λ equals 430 nm. The obtained Ф is then expressed either as counts m-1 or RFU m-1 for the Glo-Argo or Med-Bouss dataset, respectively. It represents the raw output value of the fluorometer subsequently to light absorption by phytoplankton cells.
Each regression is performed on the bioregional scale for the Glo-Argo and Glo-aphy dataset. For the Med-Bouss dataset, we merged the data acquired during the three consecutive years in order to optimize the number of data per regression and thus the robustness of the statistics. Statistics from the regressions are provided in the supplementary material.
A principal component analysis (PCA), performed with the FactoMineR package version 2.4 (Lê et al., 2008), is used to investigate the succession of phytoplankton communities at the Mediterranean Sea site (Med-Bouss dataset). All analyses were performed with the R software, version 4.1.2.
3 Results
3.1 Global variability of the slope factor
In order to investigate the influence of phytoplankton community composition on the estimation of [Chla]fluo, concomitant measurements of [Chla]fluo from BGC-Argo floats and [Chla]HPLC are merged into a global-scale dataset (Glo-Argo). This dataset covers contrasted bioregions from high to low latitudes (Figure 1A; Table 1). Thus, the variability of the slope factor in this dataset may be considered as representative of its variability on the global scale. The mean value of the slope factor is 2.2, which indicates that the SBE ECO fluorometer overestimates [Chla] on the global scale by a factor 2.2. Additionally, the slope factor shows different values depending on the bioregions (Figure 1B). There is a large variability between high and low latitude environments, with a maximum value of 2.8 observed in the Boreal Polar Province (BPLR) in the North Atlantic Ocean, and a minimum value of 1.1 in the Archipelagic Deep Basin Province (ARCH) in the subtropical Pacific Ocean. In the Mediterranean Sea, the slope factor ranges from 1.7 to 1.9 in the eastern (EMED) and western (WMED) basins, respectively. Our results are consistent with those of Roesler et al. (2017), who reported a mean global value of 2, and a regional variability characterized by higher values in polar regions and lower values in subtropical regions.
We now consider the distribution of the slope factor on a ternary diagram representing the pigment-based composition of phytoplankton communities in the Glo-Argo dataset (Figure 2). The data follow a gradient from microphytoplankton-dominated communities typical of polar and sub-polar regions, to picophytoplankton-dominated communities in the subtropical regions. In between, there are mixed and nanophytoplankton-dominated communities associated with the temperate waters of the Mediterranean Sea. The regression between the values of the slope factor and the Chla relative contribution of the three phytoplankton size classes is displayed as the background of the ternary diagram. It shows a clear pattern where the communities dominated by larger phytoplankton groups are associated with high values of the slope factor. Thus, our analysis indicates that the observed regional patterns of the slope factor (Figure 1 and Roesler et al., 2017) are consistent with the patterns associated with the composition of the phytoplankton communities (Figure 2). This suggests that the regional variability in the slope factor may in fact be influenced by phytoplankton community composition.
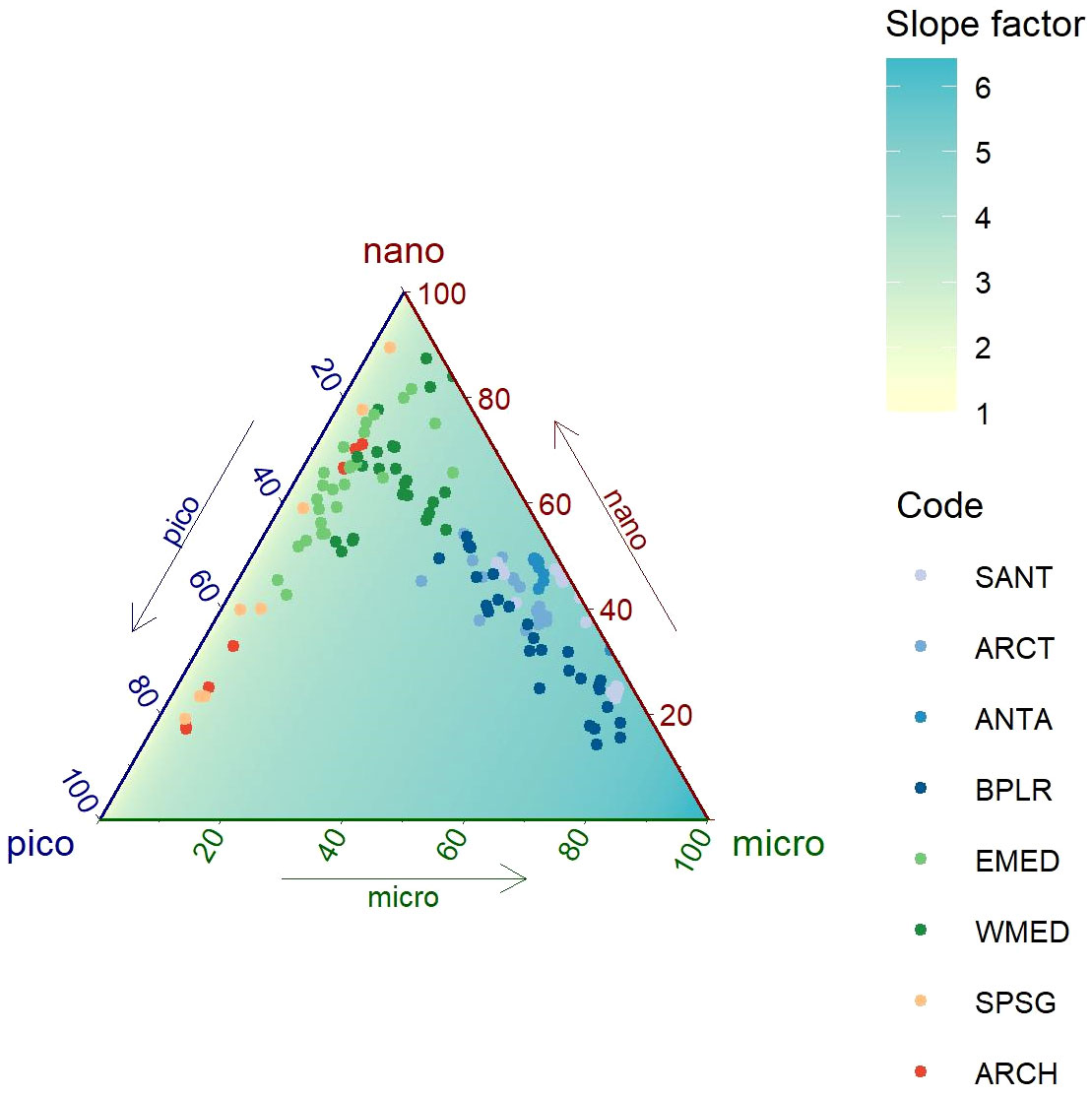
Figure 2 Ternary diagram of the pigment-based relative contribution to the Chla concentration of three phytoplankton size classes (micro-, nano-, and picophytoplankton) in the global Glo-Argo dataset. The color of the background indicates the value of the [Chla]fluo/[Chla]HPLC ratio extrapolated with a linear regression model. The color of the datapoints indicates the corresponding bioregions (ANTA, Antarctic Province; ARCH, Archipelagic Deep Basin Province; ARCT, Atlantic arctic Province; BPLR, Boreal Polar Province; EMED, Eastern Mediterranean Sea; SANT, Subantarctic Province; SPSG, South Pacific Subtropical Gyre Province; WMED, Western Mediterranean Sea).
In order to understand the mechanisms underpinning the correlation between the slope factor and phytoplankton community composition, we examine the variability of the two main photophysiological properties influencing the fluorescence signal, i.e. the Chla-specific absorption coefficient and the fluorescence quantum yield.
3.2 Variability of the phytoplankton absorption coefficient
The influence of the phytoplankton community composition on the phytoplankton Chla-specific absorption coefficient is first investigated using the Glo-aphy dataset. Similarly to the Glo-Argo database, Glo-aphy encompasses a wide variety of oceanic regimes, including polar, temperate and subtropical bioregions (Figure 3A; Table 1). The value of a*(470) follows a regional distribution (Figure 3B) and ranges from 0.024 to 0.071 m2 (mg Chla)-1, with a magnitude of variation of 2.95. On the one hand, the North Atlantic Subtropical gyre (NASE) and North Atlantic Drift (NADR) bioregions display the highest a*(470) coefficient of the dataset with values of ~0.07 m² (mg Chla)-1. These bioregions are characterized by oligotrophic waters, dominated by picophytoplankton with large concentrations of zeaxanthin. On the other hand, polar bioregions like SANT and ANTA, dominated by larger cells, show the lowest a*(470) values of ~0.02 m2 (mg Chla)-1.
