- 1First Institute of Oceanography, Ministry of Natural Resources, Qingdao, China
- 2Technology Innovation Center for Ocean Telemetry, Ministry of Natural Resources, Qingdao, China
- 3College of Oceanography and Space Informatics, China University of Petroleum, Qingdao, China
Using AIS data to mine the dynamic characteristics of fishery resource exploitation helps to carry out scientific management of fishery and realize the sustainable development of marine resources. We proposed a framework that integrates multiple AIS data processing and analysis modules, which can efficiently divide fishing voyages, determine the fishing activities and identify fishing types, and provide near real-time analysis results on the number of fishing vessels, fishing duration, voyages and so on. The framework was applied to 1.68 billion AIS trajectory data points of approximately 588,000 fishing vessels. We selected China’s sea areas overall and six fishing grounds as the research area, explored the characteristics of fishing vessel activities in winter and spring of 2019, and analyzed the impact of COVID-19 on winter-spring fishing in China in 2020. In 2019, our results showed that the number of fishing vessels in China’s sea areas gradually increased over time, with the Chinese New Year holiday affecting fishing activities at the corresponding time but having little impact on the entire month. We found that the changing laws of the fishing duration and voyages in the inshore fishing grounds were similar to those of the number of fishing vessels, which increased to varying degrees over time. Gillnetters were the most numerous fishing vessel type operating in the inshore fishing grounds with increased in spring, while seiners had an absolute advantage in the Xisha-Zhongsha fishing ground. In 2020, during the occurrence period of COVID-19, the fishing activities in China’s sea areas was almost unaffected. During the outbreak period, the number, distribution range, activity intensity, and fishing duration of fishing vessels all experienced a relatively large decline. After the epidemic was effectively controlled, they were rapidly increased. In addition, we found that compared with the Government Response Stringency Index, the number of fishing vessels and the number of new confirmed cases showed a more obvious negative correlation. By processing, mining and analyzing AIS data with high spatial-temporal granularity, this study can provide data support for the reasonable development of fishery resources, and help fishery practitioners make wise decisions when responding to unexpected emergencies (e.g. pandemics).
1 Introduction
China is one of the largest maritime states and has the largest fishery catch in the world (FAO, 2020). However, with the continuous development of the fishery economy and the gradual transformation of the economic growth mode, there are certain blind spots in the development and utilization of fishery resources in China, and these shortcomings have led to serious declines in China’s fishery resources. To ensure the sustainable development and utilization of fishery resources, it is necessary to effectively and reliably monitor fishing vessels, strengthen fishery management, and grasp the dynamic characteristics of fishery resource exploitation.
Currently, operational monitoring of fishing vessels is primarily carried out by three methods: on site monitoring, satellite remote sensing and the Automatic Identification System (AIS). The traditional maritime patrol and boarding are of high accuracy, but they are costly, risky, and monitor limited fishing vessels. When using remote sensing satellites to monitor fishing vessels, optical remote sensing and Synthetic Aperture Radar (SAR) images are used. Taking advantage of high spatial resolution, during daytime, optical satellites can directly monitor fishing vessels, providing valuable information about the vessels’ appearance (Wang et al., 2017; Xu et al., 2017; Li B. et al., 2020), and at night, mainly by detecting lights (such as fish collection lights) to obtain the distribution of fishing vessels (Yamaguchi et al., 2016; Ezequiel and Carlos, 2016). Compared with optical satellites, SAR is capable of working in all-day and all-weather conditions and can monitor target ships 24 hours a day to obtain information on the position, geometry and direction of navigation (Gerard et al., 2009; Zhao et al., 2013a; Sandirasegaram and Vachon, 2017; Chaturvedi, 2019). For optical satellite images, Zhang S. et al. (2019) used deep learning to develop a rapid regional-based convolutional neural network (R-CNN) technique for detecting ships in high-resolution remote sensing data. Li J. et al. (2021) used seasonally averaged nighttime light remote sensing data from 2016 to study the spatiotemporal patterns of illuminated fisheries in the South China Sea (SCS). To detect ships from SAR images, Chang et al. (2019) developed a deep learning framework based on You Only Look Once version 2 (YOLOv2), and the test results revealed an improvement in ship detection accuracy as well as a significant reduction in computational time when compared to Faster R-CNN. In pursuit of better ship detection results, Brekke et al. (2013) added a sub-band division method to enhance the contrast between small target ships and the background sea, based on the subaperture cross-correlation magnitude algorithm.
At present, marine vessels can be accurately detected by satellite remote sensing, but it is difficult to continuously monitor the activities of fishing vessels due to the long-time interval of its data acquisition. AIS data can provide ship information in near real-time, such as longitude, latitude, speed, heading, and the time stamp, making it possible to not only quickly obtain information on the operational activities of fishing vessels over a large area but also to monitor vessel behaviors and movement patterns on a finer scale (Tu et al., 2016; Yang et al., 2019). Thanks to the popularization of shipboard AIS equipment and the development of maritime ship communication and shore-based monitoring networks, the coverage, continuity and effectiveness of AIS data have reached unprecedented levels. Massive AIS datasets have become important data sources for studying vessel-related problems, and the mining of these data has become an important means by which fishery resource exploitation characteristics can be uncovered (Honda et al., 2017; Murray and Perera, 2021).
Currently, AIS data have been used to study the fishing intensities, exploitation trends and dynamic changes associated with fisheries. Natale et al. (2015) generated a map of fishing effort with high spatiotemporal resolution using a large AIS dataset from January to August 2014. After validating the results with detailed logbook data, it was found that the mapping accuracy was influenced by the coverage of AIS data. Similarly, Ferra et al. (2018) used AIS data from EU and non-EU fishing vessels to produce fishing activity maps of the Mediterranean Sea from 2012 to 2014. Kroodsma et al. (2018) created a dynamic footprint of global fishing effort using 22 billion AIS data from more than 70,000 industrial fishing vessels for the period of 2012~ 2016. Continuous data of 1403 trawlers, registered in the Xiangshan Port in China, were calculated fishing intensity to map the trawling effort by Zhang et al. (2016). Applying the methods of specialist experience, spatial statistics and data mining, Li X. et al. (2021) developed a thorough mining and analysis of the spatial characteristics of fishing intensity in the northern SCS. Guan et al. (2021) used 150 million AIS trajectory points to carry out the identification of the trawlers, gillnetters and seiners in the northern SCS, and the vessels’ spatial-temporal distribution, fishing durations and other activity patterns were analyzed in different seasons. The fine-scale spatial positioning data provided by AIS data can be used to analyze the spatiotemporal characteristics of fishing vessels as well as the distributions of fishery resources and fishing efforts. These data thus provide an opportunity to explore the fishery resource utilization status within a given fishing activity area.
On the other hand, the COVID-19 pandemic that started in late December 2019 created an unprecedented health crisis for human beings in recent global history (Hakovirta and Denuwara, 2020). To contain the disease, the Chinese government undertook timely isolation measures, such as implementing traffic controls, banning public events and gatherings, and closing schools and scenic spots, to reduce human mobility. These control measures effectively reduce the transmission of COVID-19 (Chinazzi et al., 2020; Tian et al., 2020). As the global COVID-19 pandemic worsens, many countries around the world have consecutively implemented similar isolation policies. Many major human activities in the fields of education, culture, transportation, and industrial manufacturing have been restricted on a global scale, and these changes have had a vast influence on almost all aspects of human society. Numerous studies have indicated that the measures taken to control the spread of the epidemic have affected fishery trade (FAO, 2021; Pérez and Vina, 2021), catches (Love et al., 2021) and port activities (Shi and Weng, 2020). For the fishing industry, according to Global Fishing Watch, as of the end of April 2020, global industrial fishing activity dropped by approximately 6.5% compared to the previous two years (2018 and 2019) due to the epidemic (Clavelle, 2021). However, this mainly represents changes in the activities of the world industrial fleet (fishing vessels over 24 m) and the impact of the epidemic on small-scale fisheries is not fully captured.
To analyze the impact of COVID-19 on fisheries, after using high-resolution PlanetScope data to monitor and compare the “fishing vessel area under zone” in three ports along the western coast of India, Avtar et al. (2021) found that nearly a quarter of the annual production of the ports was lost due to the lockdown. Das et al. (2021) conducted a rapid telephone survey of 176 wetland fishers, with the aim of assessing the impact of the COVID-19 closure on floodplain wetland fisher households. The data used in these previous studies suffered from many problems, such as small sample sizes, subjectivity, small time scales, and uncertainty factors, leading to the inability of researchers to understand the fishery situation at a macroscopic scale. The support of fine-scale spatial positioning data is thus urgently needed.
In addition, although positive progress has been made in using AIS data to analyze the current statuses of Chinese capture fisheries, some shortcomings and limitations, such as the small study area scope and the limitation to a single fishing type (trawler, seiner, etc.) are associated with these data, while the sea area of China is extensive and the fishing methods are diverse. Moreover, although a few reports have assessed the impact of the epidemic on China’s fisheries, most of the efforts published to date have focused on assessing the degree of damage in terms of postharvest, market and trade activities (Zhao et al., 2020; Liu et al., 2021), while there is a lack of high-precision and in-depth explorations of the impacts of the epidemic on fishery production.
Furthermore, there is a certain degree of seasonal variation in fishing activities. In summer, China’s sea areas are under a fishing moratorium. By law, all fishing vessels except those with fishing tackle, as well as auxiliary vessels, are required to remain ashore during that period. Autumn is the peak production season of China’s fisheries. Currently, researchers have conducted a series of analyses on China’s autumn fishery production (Wang et al., 2018; Yan et al., 2019; Su et al., 2021), while few studies have addressed winter or spring activity. In winter and spring, with the high market demand and rising prices for fisheries resources, many vessels fish at sea. Determining the characteristics of fishing during these seasons is thus conducive to improving fisheries management and protecting fishery resources. In addition, studies have corroborated that COVID-19 is transmitted primarily between people through respiratory droplets and direct contact (Huang et al., 2020; Li Q. et al., 2020; Yen et al., 2020). When the temperature is low, viruses can survive for a prolonged period of time. Thus, winter and spring are seasons of high occurrence of COVID-19 (Diao et al., 2020; Zhu and Xie, 2020; Nottmeyer and Sera, 2021). Combining the two factors above, we decided to evaluate and analyze the characteristics of fishing activities in winter and spring.
In response to the above problems, this paper first proposes a framework for processing and analyzing fishing vessel AIS data; while taking the China’s sea areas as the research area, we focus on six Chinese fishing grounds. Based on available AIS data, we analyze and mine the spatiotemporal characteristics of winter- and springtime fishing activities from the aspects of the number of fishing vessels, the vessels’ spatiotemporal distribution, and the fishing and voyage durations and conducted an objective and quantitative study of how the COVID-19 pandemic affected fishing vessel operations and thus caused fishery losses. This study is intended to provide a reference regarding the exploitation and scientific management of fishery resources and rational proposals and evidence with which policymakers and fishery practitioners can adjust their work plans and adapt to the new state caused by the crisis when responding to unexpected emergencies (e.g., pandemics).
The remainder of this paper is structured as follows: Section 2 introduces the study area, utilized data and the proposed framework by which the AIS data are processed and analyzed. Section 3 analyzes the regularity of fishing vessel activities in the winter and spring seasons of 2019. Section 4 analyzes the impacts of the epidemic on Chinese fisheries and the causes of these impacts. Finally, Section 5 presents the conclusions.
2 Data and Methods
2.1 Study Areas
China is one of the most important marine and fishery countries in the world. It has rich marine fishery resources, with a coastline of 18,000 kilometers. The sovereign territorial sea covers more than 3 million square kilometers and is divided into four sea areas: the Bohai Sea, the Yellow Sea, the East China Sea and the South China Sea.
The study area is divided into two parts (Figure 1). First, AIS data recorded by fishing vessels in China’s sea areas are used to study the winter and spring Chinese fishing activity patterns on a large scale. Second, as the latitudinal span of China’s sea areas is relatively large, there are obvious differences in the natural environments and fishery resources of various sea areas in China. Therefore, from north to south, we selected the Shidao, Lvsi-Dasha, Zhoushan, Minnan-Taiwan, Zhujiangkou and Xisha-Zhongsha fishing grounds for further analysis, as these grounds are richer in fishery resources and contain typical fishing activities. Detailed description of six fishing grounds is provided in Supplementary Material.
2.2 AIS Data
AIS data are mainly used to prevent ship collisions (Silveira et al., 2013) and monitor maritime navigation (Pelich et al., 2015; Zhang L. et al., 2019). Accumulated AIS data are also used to mine maritime traffic conflict trajectories (Lei, 2019), analysis ship behavior (Zhen et al., 2017) and curb illegal, unreported and unregulated (IUU) fishing (Akinbulire et al., 2017; Park et al., 2020). The coverage and validity of ship AIS data are important conditions for the analysis and mining of regional information and knowledge. Land-based AIS mainly receives ship information within 60 km offshore. Satellite-based AIS use satellite platforms to carry AIS receivers, allowing ship monitoring to occur anywhere in the world, particularly in areas devoid of land-based stations, such as open oceans and polar regions (Zhao et al., 2013b). To ensure the safety of fishing vessels at sea, China mandates that all fishing vessels be equipped with AIS equipment, thus ensuring the coverage of the data utilized herein. The AIS data used in this work were purchased from Bomao Xin (Beijing) Technology Co. Ltd. (http://ship.chinaports.com/) and were collected by satellite and shore-based AIS receivers worldwide in the time frame from 2018 to 2020.
Since fishing operations are mostly scheduled by Chinese fishers according to the traditional lunar calendar, we purposely selected AIS data recorded by fishing vessels operating in China’s sea areas in the corresponding periods from December to March in 2019 and 2020 (i.e., December 2018, January 2019, February 2019, March 2019, December 2019, January 2020, February 2020 and March 2020) of the lunar calendar as the research object. The purpose of this study was to obtain the spatiotemporal characteristics of fishing activities in winter and spring by fully mining these data, as this information can help governments and fishery authorities at all levels effectively manage the exploitation of fishery resources and provide a scientific basis by which the optimal management of fishery resources can be promoted. The comparison table of lunar and solar calendar time are shown in Supplementary Table 1.
