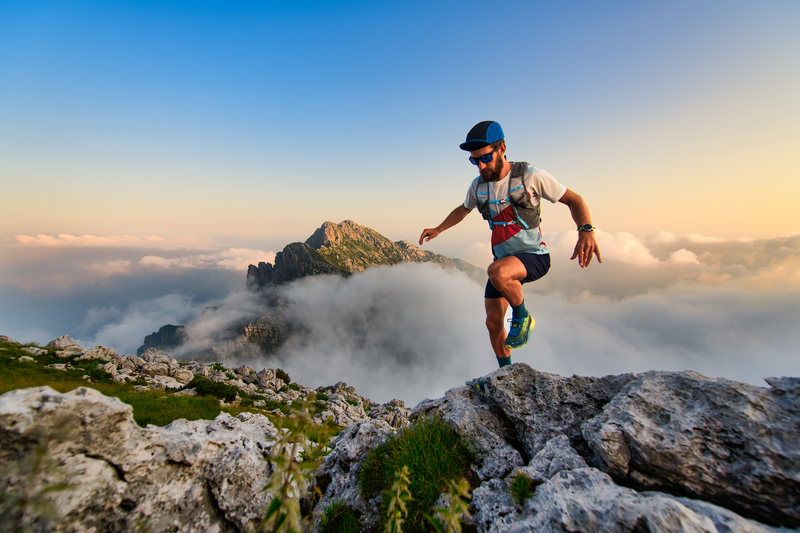
94% of researchers rate our articles as excellent or good
Learn more about the work of our research integrity team to safeguard the quality of each article we publish.
Find out more
REVIEW article
Front. Mar. Sci. , 28 June 2022
Sec. Marine Ecosystem Ecology
Volume 9 - 2022 | https://doi.org/10.3389/fmars.2022.867007
Microeukaryotes (protists) serve fundamental roles in the marine environment as contributors to biogeochemical nutrient cycling and ecosystem function. Their activities can be inferred through metatranscriptomic investigations, which provide a detailed view into cellular processes, chemical-biological interactions in the environment, and ecological relationships among taxonomic groups. Established workflows have been individually put forth describing biomass collection at sea, laboratory RNA extraction protocols, and bioinformatic processing and computational approaches. Here, we present a compilation of current practices and lessons learned in carrying out metatranscriptomics of marine pelagic protistan communities, highlighting effective strategies and tools used by practitioners over the past decade. We anticipate that these guidelines will serve as a roadmap for new marine scientists beginning in the realms of molecular biology and/or bioinformatics, and will equip readers with foundational principles needed to delve into protistan metatranscriptomics.
Metatranscriptomics, or community gene expression profiling, offers a window into transcript pool composition within mixed microbial assemblages. This information can be used to infer taxon-specific physiology and elucidate links between cell metabolism and ecosystem function. When applied to marine systems, it may offer information on the biogeochemical and ecological roles of community members across ecosystems and environmental conditions. Marine metatranscriptomic studies to date have been conducted across spatial and temporal scales (e.g., Sun et al., 2020; Becker et al., 2021; Cohen et al., 2021; Coesel et al., 2021; Groussman et al., 2021; Harke et al., 2021; Muratore et al., 2022), and in concert with experimental incubations/microcosms in which key biological, chemical and/or physical parameters are manipulated (e.g., Alexander et al., 2015b; Bertrand et al., 2015; Lampe et al., 2018). Such metatranscriptomic investigations into marine protists have expanded our understanding of their nutrient physiology (Alexander et al., 2015a; Pearson et al., 2015; Lampe et al., 2018; Caputi et al., 2019; Kolody et al., 2019), nutritional modes (Hu et al., 2018; Lambert et al., 2021), coastal bloom dynamics (Gong et al., 2017; Ji et al., 2018; Metegnier et al., 2020), and contributions to ocean biogeochemistry (Carradec et al., 2018; Cohen et al., 2021).
Marine meta-omic studies, or community omic analyses performed in the environment, emerged in the 2000s with the first successful applications of shotgun DNA sequencing to microbial communities of the open ocean (Venter et al., 2004; Rusch et al., 2007). Early marine meta-omic studies relied upon high-throughput Sanger and second generation sequencing (454 pyrosequencing and SOLiD) platforms (Venter et al., 2004; Rusch et al., 2007; Gilbert et al., 2008; McCarren et al., 2010; Stewart et al., 2010), with the first metatranscriptomic study focusing on marine microeukaryotes released in 2012 (Marchetti et al., 2012). Pyrosequencing and SOLiD platforms were later replaced by Illumina bridge amplification-based sequencing as a result of unparalleled throughput, low error rates, and mid-range read lengths (~2 x 150 bp, paired-end) that were longer than SOLiD (~85 bp), but shorter than pyrosequencing and Sanger sequencing (>400 bp) (Liu et al., 2012; Ambardar et al., 2016; Wilms, 2021). The price of sequencing has continued to decrease, while the degree of throughput has increased (Muir et al., 2016).
Marine metatranscriptomic initiatives have greatly benefited from the expansion of reference sequence libraries derived from laboratory isolates, such as the Marine Microbial Eukaryote Transcriptome Sequencing Project (MMETSP) (Keeling et al., 2014; Caron et al., 2017). The MMETSP database is composed of over 678 protistan transcriptomes from 405 unique strains (Krinos et al., 2021) and is widely used in current marine omic workflows (e.g., Lampe et al., 2018; Kolody et al., 2019; Groussman et al., 2021; Krinos et al., 2022). Oceanographic field expeditions such as Tara Oceans (Carradec et al., 2018) and bioGEOTRACES (Biller et al., 2018) are leveraging this database and other recently generated eukaryotic transcriptomes and genomes to uncover the taxonomic and functional roles of protists across ocean basins (Carradec et al., 2018; Alexander et al., 2021; Weissman et al., 2021; Blaxter et al., 2022; Delmont et al., 2022). These large field datasets describing the biographical and functional distribution of protists consist of sequence data, taxonomic and functional annotations, and contextualizing environmental metadata, and serve as invaluable community resources for researchers, students, and the public to use (Biller et al., 2018; Carradec et al., 2018; Villar et al., 2018).
The computational biology landscape is rapidly evolving as more efficient bioinformatic tools are developed and pipelines become more easily accessible. There has been a concerted community effort towards increasing reproducibility of data analysis by documenting protocols, archiving code and raw data, and making bioinformatic data products available to scientific audiences (Sandve et al., 2013; Tully et al., 2021). These resources are invaluable to new practitioners lacking formal training in molecular biology, computational biology, and/or statistics.
The interdisciplinary oceanographic community in particular would benefit from a comprehensive overview that emphasizes current approaches used to conduct microeukaryote metatranscriptomics. Here we present a compiled resource highlighting the latest practices and procedures, from sample collection to computational analysis. This effort complements other valuable environmental metatranscriptomic reviews that have been recently published (e.g., Shakya et al., 2019; Mukherjee and Reddy, 2020; Zhang et al., 2021; Wilms, 2021; Kolody et al., 2022). We anticipate these recommendations will continue to evolve in the coming years, and encourage researchers to explore current sequencing platform options and bioinformatic workflows, and to consult updated versions of tool documentation before beginning new analyses. It may be particularly valuable for those with a microbial ecology and/or oceanography background to engage in discussions and apply principles learned from computational biologists (and vice versa) to effectively innovate across these subfields. Our descriptions of protocols, pipelines and tools are not intended to be technical accounts but rather a descriptive guide for beginning and returning practitioners, and readers are referred to individual publications for further details.
The seawater volumes required to obtain suitable biomass for metatranscriptomic sequencing varies depending on the ecosystem. In offshore waters, volumes ~10 L or greater are generally recommended, while coastal systems supporting higher biomass require much lower volume (Table 1). Sample volumes that are too low have been shown to skew relative abundances of 16S rRNA amplicon sequences, with volumes >1 L generally encouraged (Padilla et al., 2015); this is likely also true for metatranscriptomic data, though has not yet been systematically verified.
While larger seawater volumes are ideal for the collection of sufficient biomass, the logistics of processing samples in the field (e.g., shipboard) must also be considered. Nucleic acid sample collection from seawater requires rapid filtration and preservation (Frias-Lopez et al., 2008), otherwise RNA degradation or unintended changes in the RNA pool may occur (Gallego Romero et al., 2014; Kolody et al., 2022), and cells may metabolically respond to changes in light and temperature during long collection times. Peristaltic pumps in conjunction with 47-142 mm in-line filter manifolds or Sterivex (Millipore) filters enable large volumes to be filtered rapidly and effectively (Table 1). Notably, filters may clog as biomass accumulates, especially for small filter size fractions, impacting filter effectiveness and sample quality. The filtration time may be reduced by splitting volumes across multiple filters. It is important to consider pump pressure levels during the filtration process, as high pressure could rupture cells before preservation, leading to RNA loss.
Increasingly, underwater battery-operated McLane filtration pumps (Saito et al., 2014), Lagrangian-like Environmental Sample Processors (Scholin et al., 2017; Kolody et al., 2019), Autonomous Underwater Vehicles (Breier et al., 2020), and other sensors (Ottesen, 2016) capable of in situ filtration at ambient temperature and pressure are being utilized. High-volume pumping mechanisms offered by McLane pumps and AUVs in particular are ideal for concentrating large amounts of biomass. These in situ approaches are useful for deep sea sampling, where depressurization during traditional seawater collection and processing may result in inaccurate assessments of community dynamics (Edgcomb et al., 2016). Filtration time is likely still important to consider in study designs using underwater pumps, as long filtrations may result in an integrated metatranscriptomic signal over time as biomass aggregates. With any sampling system used, the time needed to recover filters and preserve samples should be minimized (< 1 hour, if possible) to prevent RNA degradation.
Typical filter fraction size ranges for marine protists span 0.8 - 200 μm (Pesant et al., 2015) and generally align with the plankton size fractions (Omori and Ikeda, 1992). In some studies, no upper bound size threshold is used, and multicellular eukaryotes are included in the analysis (Table 1). It’s important to note that the specific size fraction used to capture protists will vary depending on the group of organisms intended to collect, with characterized smaller protists such as the green algae Ostreococcus approximately ~1 μm in diameter (Derelle et al., 2006), and members of the Foraminifera on the larger end of the size spectrum at >150 μm (Lo Giudice Cappelli and Austin, 2019). Size-fractionated filtering can be an advantageous strategy for capturing multiple distinct plankton size classes (Villar et al., 2018), in which filter membranes are either stacked and separated by backing filters, or arranged serially in separate filter manifolds. This approach may be valuable in concentrating biomass from specific groups of interest, but is imperfect as filters aggregate biomass especially with large seawater volumes, and smaller than intended particles may be captured on filters (Cohen et al., 2021). It is furthermore difficult to directly compare gene expression across these distinct size fractions, though may be approximated using biomass normalizations (Dupont et al., 2015).
Filter membranes made of polyethersulfone or polycarbonate are commonly used, and allow sufficient resuspension of material during the RNA extraction procedure (Table 1). RNA rapidly degrades, and instant RNA stabilization achieved via flash freezing with liquid nitrogen is recommended prior to storage at -80°C (Alvarez et al., 2015). If -80°C storage is not available on ships, RNA can be stored up to 1 week at room temperature, 1 month at 4°C, or indefinitely at -20°C using the RNAlater (Invitrogen) preservative reagent. However, RNAlater may result in inadvertent physiological changes in the RNA pool (Passow et al., 2019). Care should be taken in evaluating available storage mechanisms in the field and the potential risks of preservatives.
Biological replicates are generally required to determine statistical differences in gene expression and physiology across treatments or spatial zonation, but logistic constraints onboard research vessels often prevent repeat collection of seawater. In addition, microbial communities at a given location, depth, and time of day can rapidly shift due to the heterogeneous and highly dynamic nature of the ocean environment, complicating efforts to obtain replicated snapshots of community composition and function. This can however represent natural biological variability in a system, and samples collected from the same location may indeed serve as replicates, depending on the study objectives and spatial scope. Researchers may instead prioritize additional sampling depths, locations, or time points to capture transcript pools with high resolution. Samples collected from similar latitudes and depths may reflect similar biological properties, thus demonstrating oceanographic consistency across space and time (Cerdan-Garcia et al., 2021; Hogle et al., 2021). High frequency sampling may identify metabolic trends that either change on a diel cycle (e.g., energy partitioning), or are unaffected by temporal dynamics (e.g., chronic nutrient stress). In particular, there is significant diel periodicity in metabolic processes carried out by protists in surface waters (Kolody et al., 2019; Becker et al., 2021; Groussman et al., 2021), and time of day should therefore be considered in sampling designs.
