- 1School of Marine Sciences/Guangdong Provincial Key Laboratory of Marine Resources and Coastal Engineering, Sun Yat-sen University & Southern Marine Science and Engineering Guangdong Laboratory (Zhuhai), Zhuhai, China
- 2Shenzhen Key Laboratory of Marine Archaea Geo-Omics, Southern University of Science and Technology, Shenzhen, China
- 3Department of Ocean Science & Engineering, Southern University of Science and Technology, Shenzhen, China
- 4Southern Marine Science and Engineering Guangdong Laboratory (Guangzhou), Guangzhou, China
Bacterial communities and porewater dissolved organic matter (DOM) pool are intimately interactive in sedimentary environments. Estuarine coastal regions are an interactive area between terrestrial and marine influences in terms of DOM origins and freshness. Yet, we know little about the relationships between the bacterial communities and DOM in those regions. In this study, porewater DOM samples were collected from 42 sites in the coastal East China Sea. The porewater DOM optical properties were determined by fluorescence and absorption spectra, while the corresponding bacterial community compositions of those sediments were examined by 16S rRNA gene high-throughput sequencing. The results showed that bacterial species richness was positively correlated with multiple terrestrial indicators based on the optical properties of DOM, which implied that heterogeneous DOM from terrestrial origins might harbor a wider spectrum of bacterial taxa in marine sediments. The analysis of the co-occurrence network of the bacterial communities showed that the edges and density for samples with low DOM freshness were 3.4 times and 3 times those for the samples with high DOM freshness, respectively. This suggested that the connection among the bacterial taxa under the lower DOM freshness condition were enhanced and that reduced freshness of DOM may encourage more complimentary utilization of resources. The findings provide a new insight into such interactive processes of heterogeneous organic matter utilization meditated by microorganisms in coastal sediments.
Introduction
Marine dissolved organic matter (DOM) represents one of the largest reservoirs of reduced carbon on the surface of the earth, matching the size of the atmospheric reservoirs (Hansell et al., 2009). In marine sediments, DOM undergoes a series of transformations due to complex interaction with other components in oceans and plays an important role in global carbon cycling (Chen and Hur, 2015). DOM in sediments is traditionally considered to be recalcitrant (Weston and Joye, 2005; Chipman et al., 2010; Robador et al., 2010). However, some recent studies revealed that some parts of DOM in marine sediments have the potential to be bio-reactive (Rossel et al., 2016; Cai et al., 2019; Tobias-Hünefeldt et al., 2021). Since prokaryotes (especially for bacteria) are the pivotal component responsible for transformation of DOM in marine sediments (Wang et al., 2021), it is important to investigate the interactions, between DOM and bacteria in marine sediments. For all heterotrophic and some autotrophic bacteria in sediments, stepwise processes during the decomposition of sedimentary DOM sever as their main carbon and electron donors source (Burdige and Komada, 2015; Mahmoudi et al., 2017), and porewater DOM was believed as the most active delegate in sedimentary DOM pools (Derrien et al., 2019). Two tangled groups of bacteria processes could be summarized from conceptual frameworks: consumption and production. On the one hand, bacteria could utilize DOM as both carbon sources and electron donors (Coates et al., 2002; Ijiri et al., 2012). DOM could be either remineralized or incorporated into bacterial biomass through bacterial metabolism (Azam et al., 1983; Ducklow et al., 1986; Goldman and Dennett, 2000). On the other hand, chemoautotrophs could transform inorganic matter into DOM directly, while heterotrophs also contributed to DOM in the form of extracellular secretion like hydrolytic enzymes and siderophores (Vraspir and Butler, 2009; Arnosti, 2011). In most cases, consumption and production were coupled. For instance, microbial carbon pump proposed the important role of microbes in transforming the labile DOM into refractory DOM (Jiao et al., 2010).
Many studies have found that specific DOM compounds or compound classes may have the effect to shape specific bacterial groups, while different bacteria have preferences for specific DOM portions (Cottrell and Kirchman, 2000; Dyhrman et al., 2009; Poretsky et al., 2010; Amaral et al., 2016). However, specific DOM substrates cannot represent the chemical complexity of natural DOM for lack of environmental relevance. Similarly, natural bacterial communities generally have high biodiversity and hence possess diverse ecosystem functioning (Smith et al., 2018). Therefore, it is necessary to give both DOM and bacteria more thorough descriptions in order to have a holistic and integrative understanding of their relationship. Although it is difficult to couple complexities of DOM and bacterial community considering chemodiversity and bacterial diversity, recent advances in DOM characterization and high-throughput sequencing make it possible to do comprehensive analyses about the relationship between DOM and bacteria. With the help of optical approaches, chromophoric DOM (CDOM) could be identified from the bulk DOM pool and used to indicate properties such as molecular weight (MW), aromaticity, etc. (Li and Hur, 2017). A part of CDOM that emits fluorescence exposed to ultraviolet light is named fluorescent DOM (FDOM) and could be further characterized as different components with fluorescence spectroscopy and parallel factor analysis (PARAFAC) (Stedmon and Bro, 2008). Meanwhile, the popularity of 16S rRNA gene sequencing and associated marker-based prediction tools make exploring bacterial community compositions and structure a handy and efficient work.
For bacterial community compositions, LaBrie et al. (2021) employed optical-derived properties of DOM to reveal that bacterial spatial clusters were better related with more complex DOM. Moreover, bacterial community compositions were reported to be associated with the aromaticity of DOM indicated by the spectral slope and showed depth-specific patterns in the Atlantic Ocean (Guerrero-Feijóo et al., 2017). A laboratory study confirmed the validity of optical properties in inferring DOM sources even after intense biological modification, and it has been reported that DOM sources had impacts on extracellular enzyme activities of bacterial communities (Freixa et al., 2016; Hansen et al., 2016). Besides, the lability or freshness of DOM was believed to be a key driver of bacterial metabolism (López-Urrutia and Morán, 2007; Nguyen et al., 2012). After high-labile DOM was taken up by microbes in the epipelagic zone, a small portion of DOM may survive the thorough remineralization with a certain degree of lability, which would be transported to the deep sea and consequently further utilized by deep-sea microbial communities (Carlson et al., 2004). The destiny of fresh DOM is of great importance for the global carbon cycle and it is basically decided by bacterial uptake (Puddu et al., 2003). The uptake rates depend not only on the state of the local bacterial community, but also on the chemical composition of organic substrates (Moriarty and Bell, 1993), which reflect linkages between DOM and bacteria. Apart from modifying the bacteria community with chemical compounds traded between DOM and bacteria, interspecies relationships and co-occurrence patterns within the bacterial community may also change due to the metabolic network variance stimulated by DOM (Bai et al., 2020). Zhou et al. (2021c) found that elevated bioavailable DOM induced by eutrophication enhanced the modularity of bacterial interspecies networks. Based on an incubation experiment, there were different dominant taxa in co-occurrence networks associated with different FDOM components (Li et al., 2020b). Also, the quantity of phytoplankton-derived DOM was reported to affect the bacterial niche partitioning (Sarmento et al., 2016).
Compared with water column DOM pools, biogeochemical processes of DOM pool in sediments have been rarely revealed. As the most reactive regions within sediments (Fox et al., 2018), pore waters were believed to be an indispensable intermediate of sedimentary carbon preservation and an important part for estimating the global carbon budget (Burdige, 2001; Chen and Hur, 2015). Some previous studies have reported porewater DOM as a net upward benthic efflux to overlying water (Wang et al., 2014; Burdige and Komada, 2015). Compared to the water column, the DOM pool in sediment pore waters showed a higher average molecular weight, and a higher humidification index (HIX) (Ziegelgruber et al., 2013; Chen and Hur, 2015). There were significant differences in fluorescent components and their distribution between sediment pore water and water column (Chen and Hur, 2015; Li et al., 2015; Li et al., 2020a). While abovementioned studies have reported remarkable findings, DOM-bacteria processes in sediments remain poorly resolved and studied in this field.
Among the East Asian margin seas, the East China Sea (ECS) receives a great amount of terrestrial organic matter from rivers (Yang et al., 2011). Coupled with the complex current system and strong ocean-land interactions, ECS provides a broad gradient for our study. Considering the fact that porewater DOM is the prevailing substrate for bacteria in marine sediments, we hypothesized that the bacterial communities might be significantly controlled by the varied freshness degrees or terrestrial contributions quality of the porewater DOM in the ECS. The objectives of our study thus were to (1) clarify variations in optical-derived properties of DOM and the bacterial community and (2) elucidate the linkage between these variations in coastal sediments.
Materials and Methods
Study Area and Sample Collection
A total of 42 samples from 10 section were collected from the coastal area of ECS with a grab sampler during a cruise from August to September 2015 (Figure 1). We collected surface sediment samples (ca. 10cm) from 8-66 m depth using a CTD (Sea-bird, USA). Salinity and dissolved oxygen of overlying waters varied between 17.65‰ – 34.42‰ and 1.063 – 6.098 mg/L, respectively. Samples were stored in the refrigerator of -20°C and protected from light until further processing. Total organic carbon (TOC), carbon isotope, chlorin content and glycerol dialkyl glycerol tetraethers (GDGTs) content measurement were performed as described in our previous studies (Li et al., 2020a).
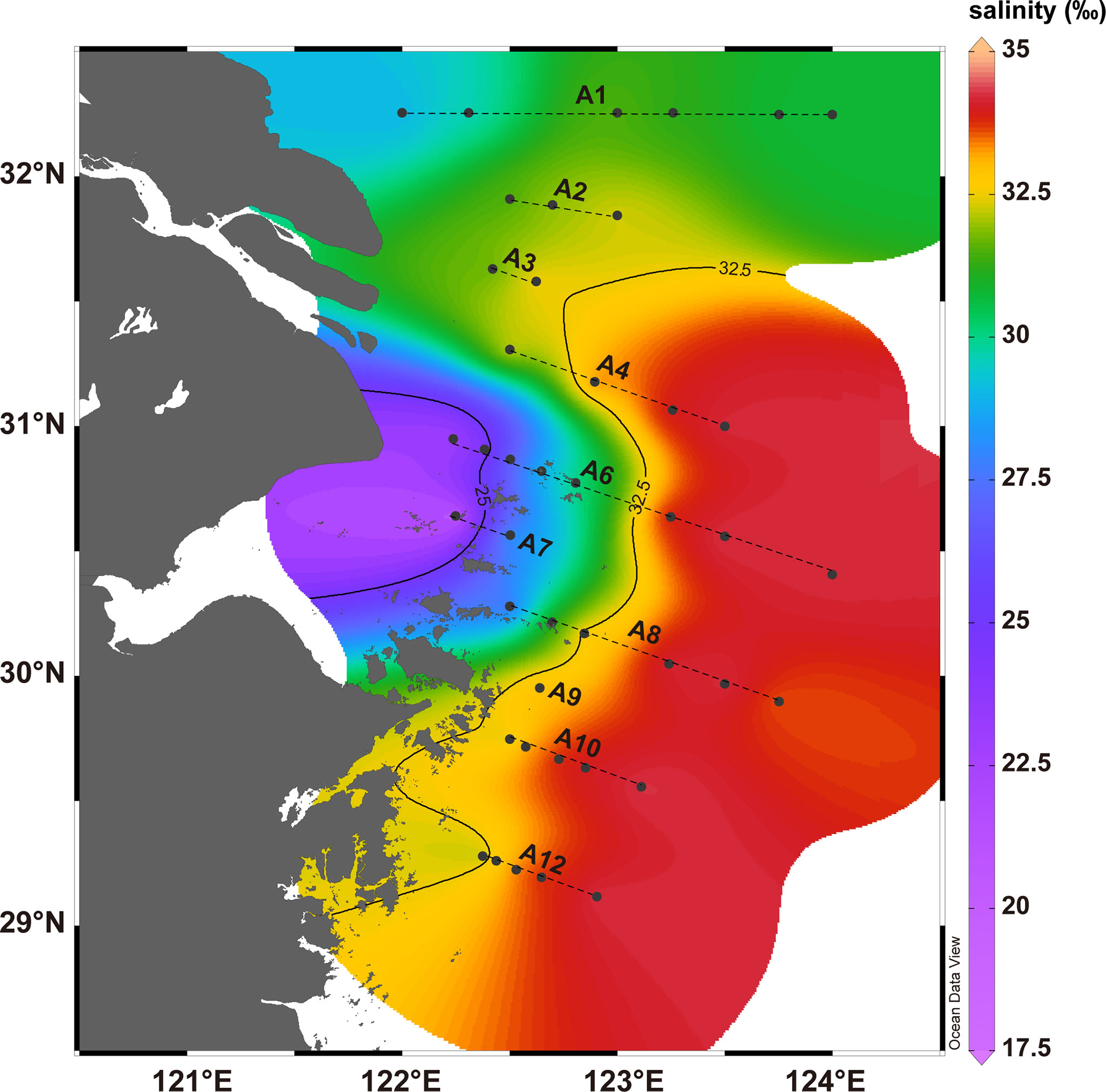
Figure 1 Map of the study area with 42 sampling sites and the spatial distribution of salinity of bottom water in the East China Sea (Ocean Data View v5.2.1).
