- 1Laboratory of Enzymology, Institute of Biological Sciences, Department of Cell Biology, University of Brasília, Brasília, Brazil
- 2Aquatic Microbial Ecology, Environmental Microbiology and Biotechnology, Department of Chemistry, University of Duisburg-Essen, Essen, Germany
- 3Genetics and Biotechnology Laboratory, Embrapa-Agroenergy, Brasília, Brazil
- 4Centre of Water and Environmental Research (ZWU), University of Duisburg-Essen, Essen, Germany
- 5Department of Genetics, Institute of Biology, Federal University of Rio de Janeiro, Rio de Janeiro, Brazil
Symbiont relationships between corals and photosynthetic microorganisms sustain coral reef existence. However, the Great Amazon Reef System (GARS) stays under a plume layer that attenuates the entry of light, and instead of corals, sponges are the major reef epifauna, for which little is known about the function of the associated microbiome. Here, we used genome-resolved metagenomics to investigate how the sponge microbiome supports its host and overcomes the reduced light availability, recovering 205 MAGs from Agelas and Geodia sponges with completeness >70% and contamination <10%. Beta diversity estimates based on the 16S rRNA genes indicated the microbiomes of Amazon and Caribbean sponges to be distinct (P<0.01), with heterotrophic lifestyles being prevalent in Amazon sponge microbiomes (P<0.05). Nevertheless, genes indicating the carbon fixation pathways 3-Hydroxypropionate/4-Hydroxybutyrate cycle, 3-Hydroxypropionate bicycle, Reductive Tricarboxylic Acid Cycle, and Calvin-Benson-Bassham cycle could be recovered in low abundance. The presence of Cyanobacteria, represented by both 16S rRNA analyses and low-quality MAGs indicated light incidence on the reef. The metabolic profile shows that the GARS sponge microbiome had genes for sulfate reduction, sulfur oxidation, nitric oxide reduction, ammonia oxidation, nitrate reduction, nitrite ammonification, nitrite oxidation, and nitrite reduction, indicating that the microbiome might play a role in detoxification of the holobiont. We conclude, that neither the plume-limited photosynthesis of the sponge microbiome nor the primary producers sustain the organic carbon input for the sponges, which likely live off plume-associated organic carbon and their heterotrophic microbiota.
Introduction
Coral reefs are one of the most diverse ecosystems on the planet (Connell, 1978). Scleractinian corals that secrete calcium carbonate layers through the epidermis of the lower half of the column and the pedal disc are principal reef-builders (Drake et al., 2020). Other organisms such as coralline algae and hexactinellid sponges can contribute to reef formation when they leave their silica and carbonate skeletons. Despite occupying about 0.2% of the seafloor, coral reefs are responsible for sheltering and feeding at least one quarter of marine life (Knowlton and Jackson, 2001).
The coral food source comes from zooxanthellae, microscopic algae that live inside of it in a symbiotic relationship, and supply 90% of the coral’s nutritional needs. Corals prefer shallow, warm, and oligotrophic waters to fix carbon by oxygenic photosynthesis using Calvin-Benson-Bassham (CBB) cycle (Stanley Jr, 2006). Muddy waters of large tropical rivers often inhibit the formation of coral reefs as sediment covers them and consequently decrease the light availability for the zooxanthella, compromising its source of nutrients. Despite the fact that tropical rivers exhibit these features, some biogenic reefs can be found at the Amazon River mouth.
Recently, scientists recognized a new biogenic reef system of 56,000 km2 in the Amazon River mouth close to offshore oil companies (i.e., BHP-Billiton, Queiroz Galvão, Ecopetrol, Total, BP, and Petrobras) - the Great Amazon Reef System (GARS) (Moura et al., 2016). A mud layer called “plume”, formed by organic and inorganic suspended material that spreads across the Atlantic Ocean, influences the formation of this reef. Instead of corals, coralline algae built the GARS foundation with small patches, platforms, and walls covered by scleractinians, sponges, octocorals, and black corals. Sponges that are adapted to this environment are the major reef epifauna. A total of 61 sponge taxa were identified in this ecosystem, with the most commonly found being Agelas spp., Aplysina spp., Callyspongia vaginalis, Clathria nicolea, Monanchora arbuscula, Oceanapia bartschi, and Geodia spp. (Moura et al., 2016). Sponges are known to filter water by choanocytes cells whose flagellar movement creates a water flux into their pores to capture dissolved organic matter (DOM), digest it (i.e., phagocytosis), and release particulate organic matter (POM) to higher trophic levels via the sponge loop (de Goeij et al., 2013).
Sponges have co-evolved with microbes for over 800 million years (Turner, 2021). The sponges’ microbiota can represent approximately 35% of the holobiont biomass (Webster and Thomas, 2016). More than 47 sponge-inhabiting phyla were described, some of which were found exclusively in these invertebrates (Reveillaud et al., 2014). Their microbiota provides the holobionts the capacity to fix carbon, metabolize nitrogen, sulfur, and phosphate (Pita et al., 2018). Moreover, sessile organisms such as sponges rely on chemical compounds produced by their microbiota to defend against predators, competitors, and invading organisms such as bacteria, viruses, and microeukaryotes. In many cases, compounds isolated from sponge’s microbiota are used as drug precursors and bioactive molecules in the pharmaceutical industry - being the most promising source for new bioactive products in the marine environment (Blunt et al., 2017). However, linking a specific microorganism from the sponge microbiota to a specific metabolic function and taxonomic group is a challenge, since many microorganisms are hard to cultivate in the laboratory, due to unknown growth requirements (Lewis and Ettema, 2019). Culture-independent techniques such as genome-resolved metagenomics allow reconstruction of draft genomes from the environment, enabling metabolic inferences for microorganisms that are little known or do not have yet cultivated representatives (Tyson et al., 2004).
In this context, the GARS sponge microbiome study provides insights into sponge microbiota’s role and provides information for ecological surveillance, and prospecting focused on biotechnological research. In this work, we used metagenome-assembled genomes (MAGs) to investigate the potential metabolic contribution of the sponges microbiome (Hügler and Sievert, 2011) to sustain this holobiont in a reduced-light environment.
Materials and Methods
Sample Collection, DNA Extraction, and Sequencing
Sponges were collected at the Amazon River mouth on September 27, 2014, latitude 1°17’59.3”N and longitude 46°46’43.9”W. The selected sponges were Agelas dispar, Agelas clathrodes, Agelas clathrodes, Geodia cf. corticostylifera, Geodia neptuni, and Geodia sp. The criterion used for sponge selection was the availability of least three individuals per genus. The collected material was placed in plastic-containers holding 20 L of seawater at approximately 24°C and transported to the laboratory. In the laboratory, sponges were transferred to a container with 250 mL of sterile seawater and left for 5 to 10 minutes to remove microorganisms from the water not associated with the sponges. The sponge tissue was then dried and dissected with a scalpel, carefully removing associated macroscopic organisms. Approximately 1 g of sponge tissue was frozen in liquid nitrogen and powdered. DNA was extracted from the powder obtained and purified using 4 M guanidine hydrochloride, 50 mM Tris-HCl pH 8.0, 0.05 M EDTA, 0.5% sodium N-lauroylsarcosine and 1% β-mercaptoethanol, followed by a phenol/chloroform step, precipitation by isopropanol, and resuspension in 50 µL of ultrapure water (Trindade-Silva et al., 2012).
Sponge-microbiome holobiont libraries were constructed for each individual sample using the TruSeq Nano DNA kit, and sequenced with Novaseq system (Illumina®) at 20 GB sequencing depth with 150 bp paired-end reads. Reads were trimmed using Sickle (https://github.com/najoshi/sickle; version 1.33) with default parameters; BBtools (https://sourceforge.net/projects/bbmap/; version 35) was to check and if present remove Illumina adaptors and phiX sequences. Each metagenome was assembled individually using MetaSpades version 3.1.3 (Nurk et al., 2017), and scaffolds smaller than 1 kb were removed.
16S Ribosomal RNA Taxonomic Composition and Beta Diversity
Metaxa2 extracted the ribosomal 16S RNA (16S rRNA) sequences from reads of the sponge-microbiome holobiont metagenomes, and compared it to the SSU default database (Bengtsson-Palme et al., 2015). We compared our data with the 16S rRNA (i.e., amplicon) microbiome associated with the sponges Agelas (SRR7068155, SRR7068157, SRR7068159, SRR7068173, SRR7068176, and SRR7068181) and Geodia (SRS9041395, SRS9041405, SRS9041416, SRS9041427, SRS9041573, and SRS9041575) from the Caribbean region (Indraningrat et al., 2019) (Supplementary Data Set S1). The Amazon plume water samples (i.e., whole metagenome sequencing) were also used for comparison (NCBI Bio-Project number PRJNA796108) (Silva et al., 2017). Those sequences were classified using Metaxa2 as described above.
