- 1CCMAR, CIMAR, Universidade do Algarve, Faro, Portugal
- 2Arctic Research Centre, Aarhus University, Aarhus, Denmark
- 3Red Sea Research Center, King Abdullah University of Science and Technology (KAUST), Thuwal, Saudi Arabia
- 4Department of Ecoscience, Aarhus University, Silkeborg, Denmark
Accelerating warming and associated loss of sea ice are expected to promote the expansion of coastal marine forests (macrophytes) along the massive Arctic coastlines. Yet, this region has received much less attention compared to other global oceans. The available future projections of Arctic macrophytes are still limited to few species and regions, and mostly focused at lower latitude ranges, thus precluding well-informed IPCC impact assessments, conservation and management. Here we aim to quantify potential distributional changes of Arctic intertidal and subtidal brown macroalgae and eelgrass by the year 2100, relative to present. We estimate habitat suitability by means of species distribution modeling, considering changes in seawater temperature, salinity, nutrients and sea ice cover under two greenhouse gas emission scenarios, one consistent with the Paris Agreement (RCP 2.6) and the other representing limited mitigation strategies (RCP 8.5). As data on substrate conditions do not exist, the models were restricted to the depth range supporting Arctic macrophytes (down to 5 m for eelgrass and 30 m for brown macroalgae). Models projected major expansions of Arctic macrophytes between 69,940 and 123,360 km2, depending on the climate scenario, with polar distribution limits shifting northwards by up to 1.5 latitude degrees at 21.81 km per decade. Such expansions in response to changing climate will likely elicit major changes in biodiversity and ecosystem functions in the future Arctic. Expansions are, however, less intense than those already realized over the past century, indicating an overall slowing down despite accelerated warming as habitats become increasingly occupied.
Introduction
The Arctic Ocean is the epicenter of global climate change, warming at three times the global average rate (IPCC, 2021). As a result, ice loss has been accelerated within the past two decades (Stroeve et al., 2012), triggering a cascade of changes to its ecosystems and beyond (Duarte et al., 2012). The Arctic contains 35% of global coastlines (Lantuit et al., 2012) and supports highly productive marine forests of eelgrass and macroalgae (intertidal and subtidal; Krause-Jensen et al., 2020). Their productivity and growth are largely constrained by freezing temperatures, sea ice scouring and light limitation due to ice cover (Krause-Jensen et al., 2020). Hence, warming and sea ice reduction can lead to an expansion of macrophytes, both as temperatures become favorable, and sea ice losses make new habitats available (Jueterbock et al., 2013; Krause-Jensen and Duarte, 2014; Assis et al., 2017a; Wilson and Lotze, 2019).
A recent assessment provided evidence of expanding macrophyte distribution limits in the Arctic along with increased abundance, productivity, and species richness over the past decades (Krause-Jensen et al., 2020). Moreover, species distribution models, although challenged by limited observation records, coarse resolution of environmental layers and lack of information on substrate in the Arctic, estimated a current (2000–2017) potential distribution area of brown macroalgae in the Arctic of 655,000 km2 (140,000 km2 intertidal, 515,000 km2 subtidal, with some overlap between the two). These areas represent an increase of about 45% for subtidal and 8% for intertidal macroalgae since 1940–1950, with poleward migration rates of biogeographic limits averaging between 18 and 23 km per decade (Krause-Jensen et al., 2020).
The poleward expansion of macrophytes (i.e., borealization of temperate communities) has important consequences for the functioning of Arctic ecosystems given their roles in e.g., carbon and nutrient cycling and storage (Filbee-Dexter et al., 2019; Vilas et al., 2020; Gilson et al., 2021). Because of their much higher C/N and C/P ratios compared to phytoplankton (Duarte, 1992), macrophytes can export far more carbon per unit of available nutrients than phytoplankton, thereby having the capacity to enhance the biological carbon pump. Indeed, macrophytes export about half of their primary production (Duarte and Cebrián, 1996) and contribute to subsidize benthic food webs and carbon sequestration in coastal sediments and the deep-sea (Krause-Jensen and Duarte, 2016; Duarte and Krause-Jensen, 2017; Ortega et al., 2019). In particular, the extended summer daylight duration in the Arctic (maximum length of a single “day” ranging from approx. 24 h at the Arctic Circle to 4,400 h (183 days) at the North Pole), leads to high growth and productivity (Krause-Jensen et al., 2016), especially where ice cover is reduced (Krause-Jensen et al., 2012). The expansion of macrophytes into the Arctic also creates habitats for associated organisms, including fish species of commercial interest (e.g., cod juveniles find refuge from predators in marine forests of macroalgae; Gotceitas et al., 1995; Teagle et al., 2017), which are also projected to expand poleward (Martins et al., 2021). At the same time, the local impacts of borealization can span from increased competition with native species (Chan et al., 2019) to the complete squeeze out of high Arctic ecosystems, resulting in the potential loss of an entire biogeographic zone (Fossheim et al., 2015).
The Paris Agreement provided a roadmap to limit warming up to 2°C above pre-industrial levels (United Nations Framework Convention on Climate Change, 2015), while considering climate change impact assessments based on policy-relevant research [e.g., Intergovernmental Panel on Climate Change (IPCC)]. To project impacts on ecosystems, species distribution models (SDMs) comparing present-day vs. projected future distributions have been performed across taxa, and under contrasting Representative Concentration Pathway (RCP) scenarios of climate change, featuring from high compliance to greenhouse gas reduction (e.g., RCP2.6) to limited mitigation strategies (e.g., RCP8.5; Handorf and Dethloff, 2012; Assis et al., 2017b; Melo-Merino et al., 2020). Despite the hypothesized poleward expansion of marine macrophytes in the future, the Arctic has received less attention compared to other global oceans (Melo-Merino et al., 2020; Starko et al., 2021). At present, projections for macrophytes are only available for few species or at regional scales (Campana et al., 2009; Müller et al., 2009; Krause-Jensen and Duarte, 2014; Assis et al., 2017a; Krause-Jensen et al., 2020) and are mostly focused on the potential impacts at lower latitude ranges, or the replacement of cold-adapted by warm-adapted taxa (Vergés et al., 2016; Filbee-Dexter et al., 2020; Pessarrodona et al., 2021). A comprehensive estimate of the expansion of macrophytes across the Arctic under contrasting scenarios of future climate change is still missing, precluding well-informed IPCC impact assessments, conservation, mitigation and management, by both international and national committees, organizations, and other stakeholders.
