- 1Departamento de Biologia Marina, Centre for Ecology and Sustainable Management of Oceanic Islands (ESMOI), Facultad Ciencias del Mar, Universidad Católica del Norte, Coquimbo, Chile
- 2Programa de Pós-Graduação em Geoquímica: Petróleo e Meio Ambiente (POSPETRO), Institute of Geosciences, Federal University of Bahia (UFBA), Salvador, Brazil
- 3Centro de Estudios Avanzados en Zonas Aridas (CEAZA), Coquimbo, Chile
- 4Departamento de Biología, Universidad de La Serena, La Serena, Chile
Seabirds are top predators in coastal and pelagic ecosystems that forage at sea but return to land regularly during the breeding season (i.e., central place foragers). This unique life history strategy is directly related to their biological traits and helps define their role as top predators in marine systems. We analysed the effects of physiographic characteristics of nesting islands (area, elevation, and distance from the continent, as predicted by the General Dynamic Model of Oceanic Island Biogeography) and oceanographic variables from waters surrounding nesting islands that tend to be characterised by steep gradients (temperature, salinity, and primary productivity) on the trait diversity of nesting seabird assemblages on islands of the south-eastern Pacific Ocean. Four biological traits related to species’ life history and feeding strategy were used to calculate two indices, the Functional Richness and Rao’s Quadratic entropy. We used fourth-corner and RLQ analysis to determine the relationship between biological traits and environmental variables. Island physiography and primary productivity in the waters surrounding nesting islands significantly affected seabird trait diversity, which gradually decreased from Chilean coastal islands to the distant Polynesian Islands. The traits for body mass and clutch size showed a significant positive relationship with primary productivity. We identified three assemblages of seabirds that had contrasting trait structures. These were defined as the Galapagos, Coastal Chile, and south-eastern Oceanic islands assemblages, and reflected the adaptations of three different species pools to specific oceanographic conditions. Our results suggest that food-related constraints might be one of the most critical environmental filters that shaped the current trait structure of nesting seabird assemblages on the islands in the eastern South Pacific Ocean.
Introduction
Seabirds represent a global group of vertebrates that are relevant top predators in marine food webs (Schreiber and Burger, 2002). Their distribution ranges from tropical to polar systems, and they occur in estuarine, coastal, and open oceanic waters (González-Solís and Shaffer, 2009; Jungblut et al., 2017). During the breeding season, seabirds regularly move between their reproductive habitat, mainly located on islands, to their foraging habitat at sea. This transboundary movement makes them essential drivers of nutrient cycling, transferring nutrients from their marine feeding grounds to the various habitats located on the islands on which they roost and breed. The islands on which seabirds breed (i.e., the distribution of breeding seabirds) are highly constrained by the availability of quality habitat to support nest sites and, for most seabirds, colonies. Habitat quality for breeding seabirds is often related to local factors such as the presence of terrestrial predators, distance from foraging grounds, and availability of suitable nest sites (Schreiber and Burger, 2002). Other factors, such as competition both for food and nest sites, also play a role in determining the distribution of breeding seabirds (Furness and Monaghan, 1987; Schreiber and Burger, 2002).
The role of seabirds as predators in marine ecosystems is directly related to biological traits that reflect life history and feeding strategies (Keith et al., 2001; Shealer, 2001; Schreiber and Burger, 2002). Body mass is an essential component of the avian condition, reflecting the inherent energy reserve available to survive and breed (Bennett and Owens, 2002). Differences in body size (i.e., structural size) among seabirds may result in differences in prey type or prey size, foraging behavior, and distance traveled during foraging (Quillfeldt et al., 2011; Cook et al., 2013). Similarly, it has been proposed that body mass and clutch size primarily reflect adaptations related to energy allocation, in which geographic regions with low food supply would favor small body size and small clutches given the energetic demands of a large offspring (Bennett and Owens, 2002; McNab, 2003). Furthermore, regions with low levels of food resources often support seabird assemblages that are comprised of species with smaller body masses (Ashmole and Ashmole, 1967; McNab, 2003). Exceptions have been observed, however, in high productivity areas of the Atlantic (e.g., São Pedro and São Paulo Archipelago, Mancini and Bugoni, 2014) and Pacific oceans (e.g., coastal islands of north central Chile, Luna-Jorquera et al., 2012), in which seabird assemblages are comprised of species with body mass ranging from a few grams to several kilograms.
The diversity of species traits is an essential driver of ecosystem functioning and responses in terrestrial and aquatic environments (Petchey and Gaston, 2006). Accordingly, an increasing number of studies that examine trait diversity have applied analytical approaches to assess the structure of species assemblages (Jiguet et al., 2007; Ding et al., 2013; Luck et al., 2013; Rayner et al., 2014). For example, the relationship between traits of a species and environmental characteristics is expected to shape communities (Mouillot et al., 2013; Gravel et al., 2016). Furthermore, studies have highlighted the role of trait diversity in modulating ecosystem functioning (Cerrano et al., 2009; Brose and Hillebrand, 2016). Thus, trait-based approaches have been applied to different marine and terrestrial systems to analyze community-level responses to environmental factors and disturbances (Levin et al., 2001; Boyer and Jetz, 2010; Mouillot et al., 2013; Dehling et al., 2014; Morais et al., 2019).
Factors that influence the distribution of seabirds can be considered within both their foraging habitat (i.e., at sea) and the breeding habitat (i.e., on land). In the south-eastern Pacific Ocean, seabirds form assemblages at sea and display biogeographic patterns at different temporal and spatial scales in response to the oceanographic features of the areas where they typically forage (Weichler et al., 2004; Serratosa et al., 2020). In contrast to studies such as these that seek to explain the drivers of seabird assemblages at sea, the factors explaining the diversity patterns of seabirds nesting on islands have seldom been studied. In oceanic islands, local species diversity is unlikely to be at equilibrium, so the classic theory of island biogeography (i.e., island area and isolation; MacArthur and Wilson, 1963; MacArthur and Wilson, 1967) may not be sufficient to explain the observed patterns. Instead, the General Dynamic Model of Oceanic Island Biogeography, GDM (Whittaker et al., 2008; Borregaard et al., 2017) provides a broader conceptual framework for studying diversity in oceanic islands. For example, Gusmao et al. (2020) may be the only study that examines the influence of island physiography (as predicted by the GDM) and oceanographic factors on the spatial variation in species richness and diversity of nesting seabird assemblages in south-eastern Pacific islands, an area that supports an extensive community of nesting seabirds. That study reported that island physiography and oceanographic factors strongly explain changes in species richness and composition across the south-eastern Pacific (Gusmao et al., 2020). However, it is still unclear whether the diversity of biological traits of nesting seabird assemblages would change across islands located in a wide spatial gradient that covers from Galapagos to southern Chile and from the Chilean coast to Pitcairn. Considering the ecological role that seabirds play in insular ecosystems and their current conservation threats, it is essential to identify patterns of biological trait diversity to understand the role of environmental factors in structuring seabird nesting assemblages in coastal and oceanic islands.
We use a trait-based approach to analyze seabird assemblages across islands of the south-eastern Pacific to elucidate how environmental factors may shape the nesting assemblages in such insular systems. Based on the GDM, we hypothesize that if the availability of nesting habitats within an island is a key driver of the trait structure of seabird assemblages, then physiographic characteristics of islands such as area and elevation would be the main predictors of seabird trait diversity. In contrast, if food availability is the main driver of the trait structure of seabird assemblages, then oceanographic variables that influence food availability (i.e., salinity, temperature, and primary productivity) would be the main predictors of seabird trait diversity. We also posited that if environmental gradients across biogeographical realms shaped seabird trait diversity, then the most notable differences in seabird trait diversity would be associated with the largest spatial scales.
Methods
Study area
We compiled information on the occurrence (i.e., presence or absence) of 53 species (Data sheet 1) of seabird nesting on 41 coastal and oceanic islands (Data sheet 2) of contrasting climate and geological history (Spalding et al., 2007; Costello et al., 2017). Although we were primarily interested in the south-eastern Pacific, we also included data from the Galápagos to increase the data set’s latitudinal gradient. The final dataset accounted for six main archipelagos (Data sheet 2): Juan Fernández, Desventuradas, Rapa Nui, Pitcairn, Galápagos, and Chilean coastal islands, which range from ~23°S to ~38°S (Figure 1).