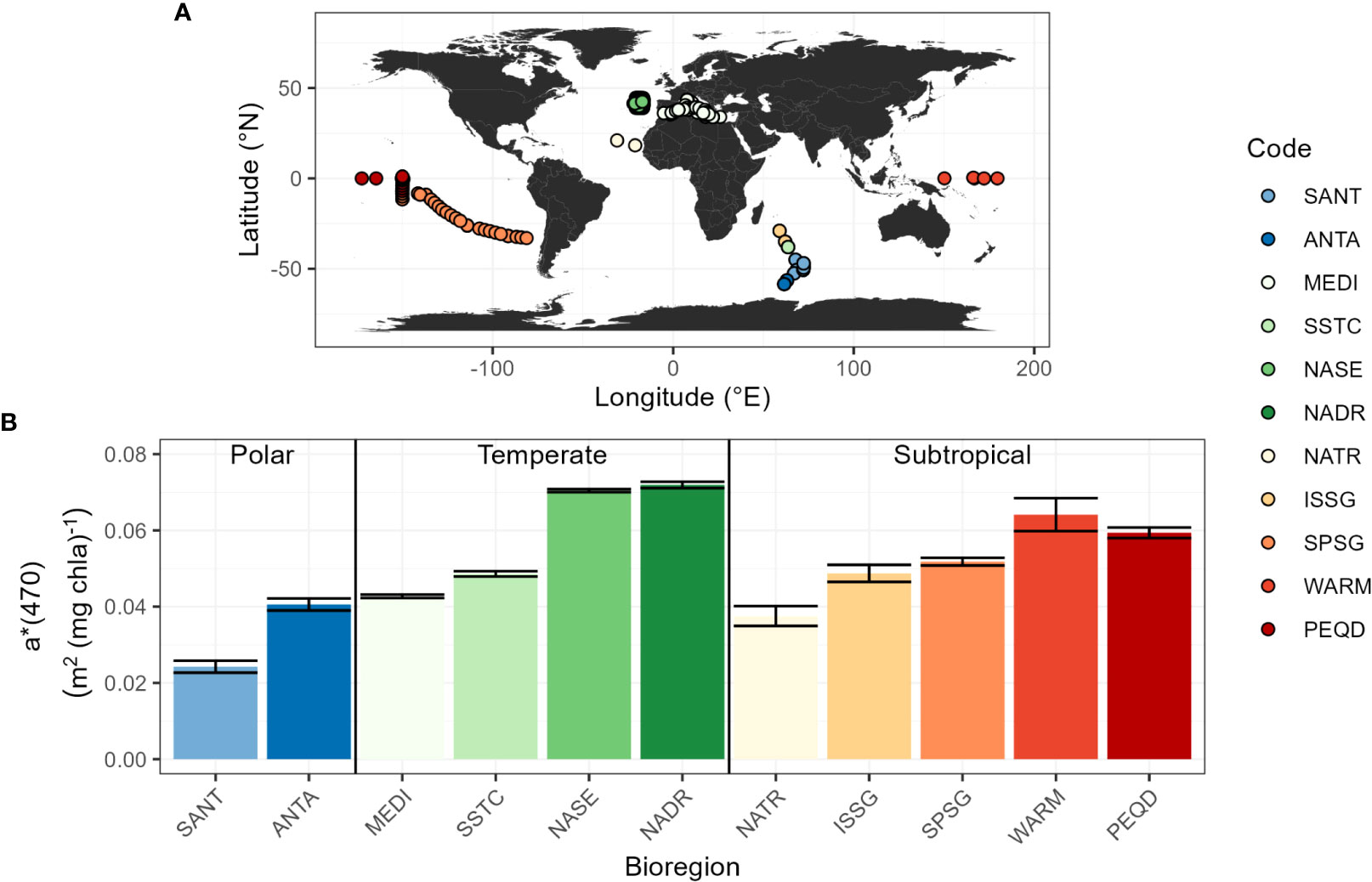
Figure 3 (A) Geographic location of the sampling stations in the Glo-Aphy database (B) Distribution of the Chla-specific absorption coefficient at 470 nm, a*(470) (m2(mg Chla)-1), calculated from a linear regression between aph(470) and the Chla concentration for each bioregion of the global Glo-aphy dataset (see Table 1 for code significance). The error bars indicate the 95% confidence limits.
In addition to the global-scale analysis, the vertical and seasonal variability of a*(470) induced by changes in the phytoplankton communities is investigated using the Mediterranean (Med-Bouss) dataset. This dataset covers three successive annual cycles and seven different depths, and presents contrasted environmental conditions due to the pronounced seasonality of this bioregion (Marty et al., 2002; Durrieu de Madron et al., 2011) with [Chla]HPLC values ranging from ~0.03 to 2.50 mg m-3. In the next paragraphs, we first describe the seasonal and vertical distribution of phytoplankton communities based on a Principle Component Analysis (PCA) and then present the distribution of the a*(470) coefficient.
A PCA projection of the pigment data from the Med-Bouss dataset is performed to visualize the distribution of the different phytoplankton communities over the seasonal cycle and the vertical dimension (Figure 4). On the first principal component (PC1), we observe a discrimination of the different sampling depths, indicating that PC1 represents the vertical distribution of the phytoplankton communities. On the second principal component (PC2), we observe a seasonal discrimination, spring and winter in the lower part of the axis and summer and fall in the upper part. Indeed, summer is characterized by stratified waters with a shallow mixed layer, whereas other seasons are characterized by a deeper mixed layer. In spring, the increase of sun light associated with nutrient-replenished upper layer resulting from a deep winter mixed layer depth (MLD) leads to the emergence of a seasonal bloom, progressively consuming the surface nutrients and eventually leading to the formation of a deep chlorophyll maximum (DCM) (Lavigne et al., 2015; Barbieux et al., 2019). In summer and fall, surface waters are typically dominated by zeaxanthin, indicative of the presence of pico-prokaryotes (Sammartino et al., 2015; Trombetta et al., 2020) whereas the concentration of 19’-BF and 19’-HF increase in deeper waters, indicating an enhanced contribution of nanophytoplankton cells such as prymnesiophytes and chrysophytes (Bustillos-Guzmán et al., 1995) to the phytoplankton assemblage at depth. In winter, we observe an increased concentration of fucoxanthin, mostly indicative of the presence of diatoms (generally microphytoplankton). Those observations are consistent with the phytoplankton succession described by Marty et al. (2002), which gives us good confidence that the PCA representation efficiently grasps the vertical as well as seasonal variations of phytoplankton communities.
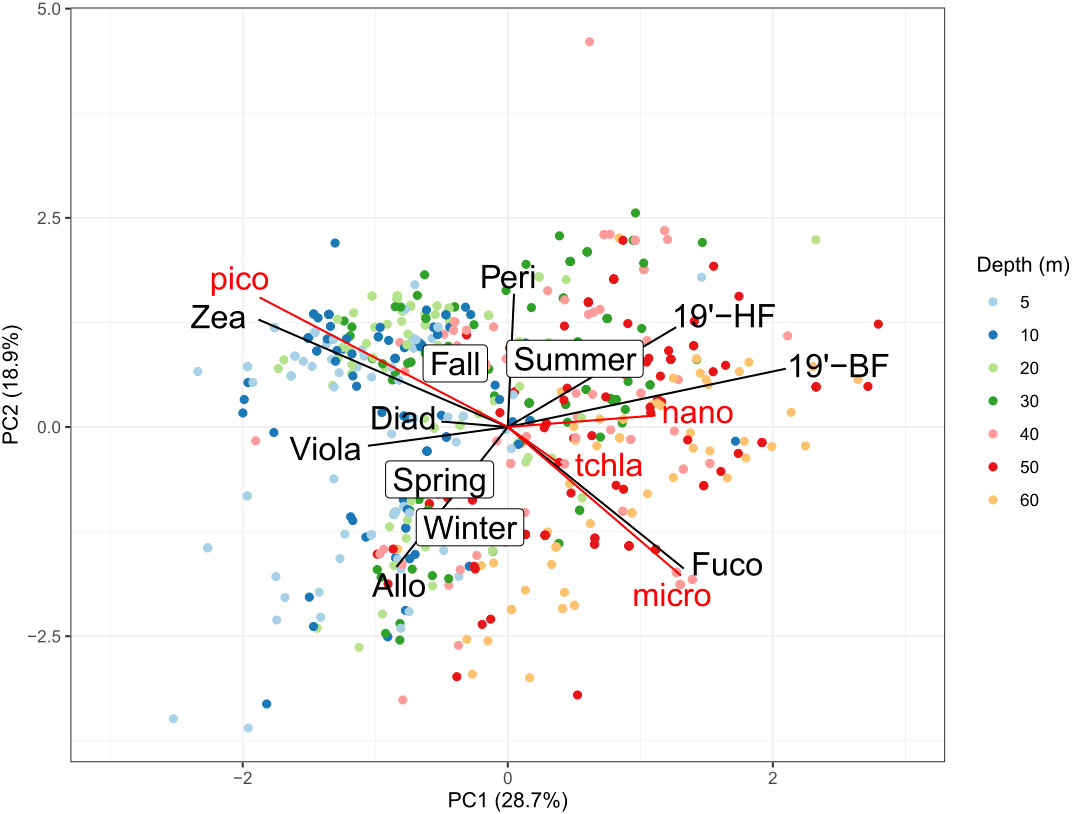
Figure 4 Orthogonal projection of the two first principal components of the PCA analysis of the concentrations of the seven diagnostic pigments in the Northwestern Mediterranean Med-Bouss database, between 5 and 60 m depth over 3 years (2013–2015). The color of each point represents the sampling depth as indicated. The relative contribution to the Chla concentration of each of the three phytoplankton size classes and the seasons are superimposed as supplementary variables onto the PCA projection space.
