- 1Marine Biology Research Group, Department of Biology, Ghent University, Ghent, Belgium
- 2Aquatic Environment and Quality, Institute for Agricultural and Fisheries Research (ILVO), Oostende, Belgium
- 3Centre for Molecular Phylogeny and Evolution (CeMoFE), Department of Biology, Ghent University, Ghent, Belgium
Resource partitioning is a mechanism for niche differentiation which can facilitate coexistence of species at local scales. Insights into resource use and niche differentiation of functionally similar and/or taxonomically closely related species are scant, especially so for small organisms that dominate marine sediments in terms of abundance and species richness. In this study we characterized the microbiomes of 10 bacterivorous nematode species co-occurring in various estuarine microhabitats using 16S rRNA metabarcoding to address their resource utilization. We investigated microbiome diversity and composition of nematodes collected from six microhabitats in the field: Fucus spiralis, Fucus vesiculosus, fresh and decomposing thalli of Ulva sp., and attached and detached leaves of Spartina anglica. The 10 species belonged to three different families, and included congeneric and morphologically cryptic species. The microbiome of species sharing their microhabitats showed a substantial overlap, suggesting that resource differentiation of species within a microhabitat was rather limited at time of sampling. Microbiomes of the same nematode species found in different microhabitats were also not strongly differentiated. Temporal divergence in resource use may occur, as suggested by temporally divergent microbiome composition of nematodes associated with Ulva sp. The observed high intraspecific variability in microbiome composition of nematodes may be the principal factor explaining the lack of microbiome differentiation among species or microhabitats and may illustrate individual specialization in resource use as a consequence of substantial intraspecific competition.
1 Introduction
Coexistence of functionally similar and/or taxonomically related species has long intrigued ecologists, because species with almost identical ecological niches/functions cannot coexist under a purely deterministic coexistence model since their intense competition would ultimately lead to exclusion (Hardin, 1960). Under the ‘niche concept’, natural selection drives the evolution of species into divergent and complementary niches (Hector and Hooper, 2002; Chase and Leibold, 2003). The more divergent their niches, the less intensely species will compete, and the more prominently intraspecific rather than interspecific competition regulates population dynamics (Leibold and McPeek, 2006). Niche differentiation insights are based on the differential tolerances of species to abiotic conditions and/or to their differential resource utilization, which can affect demographic traits and population fitness, resulting in a higher competence of one species over others, given a particular niche (Chase and Leibold, 2003). A stable species coexistence can therefore be maintained when co-occurring species are favored under different sets of environmental conditions, inhabit different structures of the same habitat (i.e., microhabitats), and/or systematically utilize different resources (Abrams, 1983; Ekschmitt and Griffiths, 1998; Chesson, 2000). None of the above is absolute, particularly when small spatial scales are considered, as species share very similar conditions, microhabitats and resources in the same habitat at the same time. Despite such niche overlap, differentiation of the ‘realized niche’ of closely related species under competition can occur, leaving some ‘space’ within the niche for competent species to persist (Hutchinson, 1959; Bruno et al., 2003). Conversely, when species with (almost) identical ecological traits and fitness compete locally (Chesson, 2000), their coexistence can be attained due to neutral dynamics (Hubbell, 2001).
In the sediments of aquatic ecosystems, nematodes are often the most abundant and diverse invertebrates (Heip et al., 1985; Traunspurger, 2000; Moens et al., 2013). A large number of species can co-occur at very small spatial scales (e.g., at submeter scales), holding a number of horizontal interactions, including competitive, facilitative and inhibitory responses (De Mesel et al., 2006; dos Santos et al., 2009; dos Santos and Moens, 2011; De Meester et al., 2015a). Based on commonly used functional guild classifications (Wieser, 1953; Bongers et al., 1991), closely related species (confamiliar, congeneric) are implicitly assumed to be confunctional. This has been recently overturned by the discovery of cryptic species within the same morphospecies complex that have sympatric distributions (Derycke et al., 2005; Derycke et al., 2007a; Derycke et al., 2012; Derycke et al., 2013; Ristau et al., 2013) and non-identical functions (De Meester et al., 2016) and exhibit differential tolerances to environmental conditions (De Meester et al., 2011; Van Campenhout et al., 2014; De Meester et al., 2015a; De Meester et al., 2015b; Monteiro et al., 2018). Evidence for microhabitat and resource differentiation among cryptic nematode species has also been provided (Derycke et al., 2016; Guden et al., 2018). Nevertheless, knowledge on niche divergence and its mechanisms between sympatric nematode species belonging to the same functional guild is scant (Venette and Ferris, 1998; Blanc et al., 2006; Estifanos et al., 2013), particularly in marine nematodes. For example, bacterivorous nematodes of the families Monhysteridae and Rhabditidae, each usually represented by several species, frequently co-occur within various microhabitats (e.g., decomposing macroalgae) in the intertidal zone of coasts and estuaries (Derycke et al., 2006; Derycke et al., 2007a; Derycke et al., 2008; dos Santos et al., 2009). At least some degree of resource differentiation has been suggested among confamiliar species of Monhysteridae (Moens et al., 1999) and among cryptic species of Litoditis marina (Derycke et al., 2016) originating from the same location and microhabitats, but the extent of such resource differentiation remains poorly understood. Furthermore, the decomposition status of macroalgae has been suggested to play a role in substrate colonization preferences of closely related nematode species (Moens et al., 1999), which may reflect resource differentiation in relation to decomposition.
High-throughput sequencing technologies (HTS) nowadays allow the characterization of microbial communities, including remnants of ingested bacteria, associated with bacterial-feeding nematodes (Derycke et al., 2016; Schuelke et al., 2018; Rzeznik-Orignac et al., 2018). Microbiomes normally comprise the genetic diversity of all microorganisms associated with a host, including the gut microflora (commensal bacteria), other (endo-/ecto-) symbiotic or pathogenic bacteria (called microbiome ‘sensu stricto’), and bacteria utilized as food or attached to it (microbiome ‘sensu lato’) (Musat et al., 2007; Derycke et al., 2016; Dirksen et al., 2016; Schulenburg and Félix, 2017). Therefore, microbiome characterization has potential to explain niche partitioning linked to resource availability and utilization, but also linked to symbiotic relationships with microbial organisms (Schuelke et al., 2018).
Here we aim to explore differences in microbiomes of bacterivorous nematode species that co-occur in the same habitats to provide insights into resource use and niche differentiation of functionally similar species. Co-occurring monhysterid and rhabditid nematodes from microhabitats in the intertidal zone of the Scheldt Estuary, the Netherlands, were collected for individual microbiome characterization, using amplicon sequencing of the bacterial 16S rRNA gene. Based on the classical coexistence theory and the niche differentiation concept, we expected that resource partitioning would be more prominent among species, thus resulting in greater microbiome differences within shared microhabitats and less among microhabitats. Furthermore, we anticipated that nematode species would exhibit some degree of plasticity in their resource use and thus, they would have partly different microbiomes in different microhabitats, a main factor for niche divergence that could contribute to microhabitat diversification. We also hypothesized that resource differentiation of species within microhabitats may vary in time, along with the natural temporal variability of the microbial community associated with algae and cordgrass in the field, or with decomposition status of the phytal microhabitat where the nematodes occur.
2 Materials and Methods
2.1 Sampling
Nematodes were sampled by collecting fractions of their potential microhabitats (here macroalgae and saltmarsh plants) in the Paulina salt marsh in the polyhaline reach of the Scheldt Estuary, the Netherlands (51°21’01.9”N 3°43’09.1”E). Samples of algae or saltmarsh material were collected at low tide at two sampling occasions representing two sampling seasons, in November 2015 (autumn) and in April 2016 (spring). Microhabitats were chosen as a function of the ‘target’ nematode species (Table 1) we were aiming to collect, based on information from a year-round monthly survey of the co-occurrence of abundant bacterivorous species in this saltmarsh (Moens et al., 1999). The sampled microhabitats included: decomposing thalli of the brown algae Fucus vesiculosus L. and Fucus spiralis L., fresh and decomposing thalli of the green alga Ulva sp. L., and decomposing but still attached as well as detached leaves of the cordgrass Spartina anglica C.E. Hubb. Decomposing Ulva thalli and old/attached Spartina leaves were only found in November. Three replicates per type of material (i.e., an entire algal thallus or, in the case of fragmented material or cordgrass leaves, an approximately equal amount per replicate) were haphazardly collected from the same location (within 1m scale for microhabitats of the same type and within few meters at the same site for different microhabitat types, e.g. cordgrass and macroalgae) on each sampling occasion, to increase the chance of obtaining enough specimens of the ‘target nematode species’. These were transferred on ice and stored frozen in the laboratory at -20°C until further processing (< 2 months after sampling). Samples of algae or cordgrass were washed carefully into a 5L glass beaker with distilled water, which was then decanted and sieved over a 32-μm mesh, and the supernatant was kept in smaller vials for direct observation of the nematodes. This procedure was repeated 5 times for each sample.
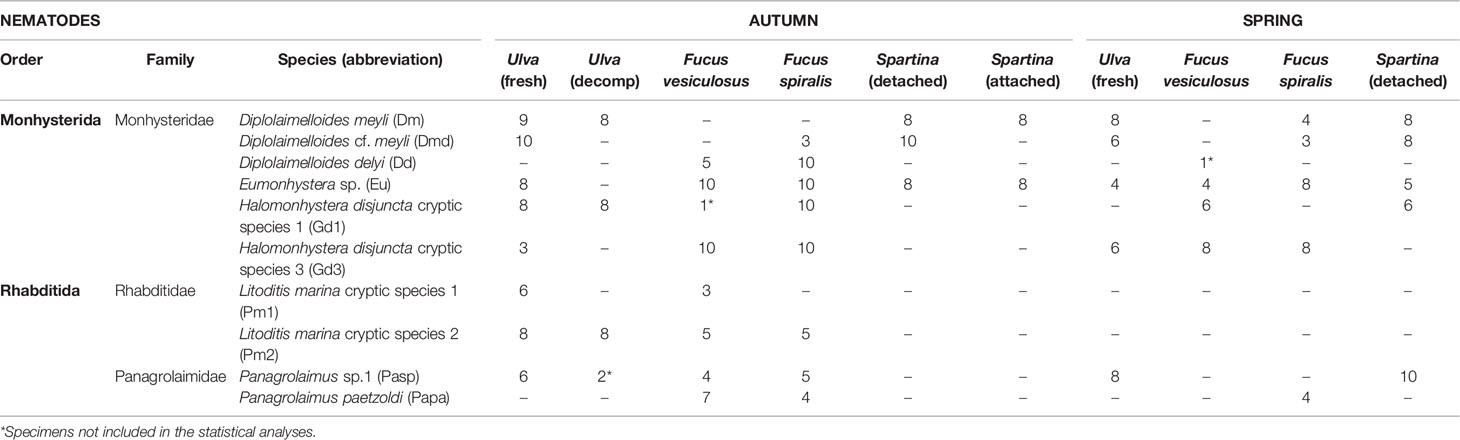
Table 1 Identified nematode species and number of specimens collected from each microhabitat type in autumn and spring.