To infer the influences of the Amazon plume water on the sponge microbiome, 16S rRNA classification data were normalized to their respective sequencing depth (i.e., relative abundance) and root-square transformed using the Hellinger method in the “decostand” function with R (R Core Team, 2021) (https://www.r-project.org/; version 4.1.2) package vegan (Oksanen et al., 2013). Shannon index was calculated using function “diversity”, and Bray-Curtis dissimilarities were calculated using the “vegdist” function and their first two Principal Coordinates were plotted (PCoA). To test for the presence of statistically significant differences between microbiomes associated with Amazon sponges, Caribbean sponges, and the Amazon Plume water, we used Permutational Multivariate Analysis of Variance (PERMANOVA) with the “adonis2” command in the vegan package using 10,000 permutations and α = 0.05. Kruskal-Wallis tested significant differences between three or more groups (i.e., Shannon index, MW-score functions), Wilcoxon tested for two groups comparisons (i.e., taxa differences between GARS and Caribbean microbiome), p-value adjusted with Benjamini-Hochberg method. We construct a Venn diagram using the venn package in R software (https://github.com/dusadrian/venn).
Metagenome Assemblies, Binning, and Analyses
We used MaxBin2 v2.2.4 with 40 and 107 markers (Wu et al., 2016) to recover metagenome assembled-genomes (MAGs). MAGs were aggregated using DASTOOL v1.1.2 (Sieber et al., 2018) and manually curated based on GC content, coverage and taxonomy using uBin v0.9.14 (Bornemann et al., 2020). MAGs were dereplicated with dRep v.3.2.2 (Olm et al., 2017), quality was checked with CheckM v1.0.13 (Parks et al., 2015), and coverage was calculated using CoverM v0.6.1 (https://github.com/wwood/CoverM).
MAGs with completeness >70% and contamination <10% were considered high-quality and used for further analysis (Bornemann et al., 2022). METABOLIC v4.0 (METabolic And BiogeOchemistry anaLyses In miCrobes) (Zhou et al., 2022) annotated and profiled metabolic traits, and assigned taxonomy to the selected MAGs. MW-score (metabolic weight score) was used to measure autotrophic and heterotrophic metabolism, as well as nitrogen and sulfur cycle functions on high-quality MAGs. MW-score is calculated by summing up all the coverage values of genes belonging to the function, and subsequently normalizing it to overall gene coverage.
METABOLIC determines carbon fixation pathways completeness by checking for the presence/absence of KEGG Orthology (KO) using hidden Markov model (HMM) profiles on KEGG modules. For dicarboxylate/4-hydroxybutyrate (DC/4-HB) cycles, Wood-Ljungdahl (WL) pathway, and reductive tricarboxylic acid (rTCA) pathways, we used METABOLIC 75% default cutoff. For 3-Hydroxypropionate/4-hydroxybutyrate (3HP/4HB), 3-Hydroxypropionate bicycle (3HP), and CBB we used custom cutoffs. Hmmsearch (https://hmmer.org/; HMMER 3.1b2) comparison with genomes that participate in each carbon fixation pathway determined custom E-values cutoff. We searched for the missing genes using hmmsearch on Hmm profiles described above with a less restricted cutoff. We defined 36% pathway completeness and key-enzyme 4-hydroxybutyryl-CoA dehydratase/vinylacetyl-CoA-Delta-isomerase (K14534) for 3HP/4HB. This choice was based on the comparison of 3HP/4HB genes with some high-quality Thermoproteota MAGs (>95% completeness and no contamination) and Nitrosopumilus maritimus (GCF_000018465.1), which is well known to participate in this pathway. For 3HP, we used 58% pathway completeness. For CBB, we selected MAGs with 70% pathway completeness and searched for the key-enzyme Rubisco using hmmsearch with a less restrictive cutoff (E-value, 1.3E-117) to classify the Rubisco type.
High-quality MAGs were assigned to a taxonomic group using GTDB-Tk database version 202 (Parks et al., 2018) on METABOLIC workflow. GTDB-Tk concatenates a set of 120 bacterial and 122 archaeal ubiquitous single-copy markers to build the phylogeny (Parks et al., 2017). We removed taxonomically distant reference genomes to improve visualization. Protein alignments were performed using MAFFT v7.405 (Katoh, 2002) with default parameters. A phylogenetic tree was obtained using FastTree v2.1.11 (Price et al., 2010), and visualized with Geneious Prime 2022.0.1 (https://www.geneious.com). We rooted the tree using the following genomes: for Archaea phylogeny Methanosarcina acetivorans (RS_GCF_000007345.1) was used, while for bacteria phylogeny we used Mycoplasma genitalium (RS_GCF_000027325.1). Genomic data (draft genomes and metagenomic reads) used in this study are deposited at NCBI under Bio-Project number PRJNA795684.
Results
The Plume Water Contributes to a Unique and High Diversity GARS Sponge Microbiome
A total of 1.26 billion fastq sponge microbiome reads were recovered. These belonged to Agelas dispar (16.27% of total), Agelas clathrodes (18.02% of total), Agelas clathrodes (17.06% of total), Geodia cf. corticostylifera (14.84% of total), Geodia neptuni (13.81% of total), and Geodia sp. (20% of total).
Based on 16S rRNA composition analysis (Figure 1), the GARS microbiomes of Agelas and Geodia sponges showed little differences at phylum level, and a high abundance of unclassified bacteria (25.8 ± 2.90%). Relative abundance shows that Proteobacteria (30.3 ± 5.1%) was the most abundant taxon in all samples. The dominant classes in the Proteobacteriota superphylum were Gammaproteobacteria (14.38 ± 2.79%), Alphaproteobacteria (7.46 ± 2.39%) and Deltaproteobacteria (4.54 ± 0.84%). Betaproteobacteria was also detected, but in fewer proportion. The other most abundant phyla were Firmicutes (16.5 ± 4.3%), Actinobacteriota (8.8 ± 1.2%), Chloroflexota (4.7 ± 0.9%), Acidobacteriota (4.0 ± 1.3%), Thermoproteota (3.9 ± 1.7%), Nitrospirota (2.1 ± 0.5%), and Spirochaetota (1.7 ± 0.7%).
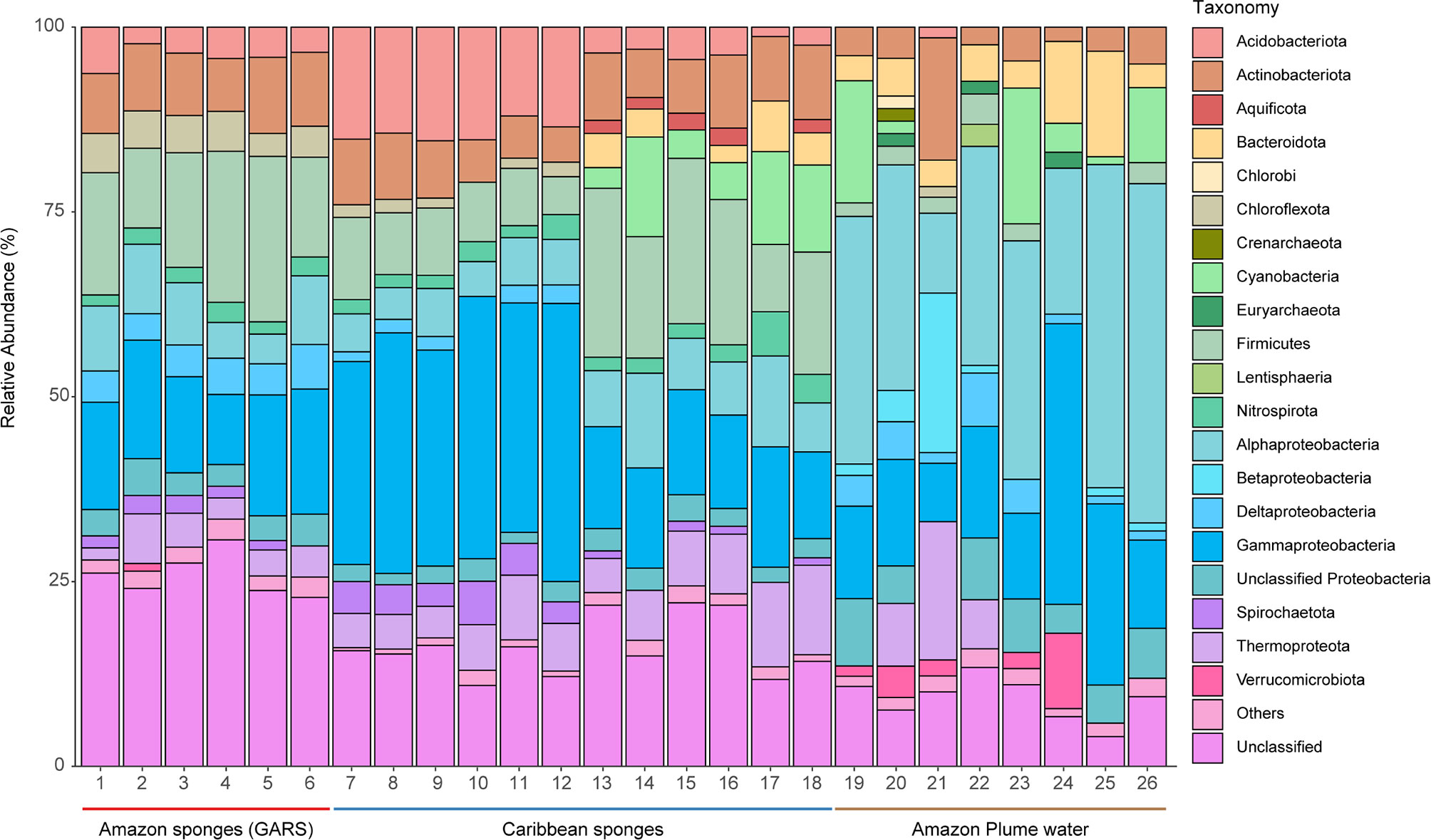
Figure 1 Relative abundance of 16S ribosomal RNA (16S rRNA) sequences in different microbiomes from GARS sponges, Caribbean sponges, and Amazon plume water. Amazon sponges (GARS) are highlighted with a red bar below: 1, Agelas dispar; 2, Agelas clathrodes 1; 3 Agelas clathrodes 2; 4, Geodia cf. corticostylifera; 5, Geodia neptuni; 6, Geodia sp. Caribbean sponges are highlighted with a blue bar below. Carribean Geodia sp. samples: 7, SRS9041575; 8, SRS9041573; 9, SRS9041427; 10, SRS9041416; 11, SRS9041405; 12, SRS9041395. Carribean Agelas sventres samples: 13, SRR7068181; 14, SRR7068176; 15, SRR7068173; 16, SRR7068159; 17, SRR7068157; 18, SRR7068155. Amazon plume water samples are highlighted with a brown bar below: 19, St15; 20, St11; 21, St10; 22, St6; 23, St5; 24, St4; 25, St3; and 26, St1. Relative abundance of 16S rRNA sequences are colored according to phyla. “Others” are microbiome phyla that were below 1% relative abundance. We split Proteobacteria superphylum into classes.