In the Med-Bouss dataset, the a*(470) coefficient ranges from 0.017 to 0.068 m2 (mg Chla)-1 (Figure 5), which is coherent with observations on the global scale in comparable regimes (Figure 3). It shows a relatively low vertical variability in winter and fall, when the water column is mixed (e.g. D’Ortenzio et al., 2005), with values comprised between 0.03 and 0.04 m2 (mg Chla)-1 in winter, and 0.04 to 0.05 m2 (mg Chla)-1 in fall (Figure 5). The a*(470) coefficient reaches a maximum of 0.07 m2 (mg Chla)-1 in summer at surface, in picophytoplankton dominated communities, and slowly decreases from fall to winter when phytoplankton communities are dominated by larger cells. Lower values are observed in winter and below 20 m in spring and summer where it decreases to less than 0.04 m2 (mg Chla)-1. This is consistent with our observations in the global-scale (Glo-aphy) database where higher values of a*(470) are encountered in picophytoplankton-dominated communities.
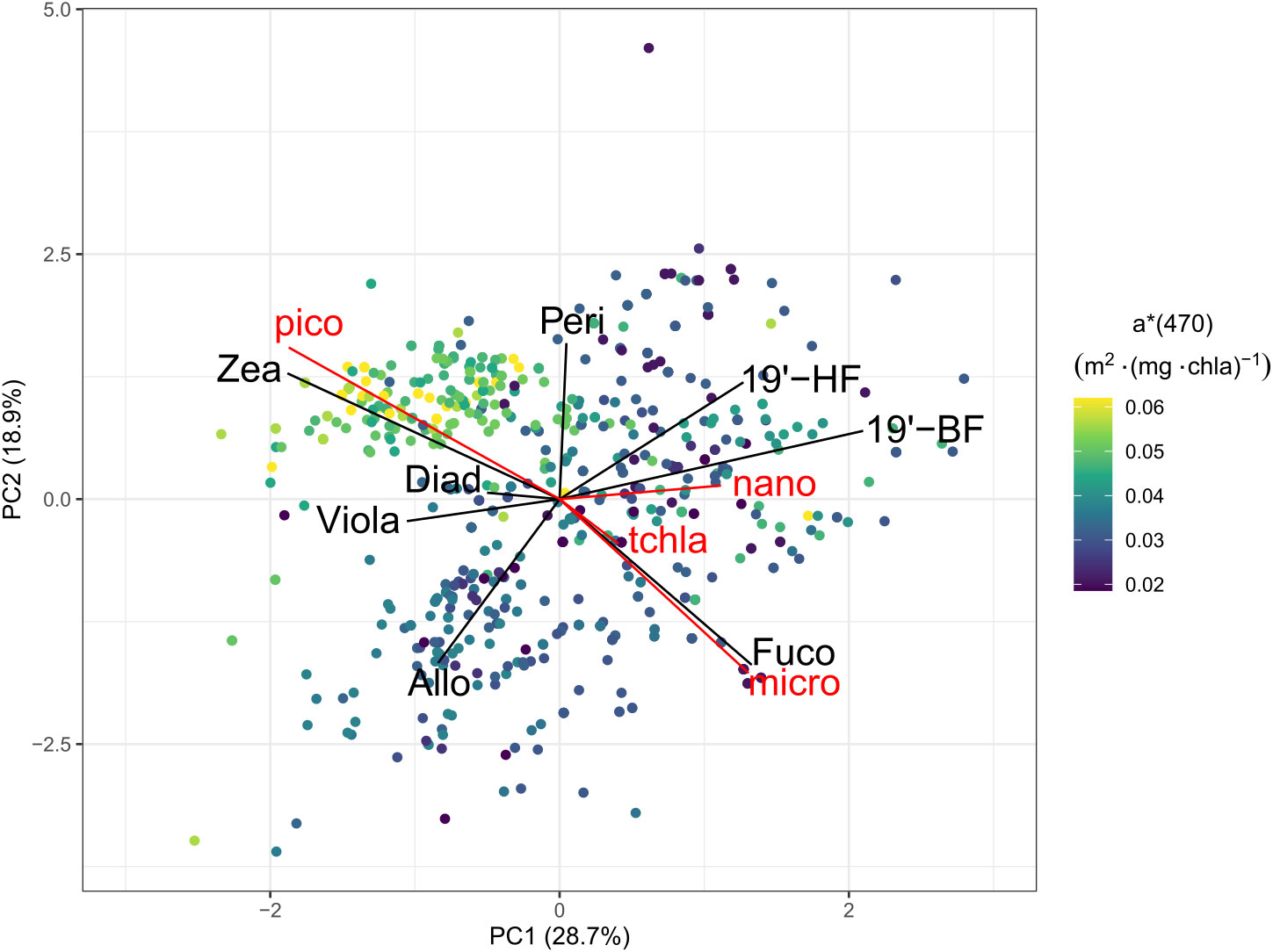
Figure 5 Orthogonal projection of the two first principal components of the PCA analysis of the concentrations of the seven diagnostic pigments in Northwestern Mediterranean Med-Bouss dataset, between 5 and 60 m depth over 3 years (2013–2015). The color of each point represents the Chla-specific absorption coefficient at 470 nm, a*(470), which did not serve in the construction of the projection. The relative contribution to Chla of each of the three phytoplankton size classes and the seasons are superimposed as supplementary variables onto the PCA projection space.
In order to determine the influence of phytoplankton pigments and, thereby, community composition on phytoplankton absorption, we consider the distribution of a*(470) in the orthogonal projection of PC1 and PC2 of the pigment based PCA (Figure 5). We observe, in particular, that the increase in a*(470) in the surface layer during the summer season coincides with the occurrence of picophytoplankton-dominated communities, with high concentrations of zeaxanthin. The highest values of a*(470) (>0.04 m2 (mg Chla)-1) are exclusively observed in such communities, while moderate to low values of a*(470) are distributed amongst nano- and micro-phytoplankton-dominated communities with no clear pattern in terms of pigments composition.
Our results indicate a significant variability of the a*(470) coefficient, not only on the global scale among the considered bioregions (Figure 3B), but also within a given bioregion, vertically within the water column as well as seasonally (Figures 5, 6). Importantly, the global-scale variability in the a*(470) coefficient (Figure 3) does not follow the same trend as the slope factor (Figure 1), which shows higher values in high latitude regions and lower values in low latitude regions. Therefore, the a*(470) coefficient is unlikely to be the only driver of the variability of the ratio between F and [Chla], i.e. the slope factor. This led us to examine the scales of variability of the fluorescence quantum yield.
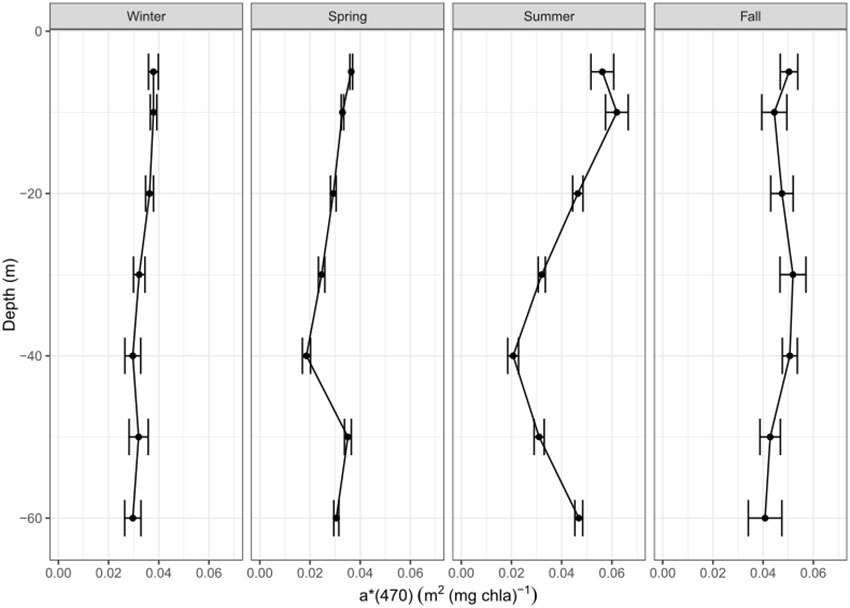
Figure 6 Vertical distribution of the phytoplankton chlorophyll-specific absorption coefficient at 470 nm, a*(470) in m2 (mg Chla)-1, for each season and sampling depth of the Med-Bouss dataset.