2.2 DNA Extraction and Nematode Identification
Morphology-based identification and DNA barcoding was used to create 345 distinct samples of nematode specimens collected from 30 algal/plant material samples. Nematodes were first identified under an inverted microscope into 10 species belonging to 3 families (i.e., Rhabditidae, Panagrolaimidae, Monhysteridae) (Table 1), and individuals of the ‘target species’ were sorted in a counting dish and hand-picked with a fine Tungsten wire under a stereomicroscope for identification to species level using DNA-barcoding. They were bathed one by one in an embryo dish with molecular-grade water to remove adhering particles and bacteria. Each specimen was transferred to 20 μl of Worm Lysis Buffer (WLB) (50 mM KCl, 10 mM Tris pH 8.3, 2.5 mM MgCl2, 0.45% NP40 (Tergitol Sigma), 0.45% Tween20) in a single microcentrifuge tube (Eppendorf AG, Hamburg, Germany), and stored in the freezer (at -20°C) before DNA extraction. DNA was extracted by adding 1 μl of proteinase K (10 mg ml-1) in the tube, which was incubated for 60 min at 65°C, then for 10 min at 95°C, and finally centrifuged for 1 min at maximum speed (13 000 rpm). DNA extracts were stored at -20°C until further processing.
1 μl of the extracted DNA per sample was used as template DNA for 24 µl of PCR mixture (containing 2.5 µl of 10 × PCR buffer (15 mM MgCl2 (Qiagen), 2.5 µl loading dye (Qiagen), 2 µl MgCl2 (25 mM), 0.5 µl dNTP (10 mM), 0.125 µl of each primer (25 µM), 0.125 µl TopTaq DNA polymerase (Qiagen), and 16.125 µl PCR grade water) for PCR amplifications. The primer set G18S4 forward (5’-GCTTGTCTCAAAGATTAAGCC-3’) (Blaxter et al., 1998) and 4R reverse (5’-GTATCTGATCGCCKTCGAWC-3’) (Armenteros et al., 2014) was used to amplify part (ca. 800 bp) of the 18S rRNA gene (small subunit or SSU). PCR amplification was performed with the following optimized cycle conditions: an initial denaturation step for 5 min at 94°C, 40 cycles of denaturation for 30 s at 94°C, annealing for 30 s at 56°C, and extension for 1 min at 72°C, and a final extension step for 10 min at 72°C. PCR products were loaded on agarose gels to test the amplification success and confirm fragment length. PCR products (single bands) were purified using an enzymatic cleanup protocol (EXOFastAP). 5 µl of the PCR product was mixed with 0.6 µl of alkaline phosphatase FastAP (1U/µl, Thermo Fisher Scientific) and 0.3 µl of Exonuclease I (20 U/µl) on ice, and incubated in a thermocycler (15 min at 37°C and 15 min at 85°C). Purified PCR products were used for bidirectional Sanger sequencing performed by Macrogen Europe. Our laboratory workflow was carefully carried out to minimize potential background contamination from air, dust, laboratory surfaces, equipment and kit reagents. Pre-sterilized disposable materials and equipment were used throughout. All surfaces and non-disposable glass or plexiglass materials were bleach-cleaned (e.g., beakers, pipettes, counting dishes, etc.) and sieves were sonicated for 5 min in between each sample processing. The Tungsten wire was cleaned with 70% ethanol followed by flame sterilization prior to picking each new specimen. To ensure a reliable species identification, the 18S sequences were blasted against Genbank to identify the nematodes using DNA barcoding. Individuals identified as Litoditis marina were further identified to the correct cryptic species using the quantitative real-time PCR (qPCR) method with species-specific primers for the ribosomal internal transcribed spacer region (ITS) (see Derycke et al., 2012). Melting curves were analyzed for the quality control of the results.
2.3 16S rRNA Gene Amplification and Library Preparation for Illumina Miseq Sequencing
We used an adapted protocol of the Earth Microbiome project for the bacterial ribosomal DNA amplification and library preparation for the Illumina Miseq sequencing (Caporaso et al., 2012; Gilbert et al., 2014). DNA extracts of 345 single nematodes were used for the amplification of 16S rRNA gene fragments. The 515F forward (5’-GTGCCAGCMGCCGCGGTAA-3’) and 806R reverse (5’-GGACTACHVGGGTWTCTAAT-3’) PCR primers were used to target the V4 region (ca. 390 bp) (Caporaso et al., 2012). The forward primer contained the 5’ Illumina adapter, the forward primer pad and primer linker, and the 515F primer. The reverse primer contained the reverse complement of the 3’ Illumina adapter, the reverse primer pad and primer linker, the 806R primer and a Golay barcode, unique for each sample (Caporaso et al., 2012), in order to identify each sample during downstream in silico analysis. PCR amplification was performed in 20 µl total reaction volume containing 11.4 µl water, 4 µl buffer (10X), 0.4 µl dNTPs (10mM), 0.2 µl Phusion (high fidelity) polymerase, 1 µl of each primer (10 μM) and 2 µl DNA template. PCR cycle conditions included: an initial denaturation step of 30 s at 98°C, followed by 35 cycles of 10 s at 98°C, 30 s at 65°C, 15 s at 72°C, and a final step of 10 min at 72°C (Derycke et al., 2016). Samples were amplified in technical triplicates, which were then pooled in one sample. Pooled PCR products were purified using a magnetic bead purification protocol with Agencourt AMPure XP beads (Beckman Coulter, CA, USA; the manufacturer’s protocol was followed). PCR library concentrations of the purified samples were measured with a Qubit 3.0 fluorometer (Thermo Fisher Scientific) and equimolarly pooled (15ng). Three libraries-pooled samples were prepared for 3 Illumina Miseq runs, which contained 100, 112 and 133 pooled nematode individuals, respectively. Separate runs were used because of the number of individual samples and the availability of Golay barcodes, necessary for the identification of individual samples during data analysis. Quality control of the three libraries was done with the Bioanalyzer (Agilent Technologies). Purification with AMPure XP beads of the libraries was repeated to remove primer dimers for pools two and three. Each library was sequenced separately on the Illumina Miseq (2 × 300 bp paired-end runs) by Edinburgh Genomics (UK).
2.4 Data Analysis
The demultiplexed Illumina paired-end sequences of the three sequencing runs were further processed together using the DADA2 pipeline (Callahan et al., 2016a) in R, v. 4.0.1 (R Core Team, 2020) and filtered/trimmed using default settings and the Bioconductor workflow (Callahan et al., 2016b), except for truncating of forward and reverse reads at 240 and 200 bp, respectively. Forward and reverse reads with >2 estimated errors were filtered and were truncated at both ends, where read quality dropped below a quality score of 2 (TrunQ = 2). Trimming and filtering was performed on paired reads jointly (both reads must pass the filter for the pair to pass). Adapter sequences were removed, denoised paired-end sequences were merged and Amplicon Sequence Variants (ASVs) were generated after separate estimations of error rates on forward and reverse reads. A Chimera removal step was performed using the consensus method with the ‘removeBimeraDenovo’ function and taxonomy was assigned to ASVs with the Naïve Bayesian RDP classifier (Wang et al., 2007) using the ‘assignTaxonomy’ function with default settings (50% confidence) and the SSU Silva database (v132) as a reference. After filtering and quality control steps, 5 samples were removed based on number of reads and therefore, the full dataset further analyzed included sequences of 340 individual nematode specimens.
2.5 Microbial Diversity Analysis
Downstream data analysis and visualization were conducted using the package ‘phyloseq’ in R (McMurdie and Holmes, 2013). Microbiome diversity was assessed in terms of alpha and beta diversity, which were then statistically compared according to research questions: (i) among species per microhabitat (1 factor: Species); (ii) among microhabitats per species (1 factor: Microhabitat); (iii) across microhabitats and time per species, for species that occurred in the same microhabitats in both sampling seasons (2 factors: Microhabitat, Time); and (iv) among species and microhabitats of different decomposition status (2 factors: Species, Status) for Ulva sp. fresh vs decomposing thalli and for Spartina anglica attached vs detached leaves. For the last research question (iv), two subsets of species co-occurring on these different microhabitats were used and microbiome diversity and composition within microhabitat were compared between ‘Species’ (Sp) and ‘Status’ (St) in a cross-factorial design. Note here that these are in fact different microhabitats per se, but we use the term ‘Status’ here for the purpose of comparison. For the above comparisons, except for (iii), data from the autumn and spring sampling occasions were analyzed separately, due to a lack of sufficient specimens from the same microhabitat on one of the two sampling seasons for most species.
Alpha diversity parameters (i.e., taxon richness as number of observed ASVs, Shannon diversity index) were estimated using the rarefied dataset at a minimum sequencing depth of 10,000 reads (32 samples with lower sequence numbers were excluded). This threshold was chosen as a trade-off to keep as many samples as possible in the dataset while the number of taxa observed in the retained samples was still in the plateau phase of the rarefaction curve (see SM2-Figure 1A). Alpha diversity of the rarefied dataset was then compared between grouping factors using Analysis of Variance (ANOVA), the assumptions of normality of the data and homogeneity of variances, tested using Shapiro–Wilk test and Levene’s test respectively, being met. Microbial community composition analysis was based on the Generalized UniFrac distances (GUniFrac) calculated with the ‘GUniFrac’ package (Chen et al., 2012), using the rarefied dataset. Generalized UniFrac distance was used instead of weighted or unweighted UniFrac distance, containing an extra parameter α (here α = 0.05) to weigh on abundant lineages, so that the distance is not dominated by highly abundant ASVs (Chen et al., 2012). Comparisons of microbiome composition across grouping factors were performed using Permutational Multivariate Analysis of Variance (PERMANOVA) based on the GUniFrac distances, using the ‘vegan’ package (Oksanen et al., 2017). Pairwise difference tests were performed on significant factors, and multivariate dispersions were tested with PERMDISP. Principal coordinates analysis (PCoA) was used to visualize community composition differences within and between grouping factors, using the ‘ade4’ package (Dray and Dufour, 2007). Differential abundance analysis for sequence count data was performed for the complete dataset (non-rarefied sequences of all species and microhabitats) using ‘DESeq2’ with standard parameters (Love et al., 2014) and a significance threshold of a=0.05 (adjusted p-value). Further plotting was performed using the R packages ‘microbiome’ (Lahti and Shetty, 2012-2019) and ‘ggplot2’ (Wickham, 2016) and basic R functions.
3 Results
3.1 Taxonomic Characterization of Microbiomes Associated With Marine Nematodes
The microbiomes of a total of 340 nematode specimens belonging to 10 species (5 genera, 3 families) from 6 different microhabitats (i.e., Fucus spiralis, Fucus vesiculosus, Ulva sp. fresh and decomposing thalli, and Spartina anglica attached and detached leaves) at two sampling moments/seasons (autumn and spring) were characterized (Table 1) and resulted in a total of 28,620,125 filtered reads for the entire dataset with an average sequencing depth of 84,176 reads (median: 68,447 reads) per nematode specimen (see SM2: Table 1). A total of 14,117 distinct ASVs were obtained (average 135 ± 106 per specimen). The vast majority of ASVs were assigned to the Bacterial domain (99.05%, 13,984 ASVs), a few were Archaea (0.34%, 49 ASVs) and the rest remaining taxonomically unassigned.