Shannon alpha diversity (Figure 2A) indicted higher diversity in the GARS sponge microbiome, followed by plume water and Caribbean sponge (Kruskal-Wallis test, chi-squared = 16.328, df = 2, P < 0.001). The GARS sponge microbiome showed to be the most The GARS sponge microbiome showed to be the most unique with 1476 taxa. The Amazon plume water influences the GARS sponge microbiome with 92 taxa uniquely shared. Sponge samples shared exclusively 488 taxa, and 186 were ubiquitous in all groups tested (Figure 2B).
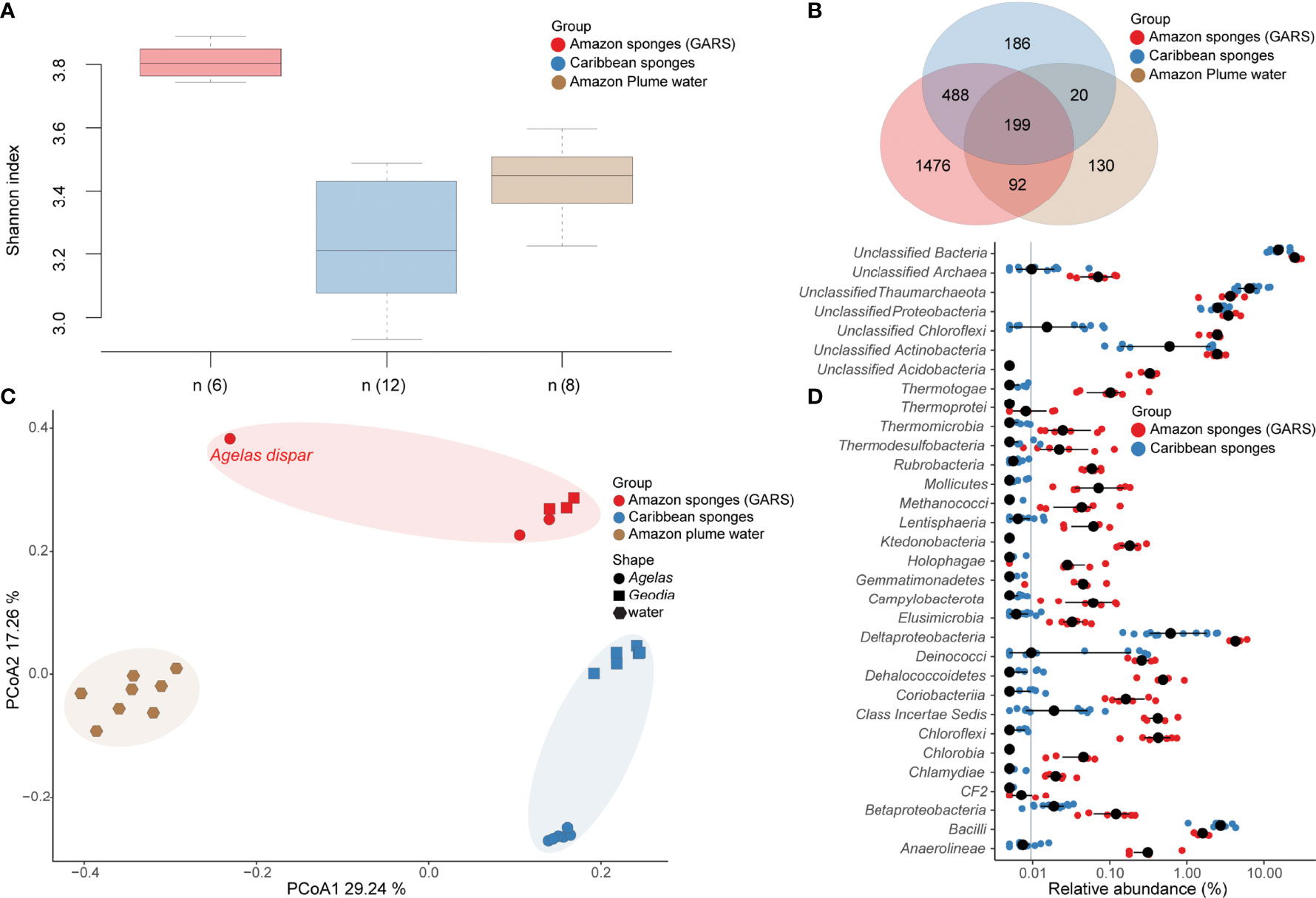
Figure 2 Microbiome composition comparison between GARS sponges Caribbean sponges and Amazon plume water samples. (A) Shannon Alpha diversity variation in sponge’s microbiome from the GARS (red) and the Caribbean (blue), as well as the Amazon plume water (brown) microbiome (Kruskal-Wallis test, chi-squared = 16.328, df = 2, P < 0.001). The (n) represents the number of samples in each box plot. Wilcoxon pairwise comparisons show that all groups tested were statistically different (P < 0.05). (B) Venn diagram of microbiome taxa that are unique or shared between sponges from the GARS (red) and the Caribbean (blue), as well as the Amazon plume water microbiome (brown). (C) Beta diversity of microbiomes associated with Agelas and Geodia sponges from the GARS (red) and the Caribbean (blue), as well as the Amazon plume water microbiome (brown) by principal coordinate analysis (PCoA) of Bray–Curtis dissimilarity (R2 = 0.43 P=0.0001). Each point represents a microbiome sample, Agelas are represented by circles, Geodia by rectangles, and water samples by hexagons. (D) Microbiome class level relative abundance differences between GARS and Caribbean sponges. GARS sponge’s microbiome is shown by red circles, while Caribbean sponges microbiome is shown by blue circles. Black circles are the median relative abundance for a specific taxa to the class level.
Beta diversity analysis (Figure 2C) showed that the Amazon sponge microbiome composition differed from the Caribbean and plume water samples (PERMANOVA, df = 2, F = 8.6343, R² = 0.42884, P < 0.001). On the class level, there were 32 taxa (Figure 2D) statistically different that contributed to the differences between the GARS and Caribbean sponge microbiome (Wilcoxon test, P < 0.05). The Amazon sponge microbiome is more similar to that of the Caribbean sponges, which are unimpacted by the plume, than to the plume water samples. Amazon Agelas and Geodia microbiomes grouped, while the Agelas dispar microbiome was found to be the most different among the six. We detected subgroups between Caribbean samples relative to the Agelas from Curacao and Geodia from Cayman Island and Belize locations (i.e., marked in blue). The Curacao Agelas samples are the six bottom blue circles in the cluster, while the Geodia samples near Cayman Island and Belize are the six top blue rectangles (Figure 2C).
Metagenome-Assembled Genome Classification
A total of 1,054 metagenome-assembled genomes (MAGs) were recovered from the Amazon reef sponge microbiome. Of these, 205 MAGs had completeness greater than 70%, and contamination below 10% (Supplementary Data Set S2). Within these 205 high-quality MAGs, we recovered bacterial genomes belonging to: Proteobacteria (49), Chloroflexota (47), Acidobacteriota (18), Actinobacteriota (16), Desulfobacterota (14), Bdellovibrionota (10), Poribacteria (10), Latescibacterota (8), Gemmatimonadota (5), Spirochaetota (4), Nitrospinota (3), Nitrospirota (3), Verrucomicrobiota (3), Deinococcota (2), Bacteroidota (1), and SAR324 (1). For archaea, we recovered Thermoproteota (10), and Nanoarchaeota (1). Within the 1,054 genomes, two Cyanobacteria MAGs were identified, but were not included in our analysis because they did not meet the quality cut-off. 16S rRNAs genes of Firmicutes origin were one of the most abundant in the metagenome, but they lack representatives in high-quality MAGs.
