- 1Key Laboratory of Sichuan Province for Fishes Conservation and Utilization in the Upper Reaches of the Yangtze River, College of Life Sciences, Neijiang Normal University, Neijiang, China
- 2Key Laboratory of Prevention and Control for Aquatic Invasive Alien Species, Ministry of Agriculture and Rural Affairs, Guangdong Modern Recreational Fisheries Engineering Technology Center, Pearl River Fisheries Research Institute, Chinese Academy of Fishery Sciences, Guangzhou, China
- 3Shenzhen Key Lab of Marine Genomics, Guangdong Provincial Key Lab of Molecular Breeding in Marine Economic Animals, BGI Academy of Marine Sciences, BGI Marine, Shenzhen, China
Midas cichlid (Amphilophus citrinellus), a popular aquarium fish, attracts extensive attention from worldwide biologists mainly due to its morphological polymorphism (dark versus gold). Continuous efforts have therefore been paid to address mechanisms of its coloration variants, while it is far away from the detailed illustration of a clear regulatory network. Some limits may come from the absence of a high-quality genome assembly and a relatively accurate gene set. In this study, we sequenced about 149 Gb of nucleotide sequences of Midas cichlid, generating a genome assembly with a total size of 933.5 Mb, which exhibits a good genome continuity with a contig N50 of 10.5 Mb. A total of 25,911 protein-coding genes were annotated and about 90% completeness was achieved, which helps to build a good gene pool for understanding expressional differences of color variation. With the assistance of the final gene set, we identified a total of 277 differential expressional genes (DEGs), of which 97 up- and 180 downregulated were determined in dark-vs-gold comparisons. Two protein-protein interaction (PPI) networks were constructed from these DEGs, and three key functional modules were classified. Hub genes within each module were evaluated, and we found that the third key module contains tyrp1b, oca2, pmela, tyr, and slc24a5, which were previously proven to be associated with melanin formation. Two downregulated DEGs (myl1 and pgam2) in the first key module may be involved in muscle movement and spermatogenesis, implying that certain side effects could result from the morphological polymorphism. The first key module, consisting of proteins encoded by upregulated DEGs that were associated with MAPK signaling, Toll-like receptor signaling, and gonadotropin-releasing hormone pathways, may contribute to a negative upstream regulation or downstream influence on melanin biosynthesis. Taken together, our new genome assembly and gene annotation of Midas cichlid provide a high-quality genetic resource for biological studies on this species, and the newly identified key networks and hub genes in dark-vs-gold comparisons enhance our understanding of the transcriptional regulatory mechanisms underlying coloration changes not only in Midas cichlid but also in other fishes from freshwater to marine ecosystems.
Introduction
Midas cichlid, Amphilophus citrinellus, as a polychromatic fish has attracted extensive attention from worldwide biologists to study speciation and coloration mechanisms due to its great amount of intraspecific variations (Maan and Sefc, 2013; Sefton, 2017). It can be mainly divided into dark- and gold-morph types, and the latter has been widely cultured for its aesthetic features that make it one of the most popular aquarium species worldwide (Oldfield, 2011). Exploration of its color regulation, a potentially conservative mechanism, is also instructive for studies on more colorful phenotypes in both freshwater and marine fishes. Meanwhile, Midas cichlid is a good genetic resource to produce derived species, such as a blood parrot that is a hybrid between Midas cichlid and its relative redhead cichlid (Paraneetroplus melanurus; Aqmal-Naser and Ahmad, 2020).
The conspicuous gold-morph of Midas cichlid has an unequal appearance frequency. Compared with dark-morph individuals, the gold-morph type occurs at a low frequency (1.9-23.9%) in previous surveys (Elmer et al., 2009; Elmer et al., 2010). The gold-morph generation is related to loss of melanic pigmentation during adolescence, while some of them keep the dark coloration during their whole life (Henning et al., 2013). The mechanism controlling morphological polymorphism of Midas cichlid is assumed to be monogenic (Thomas, 1976; Henning et al., 2013), and the putative serine/threonine kinase gene (stk) has been considered as the molecular basis for differentiation of the dark/gold phenotypes (Kautt et al., 2020). However, more recently a new defined gene “goldentouch” may also contribute to the division of dark/gold types (Kratochwil et al., 2022). A comparative transcriptomic analysis revealed significant transcriptional changes of the core genes involved in the melanosomal pathway, potentially acting as the main factors to affect the dark/gold differentiation (Henning et al., 2013). These studies render continuous endeavors to explore genetic mechanisms associated with variation of dart-to-gold phenotypes, yet it is still far away from a complete and clear illustration. Currently, two versions of genome assembly for Midas cichlid are publically available (Elmer et al., 2014; Abate and Noakes, 2021); however, their contiguity, mainly reflected by contig N50 (51.8 kb and 3.8 Mb, respectively) is relatively low. In this high-throughput sequencing era, the third-generation sequencing technology (with long sequencing reads) enables the ability to produce a longer and better contiguity, which further benefits a high-quality gene annotation for better understanding of gene expression patterns and transcriptional regulation of coloration in the representative Midas cichlid.
In the present study, we aim to sequence the whole genome of a gold-morph Midas cichlid (Figure 1A) with a high coverage depth. By a combination of Illumina and Nanopore sequencing technologies, we make efforts to construct a high-quality genome assembly with an improved continuity compared to previous versions. By using various gene prediction strategies and careful integration, we attempt to generate a non-redundant gene set, which can be effective for the study of transcriptomic differences between dark and gold types. With the assistance of this gene set, we can examine differentially expressed genes (DEGs) and evaluate key gene networks with hub genes to obtain a deeper understanding of the molecular mechanisms and biological effects underlying morphological polymorphism.
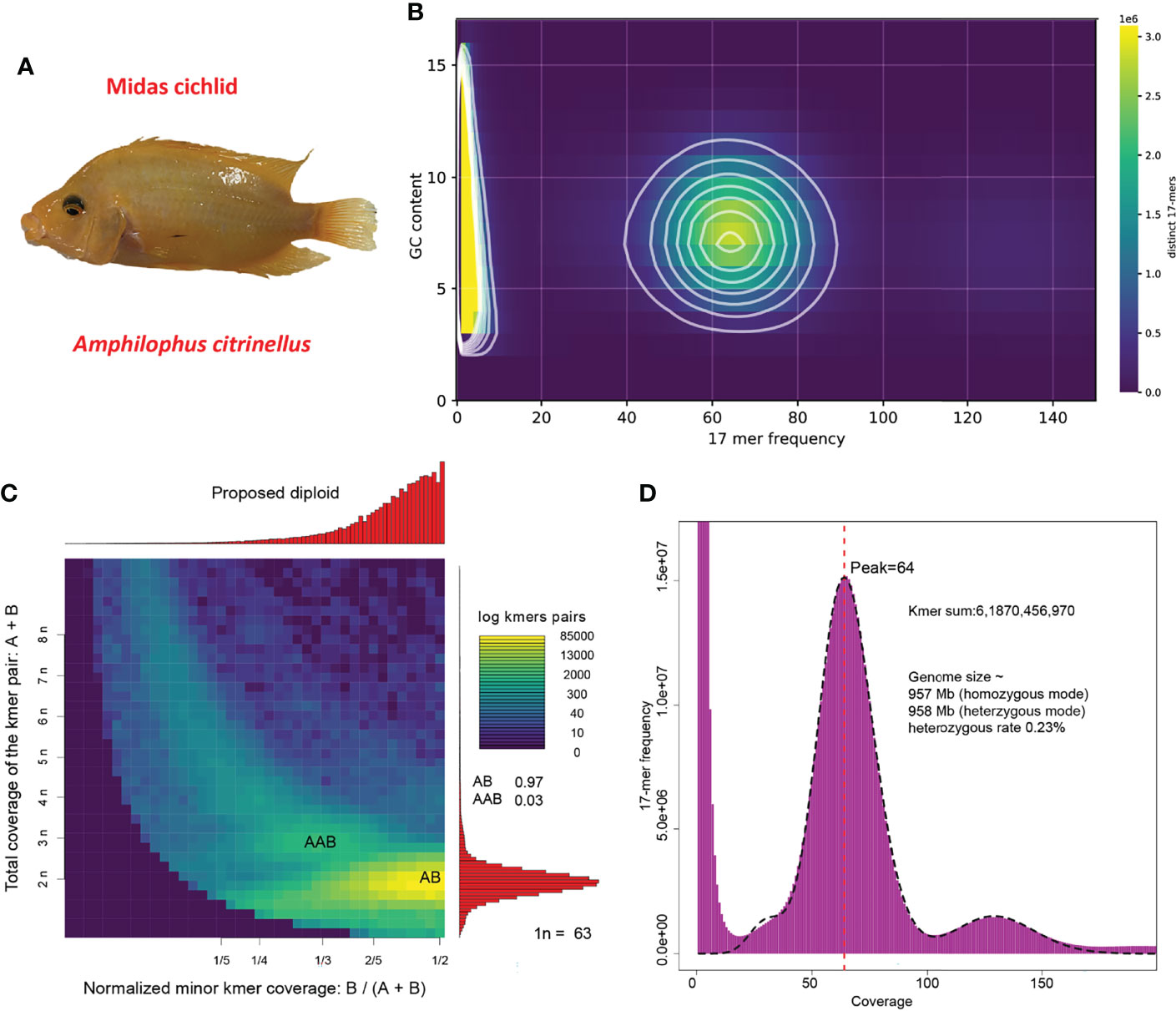
Figure 1 Specimen and genome features. (A) Image of the sequenced Midas cichlid; (B) Contamination evaluation based on GC content and 17-mer frequency; (C) Ploidy estimation; (D) Genome-size estimation and heterozygous rate evaluation.