DNA Extraction, Sequencing and Sequence Analysis
Following the manufacturer’s protocol, 0.5 g of sediment samples from each site were used to extract DNA with FastDNA Spin Kit for Soil (MP Biomedicals, USA). The V3-V4 regions of bacterial 16S rRNA genes were amplified with primers 338F (5′-ACTCCTACGGGAGGCAGCA-3′) and 806R (5′-GGACTACNVGGGTWTCTAAT-3′). The pair-end sequencing was performed on the Illlumina MiSeq platform by Shanghai Majorbio Bio-pharm Technology Co., Ltd (Shanghai, China). ‘Quantitative Insights into Microbial Ecology’ pipeline (QIIME2 2019.7.0 release) (Bolyen et al., 2019) was employed for sequence analysis together with R. DADA2 (Callahan et al., 2016) plugin in QIIME2 was applied to denoise sequencing reads and produce high-resolution amplicon sequence variants (ASVs) for subsequent analysis. Taxonomic analysis was performed with a classify-sklearn classifier trained against SILVA 16S rRNA gene reference database (Quast et al., 2012). Following quality filtering, a total of 650,936 reads from 16,741 ASVs were collected with an average of 15,498.48 reads per sample. To improve downstream diversity and composition analyses, sequences were rarefied to the lowest number of sequences per sample (n = 11,455 sequences). Thus, there were 16,703 ASVs removed (0.23% of the total number of ASVs). Additionally, richness estimators in alpha-diversity indices including Chao1, the observed richness (sobs), ace and phylogenetic diversity (pd) were also calculated. Slopes of rarefaction curves based on sobs gradually decreased increasing sequencing depth, and finally tended flatten out (Figure S1), indicating that the sequencing depth of samples was adequate. Phylogenetic Investigation of Communities by Reconstruction of Unobserved States (PICRUSt2, v2.2.0-b) were used to predict the functional potentials of the bacterial communities. Raw sequence data are available from the NCBI Sequence Read Archive under accession number PRJNA761364.
DOM Optical Characterization and Analysis
About 20 g of sediments were used to extract pore water samples by centrifugation at 4000 rpm for 20 min. After centrifugation, pore waters collected were filtered with 0.2-mm polyethersulfone membranes (Millipore Millex-GP, USA). CDOM absorbance spectra were obtained by UV2600 UV-Vis spectrophotometer (Shimadzu, Japan). The scanning range was 200-800 nm with 0 nm increments in a 1-cm cuvette and Milli-Q water (Milli-Q Integral 10, Millipore, France) was used as blank control. The CDOM absorption coefficient at the wavelength of 254 nm and 340 nm (a254 and a340) were used to estimate the CDOM concentration, with a254 refers to information of relatively simple compounds and a340 refer to that of complex molecules (Stedmon and Nelson, 2015). CDOM spectral slope from 275 nm to 295 nm (S275–295) and spectral slope ratio (SR) were calculated as suggested by (Helms et al., 2008) and both showed negative correlations with CDOM MW. We employed Excitation-emission matrices (EEMs, Figure S2) together with PARAFAC (Stedmon and Bro, 2008) to characterize FDOM as five components (C1-C5, Figure S3). Detailed information about FDOM characterization, HIX, fluorescence index (FI) are available from our previous study (Li et al., 2020a). Biological index (BIX) was calculated at the excitation wavelength of 310nm, by dividing the fluorescence intensity at the emission wavelength of 380nm by that at 430nm. BIX was a freshness index, with higher values indicating a greater proportion of recently autochthonous contribution fresh DOM and samples with BIX > 1 being considered to be marine autochthonous (Huguet et al., 2009). We categorized 42 study sites into two groups: Group Fresh (n = 26) with BIX > 1 and Group Non-Fresh (n = 16) with BIX < 1.
Statistical Analysis
To investigate the distance among samples or between different groups based on their bacterial community, non-metric multidimensional scaling ordination analysis (NMDS; Bray–Curtis distance) was conducted using the function ‘metaMDS’ within the ‘vegan’ package in R. Also, the significance of differences between Group N and Group F based on NMDS was tested with analysis of similarities (ANOSIM) with the function ‘anosim’ within the ‘vegan’ package in R (Oksanen et al., 2013). Levins’ niche breadth index for each bacterial taxon (Levins, 2020) and niche breadth at the community level (Bcom) were calculated as proposed by Jiao et al. (2020)
Before analyzing the relationship between environmental variables and bacterial communities, variables with variance inflation factors larger than 10 were removed to avoid collinearity. Then, detrended correspondence analysis (DCA) was carried out to decide whether redundancy analysis (RDA) or canonical correlation analysis (CCA) should be adopted for downstream analysis. In this study, RDA was selected in all analyses since the longest lengths of the gradient in DCA were less than 3. Spearman correlation coefficients and their corresponding p values were calculated using the ‘Hmisc’ package in R and visualized using the ‘corrplot’ package in R (Wei et al., 2017; Harrell Jr and Harrell Jr, 2019). For relationships between environmental factors and bacterial assemblages, Mantel and partial Mantel tests were performed with ‘vegan’ (Oksanen et al., 2013). Due to the data requirements during calculations, some analysis involved with δ13C employed the negative value of δ13C (labeled as -δ13C). To explore the co-occurrence network of bacteria, pairwise Spearman correlations were calculated and networks were visualized with Gephi v0.9.2. Moreover, topological roles of nodes within the networks were decided by within-module degree (Zi) and Participation Coefficient (Pi) calculated with ‘GAINT’ plugin in Cytoscape v3.8.2. A linear discriminant analysis Effect Size (LEfSe) analysis was used to identify taxa that were significantly different within two groups.
Results
General Pattern of Optical Parameters
A suite of optical parameters of the pore water from the sediments were derived by fluorescence and absorption spectra. The absorbance-derived quantitative parameters a254 and a340 showed increasing trends (r = 0.498 and r = 0.431, respectively; p < 0.01; Figure S4) from coastal area to open ocean, proclaiming higher concentration of CDOM in more off-shore sites. In brief, five components were identified with EEMs-PARAFAC, two of which were humic-like and three are protein-like. For the remainder of this article, we will refer to C1-C5 as the component itself or its fluorescence intensity of the five components: C1_r-C5_r, Humic_r and Protein_r as the proportions of total intensity (i.e., relative intensity) of the five components, humic-like components and protein-like components, respectively. The fluorescence-derived parameters HIX (0.32 – 3.61) and FI (1.07 – 2.08) indicated that this FDOM pool consisted of both terrestrial and marine end-members. Freshness index BIX ranged from 0.42 to 1.37, with an average value of 1.05. In total, 26 pore water samples had BIX values greater than 1, indicating that they were mainly of autochthonous origin and contained fresher DOM (Huguet et al., 2009). The other 16 samples, however, had BIX values lower than 1 and may contain more non-fresh DOM (Figure S5).
Bacterial Community Composition and Abundance
All ASVs were assigned to more than 50 phyla, among which five dominant phyla were Proteobacteria (25.66%), Chloroflexi (15.2%), Desulfobacterota (11.22%), Actinobacteriota (10.65%) and Acidobacteriota (9.96%) (Figure 2A). All dominant phyla had been reported in marine sedimentary environments before (Purdy et al., 2002; Li et al., 2009). At the genus level, 743 genera were classified. One genus in Order Actinomarinales was the most abundant (5.59%), followed by genus Woeseia (5.29%) from Family Woeseiaceae and genus Subgroup_10 (3.04%) from Family Thermoanaerobaculaceae (Figure 2B). Most genera were low in relative abundance, but they accounted for 47.32% of the total.
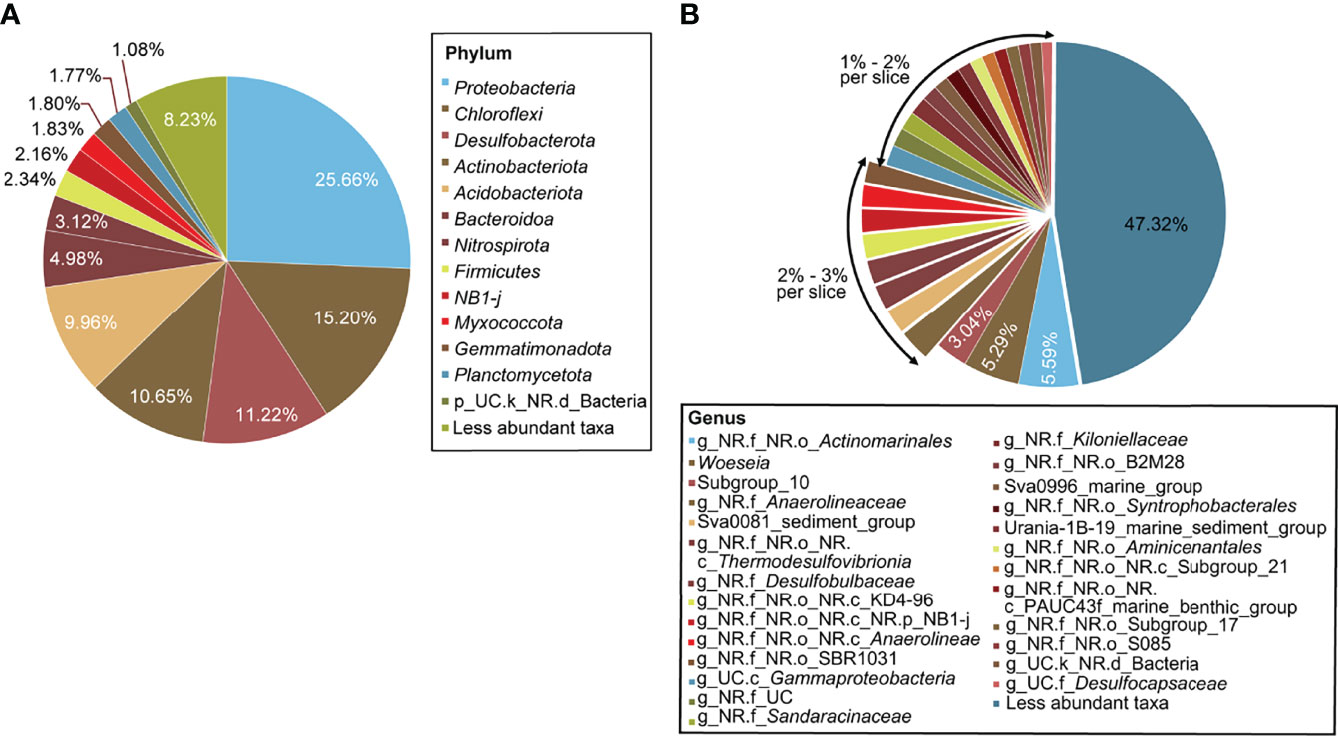
Figure 2 Pie charts of bacterial community compositions of 42 sites classified at the phylum (A) and the genera level (B). Only taxa with relative abundances more than 1% are shown and taxa at less than 1% are represented as less abundant taxa. k, kingdom; p, phylum; c, class; o, order; f, family; g, genus; NR, norank; UC, unclassified.