2.3 Methods
Fishing vessels are the vessels that carry out fish catching, processing and transportation activities in the oceans. Analyzing the movement trajectories of these vessels at sea and mining the information hidden in these trajectories can provide a scientific reference with which marine fishery resources can be analyzed. After the preprocessing steps (as shown in Supplementary Material), the obtained fishing vessel trajectory AIS dataset contained approximately 1.68 billion AIS data points, and the cumulative number of fishing vessels involved was approximately 588,000.
To ensure the efficient and accurate mining of the activity patterns of fishing vessels in China’s sea areas, we proposed a framework for processing and analyzing the fishing vessel AIS data. The overall architecture of this framework is shown in Supplementary Figure 1. More specifically, the architecture mainly includes three data processing modules and four data analysis modules; in these modules, the batch trajectory data of fishing vessels are managed and processed and the fishing operation characteristics of fishing vessels in different months and different seas are explored from multiple aspects. In this framework, the input AIS data are directly statistically analyzed to obtain basic information such as the number, size and activity region of each vessel as the datapoints are input into the voyage division module. In the voyage division module, the voyage set of each fishing vessel is obtained by the time threshold method, and the number and duration of the voyages are analyzed. Based on these voyage sets, first, the cumulative and average fishing times of the fishing vessel are calculated; next, the fishing vessel type identification model is applied to identify the operating type of the analyzed fishing vessel, and based on this information, the fishing vessel status identification module uses the speed threshold method to identify the status of each fishing vessel’s track points. In the Supplementary Material, we describe in detail the implementation of each data processing module.
3 Regularity of Fishing Vessel Activities in the Winter and Spring of 2019
To explore the spatiotemporal characteristics of Chinese fishing activities in winter and spring, we chose to analyze the AIS data recorded by fishing vessels in 2019 in terms of the number of fishing vessels, the vessels’ activity distributions, fishing durations, and voyages.
3.1 Changes in the Number of Fishing Vessels in China’s Sea Areas
Figure 2A shows the cumulative number of fishing vessels operating in China’s sea areas in each lunar month. According to experience, the first day of the first month of the lunar calendar is the Chinese New Year, the most important traditional festival in China. During the Chinese New Year, most Chinese people from all walks of life stop working to reunite with their families. Kroodsma et al. (2018) pointed out that the activity of Chinese fishing vessels declines during the Lunar New Year. However, as we can see from Figure 2A, the number of fishing vessels in lunar January, when the Chinese New Year was held in 2019, was maintained and even increased slightly compared to that of the previous month. It should be noted that our statistics were for the entire month of lunar January, while Kroodsma et al. (2018) referred only to the Chinese New Year holiday. To investigate the reasons for the above phenomenon above, we further counted the daily number of fishing vessels in lunar January (February 5 to March 6 on the solar calendar) of 2019; the results are shown in Figure 2B.
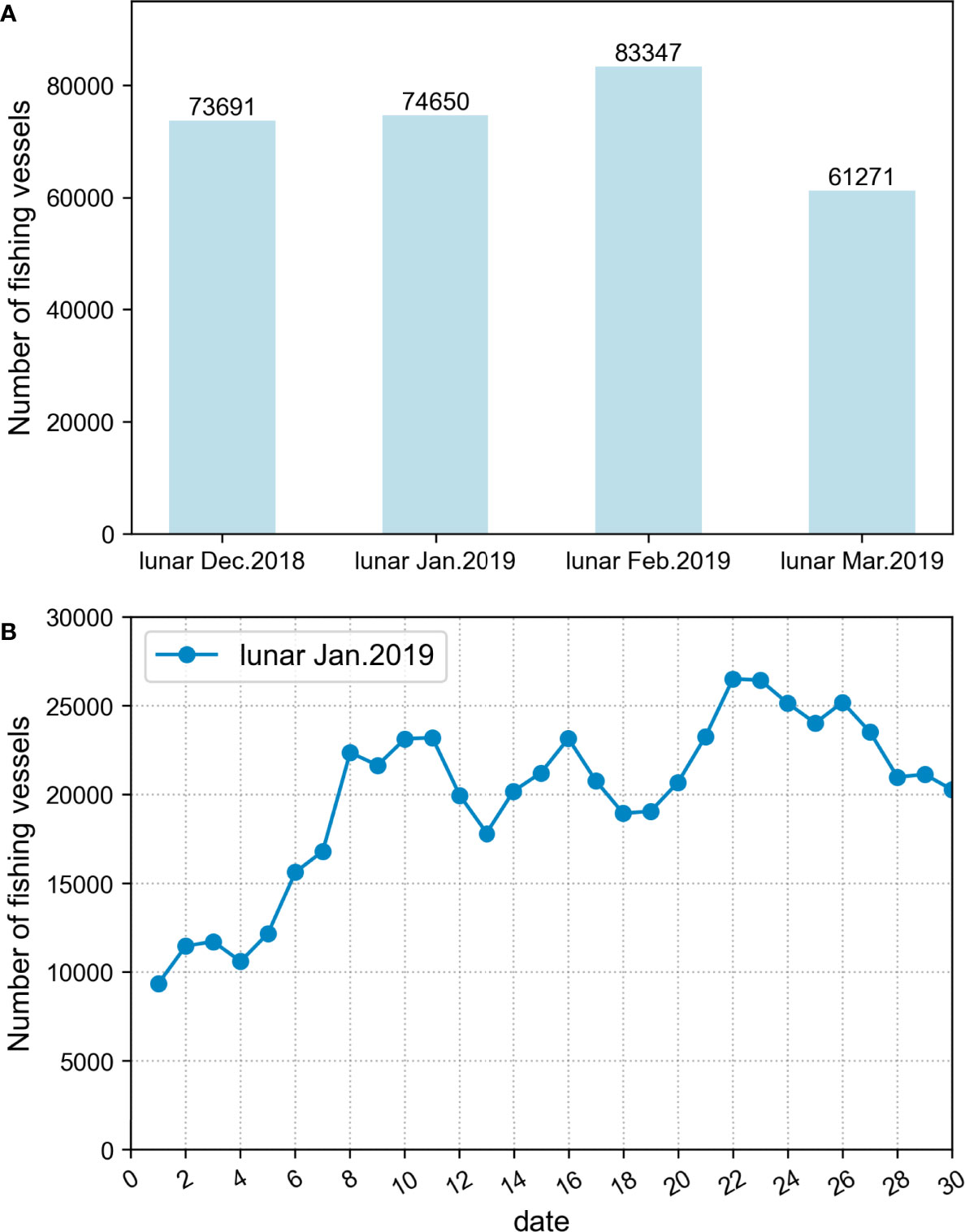
Figure 2 (A) The number of active fishing vessels in China’s sea areas in the winter and spring of 2019, and (B) the daily number of fishing vessels in China’s sea areas in lunar January of 2019.
The Chinese New Year holiday lasted from lunar January 1 to 6 (February 5 to 10 on the solar calendar). As shown in Figure 2B, the daily number of fishing vessels was indeed low during the holiday, which is consistent with previous finding. However, rapid growth of 33.23% was recorded by the second day after the holiday, which is lunar January 8 (February 12 on the solar calendar). In the following days, the daily number of fishing vessels has remained at a high level, suggesting that although some fishers reduced their fishing time to reunite with their families during the Chinese New Year, the fishers quickly returned to work to catch fish after the holidays. This information helps explain why the suspension of fishing vessels during the Chinese New Year did not reduce the number of fishing vessels in the entire month of lunar January.
In lunar February (March 7 to April 4 on the solar calendar), the number of fishing vessels increased slightly, but in lunar March (April 5 to May 4 on the solar calendar), the number of fishing vessels decreased by approximately 26.5%. According to the relevant regulations of the Chinese government, after April 1 on the solar calendar, some regions of the China’s sea areas enter a fishing moratorium, and the number of fishing vessels at sea thus begins to decrease. Until May 1 on the solar calendar, the Bohai Sea, Yellow Sea, East China Sea and the region north of the 12°N South China Sea all entered the Forbidden Fishing Period. According to Supplementary Table 1, in the third lunar month of 2019, various sea areas consecutively entered the fishing moratorium, and the adopted fishing bans thus resulted in a decrease in the number of fishing vessels recorded at sea in this month.
3.2 Vessel Activity Distributions
Figure 3 shows the distribution densities of fishing vessel trajectory points in China’s sea areas in winter and spring of 2019, thus indirectly reflecting the distribution of sites with fishing value in fishery production. From an overall viewpoint, the fishing vessel activity intensity was high in the inshore region and low in the high-sea region, and the regional differences were quite obvious. After obtaining statistics on the lengths of fishing vessels, we found that the important reason for this difference was that the fishing vessels in the study area consisted largely of small and medium-sized fishing vessels, with the proportion of vessels less than 30 m in length reaching 60%; vessels of this size can operate only in inshore waters due to the limitations associated with maneuverability, replenishment ability and vessel load. Moreover, in terms of the overall fishing situation, the intensity of fishing vessel activities in the coastal waters of the East China Sea was relatively high, especially near Zhoushan fishing grounds. In lunar March (April 5 to May 4 on the solar calendar), due to the implementation of the fishing ban, the activity density in this sea area also decreased most significantly.
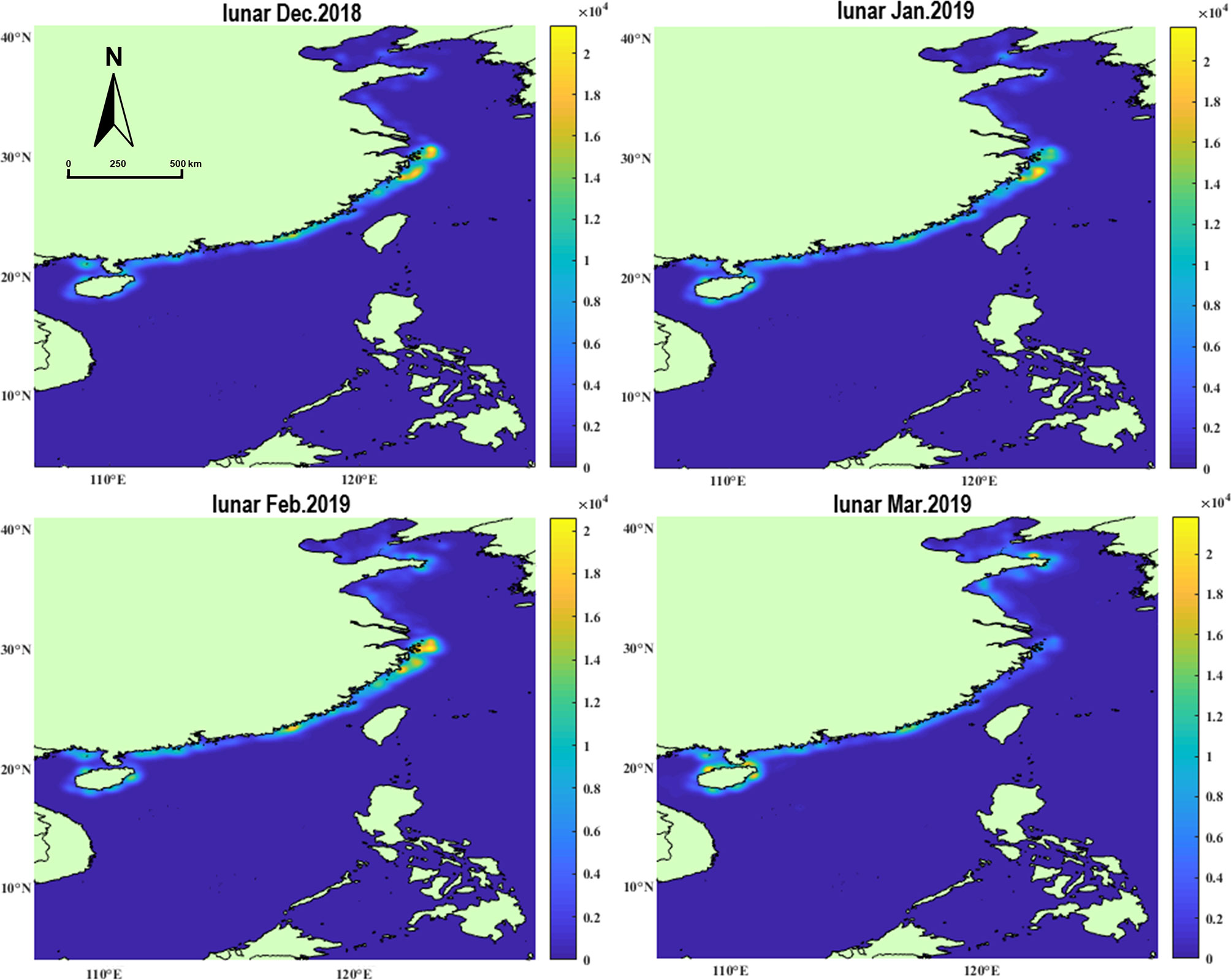
Figure 3 Distribution density map of fishing vessels trajectory points in China’s sea areas in the winter and spring of 2019 (points per km2).
Compared with other sea areas, in winter, the activity density of fishing vessels in the northern Bohai Sea was relatively low. After checking the relevant information, this was due to the frequent occurrence of strong cold currents and large-scale icing on the sea surface (Su and Wang, 2012). A large number of fishing vessels were frozen on the sea by this sea ice, making it impossible for fishers to go fishing, and a small number of fishing vessels thus went to the warmer Yellow Sea to fish. In lunar February and March (March 7 to May 4 on the solar calendar), as the sea ice melted with increasing temperatures, the activity density of the fishing vessels gradually increased.
