- 1Department of Biology, Genetics Institute, University of Florida, Gainesville, FL, United States
- 2School of Oceanography, University of Washington, Seattle, WA, United States
- 3Department of the Geophysical Sciences, University of Chicago, Chicago, IL, United States
- 4Department of Biology, University of Washington, Seattle, WA, United States
Most biological diversity on Earth is contained within microbial communities. In the ocean, these communities dominate processes related to carbon fixation and nutrient recycling. Yet, specific factors that determine community composition and metabolic activity are difficult to resolve in complex microbial populations, complicating predictions of microbial processes in a changing ocean. Microbial metabolism generates small organic molecules that reflect both the biochemical and physiological diversity as well as the taxonomic specificity of these biological processes. These small molecules serve as the conduit for taxon-specific signaling and exchange. Here, we use liquid chromatography-mass spectrometry (LC-MS)-based metabolomics to taxonomically categorize 111 metabolites that include small molecules in central and secondary metabolism across 42 taxa representing numerically dominant and metabolically important lineages of microbial autotrophs and heterotrophs. Patterns in metabolite presence-absence broadly reflected taxonomic lineages. A subset of metabolites that includes osmolytes, sulfur-containing metabolites, sugars, and amino acid derivatives provided chemotaxonomic information among phytoplankton taxa. A variety of phytohormones and signaling molecules were predominantly found in the heterotrophic bacteria and archaea, expanding knowledge of metabolites implicated in modulating interactions between microbes. This chemotaxonomic inventory of marine microbial metabolites is a key step in deciphering metabolic networks that influence ocean biogeochemical cycles.
Introduction
Microbes are the major producers and consumers in the ocean ecosystem, where they account for approximately two-thirds of total marine biomass (Bar-On and Milo, 2019). Microbial primary producers fix 50 Gt of carbon in the ocean each year, nearly equivalent to their terrestrial counterparts (Falkowski and Wilson, 1992). Heterotrophic bacteria consume approximately half of this phytoplankton-derived carbon through the microbial loop (Azam et al., 1983) in a matter of weeks, accounting for the largest flux of organic carbon in the ocean. This transformation of carbon between primary producers and heterotrophic bacteria is a key step in determining the fate of carbon in the ocean, where it is either remineralized back to carbon dioxide, exported to the deep ocean in sinking particles, or converted to refractory dissolved organic matter (Lechtenfeld et al., 2015; Zakem et al., 2021; Gonsior et al., 2022). The specific chemical structures produced by members of microbial communities form feedback networks that determine community composition and the fate of organic matter. Improving knowledge on the diversity of microbial chemotypes, or chemical phenotypes, is a key step in understanding the metabolic networks they support and predicting how changing taxonomy of primary producers will alter the carbon cycle (Henson et al., 2021).
Given the many thousands of individual microbial species and metabolites present in seawater communities, it has been challenging to connect specific metabolic processes to the microbial taxa responsible (Cavaco et al., 2022; Paoli et al., 2022). Advances in molecular and chemical tools have improved resolution and identification of microbially cycled metabolites in seawater communities (Moran et al., 2016; Durham, 2021; Vallet et al., 2021; Raina et al., 2022). Genome-based studies of marine microbial plankton have been useful in reconstructing potential metabolisms, ecological functions, and evolutionary relationships of taxonomic groups. Application of molecular tools in natural field communities have established distributions of microbial populations and co-occurrence patterns among taxa, revealing the importance of both nutrient supply and microbe-microbe interactions, respectively, in controlling community composition (Gómez-Consarnau et al., 2012; Cirri and Pohnert, 2019; Pontiller et al., 2020; Raes et al., 2021). However, there are gaps in our ability to predict microbial phenotype from genotype that include unrecognized or unannotated metabolic genes and limited knowledge of how specific taxa regulate metabolic pathways (Lewis et al., 2012). Application of metabolomics tools to marine microbial systems has advanced our recognition of abundant metabolites in marine ecosystems (Llewellyn et al., 2015; Biancarosa et al., 2018; Johnson et al., 2020; Boysen et al., 2021; Heal et al., 2021; Hughes et al., 2021) as well as our understanding of how anthropogenic contaminants impact microbial metabolism (Gauthier et al., 2020). Linking environmental inventories of microbial metabolites to regulation of ecosystem processes is a next step in understanding and predicting ocean microbiome function in our current and future ocean. In particular, identifying producers and consumers of molecules that act as substrates, or ‘currencies’ in microbial nutrient exchange is an important regulatory component of elemental cycling (Moran, 2015).
Chemical currencies cycled between specific marine microbial taxa create interdependencies in the microbial food web that influence community structure and the rate and timing of nutrient cycling (Moran, 2015; Seymour et al., 2017). For instance, 29 of 36 diatom-derived metabolites identified in supporting heterotrophic bacterial growth had differential use across bacterial taxa, illustrating that phytoplankton metabolites support resource partitioning by microbial heterotrophs (Ferrer-González et al., 2021). Further, co-culture studies between phytoplankton and bacteria have led to identification of signaling molecules and hormones that influence growth rates and exchange of nutrients between microbial taxa (Seyedsayamdost et al., 2011; Amin et al., 2015; Durham et al., 2017; Fei et al., 2020; Shibl et al., 2020). Omics-based analyses that taxonomically resolve metabolic pathways using gene expression patterns have provided an important viewpoint into the microbial producers and/or consumers of particular metabolites (Aylward et al., 2015; Moran et al., 2016). Detailed studies of labile molecules like DMSP (Levine et al., 2012; Varaljay et al., 2015), methylphosphonate (Martínez et al., 2013), 2,3-dihydroxypropane-1-sulfonate (DHPS) (Durham et al., 2019), glycine betaine (Boysen et al., 2022), and trehalose (Boysen et al., 2021) have aided in resolving the specific producers and/or consumers of these molecules in natural microbial populations through concurrent measurements of transcript and metabolite abundances. Isotope-based tools like Chip-SIP have provided high-throughput means to quantify taxon-specific incorporation of stable isotope-labeled substrates (Mayali et al., 2012). High-resolution metabolomics studies of cultures and field samples have demonstrated the value of metabolic fingerprints in distinguishing plankton (Baumeister et al., 2020) and identified underexplored sulfur-containing metabolites gonyol, dimethylsulfonioacetate (DMSAc), dimethylsulfoxonium propionate (DMSOP) (Gebser and Pohnert, 2013; Thume et al., 2018; Gebser et al., 2020) and nitrogen-containing metabolites trigonelline and homarine (Heal et al., 2021) implicated in metabolic exchange among microbial taxa.
Obtaining greater chemotaxonomic resolution of microbial representatives abundant in field populations will enhance our ability to link microbial metabolites to the taxa that produce and transform them in the ocean. To this end, we generated a targeted metabolomics dataset that includes inventories of 111 metabolites in 42 microbial taxa that represent numerically abundant lineages in natural ocean communities, with a focus on labile molecules implicated in microbial interactions and nutrient cycling. We explore the taxonomic distribution of marine metabolites and identify distinguishing metabolite classes that contribute to the chemotype of major lineages of marine microbial autotrophs and heterotrophs.
Materials and methods
Phytoplankton culturing and collection
Phytoplankton were cultured in diurnal incubators according to the medium, light, and temperature conditions in Table 1. All eukaryotic phytoplankton, with the exception of the Chrysochromulina strains, were grown in artificial seawater medium (MilliQ water that contained 28 ppt Sigma sea salts) amended with L1, f/2, or K nutrients based on recipes from the National Center for Marine Algae and Microbiota (NCMA) (Guillard, 1975; Guillard and Hargraves, 1993). Silica (+ Si) was added to the media for diatom culturing. Prochlorococcus and Synechococcus were grown in Pro99 (Moore et al., 2007) prepared with Turks Island Salt Solution and supplemented with 6 mM sterile sodium bicarbonate and 1 mM N-Tris(hydroxymethyl)methyl-3-aminopropanesulfonic acid (TAPS) (Morris and Zinser, 2013). Crocosphaera was grown in YBC-II artificial seawater medium (Chen et al., 1996) supplemented with 0.9 mM nitrate. Nutrient stocks were stored in VWR SuperClear Ultra-High Performance conical tubes. All media was made and stored in combusted borosilicate glass containers. All artificial salt water was autoclaved, and all final media was filter-sterilized using a 0.22um PVDF membrane. Cells were collected during mid-to-late exponential phase by gentle filtration onto 0.2µm Durapore (Millipore Co., MA, U.S.) filters using combusted borosilicate filter towers. All filters were flash frozen in liquid nitrogen and subsequently stored at -80°C. Cells were enumerated using flow cytometry or microscopy. Axenic status of phytoplankton was verified regularly using 4,6-diamidino-2-phenylindole (DAPI)-staining and epifluorescence microscopy, SYBR-staining and flow cytometry, along with plating on bacterial ½ YTSS agar. According to this combination of microscopy, flow cytometry, and bacterial culture tests, we did not detect contamination of phytoplankton mono-cultures. We acknowledge that low levels of contamination may occur below detection using these standard approaches, though we expect minimal contribution to the overall metabolite signal from such potential contamination.
Freshwater Chrysochromulina strains were grown in a proprietary RAC-5 medium (Hovde et al., 2019). Marine Chrysochromulina strains (C. acantha K-0643, C. rotalis K-0644 and C. simplex K-0272) were cultured in natural seawater-based L1 medium at 15°C on a 12 h/12 h light–dark cycle at ~50 µmol photons m−2 s−1. Approximately 107 cells were collected by centrifugation and frozen at −80°C until metabolite extraction.
Bacterial culturing and collection
Heterotrophic bacteria were initially grown on ½ YTSS agar at 25-30°C in the dark for one to five days, after which isolated colonies were inoculated into 20-30 mL artificial seawater medium (MilliQ water that contained 28 ppt Sigma sea salts) amended with L1 recipe nitrate, phosphate, and trace elements (Guillard, 1975; Guillard and Hargraves, 1993), 5 mM acetate, 5 mM ammonium, MEM essential amino acids (Sigma M5550; 1:200 dilution), non-essential amino acids (Sigma M7145; 1:400 dilution) and Marine Basal Mineral (MBM) vitamins. Cells were grown overnight at 30°C in the dark at 200 rpm in VWR SuperClear Ultra-High Performance conical tubes. Overnight cultures were diluted to ~0.01 optical density (OD) in triplicate tubes containing 30 mL fresh medium and grown overnight until cells reached late exponential phase (~0.2-0.4 OD). Cells were collected by gentle filtration onto 0.2µm Durapore filters using combusted borosilicate filter towers and flash frozen in liquid nitrogen and subsequently stored at -80°C until metabolite extraction. Cells were enumerated by SYBR-staining and flow cytometry.
Archaeal culturing and collection
Pure cultures of the marine ammonia-oxidizing archaeon Nitrosopumilus maritimus strain SCM1 were grown in triplicate in HEPE-buffered synthetic seawater medium supplemented with 1 mM NH4Cl at 30°C in the dark without shaking (Qin et al., 2017). Growth of strain SCM1 was monitored by measuring ammonia consumption, nitrite accumulation, and cell density. Ammonia concentration was measured using the o-phthaldialdehyde fluorescence method (Holmes et al., 1999), and nitrite concentration was measured spectrophotometrically using the Griess reagent (Grasshoff et al., 1999). Cell counts were determined using the Moviol–SYBR Green I staining protocol as previously reported (Qin et al., 2014) with a Zeiss epifluorescence microscope to count 15 random fields of view for each sample with 30 to 200 cells per field. Mid-exponential phase cells were harvested on 0.22 µm Durapore membrane filters and stored at -80°C until metabolite extraction.
