- 1Department of Animal Ecology & Systematics, Justus Liebig University Giessen, Giessen, Germany
- 2Département de Biologie des Organismes, Université Libre de Bruxelles, Brussels, Belgium
- 3Computational Science Lab, Universiteit van Amsterdam, Amsterdam, Netherlands
- 4Interuniversity Institute of Bioinformatics in Brussels – (IB)2, Brussels, Belgium
- 5Australian Research Council (ARC) Centre of Excellence for Coral Reef Studies, James Cook University, Townsville, QLD, Australia
- 6Sesoko Station, Tropical Biosphere Research Center, University of the Ryukyus, Okinawa, Japan
Morphological characters play an important role in species descriptions and are essential for a better understanding of the function, evolution and plasticity of an organism’s shape. However, in complex-shaped organisms lacking characteristic features that can be used as landmarks, quantifying morphological traits, assessing their intra- and interspecific variation, and subsequently delineating phenotypically distinct groups continue to be problematic. For such organisms, three-dimensional morphological analysis might be a promising approach to differentiate morphogroups and potentially aid the delineation of species boundaries, though identifying informative features remains a challenge. Here, we assessed the potential of 3D-based quantitative morphology to delineate a priori and/or to discriminate a posteriori morphogroups of complex-shaped and taxonomically challenging organisms, such as corals from the morphologically diverse genus Acropora. Using three closely related coral taxa previously delimited using other lines of evidence, we extracted a set of variables derived from triangulated polygon meshes and medial axis skeletons of the 3D models. From the resulting data set, univariate and multivariate analyses of 3D-based variables quantifying overall shape including curvature, branching, and complexity were conducted. Finally, informative feature selection was performed to assess the discriminative power of the selected variables. Results revealed significant interspecific differences in the means of a set of 3D-based variables, highlighting potentially informative characters that provide sufficient resolution to discriminate morphogroups congruent with independent species identification based on other lines of evidence. A combination of representative features, remarkably represented by curvature, yielded measures that assisted in differentiating closely related species despite the overall morphospaces overlap. This study shows that a well-justified combination of 3D-based variables can aid species discrimination in complex-shaped organisms such as corals and that feature screening and selection is useful for achieving sufficient resolution to validate species boundaries. Yet, the significant discriminative power displayed by curvature-related variables and their potential link to functional significance need to be explored further. Integrating informative morphological features with other independent lines of evidence appears therefore a promising way to advance not only taxonomy but also our understanding of morphological variation in complex-shaped organisms.
1 Introduction
The morphological diversity encompassed by the tree of life displays an extraordinary range of forms and shapes. Beyond contributing to characterize the biodiversity of these otherwise “endless forms” (Darwin, 1859), assessing their variation spectrum is key to gaining a better understanding of shape function and evolution (Klingenberg, 2010). Indeed, delimiting groups of individuals based on their morphological resemblance (morphogroups), or more broadly on their phenotypic distinctiveness (phena; sensu Mayr, 1969), has been traditionally the first step in taxonomic approaches and also often a preliminary step for sorting specimens in ecological, physiological, and evolutionary studies (MacLeod, 2002; Pereira et al., 2021). As such, morphology is the tie that connects the samples used for a variety of contemporary approaches, the designated type specimens used for species description, and the placement of extant species in relation to extinct life forms (Budd and Olsson, 2006; Schlick-Steiner et al., 2007; Saraswati and Srinivasan, 2016). Thus, morphological assessments are crucial to disentangle the confused and sometimes obscure categorisation of the diversity of life forms that inhabit the planet (Wheeler, 2005).
Phena, as designated taxonomic units or morphospecies, do not necessarily correspond with taxonomic categories delimited using other criteria (e.g., reciprocal monophyly, reproductive isolation, Mayr, 1969; Dubois, 2011). Indeed, finding out whether two phena are an instance of intraspecific polymorphism (e.g., sexual dimorphism, developmental stages, morphological plasticity) or correspond to two distinct species requires extra information and cannot be deduced from morphological analysis alone (Dayrat, 2005). Besides, the coupling of intraspecific variability and interspecific similarity can hamper the use of morphological features in taxonomically intricate taxa (Sites and Marshall, 2004). Yet, phena can provide primary species hypotheses (PSHs) that can be subjected to validation under a variety of scenarios (e.g., Puillandre et al., 2012).
Different phenetic approaches have been proposed to delineate a priori phena as groups of individuals characterized by intra-group diversity lower than inter-group differences (Sokal, 1986; Jensen, 2009). Such quantitative morphological analyses rely on obtaining a set of comparable measurements from all investigated specimens, which is particularly challenging in the case of complex-shaped organisms (Konglerd et al., 2017). Although traditional morphometric approaches excel at quantifying differences across a wide range of forms in the tree of life (e.g., Cardini, 2003; Migicovsky et al., 2018; Chaplin et al., 2020), they struggle to capture and describe complex geometric structures that are highly variable and lack homologous landmarks or distinctive outlines (Kaandorp, 1999; Kaandorp and Kübler, 2001; Konglerd et al., 2017).
The contrast between the morphological diversity of marine invertebrates and the shortage of informative morphological characters exemplifies many of the challenges faced by morphology-aided categorization in complex-shaped organisms (Filatov et al., 2013; Fontaneto et al., 2015). For instance, morphological plasticity in response to environmental factors such as water flow and light availability in corals can lead to large intraspecific differences in the shape of colonies, hindering unambiguous morphogroups differentiation (Miller, 1994; Todd et al., 2004; Todd, 2008; Paz-García et al., 2015b). Moreover, traditional morphological traits used to delineate coral phena are frequently at odds with molecular analyses (e.g., Forsman et al., 2009; Flot et al., 2011; Keshavmurthy et al., 2013; Erickson et al., 2021), which is particularly evident in species groups with low interspecific morphological differences (e.g., sibling or cryptic species) as well as between recently diverged species (Knowlton, 1993).
In the last decades, substantial progress in three-dimensional (3D) imaging has made it possible to document form and structure of complex-shaped organisms, revolutionizing the way morphological data is collected and analysed (Ziegler et al., 2010; Laforsch et al., 2012). While in the past this was done by hand or extracting data from two-dimensional photos and illustrations, high-throughput techniques such as magnetic resonance imaging (MRI), computed tomography (CT) scanning, structured light scanning, and photogrammetry have made it possible to capture morphology in digital and 3D data sets (e.g., Bythell et al., 2001; Faulwetter et al., 2013; Sigl et al., 2013; Reichert et al., 2016). Alternative descriptors of 3D shape and complexity, such as fractal dimension and alpha shapes, have emerged as potential approaches for quantifying morphology in complex-shaped organisms and structures (Martin-Garin et al., 2007; Reichert et al., 2016; Gardiner et al., 2018; Klinkenbuß et al., 2020; Orbach et al., 2021). Yet, previous frameworks to extract meaningful characters in the absence of identifiable landmarks and characterize phena in complex modular organisms have either gauged only a few variables from 3D-morphological data (e.g., Gutierrez-Heredia et al., 2016; Reichert et al., 2017) or been restricted to two-dimensional analyses (e.g., Reeb et al., 2018). However, in most cases, geometrical complex shapes such as corals can be only represented adequately in three dimensions (Kaandorp and Kübler, 2001; Courtney et al., 2007). Thus, the main objective of this study was to assess the applicability of 3D-morphological analyses to delineate phena among specimens of complex-shaped and taxonomically intricate organisms. For this purpose, specimens from three morphologically similar and closely-related species of Acropora corals, robustly delimited using independent evidence (Ramírez-Portilla et al., 2022), were used as a case study. Here, we specifically aimed to:
1. evaluate 3D features and perform variable selection for a prospective combination of representative characters that support morphogroups discrimination;
2. test whether the morphogroups delineated using 3D-based variables are congruent with species boundaries assessed using other sources of information; and
3. test whether the 3D-morphological analyses enable discrimination between a priori delimited species.
2 Materials and methods
2.1 Experimental design and data set
We assessed the power of 3D quantitative morphology to discriminate morphogroups using skeleton specimens of three closely related tabular Acropora species previously delineated using different lines of evidence (i.e., morphology, breeding trials, and molecular analyses): A. cf. bifurcata (n = 28), A. cf. cytherea (n = 21) and A. aff. hyacinthus (n = 25), hereafter species A, B and C respectively (for further information and comparison to type material see Table 1 at Ramírez-Portilla et al., 2022). Briefly, morphospecies were identified in the field following Veron (2000), particularly using the branch taper (either gradually narrowing or cylindrical) and the radial corallites shape (all labellate either with round, straight or flaring lips, see zoom in branches in Figure 1 in this paper and Figure 33 in Wallace, 1999). Subsequently, multivariate morphological analyses of qualitative and quantitative variables, cross-fertilization experiments, and molecular analyses using target capture and Sanger sequencing were used to identify species boundaries in the data set. For the 3D morphology assessment in this study, we documented a total of 74 skeleton fragments deposited as vouchers at the Sesoko Station, Tropical Biosphere Research Center (TBRC); collected in 2015, 2018, and 2019 from the outer reef south of Sesoko Island (26.6288 North, 127.8622 East, Okinawa, Japan). Documented specimens corresponded to medium-size fragments (min. area 8x8 cm) collected from adult colonies with similar sizes (Supplementary Table S1, photos available in Morphobank Project 4065, http://morphobank.org/permalink/?P4065).
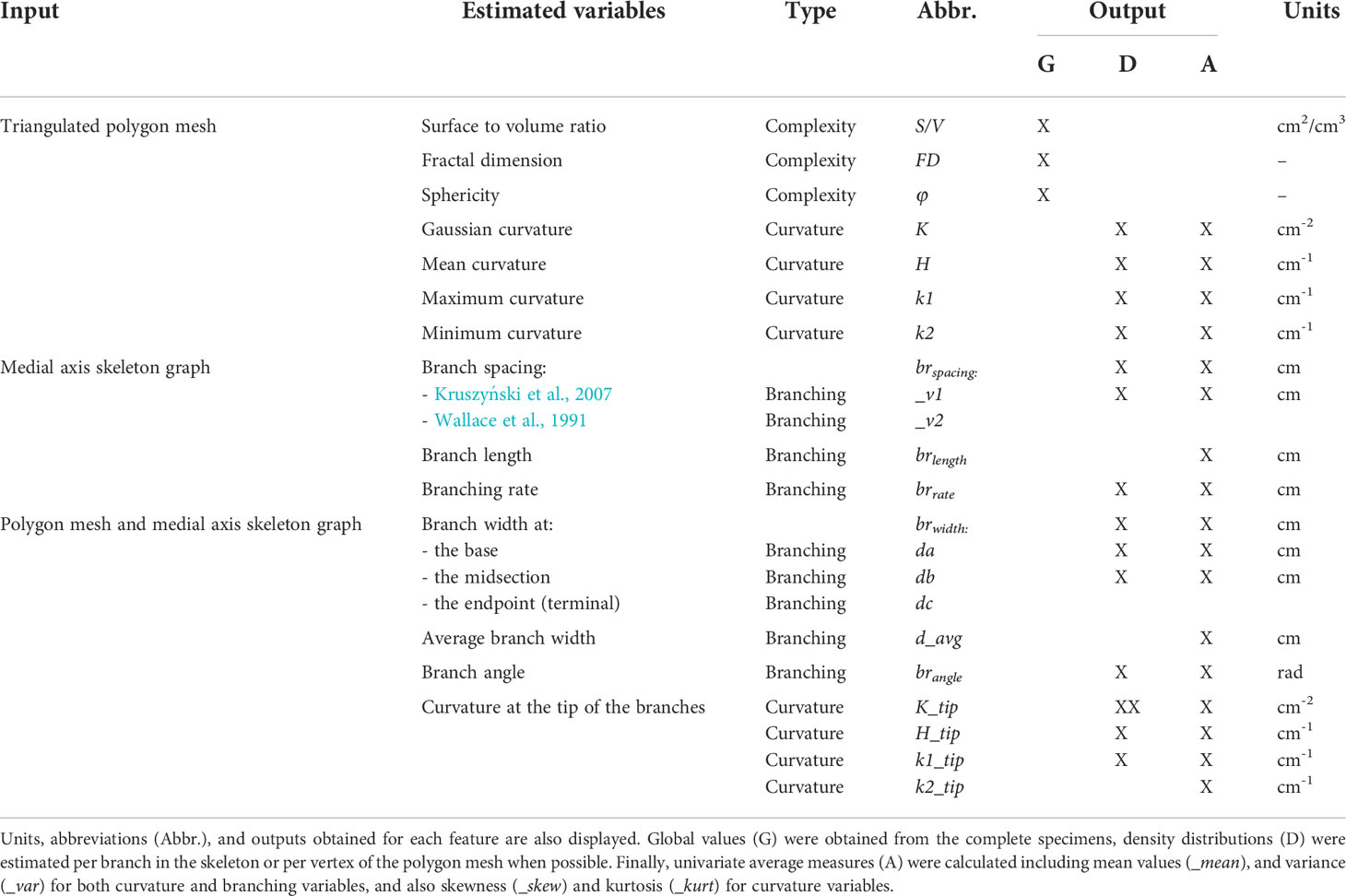
Table 1 Outline of the quantitative morphological variables assessed from the 3D data (triangulated polygon meshes and extracted medial axis skeletons) according to their type: branching, complexity, and curvature.