3.3 Six Fishing Grounds
There are a large number of fishing vessels in the vast China’s sea areas, and it is difficult to perform refined information mining on the extensive AIS data generated by these vessels. In addition, the large latitudinal spans of China’s sea areas induce substantial differences between the natural environments and fishery resources of the southern and northern waters. Therefore, we selected six important fishing grounds in China from north to south as the research objects and explored the fishing characteristics of fishing vessels in different geographical locations in China’s sea areas in winter and spring based on the numbers of fishing vessels, operation times, voyages and fishing types.
3.3.1 Changes in the Number of Fishing Vessels in Fishing Grounds
Except for Xisha-Zhongsha, the changes in the number of fishing vessels within the five inshore fishing grounds (Figure 4) had similar variation tendencies to those in China’s sea areas overall (Figure 2A). Among the six fishing grounds, the Zhoushan, which contains the richest fishery resources, had the largest number of fishing vessels, while the number of vessels in Xisha-Zhongsha, the farthest-offshore region, was lower. However, it is worth noting that the number of fishing vessels in Xisha-Zhongsha increased significantly, by approximately 181%, in lunar February (March 7 to April 4 on the solar calendar) of 2019 compared to the numbers recorded in the previous two months and continued to increase in lunar March (April 5 to May 4 on the solar calendar), indicating that the fishery exploitation intensity in this fishing area was gradually increasing.
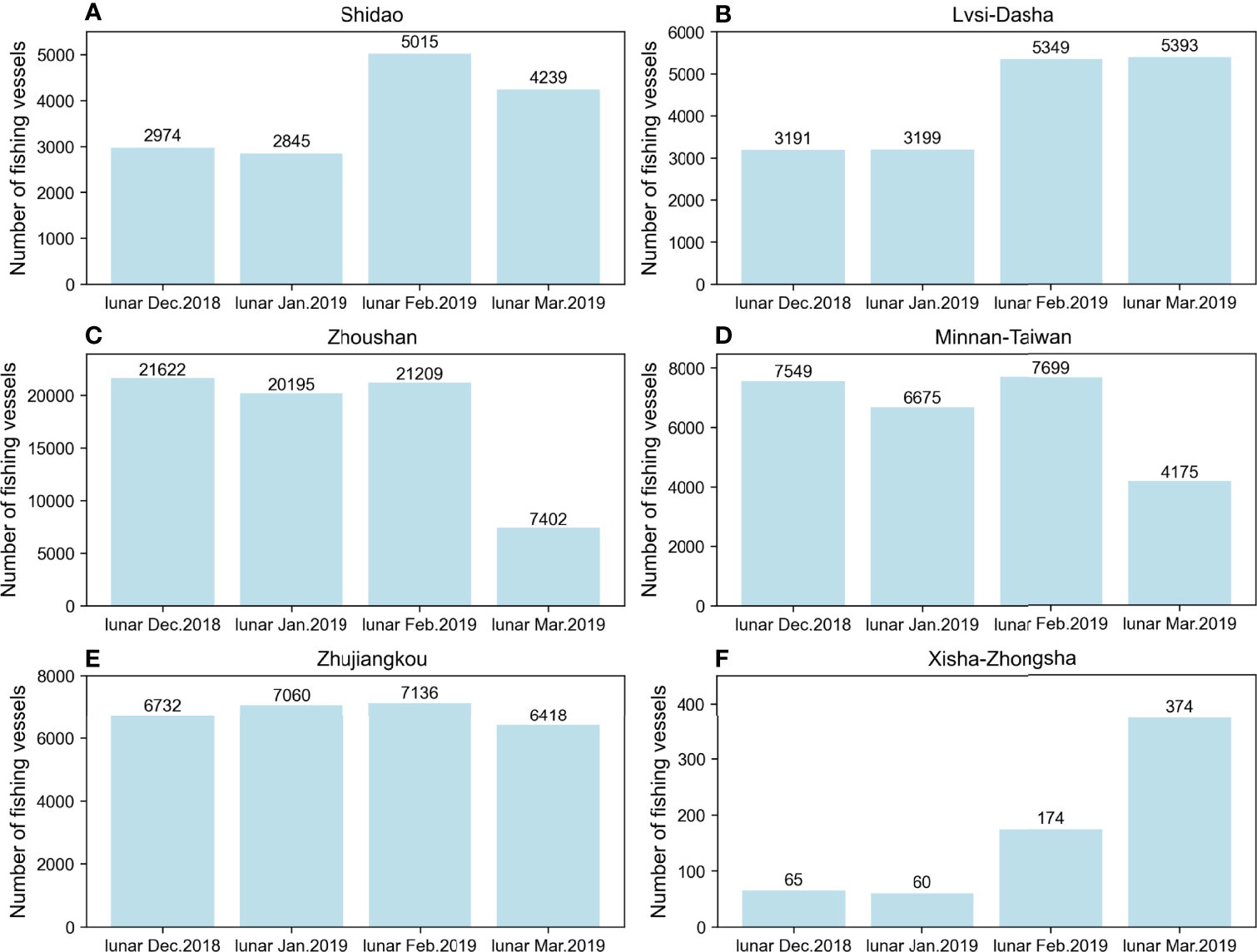
Figure 4 Number of fishing vessels in the six fishing grounds in 2019: (A) Shidao; (B) Lvsi-Dasha; (C) Zhoushan; (D) Minnan-Taiwan; (E) Zhujiangkou; (F) Xisha-Zhongsha.
Over time, the number of fishing vessels going to sea gradually increased as the temperature rose. This trend was more obvious in the northern fishing grounds with higher latitudes and greater temperature differences between winter and spring, such as Shidao and Lvsi-Dasha. In addition, from a regional perspective, the analysis of the changes in the number of fishing vessels in the inshore fishing grounds revealed that, for all fishing grounds except Zhoushan, the number of fishing vessels operating at sea in winter gradually increased as the latitude decreased.
Moreover, in lunar March (April 5 to May 4 on the solar calendar) of 2019, the numbers of fishing vessels in all five inshore fishing grounds obviously decreased to varying degrees compared to the corresponding numbers in the previous month; a very large drop was observed in the number of fishing vessels in Zhoushan, located in the East China Sea, with the number decreasing by as much as 61%. As shown in Supplementary Table 1, March of 2019 in lunar calendar corresponded to the period from April 5 to May 4 on the solar calendar. After analysis, the reason for above result may be that, according to regulations, from April 1 to September 16 on the solar calendar, the operation of fishing vessels whose main fishing objects are portunus or juvenile crabs is prohibited within the sea region from 27°N to 31°N; from April 1 to August 16 on the solar calendar, all types of fishing vessels except vessels with fishing tackles are prohibited from operating in 10 spawning ground protection areas, including Zhoushan. The sea area most affected by the regulations described above was thus Zhoushan, confirming that the implementation of seasonal fishing bans is a powerful measure for reducing fishing intensities and protecting fishery resources.
3.3.2 Fishing Duration
The fishing duration is positively correlated with the fishing effort, which is an important indicator used to measure the fishing intensity. Therefore, in the fishing time analysis module, we counted the cumulative and average fishing durations (Figure 5) of the fishing vessels in each fishing ground. Compared with other fishing grounds, the cumulative fishing duration of fishing vessels in Xisha-Zhongsha was too small to be clearly displayed in the same bar chart, so the cumulative fishing duration of fishing vessels in Xisha-Zhongsha is shown separately on the right side of Figure 5A.
Among the six major fishing grounds, Zhoushan had the longest cumulative fishing duration, followed by Zhujiangkou. These results further support the fact that Zhoushan is the largest fishing ground in China and contains abundant fishery resources. As mentioned earlier, the fishing in Shidao and Lvsi-Dasha was severely affected by bad weather factors such as ice on the sea surface and low temperatures. The cumulative fishing durations in these regions in winter were thus much lower than those in spring. However, the cumulative fishing durations of lower-latitude fishing grounds varied little in winter and spring, and the lowest values were recorded in lunar March (April 5 to May 4 on the solar calendar) due to the fishing ban policy. Overall, the change in the cumulative fishing duration of the inshore fishing grounds was similar to the change in the number of fishing vessels, but the cumulative operating time of vessels in Xisha-Zhongsha doubled in lunar March (April 5 to May 4 on the solar calendar).
The cumulative fishing duration reflects the overall fishing intensity of the fishing ground, while the average fishing duration needs to be analyzed to determine the fishing patterns of individual fishing vessels in the fishing grounds (Figure 5B). Overall, among the six fishing grounds, the average fishing duration of fishing vessels in Zhujiangkou was the highest, the average fishing durations in Shidao, Lvsi-Dasha and Zhoushan were approximately 40% of that in Zhujiangkou, and the average fishing durations in Minnan-Taiwan and Xisha-Zhongsha were the shortest. The variation in the monthly average fishing duration among the five inshore fishing grounds was relatively small, but Xisha-Zhongsha showed a clear decreasing trend over time.
After communicating with experienced fishers, we determined that the fishing time of a fishing vessel is directly proportional to the fishing capacity of the vessel, which depends mainly on the size of the vessel. According to the analysis of fishing vessels in Xisha-Zhongsha, in lunar December of 2018 (January 6 to February 4, 2019 on the solar calendar), the proportion of large fishing vessels with lengths greater than 30 m was as high as 88%. Over time, a large number of small fishing boats entered the fishing grounds, and in lunar March (April 5 to May 4 on the solar calendar) of 2019, the proportion of large fishing vessels was only 52%, leading to a significant reduction in the average fishing duration of fishing vessels.
3.3.3 Voyage Statistics
The operating voyage of a fishing vessel refers to the process of leaving a port, fishing in a fishing area, and returning to the port. However, while a voyage may involve fishing at sea over a duration generally longer than 0.5 days, it may also include the replenishment, maintenance or unloading of the fish at other ports, for which the voyage duration is generally within 0.5 days. In this paper, a voyage refers to voyages involving fishing at sea, and to reduce the impact of the nonfishing voyages, voyages with durations longer than 0.5 days were considered effective voyages. We counted the number of effective voyages for each fishing ground in each month, as shown in Figure 6.
Overall, the total number of voyages in Shidao and Lvsi-Dasha, which have relatively high latitudinal positions, increased with time. However, the lower-latitude fishing grounds, such as Zhoushan, Minnan-Taiwan and Zhujiangkou, did not show significant changes. Large temperature differences exist between winter and spring in the northern fishing grounds, and bad winter weather, such as cold spells and snowstorms, can prevent fishers from going to sea. Relatively speaking, the southern fishing grounds are warm year-round, and the winter and spring fishing activities in these regions are thus less affected by climatic factors.
Based on the voyage division results, we calculated the duration distributions of individual voyages in days, as shown in Figure 7. Figure 7 shows that overall, the voyage frequency decreased as the duration increased, and the single-voyage durations in the six fishing grounds were mainly concentrated within 8 days, accounting for approximately 92.93% of all voyages.
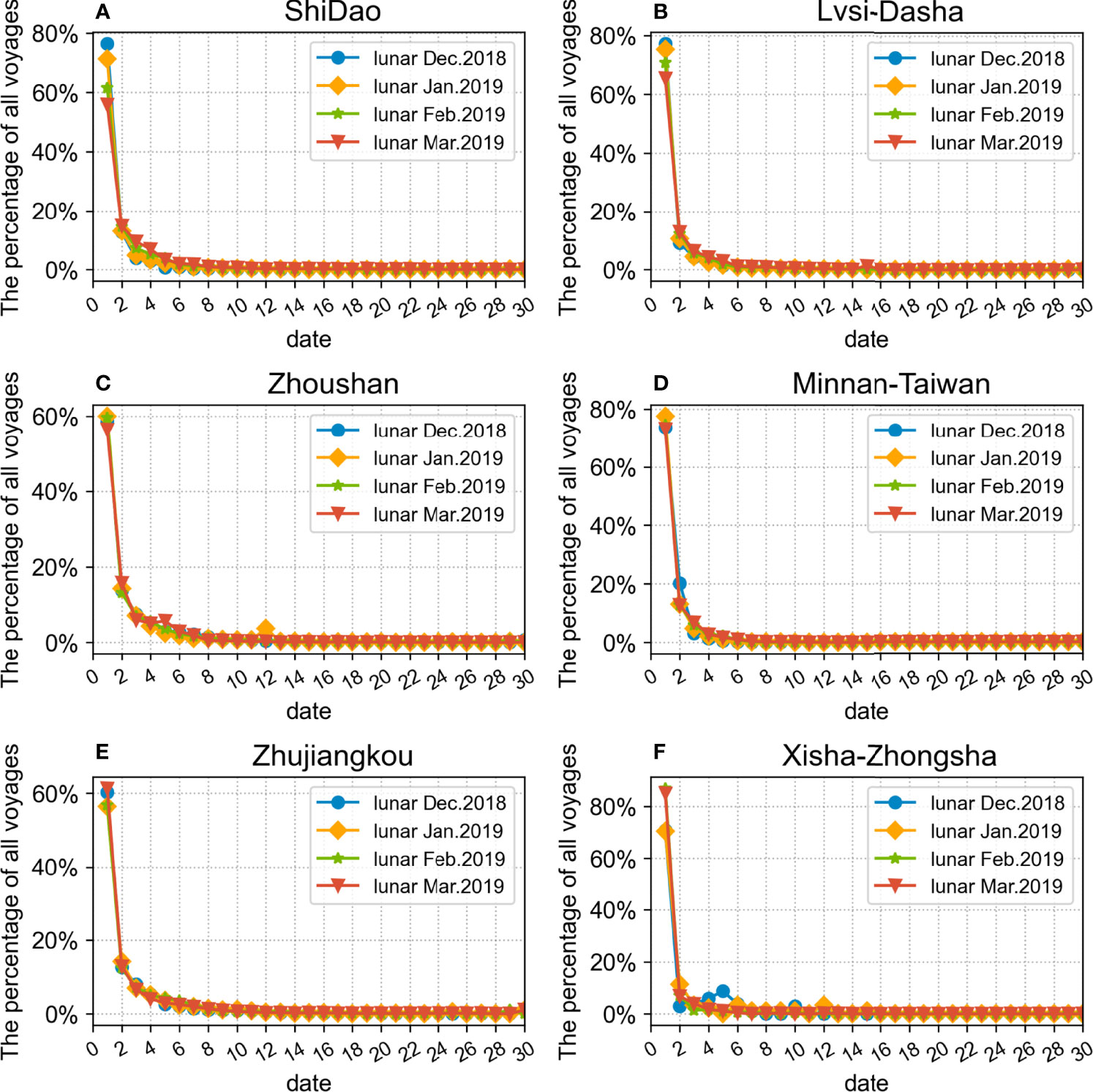
Figure 7 Statistics of the voyage duration of the six fishing grounds in 2019: (A) Shidao; (B) Lvsi-Dasha; (C) Zhoushan; (D) Minnan-Taiwan; (E) Zhujiangkou; (F) Xisha-Zhongsha.