Metabolite extraction
Filtered culture media was extracted alongside culture samples as methodological blanks. Metabolite extraction was carried out using a modified Bligh-Dyer extraction method as described in Boysen et al., 2018. This extraction method yields aqueous and organic fractions. For this study, we analyzed results from the aqueous fraction. Briefly, extraction solvents [dichloromethane (DCM) and 1:1 methanol (MeOH) to water] were prepared the day before sample extraction and stored at -20°C overnight. Bead-beating tubes were filled with a mixture of 100 µm and 400 µm silica beads, and frozen samples were placed into the tubes. Approximately 2 mL of both extraction solvents were added with isotope-labeled extraction internal standards. Samples were shaken for 30 seconds, then chilled at -20°C for 15 minutes. The homogenization step was repeated twice for a total of 30 minutes at -20°C over three bead beating cycles. Samples were then centrifuged to separate aqueous and organic phases. The aqueous phase was removed from the bead beating tube, and the remaining DCM fraction was rinsed three times with cold MeOH:H2O. The combined aqueous phase and rinses were transferred to a clean 20 mL glass vial and dried under clean N2. The dried aqueous fraction was re-dissolved in 380 µL of water and received a 20 µL spike of isotope-labeled injection standards. Standards in water and standards spiked into an aliquot of pooled sample were analyzed to set limits on the in-house quality control retention time and ion ratio ranges. Samples were analyzed within 12 or 72 hours of extraction for the HILIC and RP analyses, respectively, and stored at -80°C until analysis.
Chromatography
For reversed phase (RP) chromatography, a Waters Acquity UPLC HSS Cyano column (1.8 µm particle size, 2.1 mm x 100 mm) equipped with a Waters Acquity UPLC HSS Cyano guard column (1.8 µm particle size, 2.1 mm x 5 mm) was used with 0.1% formic acid in water (Solvent A) and 0.1% formic acid in acetonitrile (Solvent B) at a flow rate of 0.4 mL min-1. The column was held at 5% B for 2 minutes, ramped to 100% B over 16 minutes, held at 100% B for 2 minutes, and equilibrated at 5% B for 5 minutes (25 minutes total). The column was maintained at 35°C. The injection volume ranged from 5 to 15 µL, depending on amount of biomass on the filter. When starting a batch, the column was equilibrated at the starting conditions for at least 10 minutes; several water blanks were run before and throughout a batch. After a batch, the column was flushed with 100% acetonitrile for 20 to 30 minutes.
For hydrophilic interaction liquid chromatography (HILIC), a SeQuant ZIC-pHILIC column (5 mm particle size, 2.1 mm x 150 mm, from Millipore) was used with 10 mM ammonium carbonate in 85:15 acetonitrile to water (Solvent A) and 10 mM ammonium carbonate in 60:40 water to acetonitrile (Solvent B) at a flow rate of 0.15 mL min-1. The column was held at 100% A for 2 minutes, ramped to 100% B over 18 minutes, held at 100% B for 5 minutes, and equilibrated at 100% A for 25 minutes (50 minutes total). The column was maintained at 30°C. The injection volume was 2 µL for all samples and standard mixes. When starting a batch, the column was equilibrated at the starting conditions for at least 30 minutes. To improve the performance of the HILIC column, we maintained the same injection volume, kept the instrument running water blanks between samples as necessary, and injected standards in a representative matrix in addition to standards in water. After each batch, the column was flushed with 10 mM ammonium carbonate in 85:15 water to acetonitrile for 20 to 30 minutes.
Mass spectrometry
For targeted metabolomics, we used a Waters Xevo TQ-S triple quadrupole (TQS) with electrospray ionization (ESI) in selected reaction monitoring mode (SRM) with polarity switching. SRM conditions for each compound (collision energy, cone voltage, precursor and product ions) were optimized by infusion of each metabolite standard. For most metabolites, two SRM transitions were selected based on maximum peak areas. The transitions were monitored over a two- to three-minute window around the retention time for the RP and a five- to ten-minute window around the retention time for the HILIC. Scheduling was set up to ensure at least 12 data points per peak were collected. MS parameters were as follows: capillary voltage of 0.5 kV for positive mode and 1.0 kV for negative mode, source temperature of 130°C, cone gas flow at 150 L/h and desolvation gas flow at 1000 L/h for both positive and negative modes. Desolvation temperature was 500°C or 600°C for HILIC or RP, respectively.
For untargeted metabolomics, we used a Thermo QExactive HF (QE) with ESI. For HILIC, a full scan method with polarity switching was used with a scan range of 80 to 900 m/z and a resolution of 60,000. For HILIC, the capillary temperature was 320°C, the H-ESI spray voltage was 3.5 kV, and the auxiliary gas heater temperature was 90°C. The S-lens RF level was 65. Sheath gas, auxiliary gas, and sweep gas flow rates were maintained at 16, 3, and 1, respectively. For RP, a full scan method in positive mode was used with a scan range of 90 to 900 m/z and a resolution of 120,000. For RP analysis of samples, the capillary temperature was 320°C, the H-ESI spray voltage was 3.8 kV, and the auxiliary gas heater temperature was 90°C. The S-lens RF level was 65. Sheath gas, auxiliary gas, and sweep gas flow rates were maintained at 40, 10, and 1, respectively.
Data analysis
Because data were generated over the course of two years, new standards were added into the targeted analysis over time. For a subset of 22 standards that were sequentially added over the course of our measurements, we mined the untargeted metabolite data obtained on the QExactive for information about those compounds (Supplemental Table 1). Internal standards (n=28), metabolites added to culture media (e.g. vitamins, amino acids; n=41), metabolites with high background levels (n=3), and metabolites that contained missing data or were not observed across the 42 organisms (n=85) were excluded from analyses. The remaining 111 metabolites were used in subsequent analyses. For each organism, samples from corresponding media blank(s), biological replicates, and pooled mixes of replicates were treated as a batch and processed separately. For each batch/organism, QE data were first converted to .mzxml using MSConvert (Chambers et al., 2012) with positive and negative scans separated for HILIC. For MS data, each fraction [aqueous on RP, aqueous on HILIC (positive scans), and aqueous on HILIC (negative scans)] were processed separately through Skyline for small molecules (MacLean et al., 2010). For quality mass features, we searched an internal database of standards run in the exact manner on our instruments for matches to exact m/z and retention time, yielding an unequivocal identification. After integration, we used an in-house quality control (QC) filter to ensure proper metabolite identification based on retention time (RT), signal to noise ratio (S/N), absolute peak area/height, exact mass, and fragment ion ratio (for TQS) (Supplemental Table 2).
If a compound was present and met the QC thresholds, it was considered present in a biological replicate sample. A compound was flagged if it was considered comparable to the blank in the QC step. A compound was marked absent if it was otherwise not present or flagged as having an incorrect RT, low peak area, and/or poor S/N in the QC step. In the final presence/absence table, a “1” denotes a metabolite was present in all biological replicate samples, a “Blk” denotes a metabolite had a comparable peak area to the blank in more than one of the biological replicate samples, and a “0” denotes it was absent in all samples or only observed in a single biological replicate at a level comparable to the blank. In some cases, the presence/absence table includes fractions rather than a 0 or 1 if the compound was present only in some replicates. For the purposes of our analyses, fractions >0.5 were treated as “1” while fractions ≤0.5 were treated as “0” and “Blk” was also treated as “0.” For Chysochromulina data, we extracted approximately the same number of cells (107) per sample. We then used the QC-processed metabolite peak area values to compare relative differences in metabolites of interest between strains (Supplemental Table 3). For T. pseudonana data, we used the total extracted cell volume to normalize QC-processed peak areas values to compare relative differences in metabolites of interest between salinity growth conditions (Supplemental Table 4). In both cases, samples were processed and analyzed in the same QC batch. Hierarchical clustering, NMDS [vegan version 2.5-7 (Oksanen et al., 2020) and pvclust version 2.2-0 (Suzuki et al., 2019) packages], and MCA [FactoMineR version 2.4 package (Lê et al., 2008)] were performed in R (version 4.1.0).
Results
Intracellular metabolite profiles across major marine microbial lineages
We used a combined targeted and untargeted liquid chromatography-mass spectrometry-based metabolomics approach (Boysen et al., 2018) to screen the intracellular metabolite pools of 42 microbial taxa that span the three domains of life with autotrophic and heterotrophic representatives (Table 1). The taxa belong to major lineages in the oceans that contribute substantially to biomass and carbon flux in the surface ocean, many of which have publicly available genomic resources. Organisms included 14 marine autotrophic eukaryotes [red-lineage stramenopiles (e.g. diatoms), alveolates (e.g. dinoflagellates), and haptophytes (e.g. coccolithophores); green-lineage chloroplastida (e.g. prasinophytes)], seven autotrophic bacteria (e.g. cyanobacteria), 15 heterotrophic bacteria (e.g. alpha-, gamma-, beta-proteobacteria, and flavobacteria), and one marine archaeon (Table 1; Figure 1A). We cultured these thirty-seven marine organisms in mono-culture in artificial seawater media specific to the organism (Table 1). For Thalassiosira pseudonana, we included a salinity experiment where the diatom was grown in 10, 28, or 35 ppt salinity seawater to identify potential variability in metabolites due to growth conditions. Additionally, we included three marine and two freshwater strains of the haptophyte Chrysochromulina in this study. These non-coccolithophorid, mixotrophic haptophytes were maintained as bacterized cultures, with freshwater strains grown in an artificial medium and marine strains in natural seawater medium. To maintain consistency in sampling across organisms, we collected all cultures during mid-to-late exponential growth phase and during the light cycle for phytoplankton.
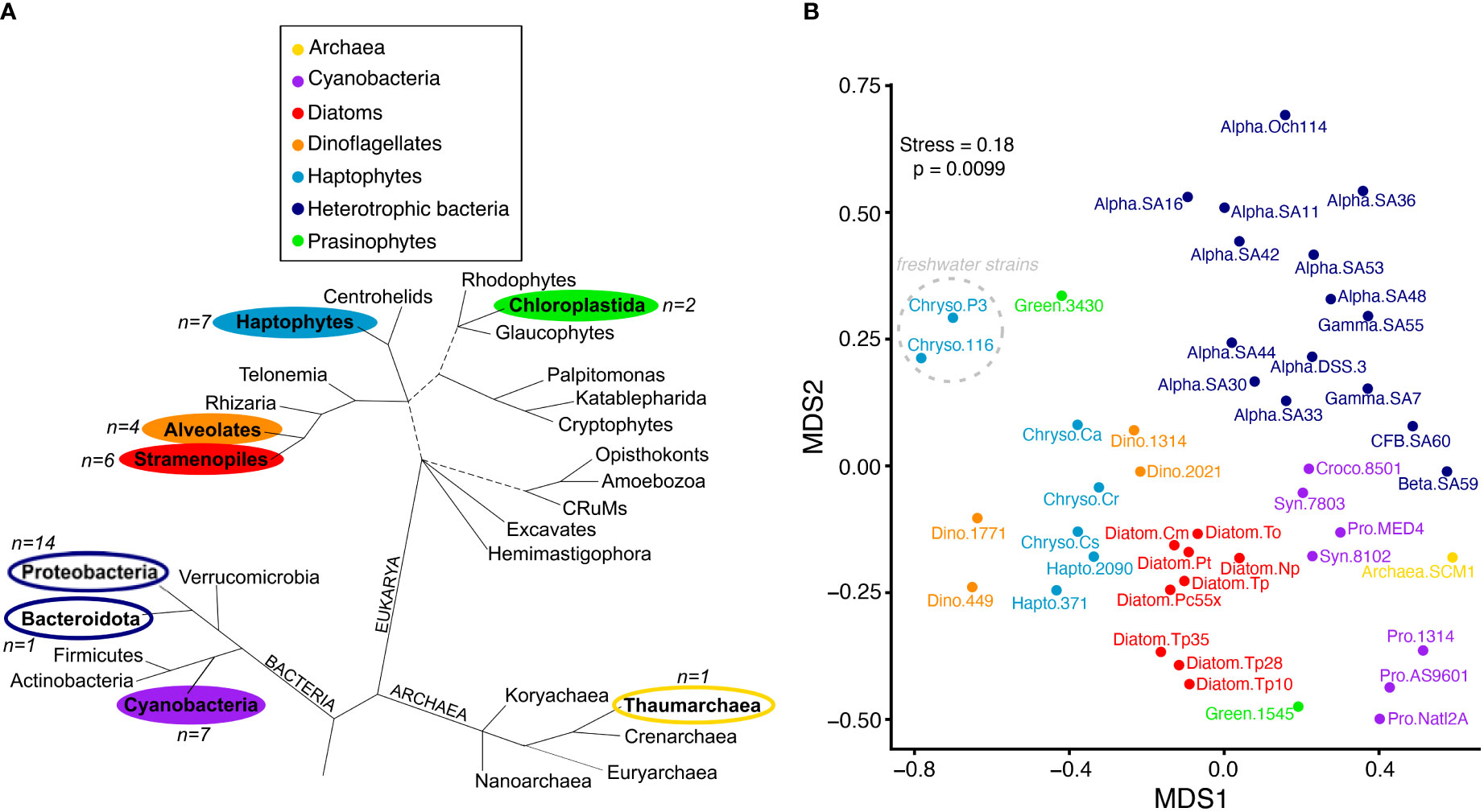
Figure 1 Metabolite profiles reflect taxonomy. (A) Major lineages of marine Bacteria, Archaea and Eukarya adapted from (Burki et al., 2020). Branch lengths are not drawn to scale, unresolved branching orders are shown as multifurcations, and uncertainties in the monophyly of groups are shown as broken lines. Lineages included in this study are in bold and colored according to the taxonomic groups shown in the legend that correspond to panel (B) The number of representatives used in this study from each lineage is indicated by n=X, with phytoplankton lineages indicated by filled colors and heterotrophic bacteria and archaea unfilled. B) NMDS ordination plot based on Jaccard distance using presence-absence of 111 metabolites. Metabolites similar to background levels were treated as absent. Organisms are labeled according to taxonomic group and strain (“group.strain” as in Table 1). The two freshwater Chrysochromulina strains are noted with the dashed gray circle. Colored taxonomic group abbreviations are the following: Cyanobacteria (purple; Croco, Crocosphaera; Pro, Prochlorococcus; Syn, Synechococcus); Archaea (yellow), Haptophytes (light blue; Chryso, Chrysochromulina; Hapto, Emiliania), Diatoms (red), Dinoflagellates (orange), Heterotrophic Bacteria (dark blue; Alpha, alphaproteobacteria; Gamma, gamaproteobacteria; Beta, betaproteobacteria; CFB, Cytophaga-Flavobacterium-Bacteroides); Prasinophytes (green).