GARS Sponge Microbiome Metabolism Analysis
As shown in Figure 3, autotrophic metabolism related genes represent a small portion of the community’s genes. 3HB/4HP and 3HP were the most significant autotrophic pathways and scored higher than rTCA (Wilcoxon test, P < 0.01). CBB metabolism scored the lowest of all autotrophic networks studied. Genes related to WL and DC/4-HB profile were absent. Gene coverage and metabolic profiles revealed absence of photosynthetic capacity in the high-quality MAGs from the plume-adapted Amazon sponge microbial community. On the other hand, heterotrophic metabolism represented by fermentation, acetate oxidation, complex carbon degradation, and aromatics degradation functions were more abundant than autotrophic gene metabolism coverage in high-quality MAGs (Kruskal-Wallis test, P < 0.05).
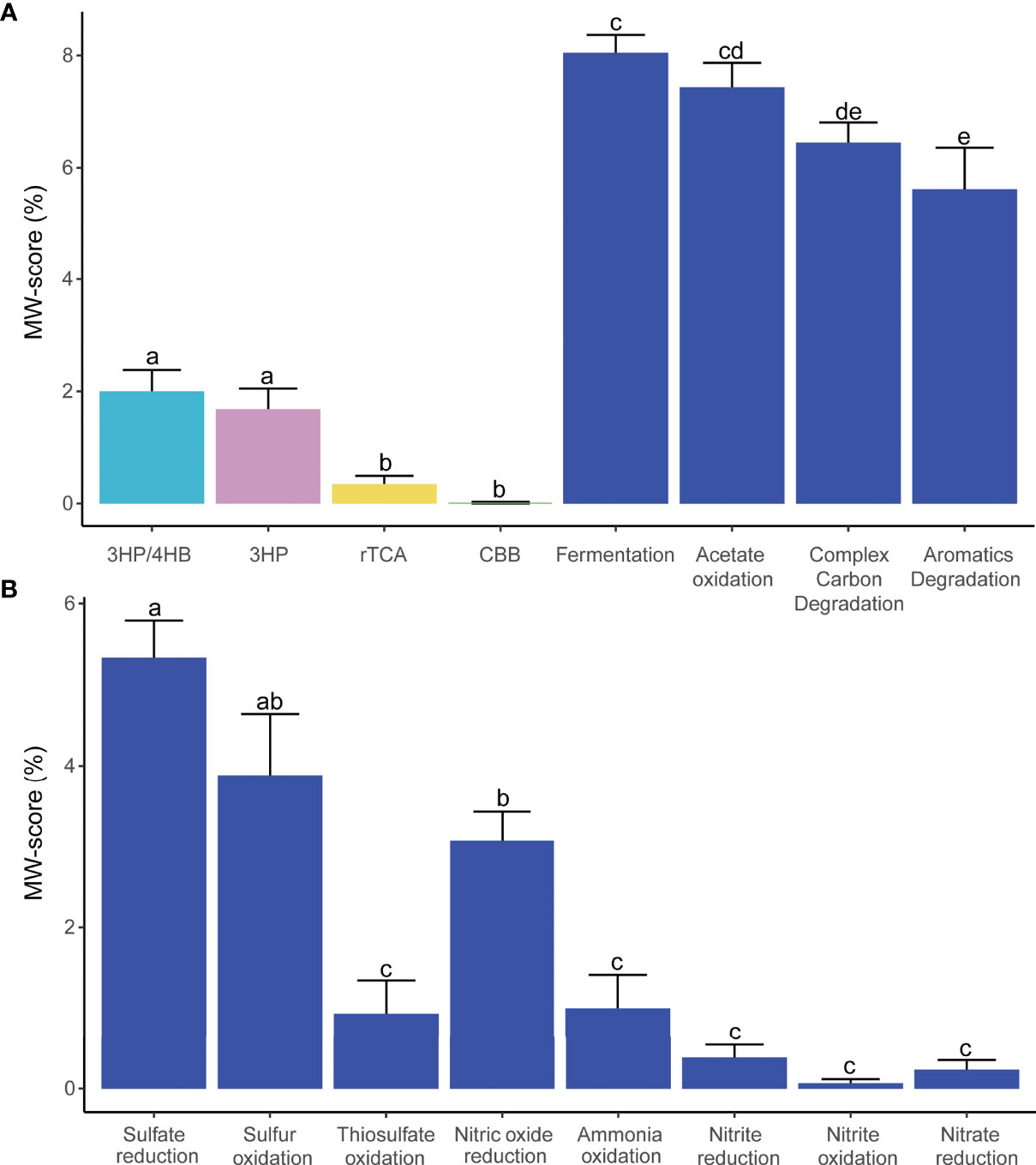
Figure 3 Microbiome metabolism profile based on metabolic weight score (MW-score). (A) Comparison between autotrophic and heterotrophic metabolisms. Autotrophic: 3-Hydroxypropionate/4-hydroxybutyrate (3HP/4HB), 3-Hydroxypropionate bicycle (3HP), Reductive tricarboxylic acid (rTCA), and Calvin–Benson–Bassham cycle (CBB); Heterotrophic: Fermentation, Acetate oxidation, Complex carbon degradation, and Aromatics degradation. (B) Comparison between sulfur and nitrogen metabolism. Bars with different letters indicate statistical differences at P < 0.05; those with the same letters indicate no statistical difference.
Sulfate reduction was the most abundant function identified in the plume-adapted Amazon sponge microbial community, with sulfur oxidation and nitric oxide reduction functions also being high. Sulfate reduction was a widespread function detected in 13 phyla with contribution from Chloroflexota (32), Gammaproteobacteria (14), Acidobacteriota (13), Thermoproteota (10), Poribacteria (9), Latescibacterota (6), Alphaproteobacteria (4), Gemmatimonadota (3), Verrucomicrobiota (3), Actinobacteriota (2), Deinococcota (2), Desulfobacterota (1), Nitrospinota (1), and Nitrospirota (1) MAGs. Sulfur oxidation was predicted in nine phyla, including Gammaproteobacteria (15), Alphaproteobacteria (14), Desulfobacterota (13), Thermoproteota (10), Actinobacteriota (9), Chloroflexota (8), Acidobacteriota (7), Bdellovibrionota (1), Latescibacterota (1), and Poribacteria (1) MAGs. We detected nine phyla predicted to participate in nitric oxide reduction function; associated with MAGs from Gammaproteobacteria (13), Desulfobacterota (10), Poribacteria (9), and Acidobacteriota (5), Alphaproteobacteria (3), Nitrospinota (3), Chloroflexota (2), Spirochaetota (2), Latescibacterota (1), and Nitrospirota (1) (Supplementary Data Set S3). We did not detect differences between reductive and oxidative metabolisms.
We detected the presence of other metabolic networks, although not as abundantly represented, such as thiosulfate oxidation, ammonia oxidation, nitrite reduction, nitrite oxidation, and nitrate reduction. Sulfide oxidation, sulfur reduction, sulfite reduction, nitrogen fixation, nitrous oxide reduction, and anammox functions were absent.
Metagenome-Assembled Genomes (MAGs) Autotrophic Metabolism Prediction
As shown in Figure 4, from 205 high-quality MAGs, 36 were predicted to participate in autotrophic metabolism pathways. We predicted the highest number of genomes with rTCA function belonging to Acidobacteriota (6), Actinobacteriota (4), Gammaproteobacteria (2), Chloroflexota (2), Latescibacterota (2), Bacteroidota (1), Verrucomicrobiota (1), and Spirochaetota (1). The key-enzymes succinate dehydrogenase/fumarate reductase, and 2-oxoglutarate/2-oxoacid ferredoxin oxidoreductase were detected for all selected MAGs, the exception was ATP-citrate lyase alpha-subunit, which was not identified. METABOLIC detected ATP-citrate lyase alpha-subunit gene in Nitrospirota and Desulfobacterota MAGs that had 3HP completeness between 66-41% and were not selected for analysis. METABOLIC did not detect ATP-citrate lyase alpha-subunit in selected high-quality MAGs, but they were detected with E-value between 2.00E-71 and 7.7E-68, using hmmsearch.