Heatmaps at the phylum and genus level (Figures S6A, B) were constructed to illustrate the similarity between samples or taxa. At the phylum level, Proteobacteria was the most distant one and it occupied a single cluster. Dominant taxa Chloroflexi was clustered together with Desulfobacterota, Actinobacteriota and Acidobacteriota. For genera, four clusters were identified without any overt patterns. On the basis of both levels, the cluster analysis indicated no tendencies for different samples to be potentially grouped according to the relative distance from the land. According to qPCR analysis, samples from ECS contained (1.3 ± 1.5) × 1010 bacterial copies per gram sediment, ranging from 7.1× 1010 (copies/g sediment) at site A8_2 to 4.9× 108 (copies/g sediment) at site A3_2. No clear spatial variation of qPCR results was reported. As suggested by Lou et al. (2018), the abundance of each taxon in the soil bacterial community could be estimated by multiplying total bacterial quantities (the copy number of total bacteria from qPCR) and the corresponding relative abundance from high-throughput sequencing. Among 42 sites, Proteobacteria was the most dominant phylum with 3.8× 108 (copies/g sediment) on average and a yet no-rank genus in Order Actinomarinales the most dominant genus with 8.6× 108 (copies/g sediment) on average. NMDS analysis showed that 42 sites could not be grouped as a separated community with many sharing sites between two clusters (Figures 3A, B).
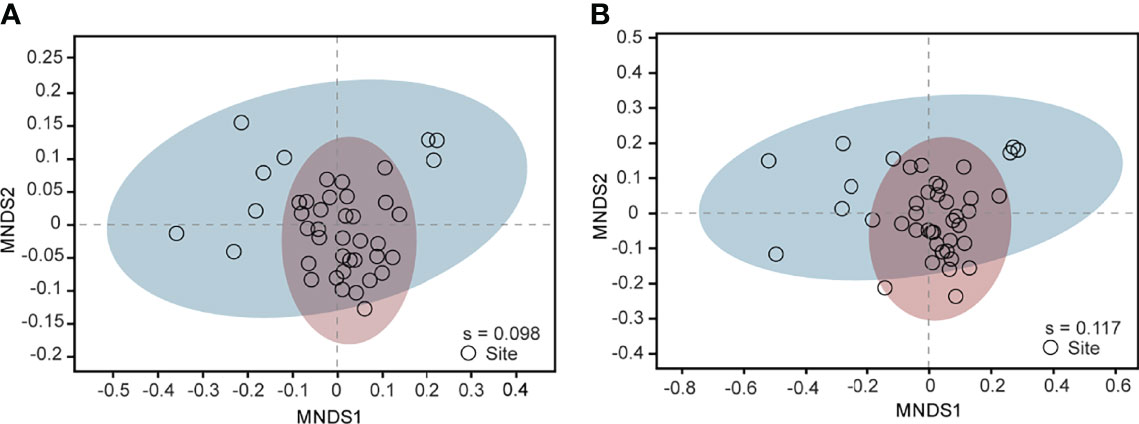
Figure 3 MNDS ordinations represent the dissimilarities according to Bray-Curtis distance among samples based on bacterial community compositions at the phylum (A) and the genus level (B). Ellipse with different colors suggest individual communities (95% confidence interval). s, stress.
Environmental Variables and Their Relationships With the Bacterial Community
The VIF analysis retained 18 variables for further analysis. RDA was carried out on these 18 environmental variables and bacteria relative abundances from the phylum to genus level, and then factors with p < 0.05 in RDA were applied to the Mantel test (Table 1), suggesting that relative abundances of bacterial community compositions at all levels were positively correlated with their corresponding factor matrix determined after processes above. Unlike relative abundances, the bacterial abundance did not show any significant correlation with the corresponding factor matrix at all levels. It seemed that relative abundances of bacterial community could better reflect the effects of environmental variables.
To further investigate the relationship between bacteria and environmental variables, a correlation heatmap (Figure 4) was potted between 11 factors and the bacterial relative abundance of the 30 most abundant orders, based on the number of significant factors. Obvious correlations were noticed universally between bacterial relative abundances and factors that survived the elimination process. Among those factors, longitude and latitude reflect the location of the sampling site and therein the tradeoff between terrestrial and marine contributions contribution to sedimentary organic matter. Crenarchaeol and branched GDGTs (bGDGTs) together determined the proxy of the Branched and Isoprenoid Tetraether index (BIT) that correlated with the relative proportion of terrestrial input, while chlorin served as a measure of sedimentary primary productivity in the study area. The range of δ13C (from -25.95‰ to -23.17‰) suggested a mixing source for porewater DOM while TOC decreased from nearshore to offshore. As a result, both GDGTs and δ13C illustrated the effect of DOM sources on bacterial communities. These seven variables could refer to DOM sources and are therefore able to affect the properties of DOM. The remaining variables, C5 and C4_r, represented respectively the intensity and relative intensity of FDOM protein-like, while BIX and FI measured freshness and differential sources of FDOM respectively.
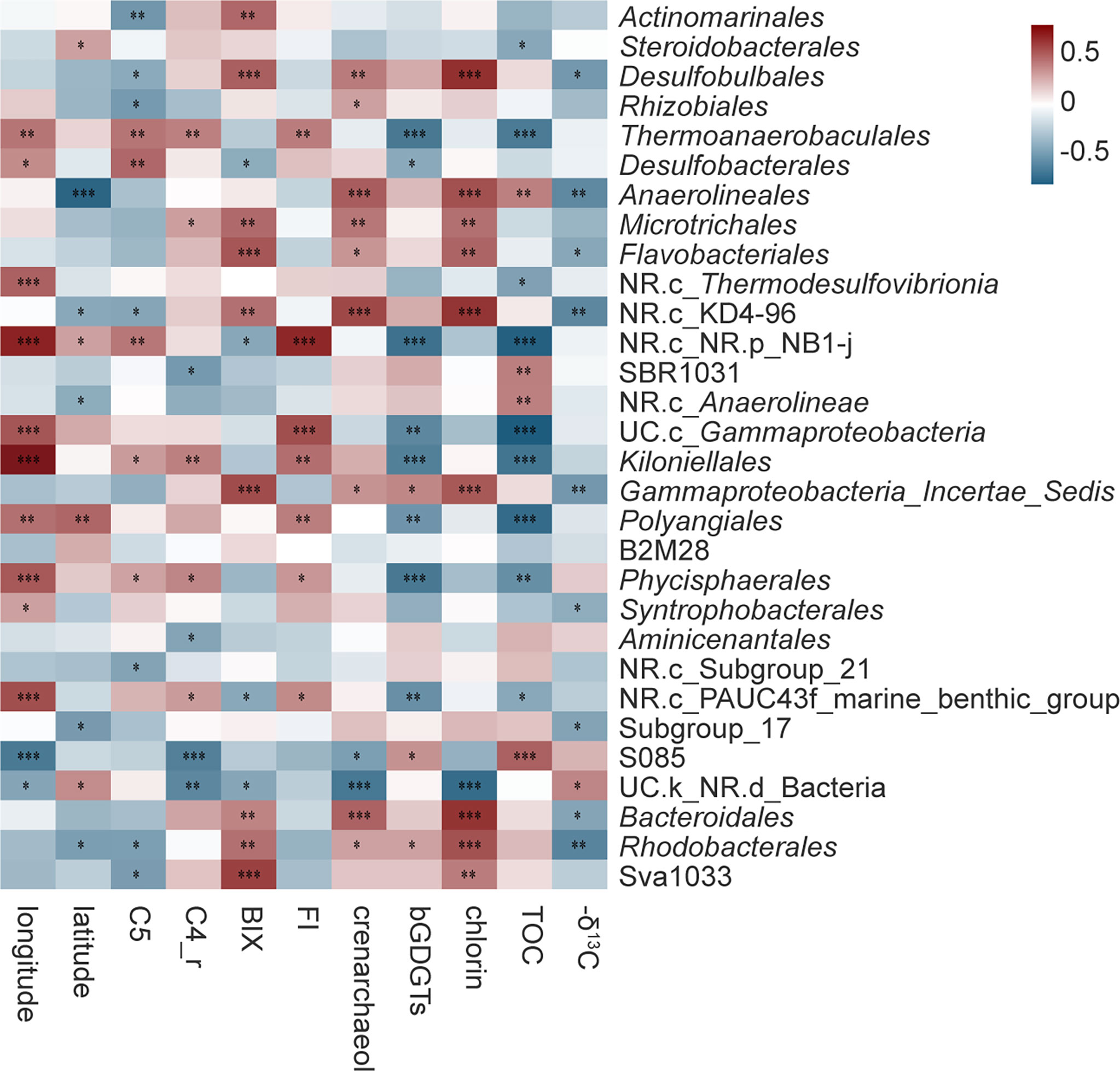
Figure 4 Correlation analysis between significant factors and bacterial taxa (top 30) at the order level. Spearman’s rank correlations are indicated by colors, with red for positive correlations and blue for negative ones. BIX, biological index; FI, fluorescence index; bGDGTs, branched glycerol dialkyl glycerol tetraethers; TOC, total organic carbon; C1-C5, fluorescence intensity in the Raman Unit of C1-C5; C1_r-C5_r, proportions of total intensity (i.e., relative intensity) of C1-C5. Significant factors are shown within brackets in the second column. Significance labels: ***p < 0.001, **p < 0.01, *p < 0.05.
Alpha-diversity indices including chao1, sobs, ace and pd were calculated for bacterial richness at the genus level. They got range of 112-26.67 for chao1, 112-262 for sobs, 112-262.93 for ace and 0.9994-1 for pd. After the process as mentioned above, factors matrix corresponding to richness matrix (i.e., chao1, sobs, ace and pd) for Mantel test included C5, bGDGTs and TOC. Mantel_r value between two matrixes was 0.2214 (p = 0.0067). This result suggested the relevance existing between bacterial richness and environmental factors. Given the nature of statistics that parts of data should be discarded to gain more important information (Stigler, 2016), details in the data will be inevitably lost more or less in the statistical process. To gain a deeper understanding of the relevance, we developed a correlation matrix for diversity indices and all 34 variations out of the consideration of too few factors retained after processes (Figure 5). Still, quite a lot of correlations showed between richness and other variables. Notably, all four richness indices were positively correlated with BIT (r = 0.583 – 0.531, p < 0.001) and Humic_r (r = 0.388 – 0.42, p < 0.05), and negatively correlated with Protein_r (r = -0.42 – -0.388, p < 0.05), suggesting higher bacterial richness in the sites with higher terrestrial input and higher humic-like proportions. According to the rank abundance distributions obtained at the genus level (Figure S7), samples with BIT > 0.1 generally had greater ranks than those with BIT < 0.1, further indicating that sites with higher terrestrial contributions harbored higher bacterial diversity. It was also notable that the community-level (or sample-level) niche breadth Bcom showed a significant negative correlation with humic-like proportion (r = -0.4252, p < 0.01; Figure S8).