Materials and Methods
Sampling and Sequencing
An adult gold-morph Midas cichlid (Figure 1A), bred in the Pearl River Fisheries Research Institute in Guangzhou city of China, was collected. It was determined as a male since its testis was anatomized (Figure S1). Its liver was sampled for Illumina next-generation sequencing (NGS), and its muscle was collected for Nanopore third-generation sequencing (TGS). Several tissues including muscle, heart, and brain were mixed for transcriptome sequencing, which enables a comprehensive coverage of mRNAs for a better gene annotation.
For the NGS, the quality of isolated genomic DNAs was verified by using an integrated strategy of the following methods: (1) DNA degradation and contamination were monitored on 1% agarose gels; (2) DNA concentration was measured by a Qubit DNA Assay Kit in Qubit 3.0 Flurometer (Invitrogen, Carlsbad, CA, USA). A total amount of 0.2 μg DNA per sample was used as the input material for library preparations. The sequencing library was generated using Next Ultra DNA Library Prep Kit (New England Biolabs, Ipswich, MA, USA) following the manufacturer’s recommendations, and index codes were added to each sample for paired-end sequencing in an Illumina HiSeq 2000 system (Illumina, San Diego, CA, USA). In brief, the genomic DNA sample was fragmented by sonication to a size of 350 bp. Sequence artifacts, including reads with adapter sequences, low-quality, and/or unrecognizable nucleotides, were removed. We used Fastp (version 0.19.7) (Chen et al., 2018) to perform basic statistics on the quality of these raw reads. The detailed steps of data processing were set as follows: (1) Discard any paired reads when either one contains adapter sequences; (2) Discard any paired reads when more than 10% of bases are uncertain in either one read; (3) Discard any paired reads if the proportion of low-quality (Phred quality < 5) bases is over 50% in either one read. For the TGS, a 20-kb library was constructed and sequenced on a Nanopore PromethION platform (Nanopore, Oxford, England) using the routine SMRT sequencing strategy.
For the transcriptome sequencing (RNA-seq), the integrity of total RNA was assessed using a Fragment Analyzer 5400 (Agilent Technologies, Santa Clara, CA, USA). Sequencing libraries were generated using NEBNext Ultra RNA Library Prep Kit (New England Biolabs) following the manufacturer’s instructions, and index codes were added to attribute sequences to each sample. Briefly, mRNAs were purified from the total RNA using poly-T oligo-attached magnetic beads. Fragmentation was carried out using divalent cations under an elevated temperature in NEBNext First Strand Synthesis Reaction Buffer (5x). First strand cDNAs were synthesized using random hexamer primers and M-MuLV Reverse Transcriptase (RNase H). Second strand cDNA synthesis was subsequently performed using DNA Polymerase I and RNase H. Remaining overhangs were converted into blunt ends via exonuclease/polymerase. After adenylation of 3’ ends of DNA fragments, NEBNext Adaptors with hairpin loop structures were ligated for hybridization. In order to select cDNA fragments of preferentially 250~300 bp in length, the library fragments were purified with an AMPure XP system (Beckman Coulter, Beverly, MA, USA). Subsequently, 3 μl of USER Enzyme (New England Biolabs) was used with size-selected, adaptor-ligated cDNAs at 37°C for 15 min followed by 5 min at 95°C before PCR. Then PCRs were performed with Phusion High-Fidelity DNA polymerase, Universal PCR primers, and Index primer pairs. At last, PCR products were purified, and then their quality was checked on an Agilent Bioanalyzer 2100 (Palo Alto, CA, USA).
Genome-Size Estimation and De Novo Genome Assembly
After obtaining the sequencing data, we firstly employed KAT (kmer analysis tools, version 2.4.2 (Mapleson et al., 2017)) to evaluate if experimental contamination was hidden in the sequencing library. Then, we used kmc version 3.0.0 (Kokot et al., 2017) to count 17-mer coverage depth and extracted heterozygous 17-mers to estimate the ploidy of the sequenced fish using Smudgeplot default version (Ranallo-Benavidez et al., 2020). Meanwhile, we applied GCE version 1.0.3 (Ranallo-Benavidez et al., 2020) to estimate the genome size and heterozygous rate based on the 17-mer coverage depth.
After ensuring the quality in sequencing and some basic genome features such as ploidy, genome size and heterozygous rate, we generated de novo assemblies using three genome-assemblers including NECAT version 0.0.1_update20200803 (Chen et al., 2021), wtdbg2 version 2.5 (Ruan and Li, 2020), and flye version 2.9-b1768 (Kolmogorov et al., 2019). We chose the best result with a high contiguity (represented by a high contig N50) and a close length to the genome-size estimate as an optimized outcome, and this assembly was used for subsequent analyses. The errors in the assembly generated from TGS were fixed by two rounds of self-correction by TGS reads, then further polished by NGS reads with three iterations using NextPolish version 1.4.0 (Hu et al., 2020).
The quality of our assembly was evaluated by several approaches. Firstly, we applied conserved single-copy genes of Actinopterygii to align our assembly, which is conducted in BUSCOs (version 3) (Seppey et al., 2019). Secondly, we used KAT to compare the k-mers that appeared in both our assembly and NGS; when a high rate of k-mers appeared in the assembly, it is proven to have a high completeness. Thirdly, we applied LAI (Ou et al., 2018) to evaluate the continuity of our assembly based on the ratio of whole LTR retrotransposons (LTR-RTs) using LTR_retriever version 2.9.0 (Ou and Jiang, 2018). Finally, we mapped previously published 37 transcriptomes from diverse tissues (see more details in Table S1) to our assembly for a quality evaluation. Meanwhile, we evaluated the two available versions of Midas cichlids genome in NCBI by comparing the indexes of contig N10, N50, N90, and BUSCOs under genome mode and LAI.
Reapeats and Gene Annotation
We firstly employed RepeatModeler version 2.0.1 (Flynn et al., 2020) calling RepeatScout version 1.0.6 (Price et al., 2005), TRF version 4.09 (Benson, 1999), RECON version 1.08 (Benson, 1999), LtrHarvest version 1.6.2 (Ellinghaus et al., 2008), and Ltr_retriever (Ou and Jiang, 2018) to build a de novo repeat library. Secondly, we searched the repeats belonging to lineages of Cichliformes including Midas cichlids from Repbase database (Bao et al., 2015) as an known library. The two libraries were combined and then searched in the assembled Midas cichlid genome with optimized parameters “-nolow -gff -poly -a -inv -e rmblast” to obtain a complete list of repeats in RepeatMasker version 4.1.1 (Chen, 2004).