Here we provide a policy-relevant biodiversity impact assessment by projecting the expansion of macrophytes based on future climate conditions within the geographic boundaries of the Pan-Arctic region, as defined by the Arctic Council. We do so by combining a newly developed distribution model (Krause-Jensen et al., 2020) of brown macroalgae and eelgrass biomes with projected changes in temperature, salinity, nutrients and sea ice cover derived from the Climate Model Intercomparison Project. Modeling full biomes, instead of applying a species-by-species approach, allowes overcoming potential biodiversity data limitations, particularly important in this data-poor region (Jayathilake and Costello, 2021; Starko et al., 2021). Near present-day distributions are compared with projected changes under the RCP 2.6 and the RCP 8.5 (Riahi et al., 2011; van Vuuren et al., 2011; Wang et al., 2018). By comparing contrasting scenarios of greenhouse gas emissions, we quantify the potential range of the future extent (i.e., coverage) of suitable habitats for macrophytes in the pan-Arctic region, the epicenter of global climate change.
Materials and Methods
Future distributional shifts of Arctic marine forests were projected under contrasting RCP scenarios with the recently developed macrophyte distribution models (Krause-Jensen et al., 2020). In this process, species were aggregated into full biomes of brown intertidal macroalgae, brown subtidal macroalgae and eelgrass (e.g., Krause-Jensen et al., 2020; Jayathilake and Costello, 2021) to overcome potential sampling bias in this data-poor region (Starko et al., 2021). Modeling was based on the ensemble of Adaptive Boosting (AdaBoost) and Boosted Regression Trees (BRT), two machine learning algorithms known for high predictive performances in SDMs (Assis et al., 2017a; Krause-Jensen et al., 2020; Fragkopoulou et al., 2021) able to fit complex interactions between predictor variables, while reducing overfitting through hyper-parametrization and forcing of monotonicity responses (Elith et al., 2008).
The algorithms fitted environmental predictor layers against a comprehensive dataset of species occurrence records gathered from the fine-tuned dataset of marine forests (Assis et al., 2020). This comprised 275,154 records of 31 brown intertidal macroalgae species, 552,542 records of 233 brown subtidal macroalgae species and 14,287 records for the eelgrass Zostera marina, which represented observations across the Arctic and temperate Northern Atlantic and Pacific realms (Spalding et al., 2007), from which species might potentially shift poleward (Krause-Jensen et al., 2020). Due to lack of absence records at the scales of the study, the same number of pseudo-absences as presences were produced in random locations where no presences were recorded (Barbet-Massin et al., 2012).
Key environmental predictors were extracted from Bio-ORACLE v2.1 (Assis et al., 2017b), a dataset at a spatial resolution of 0.08° that provides present-day climatologies from the Copernicus service, and the ensemble of multiple atmospheric-ocean general circulation models (CCSM4, GFDL-ESM2G, HadGEM2-ES, IPSL-CM5A-LR, MIROC-ESM) from the Climate Model Intercomparison Project for future RCP. Candidate predictors followed the available macrophyte distribution models (Krause-Jensen et al., 2020) to reflect physiological constraints (maximum ocean temperature and minimum salinity), essential resources (nutrients as mean nitrate concentration) and disturbance (ice cover, which also affects the light environment). The models also considered distinct environmental information for intertidal and subtidal biomes, by using surface and benthic layers (i.e., along bottom conditions for the average depth) of Bio-ORACLE (Assis et al., 2017b). Potential intertidal macroalgal areas were clipped with a gridded mask delimiting global coastlines at the resolution of Bio-ORACLE (e.g., Assis et al., 2017a). Because information on light conditions at the seafloor is not available for the future, subtidal eelgrass and macroalgae areas were clipped using a mask considering suitable depths from 0 down to 5 and 30 m depth, respectively, developed with the General Bathymetric Chart of the Oceans (GEBCO, 2019). These depth ranges are typical for Arctic eelgrass (Lalumière et al., 1994; Harris et al., 2008; Olesen et al., 2015) and brown macroalgae (Assis et al., 2017a). As substrate conditions are not mapped for the Arctic, it was not possible to delimit sandy vs. rocky areas supporting eelgrass and macroalgae, respectively.
The negative effect of spatial autocorrelation in the models was reduced by testing the spatial variability of climatic predictors as a function of distance, with correlograms estimating minimum distances at which predictors are not significantly autocorrelated (Boavida et al., 2016; Krause-Jensen et al., 2020), which were 10–12.5 km, depending on the datasets (Supplementary Figure 1). This procedure is necessary to reduce surplus information that can lead to poorly calibrated models with inappropriate inference and prediction (Dormann et al., 2007). Hence, we considered records within those distances not to be independent and, therefore, pruned records to a final database of 2,635, 2,764, and 1,410 independent records of occurrence of intertidal brown macroalgae, subtidal brown macroalgae and eelgrass, respectively.
The SDMs used a cross-validation (CV) framework with sixfold independent blocks with edges equal to the minimum correlated distance inferred per dataset (Assis et al., 2017a; Fragkopoulou et al., 2021; Martins et al., 2021). This CV framework identified the optimal combination of hyperparameters of the models by the “grid search” method, which involved tree complexity (1–6), learning rate (0.01, 0.005, and 0.001) and number of trees (50–1,000, step 50) for BRT, and degrees of freedom (1–5), shrinkage (0.1–1, step 0.1) and number of interactions (50–500, step 50) for AdaBoost. The CV framework also assessed model performance and potential for transferability (i.e., the capacity to accurately predict distributions outside the temporal window of training data) in independent data, by reporting the average sensitivity (true positive rate; Allouche et al., 2006) and the area under the curve (AUC) obtained with the optimal hyper-parameters (Assis et al., 2018; Krause-Jensen et al., 2020; Fragkopoulou et al., 2021). To further reduce overfitting, models were forced to produce monotonic responses, positive for fitting nitrate and salinity, and negative for fitting ice cover and maximum temperature (Assis et al., 2017a; Gouvêa et al., 2020).
Distribution maps for the present and under the future RCP2.6 and RCP8.5 scenarios were produced at a 5 arcmin resolution as imposed by the resolution of environmental data. The maps were produced for intertidal and subtidal macroalgae and eelgrass biomes by ensembling (mean function; Araújo and New, 2007) the responses of both AdaBoost and BRT models using the corresponding optimal parameters. Ensemble modeling is particularly important for performing future projections, as it reduces single-algorithm bias and therefore the overall uncertainty of results (Araújo and New, 2007). Maps were reclassified to reflect binomial responses—presence and absence of biome—using a threshold allowing the maximization of specificity (true negative rate) and sensitivity (Jiménez-Valverde and Lobo, 2007; Assis et al., 2017a). Distributional shifts were assessed in terms of area and latitudinal range gain by comparing the maps developed for the present with those forecasting distributions under the two RCP scenarios.