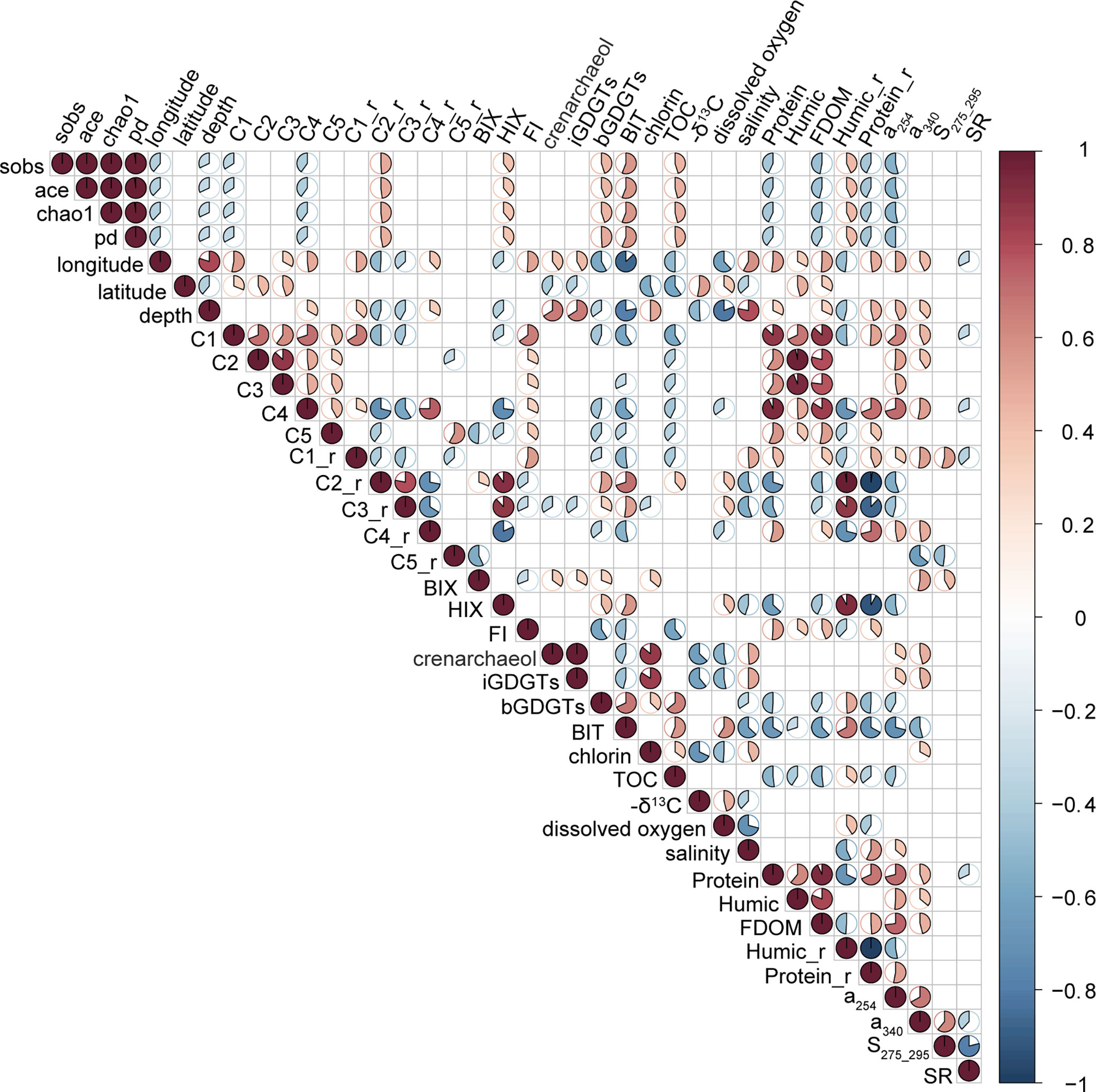
Figure 5 Correlation matrix among environmental factors and four richness estimators. Only Spearman’s rank correlations with p < 0.5 are shown. The area and color of the sector indicate the correlation coefficients, with red for positive correlations and blue for negative ones. sobs, the observed richness; pd, phylogenetic diversity; HIX, humidification index; iGDGTs, isoprenoid glycerol dialkyl glycerol tetraethers; BIT, branched and isoprenoid index; Protein, Humic & FDOM, fluorescence intensity in the Raman Unit of protein-like components, humic-like components & FDOM; Humic_r & Protein_r, proportions of total intensity (i.e., relative intensity) of protein-like components, humic-like components. a254 & a340, absorption coefficient at the wavelength of 254 nm & 340nm; S275_295, spectral slope from 275nm to 295nm; SR, spectral slope ratio.
Bacterial Co-Occurrence Networks and Comparative Analysis Between Two Groups
As indicated above, DOM properties had profound effects on composition and diversity of the bacterial community. All 42 samples were divided into two groups (Group F with BIX ≥ 1, otherwise Group N) to explore the relationship between DOM bioavailability and bacterial communities. Ordination analysis (MNDS) was performed from the level of the phylum to genus (Figures 6A, C) to validate of the grouping. Both relative abundances and abundances at all five levels varied significantly between two groups, with higher r values and lower p values for relative abundances at all five levels than that for abundances. In concordance with the dominant marine source of DOM in this area, most of the BIX values were in the highly fresh range of 0.8 to 1.2 (Figure S5). To avoid pseudo-positive results from the grouping procedure (i.e., setting the breakpoint as BIX = 1), ordination analysis was applied again with samples with BIX values between 0.8 to 1.2 ignored. From Figures 6B, D, bacterial community compositions in terms of relative abundances were still significantly different between two groups with higher r values, while the difference in abundance was no longer significant. This result confirmed the response of bacterial relative abundance to DOM with different freshness as opposed to abundances. Nevertheless, total bacterial biomass estimated by qPCR in samples of Group F were significantly higher than that of Group N (p = 0.02, Wilcoxon rank-sum test), in part due to more bioavailable substrates, although no significant differences were found in the richness indices between Group F and N (p > 0.05 for four richness indices, Wilcoxon rank-sum test).
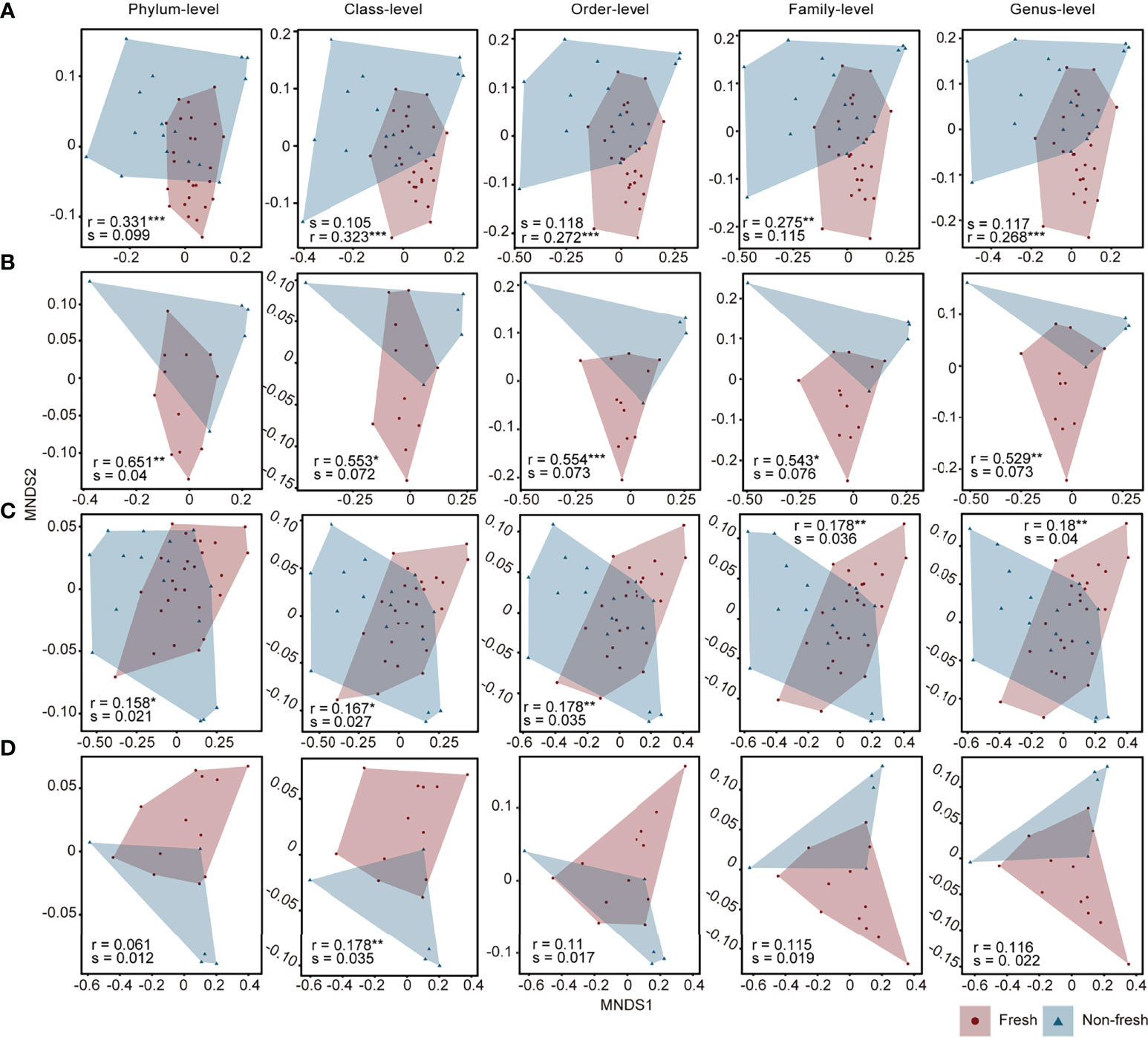
Figure 6 NMDS ordination of samples in two groups based on Bray-Curtis distance at different taxonomic levels. In form of the bacterial relative abundance, samples shown in (A) represents all 42 sites while (B) only represents samples with BIX < 0.8 or BIX > 1.2. In form of the bacterial abundance, samples shown in (C) represents all 42 sites while (D) only represents samples with BIX < 0.8 or BIX > 1.2. Red circles indicate sites of the Group F while blue triangles indicate sites of the Group N. Significance of difference between two groups based on NMDS was tested via ANOSIM. Significance labels: ***p < 0.001, **p < 0.01, *p < 0.05.
Then we performed the subsequent network analysis with relative abundances at the genus level to identify the co-occurrence patterns in two groups. It was evident Group N possessed a more complex and denser network than Group F did (Figures 7A, B). There were 583 and 544 nodes within the networks of Group N and Group F, respectively. The edges and density of Group N were 3.4 times and 3 times those of Group F, respectively. Significantly higher degrees or edges (p < 0.001, Wilcoxon rank-sum test) connected to each node appeared within the network of Group N than Group F. Based on the value of within-module degree Zi and participation coefficient Pi (Figures 7C, D), all taxa within two networks belonged to either peripherals or connectors and these two categories were believed to represent specialists and generalists, respectively (Deng et al., 2012). There were 83.6% of nodes belonged to generalists and 15.3% to specialists for Group F, while numbers for Group N were 76.5% and 23% belonged to generalists and specialists, respectively. Results from LEfSe analysis revealed 213 significantly differential bacterial features (LDA score > 2, p < 0.05) from phylum to genus levels between two groups. According to the ranking of the LDA score from large to small, the top 20 taxa were chosen to represent the impact on the difference between groups (Figure 8). Compared with Group F, Group N possessed lower relative abundances of Actinobacteriota, Bacteroidota, Alphaproteobacteria, Desulfobulbia, and Gammaproteobacteria and higher of Anaerolineae and Desulfobacteria.