We applied three distinct strategies to locate protein-coding regions in the assembled Midas cichlid genome, including de novo, homolog-based and transcripts-based predictions. Two programs, Augustus version 3.3.3 (Stanke et al., 2006) and Snap version 2006-07-28 (Korf, 2004), were used for de novo prediction. The proteins from seven representative species, including six Cichliformes and zebrafish (Table S2), were used for homolog-based prediction that was performed by Tblastn version 2.11.0 (Gish and States, 1993) and Exonerate version 2.2.0 (Slater and Birney, 2005). For the transcripts-based prediction, we firstly generated a de novo assembly of transcriptome using Trinity version 2.11.0 (Haas et al., 2013), and then the produced raw transcripts were mapped onto the assembled genome by Blat version 36 (Kent, 2002) and Gmap version 2017-11-15 (Wu and Watanabe, 2005), followed by verification and integration to assemble spliced alignments (PASA, (Haas et al., 2008)) pipeline. Finally, we employed EvidenceModeler version 1.1.1 (Haas et al., 2008) to integrate these results generated from the above three strategies, and the false predictions with stop codon(s) inside coding regions were removed. To understand the biological functions of deduced proteins, we aligned their sequences to those that were reviewed in Uniprot database using Tblastn.
Comparative Transcriptomic Analysis Between Dark- and Gold-Morph Individuals
To explore gene transcriptional difference between the dark and gold phenotypes of Midas cichlid, we downloaded available scale transcriptomes from both groups to perform a comparative transcriptome analysis; the dark- and gold-morph adult individuals were chosen, and four repeated samples were checked for both color types (See Table S3). All the transcriptomes were mapped to our Midas cichlid genome assembly by Hisat2 version 2.2.1 (Kim et al., 2019) to obtain coverage depth of every gene. Then, reads mapping counts were calculated based on gene annotation by Stringtie version 2.1.4 (Pertea et al., 2015), and gene transcription levels were represented by TPM (transcripts per kilobase per million mapped reads). DEG analysis between dark- and gold-morphs was conducted using “edgeR” (version 3.15) in the R package (Robinson et al., 2010), with 0.05 as the FDR (false discovery rate) cut-off and a log2FC (fold change) cut-off of 1. Down- and upregulated DEGs were further summarized in a heatmap using TBtools version 1.098725 (Chen et al., 2020a) and enriched by GO (Gene Ontology) analysis using GOATOOLs version 1.2.3 (Klopfenstein et al., 2018).
Hub Genes Identification
To explore functional networks of these DEGs, we imported the proteins encoded by DEGs into STRING (with zebrafish as the representative species), a search tool for the retrieval of interacting genes (http://string-db.org) (Szklarczyk et al., 2010), to reveal their protein-protein interactions. The significantly enriched terms (p-value > 0.05) about KEGG pathways or GO biological processes were used to annotate the main functions of these DEGs. The predicted PPI networks were further imported into Cytoscape version 3.9.1 (Shannon et al., 2003) for visualization, and the inside key functional modules were determined by the molecular complex detection technology (MCODE; version 1.32 (Bader and Hogue, 2003)) plug-in of Cytoscape with the following parameters “K-score = 2, degree cutoff=2, max depth = 100, score cutoff = 0.2 “. From each of the key functional modules, we identified hub genes by using the cytoHubba version 0.1 (Chin et al., 2014) plug-in of Cytoscape, and seven common algorithms (MCC, MNC, Degree, Closeness, Radiality, Stress, and EPC) were applied for practical prediction of hub genes.
Results
Sequencing Data Output and Genome Characterization
After quality control, a total of 71.9 Gb of Illumina NGS reads and 77 Gb of Nanopore TGS reads for Midas cichlid were obtained. By breaking NGS data into k-mers (length = 17, 17-mers), a matrix containing distinct 17-mer counts at varying frequency and GC content was produced. We made a density plot that highlights genuine frequency between 40-85 folds with a GC content spreading from 3 to 17 (Figure 1B). No unexpected contents beyond the main density blocks were observed, indicating no contamination in the sequencing library of Midas cichlid.
We estimated the sample of Midas cichlid (Figure 1A) as a diploid by extracting and analyzing heterozygous 17-mer pairs (Figure 1C). Subsequently, we applied heterozygous mode to estimate heterozygous rate that is about 0.23% (Figure 1D). By using the routine k-mer frequency distribution analysis (Li et al., 2010a), we estimate the genome size of Midas cichlid to be about 957 Mb (Figure 1D).
Genome Assembly, Annotation, and Quality Evaluation
After comparing genome size and contig N50 value of various genome assemblies generated from different assemblers (NECAT, wtdbg2, flye), we chose the assembly from NECAT as the best one. After polishing sequencing errors produced in TGS, the contig N50 was improved, reaching 10.6 Mb, which is much higher than any version of previously reported draft (Table 1). Based on the final assembly size of 933.5 Mb, we calculated the NGS and TGS data used in this study as about 77- and 82.5-fold depth, respectively. The total sequencing coverage depth is about 159.5-fold.
The estimated assembly completeness from k-mer (k=31, 31-mer) spectrum using kmer analysis tools (KAT) is 99.87% (Figure 2A). A Benchmarking universal single-copy orthologs (BUSCOs) estimate indicated a 98.2% completeness, which is slightly higher than the two previous versions of GCA_000751415.1 and GCA_013435755.1 (Figure 2B). When we referred to LTR assembly index (the ratio of complete LTR-RTs, LAI), the LAI estimation was failed in the GCA_000751415.1 as complete LTR-RTs are not enough for the analysis. The LAI was evaluated as 6.4 in the GCA_013435755.1. However, it was estimated as 10.6 for our assembly (see Table 1), reaching a reference genome level according to the previously established LAI standards (Ou et al., 2018). In addition, the mapping ratio of 35 transcriptomes from different samples and replicates ranged from 94% to 98% (except for one at 87.45%; see Figure 2C), suggesting a high completeness of our current assembly. Taken together, these results support achievement of a high-quality genome assembly of Midas cichlid in this study.