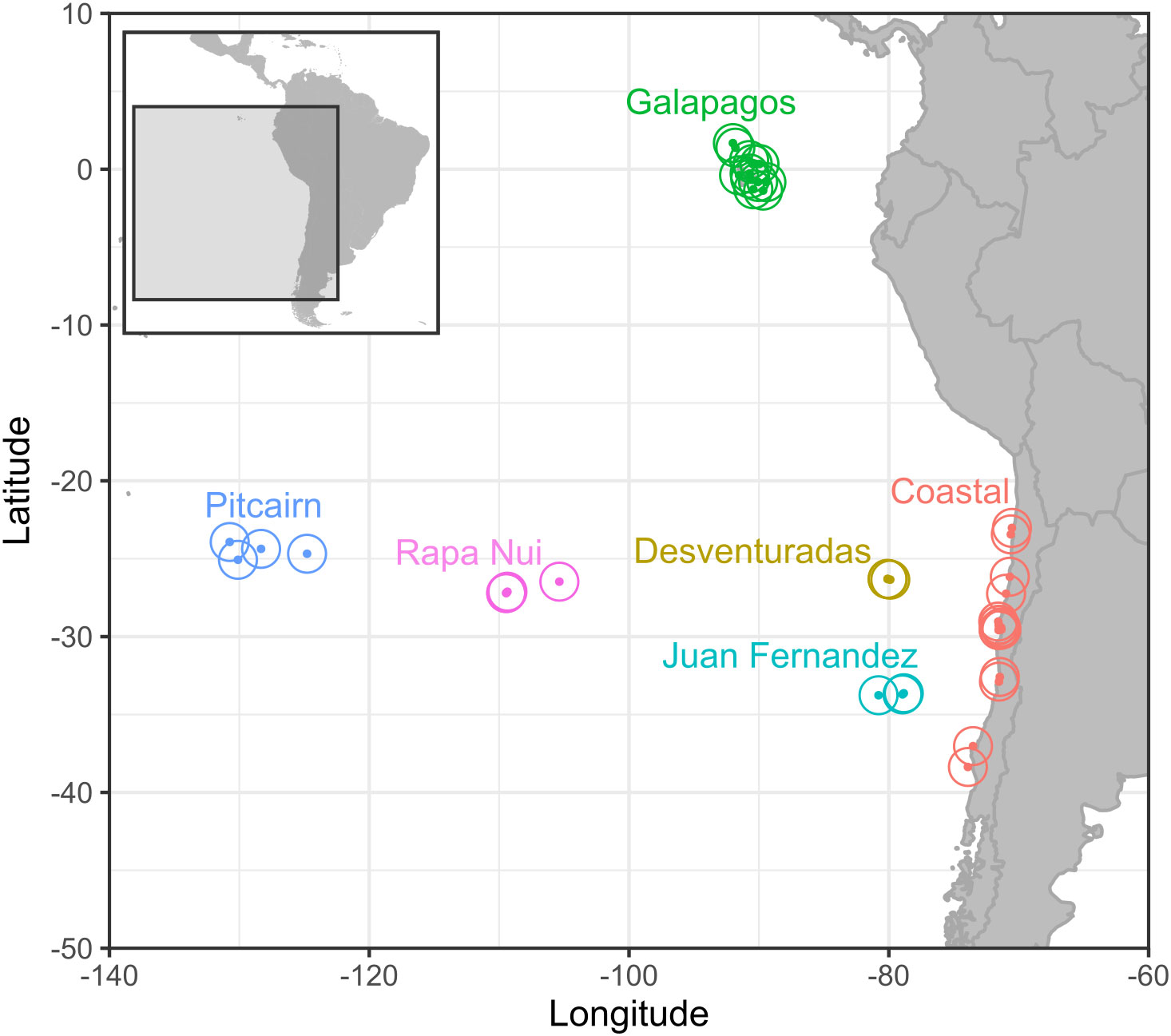
Figure 1 Location of the oceanic and coastal islands considered in the study. Islands are represented with a dot and a surrounding circle. The latter does not represent the 50 km buffer to obtain the oceanographic data.
We compiled information about the oceanographic features around each island by sampling raster maps from the Bio-ORACLE online database (Tyberghein et al., 2012; Assis et al., 2018) (Data sheet 2). Each Bio-ORACLE global-scale raster map (resolution of 5 arcmins; ~9.2 km at latitude zero) was sampled considering a 50 km buffer around the center of each island using tools of the R packages raster (Hijmans, 2021) and geosphere (Hijmans, 2019). After excluding highly collinear variables (Pearson’s correlation > |0.8|), six environmental variables were considered in our analyses (Data sheet 2): island area (km2), elevation (m), sea surface temperature (°C), sea surface salinity (SSS), primary productivity (g m-3 day-1), and distance to the mainland (km).
Seabird assemblages
We gathered information on nesting seabird occurrences on each island. This information was extracted from the literature and unpublished reports (Data sheet 1). For most Chilean islands, the information on seabirds is from our field records collected intermittently for 20 years (1999-2019) for the coastal islands and five years (2013-2018) for the oceanic islands.
We also compiled information on four biological traits for each seabird species (Data sheet 3): (1) feeding stratum within the water column, defined as below the water surf zone, around the water surf zone, and on the ground; (2) diet based on predominant prey types defined as feeding on invertebrates, vertebrate endotherms [mammals and birds], vertebrate ectotherms [reptiles and amphibians], fishes, scavenger, and unknown; (3) clutch size, average for the species, and (4) body mass in grams, average for the species. These traits were selected since they are related to species’ life history and foraging ecology (Boyer and Jetz, 2010; Ding et al., 2013; Luck et al., 2013). Data on seabird feeding stratum and diet were extracted from Wilman et al. (2014), who provided the information on feeding stratum and diet as percentages of multiple trait subcategories (e.g., % diet comprised of fish, % foraging below the water surf zone, respectively). Thus, we used principal components analyses to reduce the full set of categories (three for feeding stratum and six for diet) to fewer vectors (i.e., PCA axes) that, in all cases, explained more than 90% of the variation for each trait (see Supplementary Material Table S1). Hence, we accounted for the information on feeding stratum and diet in our trait-based analysis without unnecessarily increasing the number of dimensions in our trait space. The final trait matrix with four columns was then standardized by centering and scaling the trait values. Information about body mass and clutch size was compiled from the literature and online databases; complete table of species traits and cited references are included in the Data sheet 3.
Trait diversity
We selected two trait-based metrics to estimate seabird trait diversity, Functional Richness (FRic, Laliberté and Legendre, 2010) and Rao’s Quadratic entropy (Rao’s Q; Botta-Dukát, 2005; Ricotta and Szeidl, 2009). These indices were chosen because they reflect different aspects of the multivariate trait space formed by the trait values of all seabird species. Both metrics are distance-based multivariate indices that can be calculated using species’ occurrences and trait scores. FRic measures the convex hull hypervolume of the trait space, reflecting the general range of the trait values within a community (Mason et al., 2005; Laliberté and Legendre, 2010). Thus, increasing the range of trait values within a community would increase FRic. This index tends to be highly influenced by species richness and is very sensitive to species with particularly unique trait values (Mason et al., 2013). Rao’s Q accounts for the general differences in traits among all species observed in each assemblage. Thus, the more the species in an assemblage differ in trait values (in this case, estimated as the Euclidean distances comparing all species regarding their traits), the higher the Rao’s Q value. Although Rao’s Q is relatively independent of species richness when the number of species is high, its values can vary widely when calculated for species-poor communities (Mouchet et al., 2010; de Bello et al., 2016). Although we only considered species occurrences, Rao’s Q can also weight species abundances in the calculations. We did not use other distance-based functional diversity indices, such as Functional Evenness (FEve) and Functional Divergence (FDiv), due to their dependence on abundance data (Laliberté and Legendre, 2010), which are not available for all the species in our dataset. Both FRic and Rao’s Q were calculated using the R package FD (Laliberté and Legendre, 2010; Laliberté and Shipley, 2011).
Data analysis
The relationship between trait diversity and environmental variables was analyzed by fitting linear models. Since the empirical error distributions were similar to the theoretical normal probability distribution (visual inspection of Q-Q plots), models were fit considering a Gaussian error structure. Two types of environmental predictors were considered in the models, (i) oceanographic: salinity, primary productivity, and sea surface temperature, and (ii) island feature: area, elevation, and distance to the continent. The island elevation and the distance from the continent were square root-transformed, and the island area was transformed as log10. All covariates were scaled and centered before fitting the models. Models were validated via likelihood ratio tests comparing the fitted model to a null model with no predictors but the intercept. Additionally, we tested whether the model residuals were spatially autocorrelated by applying the Moran’s I test implemented in the R package ape (Paradis et al., 2004).
We used fourth-corner analysis (Legendre et al., 1997; Dray and Legendre, 2008; Peres-Neto et al., 2017) and RLQ analysis (Dolédec et al., 1996) to assess the relationship between seabird traits and island characteristics. These trait-based analyses have been applied to different biological models, including marine worms (Wouters et al., 2018), insects (Luiza-Andrade et al., 2017), fishes (Keck et al., 2014), and birds (Barbaro and Van Halder, 2009; Azeria et al., 2011; Hartel et al., 2014). The fourth-corner analysis assesses how assemblage trait structure is related to environmental gradients based on the information of species occurrences, environmental variables, and species’ traits (Peres-Neto et al., 2017). This analysis was performed using the function fourthcorner() in the R package ade4 (Dray and Dufour, 2007), considering Chessel’s correlations (Peres-Neto et al., 2017), 9999 permutations on sites and species (permutation methods 2 and 4, respectively), and Holm’s p-value corrections. We used RLQ-analysis to relate seabird traits to environmental factors (i.e., oceanographic factors and island physiography), considering species occurrence nesting on islands. The RLQ procedure performs a double inertia analysis of an environmental-variables-by-islands (R-table) and a species-by-traits (Q-table) matrix, with a link expressed by a species-nesting-by-islands matrix (L-table). RLQ-analysis combines three unconstrained separate ordinations, correspondence analysis of L-table and centered normed principal component analyses of Q- and R- tables, to maximize the covariance between environmental factors and trait data by using co-inertia analysis (Bernhardt-Römermann et al., 2008). First, a correspondence analysis (CA) was performed on the L-occurrence matrix, in which axis scores were used as row weights of a principal components analysis (PCA) of the R-environment matrix. Then, a principal components analysis (PCA) was performed on the Q-trait matrix using the CA site scores as column weights. These steps resulted in a constrained ordination depicting the variation patterns in trait composition across islands, with vectors indicating how environmental variables relate to each ordination axis. RLQ analysis was performed using the R package ade4 (Dray and Dufour, 2007).
All analyses were performed using R 4.1 (R Core Team, 2017), and all graphs were constructed using the R package ggplot2 (Wickham, 2009).