RNA extractions from microeukaryotic cells collected onto filters may be performed using popular commercially available kits (Table 1). Common modifications include the addition of silica or zirconia beads to lysis buffer and bead-beating to assist with the physical disruption of cell walls during the RNA extraction, which is useful for hard-shelled protists. Total RNA yields and quality may be estimated using a Nanodrop spectrophotometer (Thermo Scientific), though a Qubit fluorometer (Invitrogen), Bioanalyzer (Agilent) or Tapestation (Agilent) will produce more accurate estimates of RNA concentration, especially at lower concentrations (Hussing et al., 2018). The RiboGreen (Promega) fluorescent nucleic acid stain is another suitable option for RNA quantification with high sensitivity (Jones et al., 1998). The Bioanalyzer and Tapestation will additionally provide information on RNA quality, including RNA integrity number (RIN) scores, which reflect degree of RNA degradation. High RIN scores represent high quality RNA (Schroeder et al., 2006). RIN scores above 7 are encouraged for Illumina library preparation, although low RNA yields and partial degradation that occurs during the seawater filtration process may make it difficult to reach high RIN scores (Alberti et al., 2017). However, certain protocols allow modifications for low RIN RNA to be used in the library preparation process.
In addition to relative transcript abundances obtained through metatranscriptomic sequencing, approaches have been developed to estimate transcript copies L-1 to more directly relate metabolic information to biogeochemical measurements, and to circumvent limitations inherent to relative analyses (Satinsky et al., 2013; Gifford et al., 2014). Custom-built plasmids or commercially available mRNA internal standards (e.g., ERCC Spike-In [Thermo Scientific], ArrayControl RNA Spikes [Invitrogen], Sequins [Garvan Institute of Medical Research]) can be added to lysis buffer during the initial stages of the RNA extraction, and will reflect losses during the laboratory procedure. Assuming the exact volume filtered is known, the number of added mRNA standard copies sequenced can be used to estimate transcript concentration in the sample (Satinsky et al., 2013; Gifford et al., 2014; see below). Increasing the number of distinct mRNA standards spiked will strengthen the copies-to-sequences estimation. An appropriate rule of thumb is to add the mRNA spike concentration at a target ratio of ~1% the total RNA pool (Gifford et al., 2014). Note that the number of RNA copies per cell may differ by organism, with larger cells generally containing higher RNA concentrations (Marguerat and Bähler, 2012). Transcript pools furthermore fluctuate over the course of a day, with phytoplankton groups under different diel transcriptional regulation (Groussman et al., 2021). These factors may contribute to the copies L-1 estimate among taxa not fully scaling with absolute cell densities. It is recommended to collect direct information on biomass, including pigments and/or cell counts (flow cytometry, fluid imaging, microscopy, etc), to provide environmental context for transcript-derived copies L-1.
Popular and cost effective second generation sequencing technology platforms for metatranscriptomics include Illumina Miseq and Hiseq (Table 1), with these older platforms being replaced by the newer and more efficient Nextseq and Novaseq. Novaseq currently offers the greatest output (~20 billion reads per run), with the Miseq platform still offering the longest read lengths (2 x 300 base pairs) but Novaseq not far behind with 2 x 250 base pairs possible using the Novaseq SP flow cell.
For mRNA-Seq studies performed with the Illumina platform, RNA is converted to cDNA and fragmented as part of the sequencing library preparation process. It is important to ensure that the sequencing library preparation method chosen enriches for protein-coding RNA, or messenger RNA (mRNA), because the majority of the total RNA pool in eukaryotes consists of ribosomal RNA (rRNA) (Bush et al., 2017). Two approaches may be used: rRNA depletion, in which rRNA is removed and non-coding RNA and mRNA remain, and polyadenylated RNA (poly-A) selection, in which mRNA containing poly-A tails (characteristic of eukaryotic mRNA) is selected along with other poly-A-containing non-coding RNA (Cui et al., 2010). The methods differ in resulting RNA pools, coverage, and quantitative accuracy (Zhao et al., 2018), with poly-A selection yielding more protein-coding sequences at a given sequencing depth (Chen et al., 2020). A key difference is that rRNA depletion will select for mRNA from both microbial prokaryotes and eukaryotes, while poly-A selection will be biased towards eukaryotes containing poly-adenylated mRNA. Additionally, rRNA depletion better recovers important eukaryotic organelle transcripts without selection bias. These non-target sequences may provide valuable information especially regarding plastid expression (Smith, 2013), such as Rubisco and cytochrome oxidase, which are of biogeochemical interest (Dupont et al., 2015; Kolody et al., 2022). It is unclear whether these non-target sequences are truly able to be used comparatively across samples or whether the level of non-poly-A RNA contamination differs depending on sample matrix or other factors. Both rRNA depletion and poly-A selection are commonly used in marine microeukaryote studies (Table 1), and should be selected based on specific scientific goals and interests. Note that when analyzing metatranscriptomes processed using rRNA depletion, recovery of eukaryotes might be low, and prokaryotes may constitute the majority of the sequence library. Popular methods for rRNA depletion include Ribo-Zero (Illumina) and riboPOOLs, which are available in custom mixtures to reduce rRNA from phylogenetically diverse species (siTOOLS Biotech).
Approximately 0.1 - 1 μg of total RNA is suggested for standard whole transcriptome library preparation prior to second generation sequencing using the TruSeq Stranded mRNA library prep kit (Illumina), and as low as 25 ng using the newer Stranded mRNA Prep kit (Illumina). Both of these kits capture poly-adenylated mRNA. In many oligotrophic regions, especially deeper in the water column, obtaining high concentrations of RNA is not feasible given sampling and experimental design limitations. Specialized cDNA library preparation kits are compatible with input material as low as 250 pg total RNA in which additional linear amplification cycles are performed, such as with the SMART-Seq v4 Ultra Low Input RNA Kit (Clontech). Although more expensive, the SMART-Seq v4 approach produces similar results to those obtained using larger RNA input (Song et al., 2018) and is a suitable option for low RNA yields from oligotrophic or otherwise low biomass regions of the ocean. Intriguingly, the SMART-Seq protocol appears to capture a non-negligible pool of prokaryotic transcripts despite enrichment for poly-A RNA, although this has not been explicitly tested across library preparation kit methods; a systematic comparison using natural marine communities will be important for determining whether library preparation biases are occurring. Due to these overt differences in resulting sequence libraries between library preparation methods and mRNA enrichment strategies, it is not recommended to mix and match methods in a single experimental dataset.
An alternative aquatic metatranscriptomic approach is sequencing the entire RNA pool. This method provides valuable information on transcriptionally active taxonomic community members through dominant rRNA reads, with limited insights into the functional composition gained through the abundant mRNA captured (McCarren et al., 2010; Shi et al., 2012; Baker et al., 2013; Lanzén et al., 2013; Wu et al., 2013). This method is therefore most appropriate when functional characterization is of secondary importance to taxonomic composition.
Third generation sequencing platforms are gaining in popularity and hold great potential for long read metatranscriptomic sequencing with high accuracy and throughput, without PCR amplification bias or short read assembly challenges (Kerkhof, 2021). In contrast to the Illumina library preparation process in which RNA is required to be converted to cDNA, third generation sequencing technology can sequence RNA molecules directly. These applications to the marine environment are nascent and still being developed, with current limitations including relatively high read error rate and inter-run variability (Semmouri et al., 2020). However, preliminary findings show successful application to marine pelagic zooplankton communities, with high predicted protein content (Semmouri et al., 2020). Metatranscriptomes generated using third generation sequencing platforms will have the added benefit of detecting long 18S rRNA molecules in the sequenced RNA pool, providing a direct assessment of community composition alongside predicted proteins (Semmouri et al., 2020).
After RNA is collected from field sites, extracted in the laboratory, and sequenced, the bioinformatic process begins (Figure 1). Oceanographic research publications are generally required to include field and lab-based methods in enough detail to enable reproducibility. Unfortunately, details regarding bioinformatic tool usage are not always included in study methods, and it can therefore be difficult for others to replicate analyses and determine how each step of the bioinformatic pipeline influences downstream biological interpretations. In addition, many tools and pipelines are not created with microeukaryotes in mind, and special parameters, settings, or considerations may need to be applied. Typically tools are chosen for a specific research question or purpose, meaning that one researcher’s approach may not be suitable in other circumstances. Therefore, disclosing details and reasoning for performing these critical computational steps will help the broader community evaluate the method. It is especially helpful to include a short justification for the method chosen in study descriptions. This transparency will reduce the steep learning curves in computational biology by encouraging such consistent practices and open data sharing policies.
Figure 1 Flow chart of a metranscriptomic pipeline designed for marine microeukaryotes (right). Visual depiction of the main workflow steps, including cell extraction, sequencing, assembly, read mapping, and annotations (left). Example annotations are shown for three phytoplankton taxa (Ellis et al., 2017, Cohen et al., 2017, Wu et al., 2019, Rao, 2020). Note that many predicted proteins do not correspond to a known functional annotation. Example graphics and statistical approaches used to investigate metatranscriptomic output (bottom): Ordinations may be created using orthologous groups or functional annotations aggregated to the taxonomic level of interest. Heatmaps are constructed using normalized gene expression and can be subset to include biostatistically or biologically relevant genes. Heatmaps and ordinations are useful in identifying similarities among sampling groups. 2D section plots show differences in the relative abundance of gene expression across space (or time), highlighting shifts in ecological strategies. MA plots are created using normalized gene expression corresponding to taxonomic/functional annotations or orthologous groups, and are useful in assessing differential expression between pairwise experimental treatments or groupings. Networks are built using normalized gene expression corresponding to taxonomic/functional annotations or orthologous groups, and highlight co-occurring genes. They can be paired with metadata to identify groups of genes correlated with environmental parameters. Stacked barplots represent the relative community composition across samples, and can be produced using raw reads or normalized read counts (e.g., TPM) associated with contigs and/or predicted proteins.
There has been a concerted effort in the biological data science community to adhere to FAIR (findable, accessible, interoperable, reusable) principles in order to improve reproducibility (Garcia et al., 2020), and the oceanographic community is beginning to benefit from the adoption of these practices. In addition to fostering a culture of open data and enabling broader usage of data products, there is tremendous value in making computational code, pipelines, protocols and intermediate products available to new data scientists that are beginning their bioinformatic pursuits.
One avenue for this is sharing analysis and visualization code accompanying published studies on public servers, such as GitHub or personal websites, which is increasingly done by marine microbial ecologists. Code is most useful when annotated to facilitate readability, which is effectively done using rendered reports (Markdown) with RStudio and Jupyter Notebook. Tools such as Binder allow users to work in exact coding environments used to produce data, including loaded pre-requisites and input variables accessible through a website. Documentation in the form of personal blogs that accompany analyses are ideal tools for conveying underlying concepts and detailed thought processes going into analytical decisions, which are valuable educational resources for others in the microbial ecology field (e.g., polarmicrobes.org/antarctica-blog, merenlab.org/posts). Raw sequences are typically required to be uploaded to public repositories before publication, such as the National Center for Biotechnology Information (NCBI) Sequence Read Archive (SRA), and intermediate data files including assemblies, annotations, and read counts can similarly be shared on open-access repositories (e.g., Zenodo). In addition to these avenues for sharing computational resources, laboratory protocols can be archived on websites such as protocols.io, which brings new practitioners up to speed with technical logistics involved in sample acquisition and helps standardize sample processing.
Below we describe a typical metatranscriptomic bioinformatic analysis and provide an example workflow using second generation sequencing (Figure 1 and Supplementary Figure 1). We encourage new users to consult existing code, tutorials, and blog posts compiled by other data scientists to gain familiarity with these computational steps. Ideally, these new users will share code once their analysis is complete, and the oceanographic community as a whole will benefit from the incorporation of these open data science practices.
Once raw sequences are generated, a series of bioinformatic steps are performed to assemble reads into transcripts de novo, quantify gene expression, and assign taxonomic and functional annotations. These steps may be performed individually or as part of an automated workflow in a Linux-based environment, or within a GUI web-based platform (e.g., Galaxy). It is recommended to store all raw files in read-only format in more than one place. Many of the steps in metatranscriptomic analyses are computationally intensive and cannot be performed on the typical laptop or workstation. As such, access to a high performance computing cluster through an institution or national resource (e.g. XSEDE) or access to cloud based compute resources (e.g. Azure, Amazon Web Services) is often necessary. Broadly, the steps of assembly, quantification, and annotation require access to resources, with typical smaller scale projects benefiting from 25-40 cores and 250-400 Gb of RAM, and needs scaling with the size of the dataset and choice of tools.
Raw reads are trimmed to remove poor quality base pairs common on the ends of Illumina reads, and to clip off sequence adapters. Numerous tools are available for trimming sequences, including Trimmomatic (Bolger et al., 2014), FASTX-Toolkit (hannonlab.cshl.edu/fastx_toolkit), or cutadapt (Martin, 2011), with these tools universally compatible with Illumina sequences. The quality of sequences pre- and post-trimming can be evaluated using tools such as FastQC (www.bioinformatics.babraham.ac.uk/projects/fastqc), or evaluated in batch with MultiQC (github.com/ewels/MultiQC), which will additionally summarize total number of reads among other useful metrics. While often an aggressive approach to trimming has been taken, work in single species transcriptomes suggests that a less stringent trimming of only those reads whose Phred sequence quality score <2 or <5 is sufficient and optimal across a variety of downstream metrics (MacManes, 2014).