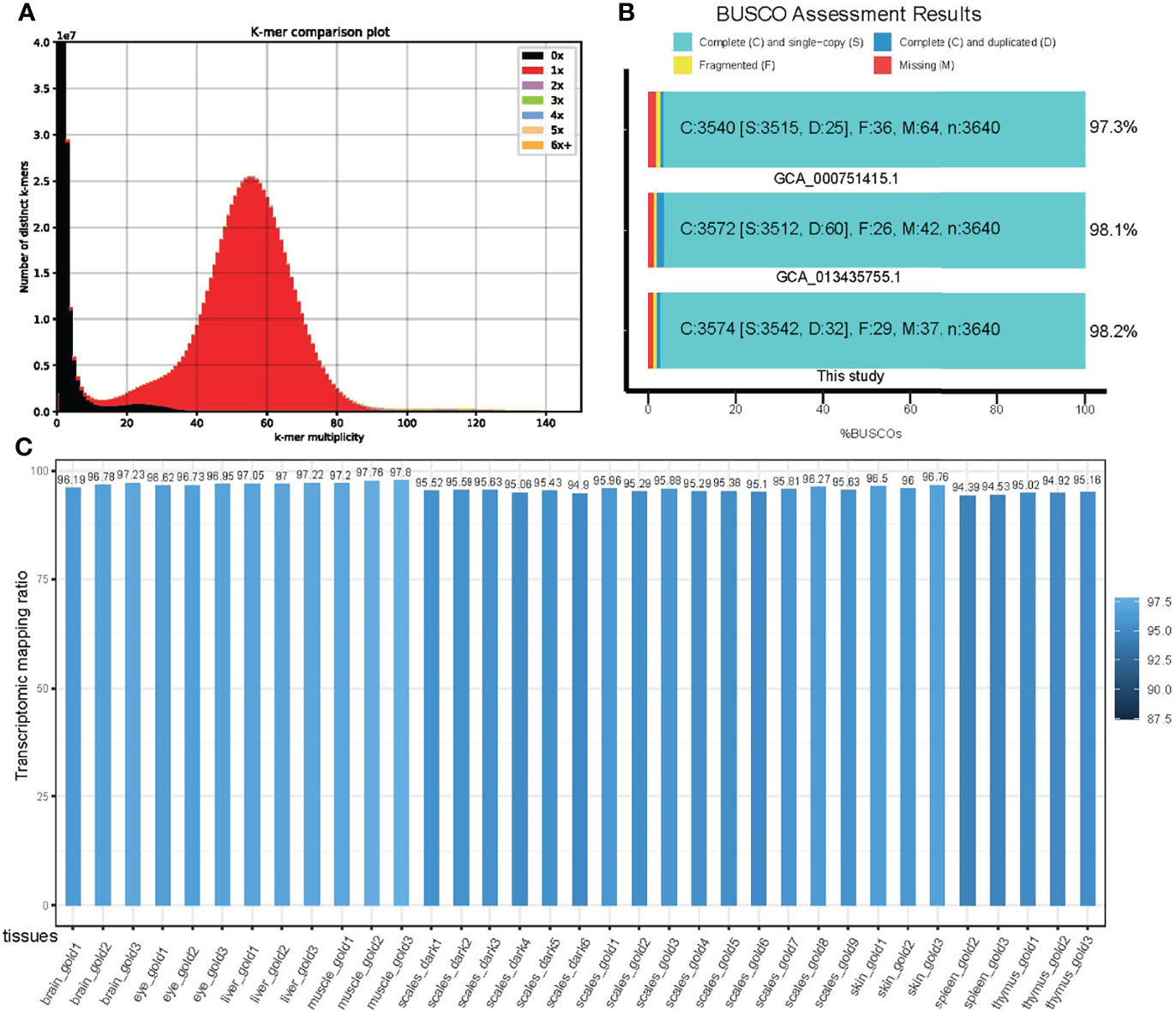
Figure 2 Quality evaluation of the genome assembly of Midas cichlid. (A) A 31-mer spectrum comparison between our assembly and short-inserted libraries; (B) BUSCO completeness comparison among our assembly and two other previously reported versions; (C) Mapping ratios of 35 transcriptomes from different tissues and replicates.
For genome elements, we totally annotated 41.03% repeats in the assembled genome of Midas cichlid. Among these repeats, DNA transposons were the most (18.2%) in abundance, Retroelements occupied the second place with 12.54%, and the rest are unknown (see more details about the landscape of repeats in Figure S1). For gene structures, we totally annotated 25,911 protein-coding genes after removal of possible false prediction such as premature stop codons within the preliminary list. The average length of these genes was 1,684.7 (Table 1). We assessed the quality of the final gene set and corresponding deduced proteins using BUSCOs under protein and transcript modes, and their completeness was estimated to be 90.6% and 90%, respectively. These results suggest establishment of a high-quality list of gene annotation, although it is unable to perform a quality comparison between our assembly and the previous versions (GCA_000751415.1 and GCA_013435755.1) due to non-disclosure of gene annotations in the latter datasets.
Identification of DEGs in the Dark-Vs-Gold Comparison
Based on our gene annotation and mapping comparison between dark- and gold-morph transcriptomes, we obtained the transcription level of each gene that was represented by transcripts per kilobase million (TPM). A total of 97 upregulated and 180 downregulated DEGs were obtained (Figures 3A, B). Interestingly, these downregulated DEGs were enriched in many biological processes (such as melanin metabolic process and melanin biosynthetic process), cellular components (such as pigment granule membrane, melanosome membrane, keratin filament, and intermediate filament), and molecular functions (such as serine-type peptidase activity and serine-type endopeptidase activity; Figure 3C). However, no significant GO terms were enriched for the upregulated DEGs.
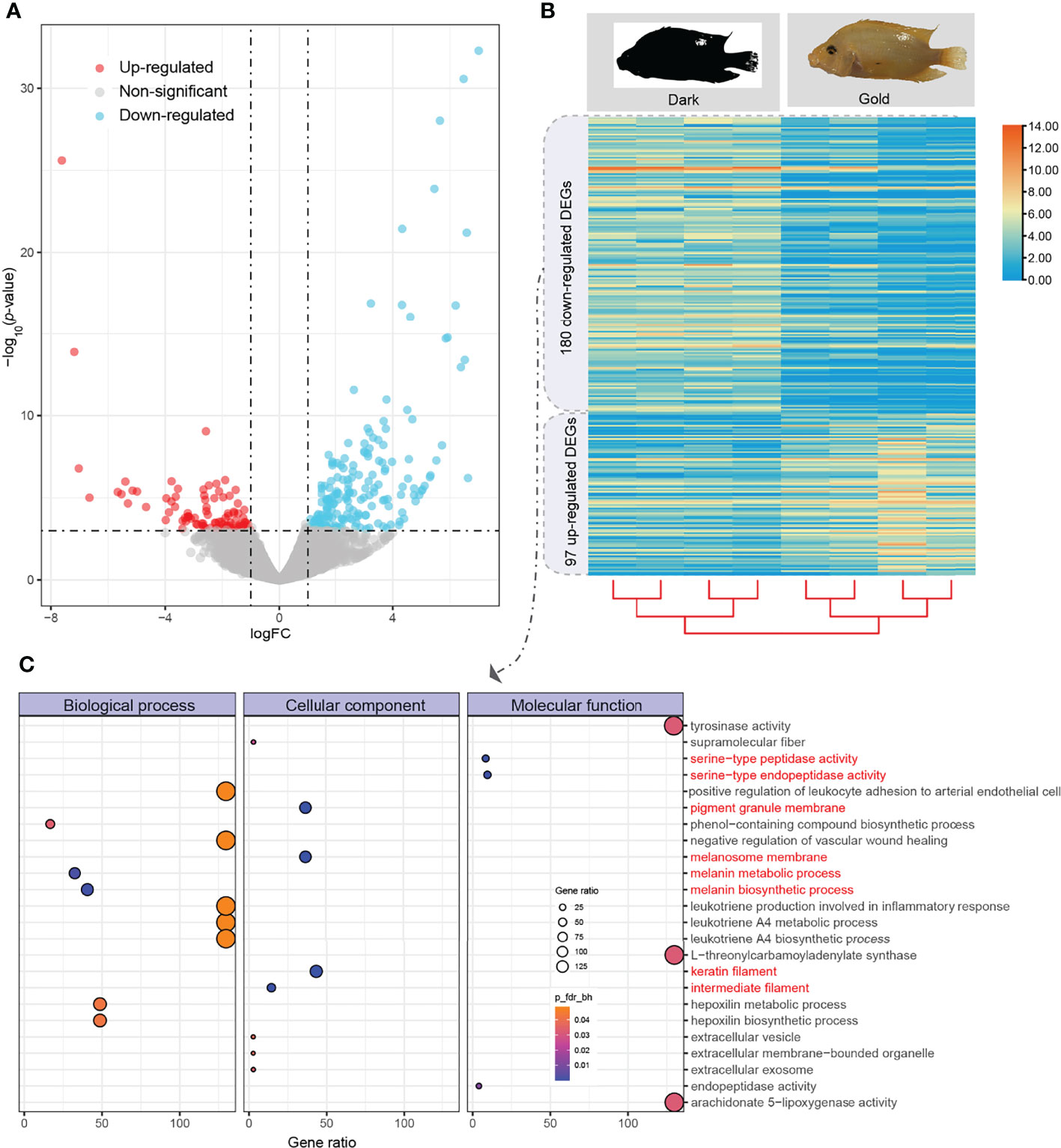
Figure 3 Identification of differentially expressed genes (DEGs) and enrichment of downregulated genes between dark and gold morphs. (A) A volcano plot of up- and downregulated genes (represented by red and blue dots, respectively); (B) A heatmap of transcriptional comparison between dark- and gold-morph individuals; (C) GO enrichment of downregulated DEGs.