Among the six fishing grounds, in the northern fishing grounds, such as Shidao and Lvsi-Dasha, the duration of a single voyage in spring was significantly greater than that in winter. Combined with the analysis results shown in Figure 6, we found that in springtime in the northern fishing grounds, as the number of fishing voyages increases, the duration of individual voyages also increases. This further supports the existence of a large difference in the fishery resources of the northern fishing grounds between winter and spring. For the southern fishing grounds, the duration distributions of individual voyages in winter and spring were basically the same, with no major changes. Furthermore, compared to the other fishing grounds, the single-voyage durations in Zhoushan and Zhujiangkou were larger.
However, for Xisha-Zhongsha, the duration of a single voyage gradually decreased with time; this was very different from the trends seen in inshore fishing grounds. As analyzed above, Xisha-Zhongsha is located far from the shore and has relatively high requirements concerning the maneuverability and replenishment capacity of fishing vessels compared to the inshore fishing grounds. However, as previously discovered, with the exploitation of fishery resources in Xisha-Zhongsha, an increasing number of small and medium-sized fishing vessels entered this fishing ground to fish. These fishing vessels may minimize the limitations of factors such as the resupply capacity by decreasing the single-voyage duration.
3.3.4 Fishing Vessel Type
Based on the fishing vessel type identification results, the quantitative compositions of trawlers, gillnetters, seiners and others in the six fishing grounds were obtained. We chose lunar December of 2018 (January 6 to February 4, 2019 on the solar calendar) as the representative winter month and lunar March of 2019 (April 5 to May 4 on the solar calendar) as the representative spring month. The fishing vessel type identification results obtained for these two months were used to examine the changes in fishing vessel types between winter and spring in the different fishing grounds, as shown in Figure 8.
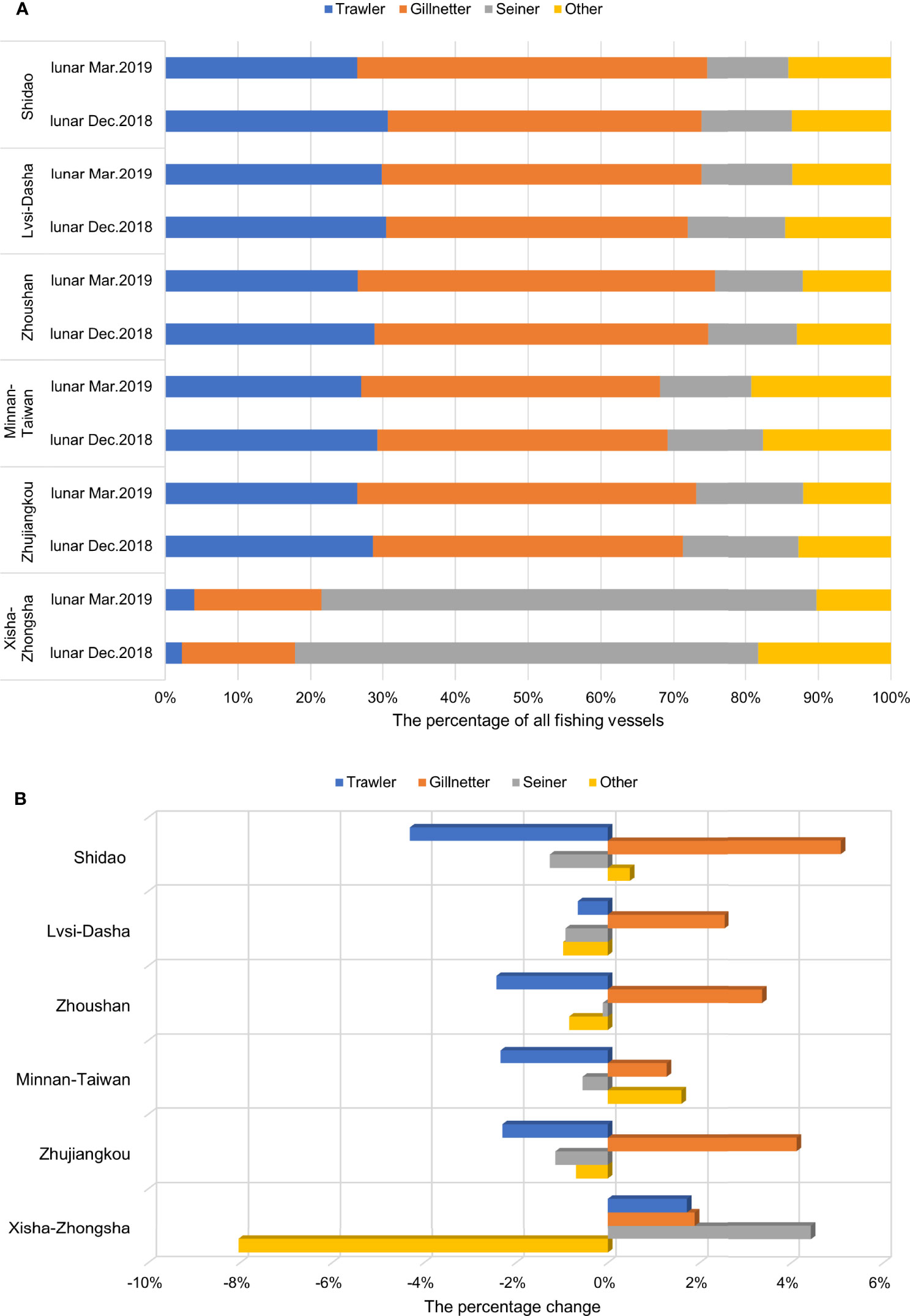
Figure 8 (A) Identification results of fishing vessel types in lunar December of 2018 (winter) and lunar March of 2019 (spring), and (B) the percentage change of the four fishing vessel types in lunar March of 2019 relative to lunar December of 2018.
In inshore fishing grounds such as Shidao, the number of gillnetters was largest, followed by the numbers of trawlers and seiners (Figure 8A). Fishing in Xisha-Zhongsha was mainly carried out by seiners, with a relatively small proportion of trawlers. These differences in the fishing vessel type compositions were evaluated by referencing relevant material. First, these differences resulted from the rugged seabed and reefs in Xisha-Zhongsha, which are not suitable for trawling. In addition, in Xisha-Zhongsha, fishes and squid with strong phototropism are dominant, and the most popular fishing method for these organisms is light-purse seining.
As shown in Figure 8B, compared with lunar December of 2018 (January 6 to February 4, 2019 on the solar calendar), the proportion of gillnetters generally increased in spring, especially in Shidao and Zhoushan, with the largest increases of 5.08% and 3.37%, respectively. We then tried to explain this change by examining the fishing methods of fishing vessels. Spring is the spawning season for fish. Shidao fishing ground was taken as an example. The Bohai Sea is China’s inland sea, with nearby geographical locations, low salinity and rich bait, and is the spawning ground of most fishery resources in the Yellow Sea. Shidao is the necessary route by which fish to swim from the Yellow Sea to the Bohai Sea. Therefore, gillnetters that lay nets in migratory waters and wait for fish to be trapped in the mesh or entangled in the netting have a greater advantage in catching migratory spawning fish.
4 Impact of COVID-19 on Winter-Spring Fishing in China in 2020
The comparative method helps to analyze the impact of the COVID-19 pandemic on fishing activities in China. By comparing the global fishing effort between 2020 and the previous three years (2017-2019), He et al. (2021) found that due to the impact of COVID-19, the overall fishing effort declined throughout 2020, with trawlers experiencing the worst decline, followed by gillnetters and longliners.
Based on the times at which the Chinese government took measures to fight the development of the COVID-19 epidemic, the critical time points related to epidemic prevention and control can be determined, as shown in Supplementary Figure 3. The four lunar months of 2020 correspond to the occurrence period, outbreak period, platform period, and decay period of the epidemic. For ease of analysis and understanding, in the following text, we directly use the names of these epidemic development periods to refer to the corresponding months and thus analyze the impact of the epidemic on fisheries during the different development periods.
The effectiveness of epidemic prevention and control depends mainly on two aspects: governmental policies and self-prevention by the population. Both of these aspects are closely related to COVID-19 developments. When the epidemic is severe, the government releases stricter epidemic prevention policies, and the public consciously adopts stricter epidemic prevention measures. We gathered publicly accessible COVID-19 data, including (1) the data of newly confirmed cases (NCC) was supplied by the National Science Foundation Spatiotemporal Innovation Center (Liu et al., 2020); and (2) the Government Response Stringency Index (GRSI) used in the Oxford COVID-19 Government Response Tracker (Hale et al., 2020). The index quantifies the response policy into numbers, which are then averaged. We used the GRSI to represent the stringency of government policy against the epidemic. For the public, NCC is the most intuitive measure of the current epidemic situation.
Therefore, based on the fishery laws in place in 2019, this section explores the changes in fishery production in 2020 from various aspects, such as the number of fishing vessels and the distribution range of fishing activities. In addition, combined with public COVID-19 data, we discuss how COVID-19 affected fishing activities in China.
4.1 Changes in the Number of Fishing Vessels in China’s Sea Areas
As shown in Supplementary Figure 3, in the early stages of the occurrence period of COVID-19 (December 26, 2019 to January 22, 2020 on the solar calendar), confirmed cases of the virus had been reported. However, because research on COVID-19 was at an early stage, there was an inadequate understanding of its harm, it did not attract considerable attention, and no nationwide prevention and control measures were taken. Therefore, during this period, COVID-19 did not immediately affect fishing activities in the China’s sea areas, and this period comprised the vast majority of the occurrence period (December 26, 2019 to January 24, 2020 on the solar calendar). As a result, China’s marine fisheries were barely affected during this period of the epidemic, and the number of fishing vessels even increased by 1.62% compared to that in the same time period in 2019 (Figure 9A). However, with the further spread and deterioration of the COVID-19, the epidemic entered the outbreak period. To prevent the spread of the novel coronavirus, provinces in mainland China gradually and successively implemented strict policies to restrict nonessential activities, transportation and production, thus inducing major effects on fishing activities. During the outbreak period, the number of active fishing vessels in China’s sea areas dropped by approximately 43.26%, to a value 43.08% lower than that recorded in lunar January (February 5 to March 6 on the solar calendar) of 2019.
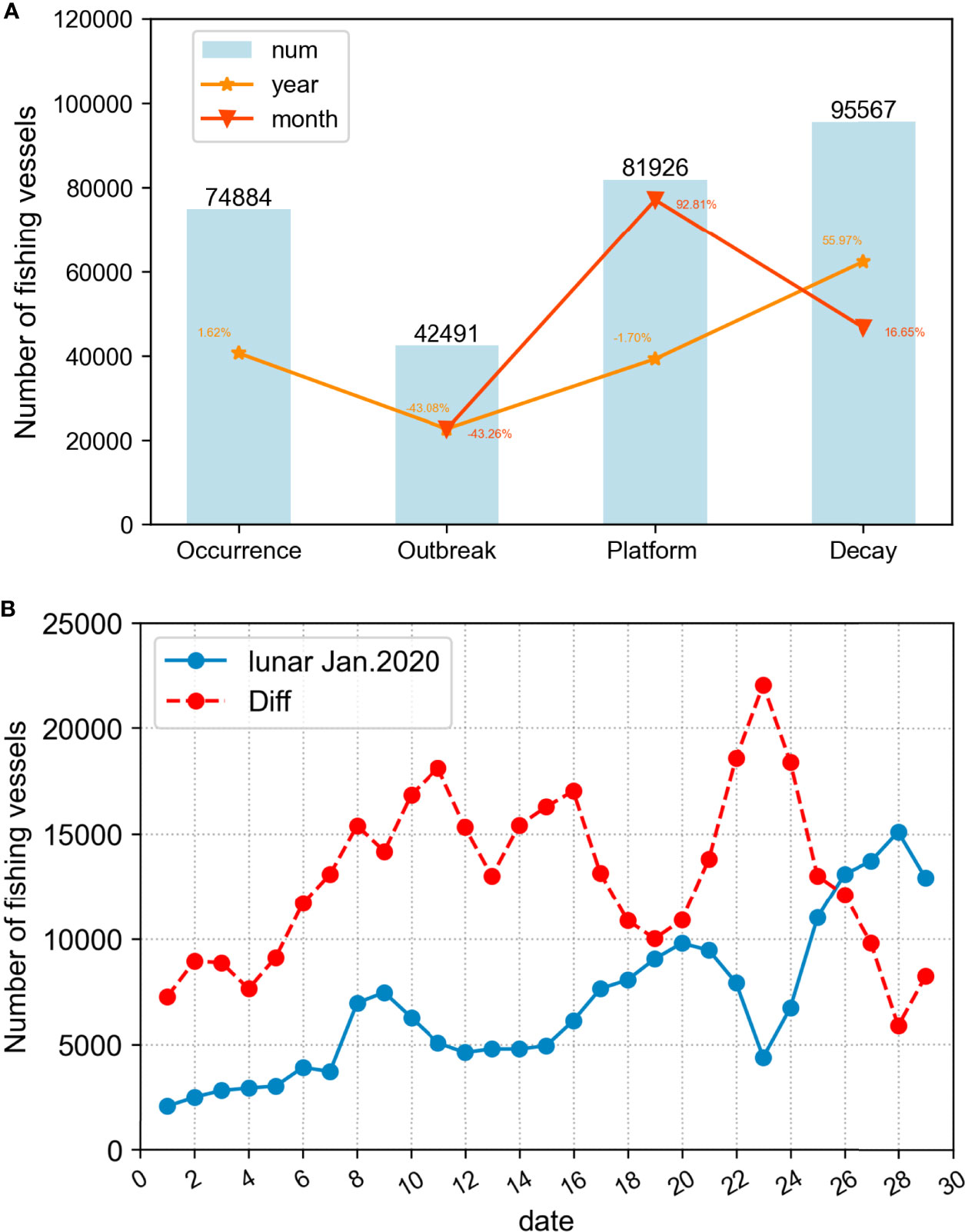
Figure 9 (A) Number of fishing vessels in China’s sea areas (num) at different epidemic development periods, where yellow represents the year-on-year increase compared to the same time period in 2019, and red represents the month-on-month increase compared to the previous epidemic period. (B) Daily number of fishing vessels in China’s sea areas in lunar January of 2020 (blue solid line) and its daily difference from lunar January of 2019(red dashed line).