Non-poly-A RNA or organelle mRNA may have been sequenced along with nuclear mRNA, especially if rRNA depletion was used as the library preparation. Ribosomal RNA is not included in most reference databases, and alignments of these sequences will be patchy and inconsistent. These sequences can be removed prior to the analysis by alignment against reference rRNA sequences using tools such as riboPicker (ribopicker.sourceforge.net), SortMeRNA (Kopylova et al., 2012), or BBDuk within the BBTools suite (jgi.doe.gov/data-and-tools/bbtools). In addition, if used, mRNA spike sequences will be useful for copies L-1 quantification, but do not need to be included in the downstream analysis. Non-target sequences can be removed by aligning against a spike reference fasta file, for example with BBMap sourceforge.net/projects/bbmap/.
Taxonomic and functional interpretations of RNA-Seq data could theoretically be achieved by aligning reads to annotated eukaryotic genomes and metagenomes, however, we lack genomic representatives that cover the extreme diversity found in the natural environment, and de novo assemblies are commonly used instead (Lau et al., 2018). The de novo assembly process relies on elongating individual short reads into contiguous sequences (contigs) using overlapping sequences of length k, or k-mers (Robertson et al., 2010). Popular assemblers for eukaryotic transcriptomes include Trinity (Grabherr et al., 2011), Trans-ABySS (Robertson et al., 2010), rnaSPAdes (Bushmanova et al., 2019), IDBA-Tran (Peng et al., 2013), and MEGAHIT (Li et al., 2015), among others (Ortiz et al., 2021), which differ in their algorithmic basis, relative performance, and the number and length of contigs generated. Although these assemblers were designed for metagenomics (MEGAHIT) or transcriptomics (Trans-ABySS, Trinity, rnaSPAdes, IDBA-Tran), they are largely compatible with environmental metatranscriptomes (Lau et al., 2018) and eukaryotic sequences (Ortiz et al., 2021), with rnaSPAdes and Trinity performing particularly well for marine microeukaryotic communities in terms of the number of high quality and annotatable contigs produced (Krinos et al., 2022).
Quality-trimmed reads should be used as input during the assembly process, with some assemblers capable of handling multiple pairs of reads (co-assembling), depending on computational constraints. For large datasets, individual assemblies can be generated from each set of reads, which could then be merged together and de-replicated by clustering at high sequence similarity (95-100%) using tools such as cd-hit (Li and Godzik, 2006) or MMseqs2 (Steinegger and Söding, 2017; Krinos et al., 2022). Broadly, assembly metrics (e.g. percent mapped reads) improve with a multi-assembler approach in which assembly output from different tools are combined (Ortiz et al., 2021; Krinos et al., 2022). In this case, the resulting assembly will contain contigs generated from all communities sampled and different assembler algorithms used. De novo assembling with metatranscriptomic reads from closely related taxa may inadvertently introduce spurious and/or chimeric contigs, in which reads from different transcripts are combined and would not reflect true protein pools. Certain transcriptomic assemblers are better at controlling chimeric contigs than others, with Trans-ABySS and IDBA-Tran stable across a range of k-mers (Wang and Gribskov, 2016).
Third generation sequencing reads can be assembled to produce high quality, long contigs using de novo assemblers such as metaFlye, which take into consideration challenges specific to long reads, such as uneven coverage among species sequenced (Kolmogorov et al., 2020). The informatic methodology will need to be reconsidered as third generation sequencing becomes more commonly applied and directly compared to older, short read-based studies. In particular, hybrid assemblies combining short and long reads benefit from the throughput/low error rates of short sequences and added structural information provided by long sequences (Prjibelski et al., 2020). This capability has been recently built into the MUFFIN metagenomic workflow (van Damme et al., 2021) and the rnaSPAdes assembler (Prjibelski et al., 2020), with the rnaSPAdes hybrid approach successfully applied to marine phytoplankton in culture (Sperfeld et al., 2021).
Trimmed reads can be aligned to the de-replicated, final assembly to estimate gene expression across samples. Alternatively, reads may be aligned to assemblies generated from individual samples, although relative comparisons across samples could then only be made at the fold change or gene ratio level. When aiming to draw direct comparisons in community composition and gene expression across a dataset, it is simplest to align to the same reference assembly, so that reads are allowed to align to the closest possible contig match, rather than only those contigs assembled from an individual sample.
Read mapping can be performed using traditional alignment tools such as Bowtie2 (Langmead and Salzberg, 2012) or BWA (Li and Durbin, 2009) which map short reads against a reference transcriptome or genome. Newer alignment options include Salmon (Patro et al., 2017) and Kallisto (Bray et al., 2016) which use quasi-mapping and pseudo-alignment approaches, respectively, rather than base-to-base alignments, performing rapid alignments while preserving high sensitivity and accuracy.
In addition to mapping reads to the de novo assembly, another option is to map metatranscriptomic reads to transcriptome and genome references directly, for example the MMETSP database. This method could identify community members present that closely match cultured species or strains of interest without the effort of de novo assembling, and can capture organisms that failed to assemble into high quality contigs (Alexander et al., 2015a; Metegnier et al., 2020; Harke et al., 2021; Muratore et al., 2022). However, is it important to note that current reference transcriptome databases are limited relative to the tremendous taxonomic diversity of eukaryotes in seawater (de Vargas et al., 2015), and this approach could therefore miss key taxa that are present.
Transcript abundance can be estimated using mRNA standards, as developed for marine prokaryotes (Satinsky et al., 2013; Gifford et al., 2014; Satinsky et al., 2017). In order to determine how much standard to add prior to sample extraction, test filters can be collected and sacrificed from representative stations/depths with differing levels of biomass (chlorophyll a fluorescence). Conversely, standards can be added after RNA is extracted, although this “quasi-standard” would no longer take into account RNA loss during the extraction procedure, and would lead to an overestimate of mRNA abundance.
Transcript abundances can be normalized by the volume filtered to approximate copies L-1:
where readsi are the raw read counts of transcript i, spike reads are the raw read counts associated with the mRNA spike standard, spike copies are the amount of mRNA copies (molecules) added to samples prior to sequencing, total reads are the number of mRNA reads in the sequence library (minus spike reads), and volume filtered is the amount of seawater filtered. Normalized counts may be used (e.g., TPM) in place of raw read counts in these absolute transcript calculations, which would account for transcript length bias (Mortazavi et al., 2008; Bartholomäus et al., 2016).
Proteins are predicted from assembled contigs (transcripts) through open reading frame (ORF) prediction software, such as TransDecoder (transdecoder.github.io) or GeneMark S-T (Tang et al., 2015). If using the metatranscriptome as a reference for a metaproteomic peptide-spectrum-matching (PSM), the ORF predictions are the FASTA database used for PSM scoring (Cohen et al., 2021).
Assigning taxonomic annotations to contigs allows for examinations into community composition across spatial and temporal gradients, or as a function of experimental perturbations. Taxonomic assignments are performed by aligning assembled contig sequences against a reference database. For identifying marine microeukaryotes in the field, it is key to use a database that includes transcriptomes or genomes from laboratory isolates or environmentally derived single-cell marine microeukaryotes. The MMETSP database includes 678 marine microeukaryote transcriptomes, including phylogenetically diverse and ecologically relevant taxa (Keeling et al., 2014; Johnson et al., 2019). Databases including MMETSP references such as EukProt (Richter et al., 2020), EukZoo (github.com/zxl124/EukZoo-database), and PhyloDB (allenlab.ucsd.edu/data) are valuable resources with additional transcriptomes and genomes present. Sequences may be aligned to these databases using tools such as BLAST (Altschul et al., 1990), DIAMOND (Buchfink et al., 2015), and MMseqs2 (Steinegger et al., 2017), with quality thresholds commonly used to assess goodness of fit including E-value cutoffs (e.g., E < 10-5), high sequence similarity percentage, and/or high bit score. EUKulele is a taxonomic annotation tool designed for marine microeukaryotes, and can perform BLAST or DIAMOND alignment searches against custom or default databases, including MMETSP (Krinos et al., 2021). For contigs with numerous hits exceeding a quality threshold, a Least Common Ancestor (LCA) approach is used to assign annotations at the lowest common taxonomic level. It is recommended to include marine bacteria, metazoa, and common contaminants in the databases for purposes of identifying these community members, if present, and avoiding misannotation. For example, the default EUKulele database includes the MarRef database of marine prokaryotic genomes (Klemetsen et al., 2018; Krinos et al., 2021). In addition, using databases containing reference transcriptomes that have been screened for multi-species presence and decontaminated are preferred (Van Vlierberghe et al., 2021).
It is crucial to remember, however, that we are ultimately limited in identifying taxonomy by what is available in our databases. If eukaryotic organisms captured in field studies are unrelated to cultured representatives included in taxonomic databases, with sufficiently low sequence similarity, they will be unannotated or misannotated. MMETSP has expanded sequence coverage of diatoms, prasinophytes, and dinoflagellates (Keeling et al., 2014), but a large number of ecologically relevant taxa remain missing from reference databases, especially those from the depths of the ocean where protistan diversity is quite high (Schoenle et al., 2021). Testing multiple databases containing different protistan reference organisms may be helpful during initial explorations of datasets. Generally, ~40-60% of marine metatranscriptomic contigs can be assigned a taxonomic annotation (e.g., Cohen et al., 2017; Carradec et al., 2018; Hu et al., 2018). The gene expression profiles captured with metatranscriptomics will represent a mixed community cellular state and the community’s dominant response to the environment. Considering the vast taxonomic and metabolic diversity across microeukaryotic species, limited reference databases may not fully capture environmentally-relevant features. Moving forward, focused efforts on sequencing additional diverse organisms from rarer lineages and further populating reference databases will improve field identifications.
Functional annotation of transcripts enables investigations into ecophysiology, resource exchanges between organisms and the environment, and contributions to elemental cycling. Similar to taxonomic assignments, functions can be assigned via sequence homology searches to protein databases including eukaryotic references such as Kyoto Encyclopedia of Genes and Genomes (KEGG) (Kanehisa et al., 2016a), Eukaryotic Ortholog Groups (KOG) (Tatusov et al., 2003), and Gene Ontology (GO) (Gene Ontology Consortium, 2004) using likelihood thresholds (e.g., E < 10-3). These databases have the advantage of grouping proteins by higher order function, allowing for broad classification into functional categories. In addition to these approaches, hidden Markov model (HMM) profiles searches executed via the HMMer software suite (Finn and Clements, 2011) in conjunction with protein databases [e.g., Pfam (Finn et al., 2014)] enable the identification of conserved protein domains, which can provide more remote information compared to local alignments. However, these conserved domains are not indicative of whole protein function and are not as easily classified into broader functional categories.
Certain existing tools and workflows perform combinations of the taxonomy and functional annotation steps listed above. For example, eggNOG-mapper provides KEGG, GO, Pfam, and other protein annotations as output using HMMer, DIAMOND, or MMSeqs2 searches against the eggNOG database (Cantalapiedra et al., 2021). KOfamScan searches query sequences against the KEGG database using HMM profiles (github.com/takaram/kofam_scan). Trinotate is a workflow for non-model organisms that carries out assemblies with Trinity and annotates using eggNOG, GO, and Pfam, among other databases (Bryant et al., 2017). Web-based servers are also available (BlastKOALA, GhostKOALA, KofamKOALA) which annotate assembly fasta files against the KEGG database using sequence homology or HMM profile searches, in a web user interface (Kanehisa et al., 2016b). Users may choose an annotation procedure that aligns with their needs, level of automation desired, and preferred computational environment.
Sequence libraries are commonly normalized to account for non-biological variability (e.g., large differences in total number of reads among samples, or library sizes) thereby allowing for relative comparisons across a dataset (Abrams et al., 2019). Popular RNA-Seq normalization and differential expression tools include EdgeR (Robinson and Oshlack, 2010; Robinson et al., 2010) and DESeq2 (Anders & Huber, 2010; Love et al., 2014), which correct for RNA composition bias during normalization, and estimate differential expression by fitting generalized linear models and assuming negative binomial distributions. False discovery rate (FDR) controlled p-values are used to account for multiple hypothesis testing within large gene expression datasets (Reiner et al., 2003). EdgeR and DESeq2 are ideal for comparative analyses in which similar marine communities are subjected to treatments or conditions in order to address experimental hypotheses. These software tools offer dispersion shrinkage estimation and outlier detection to decrease the uncertainty of differential expression annotations (Love et al., 2014). It is important to note that applying differential expression tools for community-level metatranscriptomic data requires the organization of these data into taxon-specific bins for the purposes of scaling the count matrix, thereby isolating taxon-specific gene expression changes (Klingenberg and Meinicke, 2017).
These approaches make key assumptions about most genes not being differentially expressed, and care should be taken when comparing disparate marine ecosystems (e.g., surface and deep populations, or surface waters from eastern and western ocean basins). Normalization approaches that merely account for changes in sequence library and differences in contig length, such as Transcripts Per Million (TPM) (Wagner et al., 2012), may be better suited for large spatial or temporal field studies, although these methods cannot provide statistical estimates of differential expression and may retain transcript composition biases (Cockrum et al., 2020; see below: Consolidating assemblies). Flexible and adaptive statistical techniques that take into consideration zero-inflation, such as Tweedie models, may be useful for metatranscriptomic analyses and are worth exploring in marine omic datasets (Mallick et al., 2021).