The phylum Proteobacteria was the most abundant bacterial phylum regardless of the nematode species (46.45% of the bacterial taxa), followed by Bacteroidetes (20.47%), Actinobacteria (5.97%), Firmicutes (5.67%), and Cyanobacteria (2.47%; Figure 1A). Within the phylum Proteobacteria, a high proportion of ASVs was assigned to the Gammaproteobacteria (48.40%), with the families Halomonadaceae (12.15% of Gammaproteobacteria), Shewanellaceae (3.85%) and Pseudomonadaceae (2.58%) and the genera Halomonas, Shewanella and Pseudomonas as the most common representatives (Figure 1B; SM2-Figures 5, 6). The second most abundant class within Proteobacteria was Alphaproteobacteria (39.50%), with the family Rhodobacteraceae being the most abundant within the class (33.01%), followed by Rhizobiaceae (14.81%; Figure 1C; SM2-Figures 5, 6). Within the phylum Actinobacteria, more than 70% of the ASVs belonged to the Actinobacteria class and were mainly assigned to the families Corynebacteriaceae and Micrococcaceae (more than 10 families with low prevalence < 0.05) (Figure 1D). Within the phylum Firmicutes, the most abundant ASVs were assigned to the bacterial class Bacilli (52.33%), more specifically the families Staphylococcaceae (22.41% of Bacilli) and Streptococcaceae (17.35%), represented by the genera Staphylococcus and Streptococcus, respectively (Figure 1E; SM2-Figures 5, 6). The ASVs assigned to the phylum Bacteroidetes belonged to the class Bacteroidia (96.72%), mostly represented by the families Flavobacteriaceae (34.54%), Saprospiraceae (17.11%) and Weeksellaceae (3.77%; Figure 1F; SM2-Figures 5, 6). Archaea were associated with only a few specimens of some nematode species and were mainly represented by the genus Candidatus-Nitrosopumilus, Methanobacterium (only specimens of H. disjuncta Gd3) and Methanobrevibacter (specimens of D. meyli and H. disjuncta Gd3; SM2-Figure 4).
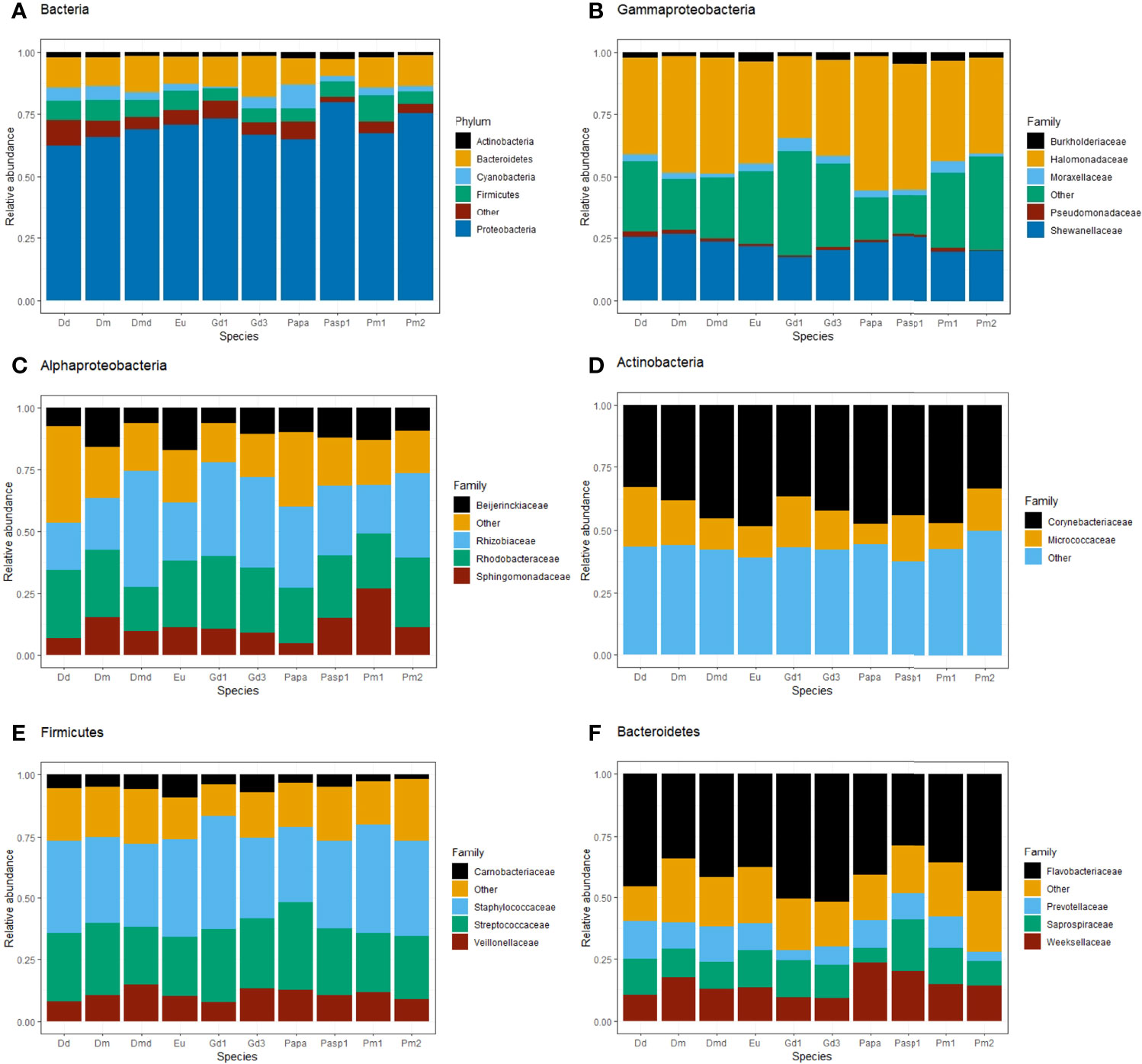
Figure 1 Relative abundance of the most abundant bacterial taxa (prevalence 0.5) of nematode microbiomes per species collected from all six microhabitats and both seasons. ASVs were assigned to phylum for all Bacteria (A) and to family level within the classes Gammaproteobacteria (B) and Alphaproteobacteria (C) and within the phyla Actinobacteria (D), Firmicutes (E) and Bacteroidetes (F). Nematode species names (see Table 1 for full names) are abbreviated as Dd, D. delyi; Dm, D. meyli; Dmd, D. cf. meyli; Eu, Eumonhystera sp.; Gd1/3, H. disjuncta Gd1/3, Papa, P. paetzoldi; Pasp1, Panagrolaimus sp.1; Pm1/2, L. marina Pm1/2. ‘Other’ refers to aggregated taxa with relative abundance < 0.005.
3.2 Microbiome Diversity of Co-Occurring Nematode Species in the Same Microhabitats
3.2.1 Comparing Nematode Microbiomes Among Their Shared Microhabitats
No significant differences were revealed in microbial diversity (Shannon-Wiener diversity) and taxon richness among co-occurring nematode species in each microhabitat, for five microhabitats collected in autumn and four collected in spring, with the only exception of microbial diversity in nematodes obtained from detached decomposing leaves of Spartina in autumn, where Eumonhystera sp. showed significantly lower Shannon diversity than D. meyli (see SM1-Table 1).
Microbiome composition, in contrast, differed significantly among co-occurring species in half of the microhabitats in both seasons (3 out of 6 microhabitats in autumn and 2 out 4 in spring) (Table 2; Figure 2; see SM1-Table 1). However, in most cases, variances were significantly heterogeneous (PERMDISP: p<0.05; Table 2; see SM1-Table 1), hence the observed differences have to be interpreted with caution. Specifically, microbial community composition was significantly different among nematode species co-occurring on decomposing F. spiralis (p = 0.013), F. vesiculosus (p = 0.009) and Ulva sp. (p = 0.003) in autumn (Table 2; Figure 2). In spring, significantly different microbiome composition was revealed for nematode species co-occurring on decomposing F. spiralis (p=0.024) and on fresh Ulva sp. (p=0.006), with significant heterogeneity of dispersions for Fucus but not for Ulva (PERMDISP: p=0.021,p=0.37 resp.; Table 2; Figure 2). The significant pairwise differences observed per microhabitat were not related to specific pairs of species and appeared unrelated to the taxonomic relatedness of the nematode species (for detailed pairwise comparisons, see SM1-Table 2). Differential abundances of microbial genera or families tested only explained 14% or 16%, respectively, of the variation of microbiomes among microhabitats for all the different nematode species together (see SM2-Figure 7).
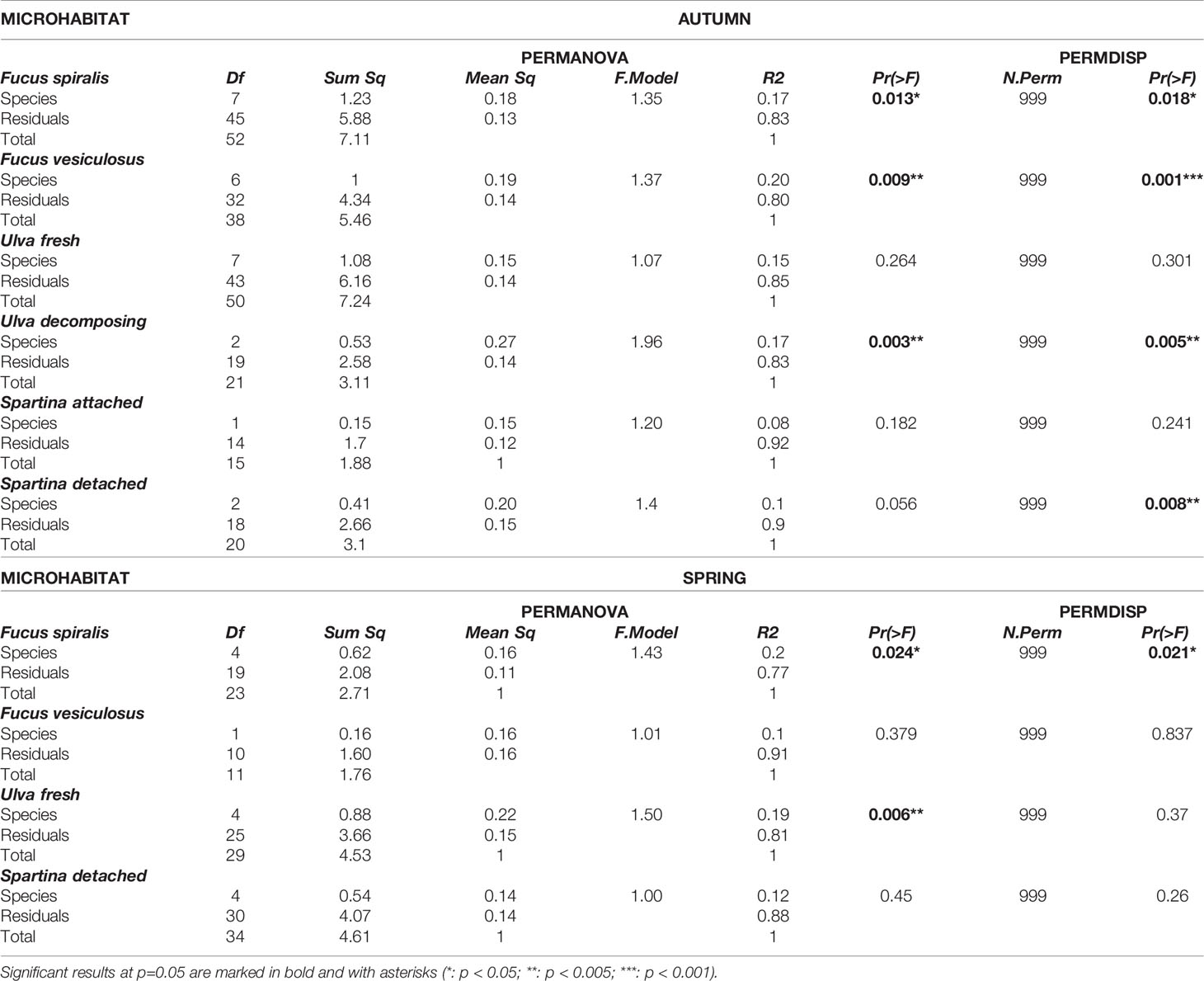
Table 2 Table of summary statistics of PERMANOVA and PERMDISP comparing microbiome composition of nematode specimens among species in each microhabitat where they co-occurred in autumn and spring.