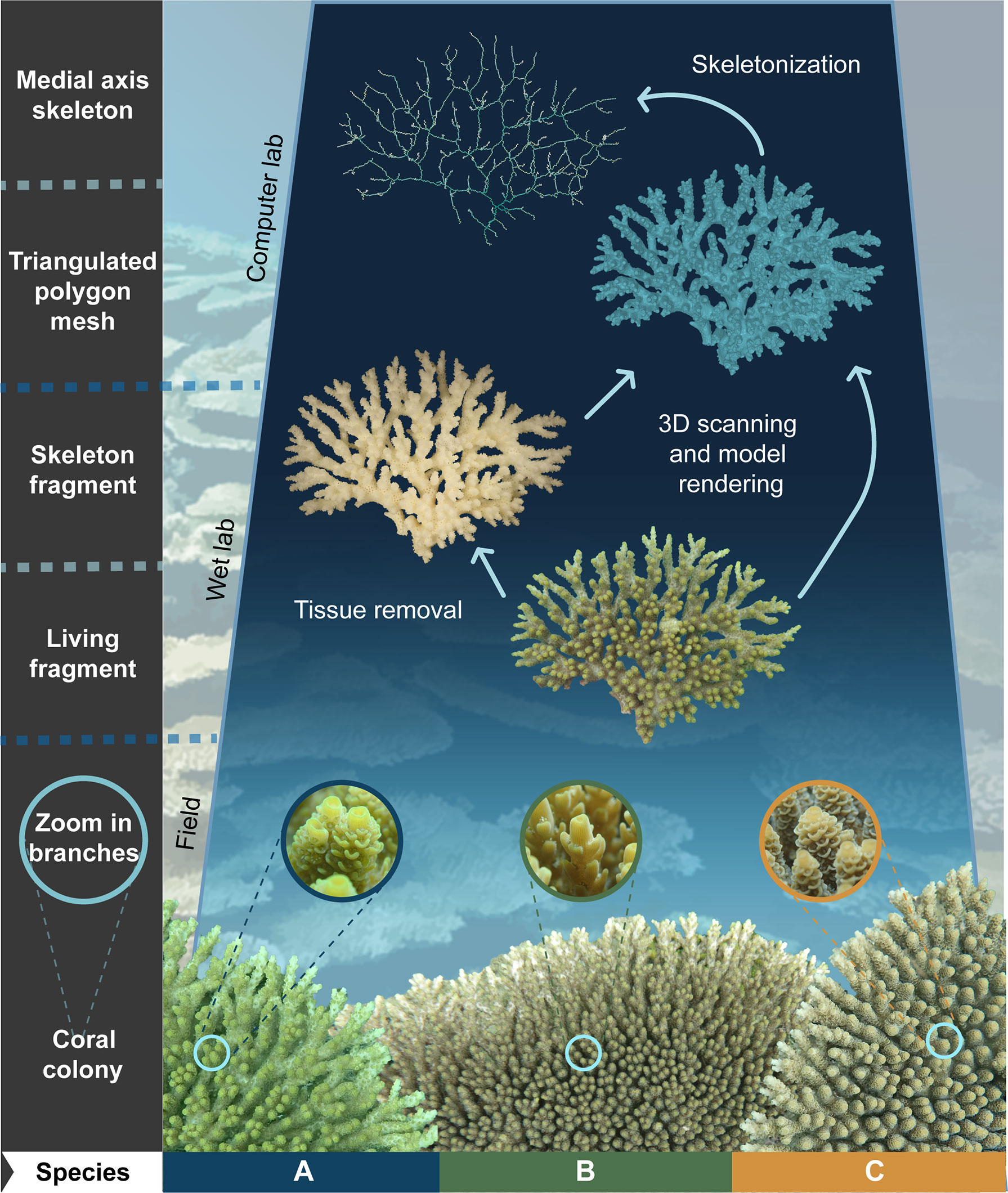
Figure 1 Schematic representation of three-dimensional (3D) model rendering and skeletonization workflow from complex-shaped organisms. Coral morphology can be analysed by 3D scanning either live (Reichert et al., 2016) or voucher skeleton specimens (as in this study; see Wet lab section). Here, three species of tabular Acropora corals (i.e., A, B, and C) identified using diagnostic characters in the field (see Coral colonies and Zoom to the branches) and later confirmed using different lines of evidence (Ramírez-Portilla et al., 2022) were used as a case study (coral photos by A.H. Baird). Downstream processing rendered 3D models as triangulated polygon meshes or medial axis skeletons (see Computer lab section) from which variable types such as curvature, branching, and complexity were estimated (see Table 1, Figure 2, and Supplementary Figure S2).
2.2 Data acquisition, model rendering, and processing
The 3D scanning of the coral fragments was performed using a handheld Artec 3D Space Spider Scanner coupled with the software Artec Studio v10 (Artec 3D, Luxembourg). For a preliminary assessment of the 3D model quality, scanning was completed using the real-time fusion mode for all fragments (Supplementary Figure S1). Following Reichert et al. (2016; 2017), the Artec Studio software was used to render and clean up the 3D models for which fusion was performed with 0.2 mm resolution (Supplementary Materials and Methods). Meshes were then exported as triangulated mesh files (either.stl or.obj; available in Morphobank http://morphobank.org/permalink/?P4205) for downstream analyses derived either from triangulated polygon meshes, medial axis skeleton graphs, or a combination of both (Figure 1 and Supplementary Figure S2).
2.3 Polygon mesh-based estimations
The resulting triangulated polygon meshes were further analysed and visualized using the Visualization Toolkit v9.1.0 (VTK; Schroeder et al., 2006) in Python v3.8 (Van Rossum and Drake, 2009) and the Insight Toolkit v5.1.2 (ITK; Ibáñez et al., 2003) in c++20 (ISO/IEC, 2020). The surface area (SA) and the volume (V) of the specimens were obtained with vtkMassProperties from which the surface-area-to-volume (S/V) ratio and the sphericity (φ) were estimated directly (Supplementary Materials and Methods).
Four common characteristics of surface curvature were estimated for each vertex of the polygon mesh following Meyer et al. (2003). First, a discrete approximation of the Gauss-Bonnet theorem and the Laplace-Beltrami operator were implemented to obtain the Gaussian (K) and mean (H) curvature for all vertices respectively (Supplementary Materials and Methods). The sign of H was then determined based on the direction of the normal vectors, which were obtained using vtkTriangleMeshPointNormals. Finally, the two principal curvatures, maximum curvature (k1) and minimum curvature (k2), were estimated considering that the Gaussian curvature (K) is defined as the product of the two principal curvatures at that location, and the mean curvature (H) corresponds to average of the two principal curvatures (Supplementary Figure S3).
2.4 Medial axis skeleton-derived estimations
To capture the topological branching structure of corals and facilitate the estimation of measures related to this type of morphology, we extracted the medial axis skeleton from the previously rendered 3D models using a voxel thinning algorithm. For this purpose, the polygon mesh was first smoothed using the vtkWindowedSincPolyDataFilter module (iterations: 100, pass-band frequency = 0.005), thereby reducing the details of the surface and potential noise while still maintaining the general shape of the coral specimens. Next, the smoothed mesh was transformed into a binary voxel image (resolution = 0.5mm×0.5mm×0.5mm) using vtkPolyDataToImageStencil (tolerance = 0). Finally, voxel thinning was performed with itkBinaryImageThinningFilter3D (Homann, 2007), an implementation of the algorithm of Lee et al. (1994) that results in single-voxel thin skeletons. The voxel skeletons were then transformed into graphs (G) by translating each voxel to a vertex (v) with coordinates corresponding to the represented location and connected by edges using the method described by Reinders et al. (2000). In this graph, a branch (b) was considered to be the set of neighbouring vertices and edges between two successive junction vertices (with a vertex degree higher than two), or between a junction and a terminal vertex (with a degree of one, Supplementary Materials and Methods).
The branches were then identified from the graph and three different morphological characters were estimated (Supplementary Materials and Methods). Branch length (brlength) was calculated as the sum of all the edge lengths. Branching rate (brrate), or how often a coral branches, was defined as the distance between the first (v0) and last vertex (vN) of the branch. Then, two definitions were used to estimate branch spacing. First, following Kruszyński et al. (2007), branch spacing (brspacing_v1) was defined as the shortest distance between the tip (vT) of the terminal branches and any vertex in the skeleton graph not belonging to the current branch. Finally, following Wallace et al. (1991), a second proxy of branch spacing (brspacing_v2) was defined as the shortest distance between the tip (vT) of a terminal branch and any other vT.
2.5 Polygon mesh and medial axis skeleton graph-based estimations
To obtain information about the branch width (brwidth), once the medial skeleton axis and the smoothed polygon mesh were obtained for each specimen, each vertex of the skeleton graph was associated with a medial thickness parameter (d(v)), which represents the diameter of the branch at v. There, the medial thickness was estimated as twice the distance to the closest point on the smoothed polygon mesh. Following Kruszyński et al. (2007), the medial thickness of the individual vertices was translated to three metrics related to the branch width (i.e., the diameter of a sphere at a certain point of the branch): the width at the base of a branch or the junction vertices (da, a−sphere), the width adjacent to the junction a, towards the midsection of the branch (db, b−sphere), and the terminal width (dc, c−sphere) or medial thickness at the tip of the branches (vT) (Figure 2, upper left). As an additional parameter, the average thickness of each branch (davg) was obtained by averaging the medial thickness of all vertices in the branch. The location of the a−sphere and b−sphere were also used to obtain the angle of the branches (bangle). The angles were obtained for all terminal branches (bT) and were defined as the smallest angle at its associated a−sphere between its b−sphere and the b−sphere of a neighbouring branch.
