- 1Centro Oceanográfico de Gijón/Xixón, Instituto Español de Oceanografía (IEO), CSIC, Gijón/Xixón, Spain
- 2Department of Earth System Science, Stanford University, Stanford, CA, United States
- 3Section for Genetics and Evolutionary Biology (EVOGENE), Department of Biosciences, University of Oslo, Oslo, Norway
- 4Institut de Ciències del Mar (ICM), CSIC, Barcelona, Spain
- 5Red Sea Research Center, Biological and Environmental Sciences and Engineering Division, King Abdullah University of Science and Technology, Thuwal, Saudi Arabia
- 6AZTI, Marine Research, Basque Research and Technology Alliance (BRTA), Sukarrieta, Spain
Trophic interactions between marine phytoplankton and heterotrophic bacteria are at the base of the biogeochemical carbon cycling in the ocean. However, the specific interactions taking place between phytoplankton and bacterial taxa remain largely unexplored, particularly out of phytoplankton blooming events. Here, we applied network analysis to a 3.5-year time-series dataset to assess the specific associations between different phytoplankton and bacterial taxa along the seasonal scale, distinguishing between free-living and particle-attached bacteria. Using a newly developed network post-analysis technique we removed bacteria-phytoplankton correlations that were primarily driven by environmental parameters, to detect potential biotic interactions. Our results indicate that phytoplankton dynamics may be a strong driver of the inter-annual variability in bacterial community composition. We found the highest abundance of specific bacteria-phytoplankton associations in the particle-attached fraction, indicating a tighter bacteria-phytoplankton association than in the free-living fraction. In the particle-associated fraction we unveiled novel potential associations such as the one between Planctomycetes taxa and the diatom Leptocylindrus spp. Consistent correlations were also found between free-living bacterial taxa and different diatoms, including novel associations such as those between SAR11 with Naviculales diatom order, and between Actinobacteria and Cylindrotheca spp. We also confirmed previously known associations between Rhodobacteraceae and Thalassiosira spp. Our results expand our view on bacteria-phytoplankton associations, suggesting that taxa-specific interactions may largely impact the seasonal dynamics of heterotrophic bacterial communities.
Introduction
Marine heterotrophic bacteria represent the largest and most diverse biomass in the ocean (Whitman et al., 1998; Venter et al., 2004). Due to their enormous taxonomical and functional diversity, marine bacteria occupy a wide range of ecological niches, from oligotrophic waters to organic matter-rich phytoplankton blooms (Martiny et al., 2006; Haggerty and Dinsdale, 2017). Some studies have shown that the dynamics of marine bacterial communities follow predictable temporal patterns (Fuhrman et al., 2015; Bunse and Pinhassi, 2017), including the rare members (Alonso-Saez et al., 2015). Recurrent environmental drivers such as resource availability or water column mixing may control the presence of different clades. For example, the SAR11 or SAR86 clades have been described to dominate bacterial communities during stratified periods (Vergin et al., 2013) due to their adaptations to grow under low nutrient conditions (Morris et al., 2002; Giovannoni et al., 2005; Dupont et al., 2012). On the other hand, members of Actinobacteria or Roseobacter grow better under water column mixing conditions (Morris et al., 2005). Despite the well-known role of environmental factors on bacterial dynamics, the relevance of biotic interactions is increasingly recognized (Lima-Mendez et al., 2015). As direct and indirect suppliers of fresh and labile dissolved organic matter (DOM), phytoplankton has been typically considered one of the most important agents controlling bacterial community composition in surface waters (Riemann et al., 2000; Teeling et al., 2012; Landa et al., 2016). Yet, there is still a lack of knowledge on specific interactions between bacteria and phytoplankton taking place in marine waters, particularly under non-bloom conditions.
The most widely studied interactions between bacteria and phytoplankton are typically mutualistic. Bacteria can provide phytoplankton with essential nutrients for growth (Cooper and Smith, 2015), including vitamin B1 and B12 (Croft et al., 2005; Cruz-Lopez and Maske, 2016) or iron-siderophore chelates (Amin et al., 2009). Bacteria-phytoplankton info-chemical signaling is also important for phytoplankton cell division (Amin et al., 2015). These associations are more usual in bacteria that live attached to phytoplankton cells, as they can establish close symbiotic interactions between the phytoplankton cells (Rooney-Varga et al., 2005; Grossart et al., 2007; Arandia-Gorostidi et al., 2017). Due to the metabolic and functional complexity of these interactions, several authors have suggested that bacteria-phytoplankton associations could be taxa-specific (Pinhassi et al., 2004; Grossart et al., 2005; Jasti et al., 2005). Therefore, rapid successions of phytoplankton and bacterial taxa, as observed under phytoplankton bloom conditions (Teeling et al., 2012), may strongly determine microbial diversity and dynamics at the short-term scale, i.e., days (Needham and Fuhrman, 2016)
To assess the great complexity in microbial community potential interactions, an increasing number of studies are applying network analysis techniques, either at the spatial scale, i.e., biogeographical patterns (Lima-Mendez et al., 2015) or in time-series data (Steele et al., 2011; Chow et al., 2013; Cram et al., 2015). This approach has been successfully used, e.g., to determine the environmental parameters controlling the dynamics of marine microorganisms (Steele et al., 2011) or to investigate the role of biotic interactions such as viral mortality on bacterial distribution (Chow et al., 2013). Potentially, network analysis may also help us understand the interactions between bacterial taxa and specific phytoplankton groups in long time-series. Yet, so far, very few studies have applied network techniques to explore taxa-specific bacteria-phytoplankton interactions. In a short-term survey in waters off the California coast, potential mutualistic and competitive interactions were observed during daily phytoplankton bloom events (Needham and Fuhrman, 2016). Long-term observations, although studied to a lesser extent, have shown the importance of environmental parameters shaping the temporal microbial dynamics (Gilbert et al., 2012), while only a recent works have described the potential importance of taxa-specific associations between bacteria and photosynthetic picoeukaryotes on the annual reoccurrence of microbial taxa (Lambert et al., 2019; Lambert et al., 2021). These works, however, were not able to discern between biotic interactions from mere co-occurrences between bacteria and phytoplankton due to preferences for similar environmental properties.
In this work, we explored specific biotic associations between heterotrophic bacterioplankton (as assessed by 16S rRNA gene amplicon sequencing) and microphytoplankton taxa (as assessed by microscopic analysis) using network analysis after removing indirect dependencies among bacteria-bacteria or driven by preferences for similar environmental conditions. The combination of a 3.5-years time-series survey with a post-analysis network technique allowed us to disentangle potential biotic interactions between phytoplankton and bacteria and their importance on shaping microbial seasonal dynamics.
Results
Environmental Variables and Phytoplankton Abundance
Samples to test the associations between bacteria, phytoplankton and environmental variables were collected monthly between July 2009 and December 2012. During this period, environmental variables followed the typical seasonality of a Northern temperate coastal site. Temperature varied from winter minima (minimum 12.1°C recorded in March 2010) to summer maxima (maximum 21.7°C recorded in September 2009, Figure 1). Inorganic nutrients concentration (see Figure 1A for nitrate and Figure S1 for nitrate, nitrite, phosphate and silicate) followed an opposite seasonality, peaking in winter and showing annual minima (e.g., no Nitrate detected in May 2012) during spring and summer stratified period. Total chlorophyll a concentration, as a proxy of phytoplankton biomass, either peaked in late winter-spring (1.9 µg l-1, February 2012) or in autumn (2.7 µg l-1, October 2009), while the lowest values were consistently found in summer (0.04-0.11 µg l-1).
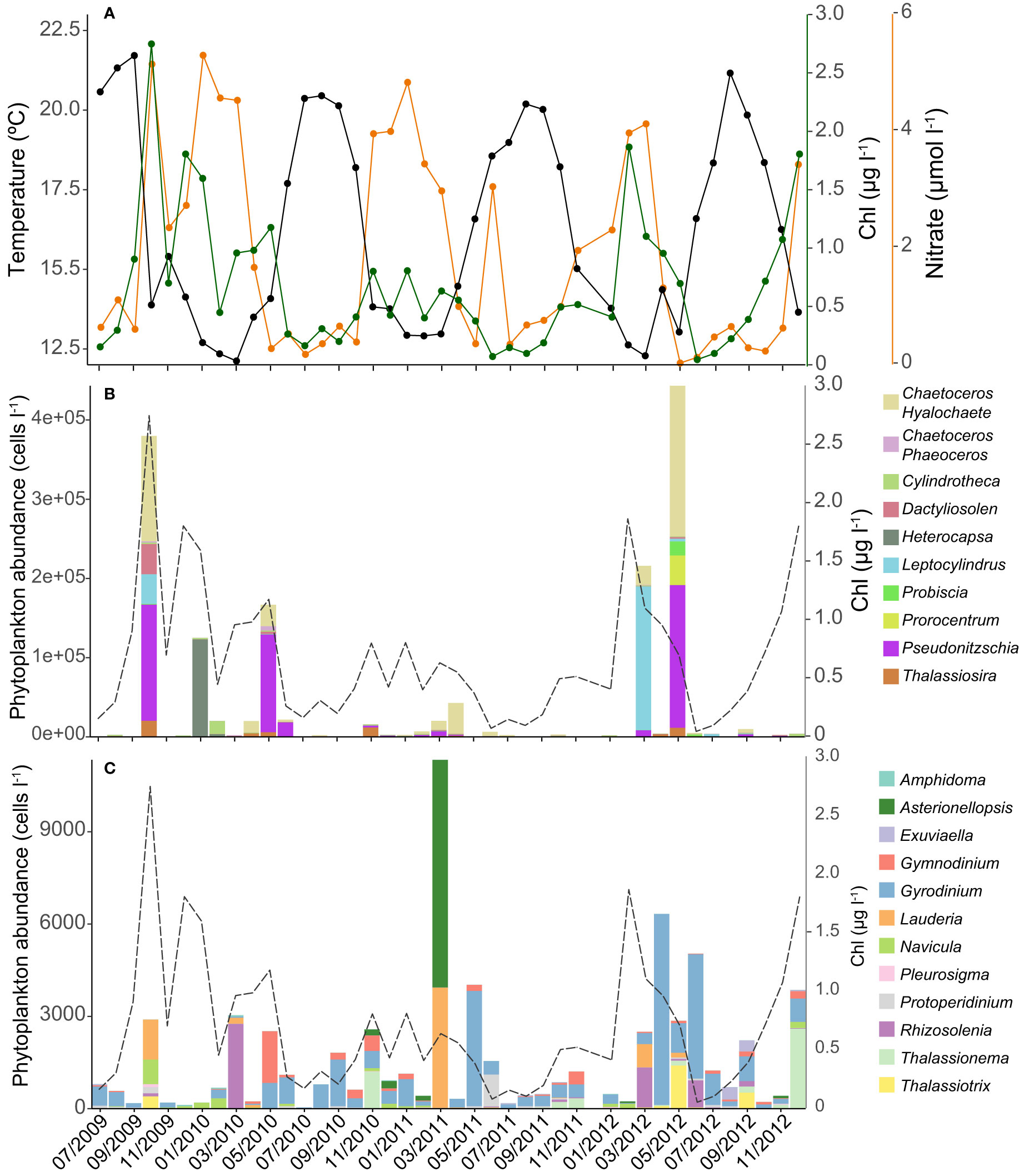
Figure 1 Temporal variation of in situ temperature and nitrate concentration (all available inorganic nutrients dynamics are shown in Figure S1) (A). Variation in total chlorophyll a concentration (green line) and most abundant phytoplankton genera (B) and other less abundant (<1.0 104 cells l-1) phytoplankton genera (C) at the time-series.