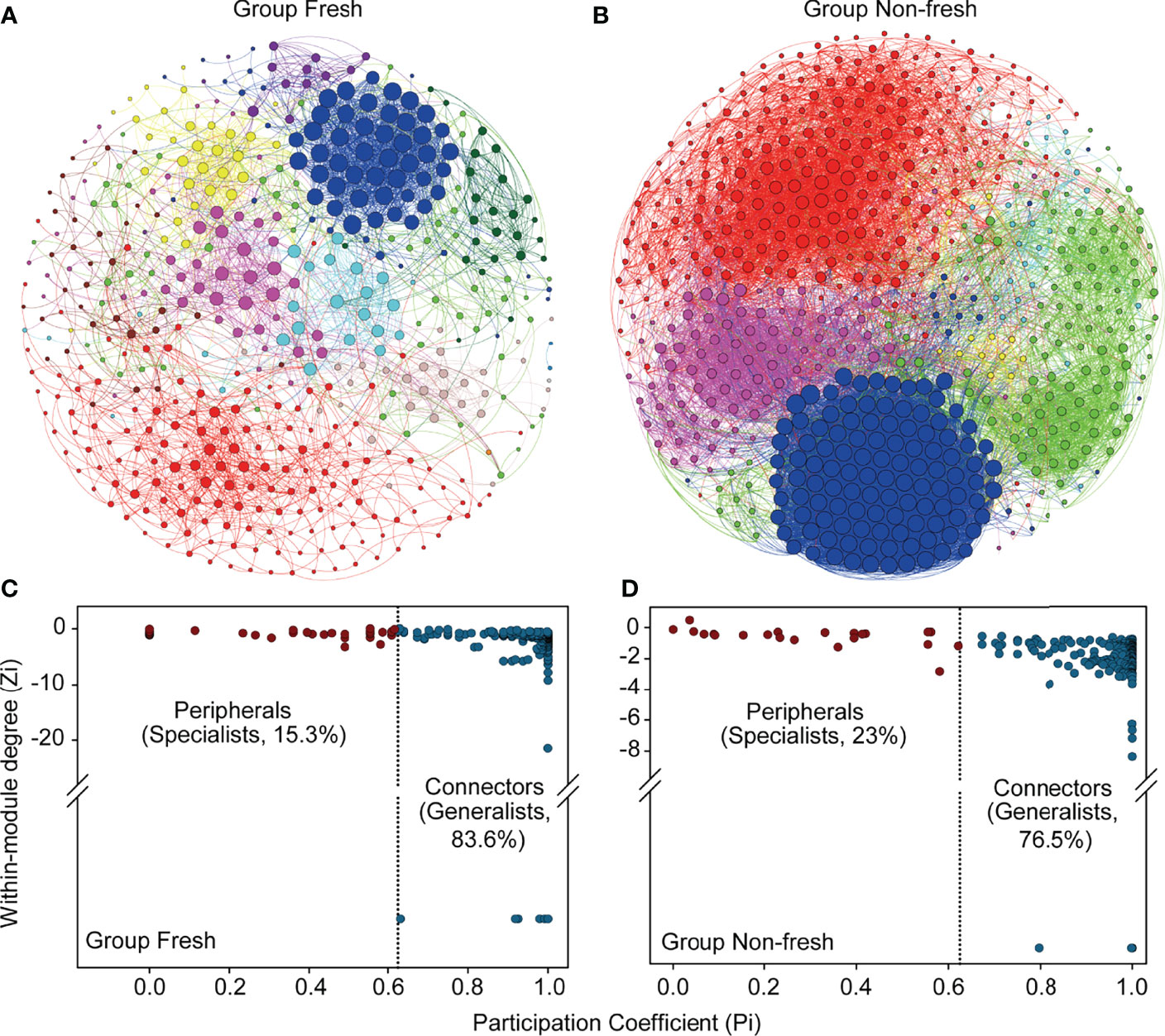
Figure 7 Bacterial co-occurrence networks of Group Fresh (A) and Group Non-fresh (B). Each node represents a genus, with larger size indicating higher degree. Edges were colored as their source nodes. Only Spearman’s rank correlations |r| > 0.6 and corresponding p < 0.05 are shown as edges. In each network, nodes with same color belong to same module. Between two networks, modules with the same color in two networks did not necessarily mean that they are the same module. Based on participation coefficient and within-module degree, topographic roles of nodes are organized into two categories: peripherals (red) and connectors (blue) for Group Fresh (C) and Group Non-fresh (D).
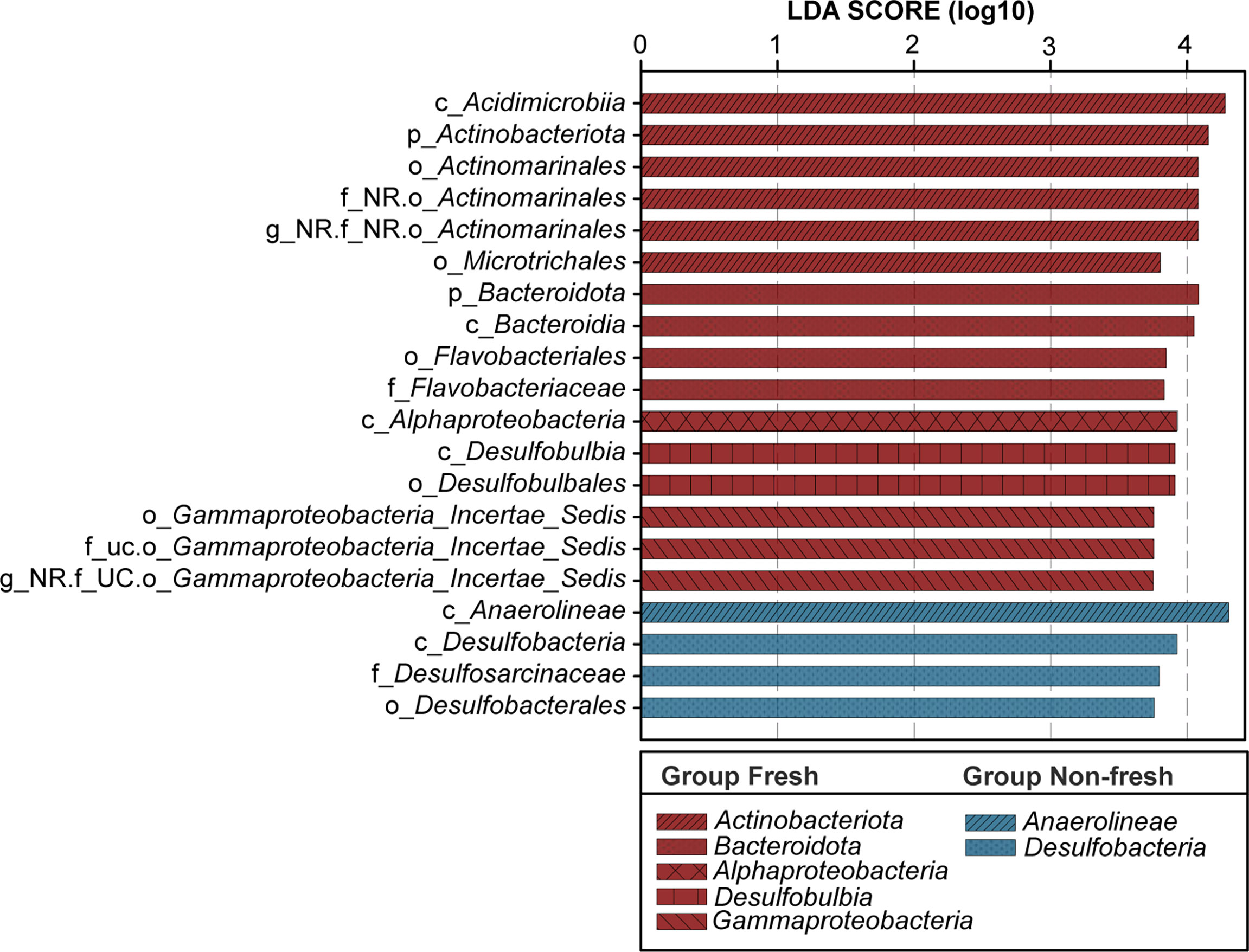
Figure 8 LEfSe analysis revealed the taxa that are significantly different between two groups base on the relative abundance. According to the ranking of LDA score from large to small, the top 20 taxa are shown in the figure with different colors and styles.
Discussion
Effects of DOM From Different Sources on Variation in Bacterial Richness and Abundance
DOM sources have been considered important in affecting the bacterial community composition and species diversity (Nagata, 2008; Landa et al., 2014). As our results showed, the relative abundances of several bacterial orders were strongly correlated with FDOM components including the marine autochthonous components C5 (Jamieson et al., 2014), as well as some FDOM-derived parameters such as BIX and FI. Also, both the relative abundances of several bacterial orders and the richness of the bacterial communities were closely correlated with variables indirectly depicting DOM sources, for example, the location of sites (relative distance from land), GDGTs-derived terrestrial proxy (BIT) and chlorin (Harris et al., 1996; Pitcher et al., 2011) representing sedimentary productivity in the sediments of the East China Sea. In line with previous studies (Landa et al., 2014; Li et al., 2020b), our results suggestthat the variations in DOM sources had significantly impact on the bacterial communities.
Species richness indicators, such as chao1, ace, sobs and pd, were positively bound up with C2_r (Figure 5), a reported terrestrial proxy (Li et al., 2020a). As a result of terrestrial vegetation diversity and regional anthropogenic activities, DOM from terrestrial environments is more chemically heterogeneous, which may further induce changes in aquatic bacterial communities (Lobbes et al., 2000; Grieve and Marsden, 2001; Lennon and Pfaff, 2005). In this study, a high terrestrial contribution might result in a high DOM heterogeneity and consequently supported more diverse bacterial taxa. Since samples with higher C2_r had lower values of Bcom (r = -0.4436, p < 0.01; Figure S8), the amendment of terrestrial DOM pool may support a narrow niche breadth at the community level. In the case of less available resources, a wide community-level niche breadth would benefit the bacterial taxa with high metabolic flexibility to acquire enough resources (Qin, 2009; Jiao et al., 2020). In this study, samples with lower terrestrial input might have lower DOM heterogeneity and nutrition. Hence, bacteria had to adopt flexible strategies and shift to the utilizing what were available to them, while the niche breadth at the community level becomes wide with insufficient resources for bacterial utilization. It was reported that DOM heterogeneity could provide diverse compounds as resources to heterotrophs and may lead to higher diversity (Smith et al., 2018). Zhao et al. (2019) showed an increase in chemodiversity after adding DOM from new sources (virus-derived DOM), although other studies reported that DOM sources had minimal effects on bacterial communities (Kirchman et al., 2004; Rink et al., 2007).
Effect of DOM Freshness on Shaping Bacterial Community Co-Occurrence Patterns in Marine Sediments
In general, a higher BIX value meant more freshly autochthonous DOM that is readily bioavailable (Sundh, 1992; Huguet et al., 2009; Romera-Castillo et al., 2011). In this study, the bacterial community compositions of those samples with lower BIX values (defined as non-freshness group, Group N) were distinctly different from those samples with higher BIX values (defined as freshness group, Group F) (Figures 6A, C), indicating the preference of bacterial communities for substrates with varying levels of freshness. Except for the community composition, the biological interactions among different bacterial taxa were also considered to be affected by DOM in natural environments (Montoya et al., 2006; Osterholz et al., 2016). A network analysis was employed to detect co-occurrence patterns of sedimentary bacterial community in this study (Figures 7A, B). A more complex and denser network was found in those non-freshness samples than in freshness samples, which suggests that the non-freshness DOM could enhance the bacterial species relationships in the sediments in the East China Sea. As DOM becomes less fresh and more refractory, a bacterial species that can adopt appropriate survival strategies of reducing energy loss would survive more easily One of the possible adaptive strategies may be to increase the exploitation of specialized resource due to genome streaming (Swan et al., 2013). This might be one possible reason for higher proportion of specialists in Group N than that in Group F. However, microbial transformation of DOM have been reported as a synergistic process involving different functional enzymes from different bacterial taxa and sometimes even utilization of simple DOM compounds required the participation of a wide phylogenetic spectrum of microbes (Cottrell and Kirchman, 2000; Zimmerman et al., 2013; Logue et al., 2016; Yang et al., 2020). To meet the DOM exploitation demand, bacteria in Group N should be more complementary to each other because they were more specialized than Group F, resulting in more interspecies connections within the network. Our results were agreeable with earlier studies indicating that fresh phytoplankton-derived DOM could be adverse to bacterial specialization (Sarmento et al., 2016). Another possible explanation for the difference between two groups may come from bacterial functional prediction. A metabolic pathway ko02024 responding to quorum sensing function in bacteria were significantly up-regulated (p < 0.01) in Group N (Figure S9). Under different physicochemical conditions, bacterial composition of functional groups would differ due to the selection of suitable metabolic pathways (Louca et al., 2016). Previous study had reported that some specialists of bacteria may produce specific DOM portions for quorum sensing (Hmelo et al., 2011). In agreement with previous studies (Zhou et al., 2021a; Zhou et al., 2021b), DOM freshness may influence bacterial metabolisms and thus be a driving force to shape the bacterial community co-occurrence. In turn, the complimentary utilization of N group DOM among different bacterial specialists would play a role in the marine cycle of DOM elements (McCarren et al., 2010).