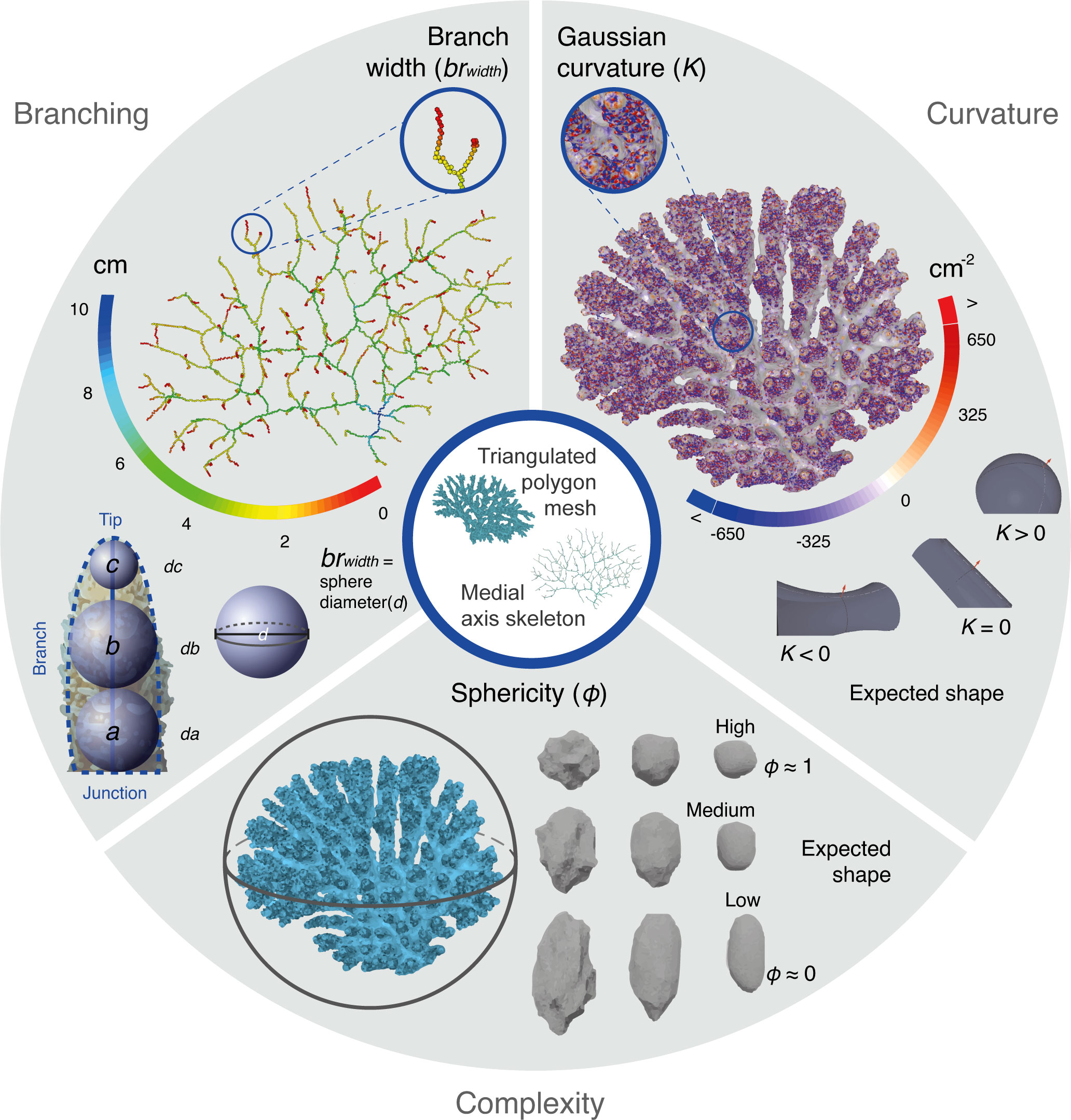
Figure 2 Types of morphological variables assessed from the 3D coral models and representative measurements. Branching estimations (upper left) such as the width (brwidth) at different points between the tip and the branch junction were performed using the diameter of spheres (d) between the medial skeleton axis (solid blue line) and the smoothed polygon mesh (dashed blue line). Measures of the surface curvature (upper right) such as the Gaussian estimations (K) were obtained from the polygon meshes (see expected shapes according to values and Supplementary Figure S3). Complexity shape measures (bottom) were estimated as global values for each coral fragment, like sphericity (φ), which captures the volume compactness by measuring how close is the shape of each coral fragment to a sphere (see expected shapes according to values).
Curvature features were also estimated at the branch tips where it was defined from the subset of the vertices on the polygon surface that were located on the tips of the branches. To identify the branch tip vertices the skeleton graph was used (Supplementary Figure S2). For each bT, a cylinder that had a diameter of da and an axis that followed the direction of the vector between vT and vN/2 was placed on vT together with a plane orthogonal to the cylinder axis. The vertices and faces that were located within the cylinder and exceeded the plane were selected using with the vtkExtractPolyDataGeometry module. From this selection, the set of connected vertices closest to vT (obtained with vtkPolyDataConnectivityFilter) were considered to be the branch tip vertices (vtip) of the polygon mesh. For these vertices, curvature values were calculated for the specimens as previously described for the polygon meshes (see section 2.3).
2.6 3D-based morphological variables assessment and feature screening
Three variable types were estimated from the 3D data set; complexity, curvature, and branching (Figure 2 and Table 1). For complexity variables, global values for each one of the coral fragments were obtained (i.e., a single value per specimen). For curvature and branching variables, estimation methods yielded results per branch in the skeleton or per vertex of the polygon mesh. Therefore, to transform these distributions into univariate measures and obtain the average values, certain features were assessed. For the branch-related measures, outliers (|Z| > 3) of each coral were removed and the mean (_mean) and variance (_var) of the distributions were obtained. For the general curvature measures, values within the 2.5–97.5th percentiles were analysed to obtain the weighted mean (_mean), variance (_var), skewness (_skew) and kurtosis (_kurt) of each distribution. For curvature measures at the branch tips, first the distribution of the number of vtip per bT was analysed to remove branches with too many vtip (Z > 3), as it indicates that reliable estimation of vtip failed. The remaining branch tip vertices were assembled and analysed in a similar fashion as the general curvature distributions.
To provide a quantitative comparison of the estimated morphological variables, both univariate values and distributions were analysed using R v4.1.0 (R Core Team, 2018) through the RStudio console v1.4.1103 (RStudio Team, 2017). The three species previously delineated in this data set (Ramírez-Portilla et al., 2022) were used as a three-level factor for the subsequent analyses.
2.6.1 Variable assessment of global and average values
For assessing differences between species, univariate analysis of variance (ANOVA; α = 0.05) and post-hoc Tukey tests (α = 0.05; stats v4.1.0; R Core Team, 2018) were performed for each variable (see Supplementary Materials and Methods). In addition, bivariate scatter plots and density plots (ggplot2 v3.3.5; Wickham, 2016) of measures with significantly different mean values between the three species were used to assess the morphospaces overlapping.
2.6.2 Variable assessment of density distributions
To weigh the informative value of measures obtained per branch in the skeleton or per vertex of the polygon mesh (D) in contrast to the univariate measures obtained per specimen (see section 2.6.1), probability density functions (pdf) were estimated. Gaussian kernel densities (KD) were estimated using the scipy.stats.kde.gaussian_kde function as implemented in SciPy v1.7.1 (Virtanen et al., 2020), where the bandwidth factor of each pdf was determined using Scott’s rule (Scott, 2015). For curvature-related distributions, the values were weighted by the surface area associated to the vertex of which the curvature values were obtained (Amixed(v)) following Meyer et al. (2003). For calculating branching rate, only branches with a minimum of 4 vertices were taken into account. To compare between species, the mean and standard deviation of the pdf were obtained within each species per step.
To test for significant interspecific differences between the distributions of the variables, ten replicates of the Mann-Whitney U test were performed using a thousand random samples for each variable measurement (scipy.stats.mannwhitneyu function). To sum up the information obtained from these tests, p-values obtained from each of the pairwise comparisons were transformed into integers according to an alpha (α) of 0.05 significance: if p-value > 0.05, then = 1 (i.e., there is a high probability that the samples come from similar distributions); if p-value ≤ 0.05, then = -1 (i.e., there is a high probability that the samples do not come from similar distributions). The integer values of the ten replicates were then added cumulatively to obtain a final value or distribution comparison score (DCS). Finally, heatmaps with samples reorganized according to the similarity displayed in the DCS pairwise comparisons using hierarchical clustering (Ward algorithm, heatmap3 v1.1.9; Zhao et al., 2021) were obtained for each of the variables and three different sets of them: all the variables (n = 15), curvature variables (n = 8), and branching variables (n = 7).
2.6.3 Screening of 3D-based morphological features
To perform feature screening for a prospective combination of representative characters that support interspecific discrimination, variables that exhibited significant differences in the ANOVA and in at least two-species comparisons in the post-hoc Tukey test were included in a “preliminary selected” subset (see Supplementary Figure S4 for a complete flow chart). Correlation between the preliminary selected variables was evaluated using Pearson coefficients (Hmisc v4.5-0; Harrell and Dupont, 2021) and a correlation plot (psych v2.1.6; Revelle, 2021). Box plots (ggplot2 v3.3.5; Wickham, 2016) were used to examine this subset of variables.
2.7 Contrasting 3D-based morphogroups and species boundaries assessed using other sources of information
To inspect clustering using the complete set of variables and the preliminary selected subset, the most likely number of groups was estimated according to 30 different indices (NbClust v3.0; Charrad et al., 2014), followed by a hierarchical clustering analysis (HCA; cluster v2.1.2; Maechler et al., 2021) using Euclidean distance and three different clustering methods (i.e., Ward, complete, and average) in which p-values were calculated via multiscale bootstrap resampling (pvclust v2.2-0; Suzuki et al., 2019).
A principal component analysis (PCA) was also performed to evaluate the ordination of the subset (stats v4.1.0; R Core Team, 2018). For this purpose, unbiased feature selection was performed using Gaussian model-based clustering (clustvarsel v2.3.4; Scrucca and Raftery, 2018) according to the Bayesian information criterion (BIC). Briefly, a set of variables that best discriminated groups using normal mixture models (NMMs) without a priori information was defined using the greedy algorithm both in forward and backward directions (Raftery and Dean, 2006; Scrucca, 2010). This set of variables was then used to reduce the dimensionality of the data using a PCA (Supplementary Figure S4).
Congruence between morphogroups discriminated using these multivariate approaches were contrasted to the three species previously delineated in this data set by mapping each coral specimen to its corresponding taxonomic assignment in each of the analyses (Ramírez-Portilla et al., 2022).
2.8 Discrimination of a priori delimited species by 3D-morphological analyses
The discriminative potential of the 3D-based variables was gauged by removing highly correlated features from the complete variable subset according to their variance inflation factor (VIF < 10; usdm v1.1-18; Naimi et al., 2014) to perform a multivariate analysis of variance (MANOVA; stats v4.1.0; R Core Team, 2018), and a linear discriminant analysis (LDA) with the maximum likelihood (ML) estimator method (MASS v7.3-54; Venables and Ripley, 2002). The accuracy of the discriminant approach was assessed by randomly partitioning the data set in a training (n = 50, 67.6% of specimens) and testing (n = 24, 32.4% of specimens) subsets and calculating the corresponding prediction accuracy tables or confusion matrices.