Results
Seabird trait diversity, expressed by FRic and Rao’s Q, varied markedly across archipelagos and islands (Figure 2). This variation was not dependent on the species richness for Rao’s Q (p > 0.05, Figure 2A) but was confirmed for FRic (Figure 2B). The highest trait diversity levels were observed on the Chilean coastal islands (median: Rao’s Q = 3.26, FRic 3.34) and Galapagos islands (Rao’s Q = 2.55, FRic = 4.05), followed by Desventuradas (Rao’s Q = 2.58, FRic = 3.18), Rapa Nui (Rao’s Q = 1.92, FRic = 2.89), Pitcairn (Rao’s Q = 2.32, FRic = 3.42), and Juán Fernández (Rao’s Q = 0.7, FRic = 0.36; Figures 2C, D).
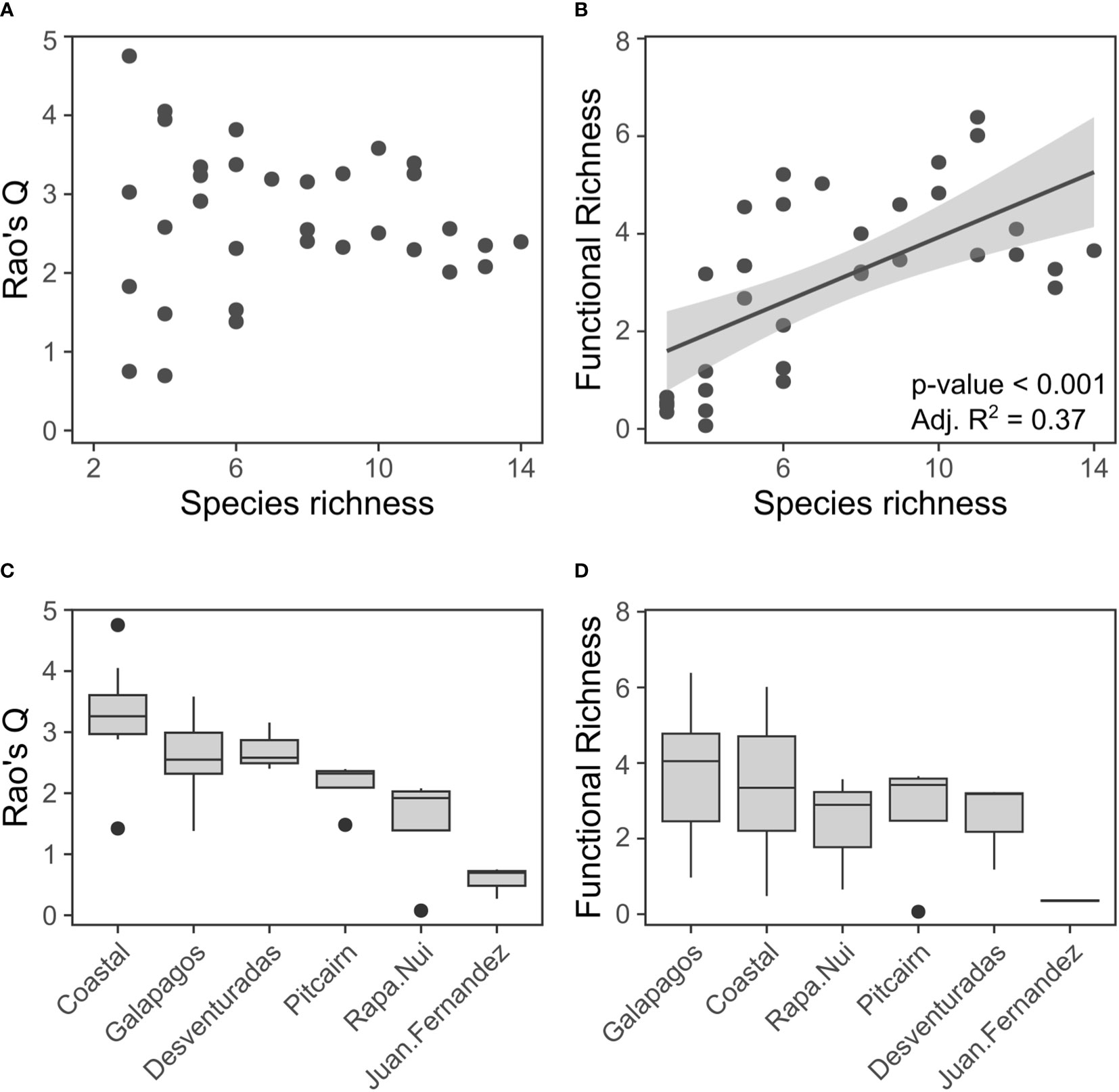
Figure 2 Changes in trait diversity of seabird assemblages across islands of the southeastern Pacific. Relationship between species richness and (A) Rao’s Q, and (B) FRic. The linear fit (black line), its associated 95% CI (gray area), and respective-value and adjusted R2 are shown for the significant fit for the FRic model. Boxplots depicting changes in trait diversity across archipelagos for (A) Rao’s Q, and (B) FRic. Midlines represent medians, boxes represent the interquartile range between quartiles 1 and 3, whiskers represent minimum and maximum non-outlier values, and dots represent outliers.
The linear model results indicated that environmental predictors explained 50% of total variation in Rao’s Q. Rao’s Q was negatively related to island elevation and positively related to primary productivity in surrounding waters (Table 1). FRic was positively related to island area and negatively related to island elevation. We detected no evidence of significant spatial autocorrelation on the residuals of the fitted models (Moran’s I test, p>0.05). See Supplementary Material Figure S1 for individual raw relationships between each environmental variable and diversity index.
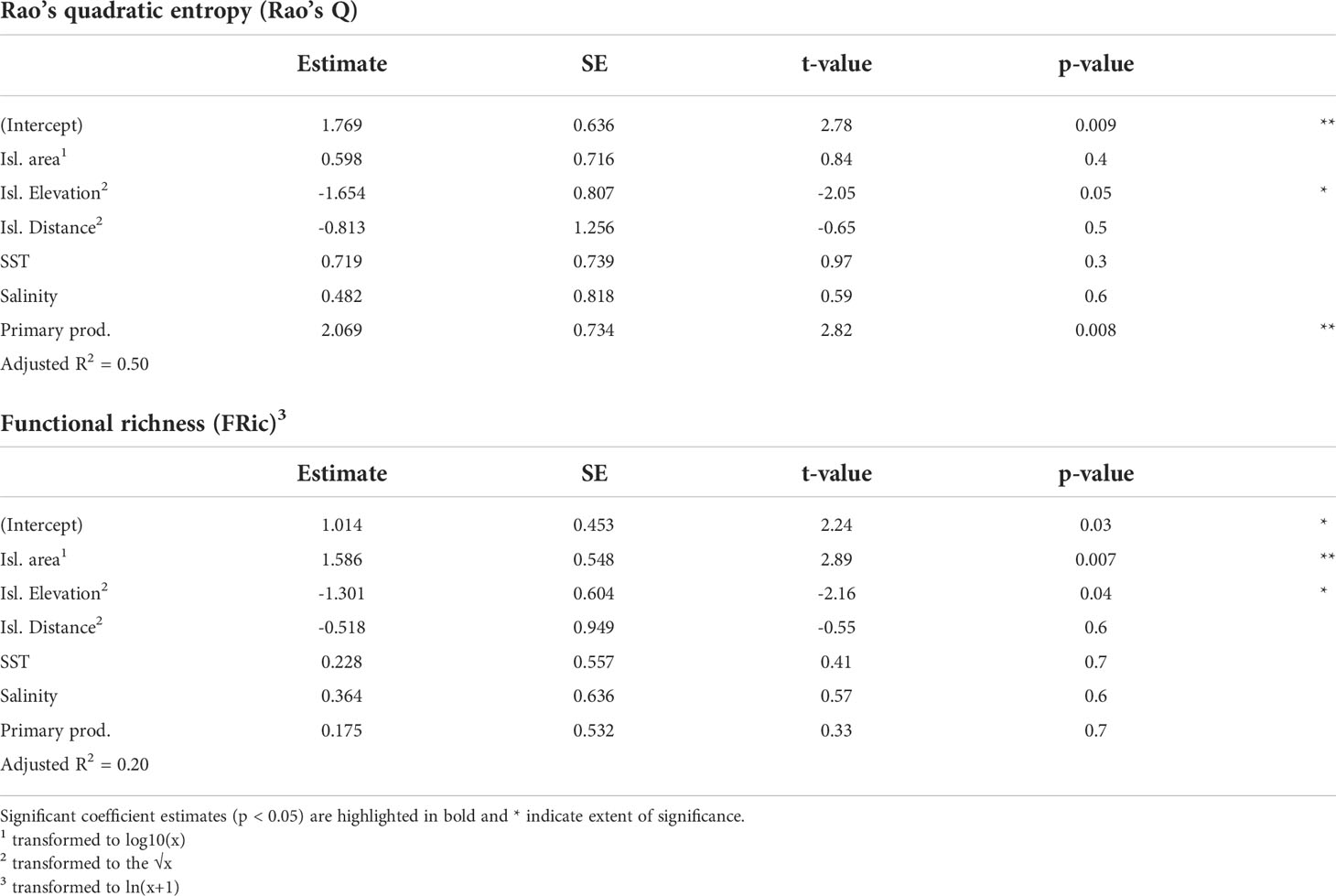
Table 1 Results of the linear models testing the effects of environmental variables on seabird trait diversity (Rao’s Q and FRic indices).
The results of the ordinations of the traits diet and feeding stratum are shown in Table S1 (Supplementary Material). For diet, PC1, which explained 90% of the total variation, described a gradient from a fish-based diet to an invertebrate-based diet. For the feeding stratum, PC1, which explained 95% of the total variation, described a gradient from the feeding stratum around the surf zone to the feeding stratum below the surf zone.