Diatoms (Bacillariophyta) were the most abundant microphytoplanktonic group, with Pseudo-nitzschia spp. as the most abundant genus (mostly during spring, 1.80*105 cells l-1 in May 2012). The genus Chaetoceros (subgenus Hyalochaete) peaked in October 2009 (1.33*105 cells l-1, Figure 1B) and Leptocylindrus spp. dominated during the bloom of March 2012 (1.79*105 cells l-1). Although other diatom genera such as Lauderia spp. and Thalassiosira spp. were considerably less abundant (Figure 1C), they peaked recurrently in the spring and autumn phytoplankton blooms. In the case of the diatom Navicula spp., we found higher concentrations in winter and autumn. Dinoflagellates were usually less abundant (ca. two orders of magnitude) than diatoms throughout the year. Heterocapsa spp. was the most abundant dinoflagellate genus, but only peaked in January 2010 (1.23*105 cells l-1), while Prorocentrum spp. was the second most abundant genus (3.80*104 cells l-1 in May 2012). The abundance of other less abundant genera, such as Gyrodinium was more homogeneous along the time-series.
Relative Abundance of Bacterial OTUs in the Free-Living and Particle-Attached Fractions
In order to identify the bacterial groups present in the free-living and particle-attached fractions, amplicon sequencing analysis was performed for the 3.5-year time series samples. While free-living samples were analyzed monthly during the period of study, the particle-attached fraction was only analyzed in 12 samples, which represented 28.5% of the 3.5-year time series samples. In order to collect reliable data about phytoplankton-associated microbial communities, this fraction was only analyzed when phytoplankton biomass was high. These 12 samples coincided mostly with spring and autumn phytoplankton bloom events. We detected 4900 OTUs of free-living bacteria at a distance cut-off of 0.01 (after resampling to 4314 reads per sample), as described in Alonso-Sáez et al. (2015). SAR11 showed the highest percentage of reads (34.6% on average), followed by Flavobacteriales (14.6%) and Rhodobacterales (7.3%). For the particle-attached bacterial fraction, we found 3797 OTUs (in a dataset with 2535 reads per sample after resampling), with Flavobacteriales as the most abundant group (21.4% of total reads), followed by Rhodobacterales (11.0%) and Alteromonadales (5.6%).
To detect seasonal changes in bacterial community composition in the time-series study, we analyzed the Principal coordinates analysis (PCoA, Figure 2) and the Bray-Curtis dissimilarity index for each season and size fraction (Figure S2). We found the lowest average dissimilarity value in summer, while the dissimilarity of spring was significantly higher (t-test, p-value=0.013, n=11). Additionally, we found the highest dissimilarity for the particle-attached bacterial fraction, exceeding the free-living ones. No seasonal changes of the particle-attached microbial community composition were analyzed due to the low number of samples.
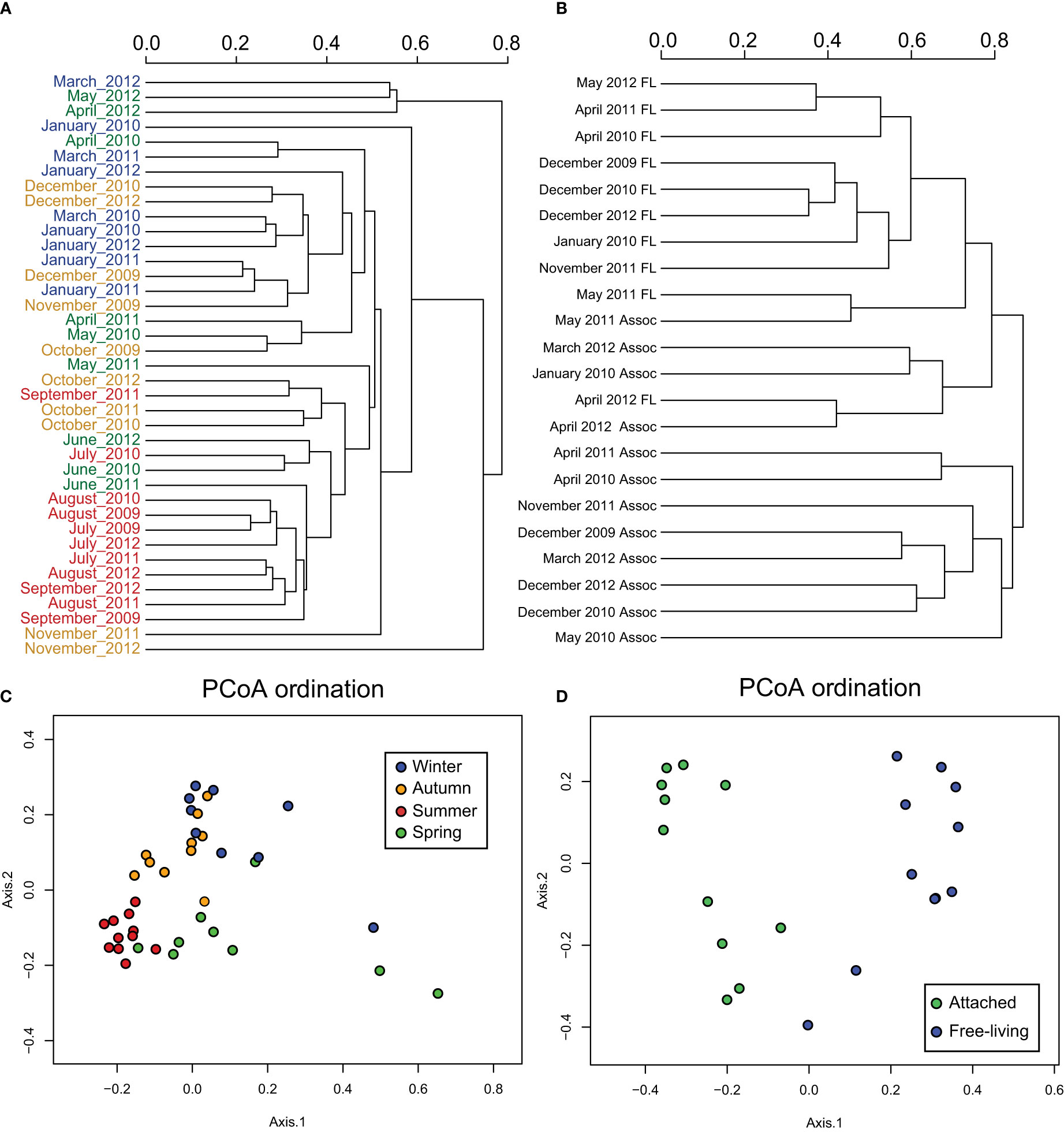
Figure 2 (A) Bray-Curtis dissimilarity tree between the free-living bacteria OTUs of each month, using different colors for each season: winter (blue), spring (green), summer (red) and autumn (orange). (B) Bray-Curtis dissimilarity tree between free-living and attached bacteria including only the dates when particle-attached samples were available. (C) Boxplot of Bray-Curtis dissimilarity values between the free-living fraction samples of individual seasons and (D) dissimilarity values between pooled free-living and particle-attached bacteria samples.
Network Analysis of Bacteria and Environmental Variables
We performed a Local similarity analysis (LSA) between 761 free-living bacterial OTUs, 273 phytoplankton groups and 5 environmental properties to infer biotic and abiotic correlations between them. The analysis resulted in 24689 significant correlations (Figure S3). In the other hand, due to the lower number of samples sequenced for the particle-attached fraction (12 samples in total), we considered the maximal information-based nonparametric exploration method (MINEv2, Reshef et al., 2011) more appropriate for this dataset.
Free-living bacteria-bacteria associations comprised in total 719 nodes (representing different OTUs in the case of bacteria) and 23073 significant edges, where 21769 of them were positive and only 1304 negative. Next, we investigated subnetworks of different phyla before and after EnDED analysis (Table 1). For example, the phyla with the lowest number of nodes, i.e., Actinobacteria, Planctomycetes, and Verrucomicrobia (range n=18-28 before EnDED n=3-10 after EnDED, Table 1), showed the highest number of edges per node and the lowest path length (L), indicating a high interconnection of these groups within the network (Table 1). By contrast, Bacteroidetes (Flavobacteriales) had a higher number of nodes (n=118 before EnDED and n=32 after EnDED) but showed the lowest edge density and relatively high L, which suggests a less interconnected network.
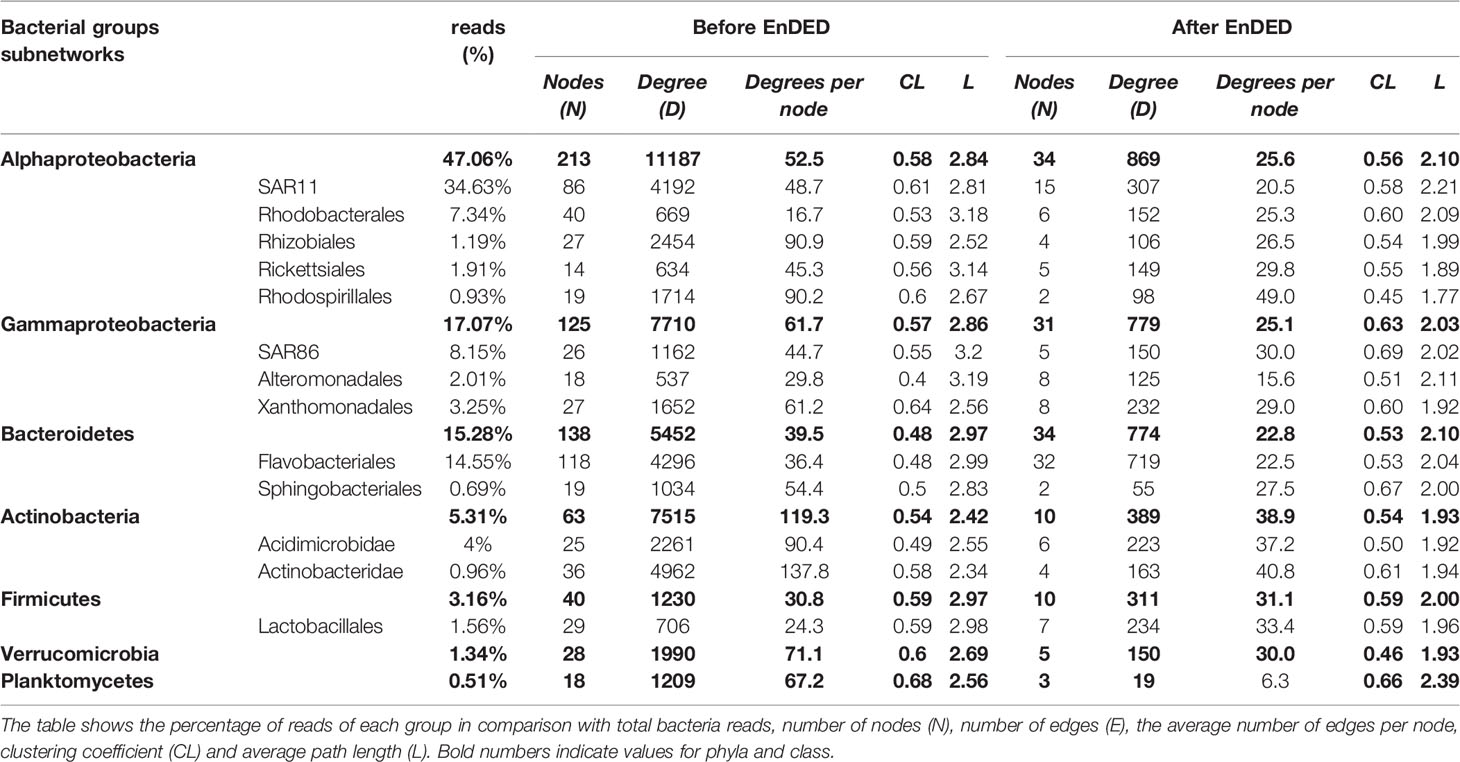
Table 1 Topological statistics for subnetworks corresponding to different bacterial groups (bold type for phyla and class) before and after applying EnDED.