3 Results
3.1 3D-based morphological variables
Overall, 53 univariate variables from one of three types (complexity, curvature, and branching, Figure 2) were estimated from the data rendered by the 3D models of the 74 coral fragments (Table 1). For each specimen, a single measure of its surface to volume ratio (S/V), fractal dimension (FD), and sphericity (φ) captured the geometric complexity of its colony shape, the irregularity of its surface, and the compactness of its volume (complexity variables). Contrastingly, average values per branch or branch tip either estimated traits such as spacing, length, width, and angle (branching) or characterized the topological concavity/convexity of coral surfaces (curvature). A total of 19 variables were derived from the polygon meshes, 8 from the medial axis skeletons, and 26 using both the polygon meshes and the medial axis skeletons. In addition, probability density functions using kernel estimates were obtained for 15 of these variables: 8 curvature variables and 7 branching variables (Table 1). Forty-one of the univariate variables did not conform one or both of the assumptions of normality and were subsequently transformed (Supplementary Table S2). Four variables were removed from downstream analyses as they did not conform either to the normality or the homogeneity of variance assumption, even after transformation (i.e., K_tip_mean, K_tip_skew, k2_tip_mean, and k2_tip_skew).
3.2 Phenotypic differences in central tendencies of 3D-based variables
To test the potential of the variables for species-level differentiation, both univariate values (i.e., global and average) and Kernel density (KD) distributions of the 3D-estimated data were examined. Using an analysis of variance (ANOVA) for univariate values, significant interspecific differences were found in the means of 42 variables when the three species previously delineated in this data set were used as a three-level factor (p-value < 0.05, df = 2; Supplementary Table S3). Further exploration using post-hoc Tukey tests (p-value < 0.05; Supplementary Table S4) indicated differences between the means of all species in 10 of the characters and between at least two pairs of species in 21 cases. In summary, more than half of the variables derived from the univariate values exhibited significant differences in the ANOVA in at least two-species comparisons in one of the two-sample tests (n = 29; preliminary selected subset).
Although no complexity or branching variable could statistically differentiate the means of all three species, at least 50% of the branching variables could differentiate two species (9/18 according to the post-hoc test). Likewise, significant differences in two to three interspecific comparisons were detected in 23 of the 33 curvature variables. In addition, although the interspecific morphospaces tended to overlap, the probability density profiles of each species were visibly distinct for most univariate variables with significant differences (Figure 3 and Supplementary Figure S5). Overall, the pair of species for which more significant differences were found in the central tendencies was A vs. C, with 64% of the comparisons with p-values < α in contrast to 47% between A vs. B and 42% between B vs. C. A high degree of correlation was found between most of these variables (Supplementary Table S5 and Supplementary Figure S8), which mainly corresponded to curvature features (20 curvature and 9 branching variables).
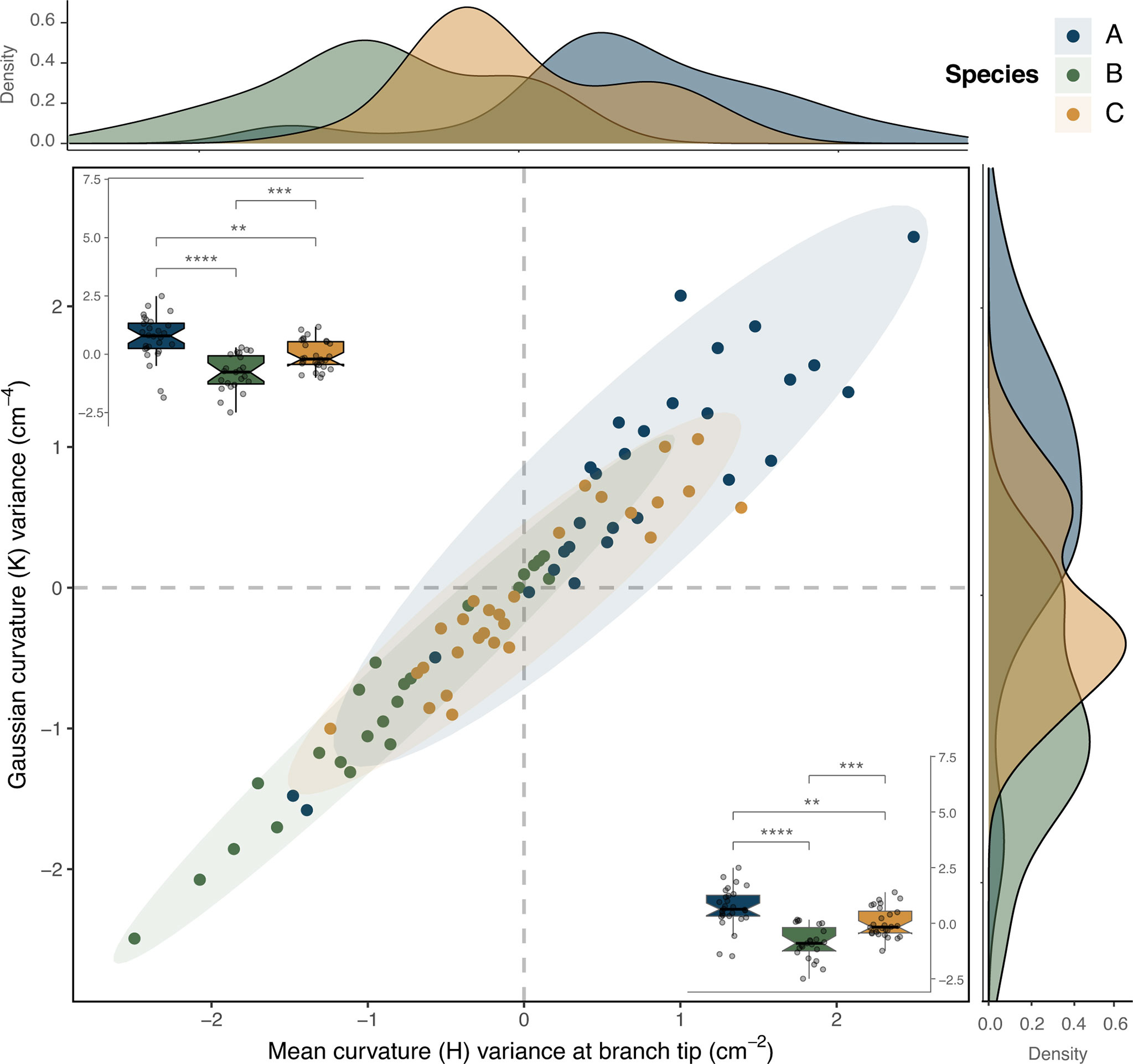
Figure 3 Comparison of two correlated curvature variables that displayed significant differences between the three species (ANOVA and post-hoc Tukey tests, α = 0.05), but where the species morphospaces overlapped: mean curvature variance at branch tip vs. Gaussian curvature variance (H_tip_var vs. K_var). In the center, a scatter plot depicts the two variables, correlation (Pearson correlation coefficient ρ = 0.96), along with their density distributions along each axis. Box plots in each corner display the significant differences in the mean values of the variables according to pairwise interspecific comparisons (p-values significance: **≤ 0.01, ***≤ 0.001, ****≤ 0.0001).
The kernel densities (KD) analysis did not reveal significant interspecific differentiation in the distribution profiles, contrasting with the univariate variables results obtained. Instead, a high degree of overlap was apparent in the distributions of most of the 3D-based morphological variables (Figure 4A and Supplementary Figure S6). The cumulative analysis of the distribution comparison scores (DCS) based on the Mann-Whitney U tests of all variables and curvature variables was able to discriminate two morphogroups (Supplementary Figure S7A and Supplementary Figure S7C, respectively). However, among the individual heatmaps plotted for each variable, there was a considerable degree of clustering between samples of the same species when assessing interspecific differences using the minimum curvature (k2) distributions (Figure 4B). Here, three clusters were observed, each comprising mainly individuals of one of the three species in the data set (from top to bottom: cluster 1 = 61.90% of species B, cluster 2 = 56% of species C, cluster 3 = 82.14% of species A).
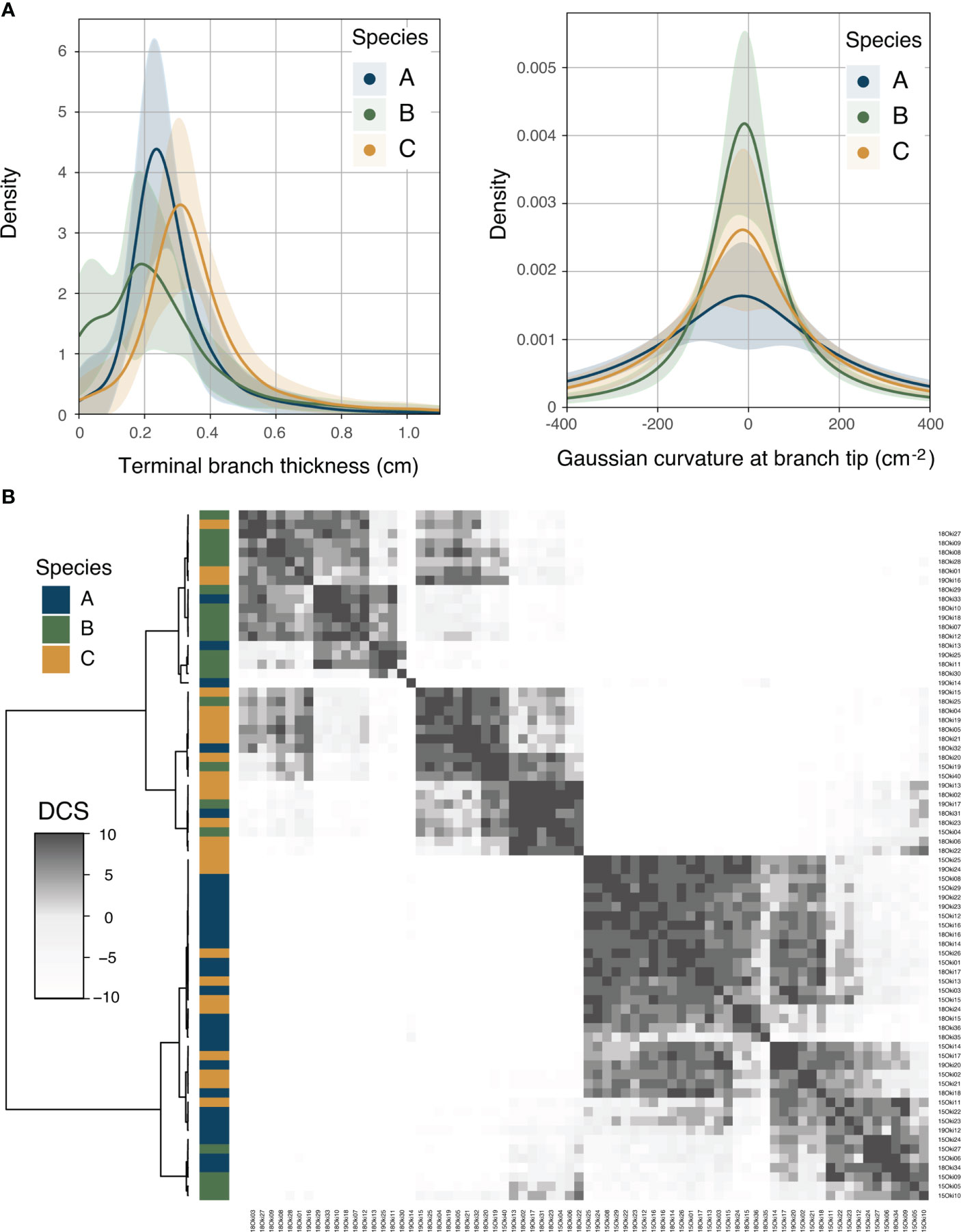
Figure 4 Kernel density distribution analyses. (A) Probability density functions of the terminal branch thickness (dc) and the Gaussian curvature at branch tip (K_tip) for each of the species. (B) Heatmap depicting recoded and summarized p-values obtained from two-sample Mann-Whitney U tests for minimum curvature (k2). Samples were re-organized using hierarchical clustering according to similarity in pairwise comparisons, calculated as the distribution comparison score (DCS) or the cumulative value of similarity according to the p-value significance cut-off (α = 0.05).