The RLQ analysis and the fourth-corner analysis indicated significant effects of environmental variables on the trait structures we assessed (Figures 3, 4). The RLQ axes explained 70% (Axis 1 = 60%, Axis 2 = 19%) of the correlation between environmental variables (matrix R) and species traits (matrix Q). Most of the variance in environmental gradients (>77%) and biological traits (>94%) was explained by the first two axes. The traits that contributed to most inertia were clutch size (45%) and body mass (25.8%); for environmental variables, primary productivity (37.2%), SST (22.9%), and island distance to the continent (21.9%) contributed to most inertia (Table 2). Gradients in environmental variables along the first axis were mostly related to increases in SST, salinity, island distance, and decreases in primary productivity (Figure 3C). All traits but diet were negatively correlated to the first axis (Figure 3D). Environmental gradients along the second axis were mostly related to increases in salinity and decreases in SST (Figure 3C). Two traits, clutch size, and diet were positively correlated to the second axis (Figure 3D).
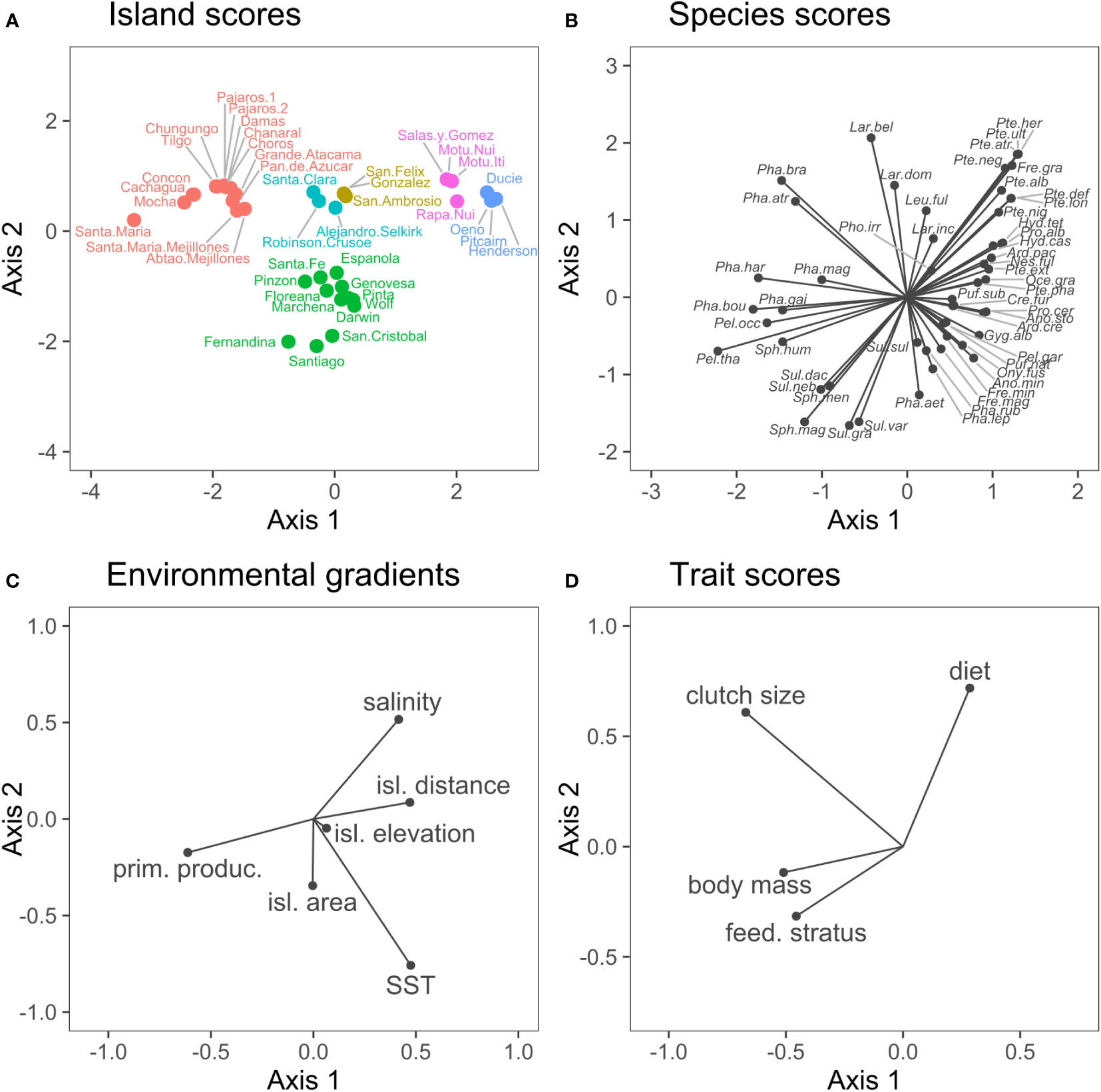
Figure 3 RLQ analysis showing the scores obtained after correspondence and principal component analysis (see Data analysis for details) for (A) islands, (B) species, (C) environmental variables, and (D) traits. Species abbreviations are defined in Data sheet 1.
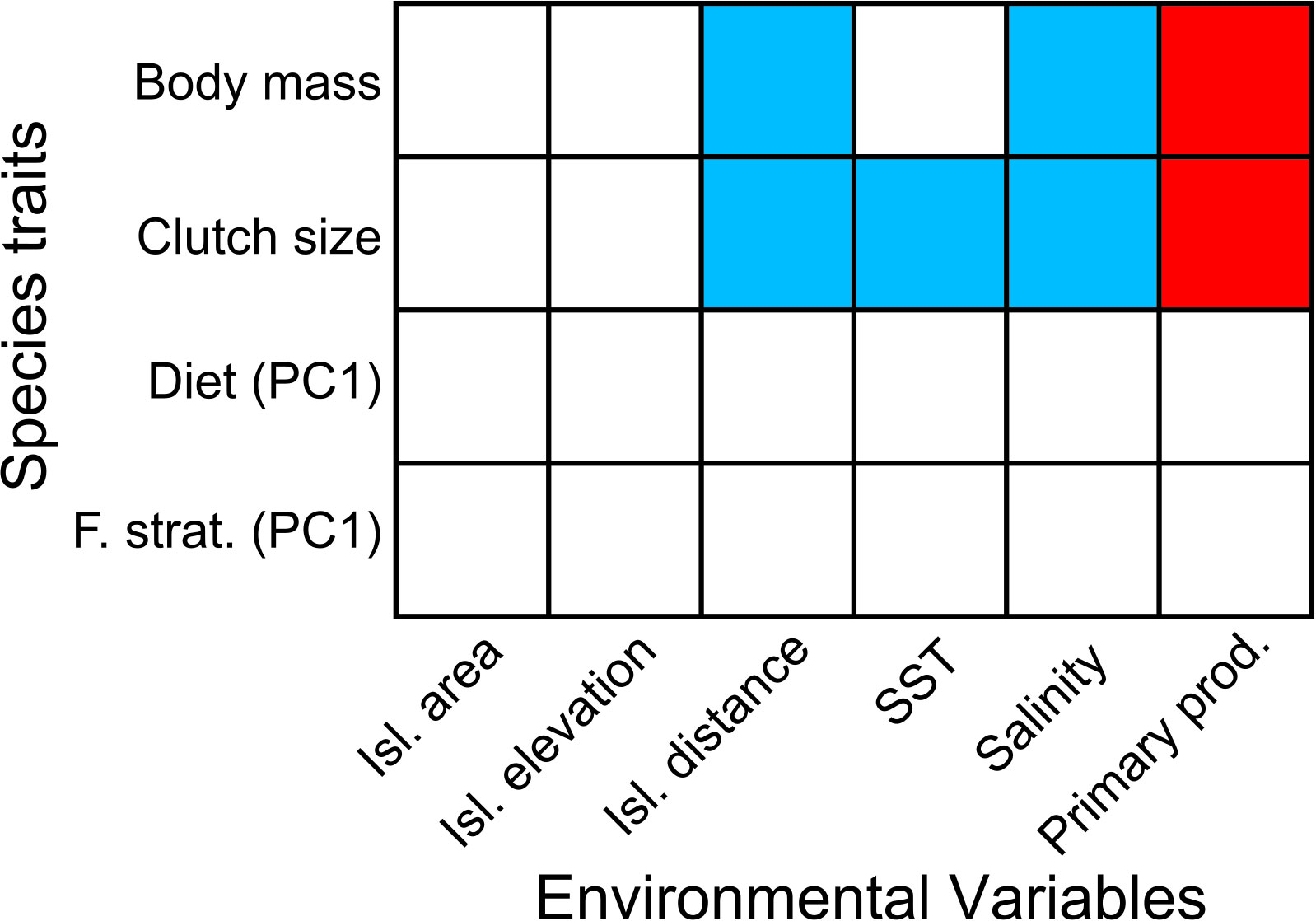
Figure 4 Results of the fourth-corner statistics analysis depicting the significant (p < 0.05) correlation between seabird traits (rows) and environmental variables (columns). Cell colors indicates if the correlations were positive (red) or negative (blue). Isl. = islands; SST = Sea surface temperature; prod. = productivity; F. strat. = Feeding stratum; PC1 = a vector after principal component analysis (see 2.2. for details and Supplementary Material Table S2).