As evident from the above descriptions of typical metatranscriptomic pipelines, many individual computational steps are required. Workflow managers are valuable for the batch execution of commands and processing steps across samples. Individual executions may otherwise be tedious, lead to inconsistencies and/or errors, and fail to be reproducible. Of the open-source workflow managers, Snakemake is a popular Python-based user-friendly option with rich documentation available (Köster and Rahmann, 2012). Many established Snakemake-based workflows are publicly available on code sharing repositories such as GitHub, and may be a useful starting point. For example, eukrhythmic is a scalable metatranscriptomic assembly workflow designed for marine microeukaryotes (Krinos et al., 2022), and many of the above outlined bioinformatic steps have been incorporated into eukrhythmic (github.com/AlexanderLabWHOI/eukrhythmic). In addition, the SqueezeMeta analysis pipeline is suitable for metatranscriptomic assembly and compatible with long read sequences (Tamames and Puente-Sánchez, 2019).
It can be difficult to interpret large assemblies in which tens of millions of contigs are produced from mixed natural communities and most contigs do not correspond to a known protein function. There are several considerations for consolidating assemblies and streamlining the analysis, including orthologous group clustering and functional/taxonomic aggregation.
A subset of assembled metatranscriptomic contigs do not encode proteins due to being fragmented and/or chimeric, and this can be a function of RNA pool composition, sequencing depth, type of assemblers used, and protein prediction algorithms and parameters applied. It can therefore be helpful to remove non-coding sequences by predicting ORFs, and strictly using ORFs for read mapping and the quantitative analysis of taxonomic and functional groups. Orthologous groups (OGs) are commonly used to further collapse proteins into protein clusters derived from common ancestors, performed using tools such as OrthoFinder (Emms and Kelly, 2019), which uses a phylogenetic approach with multiple algorithms to identify relationships between genes and species. In contrast, OrthoMCL uses a reciprocal best hits approach with the Markov Clustering Algorithm (MCL) (van Dongen 2000; Li et al., 2003). These OGs can be used to track changes in function among closely related protistan taxa. However, one consideration for OG clustering is that it is highly dependent on clustering thresholds (e.g., percent sequence similarity), with stringent parameters limiting cross-species aggregation, and very lax parameters enabling cross-species clustering (Krinos et al., 2022). OG aggregation may be particularly valuable for collapsing read counts into evolutionarily related taxonomic groups and functional genes, effectively reducing the large assembly into more biologically meaningful groupings.
Aside from OG clustering, another popular approach for condensing assemblies has been to aggregate read counts to a common taxonomic and functional classification. For example, combining reads to the supergroup, class, or family level and KEGG Orthology ID. Read counts can either be collapsed (summed) into taxonomic and functional annotations before normalization, or summed following normalization. Transcript-estimating tools such as tximport can automate this step by converting read counts associated with transcripts (contigs) to gene level annotations and generating normalized reads as community-wide TPM (Soneson et al., 2015). An important consideration is the taxonomic level of interest in which to aggregate counts, and will differ depending on research focus. The primary disadvantage of working at a fine taxonomic resolution (e.g., species) is that fewer sequences can be confidently assigned to such a level, and users may be inundated by the overwhelming number of species to analyze. On the other hand, broad class level annotations (e.g., diatoms, dinoflagellates, haptophytes) are a composite of vastly different organisms, some of which may be responding to the environment in distinct ways (Alexander et al., 2015a; Lampe et al., 2018). Testing various taxonomic annotation levels can be helpful in determining the resolution most biologically meaningful for a given dataset. This approach relieves concern about mixing different species or genera into one group, as may occur with OG clustering, and provides taxon-specific counts. However, a key issue is that a significant fraction of coding sequences do not have a known protein function (low sequence similarity to references in databases), similar to observations with assembled genomes and metagenomes (Vanni et al., 2021). This approach therefore limits biological interpretations to only a fraction of the predicted proteins included in the downstream analysis.
When working with experimental treatments or pairwise comparative conditions, MA (log ratio-mean average) plots are a straightforward approach for visualization, highlighting highly abundant and variably expressed genes in specific taxa of interest (Supplementary Figure 2). These are created by plotting log fold change by average normalized gene expression, and can be generated directly through EdgeR and DESeq2 packages. In addition, MANTA plots are a useful way to depict the MA relationship with pie charts representing the relative breakdown of taxonomic composition for each functional annotation (see github.com/AlexanderLabWHOI/2022-metaT-m3-perspective for code). Fold change comparisons are ideal for visualizing the magnitude of change in expression across samples, which can be difficult to discern from normalized transcript abundance alone. Similarly, volcano plots highlight differentially expressed genes with statistical support by graphing fold change by FDR (Supplementary Figure 2). Datasets from spatial and temporal studies may also be averaged across time or space zonations to visualize in MA plots, for example by averaging gene expression across all surface and deep communities. However, these broad groupings may conceal biologically relevant patterns across environmental features. In conjunction with these, exploratory approaches may be used to find structure across latitudes and depth, including ordinations, clustered heatmaps, and network analyses.
Ordinations are used to distill multidimensional data into two or three dimensions for visualization. Omic data is highly dimensional, with the number of annotated genes commonly reaching the thousands. Popular unconstrained ordination approaches include Principal Component Analysis (PCA) based on Euclidean distance, Principal Coordinate Analysis (PCoA) compatible with other forms of dissimilarity measurements, and Nonmetric Multidimensional Scaling (NMDS) which ranks distances in nonlinear space iteratively (Ramette, 2007). With these unconstrained ordinations, the relationship between metadata and ordination space can be assessed, for example using the envfit function of vegan (Dixon, 2003). Constrained ordinations such as Canonical Correlation Analysis (CCA) and Redundancy Analysis (RDA) can be used to evaluate how transcriptional profiles change across sample groups according to metadata, such as environmental measurements or categorical variables (Ramette, 2007). (See github.com/AlexanderLabWHOI/2022-metaT-m3-perspective for example CCA ordination tutorial). Broadly, these approaches can be used to find similarities in gene expression profiles across sample types and/or relate transcript levels to environmental conditions. Ordination plots have been utilized to show time-dependent patterns in microeukaryote gene expression (Groussman et al., 2021), taxon-specific microeukaryote functional profiles (Hu et al., 2018), and differences in surface and deep dinoflagellate metatranscriptome profiles (Cohen et al., 2021). The input data for ordinations may be normalized read counts associated with annotated or unannotated transcripts at the community level (e.g., community-wide TPM), or normalized at a given taxonomic level of interest (e.g., haptophytes).
Gene expression profiles can be effectively compared across samples using heatmaps, clustered using a statistical similarity metric and highlighting relationships among treatment groups, ocean regions, or temporal dimensions. Typically, columns represent individual samples, rows represent genes, and colors represent normalized read counts. These heatmaps can include all genes within the expression profile or subset to highlight specific genes of interest. Care should be taken when subsetting expression profiles to include differentially expressed genes, because in the absence of biostatistical tests, differential expression cannot be reliably determined. Transcripts with the highest variances can be calculated, but this will be biased towards highly expressed genes since variance increases with transcript abundance in a heteroscedastic manner (Law et al., 2014). It is therefore recommended to correct for the mean-variance relationship (as performed by EdgeR and DESeq2) or perform a transformation such as log normalization; however, log-normalizing prior to calculating variance can have the opposite effect and result in high variances of lowly expressed genes. Normalizations that effectively control for heteroscedasticity, such as the variance stabilizing transformation, are recommended to improve differential expression assessments (Zwiener et al., 2014).
Marine metatranscriptomic datasets can be quite large, consisting of billions of reads, millions of contigs, and thousands of annotated genes. Network analyses can be used to identify ecological connections between taxonomic groups, associations among functional processes, and/or link environmental metadata with expression patterns. This approach has been used to characterize the nutrient status of phytoplankton along an estuary gradient (Gong et al., 2018), identify diel metabolic patterns across protists (Kolody et al., 2019), and reveal co-occurrences between limiting resources and protistan taxa (Caputi et al., 2019). Ecological network analyses are frequently performed with Weighted Gene Correlation Network Analysis (WGCNA) (Langfelder and Horvath, 2008), igraph (Csardi and Nepusz, 2006), and sparCC (Friedman and Alm, 2012), with Cytoscape software commonly used for visualization of networks and interactions following creation (Shannon et al., 2003). Input data may be annotated transcripts with taxonomic and functional assignments and normalized read counts at the community level, and output is clusters or modules of closely related (co-occurring) associations defined by user-specified thresholds. These modules can be correlated with metadata to understand environmental conditions influencing taxonomic and/or metabolic processes. In particular, KEGG or GO-enrichments can be performed on modules to expand on how functional profiles are biologically, spatially or temporally organized.
Section plots, or 2D maps, are used to visualize changes in relative (normalized) or quantitative (copies L-1) gene expression across lateral and vertical expanses. This type of visualization is especially useful if high resolution sampling along transects was performed. Section plots can be generated using matplotlib library in Python (Saito et al., 2020), oce package in R (dankelley.github.io/oce), or software such as Ocean Data View (Schlitzer, 2018). This visualization method can highlight shifts in metabolic processes across depths, nutrient conditions, temperature regimes, or biomes (e.g., Saito et al., 2014; Santoro et al., 2017; Hogle et al., 2021). Highlighted genes may include those with strongly varying expression between zonations, or individual genes of interest hypothesized to be comparatively responsive to environmental conditions, such as biomarkers of nutrient stress (Saito et al., 2014). It is recommended to be cautious when interpreting weak and/or variable signals from genes that are not highly expressed, especially when biological replication is not available.
As discussed above, the learning curve associated with bioinformatics can be steep, and many resources exist to aid in the process. The Carpentries is an international organization that teaches foundational coding principles to learners of any background, with educational materials publicly available online (Wilson, 2006). Their lessons can equip users with basic knowledge of Unix, Python, and R, with more advanced concepts also available, including genomics, ecology and graphical visualizations (datacarpentry.org/lessons, software-carpentry.org/lessons). Additionally, universities with high performance computing clusters commonly have coding training sessions available to users. Specifically for microbiologists, the community initiative Bioinformatics Virtual Coordination Network (BVCN) aims to teach core coding and bioinformatic skills needed for omic analyses through publicly accessible lessons, tutorials, and video content available online (Tully et al., 2021). Lastly, it is key to learn how to effectively troubleshoot while coding, and user-based discussion boards are excellent venues for discovering how others have solved similar problems, asking questions, and sharing solutions. Biostars.org, Stackoverflow.com, and Github Issues have been invaluable community resources during our own bioinformatic development.
The guidelines and considerations presented here reflect the current methodology used to analyze microeukaryotic metatranscriptomes, but approaches will evolve as new tools, pipelines and databases come online. Users are encouraged to keep up with new versions of released databases and tools, and check documentation often to incorporate updates in computational steps (i.e., improved efficiency or accuracy, bug-fixes). In particular, focused community efforts on transcriptome sequencing of phylogenetically diverse protists will improve metatranscriptomic databases and strengthen our ability to track natural populations in the field. Single-cell transcriptomics (sc-RNA-Seq) performed on uncultured organisms isolated from the field will be especially valuable in expanding taxonomic and functional sequence coverage of microeukaryotes from complex communities (Wilms, 2021; Kolody et al., 2022).
Importantly, third generation sequencing technologies are promising avenues for microbial ecology that have rapidly developed over the past few years. Nanopore sequencing via the portable MinION and benchtop promethION platforms (Oxford Nanopore Technologies) and PacBio Single-Molecule Real-Time (SMRT) sequencing (Pacific Biosciences of California) will enable full length transcripts to be sequenced from mixed natural assemblages, without PCR amplification, short-read assemblies, and associated biases. Existing bioinformatic workflows will need to be updated to accommodate this new sequencing technology, as many publicly available workflows are currently designed for short read sequencing products obtained through Illumina technology. Increasingly, protocols are being developed for a combination of short and long read transcriptome sequencing of marine phytoplankton (Sperfeld et al., 2021).
The protocols outlined here have been compiled from individual studies, which are the result of years of experience at sea, in labs, and on the command line. As discussed above, there are multiple options for carrying out each step of the process, from seawater collection to bioinformatic handling. We currently lack an understanding of how the different, yet congruent, methods influence the downstream analyses and biological interpretations. A community intercalibration exercise in which multiple research groups analyze the same initial natural community using their various preferred sampling, laboratory and computational pipelines would advance our understanding of how methodology influences results, and would increase confidence in chosen practices. This is one of the goals recently discussed at the “Ocean Nucleic Acids Omics Intercalibration and Standardization” workshop funded by the National Science Foundation’s Ocean Carbon & Biogeochemistry (OCB) Program (Berube et al., 2022). The workshop report provides a roadmap for such activities, including intercalibration efforts for a variety of marine omics applications, with additional sampling and processing considerations not covered here (Berube et al., 2022). Such activities are critical for the development of future coordinated large oceanographic international programs, such as BioGeoSCAPES (www.biogeoscapes.org).