Distribution models were developed in R (Language and Environment for Statistical Computing) using the packages: biomod2, blockCV, dismo, gbm, mda, parallel, raster, sdmpredictors, SDMTools, and spThin.
Results
The distribution models developed for pan-Arctic intertidal and subtidal macroalgae, and eelgrass biomes achieved good potential for transferability, i.e., the capacity to accurately predict distribution outside the temporal window of training data inferred with cross-validation (CV true positive rate, Sensitivities > 0.85; CV area of the receiver operating characteristic curve, AUC > 0.8; Table 1). The combination of models into a unique ensemble also achieved good performance, largely matching the known distribution of Arctic intertidal and subtidal macroalgae, and eelgrass biomes, as inferred when compared to occurrence data (Sensitivities < 0.85, AUC < 0.8; Table 1).
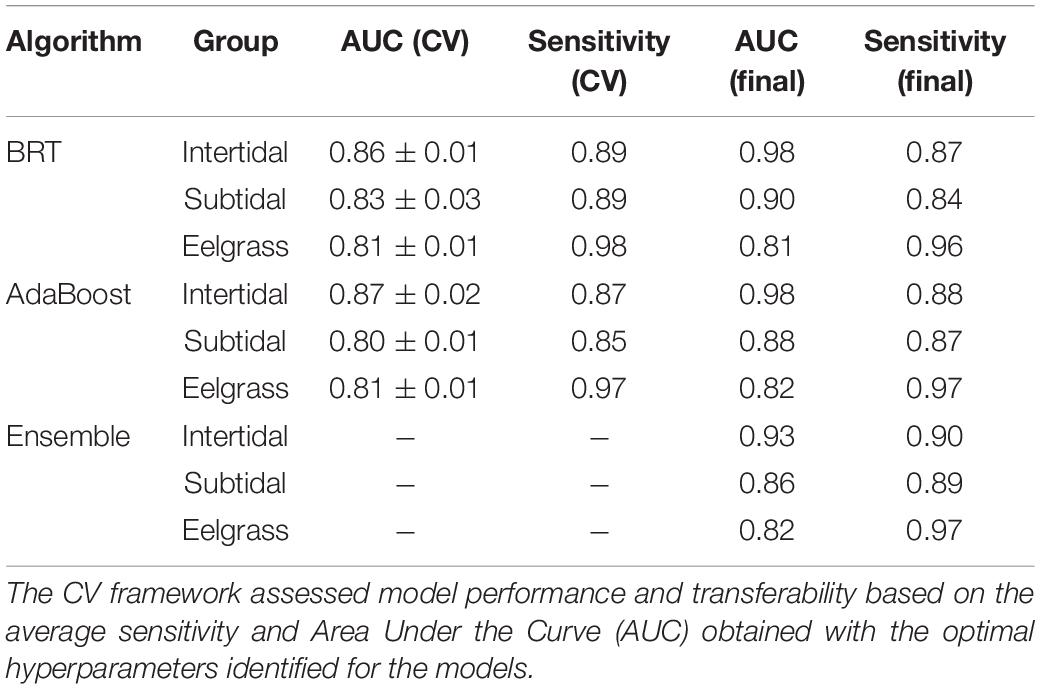
Table 1. Performance of the machine learning algorithms Adaptive Boosting (AdaBoost) and Boosted Regression Trees (BRT) inferred with cross-validation (CV) and the final predictive ensemble for pan-Arctic intertidal and subtidal brown macroalgae, and eelgrass biomes.
Sea ice and maximum temperature were particularly important in explaining the distribution of Arctic macrophytes, consistently retrieving high relative contributions to the three vegetation models (>15% contribution; Figure 1). Salinity and nutrient concentrations had marginal contributions to all models (Figure 1), in particular for intertidal macroalgae. The models showed no signs of overfitting: both maximum temperatures and ice coverage beyond modeled upper thresholds explained absences, while on a lower relative importance, increasing nutrients and salinity explained macrophyte occurrence (Supplementary Figures 2–4).
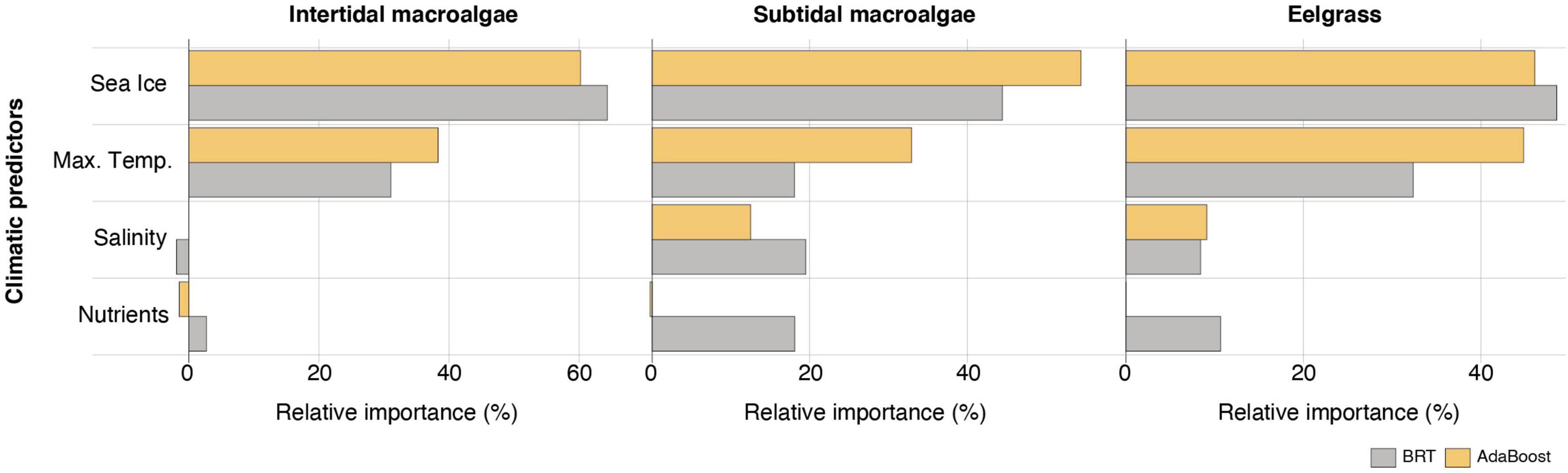
Figure 1. Relative contribution of each environmental predictor to the performance of the machine learning algorithms Boosted Regression Trees (BRT) and Adaptive Boosting (AdaBoost), for pan-Arctic intertidal and subtidal brown macroalgae, and eelgrass biomes.