Free-living bacteria correlations with environmental variables (Table 2) resulted in 344 nodes (5 environmental parameters and 338 bacterial OTUs) and 784 edges (with average absolute LS=0.58 ± 0.13). The highest percentage of edges between bacteria and environmental variables was found for bacterial OTUs and temperature (Table S1), which accounted for 31.0% and 22.6%, respectively, of total associations between bacteria and environmental variables. According to the CCA analysis, temperature also explains the distribution of the summer samples (Figure S4), while the distribution of the winter microbial community is explained by nutrient concentration. We found 69 nodes positively correlated with temperature, predominantly affiliated with Firmicutes (n=15), while OTUs negatively correlated with temperature were more abundant (65% of all OTU-Temperature edges). Although the most abundant SAR11 OTU correlated positively with temperature, we observed that this group also showed the highest number of OTUs negatively correlating with temperature (n=24), followed by Flavobacteriales (n=23) and Actinobacteridae (n=19) orders. Other environmental variables such as salinity or chlorophyll a concentration were correlated with only a few taxa. In particular, 17.9% of Verrucomicrobia OTUs correlated negatively with chlorophyll a, while inorganic nutrients concentrations correlated positively mostly with Actinobacteria and Xanthomonadales.
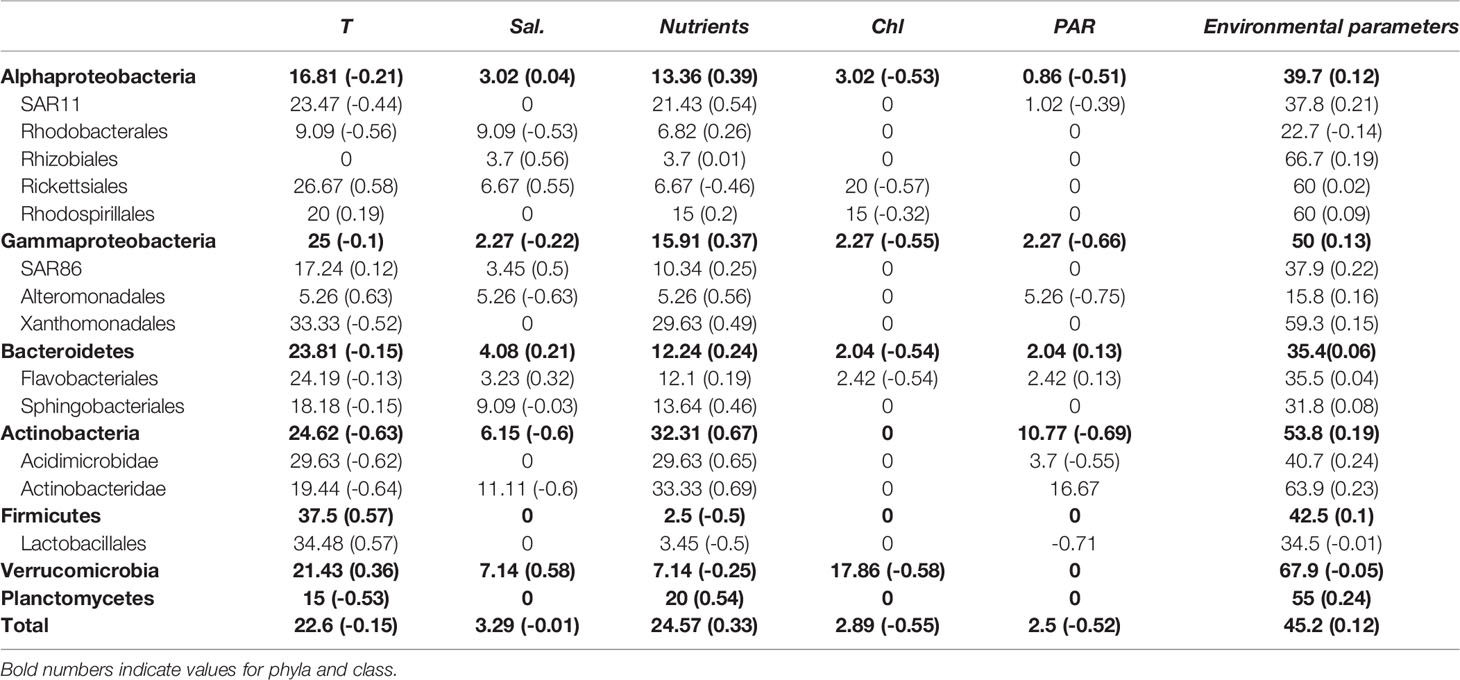
Table 2 Percentage of associations between bacterial groups and different environmental variables: Temperature (T), salinity (Sal.), nutrients, chlorophyll a concentration (Chl-a), and photoynthetically available radiation (PAR, 400-700 nm).
We also analyzed the correlations between different phytoplankton taxa with environmental parameters (Figure S5). We found that most of the groups correlated positively with inorganic nutrient concentration (nitrate, phosphate or silicate, n=8). The Naviculales showed the highest number of nodes correlated with nitrate and phosphate. Haslea spp. and Navicula spp. genera were positively correlated with both nutrients and Pleurosigma spp. with nitrate. sSome groups such as Navicula spp. or Thalassiosira spp. were negatively correlated with temperature. Silicate showed also a strong positive correlation with Haslea spp. and members of the order Dictyochales.
Network Analysis Between Bacteria in the Free-Living and Particle-Attached Fractions
To analyze the free-living bacteria-bacteria associations, we removed the edges that were due to environmental preferences using the EnDED method, (see M&M for more information). In addition, we removed isolated nodes, i.e., nodes without edges. The number of total bacterial nodes and bacterial edges decreased considerably (see Table 1). To estimate preferential associations between different microbial groups, we calculated the fraction of observed from all potential associations (i.e., edge density) between specific groups. In the free-living fraction we observed the highest edge density among low-abundant groups such as between Epsilonproteobacteria and Deltaproteobacteria, between Epsilonproteobacteria and Deltaproteobacteria with SAR202, or between distinct Nitrospina and Verrucomicrobia OTUs (Figure 3A). On the other hand, edge density between bacteria in the particle-attached subnetwork was considerably lower, with the Phycisphaerae and Verrucomicrobiales class showing the highest edge densities with other OTUs of the same class (Figure 3B).
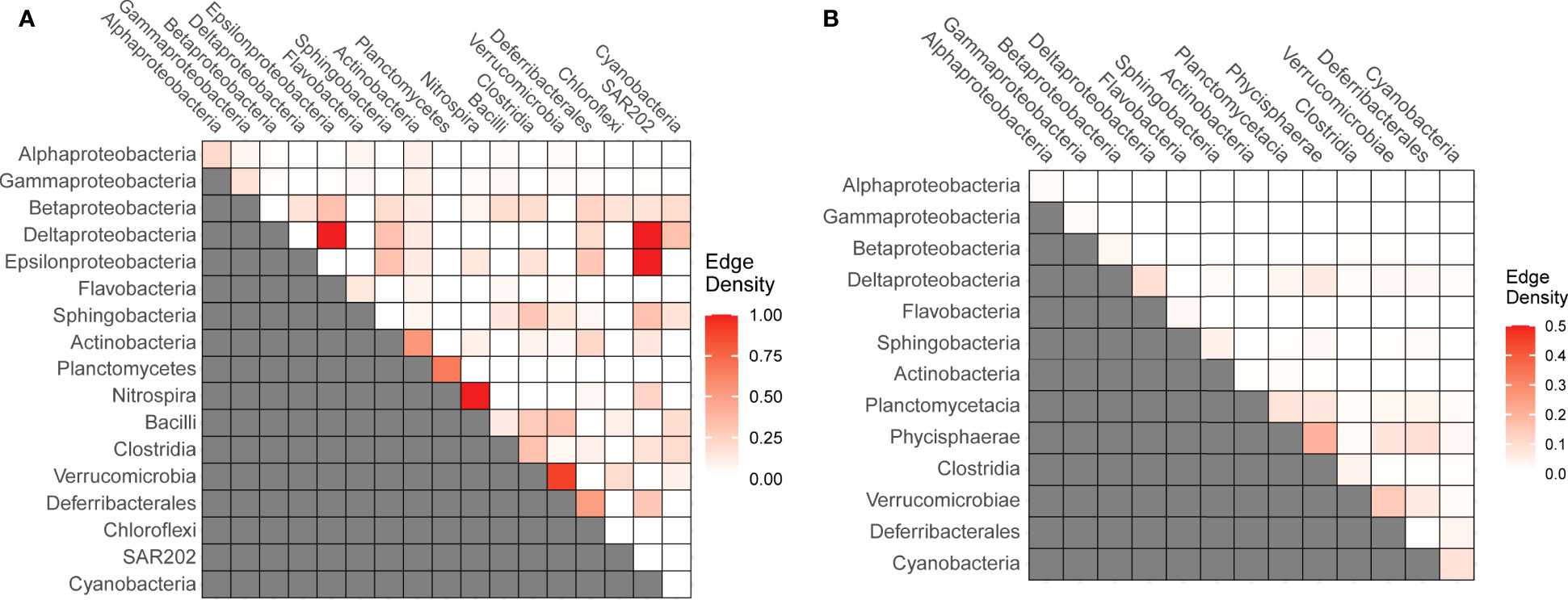
Figure 3 Edge-density indicating the fraction observed from all potential associations between specific bacterial groups in the free-living (A) and particle-attached fractions (B).