Valuable insights have been provided by microeukaryote metatranscriptomic studies to date, and exciting discoveries certainly await us in the coming years as the approach continues to be applied to diverse ecosystems and integrated with complementary methods. A eukaryotic gene catalog consisting of over 116 million expressed genes has been recently generated from the ocean, expanding our understanding of protistan biogeography and physiology as a function of environmental conditions (Carradec et al., 2018; Caputi et al., 2019). Intriguingly, half of the gene functions are not annotated, and targeted efforts into protein characterization will drastically improve our understanding of protistan ecology and their biogeochemical roles (Carradec et al., 2018). There is furthermore value in combining marine metatranscriptomics with traditional physiological and chemical approaches (Marchetti, 2019; Wilms, 2021; Kolody et al., 2022), which can strengthen and guide biogeochemical interpretations, for example in relating metatranscriptomic community composition to microscope-derived cell counts or gene expression to empirically measured enzymatic rates. Integration of metatranscriptomic information with metaproteomics enables a broader view of protistan cell metabolism (Cohen et al., 2022), with recent modeling efforts offering a mechanistic understanding of the relationships between environmentally responsive transcripts and proteins in marine microbes (Walworth et al., 2022). Similar pairings with metabolomics and/or lipidomics hold promise for relating taxonomic groups and metabolic processes to released organics, revealing trophic interactions among community members and nutrient utilization patterns (Durham et al., 2015; Heal et al., 2021; Muratore et al., 2022). Continuing to integrate and apply metatranscriptomics to biogeochemically complex and understudied marine ecosystems will undoubtedly shed light on the diverse roles marine protists play across our ocean biomes.
NC, HA, and AK conceived of the review. SH and RL generated coding resources. NC wrote the first draft. All authors contributed to the intellectual content, writing, and revising of the manuscript. All authors approved the submitted version.
We acknowledge funding support from the University of Georgia Skidaway Institute of Oceanography (to NRC), National Science Foundation (NSF) (OCE-1948025 to HA), and Department of Energy Computational Science Graduate Fellowship (DE-SC0020347 to AIK). SKH participation was supported through NSF OCE-1947776.
The authors declare that the research was conducted in the absence of any commercial or financial relationships that could be construed as a potential conflict of interest.
All claims expressed in this article are solely those of the authors and do not necessarily represent those of their affiliated organizations, or those of the publisher, the editors and the reviewers. Any product that may be evaluated in this article, or claim that may be made by its manufacturer, is not guaranteed or endorsed by the publisher.
We graciously thank Adrian Marchetti (UNC) for valuable feedback on the manuscript.
The Supplementary Material for this article can be found online at: https://www.frontiersin.org/articles/10.3389/fmars.2022.867007/full#supplementary-material
Supplementary Figure 1 | Example research questions and analysis recommendations. The standard bioinformatic workflow is used to produce the intermediate products (assembly, annotations, read mappings, etc.), but normalization and visualization strategies differ depending on research question. *Note that either non-normalized raw reads or community-wide TPM may be used for relative community composition visualization with stacked barplots, but normalized counts (taking into account differences in library sizes) is recommended for ordinations.
Supplementary Figure 2 | Example MA and volcano plots for visualizing differential expression. MA plot (A): MA plot shows the mean fold change versus mean expression with both measures on a log2 scale. Genes above the horizontal dashed line represent genes with increased expression in one treatment (numerator in the fold change calculation) and genes below represent increased expression in the other treatment (denominator in the fold change calculation). Additional modifications can aid in visualizing differentially expressed genes. For example, this plot shows genes that are not significantly differentially expressed as gray points while genes that are significantly differentially expressed are black points (P < 0.05). Data points with extremely high fold change values are plotted as triangles rather than circles. In this example, those are genes with log2 fold change values greater than 5 or less than -5. The size of the points is scaled by -log10(p-value) such that lower p-values are larger points. Although not shown here, genes of interest can be color-coded and/or labeled directly if desired. One simple and popular coloration is to show the significantly up- or down-regulated genes as two different colors, for example, red and blue respectively. MANTA plot (B): This plot is a modification of the MA plot and was introduced by Marchetti et al., 2012. The original plotting function was released as part of the manta (Microbial Assemblage Normalized Transcript Analysis) package in Bioconductor; however, use of this package to generate plots restricts users to use MANTA objects and lacks features such as the ability to handle replicates. As a result, new plotting functions inspired by the original were re-written and are available on Github (github.com/AlexanderLabWHOI/2022-metaT-m3-perspective). Here, genes that are significantly differentially expressed are shown as pie charts rather than plain black circles to display that taxonomic breakdown of the genes. This example uses the same data as the MA plot example, but now, different diatom genera are shown. Depending on the user’s analysis, different groups and/or taxonomic-levels can be used. The use of the triangles as shown in the MA plot was omitted for this plot, but extremely high log2 fold change values are 5 or -5. Volcano plot (C): Rather than highlighting the mean abundance of a gene as in the MA plot, the volcano plot emphasizes the statistical significance (p-value) of genes between two treatments. Here, genes to the right of the horizontal dashed line represent genes with increased expression in one treatment (numerator in the fold change calculation) and genes to the left represent increased expression in the other treatment (denominator in the fold change calculation). Only genes above the horizontal dashed line, -log10(0.05) representing α = 0.05, are shown in black while genes below it are shown in gray to distinguish between points that are significantly differentially expressed or not as in the MA plot. Data points with extremely low p-values and thus are high on the y-axis are plotted as triangles rather than circles. In this example, those are genes with P < 0.00001. As with the MA plot, additional color coding may be performed and may be as simple as coloring all the genes in the left treatment blue and genes in the right treatment red. Genes of interest can also be color-coded and/or labeled directly if desired.
Abrams Z. B., Johnson T. S., Huang K., Payne P. R. O., Coombes K. (2019). A Protocol to Evaluate RNA Sequencing Normalization Methods. BMC Bioinf. 20, 679. doi: 10.1186/s12859-019-3247-x
Alberti A., Poulain J., Engelen S., Labadie K., Romac S., Ferrera I., et al. (2017). Viral to Metazoan Marine Plankton Nucleotide Sequences From the Tara Oceans Expedition. Sci. Data 4, 170093. doi: 10.1038/sdata.2017.93
Alexander H., Hu S. K., Krinos A. I., Pachiadaki M., Tully B. J., Neely C. J., et al. (2021). Eukaryotic Genomes From a Global Metagenomic Dataset Illuminate Trophic Modes and Biogeography of Ocean Plankton. bioRxiv doi: 10.1101/2021.07.25.453713
Alexander H., Jenkins B. D., Rynearson T. A., Dyhrman S. T. (2015a). Metatranscriptome Analyses Indicate Resource Partitioning Between Diatoms in the Field. Proc. Natl. Acad. Sci. 112, E2182–E2190. doi: 10.1073/pnas.1421993112
Alexander H., Rouco M., Haley S. T., Wilson S. T., Karl D. M., Dyhrman S. T. (2015b). Functional Group-Specific Traits Drive Phytoplankton Dynamics in the Oligotrophic Ocean. Proc. Natl. Acad. Sci. 112, E5972–E5979. doi: 10.1073/pnas.1518165112
Altschul S. F., Gish W., Miller W., Myers E. W., Lipman D. J. (1990). Basic Local Alignment Search Tool. J. Mol. Biol. 215, 403–410. doi: 10.1016/S0022-2836(05)80360-2
Alvarez M., Schrey A. W., Richards C. L. (2015). Ten Years of Transcriptomics in Wild Populations: What Have We Learned About Their Ecology and Evolution? Mol. Ecol. doi: 10.1111/mec.13055
Ambardar S., Gupta R., Trakroo D., Lal R., Vakhlu J. (2016). High Throughput Sequencing: An Overview of Sequencing Chemistry. Indian J. Microbiol. 56, 394–404. doi: 10.1007/s12088-016-0606-4
Anders S., Huber W. (2010). Differential Expression Analysis for Sequence Count Data. Genome Biol. 11, R106. doi: 10.1186/gb-2010-11-10-r106
Baker B. J., Sheik C. S., Taylor C. A., Jain S., Bhasi A., Cavalcoli J. D., et al. (2013). Community Transcriptomic Assembly Reveals Microbes That Contribute to Deep-Sea Carbon and Nitrogen Cycling. ISME J 7, 1962–73. doi: 10.1038/ismej.2013.85
Bartholomäus A., Fedyunin I., Feist P., Sin C., Zhang G., Valleriani A., et al. (2016). Bacteria Differently Regulate mRNA Abundance to Specifically Respond to Various Stresses. Phil. Trans. R. Soc. A 374: 20150069. doi: 10.1098/rsta.2015.0069
Becker K. W., Harke M. J., Mende D. R., Muratore D., Weitz J. S., DeLong E. F., et al. (2021). Combined Pigment and Metatranscriptomic Analysis Reveals Highly Synchronized Diel Patterns of Phenotypic Light Response Across Domains in the Open Oligotrophic Ocean. ISME J. 15, 520–533. doi: 10.1038/s41396-020-00793-x
Bertrand E. M., McCrow J. P., Moustafa A., Zheng H., McQuaid J. B., Delmont T. O., et al. (2015). Phytoplankton-Bacterial Interactions Mediate Micronutrient Colimitation at the Coastal Antarctic Sea Ice Edge. Proc. Natl. Acad. Sci. USA 112, 9938–9943. doi: 10.1073/pnas.1501615112
Berube P., Gifford S., Hurwitz B., Jenkins J., Marchetti A., Santoto A. (2022). Roadmap Towards Communitywide Intercalibration and Standardization of Ocean Nucleic Acids ‘Omics Measurements. doi: 10.1575/1912/28054
Biller S. J., Berube P. M., Dooley K., Williams M., Satinsky B. M., Hackl T., et al. (2018). Marine Microbial Metagenomes Sampled Across Space and Time. Sci. Data 5, 180176. doi: 10.1038/sdata.2018.176
Blaxter M., Mieszkowska N., Di Palma F., Holland P., Durbin R., Richards T., et al. (2022). Sequence Locally, Think Globally: The Darwin Tree of Life Project. Proc. Natl. Acad. Sci. 119. doi: 10.1073/pnas.2115642118
Bolger A. M., Lohse M., Usadel B. (2014). Trimmomatic: A Flexible Trimmer for Illumina Sequence Data. Bioinformatics 30, 2114–2120. doi: 10.1093/bioinformatics/btu170
Bray N. L., Pimentel H., Melsted P., Pachter L. (2016). Near-Optimal Probabilistic RNA-Seq Quantification. Nat. Biotechnol. 34, 525–527. doi: 10.1038/nbt.3519
Breier J. A., Jakuba M. V., Saito M. A., Dick G. J., Grim S. L., Chan E. W., et al. (2020). Revealing Ocean-Scale Biochemical Structure With a Deep-Diving Vertical Profiling Autonomous Vehicle. Sci. Robot. 5. doi: 10.1126/scirobotics.abc7104
Bryant D. M., Johnson K., DiTommaso T., Tickle T., Couger M. B., Payzin-Dogru D., et al. (2017). A Tissue-Mapped Axolotl De Novo Transcriptome Enables Identification of Limb Regeneration Factors. Cell Rep 18(3):762–76. doi: 10.1016/j.celrep.2016.12.063
Buchfink B., Xie C., Huson D. H. (2015). Fast and Sensitive Protein Alignment Using DIAMOND. Nat. Methods 12, 59–60. doi: 10.1038/nmeth.3176
Bushmanova E., Antipov D., Lapidus A., Prjibelski A. D. (2019). Rnaspades: A De Novo Transcriptome Assembler and Its Application to RNA-Seq Data. Gigascience 8. doi: 10.1093/gigascience/giz100
Bush S. J., McCulloch M. E. B., Summers K. M., Hume D. A., Clark E. L. (2017). Integration of Quantitated Expression Estimates From polyA-Selected and rRNA-Depleted RNA-Seq Libraries. BMC Bioinf. 18, 301. doi: 10.1186/s12859-017-1714-9
Cantalapiedra C. P., Hernández-Plaza A., Letunic I., Huerta-Cepas J. (2021a). eggNOG-Mapper V2: Functional Annotation, Orthology Assignments, and Domain Prediction at the Metagenomic Scale. Mol. Biol. Evol.. doi: 10.1093/molbev/msab293
Caputi L., Carradec Q., Eveillard D., Kirilovsky A., Pelletier E., Pierella Karlusich J. J, et al. (2019). Community-Level Responses to Iron Availability in Open Ocean Planktonic Ecosystems. Global Biogeochem. Cycle 33(3) 391–419. doi: 10.1029/2018GB006022
Caron D. A., Alexander H., Allen A. E., Archibald J. M., Armbrust E. V., Bachy C., et al. (2017). Probing the Evolution, Ecology and Physiology of Marine Protists Using Transcriptomics. Nat. Rev. Microbiol 15, 6–20. doi: 10.1038/nrmicro.2016.160
Carradec Q., Pelletier E., Da Silva C., Alberti A., Seeleuthner Y., Blanc-Mathieu R., et al. (2018). A Global Ocean Atlas of Eukaryotic Genes. Nat. Commun. 9, 373. doi: 10.1038/s41467-017-02342-1
Cerdan-Garcia E., Baylay A., Polyviou D., Woodward E. M. S., Wrightson L., Mahaffey C., et al. (2021). Transcriptional Responses of Trichodesmium to Natural Inverse Gradients of Fe and P Availability. ISME J 16, 1055–64. doi: 10.1038/s41396-021-01151-1
Chen L., Yang R., Kwan T., Tang C., Watt S., Zhang Y., et al. (2020). Paired rRNA-Depleted and polyA-Selected RNA Sequencing Data and Supporting Multi-Omics Data From Human T Cells. Sci. Data 7, 376. doi: 10.1038/s41597-020-00719-4
Clementson L. A., Eriksen R. S. (Eds.). “Kolody, B. C., Harke, M. J., Hook, S. E., and Allen, A. E). “Chapter 13 - Transcriptomic and Metatranscriptomic Approaches in Phytoplankton: Insights and Advances,”,” in A. B. T.-A. @ in P. E. Willis (Elsevier), 435–485. doi: 10.1016/B978-0-12-822861-6.00022-4
Cockrum C., Kaneshiro K. R., Rechtsteiner A., Tabuchi T. M., Strome S. (2020). A Primer for Generating and Using Transcriptome Data and Gene Sets. Dev 147(24):dev193854. doi: 10.1242/dev.193854
Coesel S. N., Durham B. P., Groussman R. D., Hu S. K., Caron D. A., Morales R. L., et al. (2021). Diel Transcriptional Oscillations of Light-Sensitive Regulatory Elements in Open-Ocean Eukaryotic Plankton Communities. Proc. Natl. Acad. Sci. 118. doi: 10.1073/pnas.2011038118
Cohen N. R., Ellis K. A., Lampe R. H., McNair H., Twining B. S., Maldonado M. T., et al. (2017). Diatom Transcriptional and Physiological Responses to Changes in Iron Bioavailability Across Ocean Provinces. Front. Mar. Sci. 4. doi: 10.3389/fmars.2017.00360
Cohen N. R., McIlvin M. R., Moran D. M., Held N. A., Saunders J. K., Hawco N. J., et al. (2021). Dinoflagellates Alter Their Carbon and Nutrient Metabolic Strategies Across Environmental Gradients in the Central Pacific Ocean. Nat. Microbiol. 6, 173–186. doi: 10.1038/s41564-020-00814-7
Consortium G. O. (2004). The Gene Ontology (GO) Database and Informatics Resource. Nucleic Acids Res. 32, D258–D261. doi: 10.1093/nar/gkh036
Csardi G., Nepusz T. (2006). The Igraph Software Package for Complex Network Research. InterJournal Complex Syst.