3.3 Congruence between morphogroups and previously delineated species boundaries
Despite the overall significant differentiation in central tendency of the set of 3D-based morphological features (see section 3.2 and Supplementary Figure S9), none of the clusters identified by the exploratory hierarchical clustering analysis (HCA) were entirely congruent with the species delineation achieved using other lines of evidence (Supplementary Figure S10, see Ramírez-Portilla et al., 2022). Likewise, when feature selection for Gaussian model-based clustering allowed finding the optimal subset of features containing information, only two morphogroups were identified (Supplementary Table S6). Consequently, the ordination recovered by the principal component analysis (PCA), based on such feature selection methodology, showed a considerable degree of overlap between the morphospaces as defined by the reduced dimensions considered in this analysis (Supplementary Figure S11).
3.4 Potential of 3D-morphological analyses to discriminate between a priori delimited species
From the 21 variables that did not present collinearity (Variance Inflation Factor (VIF) < 10; Supplementary Table S7), 10 were also present in the preliminary selected subset and showed significant interspecific differentiation (MANOVA; Supplementary Table S8). The linear discriminant analysis (LDA) using these variables was able to distinguish three groups that matched the previously supported species delimitation with 97.30% of accuracy (Figure 5; scatter plot) and was able to predict correctly at least 75% of the observations once the model was trained and later tested (Supplementary Table S9). Variables such as the skewness of the Gaussian curvature (K_skew), the mean curvature (H_mean), and the branch angle (b_angle_mean) were the ones that mainly contributed to the discrimination according to the ordination coefficients of each linear component (Figure 5, bar plots).
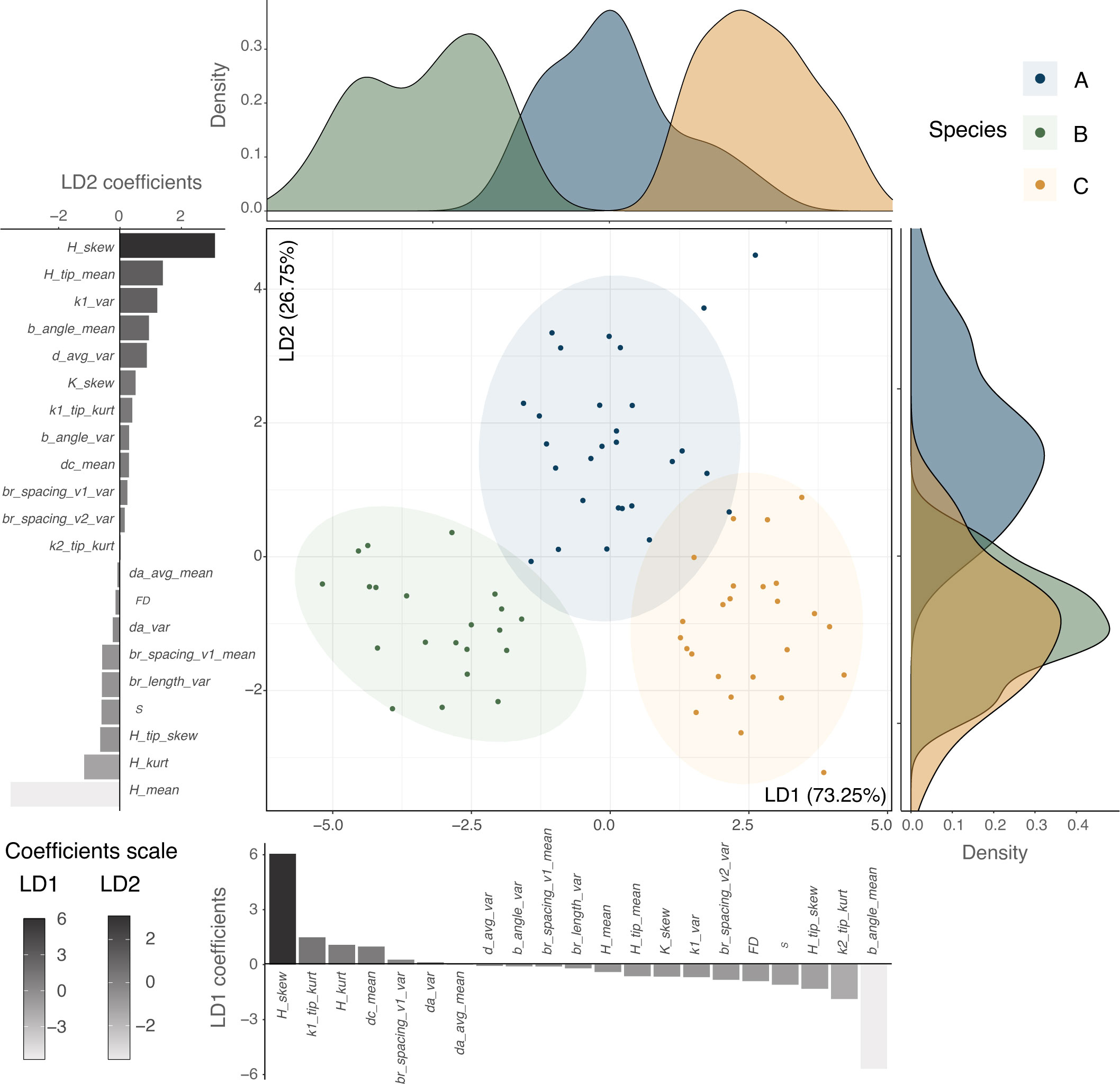
Figure 5 Discriminant multivariate analysis using a subset of variables. Linear discriminant analysis (LDA) using the variables that present no collinearity (Variance Inflation Factor (VIF) < 10, 21 variables) displaying the percentage of trace for each main discriminant. Distribution densities for each one of the linear discriminants are plotted along the axes, along with the scaling coefficients for each variable.
4 Discussion
In this study, we assessed the applicability of 3D-based variables derived from polygon meshes and medial axis skeletons to discriminate morphogroups and potentially support the delineation of species boundaries in complex-shaped and taxonomically intricate marine organisms. For this purpose, we first evaluated the interspecific morphological differentiation rendered by the 3D variables and performed feature selection for a prospective combination of representative characters that support discrimination of phena. Later, we tested whether the morphogroups delineated using 3D-morphological analysis of these variables were congruent with species boundaries assessed using other sources of information and/or whether the 3D-morphological analyses enabled to discriminate between a priori delimited species. Although we used coral skeletons as starting material, previous studies suggested the feasibility of applying our 3D methodology to living specimens, as a minimally invasive method to perform morphological assessments (Figure 1).
4.1 Interspecific morphological differentiation achieved by 3D-based variables
While evaluating the performance of individual 3D variables, both univariate and multivariate analyses were able to identify 3D-based morphological features with significant differences between a priori delimited species. This trend was particularly evident when using curvature variables, which showed differentiation in their central tendencies in most pairwise species comparisons (Supplementary Tables S3, S4), in discriminant analysis (Figure 5), and partly in comparisons between Kernel density distributions (Supplementary Figure S6 and Supplementary Figure S7C). Indeed, significant differences in attributes such as the skewness, kurtosis, and mean values of these variables suggest that they attain sufficient resolution to capture the morphological differences between the specimens of the three complex-shaped coral taxa used here in for validation. By enabling the characterization of surface profiles and owing to the relationship between curvature variables and functional traits (Hyde et al., 1997; Ankhelyi et al., 2018), these results suggest that the estimation of curvatures holds promise for improving our understanding of the relationship between morphology and potentially specific ecological traits.
Contrastingly, analyses of branch-related variables did not provide enough resolution at the species level, likely due to the high similarity between the branching patterns of these closely related taxa (Wallace, 1999). Although only branches with a minimum of four vertices were taken into account to reduce the likelihood of including spurious ones in the estimation of branching variables, these features seemed to be highly variable within species and individuals (Supplementary Figure S7). These results suggest that branch-related variables are taxonomically uninformative in this particular case, as it has been observed that species-specific patterns can emerge when comparing such variables between more distantly related taxa (Kaandorp, 1999). Besides, estimating branching variables can be more relevant to understand the function, evolution, and plasticity of an organism’s shape, particularly when studying marine taxa and their response to environmental fluctuations (Kaandorp et al., 2003; Kaandorp et al., 2005; Chindapol et al., 2013; Paz-García et al., 2015a).
Overall, analyses based on global and average univariate variables exhibited morphological differentiation consistent with a priori delineated species (Supplementary Figures S5, S9). In contrast, most non-averaged density distribution analyses did not display congruent discrimination patterns (Figure 4 and Supplementary Figure S7A), seemingly due to the high intraspecific variation and consequent overlap of the probability density functions between a priori delineated species (Supplementary Figure S6). These trends can be related to the different estimations performed in each case. The univariate values rely on average values obtained per specimen, while the probability density distributions were estimated per branch in the skeleton or per-vertex of the polygon mesh using kernel densities. Thus, since kernel densities exhibit high correspondence to data (Pradlwarter and Schuëller, 2008), they can both contain a wealth of information and display a wide range of variance that can potentially conceal the main species-specific trends in quantitative phenotypic data. Moreover, in the case of complex-shaped organisms such as the tabular Acropora corals used in this study, the variation of probability density functions can be related to the influence that shape complexity exerts on scanning reproducibility (Bythell et al., 2001). Despite the efforts to avoid the effect of self-shading (Supplementary Materials and Methods and Supplementary Figure S1), it has been observed in previous studies that the coefficient of variation between iterative scans can increase in branching corals due to a higher rate of potentially overlapping structures (Reichert et al., 2016). As a result, the shape complexity of the coral specimens could have affected the variability of the observed data.
4.2 Discriminative power of selected 3D variables
Broadly, species delimitation approaches can be differentiated into validation or discovery tools according to whether or not the samples are partitioned into taxonomic categories before performing the analysis (Carstens et al., 2013). In this study, results showed that a well-justified combination of novel 3D-based variables can aid discrimination of morphogroups of irregularly shaped organisms when based on a priori assignment of samples to categories (Ence and Carstens, 2011). In comparison to the quantitative morphological characters previously assessed from the same set of specimens by Ramírez-Portilla et al. (2022), the 3D-based variables evaluated in the current study were able to discriminate three phena congruent with other species delimitation approaches with higher overall accuracy (Figure 5 and Supplementary Table S10; 97.30% in this study vs. 94.94% in the previous). However, when morphogroups were delineated without a priori information in this study (Supplementary Figures S9–S11 and Supplementary Table S6), they were not congruent with species boundaries assessed using other sources of information. The high phenotypic heterogeneity detected within the a priori delineated species (particularly B and C) using density distributions (Supplementary Figure S5 and Supplementary Table S10) could have hampered the unambiguous and unbiased delimitation of three phena congruent with the species boundaries previously delineated using other lines of evidence (see section 4.4).