There were two similar taxa, Desulfobulbia and Desulfobacteria, which are both enriched in Group F and Group N, respectively. They previously belonged to the phylum Gammaproteobacteria but now have been proposed as two new phyla (Young, 2007; Waite et al., 2020). Although both taxa were capable of reducing sulfate, Desulfobulbia had an additional assimilation pathway reducing nitrate to ammonia (i.e., denitrification) (Waite et al., 2020). It was reported that bacterial denitrification degree was correlated positively with the consumption of the B peak of FDOM (Barnes et al., 2012). In this study, C5 corresponding to peak B was identified as a tyrosine component and was considered to be biodegradable DOM (Coble, 1996; Jamieson et al., 2014). Thus, in Group F, C5 was expected to be much higher than Group N in their initial stage. However, Wilcox test results showed that C5 in Group F was significantly lower than in Group N, which indicated a higher net consumption of C5 in the fresh F and perphaps indicated a high expression of denitrification by Desulfobulbia. Another differential taxon enriched in Group N was Anaerolineae, within which there were many taxa of filamentous bacteria in Group N. Filamentous bacteria were reported to show the high stability and low population change with varied resources load (Mielczarek et al., 2012), which may explain their enrichment in Group N owing their powerful competitiveness for varying DOM with different freshness degrees. As abundant taxa in Group F, Actinobacteriota were affected by both aromatic and protein-like substrates binding to humic compounds (Liu et al., 2019). In this study, DOM mainly originated from marine autochthonous production with a high BIX value. Thus, there were more humic-like carriers as well as more ‘passenger’ compounds like protein-like substrates, which may have supported the growth of Actinobacteriota. Alphaproteobacteria have been considered as a strong competitor for amino acids in marine ecosystems and they also subsist on almost all kinds of DOM compounds (Malmstrom et al., 2004; Kujawinski, 2011). Among the taxa, some clades like SAR11 became more important and produced more fresh DOM for other bacterial taxa (Poretsky et al., 2010). Upon exposure to marine autochthonous DOM, the abundance of both Bacteroidota and Gammaproteobacteria were elevated (Amaral et al., 2016). Both taxa were viewed as opportunistic organisms (Alonso and Pernthaler, 2006; Alonso-Sáez and Gasol, 2007) and this may explain why they were more likely to thrive in Group F with more bioavailable DOM. In this study, bacterial communities, either their co-occurrence patterns or dominant taxa, were associated with DOM freshness. These results implied that bacterial communities would probably change in their structure or composition as DOM converting from fresh to recalcitrant.
Conclusions
In this study, the bacterial communities were strongly linked to DOM optical-derived properties such as sources and freshness of DOM. Variations in the DOM properties and the bacterial communities indicated mutual responses to each other. Bacterial community compositions either as bacterial assemblages or individual bacteria taxa had significant correlations with DOM properties and the relative bacterial abundance responded to those properties more intensely than their abundance. Higher values of alpha-diversity were found in sediments with higher terrestrial contributions, which could be explain by DOM heterogeneity or nutrition within the allochthonous matter derived from variable ecosystems on land. It is possible that the high richness may enhance the complexity of the interspecies interaction since more taxa participated in the co-occurrence network. Although there were no significant differences in bacterial richness between Group F and Group N, bacterial biomass was higher in Group F due to more bioavailable substrates provided by higher freshness DOM. Network analysis identified distinct co-occurrence patterns between two groups. By comparison, bacterial taxa in Group N were connected much more frequently and had more specialists, which might be an adaptive strategy under low-resource conditions to reduce energy loss by elevating cooperation and specialization during the utilization of DOM. Differential taxa in two groups showed physiological characteristics to adopt their local environmental conditions. Our findings demonstrate that changes in DOM composition would not only modify bacterial community compositions but also enforce interspecies interactions within the bacteria community to respond to the increasing recalcitrance of DOM. Furthermore, this study also provides a framework to clarify the relationships between DOM and bacterial communities in marine sediments.
Data Availability Statement
The data presented in the study are deposited in the NCBI repository (https://www.ncbi.nlm.nih.gov/), accession number PRJNA761364.
Author Contributions
ML: Conceptualization, methodology, formal analysis, writing - original draft, visualization. WX: Conceptualization, methodology, resources, writing - review & editing, supervision, funding acquisition. CZ: Resources, writing - review & editing, funding acquisition. KY: Writing - review & editing, supervision, funding acquisition. All authors contributed to the article and approved the submitted version.
Funding
This work was supported by the National Natural Science Foundation of China (grant Nos. 92051117 (WX), 41776137 (WX), 91851210 (CZ), 42141003 (CZ), the State Key R&D project of China grant [No. 2018YFA0605800 (CZ)], the Shenzhen Key Laboratory of Marine Archaea Geo-Omics, Southern University of Science and Technology [No. ZDSYS20180208184349083(CZ)] and the Southern Marine Science and Engineering Guangdong Laboratory (Guangzhou) [K19313901(CZ)]. Kedong Yin acknowledges the support by GDNRC[2021]62 and NSFC-GD joint project (U1701247).
Conflict of Interest
The authors declare that the research was conducted in the absence of any commercial or financial relationships that could be construed as a potential conflict of interest.
Publisher’s Note
All claims expressed in this article are solely those of the authors and do not necessarily represent those of their affiliated organizations, or those of the publisher, the editors and the reviewers. Any product that may be evaluated in this article, or claim that may be made by its manufacturer, is not guaranteed or endorsed by the publisher.
Acknowledgments
We would like to thank captains and crew of R/V Zhehaike I. and the State Key Laboratory of Marine Geology (Tongji University) for the cruise support, Simin Gao, Dengxun Lai, Zhuo Cheng, Cong Liu, Penghui Li, Haijun Liu, Xizheng Lin, Peng Wang, Zhiyao Xiong, Ziqi Cui for the help during filed sampling, laboratory work and data analysis, Second Institute of Oceanography, MNR for scientific and technical assistance, online platforms of Majorbio Cloud Platform (www.majorbio.com) and Wekemo Bioimformatics Cloud (https://www.bioincloud.tech/). Computation in this study was also supported by the Centre for Computational Science and Engineering at the Southern University of Science and Technology.
Supplementary Material
The Supplementary Material for this article can be found online at: https://www.frontiersin.org/articles/10.3389/fmars.2022.919368/full#supplementary-material
References
Alonso C., Pernthaler J. (2006). Roseobacter and SAR11 Dominate Microbial Glucose Uptake in Coastal North Sea Waters. Environ. Microbiol. 8 (11), 2022–2030. doi: 10.1111/j.1462-2920.2006.01082.x
Alonso-Sáez L., Gasol J. M. (2007). Seasonal Variations in the Contributions of Different Bacterial Groups to the Uptake of Low-Molecular-Weight Compounds in Northwestern Mediterranean Coastal Waters. Appl. Environ. Microbiol. 73 (11), 3528–3535. doi: 10.1128/AEM.02627-06
Amaral V., Graeber D., Calliari D., Alonso C. (2016). Strong Linkages Between DOM Optical Properties and Main Clades of Aquatic Bacteria. Limnol. Oceanography 61 (3), 906–918. doi: 10.1002/lno.10258
Arnosti C. (2011). Microbial Extracellular Enzymes and the Marine Carbon Cycle. Annu. Rev. Mar. Sci. 3, 401–425. doi: 10.1146/annurev-marine-120709-142731
Azam F., Fenchel T., Field J. G., Gray J., Meyer-Reil L., Thingstad F. (1983). The Ecological Role of Water-Column Microbes in the Sea. Mar. Ecol. Prog. Ser. 10, 257–263. doi: 10.3354/meps010257
Bai L., Zhang Q., Ju Q., Wang C., Zhang H., Jiang H. (2020). Priming Effect of Autochthonous Organic Matter on Enhanced Degradation of 17α-Ethynylestradiol in Water-Sediment System of One Eutrophic Lake. Water Res. 184, 116153. doi: 10.1016/j.watres.2020.116153
Barnes R. T., Smith R. L., Aiken G. R. (2012). Linkages Between Denitrification and Dissolved Organic Matter Quality, Boulder Creek Watershed, Colorado. J. Geophys. Res.: Biogeosci. 117 (G1), G01014. doi: 10.1029/2011JG001749
Bolyen E., Rideout J. R., Dillon M. R., Bokulich N. A., Abnet C. C., Al-Ghalith G. A., et al. (2019). Reproducible, Interactive, Scalable and Extensible Microbiome Data Science Using QIIME 2. Nat. Biotechnol. 37 (8), 852–857. doi: 10.1038/s41587-019-0209-9
Burdige D. J. (2001). Dissolved Organic Matter in Chesapeake Bay Sediment Pore Waters. Organic Geochem. 32 (4), 487–505. doi: 10.1016/S0146-6380(00)00191-1
Burdige D. J., Komada T. (2015). “Sediment Pore Waters,” in Biogeochemistry of Marine Dissolved Organic Matter (Cambridge, MA: Academic Press), 535–577.