Within the geographic boundaries of the pan-Arctic region, models developed for present conditions (climatology of 2000–2017) predict 141,520, 515,766, and 391,180 km2 of potential suitable habitats for intertidal macroalgae, subtidal macroalgae, and eelgrass, respectively (Figure 2 and Table 2). The modeled areas of the three biomes overlap due to the relatively coarse nature of the model and the lack of substrate data that could otherwise help separate macroalgal and eelgrass biomes. Therefore, the total suitable habitat of pan-Arctic macrophytes in the present is estimated at 516,470 km2, i.e., slightly larger than the estimated suitable habitat for subtidal macroalgae (Table 2).
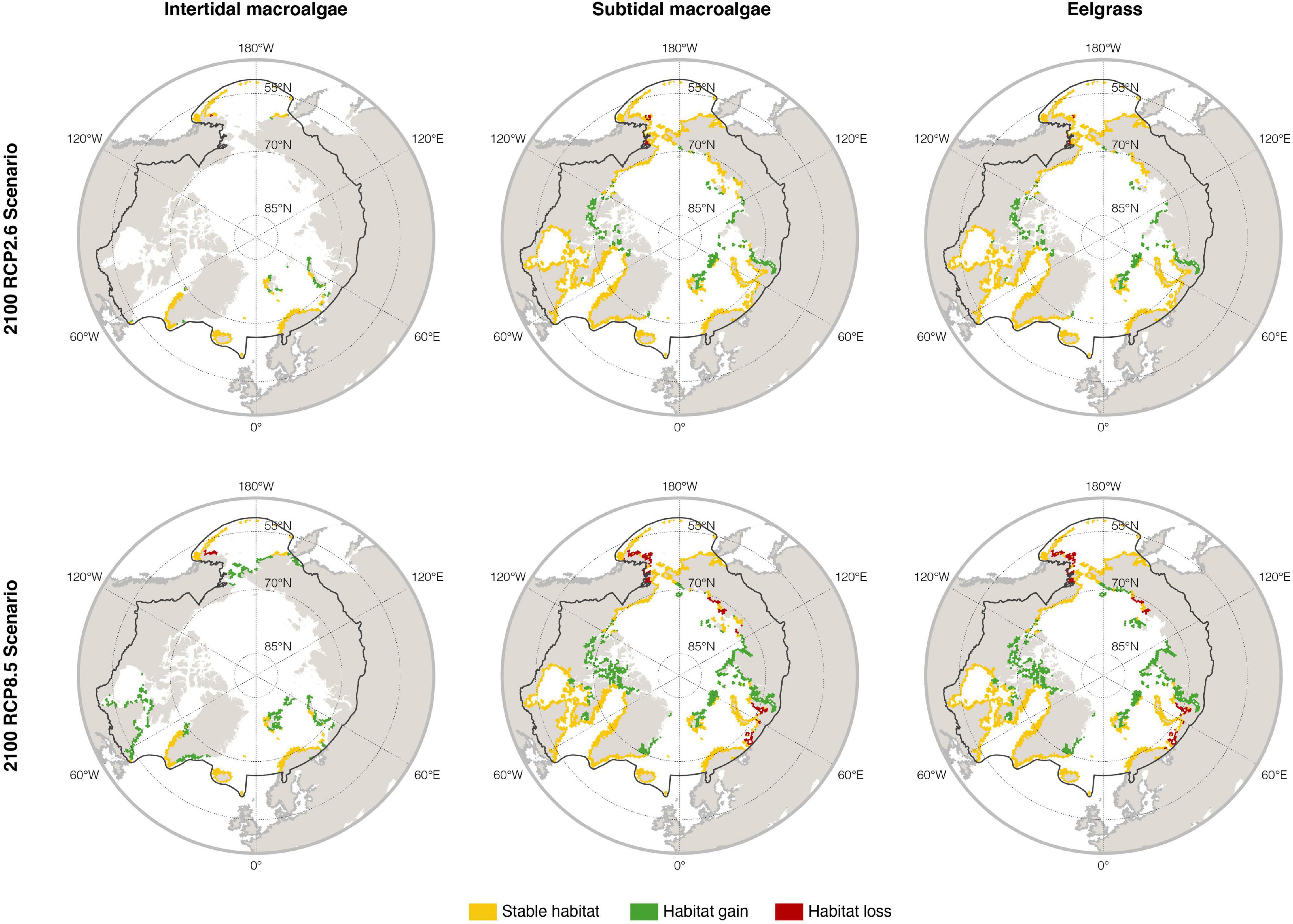
Figure 2. Potential changes in habitat suitability projected with species distribution modeling from the present (shown in yellow) to the future (2090–2100, expansion in green, losses in red, stable habitats in yellow) under contrasting scenarios of greenhouse gas emissions (RCP 2.6 and RCP 8.5) for pan-Arctic intertidal and subtidal macroalgae, and eelgrass biomes.