Although these results may seem paradoxical given the significant interspecific differences found using 3D-derived variables (see section 4.1), phenotypic differentiation in central tendencies between species defined a priori does not necessarily count as evidence of species boundaries when assessed in light of evolutionary theory (Luckow, 1995; Zapata and Jiménez, 2012; Cadena et al., 2018; Cadena and Zapata, 2021). Instead, distinct distributions of phenotypic characters (e.g., those derived from fitting quantitative data to NMMs) can constitute support for species hypotheses as long as they do not result from intraspecific polymorphisms (e.g., González-Espinosa et al., 2018) or morphological plasticity (e.g., Paz-García et al., 2015a). Here, this trend only became evident once the features that yielded information congruent with a priori species boundaries were selected and used to perform discriminant analyses (Figure 5 and Supplementary Tables S9, S10).
4.3 Potential of feature selection to improve species discrimination
The quandary of feature selection in multidimensional data sets is often the limiting factor for extending the applicability of approaches such as 3D-derived variables to a wider variety of organisms (Poon et al., 2013). Certainly, many issues in detecting species boundaries from morphological and phenotypic analyses derive from the potential exclusion of important characters during dimensionality reduction (Cadena et al., 2018). Here, given the large number of variables derived from the 3D analyses and the fact that not all of them provided discriminative and non-redundant information (Supplementary Tables S3–S7), the process of feature screening proved to be key to selecting features used to discriminate between a priori delimited species (Ramírez-Portilla et al., 2022). Feature screening and selection, however, would substantially rely on the overall morphology of the studied organisms. Assessing branching features, for example, would be inadequate for describing the 3D morphology of massive or encrusting growth forms.
Although the morphospaces overlapped when performing bivariate comparisons and other multivariate graphical representations (Figure 3 and Supplementary Figure S5), linear combinations of features after variable screening were useful to identify potentially informative characters and a combination of them that enabled discrimination of three morphogroups congruent with the species delineated a priori in the data set (Figure 5). These results support the notion that not only technological advances in 3D data acquisition and model rendering, but also feature screening and selection actually provide prospective variables to quantify morphology and discriminate groups (Valcárcel and Vargas, 2010), particularly of complex-shaped organisms lacking traditional landmarks.
Regardless, the methodology used here to estimate morphological variables from the 3D models, can be applied to understand a wider variety of phenomena such as morphological plasticity, development, and environmental effects on shape and biodiversity. Therefore, the approaches implemented in this study do not only intend to inform taxonomy, but also to provide tools that can support evolutionary, ecological, and biomonitoring aims to characterize and understand form in complex-shaped taxa in forthcoming studies.
4.4 Limitations
Independent lines of evidence that previously delineated taxonomic units in the data set (i.e., morphology, breeding trials, and molecular analyses) robustly supported the identification of three species (Ramírez-Portilla et al., 2022). Although the 3D-based variables assessed here provided enough power to discriminate morphogroups congruent with such species boundaries delineation, it was not able to delimitate the same three groups when unbiased clustering and feature selection were performed. Only two clusters or components were identified and supported by the multivariate analyses (Supplementary Figures S9, S10), even after a set of variables that best discriminated groups using normal mixture models (NMMs) without a priori information was employed (Supplementary Table S6). In this regard, the results obtained from the density distributions suggest that the heterogeneity of the phenotypic variation detected by 3D-morphological analyses within some of the species could have confounded the identification of components in the mixture (Supplementary Figure S5 and Supplementary Table S10). This would be consistent with the close ties between intraspecific variability and interspecific similarity that have hampered the widespread use of morphological features to delineate taxonomically intricate taxa (Sites and Marshall, 2004), particularly in speciose groups such as the coral genus Acropora where both high morphological intraspecific variability and interspecific similarity, particularly between closely related species, has been reported (Wallace and Willis, 1994; Wallace, 1999).
Alternatively, the scanning quality achieved in the present study could have hindered the potential of species-level delimitation given that important differences in the microstructure could be masked by the technical resolution of the underlying 3D mesh (Gutierrez-Heredia et al., 2016; Reichert et al., 2017). Indeed, features at micromorphological level, such as corallite shape and dimension, have been deemed crucial skeletal characters for discriminating between complex-shaped coral species like those of the genus Acropora (Wallace, 1999; Wolstenholme et al., 2003; Ramírez-Portilla et al., 2022). The potential masking of these features and the effect of the sample sizes used for validation, which in several cases were lower than n = 10 (Finch and Schneider, 2006), could explain the relatively low prediction accuracy achieved in this study when randomly partitioning the data set in training and testing subsets (Supplementary Tables S9, S10). These results suggest that further refinements of 3D-morphological analyses, such as increased precision and reduced errors, might even enable a priori delimitation of phena rather than a posteriori confirmation. For instance, the integration of 3D models from structured light scanning or photogrammetric approaches with high-resolution 3D methodologies such as CT scanning could solve some of the present accuracy issues (Laforsch et al., 2008; Naumann et al., 2009; Veal et al., 2010; Gutiérrez-Heredia et al., 2015; Aston et al., 2022). In the meantime, our results support the discriminative value of implementing 3D-based variables either as hypotheses testing or validation approaches rather than discovery ones.
4.5 Future perspectives
Progress in the development of methods to delineate species using morphological data is urgently needed, particularly to improve modelling of phenotypic variation in agreement with evolutionary theory (Cadena and Zapata, 2021). Due to the potential for morphological plasticity of marine taxa such as corals (Todd, 2008), assessing the discriminative power of 3D- morphological variables to distinguish species throughout the environmental ranges they occupy ought to be explored further, particularly for species with relatively wide distributions where geographical differences can mislead morphology-based species delimitation (Fukami et al., 2004; Forsman et al., 2015). Moreover, the link between 3D-based phenotypic variables and their biological, ecological, and functional significance need to be addressed in future studies that will not only intend to assess interspecific variations in morphology and their taxonomic relevance, but also their potential role in speciation and adaptation, particularly for complex-shaped organisms such as corals (Zawada et al., 2019a; Zawada et al., 2019b; Torres-Pulliza et al., 2020; Aston et al., 2022; Siqueira et al., 2022), which inhabit one of the ecosystems most threatened by climate and anthropogenic disturbances (Hughes et al., 2017; Hughes et al., 2018).
5 Conclusions
Morphological data rendered by 3D scanning approaches in this study showed great potential for discriminating phena among complex-shaped organisms. Curvature features were most prominent in differentiating morphogroups congruent with species boundaries supported by independent evidence. Yet, variable screening and selection proved key to providing sufficient resolution for discriminating closely related species that overlap in ecological and morphological traits. Although our methodology was assessed using coral species as model organisms, the approaches outlined here are in principle applicable to a wide variety of irregular and complex-shaped plant and animal taxa for which 3D data can be readily obtained. However, variables derived from 3D-morphological approaches can complement other lines of evidence but not substitute them when delineating species boundaries within an integrative framework. Ultimately, combining informative quantitative morphological features with other independent lines of evidence will advance our understanding of morphological variation in complex-shaped life forms.
Data availability statement
Data and code can be found in the GitHub repository https://github.com/catalinarp/Coral3Dmorphomeasures. In addition, the analysed triangulated polygon meshes of the studied corals specimens are available at Morphobank Project 4205 (http://morphobank.org/permalink/?P4205).
Author contributions
J-FF, JK, CR-P, and TW conceived the ideas behind the manuscript; AHB, J-FF, SH, CR-P, and FS collected the data; IMB, RB, JK, and CR-P analysed the data; CR-P and IMB led the writing of the manuscript. All authors contributed critically to the drafts and gave final approval for publication.
Funding
This work was partially supported by the Collaborative Research of the Tropical Biosphere Research Center – TBRC, University of the Ryukyus to AB. CR-P was supported by the Fonds de la Recherche Scientifique – FNRS via an “ASP” PhD fellowship (n° 1.A.835.18F) travel grants, and Fonds David et Alice Van Buuren (Fondattion Jaumotte-Demoulin). TW received support from the German Research Foundation – DFG (WI1902/14-1). AHB was funded by the Australian Research Council – ARC Centre of Excellence for Coral Reef Studies (Programme CE140100020) and the Japan Society for the Promotion of Science – JSPS Short Term Fellowship (S-15086). J-FF was supported by the Fonds de la Recherche Scientifique – FNRS under CDR Grant n°J.0272.17, by the Fédération Wallonie-Bruxelles via an ARC grant, as well as by a Fond d’Encouragement à la Recherche (FER) grant of the Université libre de Bruxelles (ULB) for the purchase of 3D scanning equipment.
Acknowledgments
We are grateful to B. Lust and colleagues at the Sesoko Research Station (TBRC) for the assistance provided in the field. We would also like to thank J. Reichert and colleagues at the Systematics and Biodiversity Lab at Justus Liebig University Giessen for sharing their expertise on 3D scanning. The support provided by H.-L. Guillaume and A. Schenkel from PANORAMA at the ULB with 3D imaging software and by C. Kastally from the University of Helsinki with R scripts was also greatly appreciated. Furthermore, we want to thank the reviewers for their insightful comments on the manuscript. Specimens used for this study were previously collected under Okinawa Prefecture permits No. 27-28 (2015), No. 30-25 (2018), and No. 31-26 (2019).
Conflict of interest
The authors declare that the research was conducted in the absence of any commercial or financial relationships that could be construed as a potential conflict of interest.
Publisher’s note
All claims expressed in this article are solely those of the authors and do not necessarily represent those of their affiliated organizations, or those of the publisher, the editors and the reviewers. Any product that may be evaluated in this article, or claim that may be made by its manufacturer, is not guaranteed or endorsed by the publisher.