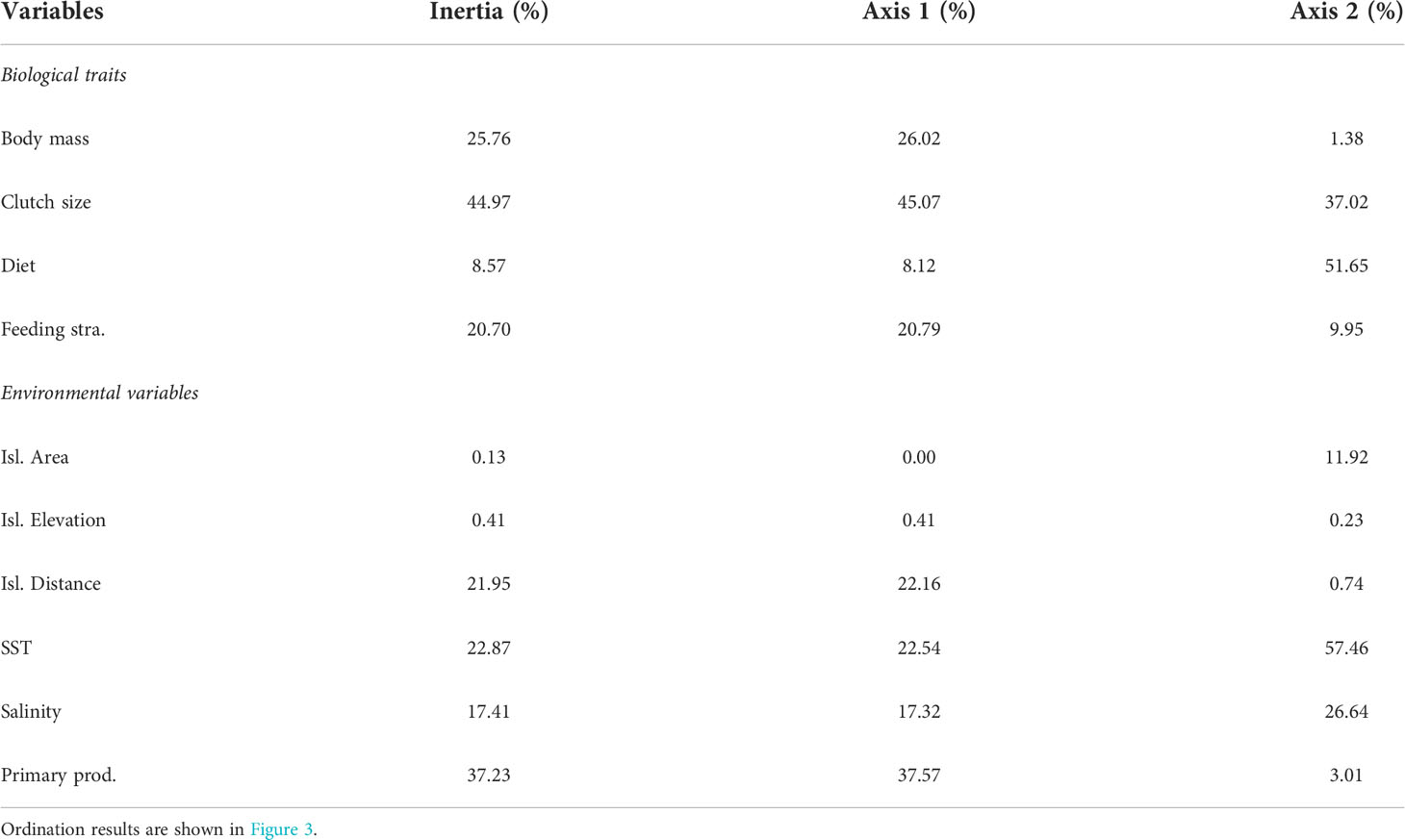
Table 2 Contributions (%) of individual traits and environmental variables to the total inertia to both RLQ axes.
The RLQ ordination (Figure 3) depicted four main clusters of islands with contrasting seabird trait structures: (1) Pitcairn and Rapa Nui archipelagos, restricted to quadrant I; (2) Coastal Chilean islands, concentrated in quadrant II; (2) Galapagos archipelago, restricted to quadrants III and IV; and (4) Desventuradas and Juan Fernández archipelagos, restricted to the center of the ordination. The distant Pitcairn and Rapa Nui archipelagos were characterized predominantly by seabirds with a diet based in invertebrates and with small body sizes. The cluster of Coastal Chilean islands was characterized by predominantly piscivorous seabirds with larger clutch size and body mass. Galapagos’ seabird assemblages were characterized by small clutch size, fish-based diet, and feeding in the deep water stratum. Desventuradas and Juan Fernández were located at the center of the ordination, depicting an assemblage structure intermediary to the other island clusters.
The results of the fourth-corner analysis (Supplementary Material Table S2) were similar to the RLQ analysis (Figure 4). Island distance, salinity, and primary productivity were significantly correlated to body mass and clutch size (p<0.01; Figure 4). Clutch size was negatively related to SST, salinity, and island distance, and positively related to primary productivity. The latter result was mainly forced by the Galápagos archipelago, whose SST is on average ~9.1°C higher than the SST of the Humboldt Current System, where the Chilean coastal islands are located. Finally, body mass was positively correlated with primary productivity but negatively with island distance and salinity. This reflects changes in community structure along the longitudinal gradient, separating the distant Polynesian islands from the other archipelagos near the South American continent (Figure 3).
Discussion
Our results show that increases in trait diversity of nesting seabird assemblages in the south-eastern Pacific are explained by increases in island area, island elevation, and distances from nesting islands to the South American continent, results that are consistent with the predictions of the GDM (Whittaker et al., 2008; Borregaard et al., 2017). Our results are also consistent with a recent study demonstrating that physiographic factors explain the species diversity of seabird assemblages nesting on islands of the south-eastern Pacific (Gusmao et al., 2020). That study found that large islands contain a high number of nesting species, reflecting a positive species-area relationship that allows for the establishment of colonies of different species. Thus, the GDM is a useful conceptual model for understanding the influence of island physiography in determining seabird trait diversity; however, oceanographic factors also play a major role shaping the seabird assemblages on oceanic and coastal islands. Indeed, we found that trait diversity among the seabird assemblages on islands was explained by the primary productivity of the waters surrounding the island. Changes in the composition of seabird assemblages observed across archipelagos reflected differences in species traits, indicating that seabird assemblages with contrasting trait structures characterize different zones of the south-eastern Pacific. In general, the greater the distance from the South American continent, the higher the predominance of an invertebrate-based diet and the smaller the body mass and clutch size. Of all the variables we assessed, primary productivity was significantly correlated with all traits but feeding strategy, suggesting that food-related constraints might be one of the most critical environmental filters that shaped the current trait structure of seabird assemblages in the south-eastern Pacific.
No significant relationship was detected between species richness and Rao’s Q, indicating that assemblages with high species richness do not necessarily present increased trait diversity (Rao’s Q index is independent of species richness; Botta-Dukát, 2005; Schleuter et al., 2010). According to the information analyzed in this study, the island elevation and the island area, variables related to local spatial scales, significantly affect trait diversity. This is consistent with theoretical models predicting that large islands with high elevations would provide a wider range of habitat types and increased topographic complexity, thus providing a range of habitats for different seabird species (Roth, 1976; Lomolino and Weiser, 2001; González-Megías et al., 2007). The significant effect of primary productivity in waters surrounding nesting islands on Rao’s Q suggests that variables related to large spatial scales also influence changes in seabird trait diversity. Differences in trait diversity across large spatial scales were observed in the Pacific for other taxonomic groups (e.g., fishes and corals; Yeager et al., 2017). Variations in trait diversity across large spatial scales are explained mainly by variables with steep environmental gradients, such as sea surface temperature and productivity (Fisher et al., 2010; Barton et al., 2013; Stuart-Smith et al., 2013). Our results suggest that the variation in trait diversity across large spatial scales is related to the environmental differences observed between Chilean coastal islands and distant oceanic islands such as Pitcairn and Rapa Nui archipelagos. Chilean coastal islands are distributed along the Humboldt Current, one of the most productive upwelling systems in the world (Penven et al., 2005; Thiel et al., 2007), while Pitcairn and Rapa Nui archipelagos are located in the oligotrophic waters of the center of the South Pacific gyre (Loret and Tanacredi, 2003). This contrast in environmental conditions indicates that productivity may be a significant environmental filter for seabirds nesting on islands of the south-eastern Pacific Ocean.
Lack (1947) proposed that food availability and quality represent a major energetic constraint for avian clutch size. Lack suggested that low food availability would favor individuals with small clutch sizes given the low energetic demand to raise a small brood. Although this hypothesis was initially proposed to explain latitudinal changes in clutch size, it could also apply to gradients in food availability, such as those that occur across oligotrophic and eutrophic marine systems. Thus, the food gradient observed from ultra-oligotrophic waters of the center of the south-eastern Pacific gyre to the eutrophic waters of the Humboldt current may modulate the observed changes in clutch size among the seabirds in the assemblages we studied. The increase in clutch size we observed with the decreasing distance from the South American continent also suggests that nest predation has little effect on explaining the seabird trait structure. A conceptual model states that birds with small clutch sizes would be favored in zones with high nest predation since the energy allocation in a single reproduction attempt is relatively small compared to birds with large clutch sizes (Martin, 1995). However, this model does not apply to our study system since the number of terrestrial predators decreases from the South American continent to the Polynesian Islands (excluding invasive species; Simeone and Luna-Jorquera, 2012; Luna et al., 2018). Therefore, the smaller body mass and clutch size of the Polynesian seabird assemblages, represented mainly by species of the Family Procellariidae, possibly reflect an energy-saving adaptation.