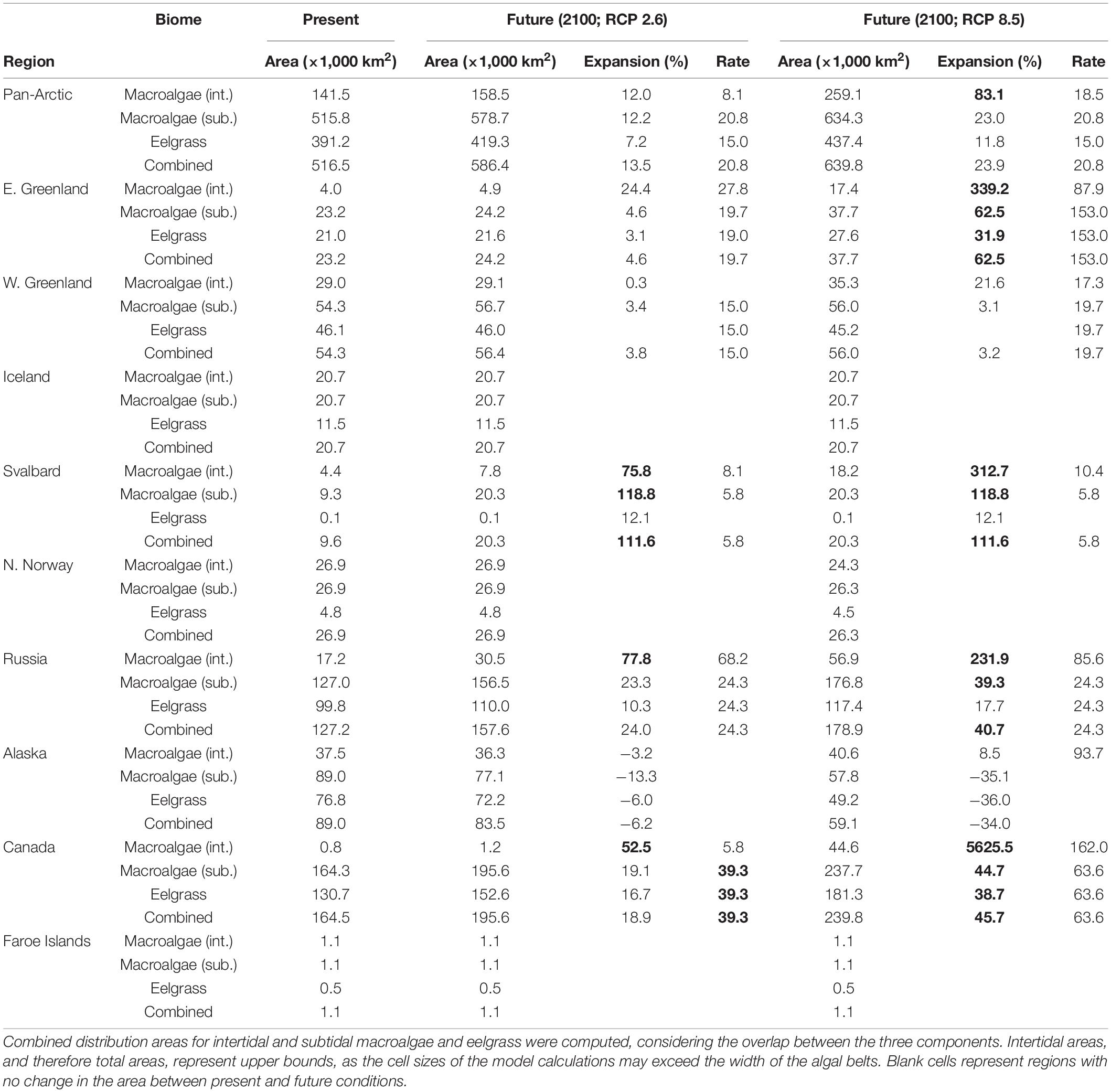
Table 2. Potential distribution area (×1,000 km2), area expansion (%; values > 25% in bold) and poleward migration rate (km decade–1) for intertidal and subtidal macroalgae, and for eelgrass, estimated with species distribution modeling for the pan-Arctic region and the Arctic sectors for the present and future (2090–2100) under contrasting scenarios of greenhouse gas emissions (RCP 2.6 and RCP 8.5).
The forecasts for the year 2100 projected a general poleward expansion of macrophytes under both contrasting emission scenarios (Figure 2). The forecasted pan-Arctic expansion was 12.0% for intertidal macroalgae, 12.2% for subtidal macroalgae and 7.2% for eelgrass, under the lower emission scenario RCP2.6 (Table 2). This involves poleward latitudinal shifts of up to 1.5 degrees by 2,100, with migration rates of 8.1 km decade–1 for intertidal macroalgae, 20.8 km decade–1 for subtidal macroalgae and 15.0 km decade–1 for eelgrass (RCP2.6; Figure 2 and Table 2). In contrast, the models predicted a potentially much larger Pan-Arctic expansion under the high-emission scenario RCP8.5, with up to 83.1% increase in area for intertidal macroalgae, 23.0% increase for subtidal macroalgae and 11.8% increase for eelgrass compared to present times. This represents migration rates of 18.5 km decade–1 for intertidal macroalgae, 20.8 km decade–1 for subtidal macroalgae and 15.0 km decade–1 for eelgrass (Figure 2 and Table 2).
Projected changes were particularly high along the shorelines of Eastern Greenland, Russia, Svalbard and Canada (potential area expansion of the combined macrophyte biome of 5–112% under RCP2.6; 41–112% under RCP8.5) associated with a maximum latitudinal expansion of up to 1.5 degrees, and migration rates of 5.8–39.3 km decade–1 under RCP2.6 and 5.8–153 km decade–1 under RCP8.5 (Figure 2 and Table 2). Projected changes were lower along the shores of Western Greenland (3–4% potential increase in both scenarios), zero for Iceland, Faroe Islands and N. Norway, and negative for Alaska under both scenarios (−6 to −34%; Table 2).
Discussion
Our study forecasts that the ongoing expansion of Arctic marine macrophytes with climate change (Krause-Jensen et al., 2020) will proceed throughout the twenty-first century under both RCP2.6 and RCP8.5 scenarios, although with variability across the Pan-Arctic region. Future poleward expansions of marine forests have been hypothesized, but until now projections have addressed few species, specific regions or lower latitude ranges (e.g., Campana et al., 2009; Müller et al., 2009; Assis et al., 2017a). By modeling the full biomes (e.g., Jayathilake and Costello, 2021) of marine forests of brown macroalgae and eelgrass under contrasting climate change scenarios, we provide new baselines for well-informed IPCC impact assessments, conservation and management in the pan-Arctic region, the epicenter of global climate change.
The performance and transferability potential of the macrophyte models was generally high, and their combination in a unique ensemble proved to be a robust approach, with predicted distributions largely consistent with the observed records (Assis et al., 2020). Overall, the most important predictors explaining the distribution of the three types of vegetation (intertidal and subtidal brown macroalgae, and eelgrass) reflect physiological constraints (ocean temperature) and disturbance (ice cover, also affecting the light environment), in line with previous studies (Krause-Jensen et al., 2020; Goldsmit et al., 2021).
The macrophyte projections were highly dependent on the emission concentration pathway scenario considered, with potential expansions of the combined pan-Arctic biome of brown intertidal and subtidal macroalgae and eelgrass ranging between 69,940 and 123,360 km2 by the end of this century. All three types of vegetation had comparable projected patterns of potential area expansion, particularly along the shorelines of Eastern Greenland, Russia, Svalbard and Canada. There, the northern range limits predicted for present-day conditions, can migrate poleward, as suitable habitats become available in the future. Additionally, these are the areas where higher warming rates and greatest sea ice reductions have been observed (Supplementary Figure 5 and Supplementary Table 1; Mudryk et al., 2018; Derksen et al., 2019; Flato et al., 2019), and where long-term research (Greenland and Russia) document higher macrophyte productivity or biomass over the past decades and suggests further increases in the years to come (Filbee-Dexter et al., 2019; Krause-Jensen et al., 2020). Conversely, in Arctic regions where habitats are currently more suitable in terms of temperature and sea ice, lower or no expansions were projected. Specifically, limited expansion was predicted along the W. Greenland coast, no expansion was projected for Iceland, Faroe Islands and N. Norway, and habitat losses were projected for Alaska (Supplementary Figure 5 and Supplementary Table 1). Such losses could be possibly driven by reductions in salinity (Spurkland and Iken, 2011) due to ice and glacier melting leading to increased riverine discharges, as observed in Alaska (Hugonnet et al., 2021; Young et al., 2021).