Cai R., Zhou W., He C., Tang K., Guo W., Shi Q., et al. (2019). Microbial Processing of Sediment-Derived Dissolved Organic Matter: Implications for its Subsequent Biogeochemical Cycling in Overlying Seawater. J. Geophys. Res.: Biogeosci. 124 (11), 3479–3490. doi: 10.1029/2019JG005212
Callahan B. J., McMurdie P. J., Rosen M. J., Han A. W., Johnson A. J. A., Holmes S. P. (2016). DADA2: High-Resolution Sample Inference From Illumina Amplicon Data. Nat. Methods 13 (7), 581–583. doi: 10.1038/nmeth.3869
Carlson C. A., Giovannoni S. J., Hansell D. A., Goldberg S. J., Parsons R., Vergin K. (2004). Interactions Among Dissolved Organic Carbon, Microbial Processes, and Community Structure in the Mesopelagic Zone of the Northwestern Sargasso Sea. Limnol. Oceanography 49 (4), 1073–1083. doi: 10.4319/lo.2004.49.4.1073
Chen M., Hur J. (2015). Pre-Treatments, Characteristics, and Biogeochemical Dynamics of Dissolved Organic Matter in Sediments: A Review. Water Res. 79, 10–25. doi: 10.1016/j.watres.2015.04.018
Chipman L., Podgorski D., Green S., Kostka J., Cooper W., Huettel M. (2010). Decomposition of Plankton-Derived Dissolved Organic Matter in Permeable Coastal Sediments. Limnol. Oceanography 55 (2), 857–871. doi: 10.4319/lo.2010.55.2.0857
Coates J. D., Cole K. A., Chakraborty R., O'Connor S. M., Achenbach L. A. (2002). Diversity and Ubiquity of Bacteria Capable of Utilizing Humic Substances as Electron Donors for Anaerobic Respiration. Appl. Environ. Microbiol. 68 (5), 2445–2452. doi: 10.1128/AEM.68.5.2445-2452.2002
Coble P. G. (1996). Characterization of Marine and Terrestrial DOM in Seawater Using Excitation-Emission Matrix Spectroscopy. Mar. Chem. 51 (4), 325–346. doi: 10.1016/0304-4203(95)00062-3
Cottrell M. T., Kirchman D. L. (2000). Natural Assemblages of Marine Proteobacteria and Members of the Cytophaga-Flavobacter Cluster Consuming Low-and High-Molecular-Weight Dissolved Organic Matter. Appl. Environ. Microbiol. 66 (4), 1692–1697. doi: 10.1128/AEM.66.4.1692-1697.2000
Deng Y., Jiang Y.-H., Yang Y., He Z., Luo F., Zhou J. (2012). Molecular Ecological Network Analyses. BMC Bioinf. 13 (1), 1–20. doi: 10.1186/1471-2105-13-113
Derrien M., Shin K.-H., Hur J. (2019). Biodegradation-Induced Signatures in Sediment Pore Water Dissolved Organic Matter: Implications From Artificial Sediments Composed of Two Contrasting Sources. Sci. Total Environ. 694, 133714. doi: 10.1016/j.scitotenv.2019.133714
Ducklow H. W., Purdie D. A., Williams P. J. L., Davies J. M. (1986). Bacterioplankton: A Sink for Carbon in a Coastal Marine Plankton Community. Science 232 (4752), 865–867. doi: 10.1126/science.232.4752.865
Dyhrman S. T., Benitez-Nelson C. R., Orchard E. D., Haley S. T., Pellechia P. J. (2009). A Microbial Source of Phosphonates in Oligotrophic Marine Systems. Nat. Geosci. 2 (10), 696–699. doi: 10.1038/ngeo639
Fox C. A., Abdulla H. A., Burdige D. J., Lewicki J. P., Komada T. (2018). Composition of Dissolved Organic Matter in Pore Waters of Anoxic Marine Sediments Analyzed by 1H Nuclear Magnetic Resonance Spectroscopy. Front. Mar. Sci. 5, 172. doi: 10.3389/fmars.2018.00172
Freixa A., Ejarque E., Crognale S., Amalfitano S., Fazi S., Butturini A., et al. (2016). Sediment Microbial Communities Rely on Different Dissolved Organic Matter Sources Along a Mediterranean River Continuum. Limnol. Oceanography 61 (4), 1389–1405. doi: 10.1002/lno.10308
Goldman J. C., Dennett M. R. (2000). Growth of Marine Bacteria in Batch and Continuous Culture Under Carbon and Nitrogen Limitation. Limnol. Oceanography 45 (4), 789–800. doi: 10.4319/lo.2000.45.4.0789
Grieve I. C., Marsden R. L. (2001). Effects of Forest Cover and Topographic Factors on TOC and Associated Metals at Various Scales in Western Scotland. Sci. Total Environ. 265 (1-3), 143–151. doi: 10.1016/S0048-9697(00)00655-0
Guerrero-Feijóo E., Nieto-Cid M., Sintes E., Dobal-Amador V., Hernando-Morales V., Álvarez M., et al. (2017). Optical Properties of Dissolved Organic Matter Relate to Different Depth-Specific Patterns of Archaeal and Bacterial Community Structure in the North Atlantic Ocean. FEMS Microbiol. Ecol. 93 (1), fiw224. doi: 10.1093/femsec/fiw224
Hansell D. A., Carlson C. A., Repeta D. J., Schlitzer R. (2009). Dissolved Organic Matter in the Ocean: A Controversy Stimulates New Insights. Oceanography 22 (4), 202–211. doi: 10.5670/oceanog.2009.109
Hansen A. M., Kraus T. E., Pellerin B. A., Fleck J. A., Downing B. D., Bergamaschi B. A. (2016). Optical Properties of Dissolved Organic Matter (DOM): Effects of Biological and Photolytic Degradation. Limnol. Oceanography 61 (3), 1015–1032. doi: 10.1002/lno.10270
Harris P., Zhao M., Rosell-Melé A., Tiedemann R., Sarnthein M., Maxwell J. (1996). Chlorin Accumulation Rate as a Proxy for Quaternary Marine Primary Productivity. Nature 383 (6595), 63–65. doi: 10.1038/383063a0
Helms J. R., Stubbins A., Ritchie J. D., Minor E. C., Kieber D. J., Mopper K. (2008). Absorption Spectral Slopes and Slope Ratios as Indicators of Molecular Weight, Source, and Photobleaching of Chromophoric Dissolved Organic Matter. Limnol. Oceanography 53 (3), 955–969. doi: 10.4319/lo.2008.53.3.0955
Hmelo L. R., Mincer T. J., Van Mooy B. A. (2011). Possible Influence of Bacterial Quorum Sensing on the Hydrolysis of Sinking Particulate Organic Carbon in Marine Environments. Environ. Microbiol. Rep. 3 (6), 682–688. doi: 10.1111/j.1758-2229.2011.00281.x
Huguet A., Vacher L., Relexans S., Saubusse S., Froidefond J.-M., Parlanti E. (2009). Properties of Fluorescent Dissolved Organic Matter in the Gironde Estuary. Organic Geochem. 40 (6), 706–719. doi: 10.1016/j.orggeochem.2009.03.002
Ijiri A., Harada N., Hirota A., Tsunogai U., Ogawa N. O., Itaki T., et al. (2012). Biogeochemical Processes Involving Acetate in Sub-Seafloor Sediments From the Bering Sea Shelf Break. Organic Geochem. 48, 47–55. doi: 10.1016/j.orggeochem.2012.04.004
Jamieson T., Sager E., Guéguen C. (2014). Characterization of Biochar-Derived Dissolved Organic Matter Using UV–visible Absorption and Excitation–Emission Fluorescence Spectroscopies. Chemosphere 103, 197–204. doi: 10.1016/j.chemosphere.2013.11.066
Jiao N., Herndl G. J., Hansell D. A., Benner R., Kattner G., Wilhelm S. W., et al. (2010). Microbial Production of Recalcitrant Dissolved Organic Matter: Long-Term Carbon Storage in the Global Ocean. Nat. Rev. Microbiol. 8 (8), 593–599. doi: 10.1038/nrmicro2386
Jiao S., Yang Y., Xu Y., Zhang J., Lu Y. (2020). Balance Between Community Assembly Processes Mediates Species Coexistence in Agricultural Soil Microbiomes Across Eastern China. ISME J. 14 (1), 202–216. doi: 10.1038/s41396-019-0522-9
Kirchman D. L., Dittel A. I., Findlay S. E., Fischer D. (2004). Changes in Bacterial Activity and Community Structure in Response to Dissolved Organic Matter in the Hudson River, New York. Aquat. Microbial Ecol. 35 (3), 243–257. doi: 10.3354/ame035243
Kujawinski E. B. (2011). The Impact of Microbial Metabolism on Marine Dissolved Organic Matter. Annu. Rev. Mar. Sci. 3, 567–599. doi: 10.1146/annurev-marine-120308-081003
LaBrie R., Bélanger S., Benner R., Maranger R. (2021). Spatial Abundance Distribution of Prokaryotes is Associated With Dissolved Organic Matter Composition and Ecosystem Function. Limnol. Oceanography 66 (3), 575–587. doi: 10.1002/lno.11624
Landa M., Cottrell M., Kirchman D., Kaiser K., Medeiros P., Tremblay L., et al. (2014). Phylogenetic and Structural Response of Heterotrophic Bacteria to Dissolved Organic Matter of Different Chemical Composition in a Continuous Culture Study. Environ. Microbiol. 16 (6), 1668–1681. doi: 10.1111/1462-2920.12242
Lennon J. T., Pfaff L. E. (2005). Source and Supply of Terrestrial Organic Matter Affects Aquatic Microbial Metabolism. Aquat. Microbial Ecol. 39 (2), 107–119. doi: 10.3354/ame039107
Levins R. (1968). Evolution in Changing Environments Some Theoretical Explorations (Princeton, NJ:Princeton University Press).
Li P., Chen L., Zhang W., Huang Q. (2015). Spatiotemporal Distribution, Sources, and Photobleaching Imprint of Dissolved Organic Matter in the Yangtze Estuary and its Adjacent Sea Using Fluorescence and Parallel Factor Analysis. PloS One 10 (6), e0130852. doi: 10.1371/journal.pone.0130852
Li P., Hur J. (2017). Utilization of UV-Vis Spectroscopy and Related Data Analyses for Dissolved Organic Matter (DOM) Studies: A Review. Crit. Rev. Environ. Sci. Technol. 47 (3), 131–154. doi: 10.1080/10643389.2017.1309186
Liu S.-j., Xi B.-D., Qiu Z.-P., He X.-S., Zhang H., Dang Q.-L., et al. (2019). Succession and Diversity of Microbial Communities in Landfills With Depths and Ages and its Association With Dissolved Organic Matter and Heavy Metals. Sci. Total Environ. 651, 909–916. doi: 10.1016/j.scitotenv.2018.09.267
Li M., Xie W., Li P., Yin K., Zhang C. (2020a). Establishing a Terrestrial Proxy Based on Fluorescent Dissolved Organic Matter From Sediment Pore Waters in the East China Sea. Water Res. 182, 116005. doi: 10.1016/j.watres.2020.116005
Li Y., Xu C., Zhang W., Lin L., Wang L., Niu L., et al. (2020b). Response of Bacterial Community in Composition and Function to the Various DOM at River Confluences in the Urban Area. Water Res. 169, 115293. doi: 10.1016/j.watres.2019.115293
Li H., Yu Y., Luo W., Zeng Y., Chen B. (2009). Bacterial Diversity in Surface Sediments From the Pacific Arctic Ocean. Extremophiles 13 (2), 233–246. doi: 10.1007/s00792-009-0225-7
Lobbes J. M., Fitznar H. P., Kattner G. (2000). Biogeochemical Characteristics of Dissolved and Particulate Organic Matter in Russian Rivers Entering the Arctic Ocean. Geochimica Cosmochimica Acta 64 (17), 2973–2983. doi: 10.1016/S0016-7037(00)00409-9
Logue J. B., Stedmon C. A., Kellerman A. M., Nielsen N. J., Andersson A. F., Laudon H., et al. (2016). Experimental Insights Into the Importance of Aquatic Bacterial Community Composition to the Degradation of Dissolved Organic Matter. ISME J. 10 (3), 533–545. doi: 10.1038/ismej.2015.131
López-Urrutia Á., Morán X. A. G. (2007). Resource Limitation of Bacterial Production Distorts the Temperature Dependence of Oceanic Carbon Cycling. Ecology 88 (4), 817–822. doi: 10.1890/06-1641
Louca S., Parfrey L. W., Doebeli M. (2016). Decoupling Function and Taxonomy in the Global Ocean Microbiome. Science 353 (6305), 1272–1277. doi: 10.1126/science.aaf4507
Lou J., Yang L., Wang H., Wu L., Xu J. (2018). Assessing Soil Bacterial Community and Dynamics by Integrated High-Throughput Absolute Abundance Quantification. PeerJ 6, e4514. doi: 10.7717/peerj.4514
Mahmoudi N., Beaupré S. R., Steen A. D., Pearson A. (2017). Sequential Bioavailability of Sedimentary Organic Matter to Heterotrophic Bacteria. Environ. Microbiol. 19 (7), 2629–2644. doi: 10.1111/1462-2920.13745
Malmstrom R. R., Kiene R. P., Kirchman D. L. (2004). Identification and Enumeration of Bacteria Assimilating Dimethylsulfoniopropionate (DMSP) in the North Atlantic and Gulf of Mexico. Limnol. Oceanography 49 (2), 597–606. doi: 10.4319/lo.2004.49.2.0597
McCarren J., Becker J. W., Repeta D. J., Shi Y., Young C. R., Malmstrom R. R., et al. (2010). Microbial Community Transcriptomes Reveal Microbes and Metabolic Pathways Associated With Dissolved Organic Matter Turnover in the Sea. Proc. Natl. Acad. Sci. 107 (38), 16420–16427. doi: 10.1073/pnas.1010732107
Mielczarek A. T., Kragelund C., Eriksen P. S., Nielsen P. H. (2012). Population Dynamics of Filamentous Bacteria in Danish Wastewater Treatment Plants With Nutrient Removal. Water Res. 46 (12), 3781–3795. doi: 10.1016/j.watres.2012.04.009
Montoya J. M., Pimm S. L., Solé R. V. (2006). Ecological Networks and Their Fragility. Nature 442 (7100), 259–264. doi: 10.1038/nature04927
Moriarty D., Bell R. (1993). “Bacterial Growth and Starvation in Aquatic Environments,” in Starvation in Bacteria (New York, NY: Plenum Press), 25–53.