Key Functional Modules and Hub Proteins of DEGs
After construction of protein-protein interaction (PPI) networks using those proteins derived from DEGs (Figures 4A, C), we identified one (Figure 4B) and two (Figures 4D, E) key functional modules for up- and downregulated DEGs, respectively. The PPI network derived from upregulated DEGs was enriched into a GO term of “regulation of transcription from RNA polymerase II promoter” and three KEGG (kyoto encyclopedia of genes and genomes) pathways including mitogen-activated protein kinases (MAPK) signaling, Toll-like receptor signaling, and gonadotropin-releasing hormone pathways. The inside key functional module contains eight genes, including fosb, jund, cebpb, jun, junbb, fos, junba, and egr1; among them, junbb, fos, junba, and egr1 were further identified as hub genes (Figure 4B).
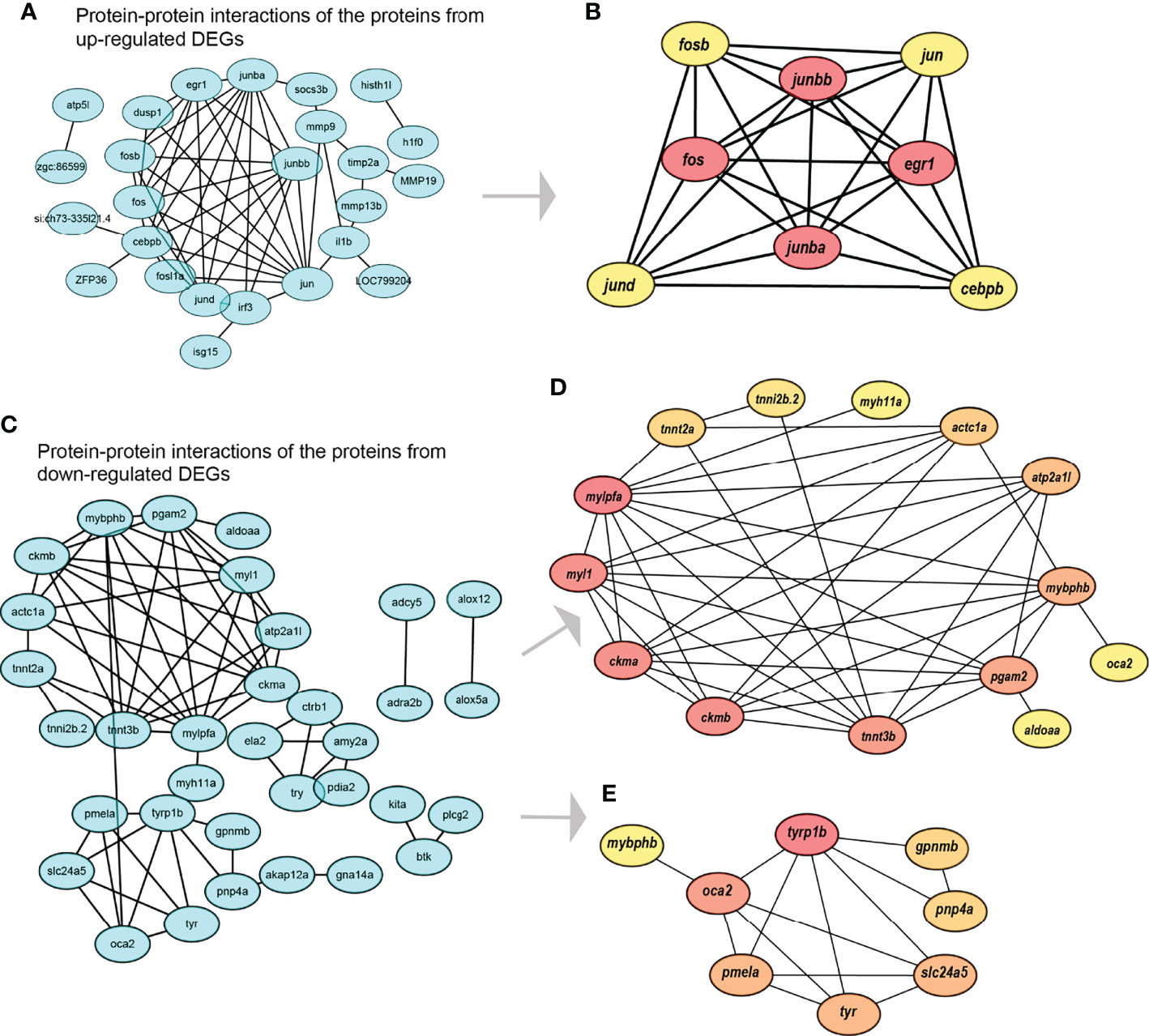
Figure 4 Construction of the protein-protein interaction (PPI) networks along with identification of key functional modules and hub genes. (A) The PPI network of those proteins derived from upregulated DEGs; (B) The identified key functional module and hub genes in (A); (C) The PPI network of those proteins derived from downregulated DEGs; (D) The first key functional module and hub genes in (C); (E) The second key functional module and hub genes in (C).
Similarly, the first key functional module derived from downregulated DEGs (Figure 4D) contains 14 genes, including tnni2b.2, tnnt2a, mylpfa, myl1, ckma, ckmb, tnnt3b, pgam2, aldoaa, mybphb, oca2, atp2a1l, actc1a, and myh11a; among them, mylpfa, myl1, ckma, ckmb, tnnt3b, pgam2, and mybphb are identified as hub genes. The second key functional module derived from downregulated DEGs (Figure 4E) contains eight genes, including tyrp1b, oca2, pmela, mybphb, tyr, slc24a5, pnp4a, and gpnmb; among them, tyrp1b, oca2, pmela, tyr, and slc24a5 are identified as hub genes.
Discussion
Midas cichlids as a model organism attracts biologists’ interests due to its outstanding coloration polymorphism. A non-reference transcriptomic comparison was performed before, revealing genes transcriptional variations during color changes [7]. However, gene specific expression in spatial and temporal patterns may lead to an incomplete scope to observe and identify DEGs. A high-quality reference genome and an effective gene set can help the practical RNA analysis for a better identification of DEGs. Despite the genome of this species was concerned with release of draft details in previous versions (GCA_000751415.1 and GCA_013435755.1), the contiguity of assembled genome (represented by a contig N50) limited both assemblies’ quality (see more comparative details in Table 1). Our new assembly using about 160-fold sequencing-depth data obviously enhanced its contiguity, with improved contig N50 at 10.6 Mb (almost threefold as long as the version of GCA_013435755.1 that is the best in public databases). Other quality evaluations including BUSCOs and LAI evaluation also indicate that our current assembly is much better than the previous ones, thereby benefiting in-depth biological studies on this specific species. In addition, we achieved a high-quality gene set of Midas cichlid, which would be efficient for uncovering key genes related to both coloration polymorphism and other biological characteristics.
In previous reports, 46 DEGs were identified between the two color morphs and the melanosomal pathway was revealed as a key factor to realize morphological differences (Henning et al., 2013). These genes included slc24a5, mreg, pmel, and tyr gene family (Aqmal-Naser and Ahmad, 2020). Our identification of downregulated DEGs is consistent with the previous results, demonstrating the melanosomal pathway plays an essential role for the morph difference in dark-vs-gold comparison. These genes are a part of the second key functional module derived from those downregulated DEGs (Figure 4E). The hub genes within this module were also proven to be crucial for coloration formation or mutation in other organisms. For instance, slc24a5, tyrp1b, oca2, tyr, and pmela were determined to affect human pigmentation (Praetorius et al., 2013); slc24a5, tyrp1b, oca2, gpnmb, and tyr are involved in pigment synthesis pathways in fishes (Braasch et al., 2007); slc24a5, tyrp1b, tyr, and pmela contribute to pigmentation in Xenopus (Williams et al., 2017); slc24a5, tyrp1b, oca2, and tyr participate in molanogenesis of black and white coat colors in mink (Song et al., 2017); slc24a5, oca2, tyr, and pmela are related to coloration of black and red skins in marine crimson snapper (Zhang et al., 2015). These genes are common in diverse species across the tree of life, revealing a relatively conserved genetic mechanism underlying coloration polymorphism in various vertebrates including freshwater and marine fishes.