The RLQ analysis separated the structure of the seabird assemblage from the Galapagos, Polynesian, and Chilean coastal islands. This separation reflects the three biogeographical realms proposed by Spalding et al. (2007) in this region; the Tropical Eastern Pacific, the Eastern Indo-Pacific, and the Temperate South American realms, respectively. The correlations of specific seabird traits with particular marine realms are consistent with the fourth corner analysis results, highlighting the adaptations of seabirds to cope with the environmental characteristics of each realm. For instance, for the seabird assemblages of Pitcairn and Rapa Nui, their small body mass and clutch sizes are consistent with relatively lower energetic demand for breeding compared to species with larger body mass and larger clutch sizes, and possibly represent an adaptation to the filtering influence of the oligotrophic waters of the center of the Pacific gyres. For highly productive systems of the provinces of the Warm Temperate south-eastern Pacific and along the Humboldt Current, increased marine productivity is positively correlated with large body mass and clutch size. The Tropical Eastern Pacific realm (Galapagos) was associated with high sea surface temperature and characterized by species with small clutch sizes. The negative relationship between clutch size and sea surface temperature is consistent with Rensch’s rules, which suggest larger clutch sizes in colder environments (Gaston et al., 2008). No evidence of Bergmann’s rule, which posits that larger body sizes of endotherms are expected in colder climates (Olson et al., 2009), was observed for our study system. The negative correlation observed between body mass and distance from the continent is also consistent with Olson et al. (2009), who suggest that birds tend to have larger median body mass on the mainland compared to oceanic islands. Thus, the trait structure of seabird assemblages of the south-eastern Pacific is a product of the local effects of island characteristics and the primary environmental filters established by the gradients observed among different biogeographical realms, which agrees with the finding of Nunes et al. (2017), i.e., that spatial phenotypic variation in seabirds may be strongly linked to oceanographic conditions.
Our study highlighted the marked differences in nesting assemblages of seabirds across different oceanic and coastal systems, reflecting the three marine biogeographical realms described in this region (Spalding et al., 2007). Since assemblages differ in species and trait structure, our results elucidate differences in the ecological roles of seabirds in these systems and the vulnerability of local seabirds colonies to the effects of climate change. In general, trait diversity is considered a better predictor of ecosystem function than species richness because traits are assumed to reflect the relationship of each species with its environment (Díaz and Cabido, 2001). Even though trait diversity metrics do not describe the direct relationship between species and functions, it is intuitive that species depicting high trait similarities tend to have similar niches (Villéger et al., 2011; Mason and De Bello, 2013). Considering the high species richness observed in Pitcairn and Rapa Nui (Gusmao et al., 2020) and the relatively low trait diversity observed in the Polynesian islands, we posit that these islands have high functional redundancy (i.e., species performing similar ecological roles; Luck et al., 2013; Pillar et al., 2013). This does not necessarily mean that the system is impoverished, but rather that these assemblages have a high chance of conserving functions when species are locally extinct (Luck et al., 2013). Assuming that the traits considered in this study are a fair representation of species niches, marine managers should be aware of islands with low species richness and high trait diversity since the local extinction of any species in such systems may have a greater probability of causing a significant loss in ecosystem functioning.
There is growing evidence showing the negative impact of anthropogenic activities on the functional diversity of birds (Matuoka et al., 2020; Stewart et al., 2022), particularly in archipelagos (Sayol et al., 2021). A recent meta-analysis demonstrated that human disturbances consistently reduce the functional diversity of bird assemblages and that impacts are higher in tropical areas (Matuoka et al., 2020). We cannot rule out these effects on our study systems. For instance, Rapanui is a textbook case of human ecocide, as the prehistoric human populations collapsed the fragile local ecosystem, leading to the extinction of most native birds and the further post-colonial invasion of other species (Plaza et al., 2021). It is likely that such species turnover leads to changes in the local functional diversity of Rapanui seabirds, as seen in other systems (Sayol et al., 2021). However, baseline information on the human impacts on other islands from our study archipelagos is unavailable.
Conclusion
Our results indicated that the trait diversity of seabird assemblages in our study system was explained by both local factors (e.g., island physiography) and large-scale environmental gradients (e.g., primary productivity as it related to the increasing distance from the South American continent). The latter also corresponded to gradients observed across three different marine biogeographical realms. Our findings reflect the adaptations of the species of seabirds in our study area to the oceanographic conditions surrounding their nesting islands. Seabirds with lower body mass and a small clutch size were associated with the distant Pitcairn and Rapa Nui archipelagos, suggesting energy-saving adaptations to cope with the oligotrophic conditions in that area. In contrast, seabirds nesting at Chilean coastal islands along the highly productive Humboldt Current were characterized by large body mass and large clutch sizes. The gradient in trait diversity observed from Polynesian islands to the Chilean coastal islands suggests the influence of environmental filters limiting trait diversity in the most distant archipelagos.
Data availability statement
The original contributions presented in the study are included in the article/Supplementary Material. Further inquiries can be directed to the corresponding author.
Author contributions
GL-J and JG designed and conceived the study. JG analyzed the data and wrote first version of the manuscript. All authors contributed to the article and approved the submitted version.
Funding
This study was funded by the Center for Ecology and Sustainable Management of Oceanic Islands ESMOI. JG was funded by the Millennium Nucleus ESMOI. MR was funded by the project “ANID-CENTROS REGIONALES R20F0008”.
Acknowledgments
We want to thank the members of the EDAM (Ecology and Diversity of Seabirds) Laboratory. They have been helping to collect data in the field and on board of ships for years, often in harsh environmental conditions. We thank the editor and two referees for constructive comments on the manuscript.
Conflict of interest
The authors declare that the research was conducted in the absence of any commercial or financial relationships that could be construed as a potential conflict of interest.
Publisher’s Note
All claims expressed in this article are solely those of the authors and do not necessarily represent those of their affiliated organizations, or those of the publisher, the editors and the reviewers. Any product that may be evaluated in this article, or claim that may be made by its manufacturer, is not guaranteed or endorsed by the publisher.
Supplementary material
The Supplementary Material for this article can be found online at: https://www.frontiersin.org/articles/10.3389/fmars.2022.897947/full#supplementary-material
Supplementary Data Sheet 1 | Compilfmars-09-897947ed data on the occurrence of seabird colonies nesting on islands of the southeastern Pacific Ocean. The consulted literature and sources are shown in the metadata tab.
Supplementary Data Sheet 2 | Compiled data on the physiography of islands of the southeastern Pacific Ocean. It also includes data on the oceanography of the surrounding waters. The consulted literature and sources are shown in the metadata tab.
Supplementary Data Sheet 3 | Compiled data on the biological traits of seabirds nesting on islands of the southeastern Pacific Ocean. The consulted literature and sources are shown.
References
Ashmole N. P., Ashmole M. J. (1967). Comparative feeding ecology of sea birds of a tropical oceanic island. Peabody Museum Nat. Hist. Bull. Yale Univ. 24, 1–131. doi: 10.2307/4511544
Assis J., Tyberghein L., Bosch S., Verbruggen H., Serrão E. A., De Clerck O. (2018). Bio-ORACLE v2.0: Extending marine data layers for bioclimatic modelling. Glob. Ecol. Biogeogr. 27, 277–284. doi: 10.1111/geb.12693
Azeria E. T., Ibarzabal J., Hébert C., Boucher J., Imbeau L., Savard J. P. L. (2011). Differential response of bird functional traits to post-fire salvage logging in a boreal forest ecosystem. Acta Oecologica 37, 220–229. doi: 10.1016/j.actao.2011.02.005
Barbaro L., Van Halder I. (2009). Linking bird, carabid beetle and butterfly life-history traits to habitat fragmentation in mosaic landscapes. Ecography (Cop.). 32, 321–333. doi: 10.1111/j.1600-0587.2008.05546.x
Barton A. D., Pershing A. J., Litchman E., Record N. R., Edwards K. F., Finkel Z. V., et al. (2013). The biogeography of marine plankton traits. Ecol. Lett. 16, 522–534. doi: 10.1111/ele.12063
Bennett P. M., Owens I. P. F. (2002). Evolutionary ecology of birds: Life histories, mating systems, and extinction (New York, U.S.A: Oxford University Press).