Cui P., Lin Q., Ding F., Xin C., Gong W., Zhang L., et al. (2010). A Comparison Between Ribo-Minus RNA-Sequencing and polyA-Selected RNA-Sequencing. Genomics 96, 259–265. doi: 10.1016/j.ygeno.2010.07.010
Delmont T. O., Gaia M., Hinsinger D. D., Frémont P., Vanni C., Fernandez-Guerra A., et al. (2022). Functional Repertoire Convergence of Distantly Related Eukaryotic Plankton Lineages Abundant in the Sunlit Ocean. Cell Genomics, 2:100123. doi: 10.1016/j.xgen.2022.100123
Derelle E., Ferraz C., Rombauts S., Rouzé P., Worden A. Z., Robbens S., et al. (2006). Genome Analysis of the Smallest Free-Living Eukaryote Ostreococcus Tauri Unveils Many Unique Features. Proc. Natl. Acad. Sci. USA 103, 11647–11652. doi: 10.1073/pnas.0604795103
de Vargas C., Audic S., Henry N., Decelle J., Mahé F., Logares R., et al. (2015). Eukaryotic Plankton Diversity in the Sunlit Ocean. Science 348, 6237. doi: 10.1126/science.1261605
Dixon P. (2003). VEGAN, a Package of R Functions for Community Ecology. J. Veg. Sci. 14, 927–930. doi: 10.1111/j.1654-1103.2003.tb02228.x
Dupont C. L., Mccrow J. P., Valas R., Moustafa A., Walworth N., Goodenough U., et al. (2015). Genomes and Gene Expression Across Light and Productivity Gradients in Eastern Subtropical Pacific Microbial Communities. ISME J. 9, 1076–1092. doi: 10.1038/ismej.2014.198
Durham B. P., Sharma S., Luo H., Smith C. B., Amin S. A., Bender S. J., et al. (2015). Cryptic Carbon and Sulfur Cycling Between Surface Ocean Plankton. Proc. Natl. Acad. Sci. 112, 453–457. doi: 10.1073/pnas.1413137112
Edgcomb V. P., Taylor C., Pachiadaki M. G., Honjo S., Engstrom I., Yakimov M. (2016). Comparison of Niskin vs. In Situ Approaches for Analysis of Gene Expression in Deep Mediterranean Sea Water Samples. Deep. Res. Part II. Top. Stud. Oceanogr 129, 213–22. doi: 10.1016/j.dsr2.2014.10.020
Ellis K. A., Cohen N. R., Moreno C., Marchetti A. (2017). Cobalamin-independent Methionine Synthase Distribution and Influence on Vitamin B12 Growth Requirements in Marine Diatoms. Protist 168, 32–47. doi: 10.1016/j.protis.2016.10.007.
Emms D. M., Kelly S. (2019). OrthoFinder: Phylogenetic Orthology Inference for Comparative Genomics. Genome Biol. 20, 238. doi: 10.1186/s13059-019-1832-y
Finn R. D., Bateman A., Clements J., Coggill P., Eberhardt R. Y., Eddy S. R., et al. (2014). Pfam: The Protein Families Database. Nucleic Acids Res. 42. doi: 10.1093/nar/gkt1223
Finn R. D., Clements J. (2011). And Eddy, sHMMER Web Server: Interactive Sequence Similarity Searching. R. Nucleic Acids Res. 39, W29–W37. doi: 10.1093/nar/gkr367
Frias-Lopez J., Shi Y., Tyson G. W., Coleman M. L., Schuster S. C., Chisholm S. W, et al. (2008). Microbial Community Gene Expression in Ocean Surface Waters. Proc. Natl. Acad. Sci. USA 105(10), 3805–10. doi: 10.1073/pnas.0708897105
Friedman J., Alm E. J. (2012). Inferring Correlation Networks From Genomic Survey Data. PloS Comput. Biol. 8, e1002687. doi: 10.1371/journal.pcbi.1002687
Gallego Romero I., Pai A. A., Tung J., Gilad Y. (2014). RNA-Seq: Impact of RNA Degradation on Transcript Quantification. BMC Biol. 12, 42. doi: 10.1186/1741-7007-12-42
Garcia L., Batut B., Burke M. L., Kuzak M., Psomopoulos F., Arcila R., et al. (2020). Ten Simple Rules for Making Training Materials FAIR. PloS Comput. Biol. 16, e1007854. doi: 10.1371/journal.pcbi.1007854
Gifford S., Satinsky B., Moran M. A. (2014). Quantitative Microbial Metatranscriptomics. Methods Mol. Biol 1096:213–29. doi: 10.1007/978-1-62703-712-9_17
Gilbert J. A., Field D., Huang Y., Edwards R., Li W., Gilna P., et al. (2008). Detection of Large Numbers of Novel Sequences in the Metatranscriptomes of Complex Marine Microbial Communities. PloS One 3(8):e3042. doi: 10.1371/journal.pone.0003042
Gong W., Browne J., Hall N., Schruth D., Paerl H., Marchetti A (2017) Molecular Insights Into a Dinoflagellate Bloom. ISME J 11, 439–52. doi: 10.1038/ismej.2016.129
Gong W., Paerl H., Marchetti A. (2018). Eukaryotic Phytoplankton Community Spatiotemporal Dynamics as Identified Through Gene Expression Within a Eutrophic Estuary. Environ. Microbiol 20(3), 1095–111. doi: 10.1111/1462-2920.14049
Grabherr M. G., Haas B. J., Yassour M., Levin J. Z., Thompson D. A., Amit I., et al. (2011). Full-Length Transcriptome Assembly From RNA-Seq Data Without a Reference Genome. Nat. Biotech. 29, 644–652. doi: 10.1038/nbt.1883
Groussman R. D., Coesel S. N., Durham B. P., Armbrust E. V. (2021). Diel-Regulated Transcriptional Cascades of Microbial Eukaryotes in the North Pacific Subtropical Gyre. Front. Microbiol. 12. doi: 10.3389/fmicb.2021.682651
Harke M. J., Frischkorn K. R., Hennon G. M. M., Haley S. T., Barone B., Karl D. M., et al. (2021). Microbial Community Transcriptional Patterns Vary in Response to Mesoscale Forcing in the North Pacific Subtropical Gyre. Environ. Microbiol. 23 (8), 4807–4822. doi: 10.1111/1462-2920.15677
Heal K. R., Durham B. P., Boysen A. K., Carlson L. T., Qin W., Ribalet F., et al. (2021). Marine Community Metabolomes Carry Fingerprints of Phytoplankton Community Composition. mSystems 6. doi: 10.1128/mSystems.01334-20
Hogle S. L., Hackl T., Bundy R. M., Park J., Braakman R., Satinsky B., et al. (2022). Siderophores as an Iron Source for Prochlorococcus in Deep Chlorophyll Maximum Layers of the Oligotrophic Ocean. ISME J. doi: 10.1038/s41396-022-01215-w
Hu S. K., Herrera E. L., Smith A. R., Pachiadaki M. G., Edgcomb V. P., Sylva S. P., et al. (2021). Protistan Grazing Impacts Microbial Communities and Carbon Cycling at Deep-Sea Hydrothermal Vents. Proc. Natl. Acad. Sci. U.S.A. 118. doi: 10.1073/pnas.2102674118
Hu S. K., Liu Z., Alexander H., Campbell V., Connell P. E., Dyhrman S. T., et al. (2018). Shifting Metabolic Priorities Among Key Protistan Taxa Within and Below the Euphotic Zone. Environ. Microbiol. 20, 2865–2879. doi: 10.1111/1462-2920.14259
Hussing C., Kampmann M.-L., Mogensen H. S., Børsting C., Morling N. (2018). Quantification of Massively Parallel Sequencing Libraries – A Comparative Study of Eight Methods. Sci. Rep. 8, 1110. doi: 10.1038/s41598-018-19574-w
Ji N., Lin L., Li L., Yu L., Zhang Y., Luo H., et al. (2018). Metatranscriptome Analysis Reveals Environmental and Diel Regulation of a Heterosigma Akashiwo (Raphidophyceae) Bloom. Environ. Microbiol 20 (3), 1078–94. doi: 10.1111/1462-2920.14045
Johnson L. K., Alexander H., Brown C. T. (2019). Re-Assembly, Quality Evaluation, and Annotation of 678 Microbial Eukaryotic Reference Transcriptomes. Gigascience 8. doi: 10.1093/gigascience/giy158
Jones L. J., Yue S. T., Cheung C. Y., Singer V. L. (1998). RNA Quantitation by Fluorescence-Based Solution Assay: RiboGreen Reagent Characterization. Anal. Biochem 265(2), 368–74. doi: 10.1006/abio.1998.2914
Kanehisa M., Sato Y., Kawashima M., Furumichi M., Tanabe M. (2016a). KEGG as a Reference Resource for Gene and Protein Annotation. Nucleic Acids Res. 44, D457–D462. doi: 10.1093/nar/gkv1070
Kanehisa M., Sato Y., Morishima K. (2016b). BlastKOALA and GhostKOALA: KEGG Tools for Functional Characterization of Genome and Metagenome Sequences. J. Mol. Biol. 428, 726–731. doi: 10.1016/j.jmb.2015.11.006
Keeling P. J., Burki F., Wilcox H. M., Allam B., Allen E. E., Amaral-Zettler L. A., et al. (2014). The Marine Microbial Eukaryote Transcriptome Sequencing Project (MMETSP): Illuminating the Functional Diversity of Eukaryotic Life in the Oceans Through Transcriptome Sequencing. PloS Biol. 12. doi: 10.1371/journal.pbio.1001889
Kerkhof L. J. (2021). Is Oxford Nanopore Sequencing Ready for Analyzing Complex Microbiomes? FEMS Microbiol. Ecol. 97. doi: 10.1093/femsec/fiab001
Klemetsen T., Raknes I. A., Fu J., Agafonov A., Balasundaram S. V., Tartari G., et al. (2018). The MAR Databases: Development and Implementation of Databases Specific for Marine Metagenomics. Nucleic Acids Res. 46, D692–D699. doi: 10.1093/nar/gkx1036
Klingenberg H., Meinicke P. (2017). How To Normalize Metatranscriptomic Count Data For Differential Expression Analysis. PeerJ 5:e3859. doi: 10.7717/peerj.3859
Kolmogorov M., Bickhart D. M., Behsaz B., Gurevich A., Rayko M., Shin S. B., et al. (2020). Metaflye: Scalable Long-Read Metagenome Assembly Using Repeat Graphs. Nat. Methods 17, 1103–10. doi: 10.1038/s41592-020-00971-x
Kolody B. C., Harke M. J., Hook S. E., Allen A. E. (2022). “Chapter 13 - Transcriptomic and Metatranscriptomic Approaches in Phytoplankton: Insights and Advances,” in, Clementson L. A., Eriksen R. S.eds. A. B. T.-A. in P. E. Willis (Elsevier) 4, 435–485. doi: 10.1016/B978-0-12-822861-6.00022-4.