Measured metabolites represent a variety of small molecules in central and secondary metabolism that include sugars, amino acids, organic acids, phytohormones, and osmolytes (Supplemental Table 1). After removal of metabolites added to culture media and those not detected in any of the 42 organisms (see Methods), there were 111 metabolites present in at least one culture with 99 found in more than one organism and 91 in more than two organisms. We did not determine absolute quantities of metabolites because accurate cell volumes were not measured for all organisms, and further, the differing biological matrices across samples cause varying ion suppression that make direct comparison of metabolite peak areas problematic (Chamberlain et al., 2019; Ghosson et al., 2021). In previous work, we quantified a subset of polar metabolites in 21 of the cultures based on measured or estimated cell volume in order to relate these data to field-based community observations (Heal et al., 2021). Here, to retain the most taxonomic diversity, we use presence-absence values based on quality-controlled peak areas in analyses presented herein, unless noted otherwise.
Metabolites reflect broad taxonomic groups
Hierarchical clustering and NMDS ordination of the presence-absence dataset showed that patterns in metabolite profiles reflect broad levels of taxonomy (Figure 1B), regardless of whether we removed metabolites detected in cultures below or near background levels from the clustering analysis or treated them as absent (Supplemental Figure 1). There was a clear separation of bacteria and archaea from the eukaryotic phytoplankton taxa, with the majority of heterotrophic bacteria, cyanobacteria, diatoms, haptophytes, and dinoflagellates forming distinct clusters. Most divergent in metabolite profile were the two green-lineage prasinophytes. This group contained fewer representatives compared to other taxonomic groups in the dataset, and additional analysis is warranted to resolve their metabolic variation given the ubiquitous contribution of this algal group to aquatic ecosystems (Leconte et al., 2020). Notably, there was a clear separation of the freshwater Chrysochromulina strains from the rest of the marine phytoplankton taxa, including the marine Chrysochromulina that clustered near Emiliania huxleyi haptophyte relatives and dinoflagellates.
Metabolites specific to phytoplankton and bacterial groups
Of the 111 metabolites measured (Figure 2 and Supplemental Table 1), we observed 14 metabolites exclusively in cyanobacteria and eukaryotic phytoplankton taxa and not in the examined heterotrophic bacteria or archaea (Figure 3 and Supplemental Table 5). When comparing between eukaryotic phytoplankton and prokaryotic groups, we excluded the five Chrysochromulina strains because they were not axenic. Of these 14 phytoplankton-derived metabolites, five were observed across both prokaryotic and eukaryotic phytoplankton taxa and included two sugars (sucrose, chitobiose) and three nitrogen-containing osmolytes and amino acid derivatives (hydroxyectoine, homarine, and trans-hydroxylproline). We note that hydroxyectoine is a metabolite produced conditionally in many organisms including bacteria and archaea (Czech et al., 2018) under high osmolarity conditions not captured with our culture conditions. We found no metabolites restricted to cyanobacteria, while nine of the fifteen phytoplankton metabolites were restricted to eukaryotic lineages. These included two nitrogen-containing carnitine derivatives (acetyl-L-carnitine and propionyl-L-carnitine) and seven sulfur-containing metabolites: the sulfonates 3-amino-1-propanesulfonate (homotaurine), methyltaurine, acetyltaurine, isethionate, and DHPS and the sulfoniums gonyol and DMSAc.
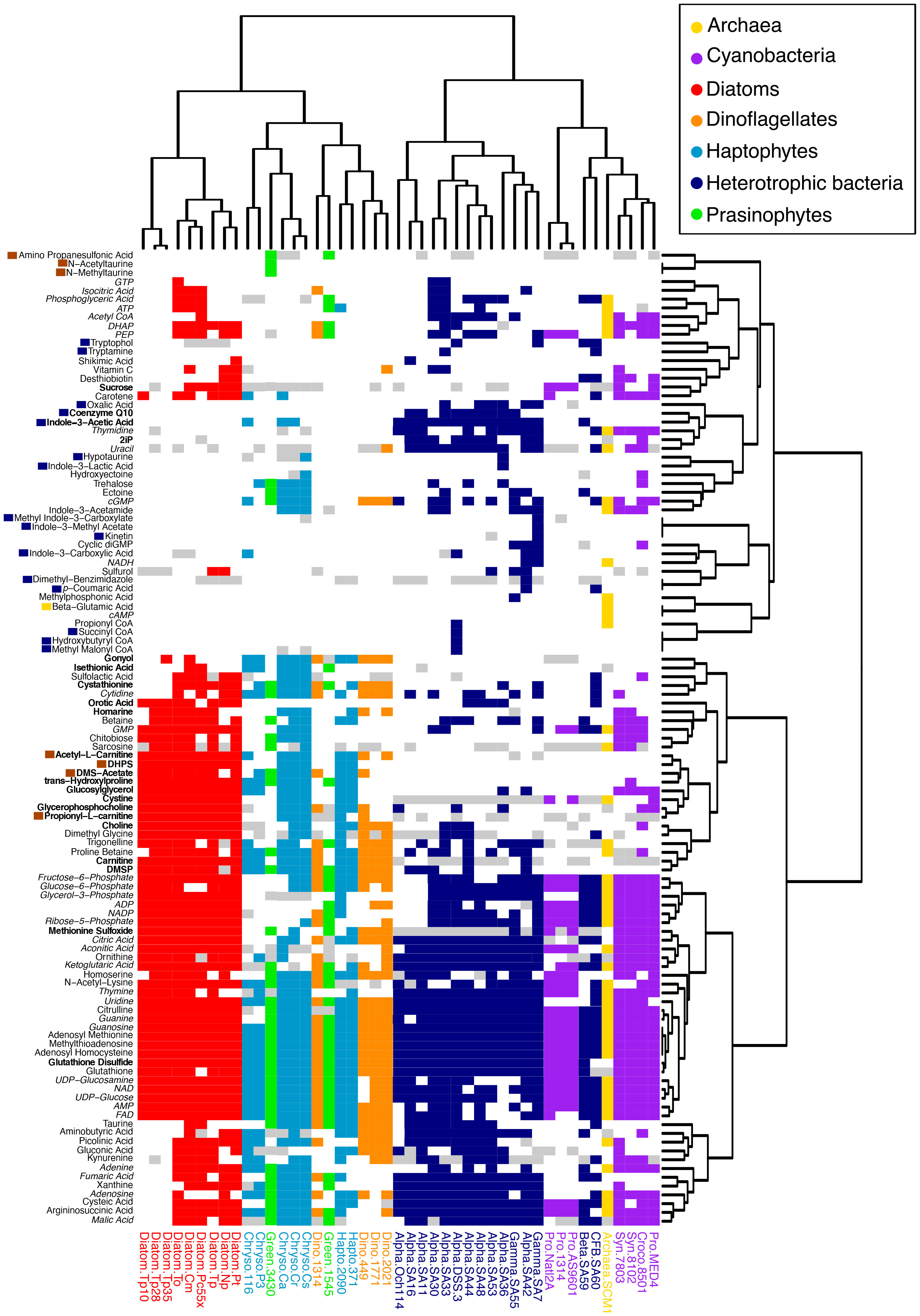
Figure 2 Distribution of 111 metabolites across 42 microbial taxa. Heatmap and hierarchical clustering dendrograms based on Jaccard distance using presence-absence of metabolites. Metabolite presence is colored according to the taxonomic groups in the legend, with white=metabolite absent and gray=metabolite similar to background levels. Metabolites in italics include universal electron transfer metabolites and nucleobases and their derivatives that are expected to be present in all organisms, though in some cases were not detected. Metabolites with dark blue boxes were restricted to heterotrophic bacteria, metabolites with brown boxes were restricted to eukaryotic phytoplankton, and one metabolite with a yellow box was restricted to the archaeal culture; note that non-axenic Chrysochromulina strains were not considered in this labeling scheme. Metabolites in bold were found to correspond with taxonomic groupings (see Figure 5). Organism labeling and coloring scheme are the same as in Figure 1.
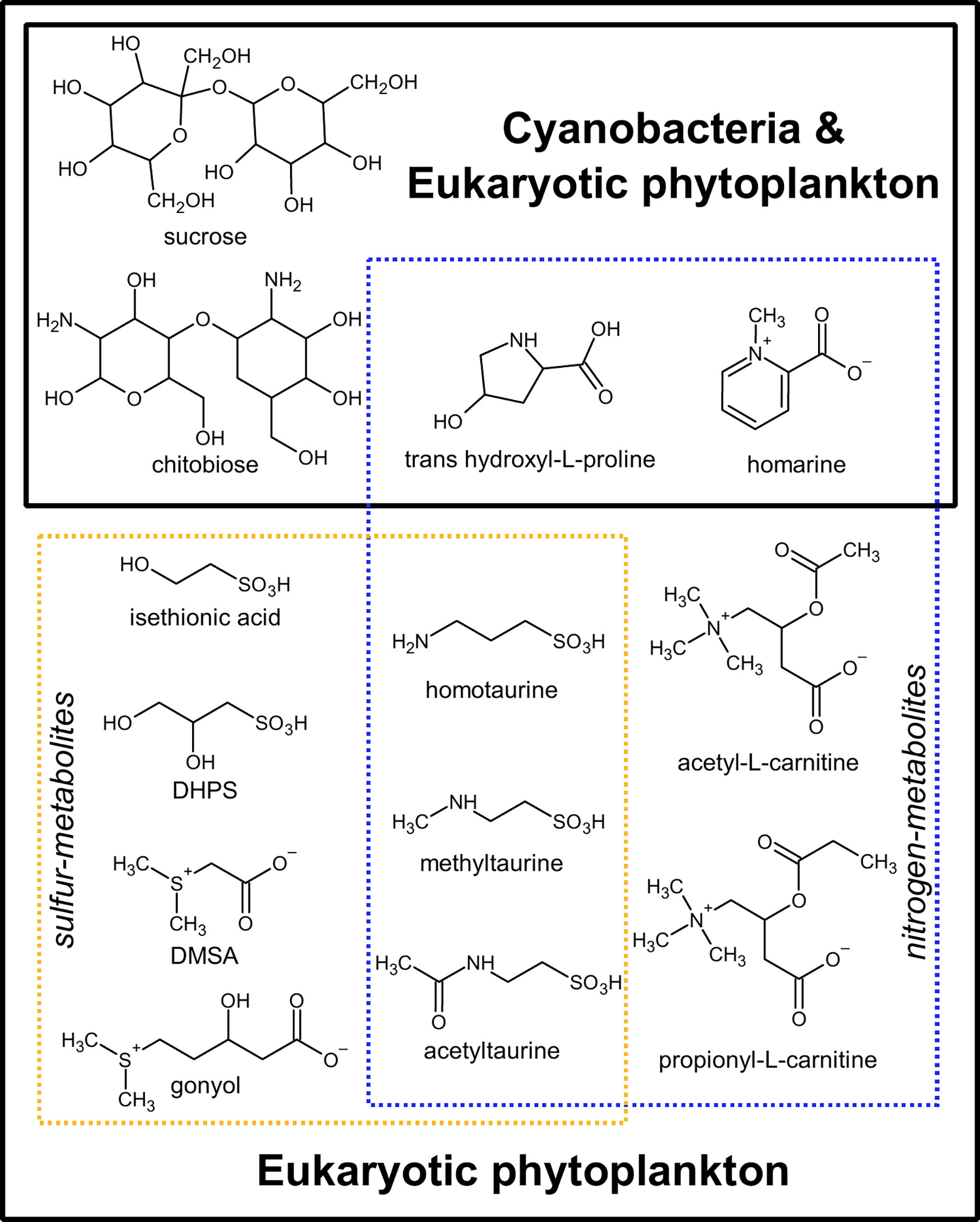
Figure 3 Thirteen metabolites exclusive to cyanobacterial and eukaryotic phytoplankton and absent in heterotrophic bacteria or archaea. Dashed blue lines: nitrogen-containing metabolites; Dashed yellow lines: sulfur-containing metabolites. Hydroxyectoine was also specific to phytoplankton in our analysis, but it was not included in the figure as there is evidence that it is conditionally produced in archaea and bacteria under high osmolarity conditions (Czech et al., 2018). DHPS, 2,3-dihydroxypropane-1-sulfonate; DMSAc, dimethylsulfonioacetate.