Network Analysis Between Bacteria and Phytoplankton Taxonomic Groups
After applying EnDED to remove indirect bacteria-phytoplankton associations, the biotic network (which includes free-living bacterial OTUs and phytoplankton genus) decreased considerably in size. Among the bacteria-phytoplankton associations we found 8 diatom groups (with 32 positive associations with bacterial taxa) and only 2 dinoflagellates (with only 3 positive associations, Figure 4). Flavobacteriales showed the highest number of correlations with distinct phytoplankton groups in the free-living fraction, including 4 diatoms (Naviculales spp., Thalassiosira spp., Chaeroceros spp. and an unknown centric diatom) and two dinoflagellates (Minuscula spp. and one unknown thecate dinoflagellate). Additionally, we found specific associations between 2 free-living SAR11 OTUs with the order Naviculales (Haslea genus). Similarly, free-living Rhodobacterales were correlated with the genus Thalassiosira (with 3 out of 4 OTUs in the network correlating with this diatom taxon). The phylum Actinobacteria showed specific correlations with Cylindrotheca spp., with the 3 OTUs present in the network correlating with this diatom taxa, representing 75% of all Cylindrotheca-bacteria associations.
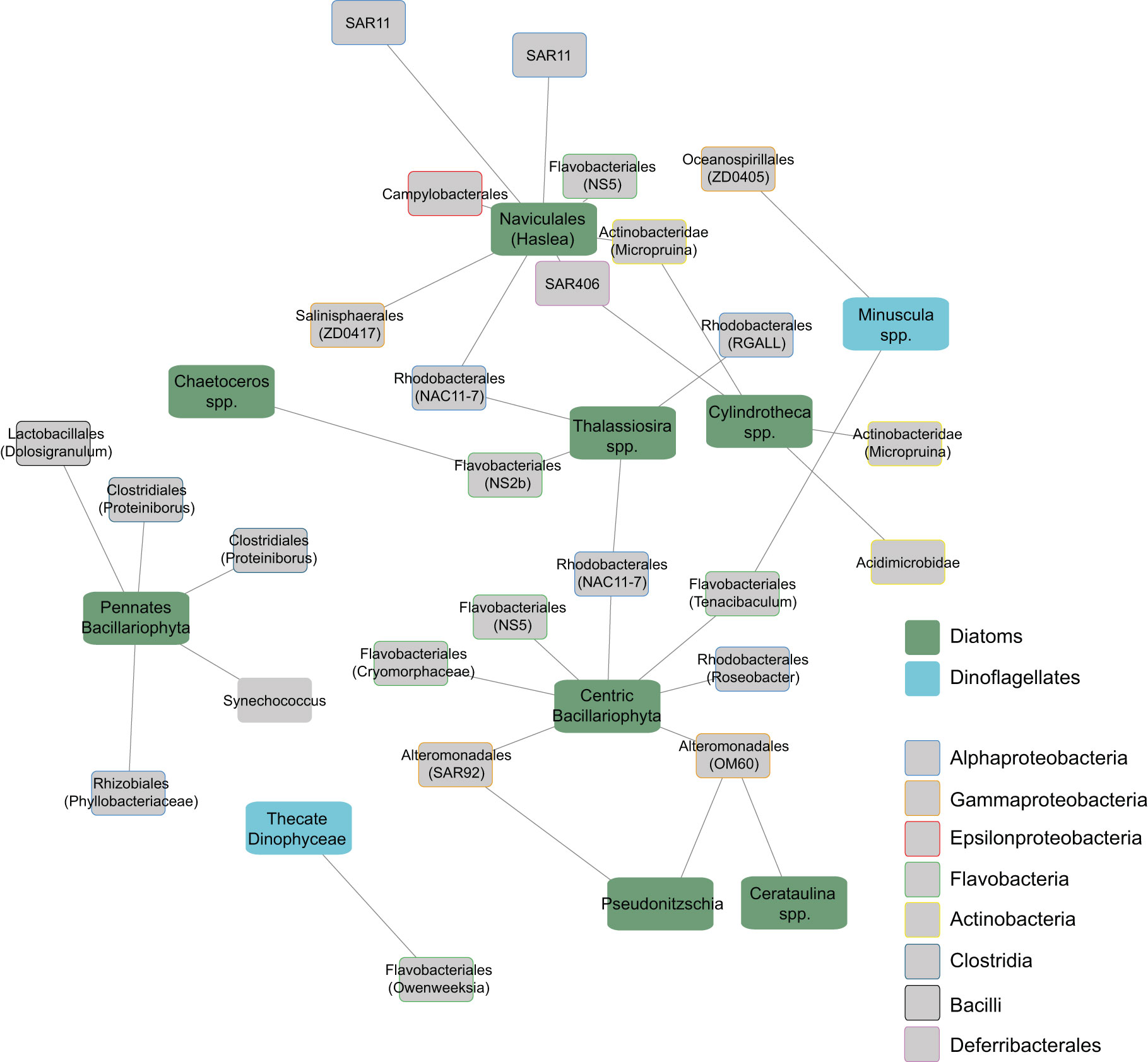
Figure 4 Subnetwork showing positive correlations between free-living bacterial OTUs and phytoplankton groups. The network was constructed with LSA, and environmentally-driven associations were removed with EnDED. Edge length reflects the association strength measured via the LS score. Phytoplankton groups are differentiated between diatoms and dinoflagellates. Bacterial groups are differentiated by classes using different box-border colors and node labels indicate the order. Names between parentheses indicate the most specific taxonomical level identified for each OTU.
In general, the phytoplankton genera showing associations with particle-attached bacteria were different from those interacting with free-living bacteria (Figure 5), with the exception of Cylindrotheca spp. and Pseudonitzia spp., which appeared associated with Actinobacteria and Alteromonadales in both fractions, respectively. Although most of the particle-attached bacteria-phytoplankton associations took place with diatoms (80% of all edges), we found that Flavobacteriales and Actinobacteridae, the groups with the largest number of edges (12 edges each) were associated indistinctly with diatoms and dinoflagellates. Among diatoms, Leptocylindrus spp. was the genus with the highest number of edges with bacterial OTUs (7 edges), including several of the Planctomycetes phylum.
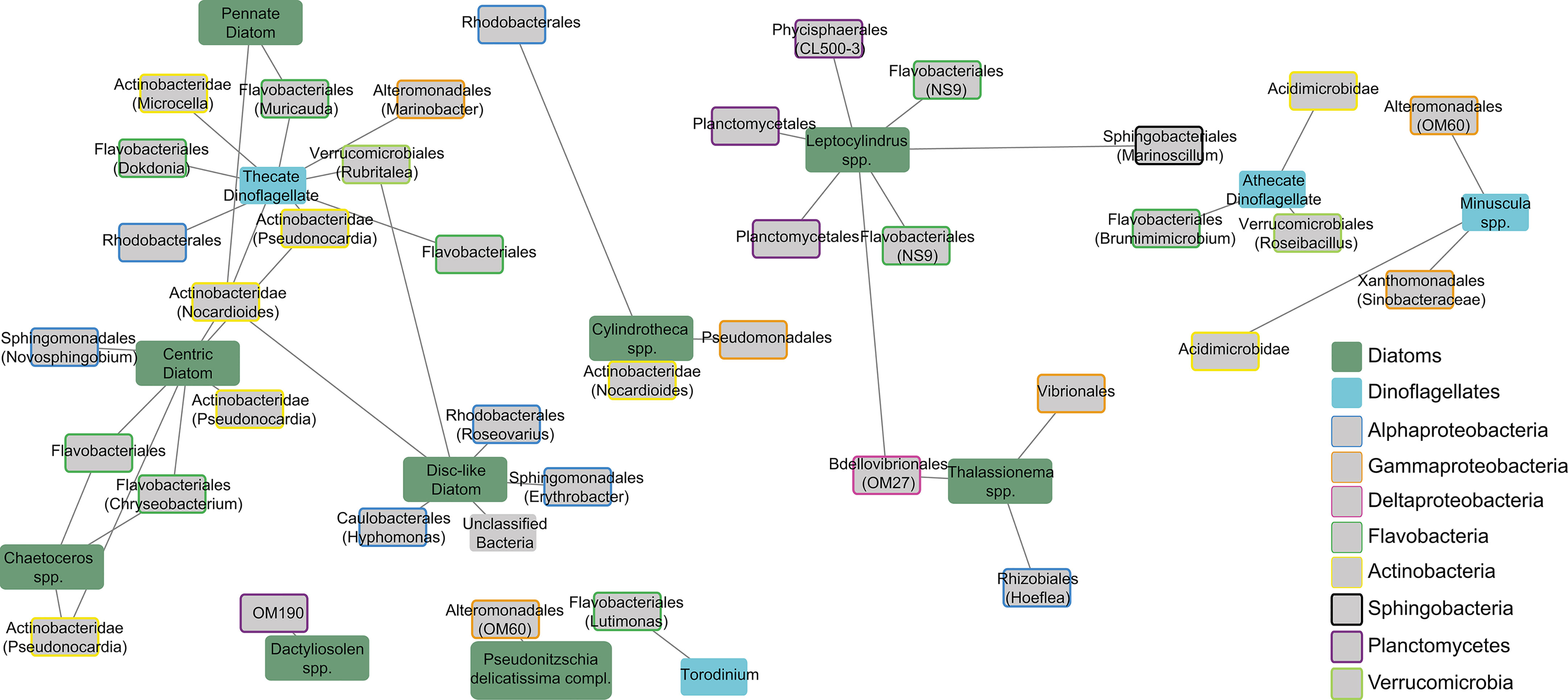
Figure 5 Subnetwork showing positive associations between particle-attached bacterial OTUs and phytoplankton groups. Edge length reflects the association strength measured via the MIC score. Phytoplankton groups are differentiated between Diatoms and Dinoflagellates. different box-border colors indicate distinct bacterial groups. Node labels indicate the order and the names between parentheses indicate the most specific taxonomical level identified for each OTU.
Discussion
The study of marine microbial temporal and spatial dynamics and their controlling drivers has regained more attention with the latest advances in network analysis (see review in Fuhrman et al., 2015). Classically, abiotic factors have been considered the main controlling variables of marine microbial dynamics (Gilbert et al., 2012; Giovannoni and Vergin, 2012; Sunagawa et al., 2015). Yet, some studies have shown that the interactions between different organisms may be at least as important as the abiotic factors (El-Swais et al., 2015; Lima-Mendez et al., 2015; Worden et al., 2015). Biotic associations occurring between heterotrophic bacteria and phytoplankton have been shown to be particularly relevant driving the microbial community dynamics during phytoplankton blooms (Bunse et al., 2016; Needham and Fuhrman, 2016), but the impact of such associations shaping the microbial community composition at seasonal and yearly time scales remain largely unknown. Furthermore, a strong yearly rhythmicity of small autotrophic and heterotrophic microbes recently shown (Lambert et al., 2019; Lambert et al., 2021), suggest re-occurrent seasonal biotic associations between bacteria and phototrophic picoeukaryotes in marine waters. Here, we unveiled novel seasonal biotic associations between bacteria and large microphytoplankton taxa in a temperate ecosystem, which have been previously addressed solely in the context of short-term phytoplankton bloom conditions.