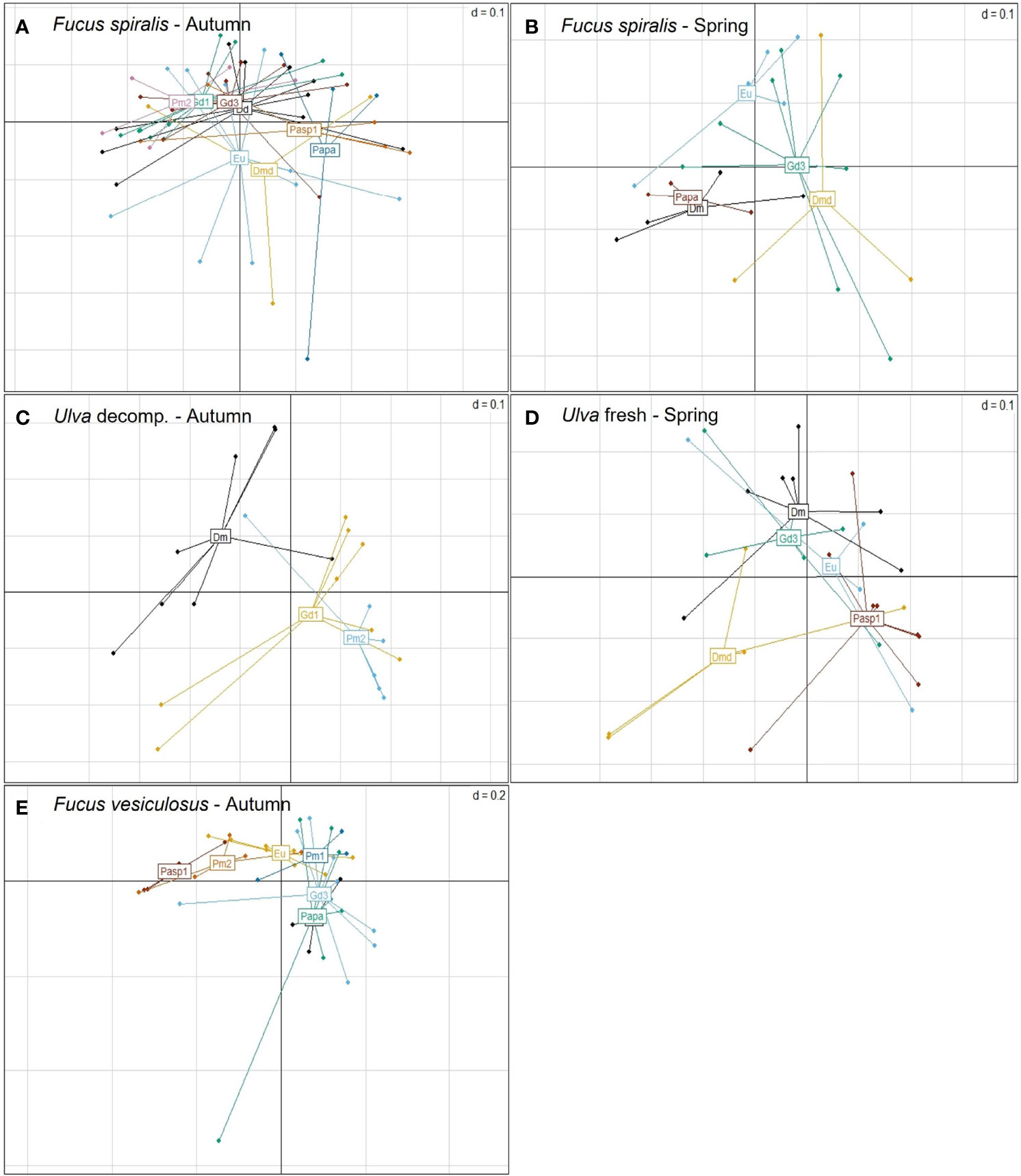
Figure 2 Principal coordinates analysis (PcoA) plots based on generalized UniFrac distances of the microbiome of single nematode specimens of co-occurring nematode species on Fucus spiralis in (A) autumn and (B) spring, on (C) decomposing Ulva in autumn and (D) fresh Ulva in spring, and on (E) Fucus vesiculosus in autumn. Nematode species are represented by different colours and abbreviated as Dd: D. delyi, Dm: D. meyli, Dmd: D. cf. meyli, Eu: Eumonhystera sp., Gd3: H. disjuncta Gd3, Papa: P. paetzoldi, Pasp1: Panagrolaimus sp.1, Pm1/Pm2: L. marina Pm1/Pm2.
3.2.2 Comparing Nematode Microbiomes Between Microhabitats in Different Decomposition Status
From the above microhabitats, two were additionally examined to compare microbiome differentiation in relation to their status of decomposition. The first subset included two monhysterid nematode species: D. meyli and H. disjuncta Gd1, and a rhabditid species: L. marina Pm2, which were encountered in the field on both fresh and decomposing Ulva sp. in autumn. The second subset included two monhysterid nematode species: D. meyli and Eumonhystera sp., which were encountered on both attached and detached Spartina leaves in autumn. No significant differences in Shannon diversity and ASV richness were revealed across different microhabitat status and species for both test cases (all ANOVAs: p>0.05; SM1-Table 3). Microbiome composition, by contrast, differed significantly between specimens collected from Ulva sp. in different decomposition status (see SM2-Figure 2) regardless of nematode species and between nematode species regardless of the decomposition status (Sp: p=0.024; St: p=0.001; Sp × St: p=0.093; PERMDISP: p>0.05; p>0.05 and p=0.003, respectively; SM1-Table 3; SM2-Figure 2A). Pairwise comparisons were only significant for two species found associated with Ulva: Pm2 vs D. meyli (SM1-Table 3). In contrast, no significant differences in microbiome composition were observed for the Spartina test case (SM1-Table 3).
3.2.3 Comparing Nematode Microbiomes Between Sampling Seasons
When looking at microbiome differences between sampling seasons, no differences were detected in Shannon diversity or microbiome composition for all species tested across microhabitats and/or sampling season (p>0.05 for all), except for one: D. cf. meyli, which exhibited a significant difference in microbiome composition as a function of the interaction between sampling season (S) and microhabitat (M) (M × S: p=0.019; M and S: p>0.05; PERMDISP: p>0.05 for all; SM1-Table 4). Significant pairwise differences were detected between seasons for specimens found on fresh Ulva (pairwise PERMANOVA: p=0.017) but not on Fucus or Spartina (p>0.05; SM1-Table 4). Moreover, significantly higher taxon richness was revealed for H. disjuncta Gd3 in autumn than in spring, regardless of the type of microhabitat (ANOVA: S: p=0.015; M and M × S: p>0.05), but no differences were detected in Shannon diversity or microbiome composition for the species across microhabitats and sampling seasons (S, M and M × S: p>0.05, PERMDISP: p>0.05 for all; SM1-Table 4).
3.2.4 Microbiome Diversity of Nematode Species Between Microhabitats
Out of 10 nematode species, only two showed significant differences in microbiome composition between the microhabitats they inhabited, i.e. L. marina Pm2 among 4 microhabitats in autumn (p=0.001; PERMDISP: p=0.043) and Panagrolaimus sp.1 between detached Spartina leaves vs fresh Ulva thalli in spring (p=0.01; PERMDISP: p=0.651; Figure 3). For L. marina Pm2, significant pairwise differences were revealed between decomposing vs fresh Ulva sp. (p=0.007) and between decomposing Ulva and the two Fucus spp. in autumn (p=0.026 and p=0.002 for F. spiralis and F. vesiculosus, resp.; Figure 3A; see SM1-Table 4). Pm2 also exhibited significant differences in taxon richness between microhabitats (ANOVA:p=0.009), more specifically between decomposing Ulva and F. vesiculosus in autumn (p=0.006; Figures 4A,B) and Panagrolaimus sp.1 exhibited significantly higher microbial diversity on Spartina than on Ulva in spring (ANOVA:p=0.025; Figures 4C,D). No significant differences in microbiome diversity and composition were detected between microhabitats for other species on either sampling season (see SM1-Table 5; SM2-Figure 3). This is likely a consequence of variances within groups being significantly heterogeneous for several species (6 out of 10 in autumn, 1 in spring), indicating a high intraspecific variability of the microbiome composition, regardless of microhabitat. This was the case for D. cf. meyli, Eumonhystera sp., H. disjuncta Gd1 and Gd3, L. marina Pm2 and Panagrolaimus sp.1 in autumn and Eumonhystera sp. in spring (PERMDISP: p<0.05 for all; SM1-Table 5). Differential abundance analysis at the microbial genus level showed significant differences between the aforementioned nematode species pairs, but overall explained only 14% of the variation among individual microbiomes for the complete dataset irrespective of the nematode taxonomic identification (named species here) and the microhabitat type (Figure 5).