Kolody B. C., McCrow J. P., Allen L. Z., Aylward F. O., Fontanez K. M., Moustafa A., et al. (2019). Diel Transcriptional Response of a California Current Plankton Microbiome to Light, Low Iron, and Enduring Viral Infection. ISME J 13, 2817–33. doi: 10.1038/s41396-019-0472-2
Kopylova E., Noé L., Touzet H. (2012). SortMeRNA: Fast and Accurate Filtering of Ribosomal RNAs in Metatranscriptomic Data. Bioinformatics 28, 24, 3211–7. doi: 10.1093/bioinformatics/bts611
Köster J., Rahmann S. (2012). Snakemake–A Scalable Bioinformatics Workflow Engine. Bioinformatics 28, 2520–2522. doi: 10.1093/bioinformatics/bts480
Krinos A. I., Cohen N. R., Follows M. J., Alexander H. (2022). Reverse Engineering Environmental Metatranscriptomes Clarifies Best Practices for Eukaryotic Assembly. bioRxiv. doi: 10.1101/2022.04.25.489326
Krinos A., Hu S., Cohen N., Alexander H. (2021). EUKulele: Taxonomic Annotation of the Unsung Eukaryotic Microbes. J. Open Source Software 6, 2817. doi: 10.21105/joss.02817
Lambert B. S., Groussman R. D., Schatz M. J., Coesel S. N., Durham B. P., Alverson A. J., et al. (2021). The Dynamic Trophic Architecture of Open-Ocean Protist Communities Revealed Through Machine-Guided Metatranscriptomics. Proc. Nat. Acad. Sci. U.S.A. 119(7), e2100916119. doi: 10.1073/pnas.2100916119
Lampe R. H., Mann E. L., Cohen N. R., Till C. P., Thamatrakoln K., Brzezinski M. A., et al. (2018). Different Iron Storage Strategies Among Bloom-Forming Diatoms. Proc. Natl. Acad. Sci. U.S.A. 115, E12275–E12284. doi: 10.1073/pnas.1805243115
Langfelder P., Horvath S. (2008). WGCNA: An R Package for Weighted Correlation Network Analysis. BMC Bioinf. 9, 559. doi: 10.1186/1471-2105-9-559
Langmead B., Salzberg S. L. (2012). Fast Gapped-Read Alignment With Bowtie 2. Nat. Methods 9, 357–359. doi: 10.1038/nmeth.1923
Lanzén A., Simachew A., Gessesse A., Chmolowska D., Jonassen I., Øvreås L. (2013). Surprising Prokaryotic and Eukaryotic Diversity, Community Structure and Biogeography of Ethiopian Soda Lakes. PloS One 8(8) e72577. doi: 10.1371/journal.pone.0072577
Lau M. C. Y., Harris R. L., Oh Y., Yi M. J., Behmard A., Onstott T. C. (2018). Taxonomic and Functional Compositions Impacted by the Quality of Metatranscriptomic Assemblies. Front. Microbiol. 9, 1235. doi: 10.3389/fmicb.2018.01235
Law C. W., Chen Y., Shi W., Smyth G. K. (2014). Voom: Precision Weights Unlock Linear Model Analysis Tools for RNA-Seq Read Counts. Genome Biol. 15, R29. doi: 10.1186/gb-2014-15-2-r29
Li H., Durbin R. (2009). Fast and Accurate Short Read Alignment With Burrows–Wheeler Transform. Bioinformatics 25, 1754–1760. doi: 10.1093/bioinformatics/btp324
Li W., Godzik A. (2006). Cd-Hit: A Fast Program for Clustering and Comparing Large Sets of Protein or Nucleotide Sequences. Bioinformatics 22, 1658–1659. doi: 10.1093/bioinformatics/btl158
Li D., Liu C.-M., Luo R., Sadakane K., Lam T.-W. (2015). MEGAHIT: An Ultra-Fast Single-Node Solution for Large and Complex Metagenomics Assembly via Succinct De Bruijn Graph. Bioinformatics 31, 1674–1676. doi: 10.1093/bioinformatics/btv033
Li L., Stoeckert C. J. Jr., Roos D. S. (2003). OrthoMCL: Identification of Ortholog Groups for Eukaryotic Genomes. Genome Res. 13, 2178–2189. doi: 10.1101/gr.1224503
Liu L., Li Y., Li S., Hu N., He Y., Pong R., et al. (2012). Comparison of Next-Generation Sequencing Systems. J. Biomed. Biotechnol. 2012, 251364. doi: 10.1155/2012/251364
Lo Giudice Cappelli E., Austin W. E. N. (2019). Size Matters: Analyses of Benthic Foraminiferal Assemblages Across Differing Size Fractions. Front. Mar. Sci. 6, 752. doi: 10.3389/fmars.2019.00752
Love M. I., Huber W., Anders S. (2014). Moderated Estimation of Fold Change and Dispersion for RNA-Seq Data With Deseq2. Genome Biol. 15. doi: 10.1186/s13059-014-0550-8
MacManes M. D. (2014). On the Optimal Trimming of High-Throughput mRNA Sequence Data. Front. Genet 5. doi: 10.3389/fgene.2014.00013
Mallick H., Chatterjee S., Chowdhury S., Chatterjee S., Rahnavard A., Hicks S. C. (2021). Differential Expression of Single-Cell RNA-Seq Data Using Tweedie Models. bioRxiv. doi: 10.1101/2021.03.28.437378
Marchetti A. (2019). A Global Perspective on Iron and Plankton Through the Tara Oceans Lens. Global Biogeochem. Cycle 33, 239–42. doi: 10.1029/2019GB006181
Marchetti A., Schruth D. M., Durkin C. A., Parker M. S., Kodner R. B., Berthiaume C. T., et al. (2012). Comparative Metatranscriptomics Identifies Molecular Bases for the Physiological Responses of Phytoplankton to Varying Iron Availability. Proc. Natl. Acad. Sci. 109(6), E317–E325. doi: 10.1073/pnas.1118408109
Marguerat S., Bähler J. (2012). Coordinating Genome Expression With Cell Size. Trends Genet. 28 (11), 560–565. doi: 10.1016/j.tig.2012.07.003
Martin M. (2011). Cutadapt Removes Adapter Sequences From High-Throughput Sequencing Reads. EMBnet.journal. doi: 10.14806/ej.17.1.200
McCarren J., Becker J. W., Repeta D. J., Shi Y., Young C. R., Malmstrom R. R., et al. (2010). Microbial Community Transcriptomes Reveal Microbes and Metabolic Pathways Associated With Dissolved Organic Matter Turnover in the Sea. Proc. Natl. Acad. Sci. 107, 16420–16427. doi: 10.1073/pnas.1010732107
Metegnier G., Paulino S., Ramond P., Siano R., Sourisseau M., Destombe C., et al. (2020). Species Specific Gene Expression Dynamics During Harmful Algal Blooms. Sci. Rep. 10, 6182. doi: 10.1038/s41598-020-63326-8
Mortazavi A., Williams B. A., McCue K., Schaeffer L., Wold B. (2008). Mapping and Quantifying Mammalian Transcriptomes by RNA-Seq. Nat. Methods 5. doi: 10.1038/nmeth.1226
Muir P., Li S., Lou S., Wang D., Spakowicz D. J., Salichos L., et al. (2016). The Real Cost of Sequencing: Scaling Computation to Keep Pace With Data Generation. Genome Biol. 17, 53. doi: 10.1186/s13059-016-0917-0
Mukherjee A., Reddy M. S. (2020). Metatranscriptomics: An Approach for Retrieving Novel Eukaryotic Genes From Polluted and Related Environments. 3. Biotech. 10, 71. doi: 10.1007/s13205-020-2057-1
Muratore D., Boysen A. K., Harke M. J., Becker K. W., Casey J. R., Coesel S. N., et al. (2022). Complex Marine Microbial Communities Partition Metabolism of Scarce Resources Over the Diel Cycle. Nat. Ecol. Evol 6, 218–29. doi: 10.1038/s41559-021-01606-w
Omori T., Ikeda M. (1992). Methods in Marine Zooplankton Ecology (Florida: Krieger Plubishing Company).
Ortiz R., Gera P., Rivera C., Santos J. C. (2021). Pincho: A Modular Approach to High Quality De Novo Transcriptomics. Genes (Basel) 12. doi: 10.3390/genes12070953
Ottesen E. A. (2016). Probing the Living Ocean With Ecogenomic Sensors. Curr. Opin. Microbiol 31, 132–9. doi: 10.1016/j.mib.2016.03.012
Padilla C. C., Ganesh S., Gantt S., Huhman A., Parris D. J., Sarode N., et al. (2015). Standard Filtration Practices may Significantly Distort Planktonic Microbial Diversity Estimates. Front. Microbiol. 6. doi: 10.3389/fmicb.2015.00547
Passow C. N., Kono T. J. Y., Stahl B. A., Jaggard J. B., Keene A. C., McGaugh S. E. (2019). Nonrandom RNAseq Gene Expression Associated With RNAlater and Flash Freezing Storage Methods. Mol. Ecol. Resour 19(2):456–64. doi: 10.1111/1755-0998.12965
Patro R., Duggal G., Love M. I., Irizarry R. A., Kingsford C. (2017). Salmon Provides Fast and Bias-Aware Quantification of Transcript Expression. Nat. Methods 14, 417–419. doi: 10.1038/nmeth.4197
Pearson G. A., Lago-Leston A., Cánovas F., Cox C. J., Verret F., Lasternas S., et al. (2015). Metatranscriptomes Reveal Functional Variation in Diatom Communities From the Antarctic Peninsula. ISME J. 9, 2275–2289. doi: 10.1038/ismej.2015.40
Peng Y., Leung H. C. M., Yiu S.-M., Lv M.-J., Zhu X.-G., Chin F. Y. L. (2013). IDBA-Tran: A More Robust De Novo De Bruijn Graph Assembler for Transcriptomes With Uneven Expression Levels. Bioinformatics 29, i326–i334. doi: 10.1093/bioinformatics/btt219
Pesant S., Not F., Picheral M., Kandels-Lewis S., Le Bescot N., Gorsky G., et al. (2015). Open Science Resources for the Discovery and Analysis of Tara Oceans Data. Sci. Data 2, 150023. doi: 10.1038/sdata.2015.23
Prjibelski A. D., Puglia G. D., Antipov D., Bushmanova E., Giordano D., Mikheenko A., et al. (2020). Extending Rnaspades Functionality for Hybrid Transcriptome Assembly. BMC Bioinf 21(302). doi: 10.1186/s12859-020-03614-2
Rabines A., Lampe R., Allen A. E. (2020a). Sterivex RNA Extraction. doi: 10.17504/protocols.io.bd9ti96n
Rabines A., Lampe R., Zeigler Allen L., Allen A. E. (2020b). NOAA-CalCOFI Ocean Genomics (NCOG) Sample Collection. doi: 10.17504/protocols.io.bmubk6sn
Ramette A. (2007). Multivariate Analyses in Microbial Ecology. FEMS Microbiol. Ecol. 62, 142–160. doi: 10.1111/j.1574-6941.2007.00375.x
Rao D. (2020) Characterizing Cobalamin Cycling by Antarctic Marine Microbes Across Multiple Scales doi: 10.1575/1912/25832
Reiner A., Yekutieli D., Benjamini Y. (2003). Identifying Differentially Expressed Genes Using False Discovery Rate Controlling Procedures. Bioinformatics 19, 368–375. doi: 10.1093/bioinformatics/btf877
Richter D. J., Berney C., Strassert J. F.H., Burki F., de Vargas C. (2020). EukProt: A Database of Genome-Scale Predicted Proteins Across the Diversity of Eukaryotic Life. bioRxiv doi: 10.1101/2020.06.30.180687.