The fact that low-abundant bacterial OTUs (such as Actinobacteridae, Verrucomicrobiales, or Planctomycetales), rather than abundant OTUs, dominated the bacteria-bacteria associations in the free-living and particle-attached networks is in agreement with previous studies (Faust et al., 2015; Lima-Mendez et al., 2015). The high edge density within the bacteria-bacteria subnetwork still remained after removing environmentally-driven associations with EnDED, supporting the view that some of these associations between low-abundant groups may be related to interactions between different taxa. Our results indicate a co-assembly between taxa like Deltaproteobacteria and SAR202, or between distinct OTUs of Nitrospira, Planctomycetes, or Verrucomicrobia, which are low-abundant yet biogeochemically relevant groups in coastal marine ecosystems (Freitas et al., 2012; Koch et al., 2015; Delmont et al., 2018). Associations between Planctomycetes and Nitrospira may be related to a potential cooperation in the cycling of nitrogen, as members of this group participate in N2 fixation (Delmont et al., 2018) and nitrification (Daims et al., 2015). In the case of SAR202 and Deltaproteobacteria, while we cannot identify the functional reason for these potential co-assemblies, it is noticeable that these two taxa usually co-occur along the vertical profile in the open ocean (Galand et al., 2010; Cardozo-Mino et al., 2021).
Low-abundant bacterial taxa also showed more associations with environmental parameters compared to high-abundant ones (Table 2). We found that a major fraction of these low-abundant taxa (72%) was negatively correlated with temperature (Figure S2). This result agrees with previous observations showing that the abundance of rare bacterial taxa is maximal in winter, when water column mixing transports bacterial taxa usually found below the pycnocline to the surface (Vergin et al., 2013; Alonso-Saez et al., 2015), increasing the overall diversity of the upper layers (Garcia et al., 2015). Although temperature was the main abiotic variable determining the free-living microbial dynamics, as previously observed in other datasets (Raes et al., 2011; Sunagawa et al., 2015), other variables such as chlorophyll a and inorganic nutrient concentrations also determined a large fraction of bacteria-environmental variable associations. For example, members of the order Verrucomicrobiales showed a high number of negative correlations with chlorophyll a and nutrient concentration, which may indicate that some Verrucomicrobiales OTUs thrive in more oligotrophic conditions, as suggested by the observed preference of this group for summer stratified conditions (Alonso-Saez et al., 2015). Surprisingly, OTUs from groups usually associated with phytoplankton biomass such as Flavobacterales and Alteromonadales (Riemann et al., 2000; Kirchman, 2002; Tada et al., 2011) did not positively correlate with chlorophyll a concentration, perhaps because chlorophyll a is not always a good indicator of the availability and lability of DOM that is incorporated by heterotrophic bacteria (Morán and Scharek, 2015). Our results coincide with other works showing that the influence of chlorophyll a concentration on microbial seasonal dynamics is not as relevant as other parameters like temperature (Steele et al., 2011; Chow et al., 2013; Krabberød et al., 2022), suggesting that chlorophyll concentration per se cannot predict the dynamics of individual bacterial OTUs.
Despite the importance of environmental variables shaping the composition of the microbial community, we found stronger correlations between distinct taxonomic groups of free-living bacteria and phytoplankton than with environmental parameters (as indicated by the higher LS score in Figure S6). Such specific associations between free-living bacteria and phytoplankton may also explain the high dissimilarity in bacterial community composition observed between different years in spring (Figure 2). For example, conspicuous changes in the dominant phytoplankton taxa were found in May 2011 and 2012, with a high contribution of the dinoflagellate Gymnodinium spp. or the diatom Pseudo-nitzschia spp., respectively, likely impacting the composition of bacterial assemblages. As rapid changes in phytoplankton species during spring blooms take place over the scale of days (Needham and Fuhrman, 2016), and these changes could not be resolved at the time scale of our monthly sampling, this may result in the apparent lack of inter-annual reoccurrence in spring.
Correlations of bacterial taxa with diatoms dominated the free-living bacteria-phytoplankton subnetwork, which may be due to the higher abundance of diatoms, with dinoflagellates only producing sporadic blooms during the 3.5-year time-series. However, a strong impact of diatoms on the free-living bacterial community should not be disregarded, as a preference for diatom-derived organic matter by most bacterial taxa has been previously shown (Sarmento et al., 2013). Rhodobacteraceae has been described as a major component of the bacterioplankton community during diatom blooms (Teeling et al., 2012; Klindworth et al., 2014; Teeling et al., 2016). Interestingly, many of these works showed a high contribution of Rhodobacteraceae during Thalassiosira sp. blooms (Riemann et al., 2000; Teeling et al., 2012), and cell-specific interactions of Rhodobacteraceae with diatoms (including Thalassiosira) through ammonium cross-feeding has been recently evidenced (Zecher et al., 2020). Our results support the idea of a preferential association between these two groups also along the seasonal scale, particularly for the Rosebacter genus (mostly NAC11-7 clade). However, the fact that Rhodobacterales was also associated with other phytoplankton groups such as Naviculales highlights their ability to closely interact with different diatoms (Buchan et al., 2005; Buchan et al., 2014). Flavobacteriales, another major group typically related to phytoplankton blooms (Abell and Bowman, 2005; Rinta-Kanto et al., 2012; Bunse et al., 2016) showed the highest number of nodes and edges with different diatom and dinoflagellate groups, suggestive of their broad ability to interact with different phytoplankton taxa. This result may also be related to the ability of Flavobacteria to use DOM from different sources of phytoplankton (Cottrell and Kirchman, 2000; Williams et al., 2013; Buchan et al., 2014).
Beyond these well-described phytoplankton-bacteria interactions, we found other interesting biotic associations with taxa that are typically not dominant under phytoplankton blooms. In particular, we found a specific association between two SAR11 OTUs representing up to 5.9% of total SAR11 abundance, with the diatom order Naviculales. It should be noted that we found no other correlations between SAR11 and phytoplankton taxa, and our results agree with previous studies showing a higher abundance of some SAR11 OTUs under the presence of diatoms (Carlson et al., 2009; Eiler et al., 2009). Some members of Naviculales produce high amounts of sulphur-containing organic compounds (Busby and Benson, 1973), which may alleviate the requirement for reduced sulphur by SAR11 for growth (Tripp et al., 2008). However, the exudates of other phytoplankton taxa are also a source of these compounds, and therefore, the underlying mechanism behind the potential specific association of SAR11 with Naviculales remains unknown.
Another interesting specific biotic association detected in the free-living bacteria-phytoplankton subnetwork was the association of Actinobacteria OTUs (which represent the 5.3% of all bacterial OTUs) with Cylindrotheca spp. Increasing abundance of Actinobacteria under the presence of a Cylindrotheca spp. inoculum has been recently described (Zhang and Chi, 2020), while our data further suggest that, under environmental conditions, some Actinobacteria (from the Acidimicrobineae and Propionibacterineae suborders) may have taxa-specific interactions with this diatom groups.
In the particle-attached fraction, the high number of edges between bacteria and phytoplankton detected (with 40% more nodes and 36% more edges than in the free-living fraction considering a maximal information coefficient, MIC>0.7) can be due to the distinct methods used for the network construction (Local similarity analysis, LSA, for free-living and Maximal Information-based Non-parametric Exploration, MINE, for particle-attached fraction). However, the larger microbial composition dissimilarity found in the particle-attached fraction than in the free-living one (Figure 2D) suggests a closer bacteria-phytoplankton interactions in the attached fraction, as observed in previous works (Jasti et al., 2005; Rooney-Varga et al., 2005; Grossart et al., 2007; Arandia-Gorostidi et al., 2017). Specific interactions between phytoplankton and their associated bacteria have already been shown in previous works (Sapp et al., 2007; Ramanan et al., 2015). These associations are often linked to the exchange of essential nutrients for growth, such as B-vitamins (Croft et al., 2005). It has also been observed that bacteria associated with the phycosphere have stronger interactions with phytoplankton cells than the free-living cells (Rooney-Varga et al., 2005; Grossart et al., 2007), most likely due a higher ability for trophic interactions within the phycosphere (Arandia-Gorostidi et al., 2017). Our results support these observations, and further suggests that taxa-specific associations are frequent in coastal areas.
Within the attached fraction network, the Flavobacteria showed the highest number of edges with phytoplankton, highlighting their role as phytoplankton-derived organic matter consumers within and outside the phycosphere. Surprisingly, Actinobacteria (mostly dominated by the Nocardioides and Pseudonocardia genera) was as abundant as Flavobacteria in the particle-attached bacteria-phytoplankton subnetwork, even if their total contribution to the attached fraction was low. Although the ability of Actinobacteria to incorporate complex alga-derived organic matter has been reported (Tada and Suzuki, 2016), they are usually more abundant as free-living cells (Needham and Fuhrman, 2016; Mestre et al., 2017). Yet, our data suggest that different Actinobacteria OTUs can develop tight associations by attachment to specific phytoplankton cells and, thus, they should be also considered as an important component of the phycospheres’ microbiome. Another interesting observation is the unique association of the Planctomycetes phyla (which includes the Planctomycetales and Phycisphaerales orders) with Leptocylindrus spp. Although associations of Planctomycetes with diatoms have been previously observed (Morris et al., 2006; Allen et al., 2012), our data indicates that this phylum may develop strong interactions with some phytoplankton groups, partially determining the seasonal abundance of this bacterial group.
In summary, this study expands previous reports describing the role of potential bacteria-phytoplankton associations in the dynamics of marine bacteria (El-Swais et al., 2015; Lima-Mendez et al., 2015) to show the role of potential taxon-specific interactions, not only in short-time periods (Bunse et al., 2016; Needham and Fuhrman, 2016) but also at annual and interannual time-scales. To predict the future dynamics of bacterial communities under a changing environment, the response of such potential interactions should be considered. While temperature has been shown to be the main environmental variable driving microbial distribution, the high number of edges between bacteria and phytoplankton and the higher absolute strength of these connections (indicated as LS value), suggest that bacteria-phytoplankton associations were at least as relevant as environmental factors in shaping the dynamics of the bacterial community. These observations could also be true for other phytoplankton groups, including pico-phytoplankton, as species-specific interactions within the phycoshere in the latter group have been reported (Lupette et al., 2016). However, stronger trophic interactions in the exchange of C and N was observed between bacteria and microphytoplankton, as compared to picophytoplankton (Arandia-Gorostidi et al., 2017, Arandia-Gorostidi et al., 2020), reflecting the ecological relevance of the associations studied here. Although more detailed experimental studies are required to explain the underlying mechanisms of these potential interactions, this work represents an important step forward to elucidate bacteria-microphytoplankton interactions in coastal oceans for a diverse taxonomic groups.