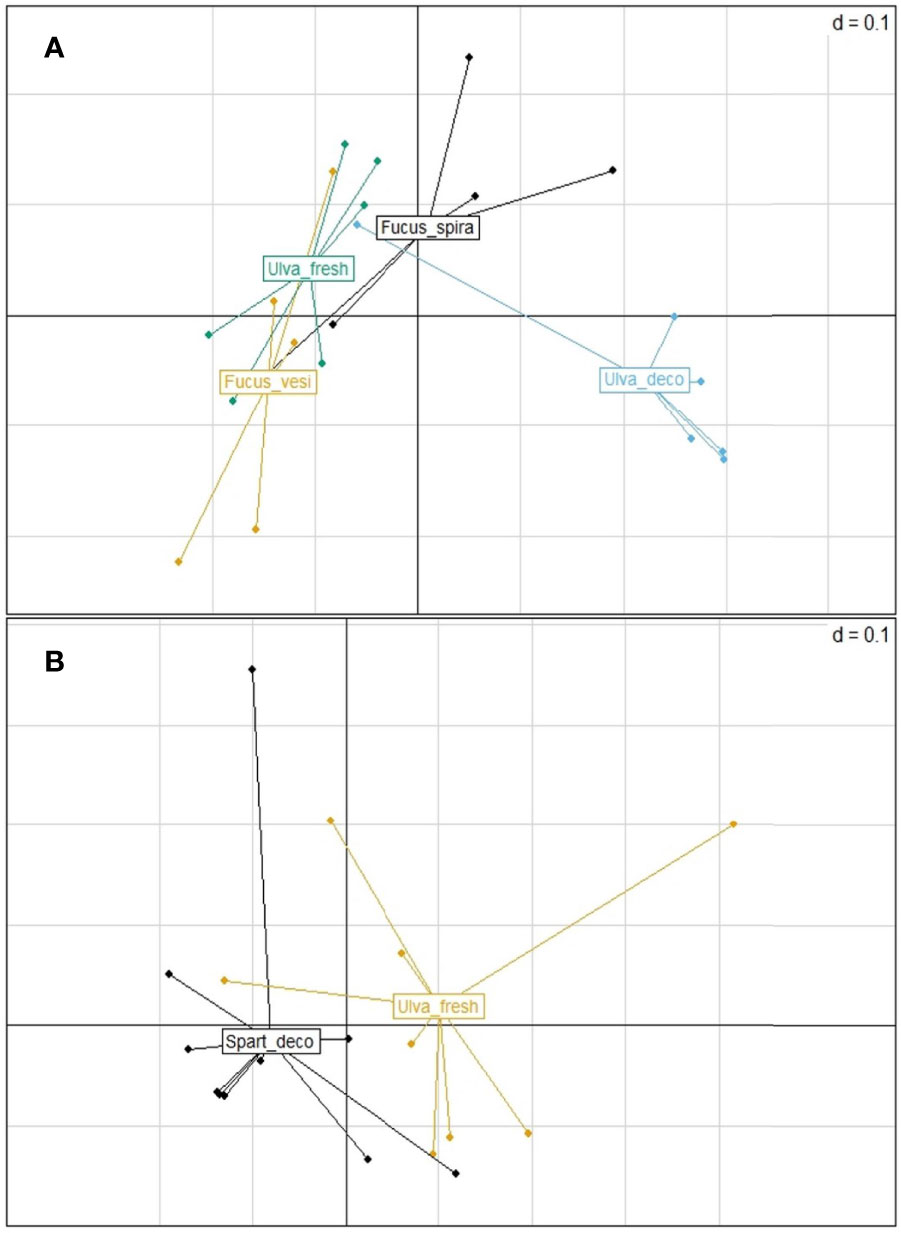
Figure 3 Principal coordinates analysis (PcoA) plots based on generalized UniFrac distances of the microbiome of (A) Litoditis marina Pm2 specimens from decomposing thalli of Fucus spiralis (in black), Fucus vesiculosus (in yellow) and from fresh and decomposing Ulva thalli (in green and in blue, resp.) in autumn and of (B) Panagrolaimus sp.1 specimens from detached Spartina leaves (in black) and fresh Ulva thalli (in yellow) in spring.
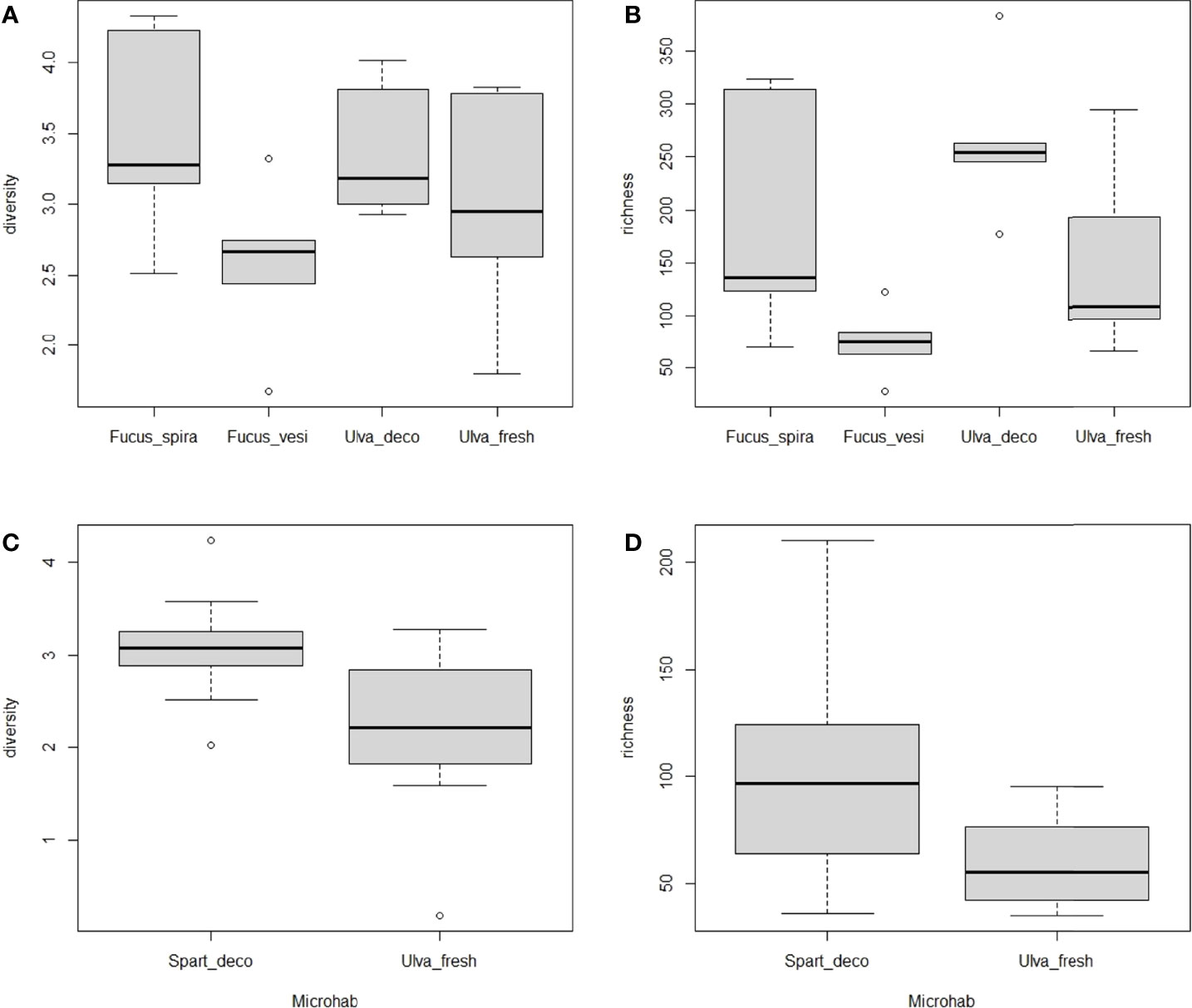
Figure 4 Box-whisker plots of alpha diversity measures (Shannon diversity index and taxon richness) for (A, B) Litoditis marina Pm2 in autumn and for (C, D) Panagrolaimus sp.1 in spring, grouped per microhabitat where the nematode species occurred (i.e. Fucus spiralis, Fucus vesiculosus, Ulva decomposing, Ulva fresh for Pm2 and detached Spartina leaves and fresh Ulva for Panagrolaimus sp).
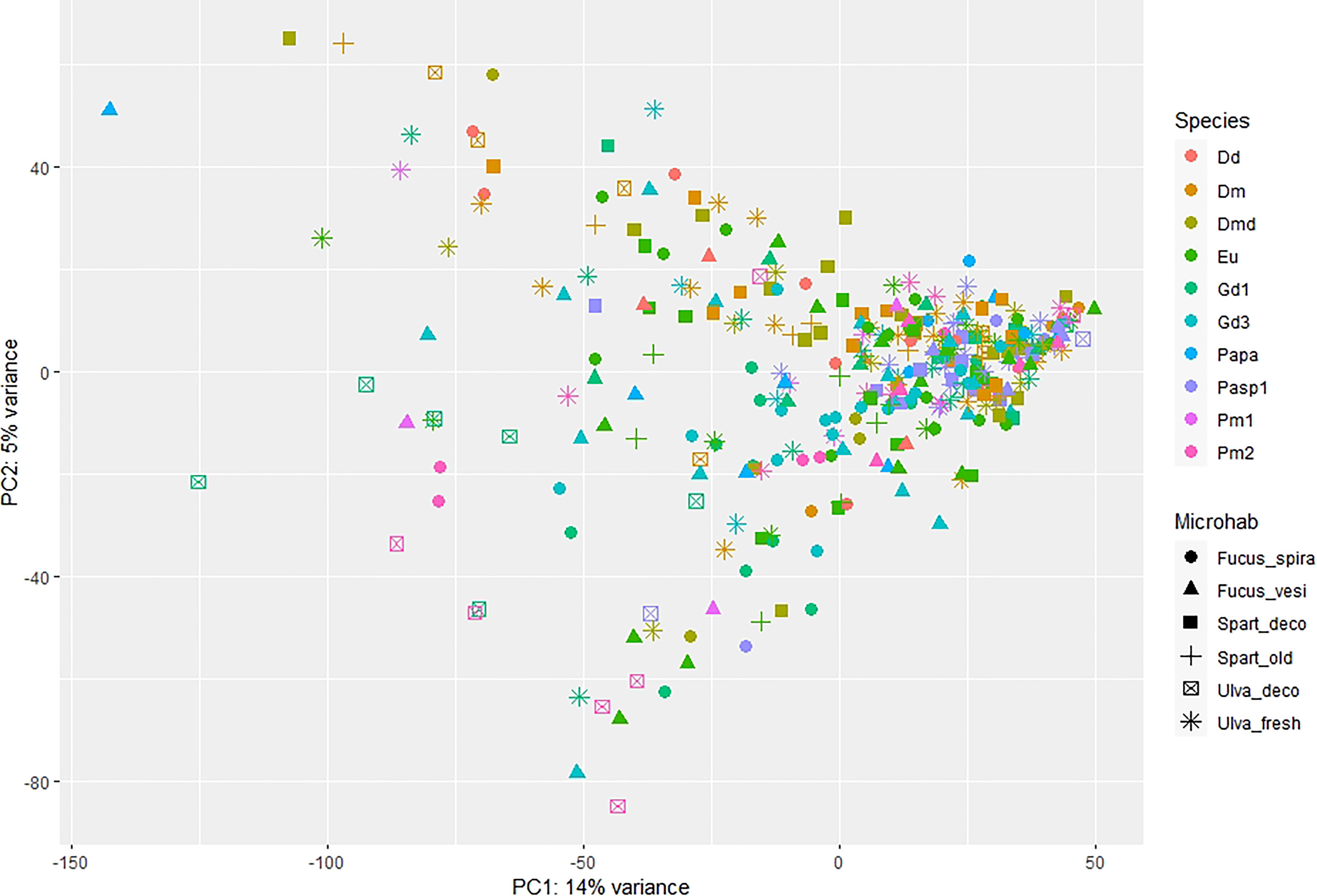
Figure 5 Principal coordinates analysis (PCA) plots based on the differential abundance data of the microbiome of the different nematode species (color) and microhabitats (shape), expressed at the taxonomically assigned genus level. Nematode species names (see Table 1 for full names) are abbreviated as Dd, D. delyi; Dm, D. meyli; Dmd, D. cf. meyli; Eu, Eumonhystera sp.; Gd1/3, H. disjuncta Gd1/3; Papa, P. paetzoldi; Pasp1, Panagrolaimus sp.1; Pm1/2, L. marina Pm1/2. Microhabitats are abbreviated as Fucus_spira, Fucus_vesi for Fucus spiralis and Fucus vesiculosus, Spart_deco, Spart_old for detached and attached Spartina leaves and Ulva_deco, Ulva_fresh for decomposing and fresh Ulva, respectively.