Since the outbreak period included the Chinese New Year holiday, the number of fishing vessels recorded during this period were affected by both the Chinese New Year holiday and the epidemic. Therefore, to explore the impact of the epidemic alone on fishery production, we counted the daily number of fishing vessels operating in lunar January of 2020 (December 26, 2019 to January 24, 2020 on the solar calendar); in Figure 9B, the red dotted line indicates the daily differences beginning in lunar January (February 5 to March 6 on the solar calendar) of 2019. As expected, the figure shows that the outbreak of the epidemic caused the number of fishing vessels active during the Chinese New Year holiday to be low. However, similar to the previous year, at the end of the holiday, the number of fishing vessels going out to sea on the 8th day increased significantly by approximately 46.63%. However, only 2 days later, on the 10th, the number of fishing vessels began to decrease, and it was not until the Lantern Festival (on the 15th) that the number of fishing vessels again started to increase. It can be seen that fishing vessels operating at sea were indeed affected by the Chinese New Year, but these vessels were more constrained by the measures taken to prevent and control the COVID-19 epidemic.
With the isolation and quarantine measures achieving obvious effects, the growth trend of the NCC on the Chinese mainland slowed considerably, and COVID-19 entered the platform period. During this period, Chinese enterprises gradually returned to work, and the number of fishing vessels going to sea increased by 92.81% from the previous period, almost returning to the level recorded in the same period in 2019. As the pandemic situation further improved and COVID-19 entered the decay period, the number of fishing vessels operating at sea further increased. After analysis, compared with large vessels such as bulk carriers and oil tankers, inshore fishing vessels have low epidemic transmission and infection risks due to the weak mobility of their personnel and their lower chance of contact with strangers. Therefore, under preventive measures, the number of fishing vessels going to sea gradually increased, even in lunar March (March 24 to April 22 on the solar calendar), when there was a significant increase of 56% compared to lunar March (April 5 to May 5 on the solar calendar) of 2019.
4.2 Vessels Activity Distributions
As shown by comparing Figures 10 with 3, the distribution density of fishing vessel trajectory points in the China’s sea areas in lunar December of 2019 (December 26, 2019 to January 22, 2020 on the solar calendar) was not significantly different from that in 2018. However, this distribution density changed significantly in lunar January (January 25 to February 22 on the solar calendar) of 2020, when the large-scale coronavirus outbreak took place in China. The hotspot distribution range of fishing vessel activity track points was significantly reduced, and the maximum point density was reduced by approximately 55%. However, when the epidemic platformed, the scope of fishing vessel activities increased, and the fishing intensity rose significantly. This phenomenon also corresponds to the previous analysis results derived for the number of fishing vessels. Fishing activities resumed to some extent after the epidemic was effectively controlled.
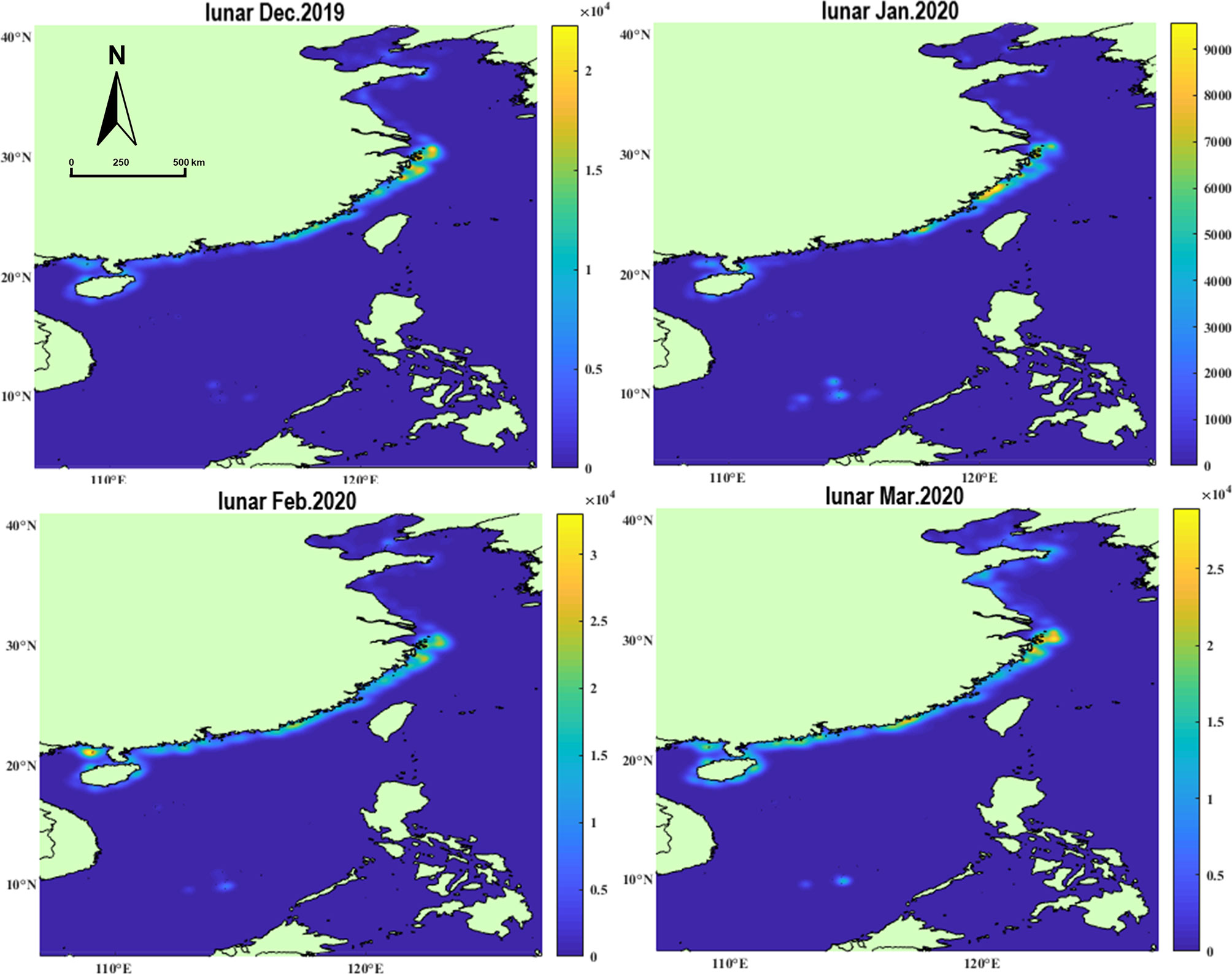
Figure 10 Distribution density map of fishing vessels trajectory points in China’s sea areas in the winter and spring of 2020(points per km2).
In addition, a newly developed “hotspot” with a relatively high density of fishing vessel trajectory points was observed in the Nansha Islands area in the South China Sea, and the density at this location increased over time. The statistical analysis of the AIS data recorded by fishing vessels in the area showed that in 2019, only a small number of large fishing vessels from China were engaged in fishing in this area, while in 2020, there was a substantial increase in the number of vessels coming to fish in the area, including small fishing vessels less than 12 m in length. At the same time, by inquiring about the registration information of fishing vessels, we learned that the fishing vessels operating in the Nansha waters mainly come from China and Vietnam, with the Chinese fishing vessels coming mainly from Guangdong Province, Guangxi Zhuang Autonomous Region and Hainan Province.
A careful analysis of the information reflected in Figure 10 revealed that the fishing operations within the Bohai and Yellow Seas were more affected by the epidemic than those in the southern waters. This was supported by the significant decrease in the scope and intensity of fishing vessel activities compared to the previous year; in January 2020, only sporadic hotspots could be seen.
4.3 Six Fishing Grounds
4.3.1 Changes in the Number of Fishing Vessels in Fishing Grounds
As shown in Figure 11, since the coastal areas were not yet significantly affected by the epidemic during the occurrence period, the number of fishing vessels recorded in inshore fishing grounds such as Shidao did not significantly change, while the number of fishing vessels in Xisha-Zhongsha increased by 196.92% over the same period in 2019 due to the increasing development intensity. During the outbreak period, 31 provinces in China launched first-level responses to the COVID‐19 emergency. The numbers of fishing vessels operating in the five inshore fishing grounds were greatly reduced, with the greatest decrease (78.38%) measured in Shidao. As the epidemic improved, the number of fishing vessels operating at sea gradually increased, while the number of fishing vessels even exceeded that measured in the corresponding period of 2019 during the decay period when the epidemic was effectively controlled.
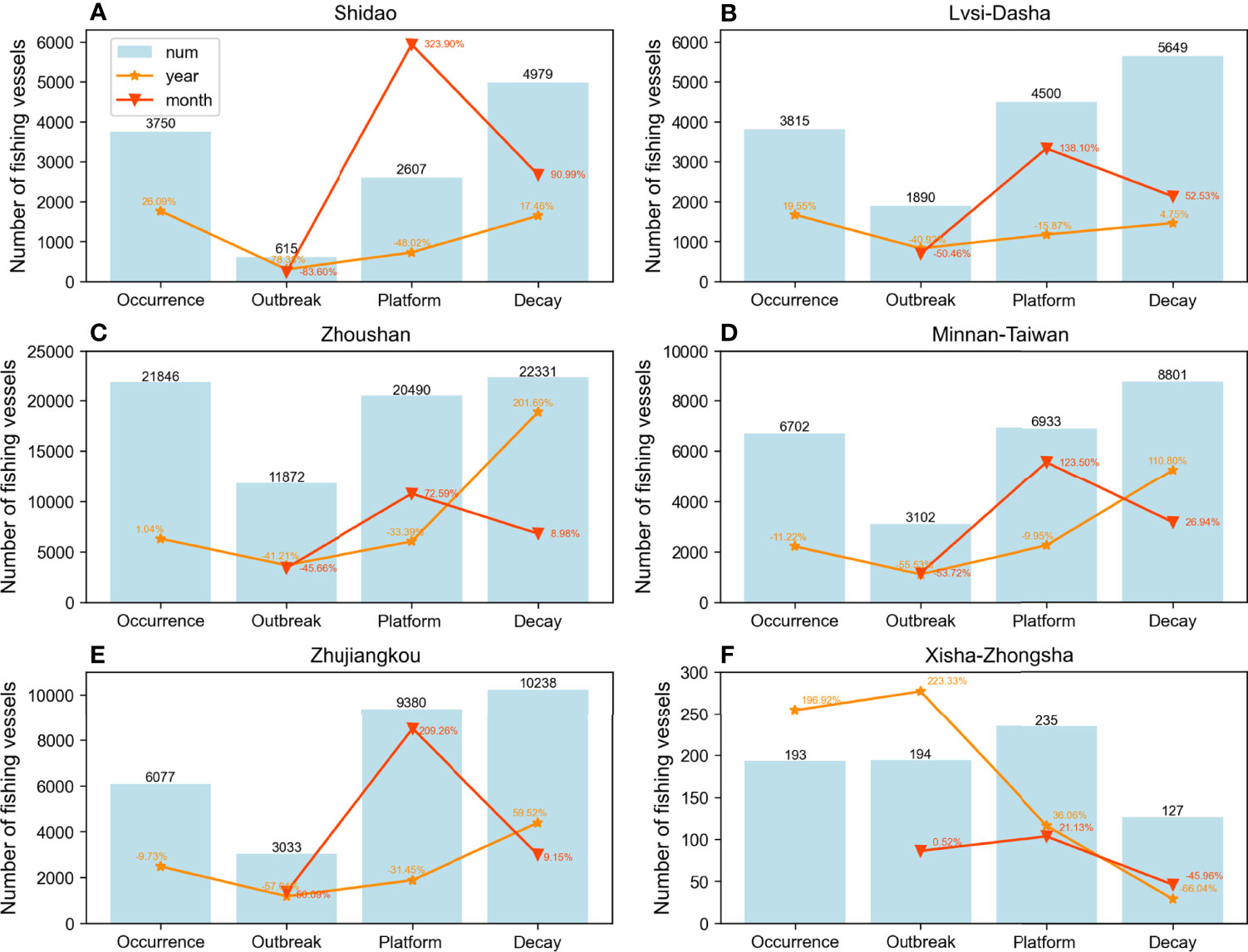
Figure 11 Number of fishing vessels within the different epidemic development periods for the six fishing grounds, where yellow represents the year-on-year increase compared to the same time period in 2019, and red represents the month-on-month increase compared to the previous epidemic period: (A) Shidao; (B) Lvsi-Dasha; (C) Zhoushan; (D) Minnan-Taiwan; (E) Zhujiangkou; (F) Xisha-Zhongsha.
However, it is worth mentioning that during the COVID-19 outbreak period, the number of fishing vessels in Xisha-Zhongsha increased rather than decreased. This indicates that the change trend of the number of fishing vessels in this region was opposite to that observed in inshore fishing grounds.