Experimental Procedures
Samples Collection and DNA Extraction
Between July 2009 and December 2012, surface seawater samples (5m depth) were collected monthly at the station E2 (43.675°N, 5.578°W) located 13 km off Gijón/Xixón (Spain) in the Southern Bay of Biscay. Samples were collected in 5 L Niskin bottles and transported to the laboratory in dark conditions within 6 hours. Additionally, 250 mL of seawater was fixed with acid lugol’s solution for phytoplankton taxonomical analysis. Seawater in situ conditions (temperature and conductivity) were measured by CTD a SeaBird 25 CTD probe and photosynthetically active radiation (PAR, 400-700 nm) was measured with a Biospherical QSP-2200 spherical quantum sensor. Chlorophyll a concentration (Chl a) was obtained by filtrating 100 ml samples through glass fibre filters (Whatman GF/F). Filters were frozen at -80°C until the analysis. Pigments were extracted by incubating in 90% acetone for 24 h in the dark at 4°C. Chl a was measured using a Perkin Elmer LB-50s spectrofluorometer calibrated with pure Chl a following the protocol by Neveux and de Billy (1986). Samples for nutrients analysis were frozen after the collection and analyzed using a Technicon autoanalyzer as detailed in Calvo-Díaz and Morán (2006).
Once in the laboratory, approximately 2 L of seawater was sequentially filtered through 3 µm pore size polycarbonate filters (GTTP, 25mm, Millipore, Eschborn, Germany), to collect bacteria attached to phytoplankton and particles, and through 0.2 µm filters (GTTP, 25mm, Millipore, Eschborn, Germany), to retain free-living bacteria. In total, we collected 39 samples of free-living bacteria (0.2 µm filters) and a subset of 12 samples coinciding with high chlorophyll concentrations for particle-attached bacteria (3 µm filters). Filters were stored at -80°C until DNA extraction, which was carried out with a PowerWater DNA Isolation Kit (Mobio, Carlsbad, CA, USA). 16S rDNA tags were amplified using the bacterial general primers 341F and 805R (Herlemann et al., 2011). Finally, amplicons were sequenced on a 454 FLX+ platform and analyzed using the MOTHUR platform (Schloss et al., 2009) in two separate sets (corresponding to the free-living and particle-attached). The analysis of both fractions was performed as described in Alonso-Saez et al., 2015. While the free-living sequences were included in Alonso-Sáez et al. (2015), the particle-attached bacteria sequence dataset is published for the first time in this study. The resulting sequences were downsized to 4314 (in the free-living fraction) and 2532 reads (in the particle-attached fraction) by random re-sampling to equal the depth of sequencing for all samples in each dataset.
Phytoplankton taxonomical analysis was carried out using the inverted microscope method (Utermöhl, 1958). Volumes between 25 mL and 100 mL of the Lugol’s fixed samples, depending on the chlorophyll concentration, were transferred to sedimentation columns. After 24 hours of cell sedimentation, phytoplankton taxa were identified and counted using a NIKON inverted microscope with a 10X and 20X magnifications.
Network Analysis
Using the local similarity analysis (LSA) program (Ruan et al., 2006), we inferred correlations between 761 free-living bacterial OTUs (present in at least 25% of the samples), 273 phytoplankton taxonomical groups, and five environmental parameters: Temperature, salinity, chlorophyll concentration, inorganic nutrient concentration (nitrate and phosphate) and photosynthetically active radiation (PAR). For each pairwise comparison, LSA calculates the local similarity (LS) score, determines the p-value and a false-discovery-rate corrected q-value (Storey and Tibshirani, 2003). Only associations with significance p and q-value < 0.05 were considered for further analysis.
Next, we identified and removed indirect edges between bacterial OTUs and/or phytoplankton groups that were due to similar or different environmental preferences using the tool EnDED (Deutschmann et al., 2021). Shortly, EnDED evaluates associations between two OTUs and/or phytoplankton taxa that are both connected to the same environmental factor and discriminates if the microbial edge (or associations) was caused by a similar or contrary environmental preferences. We used the intersection combination approach of the four methods (Sign Pattern, Overlap, Interaction Information, and Data Processing Inequality) and set the number of permutations to 10000. Before applying EnDED, we removed rare OTUs from the network, i.e., we considered only the OTUs that were present in more than 10% of the samples and had a cumulative abundance higher than 100 reads. Additionally, before the EnDED filtration we also removed the phytoplankton groups that were less abundant than 1% of the total phytoplankton abundance at least in one of the samples.
Due to the lower number of samples sequenced for the particle-attached fraction (12 samples in total) and the lack of temporal equidistance between them, as required for LSA analysis, we used the maximal information-based nonparametric exploration (MINEv2, Reshef et al., 2011) analysis for this dataset. A correlation matrix was generated using 518 OTUs (those present at least in the 25% of the samples) and 98 phytoplankton taxa. MINE was run using default parameter values for alpha, c, and TIC. All variables were analyzed as pairs. Due to the large number of correlations obtained, to build the network only strong associations (with a MIC>0.7) were considered for further analysis.
Both networks comprise nodes, which represent the variables used in the analysis (i.e., bacterial OTUs, phytoplankton groups, or environmental parameters), and edges (associations), which indicate significant correlations (positives or negatives) between the nodes. We visualized the networks using Cytoscape 3.4.0 (Shannon et al., 2003). Starting from the main network, we generated subnetworks to observe specific correlations between different bacterial OTUs, between bacterial OTUs and environmental parameters, and between bacterial OTUs and different phytoplankton groups.
Network Statistics
For the MINE analysis between particle-attached bacterial OTUs and phytoplankton, the strength of the correlation was calculated by the Mutual Information Coefficient (MIC). For LSA analysis, four statistics were calculated: 1) Local Similarity Score (LS) (Ruan et al., 2006) representing the strength of the correlations between two nodes; 2) Average shortest path length (L) represents the average smallest number of steps (number of nodes) between all node pairs; 3) average clustering coefficient (Cl) of the network quantifies the interconnection within the network, i.e., how well the network is clustered (high clustering indicates that a nodes association partners tend to be connected as well); 4) Edge-density is the fraction between observed and potential edges indicating network connectivity.
Data Availability Statement
The datasets presented in this study can be found in online repositories. The names of the repository/repositories and accession number(s) can be found below: https://www.ebi.ac.uk/ (accession: PRJEB6399).
Author Contributions
NA-G, LA-S, and XM designed the experiments and NA-G and LA-S analyzed the 16S rRNA amplicons. NA-G, AK, and RL performed the LSA analysis and ID analyzed the resulting networks with EnDED software. RS and FG performed the phytoplankton taxonomical analysis. NA-G executed the MINE analysis. All authors contributed to the manuscript writing. All authors contributed to the article and approved the submitted version.
Funding
Spanish Ministry of Economy and Competitiveness (MINECO) (CTM-2010–15840).
Conflict of Interest
The authors declare that the research was conducted in the absence of any commercial or financial relationships that could be construed as a potential conflict of interest.
Publisher’s Note
All claims expressed in this article are solely those of the authors and do not necessarily represent those of their affiliated organizations, or those of the publisher, the editors and the reviewers. Any product that may be evaluated in this article, or claim that may be made by its manufacturer, is not guaranteed or endorsed by the publisher.
Acknowledgments
We are grateful to Basque Government for supporting NA-G’s Ph.D. fellowship the Spanish Ministry of Economy and Competitiveness (MINECO) for supporting LA-S’s Juan de la Cierva and Ramón y Cajal fellowship (RYC-2012-11404) and the COMITE project (CTM-2010–15840). We are very thankful to all the staff of the R/V “José de Rioja” for their help during the sampling collection.
Supplementary Material
The Supplementary Material for this article can be found online at: https://www.frontiersin.org/articles/10.3389/fmars.2022.901201/full#supplementary-material
References
Abell G. C., Bowman J. P. (2005). Colonization and Community Dynamics of Class Flavobacteria on Diatom Detritus in Experimental Mesocosms Based on Southern Ocean Seawater. FEMS Microbiol. Ecol. 53, 379–391. doi: 10.1016/j.femsec.2005.01.008
Allen L., Allen E. E., Badger J. H., McCrow J. P., Paulsen I. T., Elbourne L. D., et al. (2012). Influence of Nutrients and Currents on the Genomic Composition of Microbes Across an Upwelling Mosaic. ISME. J. 6, 1403–1414. doi: 10.1038/ismej.2011.201
Alonso-Saez L., Diaz-Perez L., Moran X. A. (2015). The Hidden Seasonality of the Rare Biosphere in Coastal Marine Bacterioplankton. Environ. Microbiol. 17, 3766–3780. doi: 10.1111/1462-2920.12801
Amin S. A., Green D. H., Hart M. C., Kupper F. C., Sunda W. G., Carrano C. J. (2009). Photolysis of Iron-Siderophore Chelates Promotes Bacterial-Algal Mutualism. Proc. Natl. Acad. Sci. U. S. A. 106, 17071–17076. doi: 10.1073/pnas.0905512106