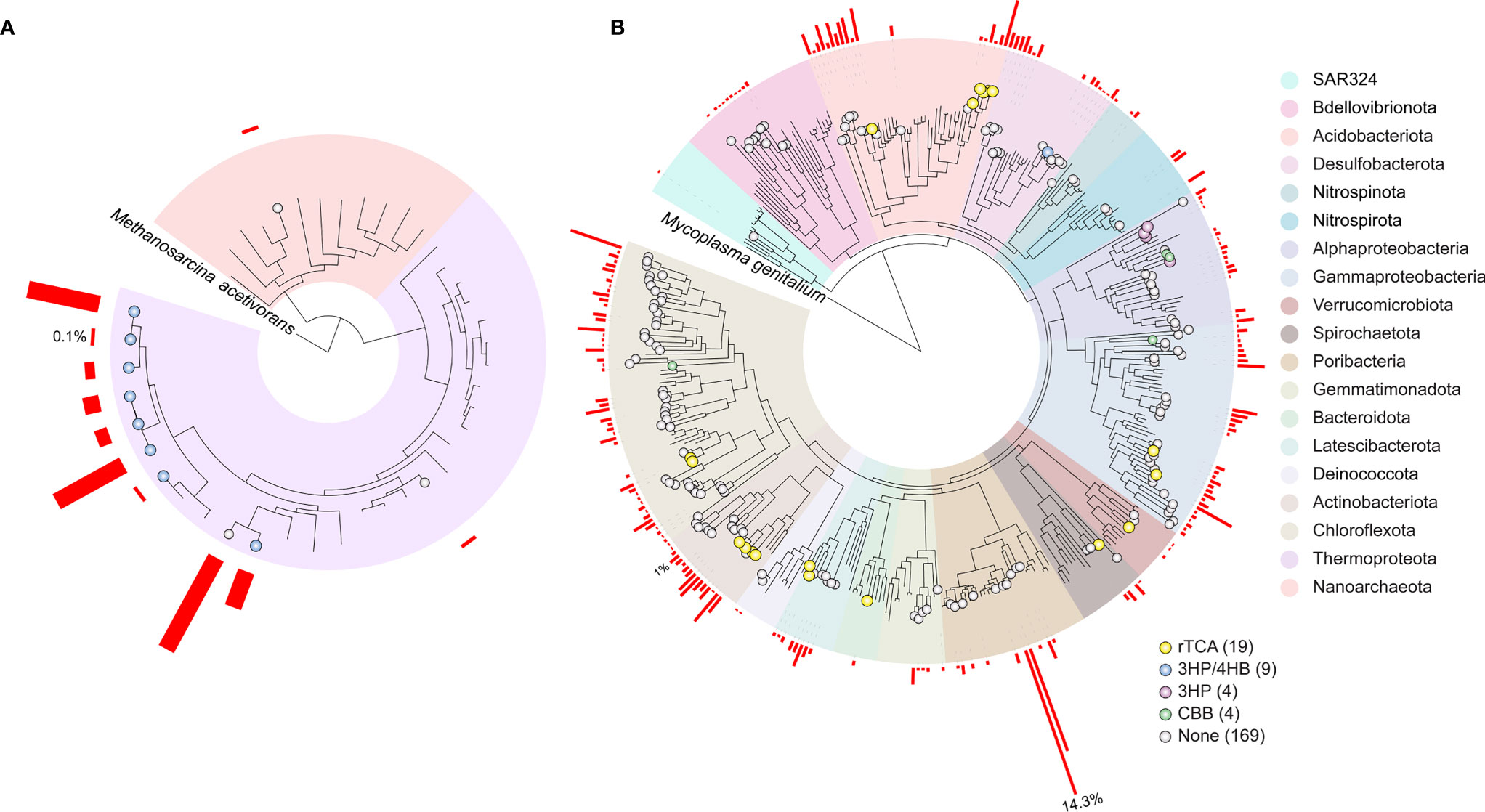
Figure 4 Phylogeny of metagenome-assembled genome (MAGs) in GARS. (A) Archaea MAGs phylogeny, (B) Bacteria MAGs phylogeny. The bacterial and archaeal trees are rooted to Methanosarcina acetivorans (RS_GCF_000007345.1) and Mycoplasma genitalium (RS_GCF_000027325.1), respectively. MAGs related to carbon fixation pathways are shown with circles: Reductive tricarboxylic acid (rTCA), yellow; Calvin–Benson–Bassham cycle (CBB), green; 3-Hydroxypropionate/4-hydroxybutyrate (3HP/4HB), blue; 3-Hydroxypropionate bicycle (3HP), purple. MAGs that did not meet the chosen METABOLIC carbon fixation cut-off are marked in grey (none). The number of MAGs in each carbon fixation pathway is shown in parentheses. Phylogeny of the MAGs is shaded by phylum, Proteobacteria were split into classes. The red bars next to each MAG represents the its abundance coverage (%) in the GARS sponge microbiome.
We selected eight Thermoproteota Nitrosopumilaceae, and one Desulfobacterota with possible involvement in the 3-hydroxypropionate/4-hydroxybutyrate (3-HP/4-HB) cycle. All selected genomes presented the key enzyme 4-hydroxybutyryl-CoA dehydratase/vinylacetyl-CoA-Delta-isomerase. These genomes showed 36% maximum completeness for the 3-HP/4-HB, but considering a less restrictive E-values cutoff, Thermoproteota genomes have all missing genes. Meanwhile, malonyl-CoA/succinyl-CoA reductase (NADPH) was absent for Desulfobacterota even in these less restrictive conditions.
The CBB cycle was identified in four MAGs. The Rubisco type I prediction was assigned to one Alphaproteobacteria Rhodobacterales and one Gammaproteobacteria Arenicellales. The Rubisco type IV prediction was assigned to one Dehalococcoidia within Chloroflexota and one Rhodobacterales.
We observed the highest completeness 3-Hydroxypropionate bicycle (3-HP) genes (58.3%) in four Rhodobacteraceae. These genomes lack the key enzymes: malonyl-CoA reductase/3-hydroxypropionate dehydrogenase (NADP+) and acrylyl-CoA reductase (NADPH)/3- hydroxypropionyl-CoA dehydratase/3-hydroxypropionyl-CoA synthetase. Considering a less restrictive gene cutoff, we detected all missing genes.
Discussion
We found that the sponge’s microbiome from the Great Amazon Reef System (GARS) is a unique community. The Amazon water turbidity-related microbiome influences the GARS sponge microbiome. However, data suggest more influences due to environmental features than to the shared microbiota. Significantly, and in contrast to other Reef systems, photosynthetic microorganisms (Cyanobacteria) were only present in low proportion in both 16S rRNA and MAG community analysis. GARS microbiome’s high diversity suggests that the plume layer does not restrict or enrich the microbiota for a specific taxonomic group. Moreover, 32 taxa on the class level were statistically different between the sponge microbiomes from GARS and traditional Caribbean reefs, indicating muddy water contributes to an adapted microbiota. Microbiome comparisons between the Caribbean (i.e., Curacao, Cayman Island, and Belize) and the Amazon region using sponges from the same genera, and two different genera, show that the findings are more general than they would be if we had made this comparison using a single genus and Caribbean location. Light in the GARS floor is faint but sufficient; a study shows the presence of photosynthetically available radiation (PAR) between 0.01 and 19.3 µE m-2 s-1 (Francini-Filho et al., 2018). Such ratio can support photosynthesis, and coralline algae reef-builders have been present in the GARS for at least 4487 years before today (Moura et al., 2016). Studies suggested high sedimentation as a principal stress factor in GARS, which results in total or partial GARS coverage in sediment (Moura et al., 2016; Omachi et al., 2019).
The data indicate a predominant heterotrophic lifestyle for the GARS sponge microbiota. The large amount of organic matter carried by the Amazon River may explain the sponge’s success in this ecosystem (Medeiros et al., 2015; Moura et al., 2016). A study revealed that dissolved organic carbon in the GARS region varies from 102.3 to 165.69 µM, and particulate organic carbon from 4.1 to 6.21 µM (unpublished data). The sponges’ microbiota also recycles and benefits from DOM (Azam et al., 1983) and contribute to more than half of the sponges’ diet (Kazanidis et al., 2018; Rix et al., 2020; Bart et al., 2021). Estimates that one sponge kilogram can filter 50,000 L of water daily and remove more than 90% of microbes in the water (Hill and Hill, 2009). Studies have demonstrated that photosynthetic net reduction and heterotrophic capacity enhancement are adaptive responses to overcome extreme conditions in turbid reefs (Burt et al., 2020).
Autotrophic microorganisms have an auxiliary role in the GARS sponge microbiome. MW-score indicates Calvin–Benson–Bassham (CBB) as the least relevant carbon fixation pathway present in the plume-adapted Amazon sponge microbial community, in stark contrast to the open ocean, where Cyanobacteria and algae are the major organisms responsible for carbon fixation through the CBB pathway (Raven, 2009; Bowler et al., 2010). Our MAGs analysis predicted CBB to occur in Rhodobacterales and Arenicellales. Rubisco type IV detected in a Chloroflexota MAG are related to functions other than carbon fixation (Tabita et al., 2007a; Tabita et al., 2007b). The presence of CBB cycle genes, especially Rubisco, in Proteobacteria and Rhodobacterales, has already been described, including the sponge microbiome (Badger and Bek, 2008; Asplund-Samuelsson and Hudson, 2021).