4.3.2 Fishing Duration
As previously described, to study the impact of COVID-19 on the fishing intensities within the six fishing grounds, the cumulative and average fishing durations (Figure 12) of fishing vessels in each fishing ground during the different epidemic development phases in 2020 were calculated. As shown in Figure 12A, the relationship between the magnitudes of the cumulative fishing durations recorded in the six fishing grounds in 2020 was consistent with that in 2019, with the longest cumulative fishing duration recorded for fishing vessels within Zhoushan. Moreover, compared to 2019, the cumulative fishing duration of the fishing grounds declined significantly during the outbreak period, and this decline even reached 90% in Zhujiangkou.
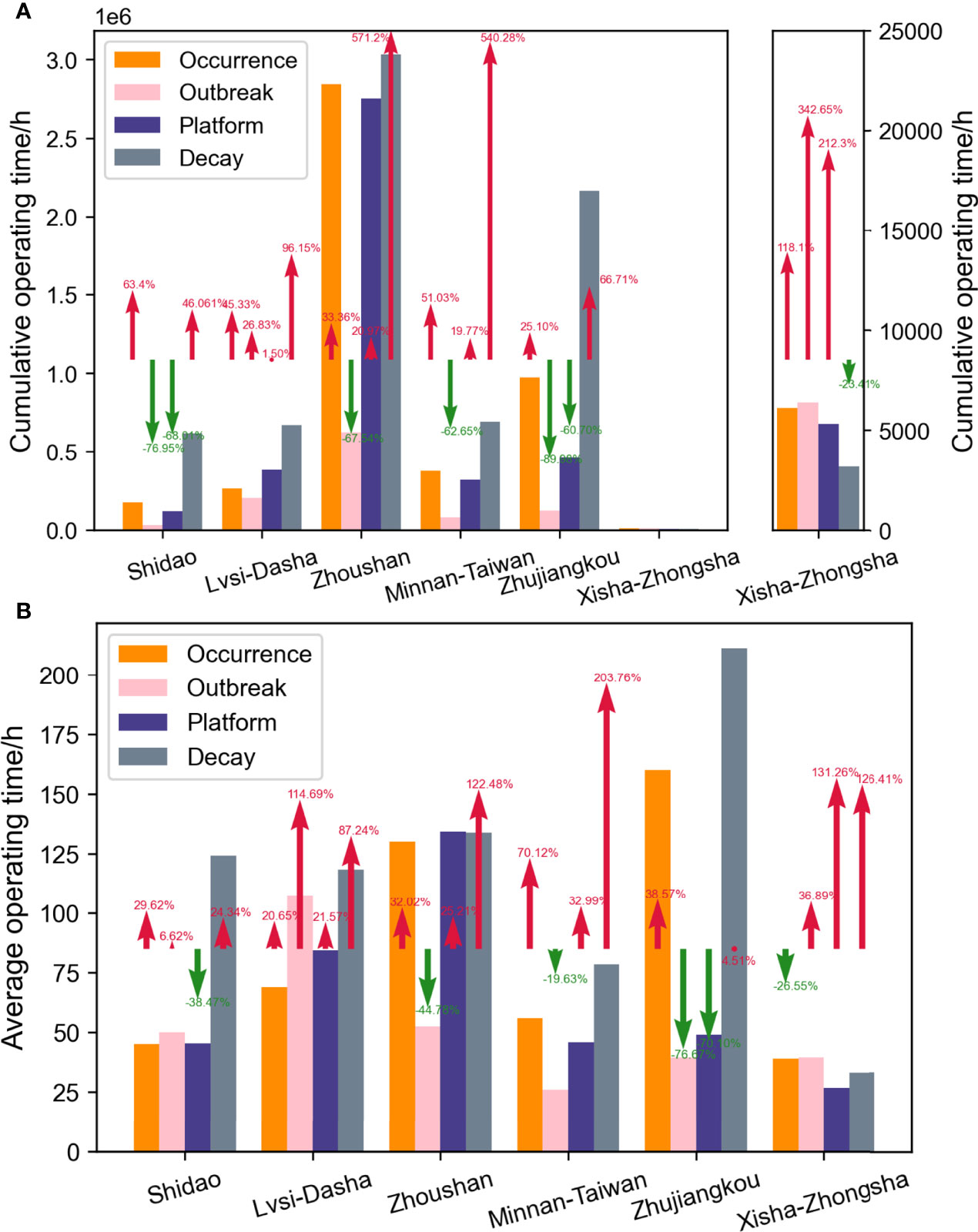
Figure 12 (A) Cumulative and (B) average fishing duration of six fishing grounds in 2020, and the arrows represent the variation relative to the same period in 2019, where the red arrows represent the increase and the green arrows represent the decrease.
Even more conspicuous, during the decay period of the epidemic, the cumulative fishing durations of fishing vessels in the six fishing grounds increased significantly. After analysis, three main reasons were found for this phenomenon. ① During the outbreak period, the number of fishing vessels going out to sea was drastically reduced, leading to a decrease in catches and causing vast economic losses to fishers. During the decay period, as the epidemic was effectively controlled in China, accelerating the “resumption of work and production” became the focus of the next step for various industries; the recovery of the fishing industry, an important part of the fishery supply chain, was particularly important. ② As shown in Supplementary Table 1, the decay period of the epidemic (lunar March of 2020) corresponded to the period from March 24 to April 22 on the solar calendar. Under Chinese law, from May 1 to August 16 (April 9 to June 27 on the lunar calendar), all types of fishing vessels except vessels with fishing tackles, as well as auxiliary vessel supporting services for fishing vessels, are required to anchor in the harbor. Therefore, except for certain sea areas in Zhoushan, the other fishing grounds were in the period during which fishing is still allowed but were about to enter the prohibited fishing season. ③ The rising water temperatures caused fish growth to flourish, and fisheries resources are thus rich at this time.
Owing to the three reasons above, we believe that during the decay period, driven by multiple factors, such as compensating for previous economic losses and coping with the upcoming fishing ban and superior natural conditions, the number of fishing vessels in various fishing grounds increased along with their fishing durations. From Figure 12B, it is obvious that the average fishing duration of fishing vessels during this period was generally higher than those in other periods and was also higher than that of the same period in 2019.
In addition, for Xisha-Zhongsha, the cumulative and average fishing durations in 2020 were generally higher than those in 2019. Combined with the fact that the number of fishing vessels in this fishing ground increased during the epidemic in 2020, as shown in Figure 11, fishery production of Xisha-Zhongsha was found to not be negatively affected by the epidemic but rather showed an increase.
4.3.3 How COVID-19 Affected China’s Fishing Activities
As mentioned above, the COVID-19 outbreak had significant repercussions on fishing in China’s sea areas. After analyzing and quantifying how large this impact was, this section was focused on how the COVID-19 affected Chinese fishing in terms of both government intervention and self-prevention by the population using collected public COVID-19 data.
The Chinese NCC in the public COVID-19 data was calculated at the provincial level, but fishing vessels from multiple provinces may have been present in each fishing ground. Therefore, we first counted the provinces to which the fishing vessels belonged and decided to use the NCC of the provinces with the most fishing vessels in each fishing ground to analyze that fishing ground. Supplementary Table 5 shows the top 3 provinces with the largest numbers of fishing vessels in each fishing ground and their proportions (number of fishing vessels from the indicated province/all fishing vessels).
We used Pearson correlation coefficients (Frey, 2018) to evaluate the correlations between the daily number of fishing vessels in each fishing ground and the known public COVID-19 data, including (1) the NCC of the province with the highest number of fishing vessels in each fishing ground and (2) the GRSI. The trend plots are shown in Figures 13, 14, the Pearson correlation coefficient and statistical significant are shown as Table 1.
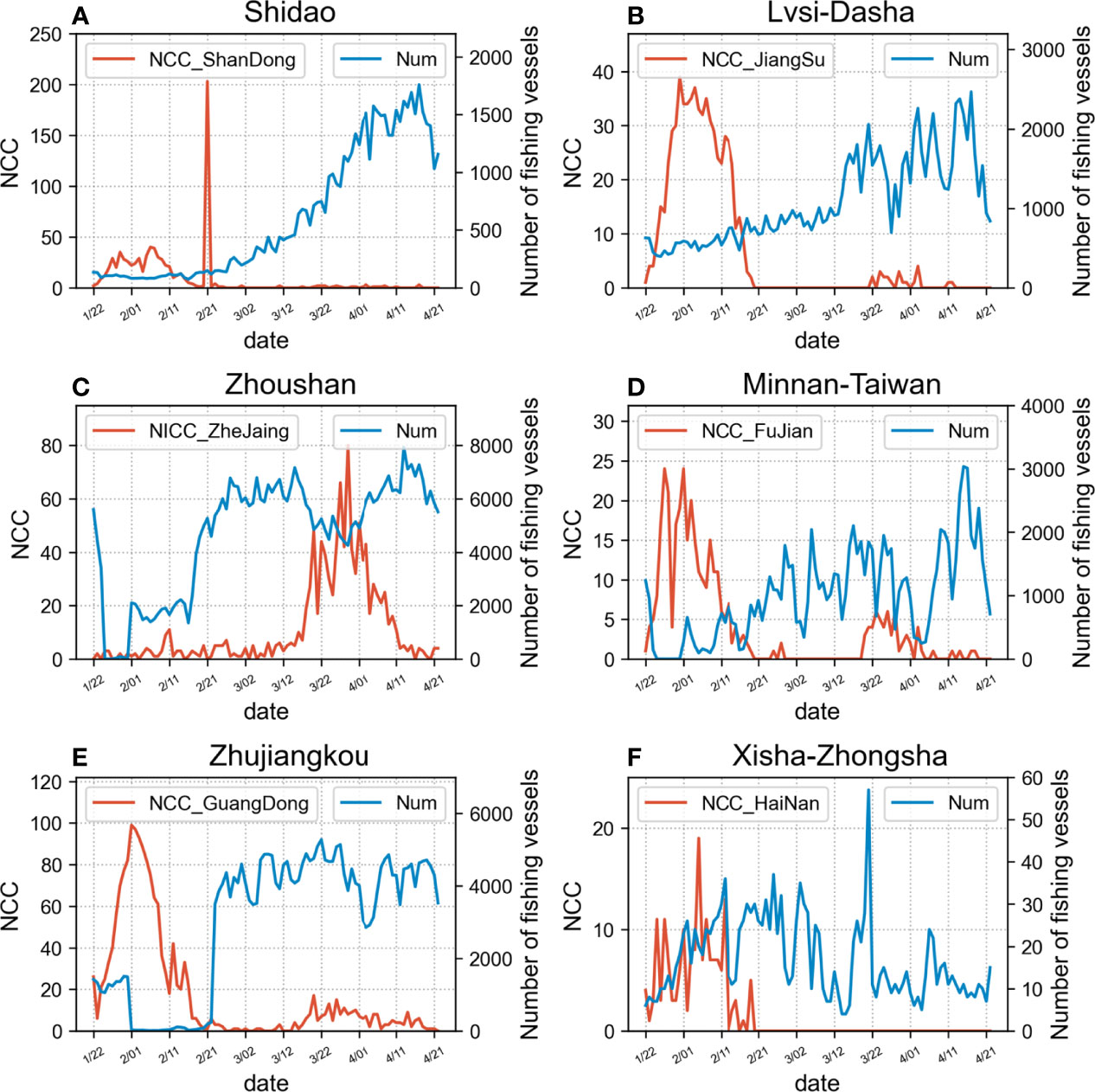
Figure 13 Graphical representation of the temporal trend of the number of fishing vessels per day (Num) and newly confirmed cases (NCC) for each fishing ground: (A) Shidao; (B) Lvsi-Dasha; (C) Zhoushan; (D) Minnan-Taiwan; (E) Zhujiangkou; (F) Xisha-Zhongsha.
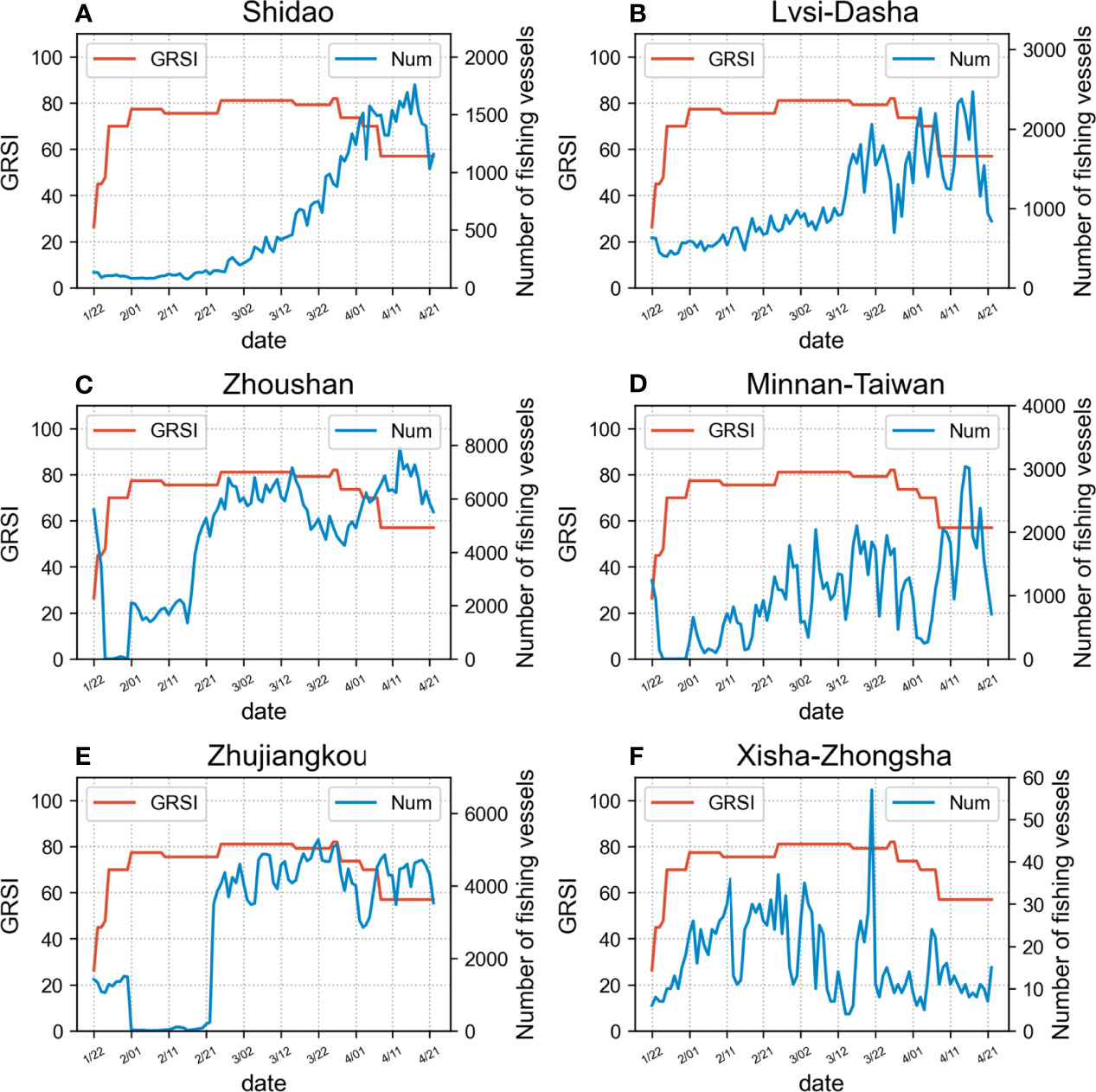
Figure 14 Graphical representation of the temporal trend of the number of fishing vessels per day (Num) and government’s intervention (Government Response Stringency Index, GRSI) for each fishing ground: (A) Shidao; (B) Lvsi-Dasha; (C) Zhoushan; (D) Minnan-Taiwan; (E) Zhujiangkou; (F) Xisha-Zhongsha.