Amin S. A., Hmelo L. R., van Tol H. M., Durham B. P., Carlson L. T., Heal K. R., et al. (2015). Interaction and Signalling Between a Cosmopolitan Phytoplankton and Associated Bacteria. Nature 522, 98–101. doi: 10.1038/nature14488
Arandia-Gorostidi N., Weber P. K., Alonso-Saez L., Moran X. A., Mayali X. (2017). Elevated Temperature Increases Carbon and Nitrogen Fluxes Between Phytoplankton and Heterotrophic Bacteria Through Physical Attachment. ISME. J. 11, 641–650. doi: 10.1038/ismej.2016.156
Arandia-Gorostidi N., Alonso-Sáez L., Stryhanyuk H., Richnow H. H., Morán X. A.G., Musat N (2020). Warming the phycosphere: Differential effect of temperature on the use of diatom‐derived carbon by two copiotrophic bacterial taxa. Environmental Microbiology 22(4). doi: 10.1111/1462-2920.14954
Buchan A., Gonzalez J. M., Moran M. A. (2005). Overview of the Marine Roseobacter Lineage. Appl. Environ. Microbiol. 71, 5665–5677. doi: 10.1128/AEM.71.10.5665-5677.2005
Buchan A., LeCleir G. R., Gulvik C. A., Gonzalez J. M. (2014). Master Recyclers: Features and Functions of Bacteria Associated With Phytoplankton Blooms. Nat. Rev. Microbiol. 12, 686–698. doi: 10.1038/nrmicro3326
Bunse C., Bertos-Fortis M., Sassenhagen I., Sildever S., Sjoqvist C., Godhe A., et al. (2016). Spatio-Temporal Interdependence of Bacteria and Phytoplankton During a Baltic Sea Spring Bloom. Front. Microbiol. 7, 517. doi: 10.3389/fmicb.2016.00517
Bunse C., Pinhassi J. (2017). Marine Bacterioplankton Seasonal Succession Dynamics. Trends Microbiol. 25, 494–505. doi: 10.1016/j.tim.2016.12.013
Busby W. F., Benson A. A. (1973). Sulfonic Acid Metabolism in the Diatom Navicula Pelliculosa. Plant Cell Physiol. 14, 1123–1132. doi: 10.1093/oxfordjournals.pcp.a074951
Calvo-Díaz A., Morán X. A. G. (2006). Seasonal Dynamics of Picoplankton in Shelf Waters of the Southern Bay of Biscay. Aquat. Microbial. Ecol. 42, 159–174. doi: 10.3354/ame042159
Cardozo-Mino M. G., Fadeev E., Salman-Carvalho V., Boetius A. (2021). Spatial Distribution of Arctic Bacterioplankton Abundance Is Linked to Distinct Water Masses and Summertime Phytoplankton Bloom Dynamics (Fram Strait, 79 Degrees N). Front. Microbiol. 12, 658803. doi: 10.3389/fmicb.2021.658803
Carlson C. A., Morris R., Parsons R., Treusch A. H., Giovannoni S. J., Vergin K. (2009). Seasonal Dynamics of SAR11 Populations in the Euphotic and Mesopelagic Zones of the Northwestern Sargasso Sea. ISME. J. 3, 283–295. doi: 10.1038/ismej.2008.117
Chow C. E., Sachdeva R., Cram J. A., Steele J. A., Needham D. M., Patel A., et al. (2013). Temporal Variability and Coherence of Euphotic Zone Bacterial Communities Over a Decade in the Southern California Bight. ISME. J. 7, 2259–2273. doi: 10.1038/ismej.2013.122
Cooper M. B., Smith A. G. (2015). Exploring Mutualistic Interactions Between Microalgae and Bacteria in the Omics Age. Curr. Opin. Plant Biol. 26, 147–153. doi: 10.1016/j.pbi.2015.07.003
Cottrell M. T., Kirchman D. L. (2000). Natural Assemblages of Marine Proteobacteria and Members of the Cytophaga-Flavobacter Cluster Consuming Low- and High-Molecular-Weight Dissolved Organic Matter. Appl. Environ. Microbiol. 66, 1692–1697. doi: 10.1128/AEM.66.4.1692-1697.2000
Cram J. A., Xia L. C., Needham D. M., Sachdeva R., Sun F., Fuhrman J. A. (2015). Cross-Depth Analysis of Marine Bacterial Networks Suggests Downward Propagation of Temporal Changes. ISME. J. 9, 2573–2586. doi: 10.1038/ismej.2015.76
Croft M. T., Lawrence A. D., Raux-Deery E., Warren M. J., Smith A. G. (2005). Algae Acquire Vitamin B12 Through a Symbiotic Relationship With Bacteria. Nature 438, 90–93. doi: 10.1038/nature04056
Cruz-Lopez R., Maske H. (2016). The Vitamin B1 and B12 Required by the Marine Dinoflagellate Lingulodinium Polyedrum Can be Provided by its Associated Bacterial Community in Culture. Front. Microbiol. 7, 560. doi: 10.3389/fmicb.2016.00560
Daims H., Lebedeva E. V., Pjevac P., Han P., Herbold C., Albertsen M., et al. (2015). Complete Nitrification by Nitrospira Bacteria. Nature 528, 504–509. doi: 10.1038/nature16461
Delmont T. O., Quince C., Shaiber A., Esen O. C., Lee S. T., Rappe M. S., et al. (2018). Nitrogen-Fixing Populations of Planctomycetes and Proteobacteria are Abundant in Surface Ocean Metagenomes. Nat. Microbiol. 3, 804–813. doi: 10.1038/s41564-018-0176-9
Deutschmann I. M., Lima-Mendez G., Krabberød A. K., Raes J., Vallina S. M., Faust K., et al. (2021). Disentangling Environmental Effects in Microbial Association Networks. Microbiome 9, 232. . doi: 10.1186/s40168-021-01141-7
Dupont C. L., Rusch D. B., Yooseph S., Lombardo M. J., Richter R. A., Valas R., et al. (2012). Genomic Insights to SAR86, an Abundant and Uncultivated Marine Bacterial Lineage. ISME. J. 6, 1186–1199. doi: 10.1038/ismej.2011.189
Eiler A., Hayakawa D. H., Church M. J., Karl D. M., Rappe M. S. (2009). Dynamics of the SAR11 Bacterioplankton Lineage in Relation to Environmental Conditions in the Oligotrophic North Pacific Subtropical Gyre. Environ. Microbiol. 11, 2291–2300. doi: 10.1111/j.1462-2920.2009.01954.x
El-Swais H., Dunn K. A., Bielawski J. P., Li W. K., Walsh D. A. (2015). Seasonal Assemblages and Short-Lived Blooms in Coastal North-West Atlantic Ocean Bacterioplankton. Environ. Microbiol. 17, 3642–3661. doi: 10.1111/1462-2920.12629
Faust K., Lahti L., Gonze D., de Vos W. M., Raes J. (2015). Metagenomics Meets Time Series Analysis: Unraveling Microbial Community Dynamics. Curr. Opin. Microbiol. 25, 56–66. doi: 10.1016/j.mib.2015.04.004
Freitas S., Hatosy S., Fuhrman J. A., Huse S. M., Welch D. B., Sogin M. L., et al. (2012). Global Distribution and Diversity of Marine Verrucomicrobia. ISME. J. 6, 1499–1505. doi: 10.1038/ismej.2012.3
Fuhrman J. A., Cram J. A., Needham D. M. (2015). Marine Microbial Community Dynamics and Their Ecological Interpretation. Nat. Rev. Microbiol. 13, 133–146. doi: 10.1038/nrmicro3417
Galand P. E., Potvin M., Casamayor E. O., Lovejoy C. (2010). Hydrography Shapes Bacterial Biogeography of the Deep Arctic Ocean. ISME. J. 4, 564–576. doi: 10.1038/ismej.2009.134
Garcia F. C., Alonso-Saez L., Moran X. A., Lopez-Urrutia A. (2015). Seasonality in Molecular and Cytometric Diversity of Marine Bacterioplankton: The Re-Shuffling of Bacterial Taxa by Vertical Mixing. Environ. Microbiol. 17, 4133–4142. doi: 10.1111/1462-2920.12984
Gilbert J. A., Steele J. A., Caporaso J. G., Steinbruck L., Reeder J., Temperton B., et al. (2012). Defining Seasonal Marine Microbial Community Dynamics. ISME. J. 6, 298–308. doi: 10.1038/ismej.2011.107
Giovannoni S. J., Tripp H. J., Givan S., Podar M., Vergin K. L., Baptista D., et al. (2005). Genome Streamlining in a Cosmopolitan Oceanic Bacterium. Science 309, 1242–1245. doi: 10.1126/science.1114057
Giovannoni S. J., Vergin K. L. (2012). Seasonality in Ocean Microbial Communities. Science 335, 671–676. doi: 10.1126/science.1198078
Grossart H. P., Levold F., Allgaier M., Simon M., Brinkhoff T. (2005). Marine Diatom Species Harbour Distinct Bacterial Communities. Environ. Microbiol. 7, 860–873. doi: 10.1111/j.1462-2920.2005.00759.x
Grossart H. P., Tang K. W., Kiorboe T., Ploug H. (2007). Comparison of Cell-Specific Activity Between Free-Living and Attached Bacteria Using Isolates and Natural Assemblages. FEMS Microbiol. Lett. 266, 194–200. doi: 10.1111/j.1574-6968.2006.00520.x
Haggerty J. M., Dinsdale E. A. (2017). Distinct Biogeographical Patterns of Marine Bacterial Taxonomy and Functional Genes. Global Ecol. Biogeogr. 26, 177–190. doi: 10.1111/geb.12528
Herlemann D. P. R., Labrenz M., Jurgens K., Bertilsson S., Waniek J. J., Andersson A. F. (2011). Transitions in Bacterial Communities Along the 2000 Km Salinity Gradient of the Baltic Sea. ISME. J. 5, 1571–1579. doi: 10.1038/ismej.2011.41
Jasti S., Sieracki M. E., Poulton N. J., Giewat M. W., Rooney-Varga J. N. (2005). Phylogenetic Diversity and Specificity of Bacteria Closely Associated With Alexandrium Spp. And Other Phytoplankton. Appl. Environ. Microbiol. 71, 3483–3494. doi: 10.1128/AEM.71.7.3483-3494.2005
Kirchman D. (2002). The Ecology of Cytophaga–Flavobacteria in Aquatic Environments. FEMS Microbiol. Ecol. 39, 91–100. doi: 10.1016/S0168-6496(01)00206-9
Klindworth A., Mann A. J., Huang S., Wichels A., Quast C., Waldmann J., et al. (2014). Diversity and Activity of Marine Bacterioplankton During a Diatom Bloom in the North Sea Assessed by Total RNA and Pyrotag Sequencing. Mar. Genomics 18 Pt B, 185–192. doi: 10.1016/j.margen.2014.08.007
Koch H., Lucker S., Albertsen M., Kitzinger K., Herbold C., Spieck E., et al. (2015). Expanded Metabolic Versatility of Ubiquitous Nitrite-Oxidizing Bacteria From the Genus Nitrospira. Proc. Natl. Acad. Sci. U.S.A. 112, 11371–11376. doi: 10.1073/pnas.1506533112
Krabberød A. K., Deutschmann I. M., Bjorbækmo M. F. M., Balagué V., Giner C. R., Ferrera I., et al. (2022). Long-Term Patterns of an Interconnected Core Marine Microbiota. Environmental Microbiome. 17(1), 22. doi: 10.1186/s40793-022-00417-1
Lambert S., Lozano J. C., Bouget F. Y., Galand P. E. (2021). Seasonal Marine Microorganisms Change Neighbours Under Contrasting Environmental Conditions. Environ. Microbiol. 23, 2592–2604. doi: 10.1111/1462-2920.15482
Lambert S., Tragin M., Lozano J. C., Ghiglione J. F., Vaulot D., Bouget F. Y., et al. (2019). Rhythmicity of Coastal Marine Picoeukaryotes, Bacteria and Archaea Despite Irregular Environmental Perturbations. ISME. J. 13, 388–401. doi: 10.1038/s41396-018-0281-z
Landa M., Blain S., Christaki U., Monchy S., Obernosterer I. (2016). Shifts in Bacterial Community Composition Associated With Increased Carbon Cycling in a Mosaic of Phytoplankton Blooms. ISME. J. 10, 39–50. doi: 10.1038/ismej.2015.105