Poleward expansions were comparable between the three types of vegetation, with rates up to 20.8 km decade–1. These rates are similar to the realized expansion of pan-Arctic subtidal macrophytes during the past decades (18.3 km decade–1; Krause-Jensen et al., 2020), but are half of the average realized rate of 42.3 km decade–1 reported for macroalgae as part of a global study (but based on only 14 macroalgal observations compiled from two studies in the subtropical to temperate NE Atlantic (Poloczanska et al., 2013; their Supplementary Table 6).
Our projections have important implications for forecasts on the functioning of future Arctic ecosystems. Marine forests of macrophytes increase the local organic matter production relative to that of phytoplankton under the ceiling imposed by nutrient supply through their much higher C/N ratios (Duarte, 1992). In addition, macrophytes contribute to carbon sequestration, with eelgrass storing carbon in their sediments, as evidenced by recent assessments in Western Greenland (Marbà et al., 2018), and macroalgae exporting carbon to sinks beyond the coastal habitat (Krause-Jensen and Duarte, 2016). Further, eelgrass contributes to the accretion and stabilization of sediments and, together with macroalgal forests, to wave attenuation and the protection of shorelines, thereby helping to prevent coastal erosion, which is an increasing problem across the Arctic with climate change (Lantuit et al., 2012). Potential expansion of macrophytes could also provide refugia from ocean acidification, particularly as these habitats expand into the high Arctic, where sustained photosynthesis under long summer daytime duration provides extended periods of elevated pH (Krause-Jensen et al., 2016). At the ecosystem level, an expansion of macrophytes in the Arctic could increase biodiversity (Dijkstra et al., 2017) through the creation of habitat for multiple associated species, including species of commercial interest, such as cod, mussels, scallops and crabs (Gotceitas et al., 1995; Teagle et al., 2017), some of which are also projected to shift poleward in the future (Martins et al., 2021), and—in the case of eelgrass—geese (Ganter, 2000), which are important game species for indigenous Arctic people. New species have already been observed in the high Arctic (e.g., Weslawski et al., 2010) and new opportunities for additional sources of income and sustainable livelihoods for Arctic communities could arise from the expansion of macroalgae and the potential of seaweed aquaculture, already introduced in Pacific Canada, Alaska and Faroe Island (Stekoll, 2019). Contrarily, the borealization of the Arctic could increase competition with native species and alter community compositions, from cold-tolerant to more temperate species (Fossheim et al., 2015). Ecological impacts could potentially involve loss of high Arctic native ecosystems (Fossheim et al., 2015) as warming proceeds.
Despite the high performance of the models, we acknowledge limitations. Macrophyte data gaps are still large in this data-poor region (Starko et al., 2021), therefore, modeling entire biomes was preferred to a species-by-species approach (Jayathilake and Costello, 2020). Additional information on biotic interactions and abiotic characteristics, such as seafloor characteristics, could improve the models and coverage estimates, but no such data are currently available (Jayathilake and Costello, 2020). For example, accounting for the estimated percentage of rocky habitat reduced the predicted distribution of marine forests of brown macroalgae in the Arctic by 52% (Krause-Jensen et al., 2020). Moreover, the cell size of the environmental data (5 arcmin) used in the models can cover broader areas than those defined by the actual depth distributions, hence, our estimates represent the upper bounds of potential suitable areas. This is particularly obvious for intertidal distributions, for which the alongshore belt of suitable habitats is often restricted, but in the models, it can overlap with subtidal distributions (e.g., Iceland). The same effect is expected between the vertical distribution of eelgrass over deeper subtidal macroalgae. Although the niches for subtidal macroalgae and eelgrass overlap for the environmental data in the models, they are expected to be segregated based on seafloor characteristics, with macroalgae dominating rocky shores and seagrass restricted to soft and sandy sediments. The Arctic coastal zone is likely approximately equally split between rocky shores and soft sediments, with the latter prevailing in the extensive shelves receiving discharge from the large Arctic rivers, particularly the Siberian coast and Svalbard (Young and Carilli, 2019). Water dynamics, such as wave energy and sea currents, may further affect the distribution of marine forests, with brown macroalgae benefiting from higher energy environments (Fragkopoulou et al., 2022) while eelgrass is confined to relatively sheltered settings (Dahl et al., 2020). Additionally, other factors such like water dynamics and particularly sea currents, could influence the dispersal potential of marine forests by either promoting or limiting their possibility of expansion in the suitable habitats here predicted for the Arctic. For example, the West Greenland Current may facilitate poleward dispersal of propagules while the east Greenland coast is dominated by southward currents not promoting poleward dispersal. Also, while the model forecasts that habitat conditions will be suitable for eelgrass, e.g., in remote Arctic settings such as Franz Josef Land, it is unlikely that natural dispersion will allow eelgrass to fill this niche.
The larger area predicted for subtidal brown macroalgae compared to eelgrass in the Arctic is determined by the much deeper distribution of brown macroalgae, i.e., to an average depth of 30 m adopted in the model, compared to 5 m for eelgrass populations (cf. section “Materials and Methods”). Yet, the 30 m depth limit used for subtidal macroalgae is conservative, as kelps may in extreme cases occur down to about 60 m in the Arctic (Krause-Jensen et al., 2019). Light conditions are key determinants of depth extension on macrophytes. But although data on underwater light levels are available for the present Arctic (Gattuso et al., 2020), these are not available in future projections and this variable could therefore not be included in the models. Future underwater light extinction could change with the variation in Arctic planktonic productivity and be locally reduced in response to increased riverine sediment discharge e.g., from melting glaciers, while reduced sea-ice cover could lead to increased incident solar radiation. Melting glaciers can also result in siltation, as fine sediments are washed into the sea, a phenomenon already observed, e.g., in Franz Josef Islands (Gagaev et al., 2019). Ice melting contributing to sea level rise could further influence light penetration in the Arctic waters. Hence, light penetration in the Arctic may increase in some areas and decrease in others, but no forecasts are available. Lastly, the area estimates do not include red algae, which may be important components of macrophyte communities in the deeper areas of the coastal zone. Green macroalgae are also not included in the models but their distribution is expected to be contained within the distribution area of the brown algae. Data on the abovementioned constrains are presently lacking, but are expected to result in further refinement of our models, once available.