With an analysis of Figure 13 and Table 1, we can observe that, in general, the number of fishing vessels operating at sea is negatively correlated with the NCC, and the trend was well reflected in Lvsi-Dasha, Minnan-Taiwan and Zhujiangkou, with the Pearson correlation coefficients of -0.5329, -0.5429 and -0.6504, with moderate correlation with Shidao (-0.3039) and low correlation with Xisha-Zhongsha (0.0075). The correlation between the number of fishing vessels in Zhoushan and NCC was less significant when analyzed using the overall data. However, we noted that in March of the solar calendar, the number of fishing vessels at sea fluctuated downward when the NCC in Zhejiang Province increased, showing a negative correlation (Figure 13C).
As shown in Figure 14 and Table 1, although the correlation with the GRSI was negative, it was not as strong as the correlation with NCC. This was an interesting phenomenon. Since the GRSI is directly related to governmental policies to implement social distancing, it substantially impacts industrial activities on land. Unlike other industries, most practitioners in China’s inshore fisheries are self-employed, with a low degree of organization, and their operations are located on the ocean. Therefore, they are less affected by the traffic control measures implemented on land. Determining whether or when to go to sea is more dependent on personal wishes, allowing a greater degree of freedom. Therefore, compared to the GRSI, the soft social constraints caused by the fear of COVID-19 due to high NCCs have a greater impact on the willingness of fishers to go to sea.
For Xisha-Zhongsha, we found that the daily number of fishing vessels had no obvious correlation with either GRSI or NCC. The data may lack representativeness due to the small number of fishing vessels located in this fishing ground; additionally, after the data analysis, we determined that there were also Vietnamese fishing vessels in this fishing ground, accounting for approximately 34.64% of all fishing vessels. Therefore, using only the GRSI of the Chinese government and the NCC data of Hainan Province in the correlation analysis resulted in large deviations.
5 Conclusion
To the best of our knowledge, this is the first study to comprehensively analyze the characteristics of wintertime and springtime fishing activities in China’s sea areas and the specific impacts of COVID-19 on fisheries. As winter and spring are important seasons for marine fishing, exploring these fishing patterns can help fishery managers grasp the dynamic characteristics of fishery resource exploitation to formulate effective policies aiming to strengthen fishery management. In addition, the unprecedented global devastation caused by COVID-19 has prompted many countries around the world, including China, to take prompt measures to contain the spread of infection and save human lives. Obviously, Chinese fisheries have suffered losses due to COVID-19. However, thus far, there has been a lack of quantitative spatiotemporal dynamic analyses regarding the impacts of the epidemic on fisheries; the mechanisms by which the epidemic affects fishing and causes fishery losses thus need to be explored.
Fishing activity analyses based on AIS data have many advantages, such as high timeliness, wide spatial ranges, and low costs. The highly accurate spatial location information of fishing operations reflected in AIS data provides an opportunity to explore the development of fishery resources. Using these data, the operational characteristics of fishing vessels can be analyzed and the distribution of marine fishery resources, fishing intensities and other spatiotemporal characteristics can be obtained. While taking the fishing vessels in China’s sea areas as the research object, this paper also selected fishing vessels in six fishing grounds distributed from north to south as the research focus. Through a statistical analysis of the AIS data recorded by fishing vessels, we comprehensively explored the changing characteristics of fishing activities in winter and spring in China’s sea areas and the impacts of the epidemic on these activities.
The AIS dataset contained the trajectory data of approximately 588,000 fishing vessels, with a total of approximately 1.68 billion AIS trajectory data points. For such a large AIS dataset, this paper proposed a processing and analysis framework in which several data processing modules and analysis modules were integrated. This framework can efficiently realize the data processing of AIS information recorded by fishing vessels, such as voyage division, fishing status recognition and fishing vessel type identification, and supports the instant monitoring and analysis of fishing vessel activity information, such as activity ranges and fishing durations. After applying the framework to the AIS dataset representing fishing vessels in China’s sea areas, we analyzed and compared the activities of fishing vessels in China’s sea areas overall and in six fishing grounds in the winter and spring of 2019, superimposed the impact analysis of the COVID-19 pandemic in 2020, and reached the following conclusions.
1. Regularity of fishing vessel activities in the winter and spring of 2019
For the fishing vessels in China’s sea areas, the analysis of the changes in the number of fishing vessels showed a gradually increasing trend over time. The Chinese New Year holiday affected the activities of fishing vessels at the corresponding time but had little impact on the entire month. Overall, the intensity of fishing vessel activities was high offshore and low in the high seas, with relatively obvious regional variances. Moreover, regarding the overall fishing situation, the intensity of fishing vessel activities in the inshore waters of the East China Sea was relatively high, but this intensity dropped significantly in lunar March due to the impact of the fishing ban policy. In addition, the fishing vessel activity densities in the Bohai Sea were quite different between winter and spring. In winter, due to severe weather, such as sea ice, some fishing vessels could not go out to fish.
Regarding the fishing vessels in the six fishing grounds, the number of fishing vessels in Zhoushan, which contains the richest fishery resources, was the highest, and the number in Xisha-Zhongsha, which is the farthest offshore, was low. The overall trends derived for the changes in the number of fishing vessels in the five inshore fishing grounds in winter and spring were consistent with those observed in the China’s sea areas overall, while the number of fishing vessels in Xisha-Zhongsha increased significantly in the spring.
From a regional perspective, as the latitude decreased, the number of fishing vessels in the fishing grounds gradually increased in winter. From the time scale, the number of fishing vessels operating at sea gradually increased as the temperature rose, and this trend was more obvious in northern fishing grounds with higher latitudes and greater temperature differences between winter and spring, such as Shidao and Lvsi-Dasha. Among the fishing grounds, the sharp decline in the number of fishing vessels in Zhoushan in lunar March (April 5 to May 4 on the solar calendar) indicated that the fishing ban effectively reduced the intensity of fishery resource development in this area. In addition, when exploring the variation law of the fishing intensity, we found that the change law of the cumulative fishing duration in the inshore fishing ground was similar to that of the number of fishing vessels. In lunar March (April 5 to May 4 on the solar calendar), the number of fishing vessels in Xisha-Zhongsha more than doubled, but the average fishing duration of a single vessel decreased significantly. Upon data analysis, we determined that this is because a large number of the middle and small fishing vessels with smaller capacities also entered the fishing grounds to fish. At the same time, the frequency of voyages decreased with the voyage duration, and the single-voyage durations in the six fishing grounds were mainly concentrated within 8 days, accounting for approximately 92.93% of all voyages.
Regarding the fishing vessel types, gillnetters were the most numerous fishing vessel type operating in the inshore fishing grounds, and their proportions increased in spring due to the superiority of this fishing method. However, due to factors such as topography and the economy, seiners were dominant in Xisha-Zhongsha.
2. Impact of COVID-19 on winter-spring fishing in China in 2020
When the epidemic was in the occurrence period, the fishing activities in China’s sea areas was almost unaffected. However, during the outbreak period, the number of fishing vessels in the China’s sea areas decreased by approximately 43.08% compared to the corresponding number in 2019, revealing a significant reduction in the distribution range and a decrease of approximately 60% in the maximum fishing vessel activity intensity. Both the number of fishing vessels and the fishing duration showed relatively large declines in the inshore fishing grounds. After the epidemic was effectively controlled, the number of fishing vessels and the fishing intensity both increased rapidly; during the decay period, when the number of domestically transmitted cases gradually decreased, these conditions even exceeded the levels recorded during the same period in 2019.
In addition, after analyzing how COVID-19 affected fishery activities, we found that compared with the GRSI, the number of fishing vessels going to sea showed a more obvious negative correlation with the NCC. The soft social constraints caused by high NCC more critically impacted fishers’ intentions to go fishing at sea.
Our analysis results provide an accurate perspective by which the characteristics of fishing vessel activities can be analyzed and accurate information on fisheries resource exploitation can be obtained; this framework can guide marine spatial planning and sustainable fishery management. In addition, this study confirms that AIS data obtained from fishing vessels are a powerful tool to investigate the impacts of COVID-19 on fishing activities.
In summary, future work will also address the shortcomings of this paper by focusing on the following aspects. More refined analysis, mining and verification processes will be carried out in conjunction with the fishing logbook data and fishery statistics held by fisheries management stakeholders. Moreover, the relationships between marine fisheries activities and marine environmental factors will be further analyzed and explored under the condition that marine environmental data are effectively obtained. Finally, future research will also combine the respective advantages of AIS, synthetic aperture radar (SAR), and nighttime light remote sensing data to identify fishing vessel types with a higher precision and achieve more comprehensive fishery monitoring.
Data Availability Statement
The raw data supporting the conclusions of this article will be made available by the authors, without undue reservation.
Author Contributions
YG interpreted the data and wrote the main manuscript. JZ, XZ, ZL, and JM developed the idea and reviewed the manuscript. GL, MB, and CC participated in data collection. All authors made substantial contributions to preparation and submission of manuscript. All authors discussed and approved the final manuscript.
Funding
This work was supported by the National Natural Science Foundation of China (No. 61971455, U2006207 and 42106177), Shandong Provincial Natural Science Foundation of China (No. ZR2021QF093), and State Key Laboratory Foundation of CEMEE (No. CEMEE2022Z0304B).
Conflict of Interest
The authors declare that the research was conducted in the absence of any commercial or financial relationships that could be construed as a potential conflict of interest.
Publisher’s Note
All claims expressed in this article are solely those of the authors and do not necessarily represent those of their affiliated organizations, or those of the publisher, the editors and the reviewers. Any product that may be evaluated in this article, or claim that may be made by its manufacturer, is not guaranteed or endorsed by the publisher.
Acknowledgments
We thank administrators and participants for their support during the study, and we are sincerely grateful to the editor and reviewers.
Supplementary Material
The Supplementary Material for this article can be found online at: https://www.frontiersin.org/articles/10.3389/fmars.2022.861395/full#supplementary-material
References
Akinbulire T., Schwartz H., Falcon R., Abielmona R. (2017). “A Reinforcement Learning Approach to Tackle Illegal, Unreported and Unregulated Fishing,” in 2017 IEEE Symposium Series on Computational Intelligence (SSCI), vol. 2017. (Honolulu, Hawaii, USA: IEEE), 1–8. doi: 10.1109/SSCI.2017.8285315
Avtar R., Singh D., Umarhadi D. A., Yunus A. P., Misra P., Desai P. N., et al. (2021). Impact of COVID-19 Lockdown on the Fisheries Sector: A CaseStudy From Three Harbors in Western India. Remote Sens. 183, 1–20. doi: 10.3390/rs13020183
Brekke C., Anfinsen S. N., Larsen Y. (2013). Subband Extraction Strategies in Ship Detection With the Subaperture Cross-Correlation Magnitude. IEEE Geosci. Remote Sens. Lett. 10, 786–790. doi: 10.1109/LGRS.2012.2223656
Chang Y., Anagaw A., Chang K., Wang Y., Hsiao C., Lee W., et al. (2019). Ship Detection Based on YOLOv2 for SAR Imagery. Remote Sensing. 11, 786. doi: 10.3390/rs11070786
Chaturvedi S. K. (2019). Study of Synthetic Aperture Radar and Automatic Identification System for Ship Target Detection. J. Ocean Eng. Scienc. 4, 173–182. doi: 10.1016/j.joes.2019.04.002
Chinazzi M., Davis J. T., Ajelli M., Gioannini C., Litvinova M., Merler S., et al. (2020). The Effect of Travel Restrictions on the Spread of the 2019 Novel Coronavirus (COVID-19) Outbreak. Science 368, 395–400. doi: 10.1126/science.aba9757
Clavelle T. (2021) COVID-19 Brings Unmatched Downturn in Global Fishing Activity. Available at: https://globalfishingwatch.org/data/covid-19-brings-unmatched-downturn-in-global-fishing-activity/ (Accessed 31 December, 2021).