3.3 Variability of the fluorescence quantum yield
We investigate the role of the second potential driver of the slope factor, the quantum yield of fluorescence, using the Med-Bouss and Glo-Argo datasets. In the Mediterranean dataset, the fluorescence quantum yield appears to vary between 5 and 12 RFU m-1 depending on the season and depth (Figure 7). The maximum values are observed near the DCM in spring (12 RFU m-1) and at depth in winter (10 RFU m-1), whereas the minimum values are observed below 20 m in summer (~5 RFU m-1). The summer season is characterized by a relatively low vertical variability. In contrast, a strong vertical pattern is found in spring, when the fluorescence quantum yield shows significantly higher values at 30-40 m than at surface (5 m). We also note that the fluorescence quantum yield shows larger values in spring than in summer. In fall, the standard deviation at most depths is larger than the vertical and seasonal variability.
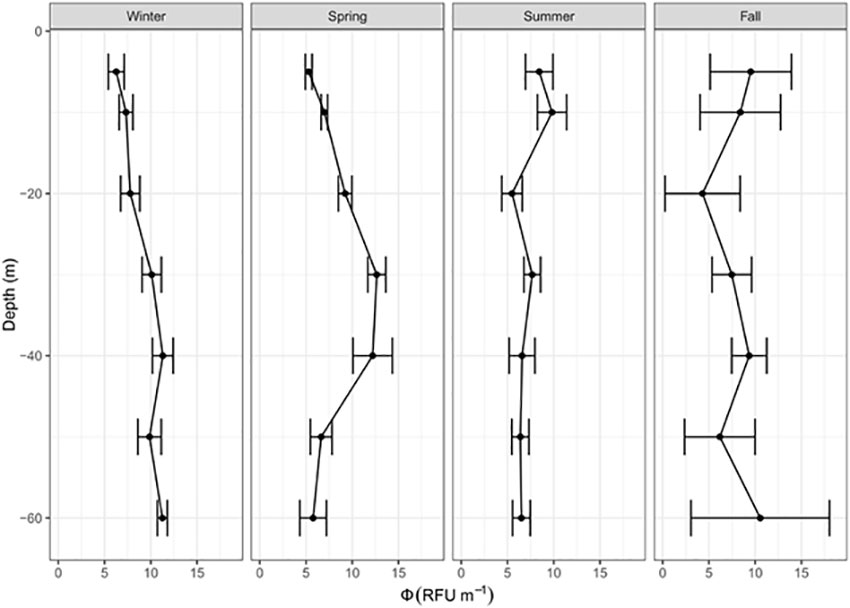
Figure 7 Vertical distribution of the quantum yield of fluorescence, Φ (RFU m-1), for the Med-Bouss dataset for each season and each sampling depth.
On a global scale, the regional variations determined from the Glo-Argo dataset for the fluorescence quantum yield are presented in Figure 8. The fluorescence quantum yield varies between 6800 counts.m-1 and 13900 counts.m-1 for the subtropical ARCH and polar BPLR bioregions, respectively. The maximum values are found in high latitude regions in contrast to subtropical regions characterized by lower values of the fluorescence quantum yield. Temperate regions have a similar value of Φ as subtropical regions with around 1000 counts m-1.
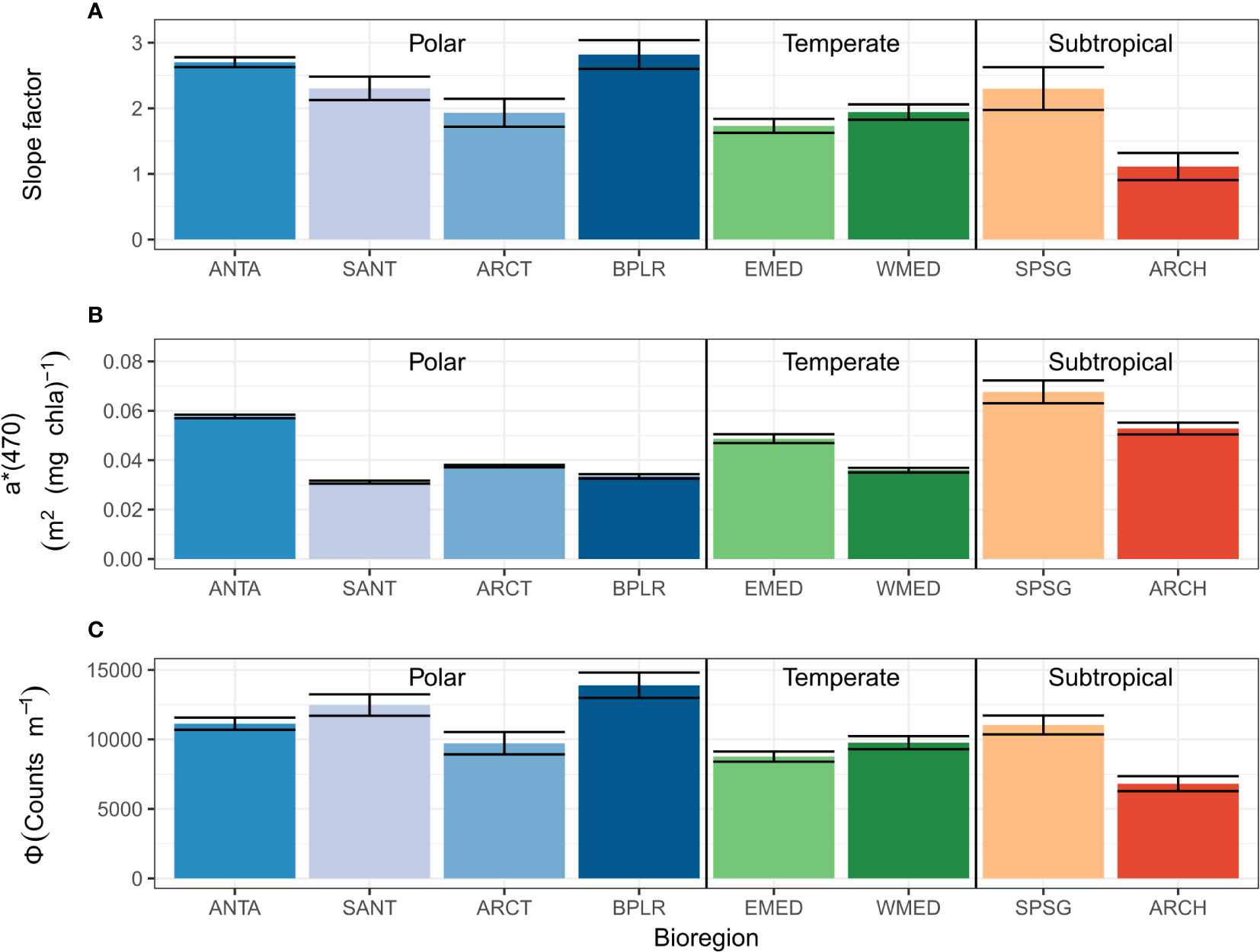
Figure 8 (A) Distribution of the slope factor, defined as the coefficient of the regression between [Chla]Fluo and [Chla]HPLC, (B) Chla-specific absorption coefficient at 470 nm, a*(470) (m2(mg Chla)-1), and (C) fluorescence quantum yield, Φ (counts m-1), for the eight bioregions of the Glo-Argo dataset (ANTA, Antarctic Province; ARCH, Archipelagic Deep Basin Province; ARCT, Atlantic arctic Province; BPLR, Boreal Polar Province; EMED, Eastern Mediterranean Sea; SANT, Subantarctic Province; SPSG, South Pacific Subtropical Gyre Province; WMED, Western Mediterranean Sea). The error bars indicate 95% confidence limits.
For comparison purposes, we present a summary of the regional distribution of slope factor (Figure 8A, same as Figure 1B), the Chla-specific absorption coefficient (Figure 8B) and fluorescence quantum yield (Figure 8C) based on the analysis of the Glo-Argo database. On the one hand, some regions located in the subtropical biome (SPSG and ARCH) show a high value of the a*(470) coefficient, yet a relatively low value of Ф. This suggests that, in such systems, the absorbed light energy is not dissipated as fluorescence, which would result in a decrease of the F-to-[Chla] ratio and, thus, of the slope factor (close to 1 in the case of ARCH). On the other hand, high-latitude regions (SANT and ARCT) show moderate to low and moderate to high values of a*(470) and Ф, respectively, suggesting that the little energy that is received by phytoplankton cells is mostly reemitted as fluorescence, which leads to an increase in the F-to-[Chla] ratio and high values of the slope factor (~3).
4 Discussion
The estimation of [Chla] based on in-situ fluorometers relies on the correlation between F and [Chla]. This correlation has been shown to be significantly variable at the global scale between different regions of the open ocean (Roesler et al., 2017). We studied here the role of the composition of phytoplankton communities on this variability through its influence on the Chla-specific absorption coefficient and fluorescence quantum yield.