Supplementary material
The Supplementary Material for this article can be found online at: https://www.frontiersin.org/articles/10.3389/fmars.2022.955582/full#supplementary-material
References
Ankhelyi M. V., Wainwright D. K., Lauder G. V. (2018). Diversity of dermal denticle structure in sharks: Skin surface roughness and three-dimensional morphology. J. Morphol. 279, 1132–1154. doi: 10.1002/jmor.20836
Aston E. A., Duce S., Hoey A. S., Ferrari R. (2022). A protocol for extracting structural metrics from 3D reconstructions of corals. Front. Mar. Sci. 9. doi: 10.3389/fmars.2022.854395
Budd G. E., Olsson L. (2006). Editorial: A renaissance for evolutionary morphology. Acta Zool. 88, 1–1. doi: 10.1111/j.1463-6395.2007.00262.x
Bythell J. C., Pan P., Lee J. (2001). Three-dimensional morphometric measurements of reef corals using underwater photogrammetry techniques. Coral Reefs 20, 193–199. doi: 10.1007/s003380100157
Cadena C. D., Zapata F. (2021). The genomic revolution and species delimitation in birds (and other organisms): Why phenotypes should not be overlooked. Ornithology 138, 1–18. doi: 10.1093/ornithology/ukaa069
Cadena C. D., Zapata F., Jiménez I. (2018). Issues and perspectives in species delimitation using phenotypic data: Atlantean evolution in darwin’s finches. Syst. Biol. 67, 181–194. doi: 10.1093/sysbio/syx071
Cardini A. (2003). The geometry of the marmot (Rodentia: Sciuridae) mandible: Phylogeny and patterns. Syst. Biol. 52, 186–205. doi: 10.1080/10635150390192807
Carstens B. C., Pelletier T. A., Reid N. M., Satler J. D. (2013). How to fail at species delimitation. Mol. Ecol. 22, 4369–4383. doi: 10.1111/mec.12413
Chaplin K., Sumner J., Hipsley C. A., Melville J. (2020). An integrative approach using phylogenomics and high-resolution X-ray computed tomography for species delimitation in cryptic taxa. Syst. Biol. 69, 294–307. doi: 10.1093/sysbio/syz048
Charrad M., Ghazzali N., Boiteau V., Niknafs A. (2014). NbClust: An r package for determining the relevant number of clusters in a data set. J. Stat. Software 61, 1–36. doi: 10.18637/jss.v061.i06
Chindapol N., Kaandorp J. A., Cronemberger C., Mass T., Genin A. (2013). Modelling growth and form of the scleractinian coral Pocillopora verrucosa and the influence of hydrodynamics. PloS Comput. Biol. 9, e1002849. doi: 10.1371/journal.pcbi.1002849
Courtney L. A., Fisher W. S., Raimondo S., Oliver L. M., Davis W. P. (2007). Estimating 3-dimensional colony surface area of field corals. J. Exp. Mar. Bio. Ecol. 351, 234–242. doi: 10.1016/j.jembe.2007.06.021
Dayrat B. (2005). Towards integrative taxonomy. Biol. J. Linn. Soc 85, 407–415. doi: 10.1111/j.1095-8312.2005.00503.x
Dubois A. (2011). Species and “strange species” in zoology: Do we need a “unified concept of species”? Comptes Rendus Palevol 10, 77–94. doi: 10.1016/j.crpv.2011.01.002
Ence D. D., Carstens B. C. (2011). SpedeSTEM: A rapid and accurate method for species delimitation. Mol. Ecol. Resour. 11, 473–480. doi: 10.1111/j.1755-0998.2010.02947.x
Erickson K. L., Pentico A., Quattrini A. M., McFadden C. S. (2021). New approaches to species delimitation and population structure of anthozoans: Two case studies of octocorals using ultraconserved elements and exons. Mol. Ecol. Resour. 21, 78–92. doi: 10.1111/1755-0998.13241
Faulwetter S., Vasileiadou A., Kouratoras M., Dailianis T., Arvanitidis C. (2013). Micro-computed tomography: Introducing new dimensions to taxonomy. Zookeys 263, 1–45. doi: 10.3897/zookeys.263.4261
Filatov M. V., Frade P. R., Bak R. P. M., Vermeij M. J. A., Kaandorp J. A. (2013). Comparison between colony morphology and molecular phylogeny in the Caribbean scleractinian coral genus Madracis. PloS One 8, e71287. doi: 10.1371/journal.pone.0071287
Finch W. H., Schneider M. K. (2006). Misclassification rates for four methods of group classification. Educ. Psychol. Meas. 66, 240–257. doi: 10.1177/0013164405278579
Flot J. F., Blanchot J., Charpy L., Cruaud C., Licuanan W. Y., Nakano Y., et al. (2011). Incongruence between morphotypes and genetically delimited species in the coral genus Stylophora: Phenotypic plasticity, morphological convergence, morphological stasis or interspecific hybridization? BMC Ecol. 11, 22. doi: 10.1186/1472-6785-11-22
Fontaneto D., Flot J.-F., Tang C. Q. (2015). Guidelines for DNA taxonomy, with a focus on the meiofauna. Mar. Biodivers. 45, 433–451. doi: 10.1007/s12526-015-0319-7
Forsman Z. H., Barshis D. J., Hunter C. L., Toonen R. J. (2009). Shape-shifting corals: molecular markers show morphology is evolutionarily plastic in Porites. BMC Evol. Biol. 9, 1–9. doi: 10.1186/1471-2148-9-45
Forsman Z., Wellington G. M., Fox G. E., Toonen R. J. (2015). Clues to unraveling the coral species problem: Distinguishing species from geographic variation in Porites across the pacific with molecular markers and microskeletal traits. PeerJ 3, e751. doi: 10.7717/peerj.751
Fukami H., Budd A. F., Levitan D. R., Jara J., Kersanach R., Knowlton N. (2004). Geographic differences in species boundaries among members of the Montastraea annularis complex based on molecular and morphological markers. Evolution 58, 324–337. doi: 10.1111/j.0014-3820.2004.tb01648.x
Gardiner J. D., Behnsen J., Brassey C. A. (2018). Alpha shapes: Determining 3D shape complexity across morphologically diverse structures. BMC Evol. Biol. 18, 184. doi: 10.1186/s12862-018-1305-z
González-Espinosa P. C., Paz-García D. A., Reyes-Bonilla H., Cabral-Tena R. A., Balart E. F. (2018). Evidence of sexual dimorphism in skeletal morphology of a gonochoric reef coral. R. Soc Open Sci. 5, 171843. doi: 10.1098/rsos.171843
Gutierrez-Heredia L., Benzoni F., Murphy E., Reynaud E. G. (2016). End to end digitisation and analysis of three-dimensional coral models, from communities to corallites. PloS One 11, e0149641. doi: 10.1371/journal.pone.0149641
Gutiérrez-Heredia L., D’Helft C., Reynaud E. G. (2015). Simple methods for interactive 3D modeling, measurements, and digital databases of coral skeletons. Limnol. Oceanogr. Methods 13, 178–193. doi: 10.1002/lom3.10017
Harrell F. E. J., Dupont C. (2021) Hmisc: Harrell miscellaneous. Available at: https://cran.r-project.org/package=Hmisc.
Homann H. (2007). Implementation of a 3D thinning algorithm. Insight J. October, 4. doi: 10.54294/xjdr5f
Hughes T. P., Anderson K. D., Connolly S. R., Heron S. F., Kerry J. T., Lough J. M., et al. (2018). Spatial and temporal patterns of mass bleaching of corals in the anthropocene. Science 359, 80–83. doi: 10.1126/science.aan8048
Hughes T. P., Barnes M. L., Bellwood D. R., Cinner J. E., Cumming G. S., Jackson J. B. C. C., et al. (2017). Coral reefs in the anthropocene. Nature 546, 82–90. doi: 10.1038/nature22901
Hyde S., Blum Z., Landh T., Lidin S., Ninham B. W., Larsson K. (1997). The language of shape: the role of curvature in condensed matter: physics, chemistry and biology (Amsterdam: Elsevier).
Ibáñez L., Schroeder W., Ng L., Cates J., Insight Software Consortium (2003). The ITK software guide. 2nd ed. Clifton Park, NY: Kitware Inc.
ISO/IEC (2020). ISO international standard ISO/IEC 14882:2020(E) – programming language c++. Available at: https://isocpp.org/std/the-standard.
Jensen R. J. (2009). Phenetics: Revolution, reform or natural consequence? Taxon 58, 50–60. doi: 10.1002/tax.581008
Kaandorp J. A. (1999). Morphological analysis of growth forms of branching marine sessile organisms along environmental gradients. Mar. Biol. 134, 295–306. doi: 10.1007/s002270050547
Kaandorp J. A., Koopman E. A., Sloot P. M. A., Bak R. P. M., Vermeij M. J. A., Lampmann L. E. H. (2003). Simulation and analysis of flow patterns around the scleractinian coral Madracis mirabilis (Duchassaing and Michelotti). Philos. Trans. R. Soc B Biol. Sci. 358, 1551–1557. doi: 10.1098/rstb.2003.1339
Kaandorp J. A., Kübler J. E. (2001). The algorithmic beauty of seaweeds, sponges, and corals. 1st ed (New York: Springer-Verlag Berlin Heidelberg). doi: 10.1007/978-3-662-04339-4
Kaandorp J. A., Sloot P. M. A., Merks R. M. H., Bak R. P. M., Vermeij M. J. A., Maier C. (2005). Morphogenesis of the branching reef coral Madracis mirabilis. Proc. R. Soc B Biol. Sci. 272, 127–133. doi: 10.1098/rspb.2004.2934
Keshavmurthy S., Yang S.-Y., Alamaru A., Chuang Y.-Y., Pichon M., Obura D., et al. (2013). DNA Barcoding reveals the coral “laboratory-rat”, Stylophora pistillata encompasses multiple identities. Sci. Rep. 3, 1520. doi: 10.1038/srep01520
Klingenberg C. P. (2010). Evolution and development of shape: Integrating quantitative approaches. Nat. Rev. Genet. 11, 623–635. doi: 10.1038/nrg2829
Klinkenbuß D., Metz O., Reichert J., Hauffe T., Neubauer T. A., Wesselingh F. P., et al. (2020). Performance of 3D morphological methods in the machine learning assisted classification of closely related fossil bivalve species of the genus Dreissena. Malacologia 63, 95–105. doi: 10.4002/040.063.0109
Knowlton N. (1993). Sibling species in the sea. Annu. Rev. Ecol. Syst. 24, 189–216. doi: 10.1146/annurev.es.24.110193.001201
Konglerd P., Reeb C., Jansson F., Kaandorp J. A. (2017). Quantitative morphological analysis of 2D images of complex-shaped branching biological growth forms: The example of branching thalli of liverworts. BMC Res. Notes 10, 103. doi: 10.1186/s13104-017-2424-0
Kruszyński K. J., Kaandorp J. A., Van Liere R. (2007). A computational method for quantifying morphological variation in scleractinian corals. Coral Reefs 26, 831–840. doi: 10.1007/s00338-007-0270-6
Laforsch C., Christoph E., Glaser C., Naumann M., Wild C., Niggl W. (2008). A precise and non-destructive method to calculate the surface area in living scleractinian corals using X-ray computed tomography and 3D modeling. Coral Reefs 27, 811–820. doi: 10.1007/s00338-008-0405-4
Laforsch C., Imhof H., Sigl R., Settles M., He M., Wanninger A. (2012). “Applications of computational 3D-modeling in organismal biology,” in Modeling and Simulation in Engineering, Ed. Alexandru C. (Rijeka: InTech), 117–142. doi: 10.5772/31092
Lee T. C., Kashyap R. L., Chu C. N. (1994). Building skeleton models via 3D medial surface axis thinning algorithms. CVGIP Graph. Model. Image Process. 56, 462–478. doi: 10.1006/cgip.1994.1042
Luckow M. (1995). Species concepts: assumptions, methods, and applications. Syst. Bot. 20, 589–605. doi: 10.2307/2419812
MacLeod N. (2002). “Phylogenetic signals in morphometric data,” in Morphology, shape and phylogeny. Eds. MacLeod N., Forey P. L. (London: CRC Press, Taylor & Francis Group).
Maechler M., Rousseeuw P., Struyf A., Hubert M., Hornik K. (2021) Cluster: cluster analysis basics and extensions. Available at: https://cran.r-project.org/package=cluster.