In comparison to the well-known genes related to coloration polymorphism in the latter key functional module (Figure 4E), the gene functions of the former key module (Figure 4D) are less known. However, two hub genes, myl1 and pgam2, have an influence on muscle contraction (Lin and Lin, 2017), which reveals not only phenotypic differences, but also muscle movement variances, between the dark- and gold morph groups. Interestingly, one of the hub genes, pgam2, was reported to be involved in spermatogenesis (Welch et al., 2000). This triggers an assumption that the fertility between dark- and gold-morph groups may also be different.
In nature, some dark-morph individuals enable a successful transmission to gold-morphs; however, a relatively large ratio of the latter individuals failed to be obtained, although the detailed reason for this failure is still unclear. In the present study, we propose that the possible cues may hide in the upregulated gene list. These hub genes, including egr1, jun, cebpb, jund, fosb, and fos, may play critical roles in regulation of the transcription of RNA polymerase II promoter (Alberini, 2009). This is involved in multiple biological processes, such as fos, jun, and jund participate in the MAPK signaling pathway (Cook et al., 1999; Zhou et al., 2007), fos and jun are involved in Toll-like receptor (TLRs) signaling (Li et al., 2010b; Fan et al., 2019), and egr1 and jun take part in gonadotropin-releasing hormone (GnRH) signaling pathway (Burger et al., 2009; Salisbury et al., 2009).
Despite a direct contribution to the coloration transmission had been assigned to the melanosomal pathway, we predict that those upregulated genes may also devote to a negative upstream regulation or downstream influence on the melanin biosynthesis in Midas cichlid. In previous reports, TLR members have been determined to play distinct roles in affecting melanocytes (i.e., melanin-producing cells), expression and melanin synthesis, and melanosome transport to modulate skin pigmentation (Koike and Yamasaki, 2020). Among them, TLR4 and TLR9 can enhance tyrosinase expression and melanogenesis through the MAPK signaling pathway and some other pathways (Koike and Yamasaki, 2020). Thus, it is reasonable to postulate that these upregulated genes related to the TLR signaling pathway such as fos and jun may play an upstream regulation to melanin biosynthesis in Midas cichlid. On the other hand, the gold-morph Midas cichlid without black skin pigmentation could be triggered by seldom secretion of melanin-concentrating hormone (MCH), which enables a low concentration of melanin in melanophores to result in a bright pallor. It has been demonstrated that MCH can directly inhibit GnRH neurons and affect expression of the GnRH signaling pathway (Wu et al., 2009). Therefore, it is possible that the downregulated DEGs in dark-morph Midas cichlid could lead to an alleviated inhibition of the GnRH signaling pathway. We thus propose that the TLR signal pathway possibly acts as an upstream modulator to affect the melanosomal pathway, causing a downstream influence on the GnRH signaling pathway. These pathways cooperate to differentially regulate the coloration of dark-vs-gold morphs in Midas cichlid. The innate immune stimulation via the TLR signal pathway may therefore work as a key contributor to cause morphological transmission in Midas cichlid.
Conclusions
In this study, with a high sequencing depth we generated an improved genome assembly of Midas Cichlid with a size of 933.5 Mb and a contig N50 of 10.5 Mb, reflecting a good continuity and high quality. Good completeness and contiguity support our current assembly at a reference genome level. The protein-coding gene annotation provided a good reference gene set for comparison of expressional differences of coloration variants, uncovering 97 up- and 180 downregulated DEGs between dark and gold morphs. We screened out five hub genes, tyrp1b, oca2, pmela, tyr, and slc24a5, which are potentially responsible for color determination in Midas cichlid. However, some other hub genes like myl1 and pgam2 have effects on muscle movement or spermatogenesis rather than coloration. The upregulated DEGs constitute a key module associated with MAPK signaling, Toll-like receptor signaling, and gonadotropin-releasing hormone pathways for involvement in multiple biological processes. In summary, we predicted the key factors in determining coloration of Midas cichlid, although exploration of the detailed mechanisms for controlling morph variation requires more investigations. Our new assembly and an improved gene set provide excellent opportunities to not only examine transcriptional regulation of coloration as in the present study, but also to study other biological aspects of Midas cichlid and other fishes from freshwater to marine ecosystems.
Data Availability Statement
The data supporting our findings in this study have been deposited at CNGB Sequence Archive (CNSA) (Guo et al., 2020) of China National GeneBank DataBase (CNGBdb) (Chen et al., 2020b) with accession number CNP0002914. The improved genome assembly and gene annotations are available at https://ftp.cngb.org/pub/CNSA/data2/CNP0002914/CNS0539345/CNA0047398.
Ethics Statement
The animal study was reviewed and approved by Neijiang Normal University.
Author Contributions
XM, QS, and YuL conceived and designed the experiments. YuL, YaL, YiL, YY, ZW, and CQ performed data analysis. YuL and YaL wrote the manuscript. QS and XM revised the manuscript. All authors contributed to the article and approved the submitted version.
Funding
This research was supported by Open Fund of Key Laboratory of Recreational Fisheries, China Ministry of Agriculture and Rural Areas (no. 2019N01), Neijiang Normal University Innovation Team Fund (2021TD03), Guangdong Provincial Special Fund for Modern Agriculture Industry Technology Innovation Team (no. 2022KJ150), and National Freshwater Genetic Resource Center (no. FGRC18537).
Conflict of Interest
The authors declare that the research was conducted in the absence of any commercial or financial relationships that could be construed as a potential conflict of interest.
Publisher’s Note
All claims expressed in this article are solely those of the authors and do not necessarily represent those of their affiliated organizations, or those of the publisher, the editors and the reviewers. Any product that may be evaluated in this article, or claim that may be made by its manufacturer, is not guaranteed or endorsed by the publisher.
Acknowledgments
We are grateful for those previously reported RNA-seq data of Midas cichlid, which are deposited in the NCBI SRA database (including SRR11962298-11962299, SRR11962300, SRR11962302-11962311, SRR11962313-11962314, SRR11962450-11962452, SRR11962454-11962463, SRR11962465-11962472) and used for genome-quality evaluation and comparative transcriptomic analysis.
Supplementary Material
The Supplementary Material for this article can be found online at: https://www.frontiersin.org/articles/10.3389/fmars.2022.950573/full#supplementary-material
References
Abate M. E., Noakes D. L. (2021). The Behavior, Ecology and Evolution of Cichlid Fishes (Dordrecht, Netherlands: Springer).