As expected from numerous previous studies (e.g. Bricaud et al., 2004; Brewin et al., 2011; Uitz et al., 2015), we observe maximum values of a*(470) in surface oligotrophic waters, i.e. in seasonally or permanently stratified open ocean conditions where picophytoplankton generally dominate the phytoplankton communities (Figure 2). The shape of the phytoplankton absorption spectrum is known to be mainly influenced by the package effect occurring in phytoplankton cells and the accessory pigment composition (Greg Mitchell and Kiefer, 1988; Bricaud et al., 1995; Cleveland, 1995; Bricaud et al., 1999). Picophytoplankton-dominated communities are characterized by a limited package effect because of a small average cell size, and large concentrations of non-photosynthetic carotenoids such as zeaxanthin, both of which lead to enhanced values of a*(λ) in the blue spectral region (Allali et al., 1997; Ciotti et al., 2002; Bricaud et al., 2004; Uitz et al., 2008). In such systems, the effect of photoacclimation combines with that of community composition and influences the vertical distribution of the a*(470) coefficient (e.g. Bricaud et al., 2004; Uitz et al., 2008; Uitz et al., 2015). First, acclimation to low light levels leads to lower a* values at depth due to an increase in the degree of pigment packaging associated with enhanced intracellular Chla content. Second, the zeaxanthin-to-Chla ratio is known to increase in high light regime (Six et al., 2004; Dubinsky and Stambler, 2009), resulting in larger a* values at surface.
Moreover, zeaxanthin is thought to be involved in photoprotection mechanisms (Demmig-Adams, 1990). This pigment enhances the energy dissipation through heat, hence diminishing the photosynthetic quantum yield (Bidigare et al., 1989; Olaizola and Yamamoto, 1994) and the fluorescence quantum yield (Kiefer and Reynolds, 1992). This is consistent with our observation that bioregions with high concentrations of zeaxanthin, such as SPSG and ARCH, are also associated with relatively low values of the fluorescence quantum yield (Figure 8). The effect of the decrease in the fluorescence quantum yield due to zeaxanthin appears to be greater than that of the increase in a*(470), resulting in a lower slope factor in picophytoplankton-dominated communities.
In the Mediterranean Sea in summer, the surface waters show high values of the Chla-specific absorption coefficient associated with a dominant contribution of picophytoplankton to the algal community and large concentrations of zeaxanthin. Yet they do not present a significantly lower fluorescence quantum yield (Figure 7), compared to the other depths and seasons, as could be expected from our results based on the global dataset (Figure 8). This result may be explained by the optical specifications of the Chelsea Aquatracka III fluorometer used during the BOUSSOLE cruises. Indeed, this fluorometer has an excitation wavelength of 430 nm that does not coincide with the absorption peak of zeaxanthin, unlike the ECO fluorometers mounted on the BGC-Argo floats that have an excitation wavelength of 470 nm. The Chelsea Aquatracka III fluorometer excites a region of the phytoplankton absorption spectrum closer to the absorption peak of Chla in solution compared to the spectral region targeted by the ECO-series fluorometer. Thus, the influence of zeaxanthin on the fluorescence response is diminished. Our results, therefore, suggest that using a fluorometer with an excitation wavelength of 430 nm may reduce the effect of zeaxanthin on the fluorescence quantum yield and, thereby, the influence of the phytoplankton community composition on the slope factor and subsequent [Chla] retrieval.
The fluorescence quantum yield has been reported to be dependent on the physiological state of phytoplankton cells (Falkowski and Kolber, 1995; Behrenfeld et al., 2009). In this study, we found a significant influence of phytoplankton community composition. Consistent trends are observed between the datasets from the Northwestern Mediterranean Sea and global ocean. In particular, higher values of Ф are found in communities characterized by a dominance of large phytoplankton cells and high [Chla], as is the case in the Northwestern Mediterranean Sea in spring or in high latitude bioregions. In contrast, lower Ф values are found in oligotrophic regions, which we attribute to the presence of photoprotective pigments, specifically zeaxanthin.
Explaining the high values of Ф in the high latitude regions remains a challenge. In the Southern Ocean, iron limitation plays an important role in the modulation of the fluorescence signal (Schallenberg, 2022). Southern Ocean phytoplankton communities often cope with iron and light colimitation (e.g. Boyd et al., 2000). In such conditions, they adapt their photosynthetic apparatus by increasing the size of the light-harvesting antenna in the photosynthetic unit, rather than by increasing the number of reaction centers, which would be iron-consuming (Strzepek et al., 2012; Strzepek et al., 2019; Schallenberg et al., 2020). An increase in the size of the antenna leads to a modification of the light transfer efficiency of the photosynthetic unit (Strzepek et al., 2012; Schallenberg et al., 2020), with a probable influence on the F-to-[Chla] ratio. Nevertheless, in this study, we observe high values of Ф in three different polar regions (SANT, ARCT, BPLR; Figure 8), not only in the Southern Ocean but also in the Arctic Ocean. This suggests that the large Ф values do not necessarily solely result from regional environmental conditions, such as iron depletion that is more specific to the Southern Ocean and may lead to atypical photophysiological properties (Behrenfeld et al., 2009). The large Ф values may also result from a common factor in these polar regions, such as a predominance of large phytoplankton cells (Figure 2). Due to the relatively small size of the Glo-Argo dataset, we could not investigate the temporal dynamics of the F-to-[Chla] ratio in relation to iron depletion or input events, which would help to disentangle the likely combined effects of iron and community composition on the fluorescence quantum yield.
In addition, the limited size of the Glo-Argo dataset hinders our ability to account for the seasonal and/or vertical variability of a*(470) and Ф in each bioregion that may lead to discrepancies between bioregions from the same biome. For instance, the SPSG bioregion presents a relatively high Ф despite belonging to the subtropical biome. The SPSG bioregion is characterized by a relatively large diversity of phytoplankton community size structure, being dominated by picophytoplankton or nanophytoplankton (Figure 2). This diversity may lead to unexpectedly high values of Ф, compared to the other subtropical region. This result is consistent with our conclusion that, beyond the physiological state of phytoplankton cells, the fluorescence quantum yield may be largely influenced by the composition of phytoplankton communities.
The present study establishes a correlation between the phytoplankton community composition and the two major drivers of the in-vivo fluorescence process, a*(λ) and Ф. The effects of those two parameters are integrated in the slope factor index, which reflects the variability of the F-to-[Chla] ratio. Thus, the [Chla]fluo estimation shows spatial, seasonal and vertical variability that reflects changes in phytoplankton community composition. Therefore, we suggest that integrating indicators of the phytoplankton community composition into the conversion of F into [Chla]fluo would contribute to improving the estimation of [Chla]fluo.
5 Conclusion and perspectives
In-situ fluorescence used in combination with autonomous platforms, such as BGC-Argo profiling floats, provides a powerful means for obtaining a global assessment of the Chla concentration, a major proxy of the phytoplankton biomass (e.g. Lombard et al., 2019; Claustre et al., 2020). However, the natural variability in the phytoplankton fluorescence response may considerably impede the estimation of the Chla concentration and, hence, needs to be apprehended (e.g. Proctor and Roesler, 2010; Roesler et al., 2017). The present study aims to better understand the influence of phytoplankton community composition on the natural variability of the in-situ fluorescence signal. In this end, we analyzed concurrent measurements of fluorescence, pigment concentrations determined by HPLC, and phytoplankton absorption spectra from various open ocean regions, and addressed the global variability in addition to the seasonal and vertical variability within a given region. Our results suggest a significant influence of phytoplankton community composition on the fluorescence signal, resulting from different photophysiological properties, i.e. the absorption coefficient and the fluorescence quantum yield, between phytoplankton groups.
Our results indicate that accounting for the composition of phytoplankton communities may help to better constrain the estimation of the Chla concentration based on in-situ fluorescence measurements. Hence, we suggest that incorporating an index of phytoplankton community composition into the conversion of F into [Chla]fluo may be a relatively simple, effective way to improve the quantification of the Chla concentration based on BGC-Argo float observations. Different methods have been introduced to derive information on the taxonomic or size structure of phytoplankton communities from BGC-Argo float measurements. In particular, Briggs et al. (2013) proposed a method to infer the mean size of the particle pool from high frequency time series of the particulate backscattering coefficient. Sauzède et al. (2015) developed a neural network-based approach to retrieve the Chla concentration attributed to three distinct phytoplankton size classes from the shape of the fluorescence profile. More recently, Rembauville et al. (2017) introduced a method that retrieves the relative contribution to the stock of particulate organic carbon of three phytoplankton size classes. While the methods of Sauzède et al. (2015) or Rembauville et al. (2017) provide comprehensive information on the community size structure from machine learning, some methods rely on the particulate backscattering-to-Chla ratio and can be used to estimate a simple optical index of the phytoplankton community composition (Cetinić et al., 2015; Lacour et al., 2017). Therefore, the global scale analysis of the covariance between such optical index of the community composition, a*(470) and Ф could allow to optimize the conversion of F into [Chla]fluo for application to BGC-Argo float fluorometers. Today, the challenge is to collect and merge the appropriate dataset. It should be large enough to cover different contrasted oceanic systems encompassing a continuum of phytoplankton community taxonomic and size structure and generate statistically robust results. The resulting relationship could allow the emergence of a real time correction of the [Chla]Fluo. Ultimately, the correction factor would reflect phytoplankton community composition variability and thus, would vary on the regional and seasonal scale. Thus, we strongly support Bittig et al. (2019) recommendation to collect seawater samples to perform phytoplankton HPLC pigments and spectral absorption analyses at BGC-Argo float deployment.