Metabolic predictions indicated 3HP/4HB as the most relevant carbon fixation pathway in the GARS sponge’s microbiome, significantly more abundant than CBB and rTCA (Wilcoxon test, P < 0.05). We predicted 3HP/4HB to occur in Thermoproteota and one Desulfobacterota MAG. The 3HP/4HB capability for the Desulfobacterota MAG remains uncertain as one of the two marker genes, the malonyl-CoA/succinyl-CoA reductase (NADPH) gene, could not be identified in this MAG. Additionally, the bacterial 4-hydroxybutyryl-CoA dehydratase is involved in the fermentation of aminobutyrate (Buckel and Golding, 2006). These findings were based on high-quality MAGs gene content and coverage observations - not expression data. Furthermore, it is important to note that genome-resolved metagenomics recovers just a fraction of the genomes present in the microbial community. The purpose of this study was to use in silico analyses to access the microbial functional capacity of samples for which there is no experimental information available.
Thaumarchaeota’s (Thermoproteota) variation in the 3HP/4HB pathway is well-described and considered the most energy-efficient aerobic carbon fixation pathway (Walker et al., 2010; Pester et al., 2011; Konneke et al., 2014). Studies have demonstrated the presence of Thaumarchaeota in the Amazon plume water and the sponge microbiome (Engelberts et al., 2020; Pinto et al., 2020). Our data indicate that these microorganisms play multiple roles in the sponge-microbiome holobiont physiology; besides carbon fixation, we predicted ammonia oxidation, sulfur oxidation, and sulfate reduction functions. Ammonia is the sponge’s main waste product, which is toxic at high levels. Ammonia oxidation can prevent its accumulation within sponge tissues. The same happens for sulfur oxidation, which prevents hydrogen sulfide levels to become high (Taylor et al., 2007).
Another predicted carbon fixation pathway was the 3-hydroxypropionate bicycle (3HP). This pathway was first described in Chloroflexus aurantiacus, a Chloroflexaceae member able to perform anoxygenic photosynthesis (Herter et al., 2001; Zarzycki et al., 2009). In our taxonomic analysis, we identified the presence of Chloroflexota MAGs related to Anaerolineae, Dehalococcoidia, and UBA223 families, not previously described as having this pathway. The MAGs predicted to encode this pathway in our GARS sponge microbiome were classified as Rhodobacteraceae. One study suggested Dinoroseobacter shibae a member of Rhodobacteraceae with mixotrophic metabolism, assimilating glucose by Entner-Doudoroff and fixing carbon by 3-hydroxypropionate bicycle (Tomasch et al., 2011). Alternative CO2 fixation pathways may facilitate the assimilation of simple organic substances, conferring a competitive advantage over obligate autotrophs or heterotrophs (Hügler and Sievert, 2011). The genomic comparison of 3HP genes between our MAGs and D. shibae showed that they are similar.
The same uncertainty occurs for rTCA genes encoding MAGs, where hmmsearch detected the ATP-citrate lyase alpha-subunit only when a less restrictive cutoff was used. The rTCA pathway was predicted to be the most taxonomically wide-spread pathway in the GARS sponge microbiome within Acidobacteriota, Gammaproteobacteria, Latescibacterota, Bacteroidota, Chloroflexota, Actinobacteriota, Verrucomicrobiota, and Spirochaetota. An incomplete rTCA cycle was found in phylum Acidobacteriota (Fernandez et al., 2020), Bacteroidota Salinibacter ruber (Bagheri et al., 2019) and other phyla like Actinobacteriota and Chloroflexota (Vikram et al., 2016). Among our low-quality MAGs, we have recovered members of Aquificota, which like Chlorobiota and Campylobacterota are often described to participate in rTCA (Hügler and Sievert, 2011). Due to the oxygen sensibility of its enzymes, the rTCA pathway is usually found in anaerobes associated with deep-sea hydrothermal vents and dark oceans and in microorganisms found in microaerophilic conditions (Berg, 2011; Erb, 2011). These data corroborate with dissolved oxygen (i.e., 3.25-3.81 mg. L-1) values reported in the GARS (unpublished data).
Prediction of the complex metabolic pathways was the main limitation of this study. Those pathways have many promiscuous genes, which makes it difficult to establish their involvement in any specific pathway. Furthermore, database profiles are incomplete. Sometimes genes that have already been shown to participate in a pathway have not yet been included in the database. An example is the 3HP/4HB pathway that lacks genes from Thaumarchaeta on the KEGG profile – making it difficult to identify non-classic pathways. Crenarchaeal Sulfolobales genes were used to construct the 3HP/4HB pathway profile found in the database used in this study. Additionally, the metabolic characterization of organisms based on their metabolic potential can not be used to predict their in vivo metabolism. Hence, further experimental validation is necessary, either culture independently through, e.g., Meta-Transcriptomics and -Proteomics, or through cultivation followed by the characterization of the culture.
In summary, our data indicate that although the plume induces a low light stress on the GARS sponge microbiome, it does not completely prevent Cyanobacteria occurrence. Specifically, we found a predominance of heterotrophic compared to autotrophic metabolism in the plume-adapted Amazon sponge microbial community. We propose that in GARS, sponges accumulate dissolved organic matter (DOM), which is assimilated by themselves as well as its heterotrophic microbiota. In addition, the sponges’ microbiota might act in the detoxification of toxic compounds released by the sponge.
Other turbid reefs present similar conditions of restricted luminosity or sedimentation to those found by GARS sponges (Zweifler (Zvifler) et al., 2021). However, none of these environments receive such a large water flux. The Amazon River discharges 20% of global freshwater into the Atlantic Ocean (Richey et al., 1990; Dai and Trenberth, 2002). This huge water flux into the ocean drastically changes environmental parameters such as salinity, pH, luminosity, oxygenation, and sedimentation, thus creating the unique environment of GARS. This study shows a different biomass accumulation strategy for GARS sponge microbiome from that of traditional sponge microbiomes. The uniqueness of the GARS sponge microbiome is ripe for biotechnological exploration, as new genes and drug precursors may be discovered. In addition, from an ecological perspective, the GARS microbiome data may be useful for surveillance efforts of potential impacts of offshore oil and gas operations near this region.
Data Availability Statement
The datasets presented in this study can be found in online repositories. The names of the repository/repositories and accession number(s) can be found below: https://www.ncbi.nlm.nih.gov/, PRJNA796108.
Author Contributions
OP and RK designed the study. Data analyses were performed by OP, TB, AP, TF, and RO. Data collection was performed by FT and MF. The manuscript was drafted by OP and revised by all authors, BQ critically reviewed and substantially edited the manuscript. All authors have read and approved the manuscript.
Funding
This study was financially supported by the Conselho Nacional de Desenvolvimento Científico e Tecnológico (CNPq), Coordenação de Aperfeiçoamento de Pessoal de Nível Superior (CAPES), which also provided financial support for the first author (OP), Fundação de Amparo à Pesquisa do Estado do Rio de Janeiro (FAPERJ), and Fundação de Apoio à Pesquisa do Distrito Federal (FAP-DF). TB and AP were supported by the Ministry of Culture and Science of North Rhine-Westphalia (Nachwuchsgruppe “Dr. Alexander Probst”).
Conflict of Interest
The authors declare that the research was conducted in the absence of any commercial or financial relationships that could be construed as a potential conflict of interest.
Publisher’s Note
All claims expressed in this article are solely those of the authors and do not necessarily represent those of their affiliated organizations, or those of the publisher, the editors and the reviewers. Any product that may be evaluated in this article, or claim that may be made by its manufacturer, is not guaranteed or endorsed by the publisher.
Acknowledgments
The authors thank Conselho Nacional de Desenvolvimento Científico e Tecnológico (CNPq), Fundação de Amparo à Pesquisa do Estado do Rio de Janeiro (FAPERJ), and Fundação de Apoio à Pesquisa do Distrito Federal (FAP-DF) for financial support. Pinto acknowledges fellowships from Coordenação de Aperfeiçoamento de Pessoal de Nível Superior (CAPES).