In summary, we provide a quantitative impact assessment forecasting the extent to which climate change will continue to drive the expansion of pan-Arctic marine forests, following the expanding trend documented along the past century (Krause-Jensen et al., 2020). Macrophyte expansion from 1940–50 to 2000–2017 has earlier been estimated at about 170,000 km2 (sum for intertidal and subtidal brown macroalgae) across the Pan-Arctic (Krause-Jensen et al., 2020) and we project further expansions between about 70,000 and 125,000 km2 until the end of this century, depending on the emission scenario. The projected future expansion represents about 1–2% of the estimated global macrophyte area (Duarte, 2017), but is less than the expansion already realized over the past century. Hence, macrophyte expansions with future climate may be slowing down, despite accelerating Arctic warming. Potential habitats, constrained by depth, are probably becoming increasingly occupied despite the Arctic permafrost comprising about 34% of the global shoreline (Lantuit et al., 2012). This is also supported by our prediction of differences in future macrophyte expansions between Arctic regions with largest relative expansions predicted for the currently coldest regions. In any case, our projections support the Arctic as an increasingly important region for marine vegetated habitats. The sustained poleward expansion of macrophytes will continue to elicit major changes in biodiversity and ecosystem functions in the future Arctic.
Data Availability Statement
Publicly available datasets were analyzed in this study. This data can be found here: Occurrence and environmental data used to perform the analyses are available at https://doi.org/10.1038/s41597-020-0459-x and https://doi.org/10.1111/geb.12693.
Author Contributions
JA, ES, CD, EF, and DK-J conceived the ideas and reviewed the writing. JA and EF analyzed the data. JA, CD, EF, and DK-J led the writing. All authors contributed to the article and approved the submitted version.
Funding
This study was supported by the Independent Research Fund Denmark through the project “CARMA” (8021-00222B) and the European Union through the project “FACE-IT” to DK-J, the Foundation for Science and Technology (FCT) through projects UID/Multi/04326/2020 to CCMAR and PTDC/BIA-CBI/6515/2020, the transitional norm DL57/2016/CP1361/CT0035 to JA and the fellowship SFRH/BD/144878/2019 to EF, and a Pew Marine Fellowship to ES.
Conflict of Interest
The authors declare that the research was conducted in the absence of any commercial or financial relationships that could be construed as a potential conflict of interest.
Publisher’s Note
All claims expressed in this article are solely those of the authors and do not necessarily represent those of their affiliated organizations, or those of the publisher, the editors and the reviewers. Any product that may be evaluated in this article, or claim that may be made by its manufacturer, is not guaranteed or endorsed by the publisher.
Supplementary Material
The Supplementary Material for this article can be found online at: https://www.frontiersin.org/articles/10.3389/fmars.2022.850368/full#supplementary-material
References
Allouche, O., Tsoar, A., and Kadmon, R. (2006). Assessing the accuracy of species distribution models: prevalence, kappa and the true skill statistic TSS. J. Appl. Ecol. 436, 1223–1232.
Araújo, M. B., and New, M. (2007). Ensemble forecasting of species distributions. Trends Ecol. Evol. 22, 42–47.
Assis, J., Araújo, M. B., and Serrão, E. A. (2017a). Projected climate changes threaten ancient refugia of kelp forests in the North Atlantic. Glob. Change Biol. 24, 1365–2486. doi: 10.1111/gcb.13818
Assis, J., Fragkopoulou, E., Frade, D., Neiva, J., Oliveira, A., Abecasis, D., et al. (2020). A fine-tuned global distribution dataset of marine forests. Sci. Data 7:119. doi: 10.1038/s41597-020-0459-x
Assis, J., Serrão, E. A., Coelho, N. C., Tempera, F., Valero, M., and Alberto, F. (2018). Past climate changes and strong oceanographic barriers structured low – latitude genetic relics for the golden kelp Laminaria ochroleuca. J. Biogeogr. 45, 2326–2336. doi: 10.1111/jbi.13425
Assis, J., Tyberghein, L., Bosch, S., Verbruggen, H., Serrão, E. A. A., and De Clerck, O. (2017b). Bio-ORACLE v2.0: extending marine data layers for bioclimatic modelling. Glob. Ecol. Biogeogr. 27, 277–284.
Barbet-Massin, M., Jiguet, F., Albert, C. H., and Thuiller, W. (2012). Selecting pseudo-absences for species distribution models: how, where and how many? Methods Ecol. Evol. 3, 327–338. doi: 10.1111/j.2041-210x.2011.00172.x
Boavida, J., Assis, J., Silva, I., and Serrão, E. A. (2016). Overlooked habitat of a vulnerable gorgonian revealed in the mediterranean and Eastern Atlantic by ecological niche modelling. Sci. Rep. 6:36460. doi: 10.1038/srep36460
Campana, G. L., Zacher, K., Fricke, A., Molis, M., Wulff, A., Quartino, L. M., et al. (2009). Drivers of colonization and succession in polar benthic macro- and microalgal communities. Bot. Mar. 52, 655–667.
Chan, F. T., Stanislawczyk, K., and Sneekes, A. C. (2019). Climate change opens new frontiers for marine species in the Arctic: current trends and future invasion risks. Glob. Chang. Biol. 25, 25–38. doi: 10.1111/gcb.14469
Dahl, M., Asplund, M. E., Björk, M., Deyanova, D., Infantes, E., Isaeus, M., et al. (2020). The influence of hydrodynamic exposure on carbon storage and nutrient retention in eelgrass (Zostera marina L) meadows on the Swedish Skagerrak coast. Sci. Rep. 10:13666. doi: 10.1038/s41598-020-70403-5
Derksen, C., Burgess, D., Duguay, C., Howell, S., Mudryk, L., and Smith, S. (2019). “Changes in snow, ice, and permafrost across Canada,” in Canada’s Changing Climate Report, eds E. Bush and D. S. Lemmen (Canada: Government of Canada).
Dijkstra, J. A., Harris, L. G., Mello, K., Litterer, A., Wells, C., and Ware, C. (2017). Invasive seaweeds transform habitat structure and increase biodiversity of associated species. J. Ecol. 105, 1668–1678.
Dormann, C., McPherson, J., Araújo, M. B., Bivand, R., Bolliger, J., Carl, G., et al. (2007). Methods to account for spatial autocorrelation in the analysis of species distributional data: a review. Ecography 30, 609–628.
Duarte, C. M. (1992). Nutrient concentration of aquatic plants: patterns across species. Limnol. Oceanogr. 37, 882–889.
Duarte, C. M. (2017). Reviews and syntheses: hidden forests, the role of vegetated coastal habitats in the ocean carbon budget. Biogeosciences 14, 301–310.