Our study shows that non-photosynthetic pigments may play an important role in the reduction of the ratio between fluorescence and Chla concentration in the surface layer of stratified oligotrophic waters. The current 470 nm excitation wavelength of the ECO-series fluorometer equipping BGC-Argo floats does not coincide with the spectral band of the Chla absorption maximum. The use of a fluorometer with an excitation closer to 430 nm would ensure to target the Chla in vivo absorption peak and, hence, likely reduce the influence of accessory pigments on the fluorescence signal (Bricaud et al., 2004; Proctor and Roesler, 2010). We recommend to investigate such possibility in the future, by deploying in the field a fluorometer with two excitation channels, one at 470 nm and one closer to 430 nm. This should be done in association with HPLC pigment measurements in order to compare the variability of the slope factors obtained from the two different excitation channels.
Eventually, taking into account the composition of phytoplankton communities for the retrieval of the Chla concentration from current in-situ fluorometers, or using new multi-channel sensors implemented on observation platforms, BGC-Argo floats in particular, will lead to substantially more robust estimates of the phytoplankton biomass on a broad range of spatial and temporal scales. Such quantitative information is critical to understand and model biogeochemical cycles in the global ocean. In a context of climate change, there is an urgent need to improve biogeochemical models whose initialization and validation will, in the future, rely more and more on BGC-Argo float observations, as this global observation system develops (Cossarini et al., 2019; Claustre et al., 2020).
Data availability statement
Publicly available datasets were analyzed in this study. This data can be found here: https://github.com/Flavi1P/fluorescence.
Author contributions
FP, JU, HC initiated the study, designed the work, and drafted the manuscript. FP gathered the different datasets, performed the data analysis and created the plots. VV and MG planned and performed seawater sampling at the BOUSSOLE site. JR and CD performed the HPLC pigment analyses. CS handled BGC-Argo data archiving and distribution, and merged the global BGC-Argo and HPLC dataset. AP prepared and tested the BGC-Argo floats prior to deployment and set up the raw data stream. All authors contributed to and approved the manuscript.
Funding
This paper represents a contribution to the following projects: OBOO funded by CNRS INSU via the LEFE-CYBER program; remOcean funded by ERC (grant 246777); NAOS funded by ANR EquipEx (grant J11R107-F); SOCLIM funded by the Fondation BNP Paribas; and BOUSSOLE funded by CNES and ESA (contract N° 4000119096/17/I-BG) with support of the French Oceanographic Fleet. FP is funded by a PhD grant from Sorbonne Université (Ecole Doctorale 129).
Acknowledgments
The float data were collected and made freely available by the International Argo Program and the national programs that contribute to it. (https://argo.ucsd.edu, https://www.ocean-ops.org). The Argo Program is part of the Global Ocean Observing System. Finally we would like to thank the chief scientists and crew members of the cruises that contributed to the acquisition of the datasets used in this study: SOCLIM, OISO, MOBYDICK, MOOSE, Bio-ArgoMed, Dewex, GreenEdge and BOUSSOLE. We acknowledge Eva Àlvarez and Aurea Ciotti for their valuable comments on the manuscript. Phytoplankton pigment analyses were performed at the SAPIGH national HPLC analytical service at the Institut de la Mer de Villefranche (IMEV).
Conflict of interest
The authors declare that the research was conducted in the absence of any commercial or financial relationships that could be construed as a potential conflict of interest.
Publisher’s note
All claims expressed in this article are solely those of the authors and do not necessarily represent those of their affiliated organizations, or those of the publisher, the editors and the reviewers. Any product that may be evaluated in this article, or claim that may be made by its manufacturer, is not guaranteed or endorsed by the publisher.
References
Allali K., Bricaud A., Claustre H. (1997). Spatial variations in the chlorophyll-specific absorption coefficients of phytoplankton and photosynthetically active pigments in the equatorial pacific. J. Geophys. Res.: Ocean. 102, 12413–12423. doi: 10.1029/97JC00380
Alpine A. E., Cloern J. E. (1985). Differences in in vivo fluorescence yield between three phytoplankton size classes. J. Plankton Res. 7, 381–390. doi: 10.1093/plankt/7.3.381
Antoine D., Guevel P., Desté J.-F., Bécu G., Louis F., Scott A. J., et al. (2008). The “BOUSSOLE” buoy–a new transparent-to-Swell taut mooring dedicated to marine optics: Design, tests, and performance at Sea. J. Atmos. Ocean. Technol. 25, 968–989. doi: 10.1175/2007JTECHO563.1
Barbieux M., Uitz J., Bricaud A., Organelli E., Poteau A., Schmechtig C., et al. (2018). Assessing the variability in the relationship between the particulate backscattering coefficient and the chlorophyll a concentration from a global biogeochemical-argo database. J. Geophys. Res. Ocean. 123, 1229–1250. doi: 10.1002/2017JC013030
Barbieux M., Uitz J., Gentili B., Pasqueron de Fommervault O., Mignot A., Poteau A., et al. (2019). Bio-optical characterization of subsurface chlorophyll maxima in the Mediterranean Sea from a biogeochemical-argo float database. Biogeosciences 16, 1321–1342. doi: 10.5194/bg-16-1321-2019
Behrenfeld M. J., Westberry T. K., Boss E. S., O’Malley R. T., Siegel D. A., Wiggert J. D., et al. (2009). Satellite-detected fluorescence reveals global physiology of ocean phytoplankton. Biogeosciences 6, 779–794. doi: 10.5194/bg-6-779-2009
Bidigare R. R., Schofield O., Prézelin B. B. (1989). Influence of zeaxanthin on quantum yield of photosynthesis of synechococcus clone WH7803 (DC2). Mar. Ecol. Prog. Ser. 56, 177–188. https://www.jstor.org/stable/24835757
Bindoff N. L., Cheung W. W. L., Kairo J. G., Arístegui J., Guinder V. A., Hallberg R., et al. (2019). Changing ocean, marine ecosystems, and dependent communities. Mar. Ecosyst. 142, 447–588. doi: 10.1017/9781009157964.007
Bittig H. C., Maurer T. L., Plant J. N., Schmechtig C., Wong A. P. S., Claustre H., et al. (2019). A BGC-argo guide: Planning, deployment, data handling and usage. Front. Mar. Sci. 6. doi: 10.3389/fmars.2019.00502
Booth B. C. (1993). “Estimating cell concentration and biomass of autotrophic plankton using microscopy,” in Handbook of methods in aquatic microbial ecology. Ed. Kemp P. F. (Boca Raton: CRC Press), 199–205.