Robertson G., Schein J., Chiu R., Corbett R., Field M., Jackman S. D., et al. (2010). De Novo Assembly and Analysis of RNA-Seq Data. Nat. Methods 7, 909–912. doi: 10.1038/nmeth.1517
Robinson M. D., McCarthy D. J., Smyth G. K. (2010). Edger: A Bioconductor Package for Differential Expression Analysis of Digital Gene Expression Data. Bioinformatics 26, 139–140. doi: 10.1093/bioinformatics/btp616
Robinson M. D., Oshlack A. (2010). A Scaling Normalization Method for Differential Expression Analysis of RNA-Seq Data. Genome Biol. 11, R25. doi: 10.1186/gb-2010-11-3-r25
Rusch D. B., Halpern A. L., Sutton G., Heidelberg K. B., Williamson S., Yooseph S., et al. (2007). The Sorcerer II Global Ocean Sampling Expedition: Northwest Atlantic Through Eastern Tropical Pacific. PloS Biol. 5, 1–34. doi: 10.1371/journal.pbio.0050077
Saito M. A., McIlvin M. R., Moran D. M., Goepfert T. J., DiTullio G. R., Post A. F., et al. (2014). Multiple Nutrient Stresses at Intersecting Pacific Ocean Biomes Detected by Protein Biomarkers. Science 345(6201):1173–7. doi: 10.1126/science.1256450
Saito M. A., McIlvin M. R., Moran D. M., Santoro A. E., Dupont C. L., Rafter P. A., et al. (2020). Abundant Nitrite-Oxidizing Metalloenzymes in the Mesopelagic Zone of the Tropical Pacific Ocean. Nat. Geosci. 13, 355–362. doi: 10.1038/s41561-020-0565-6
Sandve G. K., Nekrutenko A., Taylor J., Hovig E. (2013). Ten Simple Rules for Reproducible Computational Research. PloS Comput. Biol. 9, 1–4. doi: 10.1371/journal.pcbi.1003285
Santoro A. E., Saito M. A., Goepfert T. J., Lamborg C. H., Dupont C. L., DiTullio G. R. (2017). Thaumarchaeal Ecotype Distributions Across the Equatorial Pacific Ocean and Their Potential Roles in Nitrification and Sinking Flux Attenuation. Limnol. Oceanogr. 62, 1984–2003. doi: 10.1002/lno.10547
Satinsky B. M., Gifford S. M., Crump B. C., Moran M. A. (2013). Use of Internal Standards for Quantitative Metatranscriptome and Metagenome Analysis. Methods Enzymol. 531, 237–250. doi: 10.1016/B978-0-12-407863-5.00012-5
Satinsky B. M., Gifford S. M., Crump B. C., Smith C., Moran M. A. (2017). Internal Genomic DNA Standard for Quantitative Metagenome Analysis V.3. doi: 10.17504/protocols.io.jxdcpi6
Schoenle A., Hohlfeld M., Hermanns K., Mahé F., de Vargas C., Nitsche F., et al. (2021). High and Specific Diversity of Protists in the Deep-Sea Basins Dominated by Diplonemids, Kinetoplastids, Ciliates and Foraminiferans. Commun. Biol. 4, 501. doi: 10.1038/s42003-021-02012-5
Scholin C. A., Birch J., Jensen S., Marin R., Massion E., Pargett D., et al. (2017). The Quest to Develop Ecogenomic Sensors a 25-Year History of the Environmental Sample Processor (ESP) as a Case Study. Oceanography 30(4):100–13. doi: 10.5670/OCEANOG.2017.427
Schroeder A., Mueller O., Stocker S., Salowsky R., Leiber M., Gassmann M., et al. (2006). The RIN: An RNA Integrity Number for Assigning Integrity Values to RNA Measurements. BMC Mol. Biol. 7, 3. doi: 10.1186/1471-2199-7-3
Semmouri I., De Schamphelaere K. A.C., Mees J., Janssen C. R., and Asselman J. (2020). Evaluating the Potential of Direct RNA Nanopore Sequencing: Metatranscriptomics Highlights Possible Seasonal Differences in a Marine Pelagic Crustacean Zooplankton Community. Mar. Environ. Res. doi: 10.1016/j.marenvres.2019.104836.
Shakya M., Lo C.-C., Chain P. S. G. (2019). Advances and Challenges in Metatranscriptomic Analysis. Front. Genet. 10. doi: 10.3389/fgene.2019.00904
Shannon P., Markiel A., Ozier O., Baliga N. S., Wang J. T., Ramage D., et al. (2003). Cytoscape: A Software Environment for Integrated Models of Biomolecular Interaction Networks. Genome Res. 13. doi: 10.1101/gr.1239303
Shi Y., McCarren J., Delong E. F. (2012). Transcriptional Responses of Surface Water Marine Microbial Assemblages to Deep-Sea Water Amendment. Environ. Microbiol 14(1):191–206. doi: 10.1111/j.1462-2920.2011.02598.x
Smith D. R. (2013). RNA-Seq Data: A Goldmine for Organelle Research. Brief. Funct. Genomics 12, 454–456. doi: 10.1093/bfgp/els066
Soneson C., Love M. I., Robinson M. D. (2015). Differential Analyses for RNA-Seq: Transcript-Level Estimates Improve Gene-Level Inferences. F1000Research 4, 1521. doi: 10.12688/f1000research.7563.2
Song Y., Milon B., Ott S., Zhao X., Sadzewicz L., Shetty A., et al. (2018). A Comparative Analysis of Library Prep Approaches for Sequencing Low Input Translatome Samples. BMC Genomics 19, 696. doi: 10.1186/s12864-018-5066-2
Sperfeld M., Yahalomi D., Segev E. (2021). Resolving the Microalgal Gene Landscape at the Strain Level: A Novel Hybrid Transcriptome of Emiliania Huxleyi CCMP3266. Appl. Environ. Microbiol. 88(2):e0141821. doi: 10.1128/AEM.01418-21
Steinegger M., Söding J. (2017). MMseqs2 Enables Sensitive Protein Sequence Searching for the Analysis of Massive Data Sets. Nat. Biotechnol. 35, 1026–1028. doi: 10.1038/nbt.3988
Stewart F. J., Ottesen E. A., DeLong E. F. (2010). Development and Quantitative Analyses of a Universal rRNA-Subtraction Protocol for Microbial Metatranscriptomics. ISME J. 4, 896–907. doi: 10.1038/ismej.2010.18
Sun F., Yang H., Wang G., Shi Q. (2020). Combination Analysis of Metatranscriptome and Metagenome Reveal the Composition and Functional Response of Coral Symbionts to Bleaching During an El Niño Event. Front. Microbiol. 11. doi: 10.3389/fmicb.2020.00448
Tamames J., Puente-Sánchez F. (2019). SqueezeMeta, a Highly Portable, Fully Automatic Metagenomic Analysis Pipeline. Front. Microbiol. 9. doi: 10.3389/fmicb.2018.03349
Tang S., Lomsadze A., Borodovsky M. (2015). Identification of Protein Coding Regions in RNA Transcripts. Nucleic Acids Res. 43(12), e78. doi: 10.1093/nar/gkv227
Tatusov R. L., Fedorova N. D., Jackson J. D., Jacobs A. R., Kiryutin B., Koonin E. V., et al. (2003). The COG Database: An Updated Version Includes Eukaryotes. BMC Bioinf. 4, 41. doi: 10.1186/1471-2105-4-41
Tully B. J., Buongiorno J., Cohen A. B., Cram J. A., Garber A. I., Hu S. K., et al. (2021). The Bioinformatics Virtual Coordination Network: An Open-Source and Interactive Learning Environment. Front. Educ. 6. doi: 10.3389/feduc.2021.711618
van Damme R., Hölzer M., Viehweger A., Müller B., Bongcam-Rudloff E., Brandt C. (2021). Metagenomics Workflow for Hybrid Assembly, Differential Coverage Binning, Metatranscriptomics and Pathway Analysis (MUFFIN). PloS Comput. Biol. 17(2): e1008716. doi: 10.1371/JOURNAL.PCBI.1008716
van Dongen S. (2000). Performance Criteria for Graph Clustering and Markov Cluster Experiments. Tech. Rep. INS-R0012, Natl. Res. Inst. Math. Comput. Sci.
Van Vlierberghe M., Di Franco A., Philippe H., Baurain D. (2021). Decontamination, Pooling and Dereplication of the 678 Samples of the Marine Microbial Eukaryote Transcriptome Sequencing Project. BMC Res. Notes. doi: 10.1186/s13104-021-05717-2.
Vanni C., Schechter M. S., Acinas S. G., Barberán A., Buttigieg P. L., Casamayor E. O., et al. (2021). Unifying the Known and Unknown Microbial Coding Sequence Space. Brown TCELife 11, e67667. doi: 10.7554/eLife.67667
Venter J. C., Remington K., Heidelberg J. F., Halpern A. L., Rusch D., Eisen J. A., et al. (2004). Environmental Genome Shotgun Sequencing of the Sargasso Sea. Science 304 (5667), 66–74. doi: 10.1126/science.1093857
Villar E., Vannier T., Vernette C., Lescot M., Cuenca M., Alexandre A., et al. (2018). The Ocean Gene Atlas: Exploring the Biogeography of Plankton Genes Online. Nucleic Acids Res. 46, W289–W295. doi: 10.1093/nar/gky376
Wagner G. P., Kin K., Lynch V. J. (2012). Measurement of mRNA Abundance Using RNA-Seq Data: RPKM Measure Is Inconsistent Among Samples. Theory Biosci. 131, 281–285. doi: 10.1007/s12064-012-0162-3
Walworth N. G., Saito M. A., Lee M. D., McIlvin M. R., Moran D. M., Kellogg R. M., et al. (2022). Why Environmental Biomarkers Work: Transcriptome-Proteome Correlations and Modeling of Multi-Stressor Experiments in the Marine Bacterium Trichodesmium. J. Proteome Res. 21 (1), 77–89. doi: 10.1021/acs.jproteome.1c00517
Wang S., Gribskov M. (2016). Comprehensive Evaluation of De Novo Transcriptome Assembly Programs and Their Effects on Differential Gene Expression Analysis. Bioinformatics 33, 327–333. doi: 10.1093/bioinformatics/btw625
Weissman J. L., Dimbo E.-R. O., Krinos A. I., Neely C., Yagües Y., Nolin D., et al. (2021). Estimating the Maximal Growth Rates of Eukaryotic Microbes From Cultures and Metagenomes via Codon Usage Patterns. bioRxiv doi: 10.1101/2021.10.15.464604
Wilms S. N. (2021). A Beginner’s Guide on Integrating *Omics Approaches to Study Marine Microbial Communities: Details and Discussions From Sample Collection to Bioinformatics Analysis. Front. Mar. Sci. 8. doi: 10.3389/fmars.2021.692538
Wilson G. (2006). Software Carpentry: Getting Scientists to Write Better Code by Making Them More Productive. Comput. Sci. Eng. 8, 66–69. doi: 10.1109/MCSE.2006.122
Wu M., McCain J. S.P., Rowland E., Middag R., Sandgren M., Allen A. E., et al (2019). Manganese and Iron Deficiency in Southern Ocean Phaeocystis Antarctica Populations Revealed Through Taxon-Specific Protein Indicators. Nat. Commun. doi: 10.1038/s41467-019-11426-z.
Wu J., Gao W., Johnson R. H., Zhang W., Meldrum D. R. (2013). Integrated Metagenomic and Metatranscriptomic Analyses of Microbial Communities in the Meso- and Bathypelagic Realm of North Pacific Ocean. Mar. Drugs 11 (10), 3777–801. doi: 10.3390/md11103777
Zhang Y., Thompson K. N., Branck T., Yan Y., Nguyen L. H., Franzosa E. A., et al. (2021). Metatranscriptomics for the Human Microbiome and Microbial Community Functional Profiling. Annu. Rev. Biomed. Data Sci. 4, 279–311. doi: 10.1146/annurev-biodatasci-031121-103035
Zhao S., Zhang Y., Gamini R., Zhang B., von Schack D. (2018). Evaluation of Two Main RNA-Seq Approaches for Gene Quantification in Clinical RNA Sequencing: Polya+ Selection Versus rRNA Depletion. Sci. Rep. 8, 4781. doi: 10.1038/s41598-018-23226-4
Keywords: metatranscriptomics, phytoplankton, biological oceanography, microbial ecology, bioinformatics
Citation: Cohen NR, Alexander H, Krinos AI, Hu SK and Lampe RH (2022) Marine Microeukaryote Metatranscriptomics: Sample Processing and Bioinformatic Workflow Recommendations for Ecological Applications. Front. Mar. Sci. 9:867007. doi: 10.3389/fmars.2022.867007
Received: 31 January 2022; Accepted: 03 May 2022;
Published: 28 June 2022.
Edited by:
Angel Borja, Technological Center Expert in Marine and Food Innovation (AZTI), SpainReviewed by:
Anders Lanzén, Technology Center Expert in Marine and Food Innovation (AZTI), SpainCopyright © 2022 Cohen, Alexander, Krinos, Hu and Lampe. This is an open-access article distributed under the terms of the Creative Commons Attribution License (CC BY). The use, distribution or reproduction in other forums is permitted, provided the original author(s) and the copyright owner(s) are credited and that the original publication in this journal is cited, in accordance with accepted academic practice. No use, distribution or reproduction is permitted which does not comply with these terms.
*Correspondence: Natalie R. Cohen, Y29oZW5AdWdhLmVkdQ==; Harriet Alexander, aGFsZXhhbmRlckB3aG9pLmVkdQ==; Arianna I. Krinos, YWtyaW5vc0B3aG9pLmVkdQ==
Disclaimer: All claims expressed in this article are solely those of the authors and do not necessarily represent those of their affiliated organizations, or those of the publisher, the editors and the reviewers. Any product that may be evaluated in this article or claim that may be made by its manufacturer is not guaranteed or endorsed by the publisher.
Research integrity at Frontiers
Learn more about the work of our research integrity team to safeguard the quality of each article we publish.