There were twenty-six metabolites observed only in prokaryotic (heterotrophic bacteria, cyanobacteria, and archaeal) taxa (Figure 2 and Supplemental Table 5). Again, we excluded the Chrysochromulina strains in this analysis because they were not axenic. A single molecule, β-glutamic acid, was restricted to the marine archaeon, consistent with previous metabolomic studies (Heal et al., 2021), and sixteen metabolites were found only in heterotrophic bacteria. The most striking pattern in this subset of metabolites was the prevalence of phytohormones and signaling molecules (Figure 4). These included the signaling molecules p-coumaric acid, cytokinin kinetin, and the indole derivatives indole acetic acid (IAA), methyl indole acetic acid, indole carboxylic acid, methyl indole carboxylic acid, indole lactic acid, tryptamine, and tryptophol (all restricted to the heterotrophic bacteria) as well as indole acetamide and cytokinin 6-(γ,γ-dimethylallylamino)purine (2iP) (found across bacterial and archaeal taxa).
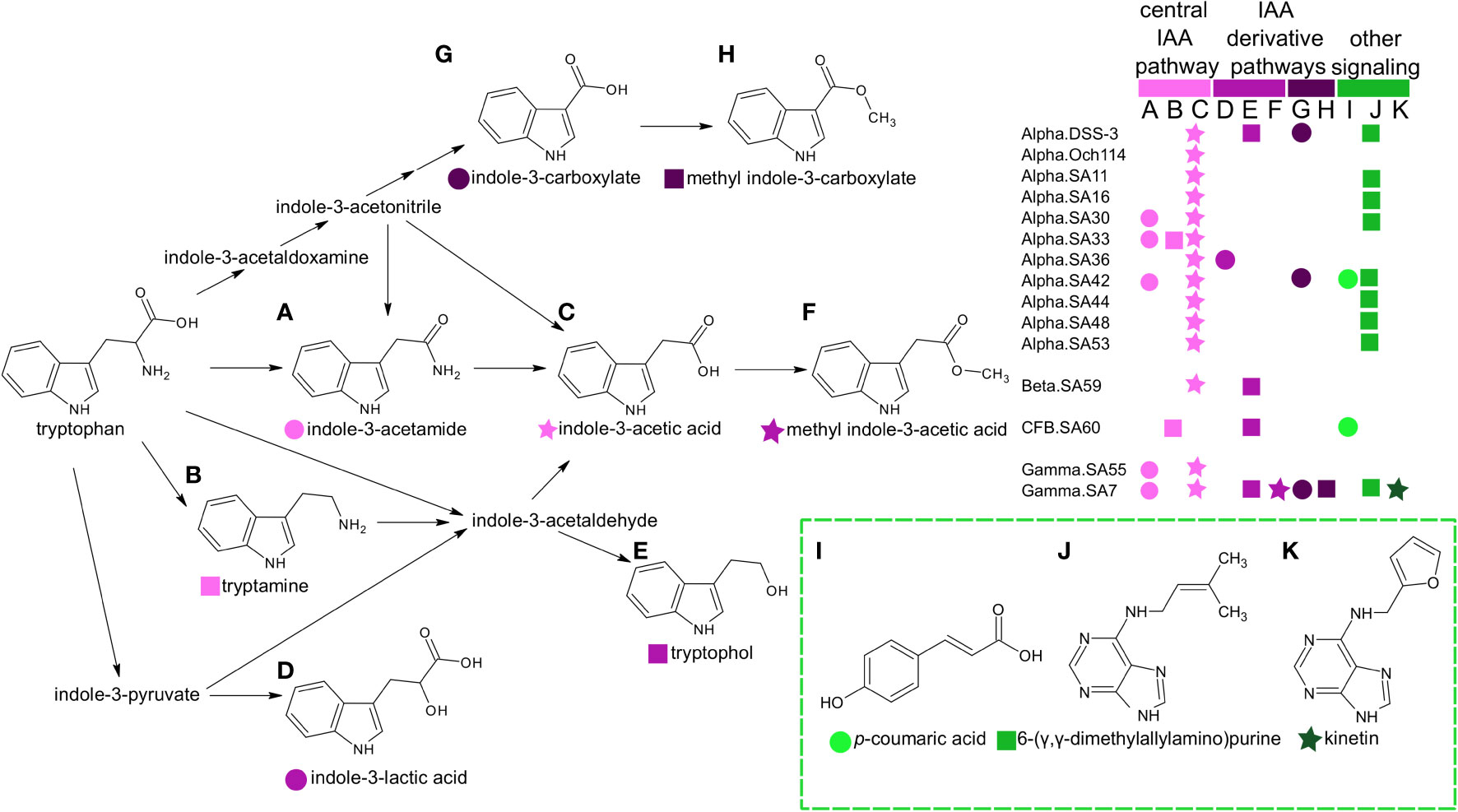
Figure 4 Distribution of phytohormones, cytokinins, and other potential signaling molecules in marine heterotrophic bacteria. Metabolites labeled A–H have shaded pink symbols based on whether they belong in the central pathways from tryptophan to IAA (light pink) or represent indole derivatives indole-3-lactic acid, tryptophol, methyl-indole-3-acetic acid (medium pink) or carboxylated forms (dark pink). These indole pathways have been previously described in bacteria and plants (Böttcher et al., 2014; Duca and Glick, 2020). Additional cytokinins and signaling molecules (labeled I–K) are colored green. The legend indicates the distribution of metabolites among the heterotrophic bacterial taxa used in this study. Bacteria are labeled according to taxonomic group and strain (“group.strain” as in Table 1). Group abbreviations are the following: Alpha, alphaproteobacteria; Gamma, gamaproteobacteria; Beta, =betaproteobacteria; CFB, Cytophaga-Flavobacterium-Bacteroides.
Metabolites from the five strains of Chrysochromulina clustered based on their marine or freshwater source (Figures 1B, 2). The two clusters grouped with other eukaryotic phytoplankton taxa, despite the presence of bacteria in each of these cultures. There were 31 metabolites differentially present between the marine and freshwater taxa (Supplemental Table 6). We found two metabolites indole-3-carboxylic acid and gluconic acid restricted to the freshwater strains, while the majority (n=29) of differential metabolites were present in one or more of the marine strains and absent from the freshwater strains. These 29 molecules included sugars (e.g. chitobiose), amino acid derivatives (e.g. citrulline, sarcosine, homoserine), and several molecules with compatible solute function (e.g. betaine, DHPS, taurine, ectoine, choline, homarine). Four of these molecules (DHPS, propionyl-L-carnitine, chitobiose, homarine) were constrained to phytoplankton taxa (Figure 3) and were most likely derived from Chrysochromulina, whereas the other molecules may represent a combined signal from the phytoplankton and bacteria. Notably, several sulfur-containing metabolites prevalent in marine eukaryotes were present across both the marine and freshwater strains (e.g. DMSP, DMSAc, gonyol, isethionate) indicating that these molecules may be widely distributed in aquatic environments, while others were only observed in marine Chrysochromulina strains (e.g. DHPS, taurine). Because we analyzed all Chrysochromulina strains in the same batch of samples and all samples contained the same number of cells, we were able to compare normalized peak area for metabolites of interest to determine whether their relative abundance differed between strains (Supplemental Table 3). Consistent with function as compatible solutes, DMSP and DMSAc all displayed higher peak area values in the marine strains as compared to the freshwater strains (35-840X DMSP and 105X DMSAc in marine versus freshwater strains). In contrast, isethionate and gonyol had similar peak area values across all Chrysochromulina strains.
The differential presence of compatible solutes across the Chrysochromulina strains led us to search a set of T. pseudonana cultures grown at three different salinities for corresponding metabolite changes. Five metabolites (homoserine, proline betaine, sarcosine, homarine, and betaine) were detected at 28 and 35 ppt but had signals below background levels in cells grown at the lowest salinity (10 ppt) (Supplemental Figure 2; Supplemental Table 4). A single metabolite, carotene, had the opposite trend and became depleted at 35 ppt salinity. These metabolome changes in T. pseudonana are consistent with increased compatible solute production with increased salinity. The samples from these salinity experiments clustered closely together and were similar to other diatoms (Figure 1B). Thus, presence-absence of these six particular metabolites did not exert a strong influence on the clustering by taxonomy, consistent with their generally broad distribution across organisms. Proline betaine and carotene were found in both marine and freshwater Chrysochromulina cultures, whereas the other four metabolites (homoserine, sarcosine, homarine, and betaine) were in common with the 29 metabolites observed only in the marine Chrysochromulina. These results are consistent with their direct use as compatible solutes (sarcosine, homarine, betaine) or their function as an intermediate in biosynthesis of compatible solutes (homoserine).
Metabolites that predict taxonomic groups
The taxonomic patterns in the presence-absence of metabolites suggested that differentially present metabolites could be used to categorize taxonomic groups (archaea, cyanobacteria, heterotrophic bacteria, diatoms, dinoflagellates, haptophytes, and prasinophytes). To explore associations between specific metabolites and taxonomic groups, we performed a multiple correspondence analysis (MCA) with the binary metabolite data (Figure 5 and Supplemental Figure 3). Many metabolites in our analysis are broadly present across taxa and/or are considered universal metabolites in microbial metabolism (Figure 2) and include electron transfer metabolites (e.g., FAD, NAD), nucleobases and their derivatives (e.g., AMP, ATP), and central carbon pathway metabolites (e.g, glycolysis and TCA cycle intermediates). Fifty-three metabolites remained after removal of these general metabolites. Using the 53 metabolites, MCA dimension 1 explained 22.7% of variance and primarily separated eukaryotic phytoplankton groups from bacteria and archaea, while dimension 2 explained 10.0% of variance and primarily separated cyanobacteria from heterotrophic bacteria and eukaryotic lineages from one another (Figure 5A). Ordination of these first two dimensions showed similar separation of taxonomic groupings to the NMDS (Figure 1B), indicating that the subset of 53 metabolites in the MCA could separate groups in a comparable manner to the NMDS that included all metabolites to preserve pairwise dissimilarity between samples.