Boyd P. W., Watson A. J., Law C. S., Abraham E. R., Trull T., Murdoch R., et al. (2000). A mesoscale phytoplankton bloom in the polar southern ocean stimulated by iron fertilization. Nature 407, 695–702. doi: 10.1038/35037500
Brewin R. J. W., Devred E., Sathyendranath S., Lavender S. J., Hardman-Mountford N. J. (2011). Model of phytoplankton absorption based on three size classes. Appl. Opt. AO 50, 4535–4549. doi: 10.1364/AO.50.004535
Brewin R. J. W., Sathyendranath S., Lange P. K., Tilstone G. (2014). Comparison of two methods to derive the size-structure of natural populations of phytoplankton. Deep Sea Res. Part I: Oceanog. Res. Pap. 85, 72–79. doi: 10.1016/j.dsr.2013.11.007
Bricaud A., Allali K., Morel A., Marie D., Veldhuis M. J. W., Partensky F., et al. (1999). Divinyl chlorophyll a-specific absorption coefficients and absorption efficiency factors for prochlorococcus marinus: kinetics of photoacclimation. Mar. Ecol. Prog. Ser. 188, 21–32. doi: 10.3354/meps188021
Bricaud A., Babin M., Claustre H., Ras J., Tièche F. (2010). Light absorption properties and absorption budget of southeast pacific waters. J. Geophys. Res.: Ocean. 115, C08009. doi: 10.1029/2009JC005517
Bricaud A., Babin M., Morel A., Claustre H. (1995). Variability in the chlorophyll-specific absorption coefficients of natural phytoplankton: Analysis and parameterization. J. Geophys. Res. 100, 13321. doi: 10.1029/95JC00463
Bricaud A., Claustre H., Ras J., Oubelkheir K. (2004). Natural variability of phytoplanktonic absorption in oceanic waters: Influence of the size structure of algal populations. J. Geophys. Res.: Ocean. 109, C11010. doi: 10.1029/2004JC002419
Bricaud A., Stramski D. (1990). Spectral absorption coefficients of living phytoplankton and nonalgal biogenous matter: A comparison between the Peru upwelling areaand the Sargasso Sea. Limnol. Oceanog. 35, 562–582. doi: 10.4319/lo.1990.35.3.0562
Briggs N. T., Slade W. H., Boss E., Perry M. J. (2013). Method for estimating mean particle size from high-frequency fluctuations in beam attenuation or scattering measurements. Appl. Opt. AO 52, 6710–6725. doi: 10.1364/AO.52.006710
Bustillos-Guzmán J., Claustre H., Marty C. (1995). Specific phytoplankton signatures and their relationship to hydrographic conditions in the coastal northwestern Mediterranean Sea. Mar. Ecol. Prog. Ser. 124, 247–258. doi: 10.3354/meps124247
Cetinić I., Perry M. J., D’Asaro E., Briggs N., Poulton N., Sieracki M. E., et al. (2015). A simple optical index shows spatial and temporal heterogeneity in phytoplankton community composition during the 2008 north Atlantic bloom experiment. Biogeosciences 12, 2179–2194. doi: 10.5194/bg-12-2179-2015
Chisholm S. W. (1992). “Phytoplankton size,” in Primary productivity and biogeochemical cycles in the Sea. Eds. Falkowski P. G., Woodhead A. D., Vivirit K. (Boston, MA: Springer US), 213–237. doi: 10.1007/978-1-4899-0762-2_12
Ciotti Á.M., Lewis M. R., Cullen J. J. (2002). Assessment of the relationships between dominant cell size in natural phytoplankton communities and the spectral shape of the absorption coefficient. Limnol Oceanogr. 47, 404–417. doi: 10.4319/lo.2002.47.2.0404
Claustre H. (1994). The trophic status of various oceanic provinces as revealed by phytoplankton pigment signatures. Limnol. Oceanog. 39, 1206–1210. doi: 10.4319/lo.1994.39.5.1206
Claustre H., Hooker S. B., Van Heukelem L., Berthon J.-F., Barlow R., Ras J., et al. (2004). An intercomparison of HPLC phytoplankton pigment methods using in situ samples: Application to remote sensing and database activities. Mar. Chem. 85, 41–61. doi: 10.1016/j.marchem.2003.09.002
Claustre H., Johnson K. S., Takeshita Y. (2020). Observing the global ocean with biogeochemical-argo. Annu. Rev. Mar. Sci. 12, 23–48. doi: 10.1146/annurev-marine-010419-010956
Cleveland J. S. (1995). Regional models for phytoplankton absorption as a function of chlorophyll a concentration. J. Geophys. Res.: Ocean. 100, 13333–13344. doi: 10.1029/95JC00532
Cosgrove J., Borowitzka M. A. (2010). “Chlorophyll fluorescence terminology: An introduction,” in Chlorophyll a fluorescence in aquatic sciences: Methods and applications. Eds. Suggett D. J., Prášil O., Borowitzka M. A. (Dordrecht: Springer Netherlands), 1–17. doi: 10.1007/978-90-481-9268-7_1
Cossarini G., Mariotti L., Feudale L., Mignot A., Salon S., Taillandier V., et al. (2019). Towards operational 3D-var assimilation of chlorophyll biogeochemical-argo float data into a biogeochemical model of the Mediterranean Sea. Ocean Model. 133, 112–128. doi: 10.1016/j.ocemod.2018.11.005
Demmig-Adams B. (1990). Carotenoids and photoprotection in plants: A role for the xanthophyll zeaxanthin. Biochim. Biophys. Acta (BBA) - Bioenerget. 1020, 1–24. doi: 10.1016/0005-2728(90)90088-L
D’Ortenzio F., Iudicone D., de Boyer Montegut C., Testor P., Antoine D., Marullo S., et al. (2005). Seasonal variability of the mixed layer depth in the Mediterranean Sea as derived from in situ profiles. Geophys. Res. Lett. 32, L12605. doi: 10.1029/2005GL022463
Dubelaar G. B. J., Jonker R. R. (2000). Flow cytometry as a tool for the study of phytoplankton. Scientia Mar. 64, 135–156. doi: 10.3989/scimar.2000.64n2135
Dubinsky Z., Stambler N. (2009). Photoacclimation processes in phytoplankton: mechanisms, consequences, and applications. Aquat. Microb. Ecol. 56, 163–176. doi: 10.3354/ame01345
Durrieu de Madron X., Guieu C., Sempéré R., Conan P., Cossa D., D’Ortenzio F., et al. (2011). Marine ecosystems’ responses to climatic and anthropogenic forcings in the Mediterranean. Prog. Oceanog. 91, 97–166. doi: 10.1016/j.pocean.2011.02.003
Escoffier N., Bernard C., Hamlaoui S., Groleau A., Catherine A. (2015). Quantifying phytoplankton communities using spectral fluorescence: the effects of species composition and physiological state. J. Plankton Res. 37, 233–247. doi: 10.1093/plankt/fbu085
Falkowski P. G. (1994). The role of phytoplankton photosynthesis in global biogeochemical cycles. Photosynthesis Res. 39, 235–258. doi: 10.1007/BF00014586
Falkowski P., Kolber Z. (1995). Variations in chlorophyll fluorescence yields in phytoplankton in the world oceans. Funct. Plant Biol. 22, 341. doi: 10.1071/PP9950341
Gorbunov M. Y., Falkowski P. G. (2022). Using chlorophyll fluorescence to determine the fate of photons absorbed by phytoplankton in the world’s oceans. Annu. Rev. Mar. Sci. 14, 213–238. doi: 10.1146/annurev-marine-032621-122346
Greg Mitchell B., Kiefer D. A. (1988). Chlorophyll α specific absorption and fluorescence excitation spectra for light-limited phytoplankton. Deep Sea Res. Part A. Oceanog. Res. Pap. 35, 639–663. doi: 10.1016/0198-0149(88)90024-6
Jeffrey S. W., Mantoura R. F. C., Wright S. W. (1997). “International council of scientific unions, and unesco,” in Phytoplankton pigments in oceanography: guidelines to modern methods (Paris: UNESCO Pub).
Johnsen G., Sakshaug E. (2007). Biooptical characteristics of PSII and PSI in 33 species (13 pigment groups) of marine phytoplankton, and the relevance for pulse-amplitude-modulated and fast-repetition-rate fluorometry1. J. Phycol 43 (6): 1236–51. doi: 10.1111/j.1529-8817.2007.00422.x?casa_token=jWEUfuxxVLQAAAAA%3AT_jXMtekOkVqD6xMOl2RGqu0mzOrF1HELQKF6t2XuSLPkPKkvXB2WvRo007g2weS17GYbbhuSX2DfAgZ
Karlson B., Cusack Bresnan C., E. (2010). Microscopic and molecular methods for quantitative phytoplankton analysis (Paris, France: Unesco), 110. doi: 10.25607/OBP-1371
Kiefer D. A., Reynolds R. A. (1992). “Advances in understanding phytoplankton fluorescence and photosynthesis,” in Primary productivity and biogeochemical cycles in the Sea environmental science research. Eds. Falkowski P. G., Woodhead A. D., Vivirito K. (Boston, MA: Springer US), 155–174. doi: 10.1007/978-1-4899-0762-2_10
Lacour L., Ardyna M., Stec K. F., Claustre H., Prieur L., Poteau A., et al. (2017). Unexpected winter phytoplankton blooms in the north Atlantic subpolar gyre. Nat. Geosci. 10, 836–839. doi: 10.1038/ngeo3035
Lavigne H., D’Ortenzio F., Ribera D’Alcalà M., Claustre H., Sauzède R., Gacic M. (2015). On the vertical distribution of the chlorophyll a concentration in the Mediterranean Sea: A basin-scale and seasonal approach. Biogeosciences 12, 5021–5039. doi: 10.5194/bg-12-5021-2015
Lê S., Josse J., Husson F. (2008). FactoMineR: A package for multivariate analysis. J. Stat. Soft. 25 (1), 1–18. doi: 10.18637/jss.v025.i01
Lombard F., Boss E., Waite A. M., Vogt M., Uitz J., Stemmann L., et al. (2019). Globally consistent quantitative observations of planktonic ecosystems. Front. Mar. Sci. 6. doi: 10.3389/fmars.2019.00196
Lorenzen C. J. (1966). A method for the continuous measurement of in vivo chlorophyll concentration. Deep Sea Res. Oceanog. Abstracts 13, 223–227. doi: 10.1016/0011-7471(66)91102-8
Mackey M., Mackey D., Higgins H., and Wright S. (1996). CHEMTAX - a program for estimating class abundances from chemical markers: Application to HPLC measurements of phytoplankton. Marine Ecology Progress Series 144, 265–83. doi: 10.3354/meps144265.