Borregaard M. K., Amorim I. R., Borges P. A. V., Cabral J. S., Fernández-Palacios J. M., Field R., et al. (2017). Oceanic island biogeography through the lens of the general dynamic model: Assessment and prospect. Biol. Rev. 92, 830–853. doi: 10.1111/brv.12256
Botta-Dukát Z. (2005). Rao’s quadratic entropy as a measure of functional diversity based on multiple traits. J. Veg. Sci. 16, 533–540. doi: 10.1111/j.1654-1103.2005.tb02393.x
Boyer A. G., Jetz W. (2010). Biogeography of body size in pacific island birds. Ecography (Cop.). 33, 369–379. doi: 10.1111/j.1600-0587.2010.06315.x
Brose U., Hillebrand H. (2016). Biodiversity and ecosystem functioning in dynamic landscapes. Philos. Trans. R. Soc B Biol. Sci. 371, 20150267. doi: 10.1098/rstb.2015.0267
Cerrano C., Danovaro R., Gambi C., Pusceddu A., Riva A., Schiaparelli S. (2009). Gold coral (Savalia savaglia) and gorgonian forests enhance benthic biodiversity and ecosystem functioning in the mesophotic zone. Biodivers. Conserv. 19, 153–167. doi: 10.1007/s10531-009-9712-5
Cook T. R., Lescroël A., Cherel Y., Kato A., Bost C.-A. (2013). Can foraging ecology drive the evolution of body size in a diving endotherm? PloS One 8, e56297. doi: 10.1371/journal.pone.0056297
Costello M. J., Tsai P., Wong P. S., Cheung A. K. L., Basher Z., Chaudhary C. (2017). Marine biogeographic realms and species endemicity. Nat. Commun. 8, 1–9. doi: 10.1038/s41467-017-01121-2
de Bello F., Carmona C. P., Lepš J., Szava-Kovats R., Pärtel M. (2016). Functional diversity through the mean trait dissimilarity: resolving shortcomings with existing paradigms and algorithms. Oecologia 180, 933–940. doi: 10.1007/s00442-016-3546-0
Dehling D. M., Töpfer T., Schaefer H. M., Jordano P., Böhning-Gaese K., Schleuning M. (2014). Functional relationships beyond species richness patterns: Trait matching in plant-bird mutualisms across scales. Glob. Ecol. Biogeogr. 23, 1085–1093. doi: 10.1111/geb.12193
Díaz S., Cabido M. (2001). Vive la différence: plant functional diversity matters to ecosystem processes. Trends Ecol. Evol. 16, 646–655. doi: 10.1016/S0169-5347(01)02283-2
Ding Z., Feeley K. J., Wang Y., Pakeman R. J., Ding P. (2013). Patterns of bird functional diversity on land-bridge island fragments. J. Anim. Ecol. 82, 781–790. doi: 10.1111/1365-2656.12046
Dolédec S., Chessel D., Ter Braak C. J. F., Champely S. (1996). Matching species traits to environmental variables: A new three-table ordination method. Environ. Ecol. Stat. 3, 143–166. doi: 10.1007/BF02427859
Dray S., Dufour A. B. (2007). The ade4 package: implementing the duality diagram for ecologists. J. Stat. Software 22, 1–20. doi: 10.18637/jss.v022.i04
Dray S., Legendre P. (2008). Testing the species traits–environment relationships: the fourth-corner problem revisited. Ecology 89, 3400–3412. doi: 10.1890/08-0349.1
Fisher J. A. D., Frank K. T., Leggett W. C. (2010). Global variation in marine fish body size and its role in biodiversity-ecosystem functioning. Mar. Ecol. Prog. Ser. 405, 1–13. doi: 10.3354/meps08601
Furness R. W., Monaghan P. (1987). Seabird ecology (New Your, U.S.A: Chapman and Hall). doi: 10.1007/978-1-4613-2093-7
Gaston K. J., Chown S. L., Evans K. L. (2008). Ecogeographical rules: Elements of a synthesis. J. Biogeogr. 35, 483–500. doi: 10.1111/j.1365-2699.2007.01772.x
González-Megías A., María Gómez J., Sánchez-Piñero F. (2007). Diversity-habitat heterogeneity relationship at different spatial and temporal scales. Ecography (Cop.). 30, 31–41. doi: 10.1111/j.2006.0906-7590.04867.x
González-Solís J., Shaffer S. A. (2009). Introduction and synthesis: Spatial ecology of seabirds at sea. Mar. Ecol. Prog. Ser. 391, 117–120. doi: 10.3354/meps08282
Gravel D., Albouy C., Thuiller W. (2016). The meaning of functional trait composition of food webs for ecosystem functioning. Philos. Trans. R. Soc B Biol. Sci. 371, 20150268. doi: 10.1098/rstb.2015.0268
Gusmao J. B., Luna-Jorquera G., Fernández C., Luna N., Plaza P., Portflitt-Toro M., et al. (2020). The role of island physiography and oceanographic factors in shaping species richness and turnover of nesting seabird assemblages on islands across the south-eastern pacific. J. Biogeogr. 47, 2611–2621. doi: 10.1111/jbi.13973
Hartel T., Hanspach J., Abson D. J., Máthé O., Moga C. I., Fischer J. (2014). Bird communities in traditional wood-pastures with changing management in Eastern Europe. Basic Appl. Ecol. 15, 385–395. doi: 10.1016/j.baae.2014.06.007
Hijmans R. J. (2021). raster: Geographic Data Analysis and Modeling. R package version 3.4-13. Available at: https://CRAN.R-project.org/package=raster.
Hijmans R. J. (2019). geosphere: Spherical Trigonometry.R package version 1.5-10. Available at : https://CRAN.R-project.org/package=geosphere.
Jiguet F., Gadot A.-S., Julliard R., Newson S. E., Couvet D. (2007). Climate envelope, life history traits and the resilience of birds facing global change. Glob. Change Biol. 13, 1672–1684. doi: 10.1111/j.1365-2486.2007.01386.x
Jungblut S., Nachtsheim D. A., Boos K., Joiris C. R. (2017). Biogeography of top predators – seabirds and cetaceans – along four latitudinal transects in the Atlantic ocean. Deep. Res. Part II Top. Stud. Oceanogr. 141, 59–73. doi: 10.1016/j.dsr2.2017.04.005
Keck B. P., Marion Z. H., Martin D. J., Kaufman J. C., Harden C. P., Schwartz J. S., et al. (2014). Fish functional traits correlated with environmental variables in a temperate biodiversity hotspot. PloS One 9, e93237. doi: 10.1371/journal.pone.0093237
Keith C. H., Schreiber E. A., Burger J. (2001). “Breeding biology, life histories, and life history-environment interactions in seabirds,” in Biology of marine birds. Eds. Schreiber E. A., Burger J. (New York, U.S.A: CRC Press), 722.
Laliberté E., Legendre P. (2010). A distance-based framework for measuring functional diversity from multiple traits. Ecology 91, 299–305. doi: 10.1890/08-2244.1
Laliberté E., Legendre P., Shipley B. (2014). FD: measuring functional diversityfrom multiple traits, and other tools for functional ecology. R package version 1.0-12.
Legendre P., Galzin R. G., Harmelin-Vivien M. L. (1997). Relating behavior to habitat: Solutions to the fourth-corner problem. Ecology 78, 547–562. doi: 10.2307/2266029
Levin L. A., Etter R. J., Rex M. A., Gooday A. J., Smith C. R., Pineda J., et al. (2001). Environmental influences on regional deep-sea species diversity. Annu. Rev. Ecol. Syst. 32, 51–93. doi: 10.1146/annurev.ecolsys.32.081501.114002
Lomolino M. V., Weiser M. D. (2001). Towards a more general species-area relationship: diversity on all islands, great and small. J. Biogeogr. 28, 431–445. doi: 10.1046/j.1365-2699.2001.00550.x
Loret J., Tanacredi J. T. (2003). Easter Island: Scientific exploration into the world’s environmental problems in microcosm. Eds. Loret J., Tanacredi J. T. (Boston, MA: Springer U). doi: 10.1007/978-1-4615-0183-1
Luck G. W., Carter A., Smallbone L. (2013). Changes in bird functional diversity across multiple land uses: interpretations of functional redundancy depend on functional group identity. PloS One 8, e63671. doi: 10.1371/journal.pone.0063671
Luiza-Andrade A., Brasil L. S., Benone N. L., Shimano Y., Farias A. P. J., Montag L. F., et al. (2017). Influence of oil palm monoculture on the taxonomic and functional composition of aquatic insect communities in eastern Brazilian Amazonia. Ecol. Indic. 82, 478–483. doi: 10.1016/j.ecolind.2017.07.006
Luna-Jorquera G., Fernández C. E., Rivadeneira M. M. (2012). Determinants of the diversity of plants, birds and mammals of coastal islands of the Humboldt current systems: implications for conservation. Biodivers. Conserv. 21, 13–32. doi: 10.1007/s10531-011-0157-2
Luna N., Varela A. I., Brokordt K., Luna-Jorquera G. (2018). Assessing potential predation risk by introduced predators on unattended eggs in the red-tailed tropicbird, phaethon rubricauda, on rapa nui (Easter island). Trop. Conserv. Sci., 1–8. doi: 10.1177/1940082918785079
MacArthur R. H., Wilson E. O. (1963). An equilibrium theory of insular zoogeography. Evol. (N. Y). 17, 373–387. doi: 10.1111/j.1558-5646.1963.tb03295.x
MacArthur R. H., Wilson E. O. (1967). The theory of island biogeography (Princeton, N.J.: Princeton University Press), 203 p.