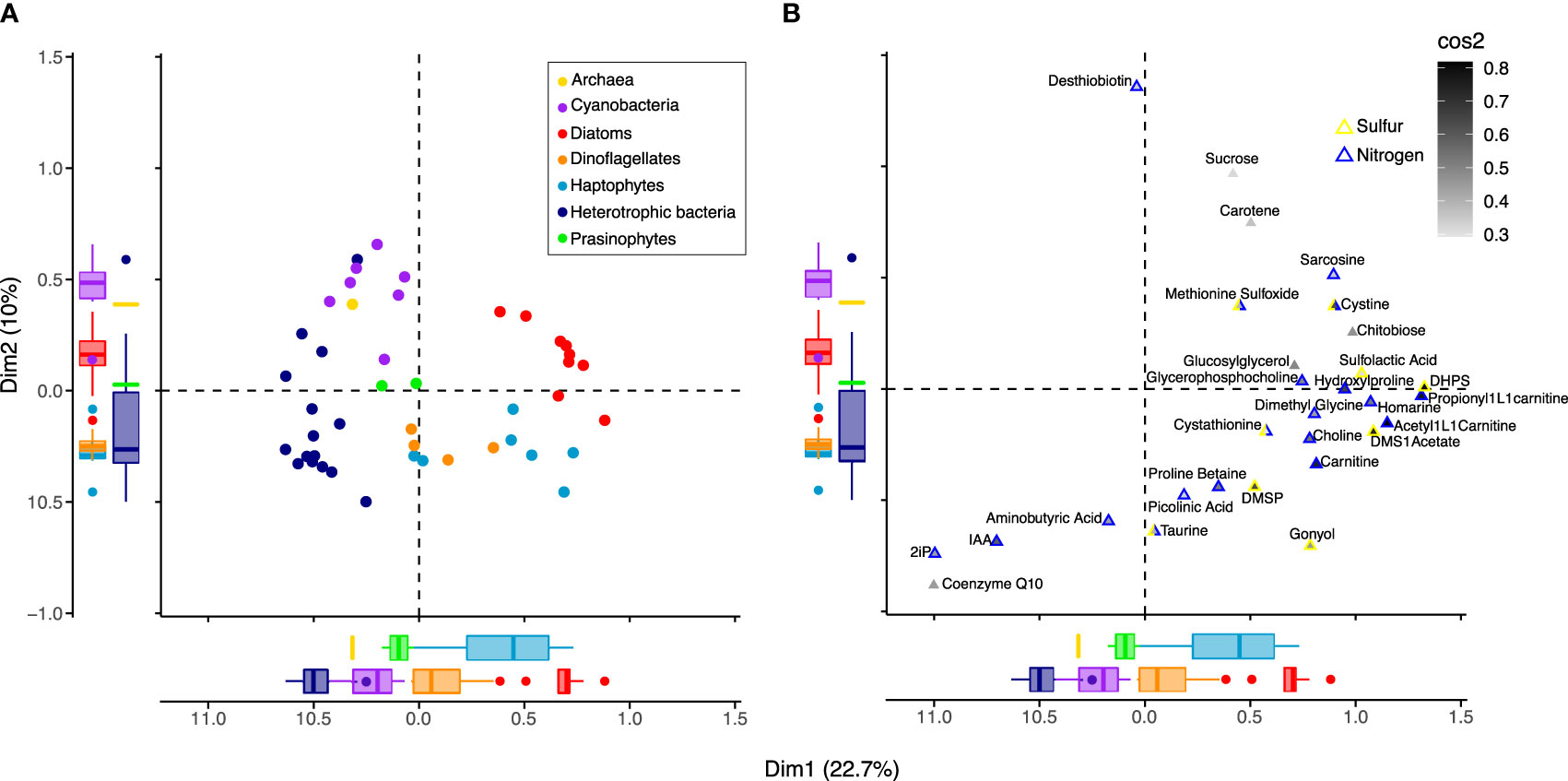
Figure 5 Associations between microbial groups and metabolites. Ordination based on Multiple Correspondence Analysis (MCA) using presence-absence of 53 metabolites in 42 organisms, with individuals (A) plotted separately from metabolites (B). Metabolites found in all organisms (n=4), metabolites found in <3 organisms (n=20), and universal metabolites (n=36; see Figure 2) were removed, and metabolites similar to background levels were treated as absent. The first two dimensions, Dim1 and Dim2, capture the greatest variation in the dataset, 22.7% and 10%, respectively. (A) Organisms (circles) are colored by group according to the legend, as in Figures 1, 2. Boxplots of the coordinates for each taxonomic group illustrate separation of groups from one another on Dim1 and Dim2. (B) Of the 42 metabolites found to have the strongest correlation with dimension axes (Supplemental Figure 4 and Supplemental Table 8), 29 were most strongly associated with Dim1 and Dim2. These 29 metabolites (triangles) are internally colored by the sum of their squared cosine (cos2) correlation values for dimensions 1 and 2 to indicate how closely their presence-absence is correlated with dimension axes. The metabolites (triangles) are externally colored according to whether they contain nitrogen (blue) and/or sulfur (yellow). The position of organisms and metabolites indicate their relationship with one another, where those with similar profiles are positioned closer together.
We next tested whether a smaller subset of metabolites could be used to categorize taxa by ranking metabolites based on their correlation strength with the MCA dimension axes (Supplemental Table 7). We incrementally removed metabolites from the MCA that contributed least to dimension axes, until there was a reduction in the separation of groups. This resulted in 42 metabolites used to separate groups (Supplemental Figure 4A), with 29 having strongest correlation values with the first two dimensions (Supplemental Figures 4B, C; Supplemental Table 8). These included chitobiose and glucosylglycerol, the nitrogen-containing metabolites choline, dimethyl glycine, sarcosine, homarine, glycerophosphocholine, trans-hydroxylproline, carnitine and two derivatives acetyl-L-carnitine and propionyl-L-carnitine, and the sulfur-containing metabolites cystine, cystathionine, methionine sulfoxide, DHPS, DMSP, gonyol, sulfolactic acid, and DMSAc associated with the positive pole of dimension 1 that corresponded with eukaryotic phytoplankton (Figure 5B; Supplemental Figure 3). Gonyol was associated with the positive pole of dimension 1 and negative pole of dimension 2, consistent with its dominance in haptophytes and dinoflagellates. Metabolites coenzyme Q10, IAA, and 2ip, found on the negative poles of dimensions 1 and 2, corresponded most closely with heterotrophic bacteria. Desthiobiton, observed in a small number of diatoms and bacteria, was associated with the positive pole of dimension 2. With positive values in both dimensions 1 and 2, sucrose and carotene corresponded with diatoms and cyanobacteria.With negative values in dimension 2, taurine, proline betaine, aminobutyric acid, and picolinic acid corresponded with heterotrophic bacteria, dinoflagellates, and a subset of prasinophytes and diatoms (Supplemental Table 5). Overall, many of the metabolites important in characterizing taxonomic groups in the MCA contained nitrogen and/or sulfur and overlapped with those specific to eukaryotic phytoplankton (Figure 3) and heterotrophic bacteria (Figure 4).
Discussion
Using the metabolomes of 42 microbial taxa, we identified groups of metabolites associated with broad levels of taxonomy. The relationship between phylogeny and metabolome has been demonstrated in phytoplankton using mass features from hybrid targeted/untargeted metabolomics data (Heal et al., 2021; Hughes et al., 2021) and in macroalgae using targeted metabolomics data (Hamid et al., 2019). By using a targeted analysis of intracellular metabolites in cultured plankton, we were able to identify specific metabolites that contribute to this relationship between phylogeny and chemotype. We identified 13 phytoplankton-derived molecules not present in the examined heterotrophic bacteria and archaea that include sugars, compatible solutes, and their derivatives (Figure 3). Conversely, we detected twenty-six prokaryotic-derived metabolites not present in the examined eukaryotic phytoplankton that were dominated by signaling molecules and hormones. Nine of the phytoplankton metabolites were constrained to eukaryotic lineages, but we did not identify cyanobacteria-specific metabolites. In contrast, untargeted metabolomics work of excreted metabolites has shown both cyanobacteria- and diatom-specific mass features (Becker et al., 2014), indicating that we have yet to identify the full suite of metabolites that differentiate microbial lineages. As additional microbial metabolites are characterized, the untargeted metabolomics dataset that we generated may serve as a resource to resolve chemotaxonomic distribution of newly identified plankton metabolites. While the taxa used in this study span major lineages in the surface ocean, some had few representatives, e.g., prasinophytes and archaea, and there are also important groups not represented in our study, e.g., SAR11. Still, these culture metabolomics data provide a scaffold for discerning metabolic pathways and corresponding genes for the transformation of metabolites of interest in field populations. For instance, using the distribution of sulfonates among microbial taxa in this dataset, we previously identified putative synthesis routes for C3-sulfonates produced by eukaryotic phytoplankton lineages that support heterotrophic bacterial associates (Durham et al., 2019).
The majority of the 13 phytoplankton-derived metabolites can act as substrates for heterotrophic bacteria, indicating that these metabolites may be useful targets for tracking phytoplankton-bacterial metabolite exchange. Indeed, several of these molecules (chitobiose, DHPS, isethionate, and acetyltaurine) have been implicated in resource partitioning among heterotrophic bacterial associates of eukaryotic phytoplankton (Ferrer-González et al., 2021). Though, it is worth noting that of the 36 diatom-derived metabolites identified to support bacterial resource partitioning (Ferrer-González et al., 2021), 29 were metabolites with broad distribution across microbial taxa such as amino acids. Thus, taxonomy can influence the types of metabolites present as well as the preferential exchange of those metabolites in establishing microbial interactions. Ten of the 13 phytoplankton-derived metabolites identified here (sucrose, DHPS, acetyltaurine, isethionate, homotaurine, DMSAc, gonyol, acetyl-L-carnitine, propionyl-L-carnitine) have been observed in field populations and display differential latitudinal and/or depth distribution in North Pacific particulate matter consistent with changes in phytoplankton taxonomic patterns (Heal et al., 2021). This handful of phytoplankton-derived molecules can serve as valuable targets for tracking metabolism of primary producer lineages to reconstruct their distributions and functions in the environment.
An interesting pattern within the phytoplankton metabolites was the differential use of nitrogen and sulfur, with sulfur-metabolites more prevalent in eukaryotic lineages and nitrogen-metabolites spanning across cyanobacteria and eukaryotic phytoplankton lineages (Figures 3, 5). Nitrogen-metabolites trans-hydroxylproline and homarine were present across eukaryotic phytoplankton (n=10/12 and 7/12, respectively) and cyanobacterial groups (n=1/7 and 2/7, respectively), while two carnitine derivates acetyl-L-carnitine (n=10/12) and propionyl-L-carnitine (n=8/12) were restricted to eukaryotes. As anticipated, we detected carnitine in the same eukaryotic cultures as its two derivates (Supplemental Table 5), and all three were associated with eukaryotic taxa in the MCA (Figure 5). While carnitine is common in eukaryotes, we note its presence in two bacterial cultures that belong to the Roseobacter group. Metabolomics-based characterization of carnitine metabolic pathways in soil bacteria has uncovered gene homologs in marine bacteria predicted to encode carnitine biosynthesis (Bazire et al., 2019), highlighting the power of metabolomics in revealing chemical phenotypes that are otherwise overlooked due to uncharacterized pathways. As additional cultures are analyzed by metabolomics approaches to fill in such gaps in our reconstruction of microbial metabolism, we may further constrain which metabolites are restricted to specific taxa.
Of the seven sulfur-metabolites specific to eukaryotic phytoplankton, three also contained a nitrogen atom (Figure 3). The prevalence of sulfur-metabolites in linking carbon transfer between eukaryotic phytoplankton and heterotrophic bacteria is a growing theme in marine biogeochemistry (Ksionzek et al., 2016; Levine, 2016; Moran and Durham, 2019). Historically, DMSP has been the most well-described marine sulfur-metabolite, in part, because its metabolism can lead to release of the volatile, climate-active gas dimethyl sulfide (DMS) (Yoch, 2002). It is an infochemical produced in high quantities by certain eukaryotic phytoplankton groups (Keller et al., 1989) and preferentially consumed by marine bacteria, with turnover rates indicating that DMSP can support over 5% of bacterial carbon demand (Kiene and Linn, 2000). We detected DMSP in 13 out of 14 eukaryotic phytoplankton and five out of 15 bacterial cultures and found it was a strong predictor of eukaryotic phytoplankton taxa (Figure 5), consistent with previous literature indicating that eukaryotic phytoplankton are major producers of DMSP in the surface ocean (Curson et al., 2018). Recognition of additional phytoplankton-derived sulfonium and sulfonate metabolites as constituents of the organic sulfur pool has altered our conceptualization of the marine sulfur cycle (Thume et al., 2018; Gebser et al., 2020; Heal et al., 2021). Here, we expand the chemotaxonomic resolution for sulfur-metabolites, particularly, those produced by eukaryotic phytoplankton (Figure 2), with DHPS and DMSAc most strongly associated with diatoms and haptophytes and gonyol with dinoflagellates (Figure 5). In addition, all three sulfonium molecules (DMSP, DMSAc, and gonyol) were present in at least one of the freshwater Chrysochromulina strains, implicating them in organic sulfur cycling in freshwater ecosystems. In comparing peak area values for these metabolites of interest, gonyol demonstrated peak areas comparable across marine and freshwater strains, in contrast to DMSAc and DMSP that had much lower peak areas in freshwater strains compared to marine. In marine bacteria, gonyol is not metabolized to volatile DMS, and it can inhibit DMS release in bacteria that catabolize DMSP (Gebser et al., 2020). This may indicate pronounced interference of volatile sulfur transformations in freshwater microbial communities, though the relevant taxa and quantitative importance of sulfoniums to freshwater systems are yet to be resolved.