Lima-Mendez G., Faust K., Henry N., Decelle J., Colin S., Carcillo F., et al. (2015). Ocean Plankton. Determinants of Community Structure in the Global Plankton Interactome. Science 348, 1262073. doi: 10.1126/science.1262073
Lupette J., Lami R., Krasovec M., Grimsley N., Moreau H., Piganeau G., et al. (2016). Marinobacter Dominates the Bacterial Community of the Ostreococcus Tauri Phycosphere in Culture. Front. Microbiol. 7. doi: 10.3389/fmicb.2016.01414
Martiny J. B., Bohannan B. J., Brown J. H., Colwell R. K., Fuhrman J. A., Green J. L., et al. (2006). Microbial Biogeography: Putting Microorganisms on the Map. Nat. Rev. Microbiol. 4, 102–112. doi: 10.1038/nrmicro1341
Mestre M., Borrull E., Sala M., Gasol J. M. (2017). Patterns of Bacterial Diversity in the Marine Planktonic Particulate Matter Continuum. ISME. J. 11, 999–1010. doi: 10.1038/ismej.2016.166
Morán X. A. G., Scharek R. (2015). Photosynthetic Parameters and Primary Production, With Focus on Large Phytoplankton, in a Temperate Mid-Shelf Ecosystem. Estuar. Coast. Shelf. Sci. 154, 255–263. doi: 10.1016/j.ecss.2014.12.047
Morris R. M., Longnecker K., Giovannoni S. J. (2006). Pirellula and OM43 are Among the Dominant Lineages Identified in an Oregon Coast Diatom Bloom. Environ. Microbiol. 8, 1361–1370. doi: 10.1111/j.1462-2920.2006.01029.x
Morris R. M., Rappe M. S., Connon S. A., Vergin K. L., Siebold W. A., Carlson C. A., et al. (2002). SAR11 Clade Dominates Ocean Surface Bacterioplankton Communities. Nature 420, 806–810. doi: 10.1038/nature01240
Morris R. M., Vergin K. L., Cho J.-C., Rappé M. S., Carlson C. A., Giovannoni S. J. (2005). Temporal and Spatial Response of Bacterioplankton Lineages to Annual Convective Overturn at the Bermuda Atlantic Time-Series Study Site. Limnol. Oceanogr. 50, 1687–1696. doi: 10.4319/lo.2005.50.5.1687
Needham D. M., Fuhrman J. A. (2016). Pronounced Daily Succession of Phytoplankton, Archaea and Bacteria Following a Spring Bloom. Nat. Microbiol. 1, 16005. doi: 10.1038/nmicrobiol.2016.5
Neveux J., de Billy G. (1986). Spectrofluorometric Determination of Chlorophylls and Pheophytins. Their Distribution in the Western Part of the Indian Ocean (July to August 1979). Deep. Sea. Res. Part A. Oceanogr. Res. Pap. 33, 1–14. doi: 10.1016/0198-0149(86)90104-4
Pinhassi J., Sala M. M., Havskum H., Peters F., Guadayol O., Malits A., et al. (2004). Changes in Bacterioplankton Composition Under Different Phytoplankton Regimens. Appl. Environ. Microbiol. 70, 6753–6766. doi: 10.1128/AEM.70.11.6753-6766.2004
Raes J., Letunic I., Yamada T., Jensen L. J., Bork P. (2011). Toward Molecular Trait-Based Ecology Through Integration of Biogeochemical, Geographical and Metagenomic Data. Mol. Syst. Biol. 7, 473. doi: 10.1038/msb.2011.6
Ramanan R., Kang Z., Kim B. H., Cho D. H., Jin L., Oh H. M, et al. (2015). Phycosphere bacterial diversity in green algae reveals an apparent similarity across habitats. Algal Research 8, 140–144. doi: 10.1016/j.algal.2015.02.003
Reshef D. N., Reshef Y. A., Finucane H. K., Grossman S. R., McVean G., Turnbaugh P. J., et al. (2011). Detecting Novel Associations in Large Data Sets. Science 334, 1518–1524. doi: 10.1126/science.1205438
Riemann L., Steward G. F., Azam F. (2000). Dynamics of Bacterial Community Composition and Activity During a Mesocosm Diatom Bloom. Appl. Environ. Microbiol. 66, 578–587. doi: 10.1128/AEM.66.2.578-587.2000
Rinta-Kanto J. M., Sun S., Sharma S., Kiene R. P., Moran M. A. (2012). Bacterial Community Transcription Patterns During a Marine Phytoplankton Bloom. Environ. Microbiol. 14, 228–239. doi: 10.1111/j.1462-2920.2011.02602.x
Rooney-Varga J. N., Giewat M. W., Savin M. C., Sood S., LeGresley M., Martin J. L. (2005). Links Between Phytoplankton and Bacterial Community Dynamics in a Coastal Marine Environment. Microb. Ecol. 49, 163–175. doi: 10.1007/s00248-003-1057-0
Ruan Q., Dutta D., Schwalbach M. S., Steele J. A., Fuhrman J. A., Sun F. (2006). Local Similarity Analysis Reveals Unique Associations Among Marine Bacterioplankton Species and Environmental Factors. Bioinformatics 22, 2532–2538. doi: 10.1093/bioinformatics/btl417
Sapp M., Schwaderer A. S., Wiltshire K. H., Hoppe H. G., Gerdts G., Wichels A. (2007). Species-Specific Bacterial Communities in the Phycosphere of Microalgae? Microbial. Ecol. 53 (4), 683–699. doi: 10.1007/s00248-006-9162-5
Sarmento H., Romera-Castillo C., Lindh M., Pinhassi J., Sala M. M., Gasol J. M., et al. (2013). Phytoplankton Species-Specific Release of Dissolved Free Amino Acids and Their Selective Consumption by Bacteria. Limnol. Oceanogr. 58, 1123–1135. doi: 10.4319/lo.2013.58.3.1123
Schloss P. D., Westcott S. L., Ryabin T., Hall J. R., Hartmann M., Hollister E. B., et al. (2009). Introducing Mothur: Open-Source, Platform-Independent, Community-Supported Software for Describing and Comparing Microbial Communities. Appl. Environ. Microbiol. 75, 7537–7541. doi: 10.1128/AEM.01541-09
Shannon P., Markiel A., Ozier O., Baliga N. S., Wang J. T., Ramage D., et al. (2003). Cytoscape: A Software Environment for Integrated Models of Biomolecular Interaction Networks. Genome Res. 13, 2498–2504. doi: 10.1101/gr.1239303
Steele J. A., Countway P. D., Xia L., Vigil P. D., Beman J. M., Kim D. Y., et al. (2011). Marine Bacterial, Archaeal and Protistan Association Networks Reveal Ecological Linkages. ISME. J. 5, 1414–1425. doi: 10.1038/ismej.2011.24
Storey J. D., Tibshirani R. (2003). Statistical Significance for Genomewide Studies. Proc. Natl. Acad. Sci. U.S.A. 100, 9440–9445. doi: 10.1073/pnas.1530509100
Sunagawa S., Coelho L. P., Chaffron S., Kultima J. R., Labadie K., Salazar G., et al. (2015). Ocean Plankton. Structure and Function of the Global Ocean Microbiome. Science 348, 1261359. doi: 10.1126/science.1261359
Tada Y., Suzuki K. (2016). Changes in the Community Structure of Free-Living Heterotrophic Bacteria in the Open Tropical Pacific Ocean in Response to Microalgal Lysate-Derived Dissolved Organic Matter. FEMS Microbiol. Ecol. 92(7), fiw099. doi: 10.1093/femsec/fiw099
Tada Y., Taniguchi A., Nagao I., Miki T., Uematsu M., Tsuda A., et al. (2011). Differing Growth Responses of Major Phylogenetic Groups of Marine Bacteria to Natural Phytoplankton Blooms in the Western North Pacific Ocean. Appl. Environ. Microbiol. 77, 4055–4065. doi: 10.1128/AEM.02952-10
Teeling H., Fuchs B. M., Becher D., Klockow C., Gardebrecht A., Bennke C. M., et al. (2012). Substrate-Controlled Succession of Marine Bacterioplankton Populations Induced by a Phytoplankton Bloom. Science 336, 608–611. doi: 10.1126/science.1218344
Teeling H., Fuchs B. M., Bennke C. M., Kruger K., Chafee M., Kappelmann L., et al. (2016). Recurring Patterns in Bacterioplankton Dynamics During Coastal Spring Algae Blooms. Elife 5, e11888. doi: 10.7554/eLife.11888
Tripp H. J., Kitner J. B., Schwalbach M. S., Dacey J. W., Wilhelm L. J., Giovannoni S. J. (2008). SAR11 Marine Bacteria Require Exogenous Reduced Sulphur for Growth. Nature 452, 741–744. doi: 10.1038/nature06776
Utermöhl H. (1958). Zur Vervollkomnung Der Quantitativen Phytoplankton-Methodik. Mitt. Int. Ver. Theor. Angew. Limnol. 9, 38.
Venter J. C., Remington K., Heidelberg J. F., Halpern A. L., Rusch D., Eisen J. A., et al. (2004). Environmental Genome Shotgun Sequencing of the Sargasso Sea. Science 304, 66–74. doi: 10.1126/science.1093857
Vergin K. L., Beszteri B., Monier A., Thrash J. C., Temperton B., Treusch A. H., et al. (2013). High-Resolution SAR11 Ecotype Dynamics at the Bermuda Atlantic Time-Series Study Site by Phylogenetic Placement of Pyrosequences. ISME. J. 7, 1322–1332. doi: 10.1038/ismej.2013.32
Whitman W. B., Coleman D. C., Wiebe W. J. (1998). Prokaryotes: The Unseen Majority. Proc. Natl. Acad. Sci. 95, 6578–6583. doi: 10.1073/pnas.95.12.6578
Williams T. J., Wilkins D., Long E., Evans F., DeMaere M. Z., Raftery M. J., et al. (2013). The Role of Planktonic Flavobacteria in Processing Algal Organic Matter in Coastal East Antarctica Revealed Using Metagenomics and Metaproteomics. Environ. Microbiol. 15, 1302–1317. doi: 10.1111/1462-2920.12017
Worden A. Z., Follows M. J., Giovannoni S. J., Wilken S., Zimmerman A. E., Keeling P. J. (2015). Environmental Science. Rethinking the Marine Carbon Cycle: Factoring in the Multifarious Lifestyles of Microbes. Science 347, 1257594. doi: 10.1126/science.1257594
Zecher K., Hayes K. R., Philipp B. (2020). Evidence of Interdomain Ammonium Cross-Feeding From Methylamine- and Glycine Betaine-Degrading Rhodobacteraceae to Diatoms as a Widespread Interaction in the Marine Phycosphere. Front. Microbiol. 11, 533894. doi: 10.3389/fmicb.2020.533894
Keywords: bacteria, phytoplankton, interactions, network analysis, DNA sequencing
Citation: Arandia-Gorostidi N, Krabberød AK, Logares R, Deutschmann IM, Scharek R, Morán XAG, González F and Alonso-Sáez L (2022) Novel Interactions Between Phytoplankton and Bacteria Shape Microbial Seasonal Dynamics in Coastal Ocean Waters. Front. Mar. Sci. 9:901201. doi: 10.3389/fmars.2022.901201
Received: 21 March 2022; Accepted: 25 May 2022;
Published: 22 July 2022.
Edited by:
Jin Zhou, Tsinghua University, ChinaReviewed by:
Cong Fei, New York University Abu Dhabi, United Arab EmiratesShu-Feng Zhang, Xiamen University, China
Copyright © 2022 Arandia-Gorostidi, Krabberød, Logares, Deutschmann, Scharek, Morán, González and Alonso-Sáez. This is an open-access article distributed under the terms of the Creative Commons Attribution License (CC BY). The use, distribution or reproduction in other forums is permitted, provided the original author(s) and the copyright owner(s) are credited and that the original publication in this journal is cited, in accordance with accepted academic practice. No use, distribution or reproduction is permitted which does not comply with these terms.
*Correspondence: Nestor Arandia-Gorostidi, bi5hcmFuZGlhODZAZ21haWwuY29t; Laura Alonso-Sáez, bGFsb25zb0BhenRpLmVz