Supplementary Material
The Supplementary Material for this article can be found online at: https://www.frontiersin.org/articles/10.3389/fmars.2022.867234/full#supplementary-material
References
Asplund-Samuelsson J., Hudson E. P. (2021). Wide Range of Metabolic Adaptations to the Acquisition of the Calvin Cycle Revealed by Comparison of Microbial Genomes. PloS Comput. Biol. 17, e1008742. doi: 10.1371/journal.pcbi.1008742
Azam F., Fenchel T., Field J., Gray J., Meyer-Reil L., Thingstad F. (1983). The Ecological Role of Water-Column Microbes in the Sea. Mar. Ecol. Prog. Ser. 10, 257–263. doi: 10.3354/meps010257
Badger M. R., Bek E. J. (2008). Multiple Rubisco Forms in Proteobacteria: Their Functional Significance in Relation to CO2 Acquisition by the CBB Cycle. J. Exp. Bot. 59, 1525–1541. doi: 10.1093/jxb/erm297
Bagheri M., Marashi S.-A., Amoozegar M. A. (2019). A Genome-Scale Metabolic Network Reconstruction of Extremely Halophilic Bacterium Salinibacter Ruber. PloS One 14, e0216336. doi: 10.1371/journal.pone.0216336
Bart M. C., Hudspith M., Rapp H. T., Verdonschot P. F. M., de Goeij J. M. (2021). A Deep-Sea Sponge Loop? Sponges Transfer Dissolved and Particulate Organic Carbon and Nitrogen to Associated Fauna. Front. Mar. Sci. 8. doi: 10.3389/fmars.2021.604879
Bengtsson-Palme J., Hartmann M., Eriksson K. M., Pal C., Thorell K., Larsson D. G. J., et al. (2015). METAXA 2: Improved Identification and Taxonomic Classification of Small and Large Subunit rRNA in Metagenomic Data. Mol. Ecol. Resour. 15, 1403–1414. doi: 10.1111/1755-0998.12399
Berg I. A. (2011). Ecological Aspects of the Distribution of Different Autotrophic CO2 Fixation Pathways. Appl. Environ. Microbiol. 77, 1925–1936. doi: 10.1128/AEM.02473-10
Blunt J. W., Copp B. R., Keyzers R. A., Munro M. H. G., Prinsep M. R. (2017). Marine Natural Products. Nat. Prod. Rep. 34, 235–294. doi: 10.1039/C6NP00124F
Bornemann T. L. V., Adam P. S., Turzynski V., Schreiber U., Figueroa-Gonzalez P. A., Rahlff J., et al. (2022). Genetic Diversity in Terrestrial Subsurface Ecosystems Impacted by Geological Degassing. Nat. Commun. 13, 284. doi: 10.1038/s41467-021-27783-7
Bornemann T. L. V., Esser S. P., Stach T. L., Burg T., Probst A. J. (2020). Ubin – A Manual Refining Tool for Metagenomic Bins Designed for Educational Purposes. Genomics. doi: 10.1101/2020.07.15.204776
Bowler C., Vardi A., Allen A. E. (2010). Oceanographic and Biogeochemical Insights From Diatom Genomes. Annu. Rev. Mar. Sci. 2, 333–365. doi: 10.1146/annurev-marine-120308-081051
Buckel W., Golding B. T. (2006). Radical Enzymes in Anaerobes. Annu. Rev. Microbiol. 60, 27–49. doi: 10.1146/annurev.micro.60.080805.142216
Burt J. A., Camp E. F., Enochs I. C., Johansen J. L., Morgan K. M., Riegl B., et al. (2020). Insights From Extreme Coral Reefs in a Changing World. Coral Reefs 39, 495–507. doi: 10.1007/s00338-020-01966-y
Connell J. H. (1978). Diversity in Tropical Rain Forests and Coral Reefs: High Diversity of Trees and Corals Is Maintained Only in a Nonequilibrium State. Science 199, 1302–1310. doi: 10.1126/science.199.4335.1302
Dai A., Trenberth K. E. (2002). Estimates of Freshwater Discharge From Continents: Latitudinal and Seasonal Variations. J. Hydrometeor. 3, 660–687. doi: 10.1175/1525-7541(2002)003<0660:EOFDFC>2.0.CO;2
de Goeij J. M., van Oevelen D., Vermeij M. J.A., Osinga R., Middelburg J. J., de Goeij A. F.P.M., et al. (2013). Surviving in a Marine Desert: The Sponge Loop Retains Resources Within Coral Reefs. Science 342,108–110. doi: 10.1126/science.1241981
Drake J. L., Mass T., Stolarski J., Von Euw S., Schootbrugge B., Falkowski P. G. (2020). How Corals Made Rocks Through the Ages. Glob. Change Biol. 26, 31–53. doi: 10.1111/gcb.14912
Engelberts J. P., Robbins S. J., de Goeij J. M., Aranda M., Bell S. C., Webster N. S. (2020). Characterization of a Sponge Microbiome Using an Integrative Genome-Centric Approach. Isme J. 14, 1100–1110. doi: 10.1038/s41396-020-0591-9
Erb T. J. (2011). Carboxylases in Natural and Synthetic Microbial Pathways. Appl. Environ. Microbiol. 77, 8466–8477. doi: 10.1128/AEM.05702-11
Fernandez L., Peura S., Eiler A., Linz A. M., McMahon K. D., Bertilsson S. (2020). Diazotroph Genomes and Their Seasonal Dynamics in a Stratified Humic Bog Lake. Front. Microbiol. 11. doi: 10.3389/fmicb.2020.01500
Francini-Filho R. B., Asp N. E., Siegle E., Hocevar J., Lowyck K., D’Avila N., et al. (2018). Perspectives on the Great Amazon Reef: Extension, Biodiversity, and Threats. Front. Mar. Sci. 5. doi: 10.3389/fmars.2018.00142
Herter S., Farfsing J., Gad’On N., Rieder C., Eisenreich W., Bacher A., et al. (2001). Autotrophic CO2 Fixation by Chloroflexus Aurantiacus: Study of Glyoxylate Formation and Assimilation via the 3-Hydroxypropionate Cycle. J. Bacteriol. 183, 4305–4316. doi: 10.1128/JB.183.14.4305-4316.2001
Hill M. S., Hill A. L. (2009). “Porifera (Sponges),” in Encyclopedia of Inland Waters (Elsevier), 423–432. doi: 10.1016/B978-012370626-3.00186-1
Hügler M., Sievert S. M. (2011). Beyond the Calvin Cycle: Autotrophic Carbon Fixation in the Ocean. Annu. Rev. Mar. Sci. 3, 261–289. doi: 10.1146/annurev-marine-120709-142712
Indraningrat A., Micheller S., Runderkamp M., Sauerland I., Becking L., Smidt H., et al. (2019). Cultivation of Sponge-Associated Bacteria From Agelas Sventres and Xestospongia Muta Collected From Different Depths. Marine Drugs 17, 578. doi: 10.3390/md17100578
Katoh K. (2002). MAFFT: A Novel Method for Rapid Multiple Sequence Alignment Based on Fast Fourier Transform. Nucleic Acids Res. 30, 3059–3066. doi: 10.1093/nar/gkf436
Kazanidis G., van Oevelen D., Veuger B., Witte U. F. M. (2018). Unravelling the Versatile Feeding and Metabolic Strategies of the Cold-Water Ecosystem Engineer Spongosorites Coralliophaga (Stephen). Deep Sea Res. Part I: Oceanograph. Res. Papers 141, 71–82. doi: 10.1016/j.dsr.2018.07.009
Konneke M., Schubert D. M., Brown P. C., Hugler M., Standfest S., Schwander T., et al. (2014). Ammonia-Oxidizing Archaea Use the Most Energy-Efficient Aerobic Pathway for CO2 Fixation. Proc. Natl. Acad. Sci. 111, 8239–8244. doi: 10.1073/pnas.1402028111
Lewis W. H., Ettema T. J. G. (2019). Culturing the Uncultured. Nat. Biotechnol. 37, 1278–1279. doi: 10.1038/s41587-019-0300-2
Medeiros P. M., Seidel M., Ward N. D., Carpenter E. J., Gomes H. R., Niggemann J., et al. (2015). Fate of the Amazon River Dissolved Organic Matter in the Tropical Atlantic Ocean: Dom in the Amazon River-Ocean Continuum. Global Biogeochem. Cycles 29, 677–690. doi: 10.1002/2015GB005115
Moura R. L., Amado-Filho G. M., Moraes F. C., Brasileiro P. S., Salomon P. S., Mahiques M. M., et al. (2016). An Extensive Reef System at the Amazon River Mouth. Sci. Adv. 2, e1501252. doi: 10.1126/sciadv.1501252
Nurk S., Meleshko D., Korobeynikov A., Pevzner P. A. (2017). Metaspades: A New Versatile Metagenomic Assembler. Genome Res. 27, 824–834. doi: 10.1101/gr.213959.116
Oksanen J., Blanchet F. G., Kindt R., Legendre P., Minchin P., O’Hara R., et al. (2013). Vegan: Community Ecology Package. R Package Version. 2.0-10. CRAN.