Another striking pattern in the dataset was the wide array of phytohormones and signaling molecules present in heterotrophic bacteria. While most of these metabolites are recognized in land plants, the majority are not described in marine microbes. The few exceptions are bacterial-derived IAA that has been shown to enhance growth rate of diatoms (Amin et al., 2015) and phytoplankton-derived p-coumaric acid that acts as an infochemical for bacterial associates (Seyedsayamdost et al., 2011). Functions for the seven additional indole derivatives and the cytokinins kinetin and 2ip have yet to be functionally described in marine bacteria. We note that IAA, indole-3-acetamide, and methyl indole-3-carboxylate have been reported in samples from the open ocean (Boysen et al., 2021), with IAA and indole-3-acetamide displaying diel rhythmicity in their abundance. Production of cytokinin 2ip has been reported in planktonic bacteria isolated from a lake (Donderski and Głuchowska, 2000). While these environmental measures indicate active use of these metabolites in natural microbial populations, their specific functions and potential use in bacterial-phytoplankton interactions are unknown. In plants, the various functional groups of indole derivatives can alter solubility and diffusion of the molecules. For instance, methyl-IAA is more nonpolar than IAA and can more easily diffuse across membranes (Yang et al., 2008), enhancing transport of IAA (in the form of methyl-IAA) to neighboring cells and more distant targets. Such differences in hormone diffusion may be important for phycosphere-mediated interactions that occur within the diffusive boundary layer of planktonic cells (Seymour et al., 2017).
It is important to note that we cultured all organisms under optimal light, temperature, and nutrient-replete conditions, not reflective of typical environmental conditions. We have presented metabolite profiles based on the presence or absence of compounds, though we recognize that many metabolites are produced under specific conditions (Johnson et al., 2016; Heal et al., 2019) and/or produced at very low concentrations potentially below our limits of detection. Indeed, both environmental conditions and biotic interactions among microbial taxa may alter metabolome composition (Pontiller et al., 2020; Boysen et al., 2021; Raina et al., 2022). For instance, there were six metabolites differentially present between T. pseudonana grown under three different salinities, demonstrating that growth conditions can alter chemotype. Nonetheless, these diatom samples clustered closely together based on their metabolite profiles (Figures 1B, 2). In a previous metabolomics analysis of five diatom species grown under varying salinity and temperature conditions, metabolomes also clustered most strongly based on species identity rather than growth conditions (Dawson et al., 2020). In a lipidomics study of 13 diatom species, nitrogen availability affected metabolite accumulation but did not have a major influence on species-specific metabolite profiles (Bromke et al., 2015). Taken together, these diatom results indicated that genotype may be stronger in determining metabolic patterns than growth condition. Additional metabolomes from multiple taxa grown under a wider variety of growth conditions will help resolve which growth condition(s), or combination of conditions, exert the greatest influence on chemotype. We also observed clear separation between freshwater and marine species of Chrysochromulina driven by differential production of compatible solutes. With the caveats that these cultures were not axenic and grown on different media types, this result indicates the importance of environmental origin when measuring chemotype. Likewise, an untargeted metabolomics analysis of multiple strains of green alga Dunaliella tertiolecta collected from regions with varying salinity suggested that chemotyping may be more important than phylogenetic identification when exploring chemical diversity (Hughes et al., 2021).
Many of the metabolites discussed herein serve as substrates in microbial metabolism and trophic exchange with potential for rapid turnover rates, warranting further quantitative analyses to determine how they may serve as biomarkers in field populations. While some of these metabolites have reported observations in seawater, we have yet to resolve their distributions, functions, and cycling in natural microbial populations. New analytical approaches including single-cell imaging metabolomics allow resolution of metabolites exchanged in microbial consortia (Baumeister et al., 2020; Vallet et al., 2021). Analytical methods have also recently become available to measure polar metabolites like sulfonates, amino acids, and sugars in the dissolved fraction of seawater (Widner et al., 2021), opening up new avenues to compare concentrations of intracellular and excreted metabolites relevant to nutrient cycling. Such tools are critical for tracking the metabolism of ecologically relevant taxa and identifying biomarkers for risk assessment in a changing ocean (Gauthier et al., 2020). Through enhanced chemotaxonomic resolution of marine metabolites presented herein, we provide a resource for connecting microbial taxa to metabolic processes that ultimately shape ocean biogeochemistry.
Data availability statement
The names of the repository/repositories and accession number(s) can be found below: www.metabolomicsworkbench.org, PR000797 and PR001372, https://github.com/bpdurham/Culture_Metabolomics_PresenceAbsence.
Author contributions
BD, AB, KH, EA, and AI designed the study. BD, RB, CD, RC, and WQ cultured organisms. BD, AB, LC, KH, RB, and WQ generated and processed the metabolomics data. BD, AB, and KH performed data analysis. BD, AB, KH, EA, and AI wrote the manuscript with contributions from all authors. All authors contributed to the article and approved the submitted version.
Funding
This work was supported by grants from the National Science Foundation (Award ID OCE PRF-1521564 to BD), the Simons Foundation (SCOPE Award ID 329108 to EV and AI, SF Award ID 385428 to AI, SF Award ID 426570 to EA, SF Award ID 823165 to BD, and SF Award ID 548565 to WQ), the Gordon and Betty Moore Foundation (GBMF3776 to EA), and the U.S. Department of Energy (DE-EE0003046 for the National Alliance for Advanced Biofuels and Bioproducts to RC).
Acknowledgments
We acknowledge laboratory assistance from R. Morales, M. Schatz, G. Workman, N. Kellogg, A. Weid, and R. Lionheart. Bacterial cultures were kindly provided by J. Becker, S. Chisholm, A. Coe, M. Moran, and M. Saito.
Conflict of interest
Co-author KH is employed by Integral Consulting.
The remaining authors declare that the research was conducted in the absence of any commercial or financial relationships that could be construed as a potential conflict of interest.
Publisher’s note
All claims expressed in this article are solely those of the authors and do not necessarily represent those of their affiliated organizations, or those of the publisher, the editors and the reviewers. Any product that may be evaluated in this article, or claim that may be made by its manufacturer, is not guaranteed or endorsed by the publisher.
Supplementary material
The Supplementary Material for this article can be found online at: https://www.frontiersin.org/articles/10.3389/fmars.2022.864796/full#supplementary-material
References
Amin S. A., Hmelo L. R., van Tol H. M., Durham B. P., Carlson L. T., Heal K. R., et al. (2015). Interaction and signalling between a cosmopolitan phytoplankton and associated bacteria. Nature 522, 98–101. doi: 10.1038/nature14488
Armbrust E. V., Berges J. A., Bowler C., Green B. R., Martinez D., Putnam N. H., et al. (2004). The genome of the diatom Thalassiosira pseudonana: ecology, evolution, and metabolism. Science 306, 79–86. doi: 10.1126/science.1101156
Aylward F. O., Eppley J. M., Smith J. M., Chavez F. P., Scholin C. A., DeLong E. F. (2015). Microbial community transcriptional networks are conserved in three domains at ocean basin scales. Proc. Natl. Acad. Sci. 112, 5443–5448. doi: 10.1073/pnas.1502883112
Azam F., Fenchel T., Field J. G., Gray J. S., Meyer-Reil L. A., Thingstad F. (1983). The ecological role of water-column microbes in the sea. Mar. Ecol. Prog. Ser. 10, 257–263. doi: 10.3354/meps010257
Bar-On Y. M., Milo R. (2019). The biomass composition of the oceans: a blueprint of our blue planet. Cell 179, 1451–1454. doi: 10.1016/j.cell.2019.11.018
Baumeister T. U. H., Vallet M., Kaftan F., Guillou L., Svatoš A., Pohnert G. (2020). Identification to species level of live single microalgal cells from plankton samples with matrix-free laser/desorption ionization mass spectrometry. Metabolomics 16, 28. doi: 10.1007/s11306-020-1646-7
Bazire P., Perchat N., Darii E., Lechaplais C., Salanoubat M., Perret A. (2019). Characterization of l-carnitine metabolism in Sinorhizobium meliloti. J. Bacteriol. 201, e00772–e00718. doi: 10.1128/JB.00772-18
Becker J. W., Berube P. M., Follett C. L., Waterbury J. B., Chisholm S. W., DeLong E. F., et al. (2014). Closely related phytoplankton species produce similar suites of dissolved organic matter. Front. Microbiol. 5. doi: 10.3389/fmicb.2014.00111
Becker J. W., Hogle S. L., Rosendo K., Chisholm S. W. (2019). Co-Culture and biogeography of Prochlorococcus and SAR11. ISME J. 13, 1506–1519. doi: 10.1038/s41396-019-0365-4
Bench S. R., Heller P., Frank I., Arciniega M., Shilova I. N., Zehr J. P. (2013). Whole genome comparison of six Crocosphaera watsonii strains with differing phenotypes. J. Phycol. 49, 786–801. doi: 10.1111/jpy.12090
Biancarosa I., Belghit I., Bruckner C. G., Liland N. S., Waagbø R., Amlund H., et al. (2018). Chemical characterization of 21 species of marine macroalgae common in Norwegian waters: benefits of and limitations to their potential use in food and feed: Chemical composition of Norwegian marine macroalgae. J. Sci. Food Agric. 98, 2035–2042. doi: 10.1002/jsfa.8798
Böttcher C., Chapman A., Fellermeier F., Choudhary M., Scheel D., Glawischnig E. (2014). The biosynthetic pathway of indole-3-carbaldehyde and indole-3-carboxylic acid derivatives in Arabidopsis. Plant Physiol. 165, 841–853. doi: 10.1104/pp.114.235630
Bowler C., Allen A. E., Badger J. H., Grimwood J., Jabbari K., Kuo A., et al. (2008). The Phaeodactylum genome reveals the evolutionary history of diatom genomes. Nature 456, 239–244. doi: 10.1038/nature07410
Boysen A. K., Carlson L. T., Durham B. P., Groussman R. D., Aylward F. O., Ribalet F., et al. (2021). Particulate metabolites and transcripts reflect diel oscillations of microbial activity in the surface ocean. mSystems 6, e00896–20. doi: 10.1128/mSystems.00896-20
Boysen A. K., Durham B. P., Kumler W., Key R. S., Heal K. R., Carlson L. T., et al. (2022). Glycine betaine uptake and metabolism in marine microbial communities. Environ. Microbiol. 24, 2380–2403. doi: 10.1111/1462-2920.16020
Boysen A. K., Heal K. R., Carlson L. T., Ingalls A. E. (2018). Best-matched internal standard normalization in liquid chromatography-mass spectrometry metabolomics applied to environmental samples. Anal. Chem. 90, 1363–1369. doi: 10.1021/acs.analchem.7b04400
Bromke M. A., Sabir J. S., Alfassi F. A., Hajarah N. H., Kabli S. A., Al-Malki A. L., et al. (2015). Metabolomic profiling of 13 diatom cultures and their adaptation to nitrate-limited growth conditions. PLoS One 10, e0138965. doi: 10.1371/journal.pone.0138965
Burki F., Roger A. J., Brown M. W., Simpson A. G. B. (2020). The new tree of eukaryotes. Trends Ecol. Evol. 35, 43–55. doi: 10.1016/j.tree.2019.08.008
Cavaco M. A., Bhatia M. P., Hawley A. K., Torres-Beltrán M., Johnson W. M., Longnecker K., et al. (2022)Pathway-centric analysis of microbial metabolic potential and expression along nutrient and energy gradients in the Western Atlantic ocean (Accessed August 4, 2022).