4 Discussion
4.1 The Microbiome of Bacterivorous Nematodes Is Highly Diverse
Our data revealed a highly diverse microbial community (13,984 ASVs were assigned to bacterial taxa) associated with intertidal nematode specimens of different taxonomical identities (average min.120 and average max. 197 ASVs were assigned per specimen across 10 nematode species) and a high number of reads (see SM2-Figure 1). The average number of raw reads per sample (84,176 reads) was high, although comparisons with other studies on microbiome of nematode individuals from marine habitats can not be directly made due to different methods (e.g. average 31,790 reads using the Illumina Miseq platform and OTUs in Schuelke et al. (2018); 5,744 reads using the 454 GS-FLX platform and OTUs in Derycke et al., 2016). Microbiomes of bacterivorous nematodes comprise not only bacteria that are part of their diet (microbiome ‘sensu lato’), but also host-associated bacteria that hold ecological interactions (e.g., commensal, symbiotic or pathogenic) with nematodes (microbiome ‘sensu stricto’) (Musat et al., 2007; Cabreiro and Gems, 2013; Derycke et al., 2016). Gut-symbiotic bacteria (i.e., gut microflora) can also be related to resource utilization of bacterivorous nematodes and can substantially contribute to their microbiomes (Baquiran et al., 2013; Cabreiro and Gems, 2013). Hence, we assume that a significant part of the microbiome of our nematode specimens are either food-derived or potentially involved in the resource utilization process, as also suggested by Derycke et al. (2016).
4.2 Microbiomes of Co-Occurring Bacterivorous Nematode Species Display Strong Overlap and a High Intraspecific Variability
Niche differentiation is a mechanism enabling local coexistence of closely related species (Chesson, 2000; Leibold and McPeek, 2006). We anticipated a degree of microbiome differentiation among species that share the same microhabitat, resulting at least in part from a differential resource use. Our results do not provide strong support for this hypothesis, as microbiomes did not show a significantly distinct composition among co-occurring species in half the number of microhabitats examined in autumn and in spring, exceptions being the two Fucus species and decomposing Ulva sp. in autumn, and F. spiralis and fresh Ulva sp. in spring.
Nevertheless, differences in microbiome composition of nematode species related with different stages of habitat decomposition were observed in our study. The state of decomposition per se means that some bacterial activity is already in place and thus slight differences in microbiomes for some nematode species could be expected. Yet, differences in nematode microbiomes related to decomposition status were only observed for species found on green macroalgae Ulva sp. Different microhabitat preferences related to different stages in the decomposition process have been suggested for closely related bacterivorous species associated with cordgrass or macroalgae. Specifically, Diplolaimelloides meyli was suggested to be associated with early-stage, and Diplolaimella dievengatensis and Diplolaimelloides oschei with later stages of the decomposition process of Spartina leaves (Moens et al., 1999). Litoditis marina is known as a particularly successful colonizer of early-stage decomposition of macroalgae (Derycke et al., 2007b) and Pm2 exhibited differences in microbiome composition between early and later decomposition stage of Ulva in our study. That was also the case for D. meyli and Halomonhystera disjuncta - Gd1, suggesting that resource divergence may be related to some extent to microhabitat partitioning.
In agreement with previous studies on L. marina and C. elegans (Derycke et al., 2016; Dirksen et al., 2016), we observed a high intraspecific variability in microbiome composition, which may provide an explanation for the lack of clear patterns of microbiome divergence among species. When interpreted as a consequence of differences in the microbiome ‘sensu lato’, this high intraspecific variability in microbiome composition may result from a substantial intraspecific competition (Svanbäck and Bolnick, 2007). Strong intraspecific competition may prevent individuals from exploiting the whole range of available resources (Svanbäck and Bolnick, 2007; Guden et al., 2021). The resulting interindividual resource specialization can expand the niche breadth of the total population (Bolnick et al., 2007; Svanbäck and Bolnick, 2007). Population niche breadth is thought to represent a balance between the diversifying effect of intraspecific competition and the constraints imposed by interspecific competition. Trade-offs between resource utilization and competitive success have been suggested to favor generalist populations with specialist individuals (Bolnick et al., 2003). From that perspective, the combination of a large interindividual microbiome variation with limited interspecific microbiome divergence may be suggestive of a general importance of intraspecific competition in the communities of bacterivorous nematodes studied here.
Species-specific nematode-microbiota associations may allow organisms to expand their resource utilization or niche. It has been suggested that putative mutualists may facilitate food digestion by nematodes through secretion of toxins, antibiotics or digestive enzymes, but they may also protect their hosts against pathogenic bacteria (Baquiran et al., 2013). Microbiome taxa may also be involved in mediating differential tolerances of their hosts to environmental conditions (Derycke et al., 2016; Dirksen et al., 2016), which can also be important for niche partitioning. For instance, bacteria of the genus Acinetobacter were found in association with Pm1 (and Pm3) by Derycke et al. (2016) and in the present study, have been suspected to be involved in abiotic tolerances of Pm1 (Derycke et al., 2016). Putative symbiotic associations of free-living marine nematodes and specific bacterial/archaeal taxa have also been found related to important ecological functions (e.g. oxidizing, nutrient cycling), which suggests that although these relationships remain largely unexplored, host-associated microbiomes can diversify species niche (Schuelke et al., 2018). Therefore, it is possible that symbionts play a role in niche partitioning of co-occurring nematodes, not only through their contribution to their host’s resource use, but also through influences on other aspects of their niche.
4.3 The Microbiome of Most Nematode Species Does Not Differ Between Microhabitats
The microbiomes of most nematode species did not differ among microhabitats, in contrast with our expectations of microbiome divergence as an indication of differential resource availability. On the contrary, each species showed a large overlap of microbiome diversity and composition across the microhabitats they occupied. There were only two (Rhabditida) nematode species in our study with clear differences in their microbiome depending on the microhabitat from which they were collected. The lack of nematode microbiome differentiation among microhabitats could be explained by two hypotheses: (a) a lack of microbiome differences associated with the microhabitats, and (b) the high intraspecific variability in microbiome composition of the nematodes. The first hypothesis is less probable as clear differences in epibiotic microbial communities associated with species and even (sub)structures of macroalgae have been reported in the literature (Lachnit et al., 2009; Lachnit et al., 2011), demonstrating algal species-specific and structure-specific microbiomes. Those microbiome differences between microhabitats, however, are not reflected in the microbiomes of the nematodes, which suggests that the nematode species studied here either utilize the same resources in different microhabitats, or that their resource use is so variable that no significant differences occur. Another study on the microbiomes of a large number of free-living marine nematode species showed a lack of habitat-microbiome correlation not only for bacterivorous nematodes but also for other functional groups (i.e. herbivores, predators, omnivores; Schuelke et al., 2018). According to their results, the structure and diversity of microbial communities associated with free-living marine nematodes from various geographic locations were not correlated with habitat type, albeit they were collected at much larger spatial scales (e.g., intertidal sediments, methane seeps, deep sea). Our data extent these results and show that also nematode species belonging to the same functional group (bacterivores) and collected from different habitats in one geographic location have microbiomes that are unlinked to (micro)habitat type. We therefore agree with the suggestion by Schuelke et al. (2018) that free-living nematodes in marine habitats may rather exhibit microbiome composition plasticity and be generalists in their feeding, which may also be the case for the bacterivorous nematodes examined in our study. Studies on entomophile nematodes have also demonstrated a lack of correlation of nematode-associated microbiomes and their respective (micro)habitats (Koneru et al., 2016; Meyer et al., 2017). By contrast, diverse microbiomes of plant-parasitic nematodes and of the well-studied soil nematode C. elegans did differ in relation to their microbial environments (Berg et al., 2016; Elhady et al., 2017). Microbiome structure was also related to habitat (and species) for freshwater and soil tardigrades and marine isopods (Mattila et al., 2014; Vecchi et al., 2018). On the other hand, the high intraspecific variation observed in microbiome composition suggests that nematodes more likely do not utilize the same resources but extend their trophic niche by individual resource specialization, which explains the lack of significant microbiome differentiation between microhabitats. Future research on nematode microbiomes, including a broader range of habitats and other feeding guilds (i.e. detritivores, predators, omnivores), would be interesting to draw clearer conclusions on the role of trophic niche specialization of nematodes for their coexistence patterns.
Although we could test temporal variability in nematode microbiomes for only four species, all belonging to the same family (Monhysteridae), there was only one observed case of temporal variability in resource use which may result in temporal niche differentiation among co-occurring species on Ulva sp. Priority effects may also play a role during colonization of macroalgae (Derycke et al., 2007b) or any substratum by competing species, which can also result in temporal differentiation in resource utilization. A pattern of temporal differentiation in microhabitat colonization has also been reported for co-occurring Litoditis marina cryptic species in the field (Guden et al., 2018).
Conclusion
Co-occurring free-living nematodes from an intertidal habitat did not show clear resource differentiation patterns among species sharing the same microhabitats, nor did single species among the different microhabitats they occupied. Contrary to our expectations, microbiomes of bacterial-feeding nematodes were not strongly species-specific nor microhabitat-specific, apart from an indication that nematode microbiomes differ in relation to the decomposition process of their phytal substratum. However, a pronounced intraspecific variability in microbiome diversity and composition was observed for the examined estuarine nematode species. We suggest that this intraspecific variability, most likely resulting from intraspecific competition, is potentially the main driver promoting coexistence of sympatric species.