Alberini C. M. (2009). Transcription Factors in Long-Term Memory and Synaptic Plasticity. Physiol. Rev. 89, 121–145. doi: 10.1152/physrev.00017.2008
Aqmal-Naser M., Ahmad A. B. (2020). First Report of the Hybrid Blood Parrot Cichlid From a Rice Agroecosystem in Seberang Perai Tengah, Penang, Peninsular Malaysia, With Notes on Syntopic Midas Cichlid, Amphilophus Citrinellus (Gunther 1864). BioInvasions. Rec. 9, 588−598. doi: 10.3391/bir.2020.9.3.15
Bader G. D., Hogue C. W. (2003). An Automated Method for Finding Molecular Complexes in Large Protein Interaction Networks. BMC Bioinf. 4, 1–27. doi: 10.1186/1471-2105-4-2
Bao W., Kojima K. K., Kohany O. (2015). Repbase Update, a Database of Repetitive Elements in Eukaryotic Genomes. Mobile. DNA 6, 1–6. doi: 10.1186/s13100-015-0041-9
Benson G. (1999). Tandem Repeats Finder: A Program to Analyze DNA Sequences. Nucleic Acids Res. 27, 573–580. doi: 10.1093/nar/27.2.573
Braasch I., Schartl M., Volff J.-N. (2007). Evolution of Pigment Synthesis Pathways by Gene and Genome Duplication in Fish. BMC Evolution. Biol. 7, 1–18. doi: 10.1186/1471-2148-7-74
Burger L. L., Haisenleder D. J., Aylor K. W. (2009). Regulation of Lhb and Egr1 Gene Expression by GNRH Pulses in Rat Pituitaries is Both C-Jun N-Terminal Kinase (JNK)-And Extracellular Signal-Regulated Kinase (ERK)-Dependent. Biol. Reprod. 81, 1206–1215. doi: 10.1095/biolreprod.109.079426
Chen N. (2004). Using Repeat Masker to Identify Repetitive Elements in Genomic Sequences. Curr. Protoc. Bioinf. 5, 4−10. doi: 10.1002/0471250953.bi0410s05
Chen C., Chen H., Zhang Y., Thomas H. R., Frank M. H., He Y., et al. (2020a). TBtools: An Integrative Toolkit Developed for Interactive Analyses of Big Biological Data. Mol. Plant 13, 1194–1202. doi: 10.1016/j.molp.2020.06.009
Chen F. Z., You L. J., Yang F., Wang L. N., Guo X. Q., Gao F., et al. (2020b). CNGBdb: China national genebank database. Hereditas. 42, 799−809. doi: 10.1093/database/baaa05
Chen Y., Nie F., Xie S. -Q., Zheng Y. F., Dai Q., Bray T., et al. (2021). Efficient Assembly of Nanopore Reads via Highly Accurate and Intact Error Correction. Nat. Commun. 12, 1–10. doi: 10.1038/s41467-020-20236-7
Chen S., Zhou Y., Chen Y., Gu J. (2018). Fastp: An Ultra-Fast All-in-One FASTQ Preprocessor. Bioinformatics 34, i884–i890. doi: 10.1093/bioinformatics/bty560
Chin C., Chen S., Wu H., Ho C., Ko M., Lin C. (2014). Cytohubba: Identifying Hub Objects and Sub-Networks From Complex Interactome. BMC Syst. Biol. 8, 1–7. doi: 10.1186/1752-0509-8-S4-S11
Cook S. J., Aziz N., Mcmahon M. (1999). The Repertoire of Fos and Jun Proteins Expressed During the G1 Phase of the Cell Cycle is Determined by the Duration of Mitogen-Activated Protein Kinase Activation. Mol. Cell. Biol. 19, 330–341. doi: 10.1128/MCB.19.1.330
Ellinghaus D., Kurtz S., Willhoeft U. (2008). LTRharvest, an Efficient and Flexible Software for De Novo Detection of LTR Retrotransposons. BMC Bioinf. 9, 1–14. doi: 10.1186/1471-2105-9-18
Elmer K. R., Fan S., Kusche H., Spreitzer M. L., Kautt A. F., Franchini P., et al. (2014). Parallel Evolution of Nicaraguan Crater Lake Cichlid Fishes via non-Parallel Routes. Nat. Commun. 5, 5168. doi: 10.1038/ncomms6168
Elmer K. R., Kusche H., Lehtonen T. K., Meyer A. (2010). Local Variation and Parallel Evolution: Morphological and Genetic Diversity Across a Species Complex of Neotropical Crater Lake Cichlid Fishes. Philos. Trans. R. Soc. B.: Biol. Sci. 365, 1763–1782. doi: 10.1098/rstb.2009.0271
Elmer K. R., Lehtonen T. K., Meyer A. (2009). Color Assortative Mating Contributes to Sympatric Divergence of Neotropical Cichlid Fish. Evolution.: Int. J. Organic. Evol. 63, 2750–2757. doi: 10.1111/j.1558-5646.2009.00736.x
Fan H., Lv Z., Gan L., Ning C., Li Z., Yang S.M., et al. (2019). A Novel lncRNA Regulates the Toll-Like Receptor Signaling Pathway and Related Immune Function by Stabilizing FOS mRNA as a Competitive Endogenous RNA. Front. Immunol. 10, 838. doi: 10.3389/fimmu.2019.00838
Flynn J. M., Hubley R., Goubert C., Rosen J., Clark A. G., Feschotte C., et al. (2020). RepeatModeler2 for Automated Genomic Discovery of Transposable Element Families. Proc. Natl. Acad. Sci. 117, 9451–9457. doi: 10.1073/pnas.1921046117
Gish W., States D. J. (1993). Identification of Protein Coding Regions by Database Similarity Search. Nat. Genet. 3, 266–272. doi: 10.1038/ng0393-266
Guo X., Chen F., Gao F., Li L., Liu K., You L., et al. (2020). CNSA: A Data Repository for Archiving Omics Data. Database, baaa055,1−5. doi: 10.1093/database/baaa055
Haas B. J., Papanicolaou A., Yassour M., Grabherr M., Blood P. D., Bowden J., et al. (2013). De Novo Transcript Sequence Reconstruction From RNA-Seq Using the Trinity Platform for Reference Generation and Analysis. Nat. Protoc. 8, 1494–1512. doi: 10.1038/nprot.2013.084
Haas B. J., Salzberg S. L., Zhu W., Pertea M., Allen J. E., Orvis J., et al. (2008). Automated Eukaryotic Gene Structure Annotation Using EVidenceModeler and the Program to Assemble Spliced Alignments. Genome Biol. 9, 1–22. doi: 10.1186/gb-2008-9-1-r7
Henning F., Jones J. C., Franchini P. (2013). Transcriptomics of Morphological Color Change in Polychromatic Midas Cichlids. BMC Genomics 14, 1–14. doi: 10.1186/1471-2164-14-171
Hu J., Fan J., Sun Z., (2020). NextPolish: A Fast and Efficient Genome Polishing Tool for Long-Read Assembly. Bioinformatics 36, 2253–2255. doi: 10.1093/bioinformatics/btz891
Kautt A. F., Kratochwil C. F., Nater A., Machado-Schiaffino G., Olave M., Henning F., et al. (2020). Contrasting Signatures of Genomic Divergence During Sympatric Speciation. Nature 588, 106–111. doi: 10.1038/s41586-020-2845-0
Kent W. J. (2002). BLAT—the BLAST-Like Alignment Tool. Genome Res. 12, 656–664. doi: 10.1101/gr.229202
Kim D., Paggi J. M., Park C. (2019). Graph-Based Genome Alignment and Genotyping With HISAT2 and HISAT-Genotype. Nat. Biotechnol. 37, 907–915. doi: 10.1038/s41587-019-0201-4
Klopfenstein D., Zhang L., Pedersen B. S., Ramírez F., Warwick Vesztrocy A., Naldi A., et al. (2018). GOATOOLS: A Python Library for Gene Ontology Analyses. Sci. Rep. 8, 1–17. doi: 10.1038/s41598-018-28948-z
Koike S., Yamasaki K. (2020). Melanogenesis Connection With Innate Immunity and Toll-Like Receptors. Int. J. Mol. Sci. 21,1−18. doi: 10.3390/ijms21249769
Kokot M., Długosz M., Deorowicz S. (2017). KMC 3: Counting and Manipulating K-Mer Statistics. Bioinformatics 33, 2759–2761. doi: 10.1093/bioinformatics/btx304
Kolmogorov M., Yuan J., Lin Y. (2019). Assembly of Long, Error-Prone Reads Using Repeat Graphs. Nat. Biotechnol. 37, 540–546. doi: 10.1038/s41587-019-0072-8
Kratochwil C. F., Kautt A. F., Nater A., Härer A., Liang Y., Henning F., et al. (2022). An Intronic Transposon Insertion Associates With a Trans-Species Color Polymorphism in Midas Cichlid Fishes. Nat. Commun. 13, 1–8. doi: 10.1038/s41467-021-27685-8
Li R., Fan W., Tian G., Zhu H., He L., Cai J., et al. (2010a). The Sequence and De Novo Assembly of the Giant Panda Genome. Nature 463, 311–317. doi: 10.1038/nature08696