Chamberlain C. A., Rubio V. Y., Garrett T. J. (2019). Impact of matrix effects and ionization efficiency in non-quantitative untargeted metabolomics. Metabolomics 15, 135. doi: 10.1007/s11306-019-1597-z
Chambers M. C., Maclean B., Burke R., Amodei D., Ruderman D. L., Neumann S., et al. (2012). A cross-platform toolkit for mass spectrometry and proteomics. Nat. Biotechnol. 30, 918–920. doi: 10.1038/nbt.2377
Chen Y.-B., Zehr J. P., Mellon M. (1996). Growth and nitrogen fixation of the diazotrophic filamentous nonheterocystous cyanobacterium Trichodesmium sp. IMS 101 in defined media: Evidence for a circadian rhythm. J. Phycol. 32, 916–923. doi: 10.1111/j.0022-3646.1996.00916.x
Cirri E., Pohnert G. (2019). Algae–bacteria interactions that balance the planktonic microbiome. New Phytol. 223, 100–106. doi: 10.1111/nph.15765
Curson A. R. J., Williams B. T., Pinchbeck B. J., Sims L. P., Martínez A. B., Rivera P. P. L., et al. (2018). DSYB catalyses the key step of dimethylsulfoniopropionate biosynthesis in many phytoplankton. Nat. Microbiol. 3, 430–439. doi: 10.1038/s41564-018-0119-5
Czech L., Hermann L., Stöveken N., Richter A. A., Höppner A., Smits S. H. J., et al. (2018). Role of the extremolytes ectoine and hydroxyectoine as stress protectants and nutrients: Genetics, phylogenomics, biochemistry, and structural analysis. Genes 9, 177. doi: 10.3390/genes9040177
Dawson H. M., Heal K. R., Torstensson A., Carlson L. T., Ingalls A. E., Young J. N. (2020). Large Diversity in nitrogen- and sulfur-containing compatible solute profiles in polar and temperate diatoms. Integr. Comp. Biol. 60, 1401–1413. doi: 10.1093/icb/icaa133
Deodato C. R., Barlow S. B., Hovde B. T., Cattolico R. A. (2019). Naked Chrysochromulina (Haptophyta) isolates from lake and river ecosystems: An electron microscopic comparison including new observations on the type species of this taxon. Algal Res. 40, 101492. doi: 10.1016/j.algal.2019.101492
Donderski W., Głuchowska M. (2000). Production of cytokinin-like substances by planktonic bacteria isolated from lake jeziorak. Pol. J. Environ. Stud. 9, 369–376.
Duca D. R., Glick B. R. (2020). Indole-3-acetic acid biosynthesis and its regulation in plant-associated bacteria. Appl. Microbiol. Biotechnol. 104, 8607–8619. doi: 10.1007/s00253-020-10869-5
Durham B. P. (2021). Deciphering metabolic currencies that support marine microbial networks. mSystems 6, e0076321. doi: 10.1128/mSystems.00763-21
Durham B. P., Boysen A. K., Carlson L. T., Groussman R. D., Heal K. R., Cain K. R., et al. (2019). Sulfonate-based networks between eukaryotic phytoplankton and heterotrophic bacteria in the surface ocean. Nat. Microbiol. 4, 1706–1715. doi: 10.1038/s41564-019-0507-5
Durham B. P., Dearth S. P., Sharma S., Amin S. A., Smith C. B., Campagna S. R., et al. (2017). Recognition cascade and metabolite transfer in a marine bacteria-phytoplankton model system. Environ. Microbiol. 19, 3500–3513. doi: 10.1111/1462-2920.13834
Eikrem W., Throndsen J. (1999). The morphology of Chrysochromulina rotalis sp. nov. (Prymnesiophyceae, haptophyta), isolated from the skagerrak. Sarsia 84, 445–449. doi: 10.1080/00364827.1999.10807349
Estep K. W., Davis P. G., Hargraves P. E., Sieburth J. M. (1984). Chloroplast containing microflagellates in natural populations of north Atlantic nanoplankton, their identification and distribution; including a description of five new species of Chrysochromulina (Prymnesiophyceae). Protistologica 20, 613–634.
Falkowski P. G., Wilson C. (1992). Phytoplankton productivity in the north pacific ocean since 1900 and implications for absorption of anthropogenic CO2. Nature 358, 741–743. doi: 10.1038/358741a0
Fei C., Ochsenkühn M. A., Shibl A. A., Isaac A., Wang C., Amin S. A. (2020). Quorum sensing regulates “swim-or-stick” lifestyle in the phycosphere. Environ. Microbiol. 22, 4761–4778. doi: 10.1111/1462-2920.15228
Ferrer-González F. X., Widner B., Holderman N. R., Glushka J., Edison A. S., Kujawinski E. B., et al. (2021). Resource partitioning of phytoplankton metabolites that support bacterial heterotrophy. ISME J. 15, 762–773. doi: 10.1038/s41396-020-00811-y
Gauthier L., Tison-Rosebery J., Morin S., Mazzella N. (2020). Metabolome response to anthropogenic contamination on microalgae: a review. Metabolomics 16, 8. doi: 10.1007/s11306-019-1628-9
Gebser B., Pohnert G. (2013). Synchronized regulation of different zwitterionic metabolites in the osmoadaption of phytoplankton. Mar. Drugs 11, 2168–2182. doi: 10.3390/md11062168
Gebser B., Thume K., Steinke M., Pohnert G. (2020). Phytoplankton-derived zwitterionic gonyol and dimethylsulfonioacetate interfere with microbial dimethylsulfoniopropionate sulfur cycling. MicrobiologyOpen 9, e1014. doi: 10.1002/mbo3.1014
Ghosson H., Guitton Y., Ben A. Jrad, Patil C., Raviglione D., Salvia M.-V., et al. (2021). Electrospray ionization and heterogeneous matrix effects in liquid chromatography/mass spectrometry based meta-metabolomics: A biomarker or a suppressed ion? Rapid Commun. Mass Spectrom. 35, e8977. doi: 10.1002/rcm.8977
Gómez-Consarnau L., Lindh M. V., Gasol J. M., Pinhassi J. (2012). Structuring of bacterioplankton communities by specific dissolved organic carbon compounds: Specialist and generalist DOC-degrading bacteria. Environ. Microbiol. 14, 2361–2378. doi: 10.1111/j.1462-2920.2012.02804.x
Gonsior M., Powers L., Lahm M., McCallister S. L. (2022). New perspectives on the marine carbon cycle–the marine dissolved organic matter reactivity continuum. Environ. Sci. Technol. 56, 5371–5380. doi: 10.1021/acs.est.1c08871
Grasshoff K., Ehrhardt M., Kremling K., Anderson L. G. (1999). Methods of seawater analysis (Weinheim; New York: Wiley-VCH).
Guillard R. R. L. (1975). “Culture of phytoplankton for feeding marine invertebrates,” in Culture of marine invertebrate animals: Proceedings — 1st conference on culture of marine invertebrate animals greenport. Eds. Smith W. L., Chanley M. H. (Boston, MA: Springer US), 29–60. doi: 10.1007/978-1-4615-8714-9_3
Guillard R. R. L., Hargraves P. E. (1993). Stichochrysis immobilis is a diatom, not a chrysophyte. Phycologia 32, 234–236. doi: 10.2216/i0031-8884-32-3-234.1
Hamid S. S., Wakayama M., Ichihara K., Sakurai K., Ashino Y., Kadowaki R., et al. (2019). Metabolome profiling of various seaweed species discriminates between brown, red, and green algae. Planta 249, 1921–1947. doi: 10.1007/s00425-019-03134-1
Heal K. R., Durham B. P., Boysen A. K., Carlson L. T., Qin W., Ribalet F., et al. (2021). Marine community metabolomes carry fingerprints of phytoplankton community composition. mSystems 6. doi: 10.1128/mSystems.01334-20
Heal K. R., Kellogg N. A., Carlson L. T., Lionheart R. M., Ingalls A. E. (2019). Metabolic consequences of cobalamin scarcity in the diatom Thalassiosira pseudonana as revealed through metabolomics. Protist 170, 328–348. doi: 10.1016/j.protis.2019.05.004
Henson S. A., Cael B. B., Allen S. R., Dutkiewicz S. (2021). Future phytoplankton diversity in a changing climate. Nat. Commun. 12, 5372. doi: 10.1038/s41467-021-25699-w
Holmes R. M., Aminot A., Kérouel R., Hooker B. A., Peterson B. J. (1999). A simple and precise method for measuring ammonium in marine and freshwater ecosystems. Can. J. Fish. Aquat. Sci. 56, 1801–1808. doi: 10.1139/f99-128
Hovde B. T., Deodato C. R., Andersen R. A., Starkenburg S. R., Barlow S. B., Cattolico R. A. (2019). Chrysochromulina: Genomic assessment and taxonomic diagnosis of the type species for an oleaginous algal clade. Algal Res. 37, 307–319. doi: 10.1016/j.algal.2018.11.023
Hovde B. T., Deodato C. R., Hunsperger H. M., Ryken S. A., Yost W., Jha R. K., et al. (2015). Genome sequence and transcriptome analyses of Chrysochromulina tobin: Metabolic tools for enhanced algal fitness in the prominent order Prymnesiales (Haptophyceae). PloS Genet. 11, e1005469. doi: 10.1371/journal.pgen.1005469
Hughes A. H., Magot F., Tawfike A. F., Rad-Menéndez C., Thomas N., Young L. C., et al. (2021). Exploring the chemical space of macro- and micro-algae using comparative metabolomics. Microorganisms 9, 311. doi: 10.3390/microorganisms9020311
Johnson W. M., Kido Soule M. C., Kujawinski E. B. (2016). Evidence for quorum sensing and differential metabolite production by a marine bacterium in response to DMSP. ISME J. 10, 2304–2316. doi: 10.1038/ismej.2016.6
Johnson W. M., Longnecker K., Soule M. C. K., Arnold W. A., Bhatia M. P., Hallam S. J., et al. (2020). Metabolite composition of sinking particles differs from surface suspended particles across a latitudinal transect in the south Atlantic. Limnol. Oceanogr. 65, 111–127. doi: 10.1002/lno.11255
Keeling P. J., Burki F., Wilcox H. M., Allam B., Allen E. E., Amaral-Zettler L. A., et al. (2014). The marine microbial eukaryote transcriptome sequencing project (MMETSP): Illuminating the functional diversity of eukaryotic life in the oceans through transcriptome sequencing. PloS Biol. 12, e1001889. doi: 10.1371/journal.pbio.1001889
Keller M. D., Bellows W. K., Guillard R. R. L. (1989). Dimethyl sulfide production in marine phytoplankton Biogenic sulfur in the environment ACS symposium series (American Chemical Society), 167–182. doi: 10.1021/bk-1989-0393.ch011
Kettler G. C., Martiny A. C., Huang K., Zucker J., Coleman M. L., Rodrigue S., et al. (2007). Patterns and implications of gene gain and loss in the evolution of Prochlorococcus. PloS Genet. 3, e231. doi: 10.1371/journal.pgen.0030231
Kiene R. P., Linn L. J. (2000). Distribution and turnover of dissolved DMSP and its relationship with bacterial production and dimethylsulfide in the gulf of Mexico. Limnol. Oceanogr. 45, 849–861. doi: 10.4319/lo.2000.45.4.0849
Ksionzek K. B., Lechtenfeld O. J., McCallister S. L., Schmitt-Kopplin P., Geuer J. K., Geibert W., et al. (2016). Dissolved organic sulfur in the ocean: Biogeochemistry of a petagram inventory. Science 354, 456–459. doi: 10.1126/science.aaf7796
Leadbeater B. S. C., Manton I. (1971). Fine structure and light microscopy of a new species of Chrysochromulina (C. acantha). Arch. Für Mikrobiol. 78, 58–69. doi: 10.1007/BF00409088
Lechtenfeld O. J., Hertkorn N., Shen Y., Witt M., Benner R. (2015). Marine sequestration of carbon in bacterial metabolites. Nat. Commun. 6, 6711. doi: 10.1038/ncomms7711
Leconte J., Benites L. F., Vannier T., Wincker P., Piganeau G., Jaillon O. (2020). Genome resolved biogeography of Mamiellales. Genes 11, 66. doi: 10.3390/genes11010066
Lê S., Josse J., Husson F. (2008). FactoMineR: An r package for multivariate analysis. J. Stat. Software 25, 1–18. doi: 10.18637/jss.v025.i01
Levine N. M. (2016). Putting the spotlight on organic sulfur. Science 354, 418–419. doi: 10.1126/science.aai8650
Levine N. M., Varaljay V. A., Toole D. A., Dacey J. W. H., Doney S. C., Moran M. A. (2012). Environmental, biochemical and genetic drivers of DMSP degradation and DMS production in the Sargasso Sea. Environ. Microbiol. 14, 1210–1223. doi: 10.1111/j.1462-2920.2012.02700.x
Lewis N. E., Nagarajan H., Palsson B. O. (2012). Constraining the metabolic genotype–phenotype relationship using a phylogeny of in silico methods. Nat. Rev. Microbiol. 10, 291–305. doi: 10.1038/nrmicro2737
Llewellyn C. A., Sommer U., Dupont C. L., Allen A. E., Viant M. R. (2015). Using community metabolomics as a new approach to discriminate marine microbial particulate organic matter in the western English channel. Prog. Oceanogr. 137, 421–433. doi: 10.1016/j.pocean.2015.04.022
Lommer M., Specht M., Roy A.-S., Kraemer L., Andreson R., Gutowska M. A., et al. (2012). Genome and low-iron response of an oceanic diatom adapted to chronic iron limitation. Genome Biol. 13, R66. doi: 10.1186/gb-2012-13-7-r66
MacLean B., Tomazela D. M., Shulman N., Chambers M., Finney G. L., Frewen B., et al. (2010). Skyline: an open source document editor for creating and analyzing targeted proteomics experiments. Bioinforma. Oxf. Engl. 26, 966–968. doi: 10.1093/bioinformatics/btq054
Martínez A., Ventouras L.-A., Wilson S. T., Karl D. M., DeLong E. F. (2013). Metatranscriptomic and functional metagenomic analysis of methylphosphonate utilization by marine bacteria. Front. Microbiol. 4. doi: 10.3389/fmicb.2013.00340
Mayali X., Weber P. K., Brodie E. L., Mabery S., Hoeprich P. D., Pett-Ridge J. (2012). High-throughput isotopic analysis of RNA microarrays to quantify microbial resource use. ISME J. 6, 1210–1221. doi: 10.1038/ismej.2011.175
Moore L. R., Coe A., Zinser E. R., Saito M. A., Sullivan M. B., Lindell D., et al. (2007). Culturing the marine cyanobacterium Prochlorococcus. Limnol. Oceanogr. Methods 5, 353–362. doi: 10.4319/lom.2007.5.353
Moran M. A., Durham B. P. (2019). Sulfur metabolites in the pelagic ocean. Nat. Rev. Microbiol. 17, 665–678. doi: 10.1038/s41579-019-0250-1
Moran M. A., Kujawinski E. B., Stubbins A., Fatland R., Aluwihare L. I., Buchan A., et al. (2016). Deciphering ocean carbon in a changing world. Proc. Natl. Acad. Sci. U. S. A. 113, 3143–3151. doi: 10.1073/pnas.1514645113
Morris J. J., Zinser E. R. (2013). Continuous hydrogen peroxide production by organic buffers in phytoplankton culture media. J. Phycol. 49, 1223–1228. doi: 10.1111/jpy.12123
Oksanen J., Blanchet F. G., Friendly M., Kindt R., Legendre P., McGlinn D., et al. (2020) Vegan: Community ecology package. Available at: https://CRAN.R-project.org/package=vegan.