Data Availability Statement
The data that support the findings of this study are publicly available online: Sanger 18S sequences at BOLD dataset [DS-MOLECOL] and GenBank [accession numbers: ON713487 - ON713781] and HTS raw reads at NIH Sequence Read Archive [BioProject “Microbiome partitioning of coexisting nematode species” - accession number: PRJNA835312].
Author Contributions
A-MV designed and performed this research, the data analyses and wrote the paper. TM and SD contributed to the design of the research and the writing of the manuscript. AR contributed to lab analyses, NM to data analyses and RG together with all co-authors revised the manuscript. TM provided funding for this research. All authors contributed to the article and approved the submitted version.
Funding
Financial support for this study was obtained from the Flemish Science Fund FWO through projects G0C3218N and 3G.0192.09, and from the Special Research Fund of Ghent University (BOF) through project 17GOA-026. The research leading to results presented in this publication was carried out with infrastructure funded by FWO (project G0H3817N) in the framework of EMBRC Belgium - international research infrastructure I001621N.
Conflict of Interest
The authors declare that the research was conducted in the absence of any commercial or financial relationships that could be construed as a potential conflict of interest.
Publisher’s Note
All claims expressed in this article are solely those of the authors and do not necessarily represent those of their affiliated organizations, or those of the publisher, the editors and the reviewers. Any product that may be evaluated in this article, or claim that may be made by its manufacturer, is not guaranteed or endorsed by the publisher.
Acknowledgments
High-throughput sequencing was carried out by Edinburgh Genomics, The University of Edinburgh. This article is based on work that was part of the Ph.D. thesis of AMV.
Supplementary Material
The Supplementary Material for this article can be found online at: https://www.frontiersin.org/articles/10.3389/fmars.2022.881566/full#supplementary-material
References
Abrams P. (1983). The Theory of Limiting Similarity. Annu. Rev. Ecol. Systematics 14, 359–376. doi: 10.1146/annurev.es.14.110183.002043
Armenteros M., Rojas-Corzo A., Ruiz-Abierno A., Derycke S., Backeljau T., Decraemer W. (2014). Systematics and DNA Barcoding of Free-Living Marine Nematodes With Emphasis on Tropical Desmodorids Using Nuclear SSU rDNA and Mitochondrial COI Sequences. Nematology 16 (8), 979–989. doi: 10.1163/15685411-00002824
Baquiran J. P., Thater B., Sedky S., De Ley P., Crowley D., Orwin P. M. (2013). Culture-Independent Investigation of the Microbiome Associated With the Nematode Acrobeloides Maximus. PLoS One 8 (7), 1–11. doi: 10.1371/journal.pone.0067425
Berg M., Stenuit B., Ho J., Wang A., Parke C., Knight M., et al. (2016). Assembly of the Caenorhabditis Elegans Gut Microbiota From Diverse Soil Microbial Environments. ISME J. 10 (8), 1998–2009. doi: 10.1038/ismej.2015.253
Blanc C., Sy M., Djigal D., Brauman A., Normand P., Villenave C. (2006). Nutrition on Bacteria by Bacterial-Feeding Nematodes and Consequences on the Structure of Soil Bacterial Community. Eur. J. Soil Biol. 42, 70–78. doi: 10.1016/j.ejsobi.2006.06.003
Blaxter M. L., De Ley P., Garey J. R., Liu L. X., Scheldeman P., Vierstraete A., et al. (1998). A Molecular Evolutionary Framework for the Phylum Nematoda. Nature 392 (6671), 71–75. doi: 10.1038/32160
Bolnick D. I., Svanback R., Araujo M. S., Persson L. (2007). Comparative Support for the Niche Variation Hypothesis That More Generalized Populations Also are More Heterogeneous. Proc. Natl. Acad. Sci. 104 (24), 10075–10079. doi: 10.1073/pnas.0703743104
Bolnick D. I., Svanbäck R., Fordyce J. A., Yang L. H., Davis J. M., Hulsey C. D., et al. (2003). The Ecology of Individuals: Incidence and Implications of Individual Specialization. Am. Nat. 161 (1), 1–28. doi: 10.1086/343878
Bongers T., Alkemade R., Yeates G. W. (1991). Interpretation of Disturbance-Induced Maturity Decrease in Marine Nematode Assemblages by Means of the Maturity Index. Mar. Ecol. Prog. Ser. 76, 135–142. doi: 10.3354/meps076135
Bruno J. F., Stachowicz J. J., Bertness M. D. (2003). Inclusion of Facilitation Into Ecological Theory. Trends Ecol. Evol. 18 (3), 119–125. doi: 10.1016/S0169-5347(02)00045-9
Cabreiro F., Gems D. (2013). Worms Need Microbes Too: Microbiota, Health and Aging in Caenorhabditis Elegans. EMBO Mol. Med. 5 (9), 1300–1310. doi: 10.1002/emmm.201100972
Callahan B. J., McMurdie P. J., Rosen M. J., Han A. W., Johnson A. J. A., Holmes S. P. (2016a). DADA2: High-Resolution Sample Inference From Illumina Amplicon Data. Nat. Methods 13 (7), 581–583. doi: 10.1038/nmeth.3869
Callahan B. J., Sankaran K., Fukuyama J. A., McMurdie P. J., Holmes S. P. (2016b). Bioconductor Workflow for Microbiome Data Analysis: From Raw Reads to Community Analyses. F1000Research 5, 1492. doi: 10.12688/f1000research.8986.2
Caporaso J. G., Lauber C. L., Walters W. A., Berg-Lyons D., Huntley J., Fierer N., et al. (2012). Ultra-High-Throughput Microbial Community Analysis on the Illumina HiSeq and MiSeq Platforms. ISME J. 6 (8), 1621–1624. doi: 10.1038/ismej.2012.8
Chase J. M., Leibold M. A. (2003). Ecological Niches: Linking Classical and Contemporary Approaches. Ed. Thompson J. N. (London: The University of Chicago Press). Ltd.
Chen J., Bittinger K., Charlson E. S., Hoffmann C., Lewis J., Wu G. D., et al. (2012). Associating Microbiome Composition With Environmental Covariates Using Generalized UniFrac Distances. Bioinformatics 28 (16), 2106–2113. doi: 10.1093/bioinformatics/bts342
Chesson P. (2000). Mechanisms of Maintenance of Species Diversity. Annu. Rev. Ecol. Systematics 31, 343–358. doi: 10.1146/annurev.ecolsys.31.1.343
De Meester N., Derycke S., Bonte D., Moens T. (2011). Salinity Effects on the Coexistence of Cryptic Species: A Case Study on Marine Nematodes. Mar. Biol. 158 (12), 2717–2726. doi: 10.1007/s00227-011-1769-5
De Meester N., Derycke S., Rigaux A., Moens T. (2015a). Active Dispersal is Differentially Affected by Inter- and Intraspecific Competition in Closely Related Nematode Species. Oikos 124 (5), 561–570. doi: 10.1111/oik.01779
De Meester N., Derycke S., Rigaux A., Moens T. (2015b). Temperature and Salinity Induce Differential Responses in Life Histories of Cryptic Nematode Species. J. Exp. Mar. Biol. Ecol. 472, 54–62. doi: 10.1016/j.jembe.2015.07.002
De Meester N., Gingold R., Rigaux A., Derycke S., Moens T. (2016). Cryptic Diversity and Ecosystem Functioning: A Complex Tale of Differential Effects on Decomposition. Oecologia 182 (2), 1–13. doi: 10.1007/s00442-016-3677-3
De Mesel I., Derycke S., Swings J., Vincx M., Moens T. (2006). Role of Nematodes in Decomposition Processes: Does Within-Trophic Group Diversity Matter? Mar. Ecol. Prog. Ser. 321, 157–166. doi: 10.3354/meps321157
Derycke S., Backeljau T., Moens T. (2013). Dispersal and Gene Flow in Free-Living Marine Nematodes. Front. Zool. 10 (1), 1. doi: 10.1186/1742-9994-10-1
Derycke S., Backeljau T., Vlaeminck C., Vierstraete A., Vanfleteren J., Vincx M., et al. (2007a). Spatiotemporal Analysis of Population Genetic Structure in Geomonhystera Disjuncta (Nematoda, Monhysteridae) Reveals High Levels of Molecular Diversity. Mar. Biol. 151 (5), 1799–1812. doi: 10.1007/s00227-007-0609-0
Derycke S., De Meester N., Rigaux A., Creer S., Bik H., Thomas W., et al. (2016). Coexisting Cryptic Species of the Litoditis Marina Complex (Nematoda) Show Differential Resource Use and Have Distinct Microbiomes With High Intraspecific Variability. Mol. Ecol. 25, 2093–2110. doi: 10.1111/mec.13597
Derycke S., Remerie T., Backeljau T., Vierstraete A., Vanfleteren J., Vincx M., et al. (2008). Phylogeography of the Rhabditis (Pellioditis) Marina Species Complex: Evidence for Long-Distance Dispersal, and for Range Expansions and Restricted Gene Flow in the Northeast Atlantic. Mol. Ecol. 17 (14), 3306–3322. doi: 10.1111/j.1365-294X.2008.03846.x
Derycke S., Remerie T., Vierstraete A., Backeljau T., Vanfleteren J. R., Vincx M., et al. (2005). Mitochondrial DNA Variation and Cryptic Speciation Within the Free-Living Marine Nematode Pellioditis Marina. Mar. Ecol. Prog. Ser. 300, 91–103. doi: 10.3354/meps300091
Derycke S., Backeljau T., Vlaeminck C., Vierstraete A., Vanfleteren J., Vincx M., et al (2006). Seasonal Dynamics of Population Genetic Structure in Cryptic Taxa of The Pellioditis Marina Complex (Nematoda: Rhabditida). Genetica 128 (1), 307–321. doi: 10.1007/s10709-006-6944-0
Derycke S., Sheibani Tezerji R., Rigaux A., Moens T. (2012). Investigating the Ecology and Evolution of Cryptic Marine Nematode Species Through Quantitative Real-Time PCR of the Ribosomal ITS Region. Mol. Ecol. Resour. 12 (4), 607–619. doi: 10.1111/j.1755-0998.2012.03128.x
Derycke S., Van Vynckt R., Vanaverbeke J., Vincx M., Moens T. (2007b). Colonisation Patterns of Nematoda on Decomposing Algae in the Estuarine Environment: Community Build Up and Genetic Structure of the Dominant Species Pellioditis Marina. Limnol. Oceanog. 52, 992–1001. doi: 10.4319/lo.2007.52.3.0992
Dirksen P., Marsh S. A., Braker I., Heitland N., Wagner S., Nakad R., et al. (2016). The Native Microbiome of the Nematode Caenorhabditis Elegans: Gateway to a New Host-Microbiome Model. BMC Biol. 14 (1), 1–16. doi: 10.1186/s12915-016-0258-1
dos Santos G. A. P., Derycke S., Genevois V. G. F., Coelho L. C. B. B., Correia M. T. S., Moens T. (2009). Interactions Among Bacterial-Feeding Nematode Species at Different Levels of Food Availability. Mar. Biol. 156 (4), 629–640. doi: 10.1007/s00227-008-1114-9
dos Santos G. A. P., Moens T. (2011). Populations of Two Prey Nematodes and Their Interaction are Controlled by a Predatory Nematode. Mar. Ecol. Prog. Ser. 427, 117–131. doi: 10.3354/meps08991
Dray S., Dufour A. B. (2007). The Ade4 Package: Implementing the Duality Diagram for Ecologists. J. Stat. Software 22 (4), 1–20. doi: 10.18637/jss.v022.i04
Ekschmitt K., Griffiths B. S. (1998). Soil Biodiversity and its Implications for Ecosystem Functioning in a Heterogeneous and Variable Environment. Appl. Soil Ecol. 10, 201–215. doi: 10.1016/S0929-1393(98)00119-X
Elhady A., Giné A., Topalovic O., Jacquiod S., Sørensen S. J., Sorribas F. J., et al. (2017). Microbiomes Associated With Infective Stages of Root-Knot and Lesion Nematodes in Soil. PLoS One 12 (5), e0177145. doi: 10.1371/journal.pone.0177145
Estifanos T. K., Traunspurger W., Peters L. (2013). Selective Feeding in Nematodes: A Stable Isotope Analysis of Bacteria and Algae as Food Sources for Free-Living Nematodes. Nematology 15 (1), 1–13. doi: 10.1163/156854112X639900
Gilbert J. A., Jansson J. K., Knight R. (2014). The Earth Microbiome Project: Successes and Aspirations. BMC Biol. 12 (1), 1–4. doi: 10.1186/s12915-014-0069-1
Guden R. M., Derycke S., Moens T. (2021). A Multi-Faceted Approach to Understand How Resource Diversity can Mediate the Coexistence of Cryptic Marine Nematode Species. Front. Mar. Sci. 1858. doi: 10.3389/fmars.2021.777425
Guden R. M., Vafeiadou A. M., De Meester N., Derycke S., Moens T. (2018). Living Apart-Together: Microhabitat Differentiation of Cryptic Nematode Species in a Saltmarsh Habitat. PLoS One 13 (9), e0204750. doi: 10.1371/journal.pone.0204750
Hardin G. (1960). The Competitive Exclusion Principle. Science 131, 1292–1297. doi: 10.1126/science.131.3409.1292
Hector A., Hooper R. (2002). Darwin and the First Ecological Experiment. Science 295, 639–640. doi: 10.1126/science.1064815
Heip C., Vincx M., Vranken G. (1985). The Ecology of Marine Nematodes. Oceanography Mar. Biol.: an Annu. Rev. 23, 399–489.