Nagata T. (2008). Organic Matter–Bacteria Interactions in Seawater (New York, NY:John Wiley & Sons, Inc.), 207–241.
Nguyen D., Maranger R., Tremblay J.É., Gosselin M. (2012). Respiration and Bacterial Carbon Dynamics in the Amundsen Gulf, Western Canadian Arctic. J. Geophys. Res.: Oceans 117 (C9), C00G16. doi: 10.1029/2011JC00734
Oksanen J., Blanchet F. G., Kindt R., Legendre P., Minchin P. R., O’hara R., et al. (2013). Package ‘Vegan’. Community Ecol. Package Version 2 (9), 1–295.
Osterholz H., Singer G., Wemheuer B., Daniel R., Simon M., Niggemann J., et al. (2016). Deciphering Associations Between Dissolved Organic Molecules and Bacterial Communities in a Pelagic Marine System. ISME J. 10 (7), 1717–1730. doi: 10.1038/ismej.2015.231
Pitcher A., Hopmans E. C., Mosier A. C., Park S.-J., Rhee S.-K., Francis C. A., et al. (2011). Core and Intact Polar Glycerol Dibiphytanyl Glycerol Tetraether Lipids of Ammonia-Oxidizing Archaea Enriched From Marine and Estuarine Sediments. Appl. Environ. Microbiol. 77 (10), 3468–3477. doi: 10.1128/AEM.02758-10
Poretsky R. S., Sun S., Mou X., Moran M. A. (2010). Transporter Genes Expressed by Coastal Bacterioplankton in Response to Dissolved Organic Carbon. Environ. Microbiol. 12 (3), 616–627. doi: 10.1111/j.1462-2920.2009.02102.x
Puddu A., Zoppini A., Fazi S., Rosati M., Amalfitano S., Magaletti E. (2003). Bacterial Uptake of DOM Released From P-Limited Phytoplankton. FEMS Microbiol. Ecol. 46 (3), 257–268. doi: 10.1016/S0168-6496(03)00197-1
Purdy K., Embley T., Nedwell D. (2002). The Distribution and Activity of Sulphate Reducing Bacteria in Estuarine and Coastal Marine Sediments. Antonie Van Leeuwenhoek 81 (1), 181–187. doi: 10.1023/A:1020550215012
Quast C., Pruesse E., Yilmaz P., Gerken J., Schweer T., Yarza P., et al. (2012). The SILVA Ribosomal RNA Gene Database Project: Improved Data Processing and Web-Based Tools. Nucleic Acids Res. 41 (D1), D590–D596. doi: 10.1093/nar/gks1219
Rink B., Seeberger S., Martens T., Duerselen C.-D., Simon M., Brinkhoff T. (2007). Effects of Phytoplankton Bloom in a Coastal Ecosystem on the Composition of Bacterial Communities. Aquat. Microbial Ecol. 48 (1), 47–60. doi: 10.3354/ame048047
Robador A., Brüchert V., Steen A. D., Arnosti C. (2010). Temperature Induced Decoupling of Enzymatic Hydrolysis and Carbon Remineralization in Long-Term Incubations of Arctic and Temperate Sediments. Geochimica Cosmochimica Acta 74 (8), 2316–2326. doi: 10.1016/j.gca.2010.01.022
Romera-Castillo C., Sarmento H., Alvarez-Salgado X. A., Gasol J. M., Marrasé C. (2011). Net Production and Consumption of Fluorescent Colored Dissolved Organic Matter by Natural Bacterial Assemblages Growing on Marine Phytoplankton Exudates. Appl. Environ. Microbiol. 77 (21), 7490–7498. doi: 10.1128/AEM.00200-11
Rossel P. E., Bienhold C., Boetius A., Dittmar T. (2016). Dissolved Organic Matter in Pore Water of Arctic Ocean Sediments: Environmental Influence on Molecular Composition. Organic Geochem. 97, 41–52. doi: 10.1016/j.orggeochem.2016.04.003
Sarmento H., Morana C., Gasol J. M. (2016). Bacterioplankton Niche Partitioning in the Use of Phytoplankton-Derived Dissolved Organic Carbon: Quantity is More Important Than Quality. ISME J. 10 (11), 2582–2592. doi: 10.1038/ismej.2016.66
Smith H. J., Tigges M., D'Andrilli J., Parker A., Bothner B., Foreman C. (2018). Dynamic Processing of DOM: Insight From Exometabolomics, Fluorescence Spectroscopy, and Mass Spectrometry. Limnol. Oceanography Lett. 3 (3), 225–235. doi: 10.1002/lol2.10082
Stedmon C. A., Bro R. (2008). Characterizing Dissolved Organic Matter Fluorescence With Parallel Factor Analysis: A Tutorial. Limnol. Oceanography: Methods 6 (11), 572–579. doi: 10.4319/lom.2008.6.572
Stedmon C. A., Nelson N. B. (2015). “The Optical Properties of DOM in the Ocean,” in Biogeochemistry of Marine Dissolved Organic Matter (Cambridge, MA: Academic Press), 481–508.
Stigler S. M. (2016). The Seven Pillars of Statistical Wisdom (Boston, MA:Harvard University Press).
Sundh I. (1992). Biochemical Composition of Dissolved Organic Carbon Derived From Phytoplankton and Used by Heterotrophic Bacteria. Appl. Environ. Microbiol. 58 (9), 2938–2947. doi: 10.1128/aem.58.9.2938-2947.1992
Swan B. K., Tupper B., Sczyrba A., Lauro F. M., Martinez-Garcia M., González J. M., et al. (2013). Prevalent Genome Streamlining and Latitudinal Divergence of Planktonic Bacteria in the Surface Ocean. Proc. Natl. Acad. Sci. 110 (28), 11463–11468. doi: 10.1073/pnas.1304246110
Tobias-Hünefeldt S. P., Wing S. R., Baltar F., Morales S. E. (2021). Changes in Microbial Community Phylogeny and Metabolic Activity Along the Water Column Uncouple at Near Sediment Aphotic Layers in Fjords. Sci. Rep. 11 (1), 1–7. doi: 10.1038/s41598-021-98519-2
Vraspir J. M., Butler A. (2009). Chemistry of Marine Ligands and Siderophores. Annu. Rev. Mar. Sci. 1, 43–63. doi: 10.1146/annurev.marine.010908.163712
Waite D. W., Chuvochina M., Pelikan C., Parks D. H., Yilmaz P., Wagner M., et al. (2020). Proposal to Reclassify the Proteobacterial Classes Deltaproteobacteria and Oligoflexia, and the Phylum Thermodesulfobacteria Into Four Phyla Reflecting Major Functional Capabilities. Int. J. Systematic Evolutionary Microbiol. 70 (11), 5972–6016. doi: 10.1099/ijsem.0.004213
Wang W., Tao J., Yu K., He C., Wang J., Li P., et al. (2021). Vertical Stratification of Dissolved Organic Matter Linked to Distinct Microbial Communities in Subtropic Estuarine Sediments. Front. Microbiol. 12. doi: 10.3389/fmicb.2021.697860
Wang Y., Zhang D., Shen Z., Chen J., Feng C. (2014). Characterization and Spacial Distribution Variability of Chromophoric Dissolved Organic Matter (CDOM) in the Yangtze Estuary. Chemosphere 95, 353–362. doi: 10.1016/j.chemosphere.2013.09.044
Wei T., Simko V., Levy M., Xie Y., Jin Y., Zemla J. (2017). Package ‘Corrplot’. Statistician 56 (316), e24.
Weston N. B., Joye S. B. (2005). Temperature-Driven Decoupling of Key Phases of Organic Matter Degradation in Marine Sediments. Proc. Natl. Acad. Sci. 102 (47), 17036–17040. doi: 10.1073/pnas.0508798102
Yang J., Jiang H., Liu W., Huang L., Huang J., Wang B., et al. (2020). Potential Utilization of Terrestrially Derived Dissolved Organic Matter by Aquatic Microbial Communities in Saline Lakes. ISME J. 14 (9), 2313–2324. doi: 10.1038/s41396-020-0689-0
Yang S., Tang M., Yim W. W.-S., Zong Y., Huang G., Switzer A. D., et al. (2011). Burial of Organic Carbon in Holocene Sediments of the Zhujiang (Pearl River) and Changjiang (Yangtze River) Estuaries. Mar. Chem. 123 (1-4), 1–10. doi: 10.1016/j.marchem.2010.07.001
Young K. D. (2007). Bacterial Morphology: Why Have Different Shapes? Curr. Opin. Microbiol. 10 (6), 596–600. doi: 10.1016/j.mib.2007.09.009
Zhao Z., Gonsior M., Schmitt-Kopplin P., Zhan Y., Zhang R., Jiao N., et al. (2019). Microbial Transformation of Virus-Induced Dissolved Organic Matter From Picocyanobacteria: Coupling of Bacterial Diversity and DOM Chemodiversity. ISME J. 13 (10), 2551–2565. doi: 10.1038/s41396-019-0449-1
Zhou L., Zhou Y., Tang X., Zhang Y., Jang K.-S., Székely A. J., et al. (2021a). Resource Aromaticity Affects Bacterial Community Successions in Response to Different Sources of Dissolved Organic Matter. Water Res. 190, 116776. doi: 10.1016/j.watres.2020.116776
Zhou L., Zhou Y., Tang X., Zhang Y., Jeppesen E. (2021b). Biodegradable Dissolved Organic Carbon Shapes Bacterial Community Structures and Co-Occurrence Patterns in Large Eutrophic Lake Taihu. J. Environ. Sci. 107, 205–217. doi: 10.1016/j.jes.2021.02.011
Zhou L., Zhou Y., Tang X., Zhang Y., Zhu G., Székely A. J., et al. (2021c). Eutrophication Alters Bacterial Co-Occurrence Networks and Increases the Importance of Chromophoric Dissolved Organic Matter Composition. Limnol. Oceanography 66 (6), 2319–32. doi: 10.1002/lno.11756
Ziegelgruber K. L., Zeng T., Arnold W. A., Chin Y.-P. (2013). Sources and Composition of Sediment Pore-Water Dissolved Organic Matter in Prairie Pothole Lakes. Limnol. Oceanography 58 (3), 1136–1146. doi: 10.4319/lo.2013.58.3.1136
Keywords: sediment, pore water, dissolved organic matter (DOM), bacterial community, co-occurrence networks
Citation: Li M, Xie W, Zhang C and Yin K (2022) Linking Bacterial Communities to Optical-Derived Properties of Porewater DOM in Sediments in the Coastal East China Sea. Front. Mar. Sci. 9:919368. doi: 10.3389/fmars.2022.919368
Received: 27 April 2022; Accepted: 23 May 2022;
Published: 21 June 2022.
Edited by:
Takuro Nunoura, Japan Agency for Marine-Earth Science and Technology (JAMSTEC), JapanReviewed by:
Jin Zhou, Tsinghua University, ChinaPatrick G. Hatcher, Old Dominion University, United States
Copyright © 2022 Li, Xie, Zhang and Yin. This is an open-access article distributed under the terms of the Creative Commons Attribution License (CC BY). The use, distribution or reproduction in other forums is permitted, provided the original author(s) and the copyright owner(s) are credited and that the original publication in this journal is cited, in accordance with accepted academic practice. No use, distribution or reproduction is permitted which does not comply with these terms.
*Correspondence: Wei Xie, eGlld2VpOUBtYWlsLnN5c3UuZWR1LmNu; Kedong Yin, eWlua2RAbWFpbC5zeXN1LmVkdS5jbg==