Li X., Jiang S., Tapping R. I. (2010b). Toll-Like Receptor Signaling in Cell Proliferation and Survival. Cytokine 49, 1–9. doi: 10.1016/j.cyto.2009.08.010
Lin Z., Lin Y. (2017). Identification of Potential Crucial Genes Associated With Steroid-Induced Necrosis of Femoral Head Based on Gene Expression Profile. Gene 627, 322–326. doi: 10.1016/j.gene.2017.05.026
Maan M. E., Sefc K. M. (2013). Colour Variation in Cichlid Fish: Developmental Mechanisms, Selective Pressures and Evolutionary Consequences. Semin. in Cell Dev. Biol. 24, 6−7. doi: 10.1016/j.semcdb.2013.05.003
Mapleson D., Garcia Accinelli G., Kettleborough G., Wright J., Clavijo B. J. (2017). KAT: A K-Mer Analysis Toolkit to Quality Control NGS Datasets and Genome Assemblies. Bioinformatics 33, 574–576. doi: 10.1093/bioinformatics/btw663
Oldfield R. G. (2011). Aggression and Welfare in a Common Aquarium Fish, the Midas Cichlid. J. Appl. Anim. Welfare. Sci. 14, 340–360. doi: 10.1080/10888705.2011.600664
Ou S., Chen J., Jiang N. (2018). Assessing Genome Assembly Quality Using the LTR Assembly Index (LAI). Nucleic Acids Res. 46, 126–126. doi: 10.1093/nar/gky730
Ou S., Jiang N. (2018). LTR_retriever: A Highly Accurate and Sensitive Program for Identification of Long Terminal Repeat Retrotransposons. Plant Physiol. 176, 1410–1422. doi: 10.1104/pp.17.01310
Pertea M., Pertea G. M., Antonescu C. M., Chang T.-C., Mendell J. T., Salzberg S. L. (2015). StringTie Enables Improved Reconstruction of a Transcriptome From RNA-Seq Reads. Nat. Biotechnol. 33, 290–295. doi: 10.1038/nbt.3122
Praetorius C., Grill C., Stacey S. N., Metcalf A. M., Gorkin D. U., Robinson K. C., et al. (2013). A Polymorphism in IRF4 Affects Human Pigmentation Through a Tyrosinase-Dependent MITF/TFAP2A Pathway. Cell 155, 1022–1033. doi: 10.1016/j.cell.2013.10.022
Price A. L., Jones N. C., Pevzner P. A. (2005). De Novo Identification of Repeat Families in Large Genomes. Bioinformatics 21, 351–358. doi: 10.1093/bioinformatics/bti1018
Ranallo-Benavidez T. R., Jaron K. S., Schatz M. C. (2020). GenomeScope 2.0 and Smudgeplot for Reference-Free Profiling of Polyploid Genomes. Nat. Commun. 11, 1–10. doi: 10.1038/s41467-020-14998-3
Robinson M. D., Mccarthy D. J., Smyth G. K. (2010). Edger: A Bioconductor Package for Differential Expression Analysis of Digital Gene Expression Data. Bioinformatics 26, 139–140. doi: 10.1093/bioinformatics/btp616
Ruan J., Li H. (2020). Fast and Accurate Long-Read Assembly With Wtdbg2. Nat. Methods 17, 155–158. doi: 10.1038/s41592-019-0669-3
Salisbury T. B., Binder A. K., Grammer J. C., Nilson J. H. (2009). GnRH-Regulated Expression of Jun and JUN Target Genes in Gonadotropes Requires a Functional Interaction Between TCF/LEF Family Members and β-Catenin. Mol. Endocrinol. 23, 402–411. doi: 10.1210/me.2008-0370
Sefton M. (2017). Investigating the Developmental and Gene Regulatory Basis of Color Diversification in Cichlid Fish: A Framework for Evolutionary Developmental Studies in the Midas Cichlid Species Complex (Amphilophus Spp.) (Germany: Doctoral dissertation, University of Konstanz).
Seppey M., Manni M., Zdobnov E. M. (2019). BUSCO: Assessing Genome Assembly and Annotation Completeness. Gene predict., 227–245. doi: 10.1007/978-1-4939-9173-0_14
Shannon P., Markiel A., Ozier O., Baliga N. S., Wang J. T., Ramage D., et al, (2003). Cytoscape: A Software Environment for Integrated Models of Biomolecular Interaction Networks. Genome Res. 13, 2498–2504. doi: 10.1101/gr.1239303
Slater G. S. C., Birney E. (2005). Automated Generation of Heuristics for Biological Sequence Comparison. BMC Bioinf. 6, 1–11. doi: 10.1186/1471-2105-6-31
Song X., Xu C., Liu Z., Yue Z., Liu L., Yang T., et al. (2017). Comparative Transcriptome Analysis of Mink (Neovison Vison) Skin Reveals the Key Genes Involved in the Melanogenesis of Black and White Coat Colour. Sci. Rep. 7, 1–11. doi: 10.1038/s41598-017-12754-0
Stanke M., Keller O., Gunduz I., Hayes A., Waack S., Morgenstern B. (2006). AUGUSTUS: Ab Initio Prediction of Alternative Transcripts. Nucleic Acids Res. 34, 435–439. doi: 10.1093/nar/gkl200
Szklarczyk D., Franceschini A., Kuhn M., Simonovic M., Roth A., Minguez P., et al. (2010). The STRING Database in 2011: Functional Interaction Networks of Proteins, Globally Integrated and Scored. Nucleic Acids Res. 39, 561–568. doi: 10.1093/nar/gkq973
Thomas B. T. (Ed.) (1976). Investigations of the Ichthyofauna of Nicaraguan Lakes (University of Nebraska-Lincoln, Nebraska, USA: University of Nebraska).
Welch J. E., Brown P. L., O’brien D. A., Magyar P. L., Bunch D. O., Mori C., et al. (2000). Human Glyceraldehyde 3-Phosphate Dehydrogenase-2 Gene is Expressed Specifically in Spermatogenic Cells. J. androl. 21, 328–338. doi: 10.1002/j.1939-4640.2000.tb02111.x
Williams R. M., Winkfein R. J., Ginger R. S., Green M. R., Schnetkamp P. P., Wheeler G. N. (2017). A Functional Approach to Understanding the Role of NCKX5 in Xenopus Pigmentation. PloS One 12, e0180465. doi: 10.1371/journal.pone.0180465
Wu M., Dumalska I., Morozova E., Van Den Pol A., Alreja M. (2009). Melanin-Concentrating Hormone Directly Inhibits GnRH Neurons and Blocks Kisspeptin Activation, Linking Energy Balance to Reproduction. Proc. Natl. Acad. Sci. U.S.A. 106, 17217–17222. doi: 10.1073/pnas.0908200106
Wu T. D., Watanabe C. K. (2005). GMAP: A Genomic Mapping and Alignment Program for mRNA and EST Sequences. Bioinformatics 21, 1859–1875. doi: 10.1093/bioinformatics/bti310
Zhang Y., Wang Z., Guo Y., Liu L., Yu J., Zhang S., et al. (2015). Morphological Characters and Transcriptome Profiles Associated With Black Skin and Red Skin in Crimson Snapper (Lutjanus Erythropterus). Int. J. Mol. Sci. 16, 26991–27004. doi: 10.3390/ijms161126005
Keywords: Midas cichlid, morphological polymorphism, high-quality genome, melanin, transcriptome
Citation: Lv Y, Li Y, Liu Y, Wen Z, Yang Y, Qin C, Shi Q and Mu X (2022) An Updated Genome Assembly Improves Understanding of the Transcriptional Regulation of Coloration in Midas Cichlid. Front. Mar. Sci. 9:950573. doi: 10.3389/fmars.2022.950573
Received: 23 May 2022; Accepted: 20 June 2022;
Published: 26 July 2022.
Edited by:
Hongyu Ma, Shantou University, ChinaReviewed by:
Changwei Shao, Yellow Sea Fisheries Research Institute (CAFS), ChinaKarsoon Tan, Shantou University, China
Copyright © 2022 Lv, Li, Liu, Wen, Yang, Qin, Shi and Mu. This is an open-access article distributed under the terms of the Creative Commons Attribution License (CC BY). The use, distribution or reproduction in other forums is permitted, provided the original author(s) and the copyright owner(s) are credited and that the original publication in this journal is cited, in accordance with accepted academic practice. No use, distribution or reproduction is permitted which does not comply with these terms.
*Correspondence: Qiong Shi, c2hpcWlvbmdAZ2Vub21pY3MuY24=; Xidong Mu, bXV4ZEBwcmZyaS5hYy5jbg==
†These authors have contributed equally to this work