Hubbell S. P. (2001). The Unified Neutral Theory of Biodiversity and Biogeography (Volume 17) (Princeton, New Jersey, USA: Princeton University Press).
Hutchinson G. E. (1959). Homage to Santa Rosalia or Why are There So Many Kinds of Animals? Am. Nat. 93 (870), 145–159. doi: 10.1086/282070
Koneru S. L., Salinas H., Flores G. E., Hong R. L. (2016). The Bacterial Community of Entomophilic Nematodes and Host Beetles. Mol. Ecol. 25 (10), 2312–2324. doi: 10.1111/mec.13614
Lachnit T., Blümel M., Imhoff J. F., Wahl M. (2009). Specific Epibacterial Communities on Macroalgae: Phylogeny Matters More Than Habitat. Aquat. Biol. 5 (2), 181–186. doi: 10.3354/ab00149
Lachnit T., Meske D., Wahl M., Harder T., Schmitz R. (2011). Epibacterial Community Patterns on Marine Macroalgae are Host-Specific But Temporally Variable. Environ. Microbiol. 13 (3), 655–665. doi: 10.1111/j.1462-2920.2010.02371.x
Leibold M. A., McPeek M. A. (2006). Coexistence of the Niche and Neutral Perspectives in Community Ecology. Ecology 87 (6), 1399–1410. doi: 10.1890/0012-9658(2006)87[1399:cotnan]2.0.co;2
Love M. I., Huber W., Anders S. (2014). Moderated Estimation of Fold Change and Dispersion for RNA-Seq Data With Deseq2. Genome Biol. 15,550. doi: 10.1186/s13059-014-0550-8
Mattila J. M., Zimmer M., Vesakoski O., Jormalainen V. (2014). Habitat-Specific Gut Microbiota of the Marine Herbivore Idotea Balthica (Isopoda). J. Exp. Mar. Biol. Ecol. 455, 22–28. doi: 10.1016/j.jembe.2014.02.010
McMurdie P. J., Holmes S. (2013). Phyloseq: An R Package for Reproducible Interactive Analysis and Graphics of Microbiome Census Data. PLoS One 8 (4), e61217. doi: 10.1371/journal.pone.0061217
Meyer J. M., Baskaran P., Quast C., Susoy V., Rödelsperger C., Glöckner F. O., et al. (2017). Succession and Dynamics of Pristionchus Nematodes and Their Microbiome During Decomposition of Oryctes Borbonicus on La Réunion Island. Environ. Microbiol. 19 (4), 1476–1489. doi: 10.1111/1462-2920.13697
Moens T, Braeckman U, Derycke S, Fonseca G, Gallucci F, Gingold R, et al (2013). Ecology of Free-Living Marine Nematodes. Nematoda 2, 109–152.
Moens T., Verbeeck L., De Maeyer A., Swings J., Vincx M. (1999). Selective Attraction of Marine Bacterivorous Nematodes to Their Bacterial Food. Mar. Ecol. Prog. Ser. 176, 165–178. doi: 10.3354/meps176165
Monteiro L. C., Van Butsel J., De Meester N., Traunspurger W., Derycke S., Moens T. (2018). Differential Heavy-Metal Sensitivity in Two Cryptic Species of the Marine Nematode Litoditis Marina as Revealed by Developmental and Behavioural Assays. J. Exp. Mar. Biol. Ecol. 502, 203–210. doi: 10.1016/j.jembe.2017.05.016
Musat N., Giere O., Gieseke A., Thiermann F., Amann R., Dubilier N. (2007). Molecular and Morphological Characterization of the Association Between Bacterial Endosymbionts and the Marine Nematode Astomonema Sp. From the Bahamas: Brief Report. Environ. Microbiol. 9 (5), 1345–1353. doi: 10.1111/j.1462-2920.2006.01232.x
Oksanen J., Guillaume Blanchet F., Friendly M., Kindt R., Legendre P., McGlinn D. (2017). Vegan: Community Ecology Package. R Package Version 2.4–3, https://CRAN. Rproject. org/package=vegan.
R Core Team (2020). R: A Language and Environment for Statistical Computing (Vienna, Austria: R Foundation for Statistical Computing). Available at: https://www.R-project.org/.
Ristau K., Steinfartz S., Traunspurger W. (2013). First Evidence of Cryptic Species Diversity and Significant Population Structure in a Widespread Freshwater Nematode Morphospecies (Tobrilus Gracilis). Mol. Ecol. 22 (17), 4562–4575. doi: 10.1111/mec.12414
Rzeznik-Orignac J., Puisay A., Derelle E., Peru E., Le Bris N., Galand P. E. (2018). Co-Occurring Nematodes and Bacteria in Submarine Canyon Sediments. PeerJ 6, e5396. doi: 10.7717/peerj.5396
Schuelke T., Pereira T. J., Hardy S. M., Bik H. M. (2018). Nematode-Associated Microbial Taxa do Not Correlate With Host Phylogeny, Geographic Region or Feeding Morphology in Marine Sediment Habitats. Mol. Ecol. 27 (8), 1930–1951. doi: 10.1111/mec.14539
Schulenburg H., Félix M.-A. (2017). The Natural Biotic Environment of Caenorhabditis Elegans. Genetics 206 (1), 55–86. doi: 10.1534/genetics.116.195511
Svanbäck R., Bolnick D. I. (2007). Intraspecific Competition Drives Increased Resource Use Diversity Within a Natural Population. Proc. R. Soc. B: Biol. Sci. 274 (1611), 839–844. doi: 10.1098/rspb.2006.0198
Traunspurger W. (2000). The Biology and Ecology of Lotic Nematodes. Freshw. Biol. 44 (1), 29–45. doi: 10.1371/journal.pone.0111889
Van Campenhout J., Derycke S., Moens T., Vanreusel L. (2014). Differences in Life-Histories Refute Ecological Equivalence of Cryptic Species and Provide Clues to the Origin of Bathyal Halomonhystera (Nematoda). PLoS one 9 (11), e111889. doi: 10.1371/journal.pone.0111889
Vecchi M., Newton I. L. G., Cesari M., Rebecchi L., Guidetti R. (2018). The Microbial Community of Tardigrades: Environmental Influence and Species Specificity of Microbiome Structure and Composition. Microbial Ecol. 76 (2), 467–481. doi: 10.1007/s00248-017-1134-4
Venette R. C., Ferris H. (1998). Influence of Bacterial Type and Density on Population Growth of Bacterial-Feeding Nematodes. Soil Biol. Biochem. 30 (7), 949–960. doi: 10.1016/S0038-0717(97)00176-4
Wang Q., Garrity G. M., Tiedje J. M., Cole J. R. (2007). Naive Bayesian Classifier for Rapid Assignment of rRNA Sequences Into the New Bacterial Taxonomy. Appl. Environ. Microbiol. 73 (16), 5261–5267. doi: 10.1128/AEM.00062-07
Wickham H. (2016). Ggplot2: Elegant Graphics for Data Analysis (New York:Springer-Verlag New York). Available at: https://ggplot2.tidyverse.org.
Keywords: coexistence, resource partitioning, free-living nematodes, next-generation sequencing, niche differentiation, microhabitats
Citation: Vafeiadou A-M, Derycke S, Rigaux A, De Meester N, Guden RM and Moens T (2022) Microbiome Differentiation Among Coexisting Nematode Species in Estuarine Microhabitats: A Metagenetic Analysis. Front. Mar. Sci. 9:881566. doi: 10.3389/fmars.2022.881566
Received: 22 February 2022; Accepted: 19 May 2022;
Published: 30 June 2022.
Edited by:
Francisco J. A. Nascimento, Stockholm University, SwedenReviewed by:
Tiago Jose Pereira, University of Georgia, United StatesVera G. Fonseca, Centre for Environment, Fisheries and Aquaculture Science (CEFAS), United Kingdom
Copyright © 2022 Vafeiadou, Derycke, Rigaux, De Meester, Guden and Moens. This is an open-access article distributed under the terms of the Creative Commons Attribution License (CC BY). The use, distribution or reproduction in other forums is permitted, provided the original author(s) and the copyright owner(s) are credited and that the original publication in this journal is cited, in accordance with accepted academic practice. No use, distribution or reproduction is permitted which does not comply with these terms.
*Correspondence: Anna-Maria Vafeiadou, YW5uYW1hcmlhLnZhZmVpYWRvdUB1Z2VudC5iZQ==