Martin-Garin B., Lathuilière B., Verrecchia E. P., Geister J. (2007). Use of fractal dimensions to quantify coral shape. Coral Reefs 26, 541–550. doi: 10.1007/s00338-007-0256-4
Meyer M., Desbrun M., Schröder P., Barr A. H. (2003). “Discrete differential-geometry operators for triangulated 2-manifolds,” in Visualization and mathematics III. Eds. Hege H. C., Polthier K. (Berlin-Heidelberg: Springer), 35–57. doi: 10.1007/978-3-662-05105-4_2
Migicovsky Z., Li M., Chitwood D. H., Myles S. (2018). Morphometrics reveals complex and heritable apple leaf shapes. Front. Plant Sci. 8, 2185. doi: 10.3389/fpls.2017.02185
Miller K. (1994). Morphological variation in the coral genus Platygyra: Environmental influences and taxonomic implications. Mar. Ecol. Prog. Ser. 110, 19–28. doi: 10.3354/meps110019
Naimi B., Hamm N., Groen T. A., Skidmore A. K., Toxopeus A. G. (2014). Where is positional uncertainty a problem for species distribution modelling. Ecography (Cop.). 37, 191–203. doi: 10.1111/j.1600-0587.2013.00205.x
Naumann M. S., Niggl W., Laforsch C., Glaser C., Wild C. (2009). Coral surface area quantification-evaluation of established techniques by comparison with computer tomography. Coral Reefs 28, 109–117. doi: 10.1007/s00338-008-0459-3
Orbach D. N., Brassey C. A., Gardiner J. D., Brennan P. L. R. (2021). 3D genital shape complexity in female marine mammals. Ecol. Evol. 11, 3210–3218. doi: 10.1002/ece3.7269
Paz-García D. A., Aldana-Moreno A., Cabral-Tena R. A., García-De-León F. J., Hellberg M. E., Balart E. F. (2015a). Morphological variation and different branch modularity across contrasting flow conditions in dominant Pocillopora reef-building corals. Oecologia 178, 207–218. doi: 10.1007/s00442-014-3199-9
Paz-García D. A., Hellberg M. E., García-de-León F. J., Balart E. F. (2015b). Switch between morphospecies of Pocillopora corals. Am. Nat. 186, 434–440. doi: 10.1086/682363
Pereira C. L., Gilbert M. T. P., Araújo M. B., Matias M. G. (2021). Fine-tuning biodiversity assessments: A framework to pair eDNA metabarcoding and morphological approaches. Methods Ecol. Evol. 12, 2397–2409. doi: 10.1111/2041-210X.13718
Poon L. K. M., Zhang N. L., Liu T., Liu A. H. (2013). Model-based clustering of high-dimensional data: Variable selection versus facet determination. Int. J. Approx. Reason. 54, 196–215. doi: 10.1016/j.ijar.2012.08.001
Pradlwarter H. J., Schuëller G. I. (2008). The use of kernel densities and confidence intervals to cope with insufficient data in validation experiments. Comput. Methods Appl. Mech. Eng. 197, 2550–2560. doi: 10.1016/j.cma.2007.09.028
Puillandre N., Modica M. V., Zhang Y., Sirovich L., Boisselier M. C., Cruaud C., et al. (2012). Large-scale species delimitation method for hyperdiverse groups. Mol. Ecol. 21, 2671–2691. doi: 10.1111/j.1365-294X.2012.05559.x
Raftery A. E., Dean N. (2006). Variable selection for model-based clustering. J. Am. Stat. Assoc. 101, 168–178. doi: 10.1198/016214506000000113
Ramírez-Portilla C., Baird A. H., Cowman P. F., Quattrini A. M., Harii S., Sinniger F., et al. (2022). Solving the coral species delimitation conundrum. Syst. Biol. 71, 461–475. doi: 10.1093/sysbio/syab077
R Core Team (2018) R: A language and environment for statistical computing. Available at: https://www.r-project.org/.
Reeb C., Kaandorp J., Jansson F., Puillandre N., Dubuisson J.-Y., Cornette R., et al. (2018). Quantification of complex modular architecture in plants. New Phytol. 218, 859–872. doi: 10.1111/nph.15045
Reichert J., Backes A. R., Schubert P., Wilke T. (2017). The power of 3D fractal dimensions for comparative shape and structural complexity analyses of irregularly shaped organisms. Methods Ecol. Evol. 8, 1650–1658. doi: 10.1111/2041-210X.12829
Reichert J., Schellenberg J., Schubert P., Wilke T. (2016). 3D scanning as a highly precise, reproducible, and minimally invasive method for surface area and volume measurements of scleractinian corals. Limnol. Oceanogr. Methods 14, 518–526. doi: 10.1002/lom3.10109
Reinders F., Jacobson M. E. D., Post F. H. (2000). “Skeleton graph generation for feature shape description,” in Data visualization 2000. Eds. Leeuw W.C. de, Liere R.v. (Amsterdam: Springer Netherlands), 73–82. doi: 10.1007/978-3-7091-6783-0_8
Revelle W. (2021) Psych: procedures for psychological, psychometric, and personality research. Available at: https://cran.r-project.org/package=psych.
RStudio Team (2017) RStudio: Integrated development for r. Available at: http://www.rstudio.com/.
Saraswati P. K., Srinivasan M. S. (2016). “Morphology, taxonomy and concepts of species,” in Micropaleontology (Cham: Springer International Publishing), 53–65. doi: 10.1007/978-3-319-14574-7_4
Schlick-Steiner B. C., Seifert B., Stauffer C., Christian E., Crozier R. H., Steiner F. M. (2007). Without morphology, cryptic species stay in taxonomic crypsis following discovery. Trends Ecol. Evol. 22, 391–392. doi: 10.1016/j.tree.2007.05.004
Schroeder W., Martin K., Lorensen B. (2006). The visualization toolkit. 4th ed (Clifton Park, NY: Kitware Inc).
Scott D. W. (2015). Multivariate density estimation: theory, practice, and visualization (New York: John Wiley & Sons, Inc).
Scrucca L. (2010). Dimension reduction for model-based clustering. Stat. Comput. 20, 471–484. doi: 10.1007/s11222-009-9138-7
Scrucca L., Raftery A. E. (2018). Clustvarsel: A package implementing variable selection for Gaussian model-based clustering in R. J. Stat. Software 84, 1–28. doi: 10.18637/jss.v084.i01
Sigl R., Imhof H., Settles M., Laforsch C. (2013). A novel, non-invasive and in vivo approach to determine morphometric data in starfish. J. Exp. Mar. Bio. Ecol. 449, 1–9. doi: 10.1016/j.jembe.2013.08.002
Siqueira A. C., Kiessling W., Bellwood D. R. (2022). Fast-growing species shape the evolution of reef corals. Nat. Commun. 13, 1–8. doi: 10.1038/s41467-022-30234-6
Sites J. W., Marshall J. C. (2004). Operational criteria for delimiting species. Annu. Rev. Ecol. Evol. Syst. 35, 199–227. doi: 10.1146/annurev.ecolsys.35.112202.130128
Sokal R. R. (1986). Phenetic taxonomy: Theory and methods. Annu. Rev. Ecol. Syst. 17, 423–442. doi: 10.1146/annurev.es.17.110186.002231
Suzuki R., Terada Y., Shimodaira H. (2019) Pvclust: Hierarchical clustering with p-values via multiscale bootstrap resampling. Available at: https://cran.r-project.org/package=pvclust.
Todd P. A. (2008). Morphological plasticity in scleractinian corals. Biol. Rev. 83, 315–337. doi: 10.1111/j.1469-185X.2008.00045.x
Todd P. A., Ladle R. J., Lewin-Koh N. J. I., Chou L. M. (2004). Genotype × environment interactions in transplanted clones of the massive corals Favia speciosa and Diploastrea heliopora. Mar. Ecol. Prog. Ser. 271, 167–182. doi: 10.3354/meps271167
Torres-Pulliza D., Dornelas M. A., Pizarro O., Bewley M., Blowes S. A., Boutros N., et al. (2020). A geometric basis for surface habitat complexity and biodiversity. Nat. Ecol. Evol. 4, 1495–1501. doi: 10.1038/s41559-020-1281-8
Valcárcel V., Vargas P. (2010). Quantitative morphology and species delimitation under the general lineage concept: optimization for Hedera (Araliaceae). Am. J. Bot. 97, 1555–1573. doi: 10.3732/ajb.1000115
Veal C. J., Holmes G., Nunez M., Hoegh-Guldberg O., Osborn J. (2010). A comparative study of methods for surface area and three dimensional shape measurement of coral skeletons. Limnol. Oceanogr. Methods 8, 241–253. doi: 10.4319/lom.2010.8.241
Venables W. N., Ripley B. D. (2002). Modern applied statistics with S (Fourth. New York: Springer). Available at: https://www.stats.ox.ac.uk/pub/MASS4/.
Veron J. E. N. (2000). Corals of the world (Townsville: Australian Institute of Marine Science (AIMS).
Virtanen P., Gommers R., Oliphant T. E., Haberland M., Reddy T., Cournapeau D., et al. (2020). SciPy 1.0: Fundamental algorithms for scientific computing in Python. Nat. Methods 17, 261–272. doi: 10.1038/s41592-019-0686-2
Wallace C. C. (1999). Staghorn corals of the world: A revision of the genus Acropora. (Collibgwood, Victoria: CSIRO Publishing). doi: 10.1071/9780643101388
Wallace C., Pandolfi J., Young A., Wolstenholme J. (1991). Indo-pacific coral biogeography: A case study from the Acropora selago group. Aust. Syst. Bot. 4, 199. doi: 10.1071/SB9910199
Wallace C. C., Willis B. L. (1994). Systematics of the coral genus Acropora: implications of new biological findings for species concepts. Annu. Rev. Ecol. Syst. 25, 237–262. doi: 10.1146/annurev.es.25.110194.001321
Wheeler Q. D. (2005). Losing the plot: DNA “barcodes” and taxonomy. Cladistics 21, 405–407. doi: 10.1111/j.1096-0031.2005.00075.x
Wickham H. (2016). ggplot2: elegant graphics for data analysis (New York: Springer-Verlag New York). Available at: https://ggplot2.tidyverse.org.
Wolstenholme J. K., Wallace C. C., Chen C. A. (2003). Species boundaries within the Acropora humilis species group (Cnidaria; scleractinia): A morphological and molecular interpretation of evolution. Coral Reefs 22, 155–166. doi: 10.1007/s00338-003-0299-0
Zapata F., Jiménez I. (2012). Species delimitation: Inferring gaps in morphology across geography. Syst. Biol. 61, 179–194. doi: 10.1093/sysbio/syr084
Zawada K. J. A., Dornelas M., Madin J. S. (2019a). Quantifying coral morphology. Coral Reefs 38, 1281–1292. doi: 10.1007/s00338-019-01842-4
Zawada K. J. A., Madin J. S., Baird A. H., Bridge T. C. L., Dornelas M. (2019b). Morphological traits can track coral reef responses to the anthropocene. Funct. Ecol. 33, 962–975. doi: 10.1111/1365-2435.13358
Zhao S., Yin L., Guo Y., Sheng Q., Shyr Y. (2021) heatmap3: an improved heatmap package. Available at: https://cran.r-project.org/package=heatmap3.
Keywords: species delimitation, quantitative morphology, phenotypic variation, 3D scanning, skeletonization algorithms, feature selection, surface curvature
Citation: Ramírez-Portilla C, Bieger IM, Belleman RG, Wilke T, Flot J-F, Baird AH, Harii S, Sinniger F and Kaandorp JA (2022) Quantitative three-dimensional morphological analysis supports species discrimination in complex-shaped and taxonomically challenging corals. Front. Mar. Sci. 9:955582. doi: 10.3389/fmars.2022.955582
Received: 28 May 2022; Accepted: 12 August 2022;
Published: 06 September 2022.
Edited by:
David A. Paz-García, Centro de Investigación Biológica del Noroeste (CIBNOR), MexicoReviewed by:
Ann Budd, The University of Iowa, United StatesSylvain Agostini, Shimoda Marine Research Center, University of Tsukuba, Tsukuba, Japan
Copyright © 2022 Ramírez-Portilla, Bieger, Belleman, Wilke, Flot, Baird, Harii, Sinniger and Kaandorp. This is an open-access article distributed under the terms of the Creative Commons Attribution License (CC BY). The use, distribution or reproduction in other forums is permitted, provided the original author(s) and the copyright owner(s) are credited and that the original publication in this journal is cited, in accordance with accepted academic practice. No use, distribution or reproduction is permitted which does not comply with these terms.
*Correspondence: Catalina Ramírez-Portilla, Y2F0YWxpbmEucnpwbEBnbWFpbC5jb20=; Jaap A. Kaandorp, Si5BLkthYW5kb3JwQHV2YS5ubA==