Palenik B., Brahamsha B., Larimer F. W., Land M., Hauser L., Chain P., et al. (2003). The genome of a motile marine Synechococcus. Nature 424, 1037–1042. doi: 10.1038/nature01943
Palenik B., Grimwood J., Aerts A., Rouzé P., Salamov A., Putnam N., et al. (2007). The tiny eukaryote Ostreococcus provides genomic insights into the paradox of plankton speciation. Proc. Natl. Acad. Sci. 104, 7705–7710. doi: 10.1073/pnas.0611046104
Paoli L., Ruscheweyh H.-J., Forneris C. C., Hubrich F., Kautsar S., Bhushan A., et al. (2022). Biosynthetic potential of the global ocean microbiome. Nature 607, 111–118. doi: 10.1038/s41586-022-04862-3
Pontiller B., Martínez-García S., Lundin D., Pinhassi J. (2020). Labile dissolved organic matter compound characteristics select for divergence in marine bacterial activity and transcription. Front. Microbiol. 236511. doi: 10.3389/fmicb.2020.588778
Qin W., Amin S. A., Martens-Habbena W., Walker C. B., Urakawa H., Devol A. H., et al. (2014). Marine ammonia-oxidizing archaeal isolates display obligate mixotrophy and wide ecotypic variation. Proc. Natl. Acad. Sci. 111, 12504–12509. doi: 10.1073/pnas.1324115111
Qin W., Heal K. R., Ramdasi R., Kobelt J. N., Martens-Habbena W., Bertagnolli A. D., et al. (2017). Nitrosopumilus maritimus gen. nov., sp. nov., nitrosopumilus cobalaminigenes sp. nov., Nitrosopumilus oxyclinae sp. nov., and Nitrosopumilus ureiphilus sp. nov., four marine ammonia-oxidizing archaea of the phylum Thaumarchaeota. Int. J. Syst. Evol. Microbiol., 67, 5067–5079. doi: 10.1099/ijsem.0.002416
Raes E. J., Karsh K., Sow S. L. S., Ostrowski M., Brown M. V., van de Kamp J., et al. (2021). Metabolic pathways inferred from a bacterial marker gene illuminate ecological changes across south pacific frontal boundaries. Nat. Commun. 12, 2213. doi: 10.1038/s41467-021-22409-4
Raina J.-B., Lambert B. S., Parks D. H., Rinke C., Siboni N., Bramucci A., et al. (2022). Chemotaxis shapes the microscale organization of the ocean’s microbiome. Nature 605, 132–138. doi: 10.1038/s41586-022-04614-3
Read B. A., Kegel J., Klute M. J., Kuo A., Lefebvre S. C., Maumus F., et al. (2013). Pan genome of the phytoplankton Emiliania underpins its global distribution. Nature 499, 209–213. doi: 10.1038/nature12221
Rivers A. R., Smith C. B., Moran M. A. (2014). An updated genome annotation for the model marine bacterium Ruegeria pomeroyi DSS-3. Stand. Genomic Sci. 9, 11. doi: 10.1186/1944-3277-9-11
Rocap G., Larimer F. W., Lamerdin J., Malfatti S., Chain P., Ahlgren N. A., et al. (2003). Genome divergence in two Prochlorococcus ecotypes reflects oceanic niche differentiation. Nature 424, 1042–1047. doi: 10.1038/nature01947
Seyedsayamdost M. R., Case R. J., Kolter R., Clardy J. (2011). The Jekyll-and-Hyde chemistry of Phaeobacter gallaeciensis. Nat. Chem. 3, 331–335. doi: 10.1038/nchem.1002
Seymour J. R., Amin S. A., Raina J.-B., Stocker R. (2017). Zooming in on the phycosphere: the ecological interface for phytoplankton–bacteria relationships. Nat. Microbiol. 2, 1–12. doi: 10.1038/nmicrobiol.2017.65
Shibl A. A., Isaac A., Ochsenkühn M. A., Cárdenas A., Fei C., Behringer G., et al. (2020). Diatom modulation of select bacteria through use of two unique secondary metabolites. Proc. Natl. Acad. Sci. 117, 27445–27455. doi: 10.1073/pnas.2012088117
Suzuki R., Terada Y., Shimodaira H. (2019) Pvclust: Hierarchical clustering with p-values via multiscale bootstrap resampling. Available at: https://CRAN.R-project.org/package=pvclust.
Swingley W. D., Sadekar S., Mastrian S. D., Matthies H. J., Hao J., Ramos H., et al. (2007). The complete genome sequence of Roseobacter denitrificans reveals a mixotrophic rather than photosynthetic metabolism. J. Bacteriol. 189, 683–690. doi: 10.1128/JB.01390-06
Thume K., Gebser B., Chen L., Meyer N., Kieber D. J., Pohnert G. (2018). The metabolite dimethylsulfoxonium propionate extends the marine organosulfur cycle. Nature 563, 412–415. doi: 10.1038/s41586-018-0675-0
Vallet M., Kaftan F., Grabe V., Ghaderiardakani F., Fenizia S., Svatoš A., et al. (2021). A new glance at the chemosphere of macroalgal-bacterial interactions: In situ profiling of metabolites in symbiosis by mass spectrometry. Beilstein J. Org. Chem. 17, 1313–1322. doi: 10.3762/bjoc.17.91
Varaljay V. A., Robidart J., Preston C. M., Gifford S. M., Durham B. P., Burns A. S., et al. (2015). Single-taxon field measurements of bacterial gene regulation controlling DMSP fate. ISME J. 9, 1677–1686. doi: 10.1038/ismej.2015.23
Walker C. B., dela Torre J.R., Klotz M. G., Urakawa H., Pinel N., Arp D. J., et al. (2010). Nitrosopumilus maritimus genome reveals unique mechanisms for nitrification and autotrophy in globally distributed marine crenarchaea. Proc. Natl. Acad. Sci. 107, 8818–8823. doi: 10.1073/pnas.0913533107
Widner B., Kido Soule M. C., Ferrer-González F. X., Moran M. A., Kujawinski E. B. (2021). Quantification of amine- and alcohol-containing metabolites in saline samples using pre-extraction benzoyl chloride derivatization and ultrahigh performance liquid chromatography tandem mass spectrometry (UHPLC MS/MS). Anal. Chem. 93, 4809–4817. doi: 10.1021/acs.analchem.0c03769
Worden A. Z., Lee J.-H., Mock T., Rouzé P., Simmons M. P., Aerts A. L., et al. (2009). Green evolution and dynamic adaptations revealed by genomes of the marine picoeukaryotes Micromonas. Science 324, 268–272. doi: 10.1126/science.1167222
Yang Y., Xu R., Ma C., Vlot A. C., Klessig D. F., Pichersky E. (2008). Inactive methyl indole-3-acetic acid ester can be hydrolyzed and activated by several esterases belonging to the AtMES esterase family of Arabidopsis. Plant Physiol. 147, 1034–1045. doi: 10.1104/pp.108.118224
Yoch D. C. (2002). Dimethylsulfoniopropionate: Its sources, role in the marine food web, and biological degradation to dimethylsulfide. Appl. Environ. Microbiol. 68, 5804–5815. doi: 10.1128/AEM.68.12.5804-5815.2002
Keywords: metabolomics, phytoplankton, organic sulfur, sulfonates, hormones, infochemicals, bacteria
Citation: Durham BP, Boysen AK, Heal KR, Carlson LT, Boccamazzo R, Deodato CR, Qin W, Cattolico RA, Armbrust EV and Ingalls AE (2022) Chemotaxonomic patterns in intracellular metabolites of marine microbial plankton. Front. Mar. Sci. 9:864796. doi: 10.3389/fmars.2022.864796
Received: 28 January 2022; Accepted: 22 August 2022;
Published: 15 September 2022.
Edited by:
Jose M. Gonzalez, University of La Laguna, SpainReviewed by:
Lisa R. Moore, Macquarie University, AustraliaGwenael Piganeau, UMR7232 Biologie Intégrative des Organismes Marins (BIOM), France
Assaf Vardi, Weizmann Institute of Science, Israel
Copyright © 2022 Durham, Boysen, Heal, Carlson, Boccamazzo, Deodato, Qin, Cattolico, Armbrust and Ingalls. This is an open-access article distributed under the terms of the Creative Commons Attribution License (CC BY). The use, distribution or reproduction in other forums is permitted, provided the original author(s) and the copyright owner(s) are credited and that the original publication in this journal is cited, in accordance with accepted academic practice. No use, distribution or reproduction is permitted which does not comply with these terms.
*Correspondence: Bryndan P. Durham, Yi5kdXJoYW1AdWZsLmVkdQ==
†Present address: Bryndan P. Durham, Department of Biology, Genetics Institute, University of Florida, Gainesville, FL, United States Angela K. Boysen, Department of Chemistry, Pacific Lutheran University, Tacoma, WA, United States Katherine R. Heal, Integral Consulting Inc, Seattle, WA, United States Rachel Boccamazzo, Department of Physiology and Biophysics, University of Washington, Seattle, WA, United StatesWei Qin, Department of Microbiology and Plant Biology, University of Oklahoma, Norman, OK, United States