Das B. K., Roy A., Som S., Chandra G., Kumari S., Sarkar U. K., et al. (2021). Impact of COVID-19 Lockdown on Small-Scale Fishers (SSF) Engaged in Floodplain Wetland Fisheries: Evidences From Three States in India (Haidelberg, Germany: Environmental Science and Pollution Research), 1–12. doi: 10.21203/rs.3.rs-569637/v1
Diao Y., Kodera S., Anzai D., Gomez-Tames J., Rashed E. A., Hirata A. (2020). Influence of Population Density, Temperature, and Absolute Humidity on Spread and Decay Durations of COVID-19: A Comparative Study of Scenarios in China, England, Germany, and Japan. One Health 12 (13), 100203. doi: 10.1016/j.onehlt.2020.100203
Ezequiel C., Carlos A. (2016). Use of VIIRS DNB Satellite Images to Detect Jigger Ships Involved in the Illex Argentinus Fishery Vol. 4 (Amsterdam, Netherlands: Remote Sensing Applications Society & Environment), 167–178. doi: 10.1016/j.rsase.2016.09.002
FAO (2020). “The State of World Fisheries and Aquaculture 2020,” in Sustainability in Action (Rome: Food and Agriculture Organization). doi: 10.4060/ca9229en
FAO (2021). The Impact of COVID-19 on Fisheries and Aquaculture Food Systems, Possible Responses: Information Paper November 2020 (Rome: Food and Agriculture Organization). doi: 10.4060/cb2537en
Ferra C., Tassetti A. N., Grati F., Pellini G., Polidori P., Scarells G., et al. (2018). Mapping Change in Bottom Trawling Activity in the Mediterranean Sea Through AIS Data. Marine Policy 94, 275–281. doi: 10.1016/j.marpol.2017.12.013
Frey B. B. (2018). “Pearson Correlation Coefficient,” in In The SAGE Encyclopedia of Educational Research, Measurement, and Evaluation (Berlin, Germany: Springer), 1299–1233.
Gerard M., José B. M., Antonio T. (2009). Operational Ship Monitoring System Based on Synthetic Aperture Radar Processing. Remote Sensing. 1 (3), 375–375. doi: 10.3390/rs1030375
Guan Y., Zhang J., Zhang X., Li Z., Meng J., Liu G., et al. (2021). Identification of Fishing Vessel Types and Analysis of Seasonal Activities in the Northern South China Sea Based on AIS Data: A Case Study of 2018. Remote Sens. 13, 1952. doi: 10.3390/rs13101952
Hakovirta M., Denuwara N. (2020). How COVID-19 Redefines the Concept of Sustainability. Sustainability 12, 3727. doi: 10.3390/su12093727
Hale T., Petherick A., Phillips T., Webster S. (2020) Oxford COVID-19 Government Response Tracker, Blavatnik School of Government. Available at: www.bsg.ox.ac.uk/covidtracker.
He B., Yan F., Yu H., Su F., Lyne V., Cui Y., et al. (2021). Global Fisheries Responses to Culture, Policy and COVID-19 From 2017 to 2020. Remote Sens. 13, 4507. doi: 10.3390/rs13224507
Honda K., Shoji R., Inaishi M. (2017). A Study of Standard Ship Track With AIS Data. J. Japan Institute Navigation 137, 97–102. doi: 10.9749/jin.137.97
Huang C., Wang Y., Li X., Ren L., Zhao J., Hu Y., et al. (2020). Clinical Features of Patients Infected With 2019 Novel Coronavirus in Wuhan, China. Lancet 395, 497–506. doi: 10.1016/s0140-6736(20)30183-5
Kroodsma D. A., Mayorga J., Hochberg T., Miller N. A., Boerder K., Ferretti F., et al. (2018). Tracking the Global Footprint of Fisheries. Science 359, 904–907. doi: 10.1126/science.aao5646
Lei P. R. (2019). Mining Maritime Traffic Conflict Trajectories From a Massive AIS Data. Knowledge Inf. Systems 62, 259–258. doi: 10.1007/s10115-019-01355-0
Li J., Cai Y., Zhang P., Zhang Q., Jing Z., Wu Q., et al. (2021). Satellite Observation of a Newly Developed Light-Fishing “Hotspot” Open South China Sea Remote Sens. Environment. 256, 112312. doi: 10.1016/j.rse.2021.112312
Li Q., Guan X., Wu P., Wang X., Zhou L., Tong Y., et al. (2020). Early Transmission Dynamics in Wuhan, China, of Novel Coronavirus–Infected Pneumonia. N Engl. J. Med 382, 1199–1207. doi: 10.1056/NEJMoa2001316
Liu Q., Liu W., Sha D., Kumar S., Chang E., Arora V., et al. (2020). An Environmental Data Collection for COVID-19 Pandemic Research. Data’ 5, 68. doi: 10.3390/data5030068
Liu J., Zhang J., Chen J. (2021). Novel Coronavirus Pneumonia Affects International Supply Chain Changes of Aquatic Products and Analysis of China’s Aquatic Products Supply Situation. World Agric. 2021, 20–27. doi: 10.13856/j.cn11-1097/s.2021.01.003
Li B., Xie X., Wei X., Tang W. (2020). Ship Detection and Classification From Optical Remote Sensing Images: A Survey - ScienceDirect. Chin. J. Aeronautics 34, 145–163. doi: 10.1016/j.cja.2020.09.022
Li X., Zhou L., Xiao Y., Wu W., Su F., Shi W. (2021). Spatial Characteristics Mining of Fishing Intensity in the Northern South China Sea Based on Fishing Vessels AIS Data. J. Geo-information Sci. 23, 850–859. doi: 10.12082/dqxxkx.2021.200328
Love D. C., Allison E. H., Asche F., Belton B., Cottrell R. S., Froehlich H. E., et al. (2021). Emerging COVID-19 Impacts, Responses, and Lessons for Building Resilience in the Seafood System. Glob. Food Secur. 28, 100494. doi: 10.31235/osf.io/x8aew
Murray B., Perera L. P. (2021). An AIS-Based Deep Learning Framework for Regional Ship Behavior Prediction. System Saf. 215, 107819. doi: 10.1016/j.ress.2021.107819
Natale F., Gibin M., Alessandrini A., Vespe M., Paulrud A. (2015). Mapping Fishing Effort Through AIS Data. PloS One 10, 130746. doi: 10.1371/journal.pone.0130746
Nottmeyer L. N., Sera F. (2021). Influence of Temperature, and of Relative and Absolute Humidity on COVID-19 Incidence in England - A Multi-City Time-Series Study. Environ. Res 196, 110977. doi: 10.1016/j.envres.2021.110977
Park J., Lee J., Seto K., Hochberg T., Wong B. A., Miller N., et al. (2020). Illuminating Dark Fishing Fleets in North Korea. Sci. Advances 6 (30), eabb1197. doi: 10.1126/sciadv.abb1197
Pelich R., Longepe N., Mercier G., Hajduch G., Garello R. (2015). AIS-Based Evaluation of Target Detectors and SAR Sensors Characteristics for Maritime Surveillance. IEEE J. Selected Topics Appl. Earth Observations Remote Sensing. 8 (8), 3892–3901. doi: 10.1109/JSTARS.2014.2319195
Pérez F. R., Vina M. (2021). From Below and From Within: Fishing Communities Under the COVID-19 Pandemic and Other Globalizations in Southern Manabí, Ecuador. Territ. Politics Gov. 368, 1–20. doi: 10.1080/21622671.2021.1960595
Sandirasegaram N., Vachon P. W. (2017). Validating Targets Detected by SAR Ship Detection Engines. Can. J. Remote Sensing. 43, 451–454. doi: 10.1080/07038992.2017.1342204
Shi K., Weng J. (2020). Impacts of the COVID-19 Epidemic on Merchant Ship Activity and Pollution Emissions in Shanghai Port Waters. Sci. Total Environ. 790, 148198. doi: 10.1016/j.scitotenv.2021.148198
Silveira P., Teixeira A. P., Soares C. G. (2013). Use of AIS Data to Characterise Marine Traffic Patterns and Ship Collision Risk Off the Coast of Portugal. J. Navigation 66 (6), 879–898. doi: 10.1017/S0373463313000519
Su C., Shan X., Yang T., Han Q. (2021). Interdecadal Changes in Keystone Species of Fish Community During Autumn in the Yellow Sea. Prog. Fishery Sci. 42, 1–14. doi: 10.19663/j.issn2095-9869.20200525001
Su H., Wang Y. (2012). Using MODIS Data to Estimate Sea Ice Thickness in the Bohai Sea (China) in the 2009–2010 Winter. J. Geophys. l Res.: Oceans 117, C10018. doi: 10.1029/2012JC008251
Tian H., Liu Y., Li Y., Wu C., Chen B., Kraemer M. U. G., et al. (2020). An Investigation of Transmission Control Measures During the First 50 Days of the COVID-19 Epidemic in China. Science 2020, 638–642. doi: 10.1126/science.abb6105
Tu E., Zhang G., Rachmawati L., Rajabally E. (2016). Exploiting AIS Data for Intelligent Maritime Navigation: A Comprehensive Survey From Data to Methodology. IEEE Trans. Intelligent Transportation Systems 19, 1559–1582. doi: 10.1109/TITS.2017.2724551
Wang H., Bi F., Cao M., Tang Y. (2018). Survey and Acoustic Assessment of Fishery Resources in Marine Ranching Area Surrounding Zhangzi Island in Autumn. J. Dalian Ocean University 33, 802–807. doi: 10.16535/j.cnki.dlhyxb.2018.06.019
Wang H., Zhu M., Lin, Chen D. (2017). Ship Detection in Optical Remote Sensing Image Based on Visual Saliency and AdaBoost Classifier. Optoelectronics Letters. 13, 151–155. doi: 10.1007/s11801-017-7014-9
Xu F., Liu J., Dong C., Wang X. (2017). Ship Detection in Optical Remote Sensing Images Based on Wavelet Transform and Multi-Level False Alarm Identification. Remote Sensing. 9 (10), 985. doi: 10.3390/rs9100985
Yamaguchi T., Asanuma I., Park J., Mackin K. J., Mittleman J. (2016). Estimation of Vessel Traffic Density from Suomi NPP VIIRS Day/Night Band. In: Proceedings of the Oceans Mts/IEEE Monterey (CA, USA: Monterey), 1–5. doi: 10.1109/OCEANS.2016.7761309
Yang D., Wu L., Wang S., Jia H., Li K. (2019). How Big Data Enriches Maritime Research – a Critical Review of Automatic Identification System (AIS) Data Applications. Transport Rev. 39, 775–773. doi: 10.1080/01441647.2019.1649315
Yan L., Liu Z., Yan J., Cheng J. (2019). Effects of Prolonging the Trawl Net Summer Fishing Moratorium Period in the East China Sea on the Conservation of Fishery Resources. J. Fishery Sci. China 26, 118–123. doi: 10.3724/SP.J.1118.2019.18243
Yen M., Schwartz J., Chen S., King C., Yang G., Hsueh P. (2020). Interrupting COVID-19 Transmission by Implementing Enhanced Traffic Control Bundling: Implications for Global Prevention and Control Efforts. J. Microbiol. Immunol. Infect. 53 (3), 377–380. doi: 10.1016/j.jmii.2020.03.011
Zhang S., Jin S., Zhang H., Wei F., Yang S. (2016). Distribution of Bottom Trawling Effort in the Yellow Sea and East China Sea. PloS One 11, e0166640. doi: 10.1371/journal.pone.0166640
Zhang L., Meng Q., Fang F. T. (2019). Big AIS Data Based Spatial-Temporal Analyses of Ship Traffic in Singapore Port Waters. Transportation Res. Part E: Logistics Transportation Rev. 129, 287–304. doi: 10.1016/j.tre.2017.07.011
Zhang S., Wu R., Xu K., Wang J. (2019). R-CNN-Based Ship Detection From High Resolution Remote Sensing Imagery. Remote Sensing. 11, 631. doi: 10.3390/rs11060631
Zhao Z., Ji K., Xing X., Zou H., Zhou S. (2013a). Ship Surveillance by Integration of Space-Borne SAR and AIS – Review of Current Research. J. Navigation 67, 177–189. doi: 10.1017/S0373463313000659
Zhao Z., Ji K., Xing X., Zou H., Zhou S. (2013b). Ship Surveillance by Integration of Space-Borne SAR and AIS—Further Research. J. Navigation 67, 295–309. doi: 10.1017/s0373463313000702
Zhao H., Yang Z., Liu H., Chen Y., Wang W., Kuang L., et al. (2020). Novel Coronavirus Pneumonia Impact on China’s Import and Export Slobber Industry and Response. J. Food Saf. Quality 11, 8180–8150. doi: 10.1056/NEJMoa2001017
Zhen R., Jin Y., Hu Q., Nikitakeos N. (2017). Maritime Anomaly Detection Within Coastal Waters Based on Vessel Trajectory Clustering and Naïve Bayes Classifier. J. Navigation 70 (3), 648–670. doi: 10.1017/S0373463316000850
Keywords: Automatic Identification System (AIS) data, winter-spring fishing, China’s sea areas, fishing grounds, COVID-19 pandemic
Citation: Guan Y, Zhang J, Zhang X, Li Z, Meng J, Liu G, Bao M and Cao C (2022) Study on the Activity Laws of Fishing Vessels in China’s Sea Areas in Winter and Spring and the Effects of the COVID-19 Pandemic Based on AIS Data. Front. Mar. Sci. 9:861395. doi: 10.3389/fmars.2022.861395
Received: 24 January 2022; Accepted: 30 March 2022;
Published: 28 April 2022.
Edited by:
Yen-Chiang Chang, Dalian Maritime University, ChinaReviewed by:
Yi-Che Shih, National Academy of Marine Research (NAMR), TaiwanYitong Chen, Ocean University of China, China
Copyright © 2022 Guan, Zhang, Zhang, Li, Meng, Liu, Bao and Cao. This is an open-access article distributed under the terms of the Creative Commons Attribution License (CC BY). The use, distribution or reproduction in other forums is permitted, provided the original author(s) and the copyright owner(s) are credited and that the original publication in this journal is cited, in accordance with accepted academic practice. No use, distribution or reproduction is permitted which does not comply with these terms.
*Correspondence: Xi Zhang, eGkuemhhbmdAZmlvLm9yZy5jbg==