Olm M. R., Brown C. T., Brooks B., Banfield J. F. (2017). Drep: A Tool for Fast and Accurate Genomic Comparisons That Enables Improved Genome Recovery From Metagenomes Through De-Replication. Isme J. 11, 2864–2868. doi: 10.1038/ismej.2017.126
Omachi C. Y., Asp N. E., Siegle E., Couceiro M. A. A., Francini-Filho R. B., Thompson F. L. (2019). Light Availability for Reef-Building Organisms in a Plume-Influenced Shelf. Continent. Shelf Res. 181, 25–33. doi: 10.1016/j.csr.2019.05.005
Parks D. H., Chuvochina M., Waite D. W., Rinke C., Skarshewski A., Chaumeil P.-A., et al. (2018). A Standardized Bacterial Taxonomy Based on Genome Phylogeny Substantially Revises the Tree of Life. Nat. Biotechnol. 36, 996–1004. doi: 10.1038/nbt.4229
Parks D. H., Imelfort M., Skennerton C. T., Hugenholtz P., Tyson G. W. (2015). CheckM: Assessing the Quality of Microbial Genomes Recovered From Isolates, Single Cells, and Metagenomes. Genome Res. 25, 1043–1055. doi: 10.1101/gr.186072.114
Parks D. H., Rinke C., Chuvochina M., Chaumeil P.-A., Woodcroft B. J., Evans P. N., et al. (2017). Recovery of Nearly 8,000 Metagenome-Assembled Genomes Substantially Expands the Tree of Life. Nat. Microbiol. 2, 1533–1542. doi: 10.1038/s41564-017-0012-7
Pester M., Schleper C., Wagner M. (2011). The Thaumarchaeota: An Emerging View of Their Phylogeny and Ecophysiology. Curr. Opin. Microbiol. 14, 300–306. doi: 10.1016/j.mib.2011.04.007
Pinto O. H. B., Silva T. F., Vizzotto C. S., Santana R. H., Lopes F. A. C., Silva B. S., et al. (2020). Genome-Resolved Metagenomics Analysis Provides Insights Into the Ecological Role of Thaumarchaeota in the Amazon River and Its Plume. BMC Microbiol. 20, 13. doi: 10.1186/s12866-020-1698-x
Pita L., Rix L., Slaby B. M., Franke A., Hentschel U. (2018). The Sponge Holobiont in a Changing Ocean: From Microbes to Ecosystems. Microbiome 6, 46. doi: 10.1186/s40168-018-0428-1
Price M. N., Dehal P. S., Arkin A. P. (2010). FastTree 2 – Approximately Maximum-Likelihood Trees for Large Alignments. PloS One 5, e9490. doi: 10.1371/journal.pone.0009490
R Core Team (2021). R: A Language and Environment for Statistical Computing (Vienna, Austria: R Version 4.1.2, R Foundation for Statistical Computing). Available at: https://www.r-project.org/.
Raven J. (2009). Contributions of Anoxygenic and Oxygenic Phototrophy and Chemolithotrophy to Carbon and Oxygen Fluxes in Aquatic Environments. Aquat. Microb. Ecol. 56, 177–192. doi: 10.3354/ame01315
Reveillaud J., Maignien L., Eren A. M., Huber J. A., Apprill A., Sogin M. L., et al. (2014). Host-Specificity Among Abundant and Rare Taxa in the Sponge Microbiome. Isme J. 8, 1198–1209. doi: 10.1038/ismej.2013.227
Richey J. E., Hedges J. I., Devol A. H., Quay P. D., Victoria R., Martinelli L., et al. (1990). Biogeochemistry of Carbon in the Amazon River. Limnol. Oceanogr. 35, 352–371. doi: 10.4319/lo.1990.35.2.0352
Rix L., Ribes M., Coma R., Jahn M. T., de Goeij J. M., van Oevelen D., et al. (2020). Heterotrophy in the Earliest Gut: A Single-Cell View of Heterotrophic Carbon and Nitrogen Assimilation in Sponge-Microbe Symbioses. Isme J. 14, 2554–2567. doi: 10.1038/s41396-020-0706-3
Sieber C. M. K., Probst A. J., Sharrar A., Thomas B. C., Hess M., Tringe S. G., et al. (2018). Recovery of Genomes From Metagenomes via a Dereplication, Aggregation and Scoring Strategy. Nat. Microbiol. 3, 836–843. doi: 10.1038/s41564-018-0171-1
Silva B. S., de O., Coutinho F. H., Gregoracci G. B., Leomil L., de Oliveira L. S., et al. (2017). Virioplankton Assemblage Structure in the Lower River and Ocean Continuum of the Amazon. mSphere 2. doi: 10.1128/mSphere.00366-17
Stanley G.D. Jr (2006). Photosymbiosis and the Evolution of Modern Coral Reefs. Evolution 1, 3. doi: 10.1126/science.1123701
Tabita F. R., Hanson T. E., Li H., Satagopan S., Singh J., Chan S. (2007a). Function, Structure, and Evolution of the RubisCO-Like Proteins and Their RubisCO Homologs. Microbiol. Mol. Biol. Rev. 71, 576–599. doi: 10.1128/MMBR.00015-07
Tabita F. R., Satagopan S., Hanson T. E., Kreel N. E., Scott S. S. (2007b). Distinct Form I, II, III, and IV Rubisco Proteins From the Three Kingdoms of Life Provide Clues About Rubisco Evolution and Structure/Function Relationships. J. Exp. Bot. 59, 1515–1524. doi: 10.1093/jxb/erm361
Taylor M. W., Radax R., Steger D., Wagner M. (2007). Sponge-Associated Microorganisms: Evolution, Ecology, and Biotechnological Potential. Microbiol. Mol. Biol. Rev. 71, 295–347. doi: 10.1128/MMBR.00040-06
Tomasch J., Gohl R., Bunk B., Diez M. S., Wagner-Döbler I. (2011). Transcriptional Response of the Photoheterotrophic Marine Bacterium Dinoroseobacter Shibae to Changing Light Regimes. Isme J. 5, 1957–1968. doi: 10.1038/ismej.2011.68
Trindade-Silva A. E., Rua C., Silva G. G. Z., Dutilh B. E., Moreira A. P. B., Edwards R. A., et al. (2012). Taxonomic and Functional Microbial Signatures of the Endemic Marine Sponge Arenosclera Brasiliensis. PloS One 7, e39905. doi: 10.1371/journal.pone.0039905
Turner E. C. (2021). Possible Poriferan Body Fossils in Early Neoproterozoic Microbial Reefs. Nature 596, 87–91. doi: 10.1038/s41586-021-03773-z
Tyson G. W., Chapman J., Hugenholtz P., Allen E. E., Ram R. J., Richardson P. M., et al. (2004). Community Structure and Metabolism Through Reconstruction of Microbial Genomes From the Environment. Nature 428, 37–43. doi: 10.1038/nature02340
Vikram S., Guerrero L. D., Makhalanyane T. P., Le P. T., Seely M., Cowan D. A. (2016). Metagenomic Analysis Provides Insights Into Functional Capacity in a Hyperarid Desert Soil Niche Community: Functional Capacity in Namib Hypoliths. Environ. Microbiol. 18, 1875–1888. doi: 10.1111/1462-2920.13088
Walker C. B., de la Torre J. R., Klotz M. G., Urakawa H., Pinel N., Arp D. J., et al. (2010). Nitrosopumilus Maritimus Genome Reveals Unique Mechanisms for Nitrification and Autotrophy in Globally Distributed Marine Crenarchaea. Proc. Natl. Acad. Sci. 107, 8818–8823. doi: 10.1073/pnas.0913533107
Webster N. S., Thomas T. (2016). The Sponge Hologenome. mBio 7 (2), e00135–16. doi: 10.1128/mBio.00135-16
Wu Y.-W., Simmons B. A., Singer S. W. (2016). MaxBin 2.0: An Automated Binning Algorithm to Recover Genomes From Multiple Metagenomic Datasets. Bioinformatics 32, 605–607. doi: 10.1093/bioinformatics/btv638
Zarzycki J., Brecht V., Müller M., Fuchs G. (2009). Identifying the Missing Steps of the Autotrophic 3-Hydroxypropionate CO 2 Fixation Cycle in Chloroflexus Aurantiacus. Proc. Natl. Acad. Sci. U. S. A. 106, 21317–21322. doi: 10.1073/pnas.0908356106
Zhou Z., Tran P. Q., Breister A. M., Liu Y., Kieft K., Cowley E. S., et al. (2022). METABOLIC: High-Throughput Profiling of Microbial Genomes for Functional Traits, Metabolism, Biogeochemistry, and Community-Scale Functional Networks. Microbiome 1, 10–33. doi: 10.1186/s40168-021-01213-8
Keywords: Carbon fixation, Host-associated, Metagenome-assembled genomes (MAGs), Sponge microbiome, the Great Amazon Reef System (GARS)
Citation: Pinto OHB, Bornemann TLV, Oliveira RS, Frederico TD, Quirino BF, Probst AJ, de Freitas MAM, Thompson FL and Kruger RH (2022) Plume Layer Influences the Amazon Reef Sponge Microbiome Primary Producers. Front. Mar. Sci. 9:867234. doi: 10.3389/fmars.2022.867234
Received: 31 January 2022; Accepted: 13 April 2022;
Published: 19 May 2022.
Edited by:
Sébastien Duperron, Muséum National d’Histoire Naturelle, FranceReviewed by:
Cara Fiore, Appalachian State University, United StatesMarie-Charlotte Cheutin, Université de Montpellier, CNRS, France
Copyright © 2022 Pinto, Bornemann, Oliveira, Frederico, Quirino, Probst, de Freitas, Thompson and Kruger. This is an open-access article distributed under the terms of the Creative Commons Attribution License (CC BY). The use, distribution or reproduction in other forums is permitted, provided the original author(s) and the copyright owner(s) are credited and that the original publication in this journal is cited, in accordance with accepted academic practice. No use, distribution or reproduction is permitted which does not comply with these terms.
*Correspondence: Ricardo H. Kruger, a3J1Z2VyQHVuYi5icg==