Marty J.-C., Chiavérini J., Pizay M.-D., Avril B. (2002). Seasonal and interannual dynamics of nutrients and phytoplankton pigments in the western Mediterranean Sea at the DYFAMED time-series station, (1991–1999). Deep Sea Res. Part II: Top. Stud. Oceanog. 49, 1965–1985. doi: 10.1016/S0967-0645(02)00022-X
Mayot N., D’Ortenzio F., Uitz J., Gentili B., Ras J., Vellucci V., et al. (2017). Influence of the phytoplankton community structure on the spring and annual primary production in the northwestern Mediterranean Sea. J. Geophys. Res.: Ocean. 122, 9918–9936. doi: 10.1002/2016JC012668
Mignot A., Ferrari R., Claustre H. (2018). Floats with bio-optical sensors reveal what processes trigger the north Atlantic bloom. Nat. Commun. 9, 190. doi: 10.1038/s41467-017-02143-6
Morel A., Maritorena S. (2001). Bio-optical properties of oceanic waters: A reappraisal. J. Geophys. Res.: Ocean. 106, 7163–7180. doi: 10.1029/2000JC000319
Olaizola M., Yamamoto H. Y. (1994). Short-term response of the diadinoxanthin cycle and fluorescence yield to high irradiance in chaetoceros muelleri (bacillariophyceae)1. J. Phycol. 30, 606–612. doi: 10.1111/j.0022-3646.1994.00606.x
Proctor C. W., Roesler C. S. (2010). New insights on obtaining phytoplankton concentration and composition from in situ multispectral chlorophyll fluorescence: In situ phytoplankton composition. Limnol. Oceanog.: Methods 8, 695–708. doi: 10.4319/lom.2010.8.0695
Ras J., Claustre H., Uitz J. (2008). Spatial variability of phytoplankton pigment distributions in the subtropical south pacific ocean: comparison between in situ and predicted data Biogeosciences, 5, 353–69. doi: 10.5194/bg-5-353-2008
Rembauville M., Briggs N., Ardyna M., Uitz J., Catala P., Penkerc’h C., et al. (2017). Plankton assemblage estimated with BGC-argo floats in the southern ocean: Implications for seasonal successions and particle export: PLANKTON ASSEMBLAGE BGC-ARGO. J. Geophys. Res.: Ocean. 122, 8278–8292. doi: 10.1002/2017JC013067
Roemmich D., Alford M. H., Claustre H., Johnson K., King B., Moum J., et al (2019). On the Future of Argo: A Global, Full-Depth, Multi-Disciplinary Array. Frontiers in Marine Science 6. Available at: https://www.frontiersin.org/articles/10.3389/fmars.2019.00439
Roesler C., Uitz J., Claustre H., Boss E., Xing X., Organelli E., et al. (2017). Recommendations for obtaining unbiased chlorophyll estimates from in situ chlorophyll fluorometers: A global analysis of WET labs ECO sensors: Unbiased chlorophyll from in situ fluorometers. Limnol. Oceanog.: Methods 15, 572–585. doi: 10.1002/lom3.10185
Röttgers R., Gehnke S. (2012). Measurement of light absorption by aquatic particles: improvement of the quantitative filter technique by use of an integrating sphere approach. Appl. Opt. AO 51, 1336–1351. doi: 10.1364/AO.51.001336
Roy S., Llewellyn C., Egeland E. S., Johnsen G. (Eds.) (2011). Phytoplankton pigments: Characterization, chemotaxonomy and applications in oceanography (Cambridge: Cambridge University Press). doi: 10.1017/CBO9780511732263
Roy S., Sathyendranath S., Bouman H., Platt T. (2013). The global distribution of phytoplankton size spectrum and size classes from their light-absorption spectra derived from satellite data. Remote Sens. Environ. 139, 185–197. doi: 10.1016/j.rse.2013.08.004
Sammartino M., Di Cicco A., Marullo S., Santoleri R. (2015). Spatio-temporal variability of micro-, nano- and pico-phytoplankton in the Mediterranean Sea from satellite ocean colour data of SeaWiFS. Ocean Sci. 11, 759–778. doi: 10.5194/os-11-759-2015
Sauzède R., Claustre H., Jamet C., Uitz J., Ras J., Mignot A., et al. (2015). Retrieving the vertical distribution of chlorophyll a concentration and phytoplankton community composition from in situ fluorescence profiles: A method based on a neural network with potential for global-scale applications. J. Geophys. Res.: Ocean. 120, 451–470. doi: 10.1002/2014JC010355
Schallenberg C., Strzepek R. F., Bestley S., Wojtasiewicz B., and Trull T. W. (2022). Iron limitation drives high fluorescence/chlorophyll ratios in the southern ocean and points the way to improved phytoplankton biomass estimates from BGC-argo floats. Geophysical Research Letters 49, e2021GL097616. doi: 10.1029/2021GL097616.
Schallenberg C., Strzepek R. F., Schuback N., Clementson L. A., Boyd P. W., Trull T. W. (2020). Diel quenching of southern ocean phytoplankton fluorescence is related to iron limitation. Biogeosciences 17, 793–812. doi: 10.5194/bg-17-793-2020
Schmechtig C., Claustre H., Poteau A., D’Ortenzio F. (2014). Bio-argo quality control manual for the chlorophyll-a concentration. doi: 10.13155/35385
Schuback N., Tortell P. D., Berman-Frank I., Campbell D. A., Ciotti A., Courtecuisse E., et al. (2021). Single-turnover variable chlorophyll fluorescence as a tool for assessing phytoplankton photosynthesis and primary productivity: Opportunities, caveats and recommendations. Front. Mar. Sci. 8. doi: 10.3389/fmars.2021.690607
Six C., Thomas J. C., Brahamsha B., Lemoine Y., Partensky F. (2004). Photophysiology of the marine cyanobacterium synechococcus sp. WH8102, a new model organism. Aquat. Microbial Ecol. 35, 17–29. doi: 10.3354/ame035017
Strzepek R. F., Boyd P. W., Sunda W. G. (2019). Photosynthetic adaptation to low iron, light, and temperature in southern ocean phytoplankton. Proc. Natl. Acad. Sci. 116, 4388–4393. doi: 10.1073/pnas.1810886116
Strzepek R. F., Hunter K. A., Frew R. D., Harrison P. J., Boyd P. W. (2012). Iron-light interactions differ in southern ocean phytoplankton. Limnol. Oceanog. 57, 1182–1200. doi: 10.4319/lo.2012.57.4.1182
Taillandier V., Wagener T., D’Ortenzio F., Mayot N., Legoff H., Ras J., et al. (2018). Hydrography and biogeochemistry dedicated to the Mediterranean BGC-argo network during a cruise with RV <i<Tethys 2</i< in may 2015. Earth Syst. Sci. Data 10, 627–641. doi: 10.5194/essd-10-627-2018
Trombetta T., Vidussi F., Roques C., Scotti M., Mostajir B. (2020). Marine microbial food web networks during phytoplankton bloom and non-bloom periods: Warming favors smaller organism interactions and intensifies trophic cascade. Front. Microbiol. 11. doi: 10.3389/fmicb.2020.502336
Uitz J., Claustre H., Morel A., Hooker S. B. (2006). Vertical distribution of phytoplankton communities in open ocean: An assessment based on surface chlorophyll. J. Geophys. Res. 111, C08005. doi: 10.1029/2005JC003207
Uitz J. U., Huot Y., Bruyant F., Babin M., Claustre H. (2008). Relating phytoplankton photophysiological properties to community structure on large scales. Limnol. Oceanog. 53, 614–630. doi: 10.4319/lo.2008.53.2.0614
Uitz J., Stramski D., Reynolds R. A., Dubranna J. (2015). Assessing phytoplankton community composition from hyperspectral measurements of phytoplankton absorption coefficient and remote-sensing reflectance in open-ocean environments. Remote Sens. Environ. 171, 58–74. doi: 10.1016/j.rse.2015.09.027
Vidussi F., Claustre H., Manca B. B., Luchetta A., Marty J.-C. (2001). Phytoplankton pigment distribution in relation to upper thermocline circulation in the eastern Mediterranean Sea during winter. J. Geophys. Res.: Ocean. 106, 19939–19956. doi: 10.1029/1999JC000308
Xing X., Claustre H., Blain S., D’Ortenzio F., Antoine D., Ras J., et al. (2012). Quenching correction for in vivo chlorophyll fluorescence acquired by autonomous platforms: A case study with instrumented elephant seals in the kerguelen region (Southern ocean): Quenching correction for chlorophyll fluorescence. Limnol. Oceanog.: Methods 10, 483–495. doi: 10.4319/lom.2012.10.483
Keywords: Phytoplankton biomass, in-situ fluorescence, BGC-Argo floats, phytoplankton community composition, chlorophyll-a concentration, phytoplankton absorption
Citation: Petit F, Uitz J, Schmechtig C, Dimier C, Ras J, Poteau A, Golbol M, Vellucci V and Claustre H (2022) Influence of the phytoplankton community composition on the in situ fluorescence signal: Implication for an improved estimation of the chlorophyll-a concentration from BioGeoChemical-Argo profiling floats. Front. Mar. Sci. 9:959131. doi: 10.3389/fmars.2022.959131
Received: 01 June 2022; Accepted: 31 August 2022;
Published: 21 September 2022.
Edited by:
Takafumi Hirata, Hokkaido University, JapanReviewed by:
Eva Álvarez, Istituto Nazionale di Oceanografia e di Geofisica Sperimentale, ItalyAurea Maria Ciotti, Center for Marine Biology, University of São Paulo, Brazil
Copyright © 2022 Petit, Uitz, Schmechtig, Dimier, Ras, Poteau, Golbol, Vellucci and Claustre. This is an open-access article distributed under the terms of the Creative Commons Attribution License (CC BY). The use, distribution or reproduction in other forums is permitted, provided the original author(s) and the copyright owner(s) are credited and that the original publication in this journal is cited, in accordance with accepted academic practice. No use, distribution or reproduction is permitted which does not comply with these terms.
*Correspondence: Flavien Petit, flavien.petit@imev-mer.fr