Mancini P. L., Bugoni L. (2014). Resources partitioning by seabirds and their relationship with other consumers at and around a small tropical archipelago. ICES J. Mar. Sci. 71, 2599–2607. doi: 10.1093/icesjms/fsu105
Martin T. E. (1995). Avian life history evolution in relation to nest sites, nest predation, and food. Ecol. Monogr. 65, 101–127. doi: 10.2307/2937160
Mason N. W. H., De Bello F. (2013). Functional diversity: A tool for answering challenging ecological questions. J. Veg. Sci. 24, 777–780. doi: 10.1111/jvs.12097
Mason N. W. H. H., De Bello F., Mouillot D., Pavoine S., Dray S. (2013). A guide for using functional diversity indices to reveal changes in assembly processes along ecological gradients. J. Veg. Sci. 24, 794–806. doi: 10.1111/jvs.12013
Mason N. W. H., Mouillot D., Lee W. G., Wilson J. B. (2005). Functional richness, functional evenness and functional divergence: the primary components of functional diversity. Oikos 111, 112–118. doi: 10.1111/j.0030-1299.2005.13886.x
Matuoka M. A., Benchimol M., Almeida-Rocha J. M.de, Morante-Filho J. C. (2020). Effects of anthropogenic disturbances on bird functional diversity: A global metaanalysis. Ecol. Indic. 116, 106471. doi: 10.1016/j.ecolind.2020.106471
McNab B. K. (2003). The physiological ecology of vertebrates: A view from energetics. J. Mammal. 84, 774–775. doi: 10.1644/1545-1542(2003)084<0774:TPEOVA>2.0.CO;2
Morais G. C., Gusmao J. B., Oliveira V. M., Lana P. (2019). Macrobenthic functional trait diversity at multiple scales along a subtropical estuarine gradient. Mar. Ecol. Prog. Ser. 624, 23–37. doi: 10.3354/meps13033
Mouchet M. A., Villéger S., Mason N. W. H., Mouillot D. (2010). Functional diversity measures: an overview of their redundancy and their ability to discriminate community assembly rules. Funct. Ecol. 24, 867–876. doi: 10.1111/j.1365-2435.2010.01695.x
Mouillot D., Graham N. A. J., Villéger S., Mason N. W. H., Bellwood D. R. (2013). A functional approach reveals community responses to disturbances. Trends Ecol. Evol. 28, 167–177. doi: 10.1016/j.tree.2012.10.004
Nunes G. T., Mancini P. L, Bugoni L. (2017). When Bergmann's rule fails: evidences of environmental selection pressures shaping phenotypic diversification in a widespread seabird. Ecography 40, 365–375. doi: 10.1111/ecog.02209
Olson V. A., Davies R. G., Orme C. D. L., Thomas G. H., Meiri S., Blackburn T. M., et al. (2009). Global biogeography and ecology of body size in birds. Ecol. Lett. 12, 249–259. doi: 10.1111/j.1461-0248.2009.01281.x
Paradis E., Claude J., Strimmer K. (2004). APE: Analyses of phylogenetics and evolution in r language. Bioinformatics 20, 289–290. doi: 10.1093/bioinformatics/btg412
Penven P., Echevin V., Pasapera J., Colas F., Tam J. (2005). Average circulation, seasonal cycle, and mesoscale dynamics of the Peru current system: A modeling approach. J. Geophys. Res. C Ocean. 110, 1–21. doi: 10.1029/2005JC002945
Peres-Neto P. R., Dray S., Braak C. J. F. (2017). Linking trait variation to the environment: critical issues with community-weighted mean correlation resolved by the fourth-corner approach. Ecography (Cop.). 40, 806–816. doi: 10.1111/ecog.02302
Petchey O. L., Gaston K. J. (2006). Functional diversity: back to basics and looking forward. Ecol. Lett. 9, 741–758. doi: 10.1111/j.1461-0248.2006.00924.x
Pillar V. D., Blanco C. C., Müller S. C., Sosinski E. E., Joner F., Duarte L. D. S. (2013). Functional redundancy and stability in plant communities. J. Veg. Sci. 24, 963–974. doi: 10.1111/jvs.12047
Plaza P., Serratosa J., Gusmao J. B., Duffy D. C., Arce P., Luna-Jorquera G. (2021). Temporal changes in seabird assemblage structure and trait diversity in the rapa nui (Easter island) multiple-use marine protected area. Aquat. Conserv. Mar. Freshw. Ecosyst. 31, 378–388. doi: 10.1002/aqc.3501
Quillfeldt P., Schroff S., van Noordwijk H., Michalik A., Ludynia K., Masello J. (2011). Flexible foraging behaviour of a sexually dimorphic seabird: large males do not always dive deep. Mar. Ecol. Prog. Ser. 428, 271–287. doi: 10.3354/meps09058
Rayner L., Lindenmayer D. B., Wood J. T., Gibbons P., Manning A. D. (2014). Are protected areas maintaining bird diversity? Ecography (Cop.). 37, 43–53. doi: 10.1111/j.1600-0587.2013.00388.x
R Core Team (2021). R: A language and environment for statistical computing. (Vienna, Austria: R Foundation for Statistical Computing). Available at: https://www.R-project.org/.
Ricotta C., Szeidl L. (2009). Diversity partitioning of rao’s quadratic entropy. Theor. Popul. Biol. 76, 299–302. doi: 10.1016/j.tpb.2009.10.001
Roth R. R. (1976). Spatial heterogeneity and bird species diversity. Ecology 57, 773–782. doi: 10.2307/1936190
Sayol F., Cooke R. S.C., Pigot A. L., Blackburn T. M., Tobias J. A., Steinbauer M. J., et al. (2021). Loss of functional diversity through anthropogenic extinctions of island birds is not offset by biotic invasions. Sci. Adv. 7. doi: 10.1126/sciadv.abj5790
Schleuter D., Daufresne M., Massol F., Argillier C. (2010). A user’s guide to functional diversity indices. Ecol. Monogr. 80, 469–484. doi: 10.1890/08-2225.1
Schreiber E. A., Burger J. (2002). Biology of marine birds (Boca Raton: CRC Press). doi: 10.1201/9781420036305
Serratosa J., Hyrenbach K. D., Miranda-Urbina D., Portflitt-Toro M., Luna N., Luna-Jorquera G. (2020). Environmental drivers of seabird At-Sea distribution in the Eastern south pacific ocean: Assemblage composition across a longitudinal productivity gradient. Front. Mar. Sci. 6. doi: 10.3389/fmars.2019.00838
Shealer D. (2001). “Foraging behavior and food of seabirds,” in Biology of marine birds. Eds. Schreiber E. A., Burger J. (Boca Raton: CRC Press), 137–178. doi: 10.1201/9781420036305.ch6
Simeone A., Luna-Jorquera G. (2012). Estimating rat predation on Humboldt penguin colonies in north-central Chile. J. Ornithol. 153, 1079–1085. doi: 10.1007/s10336-012-0837-z
Spalding M. D., Fox H. E., Allen G. R., Davidson N., Ferdaña Z. A., Finlayson M., et al. (2007). Marine ecoregions of the world: A bioregionalization of coastal and shelf areas. Bioscience 57, 573–583. doi: 10.1641/B570707
Stewart P. S., Voskamp A., Santini L., Biber M. F., Devenish A. J. M., Hof C., et al. (2022). Global impacts of climate change on avian functional diversity. Ecol. Lett. 25, 673–685. doi: 10.1111/ele.13830
Stuart-Smith R. D., Bates A. E., Lefcheck J. S., Duffy J. E., Baker S. C., Thomson R. J., et al. (2013). Integrating abundance and functional traits reveals new global hotspots of fish diversity. Nature 501, 539–542. doi: 10.1038/nature12529
Thiel M., Macaya E. C, Acuña E., Arntz W. E, Bastias H., Brokordt K., et al. (2007). The Humboldt current system of northern and central Chile: oceanographic processes, ecological interactions and socioeconomic feedback. In: Gibson R. N., Atkinson R. J. A., Gordon J. D. M. (Eds.), Oceanography and Marine Biology an Annual Review 45 (Taylor and Francis), 195–344. doi: 10.1201/9781420050943
Tyberghein L., Verbruggen H., Pauly K., Troupin C., Mineur F., De Clerck O. (2012). Bio-ORACLE: A global environmental dataset for marine species distribution modelling. Glob. Ecol. Biogeogr. 21, 272–281. doi: 10.1111/j.1466-8238.2011.00656.x
Villéger S., Novack-Gottshall P. M., Mouillot D. (2011). The multidimensionality of the niche reveals functional diversity changes in benthic marine biotas across geological time. Ecol. Lett. 14, 561–568. doi: 10.1111/j.1461-0248.2011.01618.x
Weichler T., Garthe S., Luna-Jorquera G., Moraga J. (2004). Seabird distribution on the Humboldt current in northern Chile in relation to hydrography, productivity, and fisheries. ICES J. Mar. Sci 61, 148–154. doi: 10.1016/j.icesjms.2003.07.001
Whittaker R. J., Triantis K. A., Ladle R. J. (2008). A general dynamic theory of oceanic island biogeography. J. Biogeogr. 35, 977–994. doi: 10.1111/j.1365-2699.2008.01892.x
Wickham H. (2009). ggplot2: Elegant graphics for data analysis (New York, NY: Springer New York). doi: 10.1007/978-0-387-98141-3
Wilman H., Belmaker J., Simpson J., de la Rosa C., Rivadeneira M. M., Jetz W. (2014). EltonTraits 1.0: Species-level foraging attributes of the world’s birds and mammals. Ecology 95, 2027–2027. doi: 10.1890/13-1917.1
Wouters J. M., Gusmao J. B., Mattos G., Lana P. (2018). Polychaete functional diversity in shallow habitats: Shelter from the storm. J. Sea Res. 135, 18–30. doi: 10.1016/j.seares.2018.02.005
Keywords: macroecology, functional diversity (FD), trait diversity, oceanic islands, coastal islands, RLQ, fourth-corner analysis
Citation: Gusmao JB, Luna-Jorquera G and Rivadeneira MM (2022) Oceanographic gradients explain changes in the biological traits of nesting seabird assemblages across the south-eastern Pacific. Front. Mar. Sci. 9:897947. doi: 10.3389/fmars.2022.897947
Received: 16 March 2022; Accepted: 12 October 2022;
Published: 27 October 2022.
Edited by:
Patrick Jodice, United States Geological Survey (USGS), United StatesReviewed by:
Luke Bower, United States Geological Survey (USGS), United StatesPatricia Mancini, Federal University of Rio de Janeiro, Brazil
Copyright © 2022 Gusmao, Luna-Jorquera and Rivadeneira. This is an open-access article distributed under the terms of the Creative Commons Attribution License (CC BY). The use, distribution or reproduction in other forums is permitted, provided the original author(s) and the copyright owner(s) are credited and that the original publication in this journal is cited, in accordance with accepted academic practice. No use, distribution or reproduction is permitted which does not comply with these terms.
*Correspondence: Guillermo Luna-Jorquera, Z2x1bmFAdWNuLmNs