Duarte, C. M., and Cebrián, J. (1996). The fate of marine autotrophic production. Limnol. Oceanogr. 41, 1758–1766. doi: 10.4319/lo.1996.41.8.1758
Duarte, C. M., and Krause-Jensen, D. (2017). Export from seagrass meadows contributes to marine carbon sequestration. Front. Mar. Sci. 4:13. doi: 10.1016/j.scitotenv.2020.140185
Duarte, C. M., Lenton, T. M., Wadhams, P., and Wassmann, P. (2012). Abrupt climate change in the Arctic. Nat. Clim. Chang. 2, 60–62.
Elith, J., Leathwick, J. R., and Hastie, T. (2008). A working guide to boosted regression trees. J. Anim. Ecol. 77, 802–813. doi: 10.1111/j.1365-2656.2008.01390.x
Filbee-Dexter, K., Wernberg, T., and Grace, S. P. (2020). Marine heatwaves and the collapse of marginal North Atlantic kelp forests. Sci. Rep. 10:13388. doi: 10.1038/s41598-020-70273-x
Filbee-Dexter, K., Wernberg, T., Fredriksen, S., Norderhaug, K. M., and Pedersen, M. F. (2019). Arctic kelp forests: diversity, resilience and future. Glob. Planetary Change 172, 1–14. doi: 10.1016/j.gloplacha.2018.09.005
Flato, G., Gillett, N., Arora, V., Cannon, A., and Anstey, J. (2019). “Modelling future climate change,” in Canada’s Changing Climate Report, Chap. 3, eds E. Bush and D. S. Lemmen (Ottawa: Government of Canada), 74–111.
Fossheim, M., Primicerio, R., Johannesen, E., Ingvaldsen, R. B., Aschan, M. M., and Dolgov, A. V. (2015). Recent warming leads to a rapid borealization of fish communities in the Arctic. Nat. Clim. Change 5, 1–6.
Fragkopoulou, E., Serrão, E. A., De Clerck, O., Costello, M. J., Araújo, M. B., Duarte, C. M., et al. (2022). Global biodiversity patterns of marine forests of brown macroalgae. Glob. Ecol. Biogeogr. 1–13. doi: 10.1111/geb.13450
Fragkopoulou, E., Serrão, E. A., Horta, P. A., Koerich, G., and Assis, J. (2021). Bottom trawling threatens future climate refugia of rhodoliths globally. Front. Mar. Sci. 7:594537.
Gagaev, S. Y., Grebelny, S. D., Sirenko, B. I., Potin, V. V., and Savinkin, O. V. (2019). Benthic habitats in the Tikhaya Bight (the Hooker Island, Franz Josef Land). Proc. Zool. Institute Russian Acad. Sci. 323, 3–15. doi: 10.31610/trudyzin/2019.323.1.3
Ganter, B. (2000). Seagrass (Zostera spp.) as food for brent geese (Branta bernicla): an overview. Helgoland Mar. Res. 54, 63–70.
Gattuso, J. P., Gentili, B., Antoine, D., and Doxaran, D. (2020). Global distribution of photosynthetically available radiation on the seafloor. Earth Syst. Sci. Data 12, 1697–1709.
GEBCO (2019). General Bathymetric Chart of the Oceans B. O. D. Centre, Ed. Liverpool: British Oceanographic Data Centre.
Gilson, A. R., Smale, D. A., and O’Connor, N. (2021). Ocean warming and species range shifts affect rates of ecosystem functioning by altering consumer–resource interactions. Ecology 102:e03341. doi: 10.1002/ecy.3341
Goldsmit, J., Schlegel, R. W., Filbee-Dexter, K., MacGregor, K. A., Johnson, L. E., Mundy, C. J., et al. (2021). Kelp in the Eastern Canadian arctic: current and future predictions of habitat suitability and cover. Front. Mar. Sci. 8:1453.
Gotceitas, V., Fraser, S., and Brown, J. A. (1995). Habitat use by juvenile Atlantic cod (Gadus morhua) in the presence of an actively foraging and non-foraging predator. Mar. Biol. 123, 421–430.
Gouvêa, L. P., Assis, J., Gurgel, C. F. D., Serrão, E. A., Silveira, T. C. L., Santos, R., et al. (2020). Golden carbon of Sargassum forests revealed as an opportunity for climate change mitigation. Sci. Total Environ. 729:138745.
Handorf, D., and Dethloff, K. (2012). How well do state-of-the-art atmosphere-ocean general circulation models reproduce atmospheric teleconnection patterns? Dynamic Meteorol. Oceanogr. 64:19777.
Harris, P. M., Neff, A. D., Johnson, S. W., and Thedinga, J. F. (2008). Eelgrass Habitat and Faunal Assemblages in the City and Borough of Juneau, Alaska. Washington, DC: NOAA.
Hugonnet, R., McNabb, R., and Berthier, E. (2021). Accelerated global glacier mass loss in the early twenty-first century. Nature 592, 726–731. doi: 10.1038/s41586-021-03436-z
IPCC (2021). Climate Change 2021. The Physical Science Basis. Contribution of Working Group I to the Sixth Assessment Report of the Intergovernmental Panel on Climate Change. Cambridge: Cambridge University Press.
Jayathilake, D. R. M., and Costello, M. J. (2020). A modelled global distribution of the kelp biome. Biol. Conserv. 252:108815.
Jayathilake, D. R. M., and Costello, M. J. (2021). Version 2 of the world map of laminarian kelp benefits from more Arctic data and makes it the largest marine biome. Biol. Conserv. 257:109099.
Jiménez-Valverde, A., and Lobo, J. M. (2007). Threshold criteria for conversion of probability of species presence to either-or presence-absence. Acta Oecol. 31, 361–369.
Jueterbock, A., Tyberghein, L., Verbruggen, H., Coyer, J. A., Olsen, J. L., and Hoarau, G. (2013). Climate change impact on seaweed meadow distribution in the North Atlantic rocky intertidal. Ecol. Evol. 3, 1356–1373. doi: 10.1002/ece3.541
Krause-Jensen, D., and Duarte, C. M. (2014). Expansion of vegetated coastal ecosystems in the future Arctic. Front. Mar. Sci. 1:77. doi: 10.1111/gcb.14279
Krause-Jensen, D., and Duarte, C. M. (2016). Substantial role of macroalgae in marine carbon sequestration. Nat. Geosci. 9, 737–742.
Krause-Jensen, D., Archambault, P., Assis, J., Bartsch, I., Bischof, K., Filbee-dexter, K., et al. (2020). Imprint of climate change on Pan-Arctic marine vegetation. Front. Mar. Sci. 7:617324.
Krause-Jensen, D., Marbà, N., Olesen, B., Sejr, M. K., Christensen, P. B., Rodrigues, J., et al. (2012). Seasonal sea ice cover as principal driver of spatial and temporal variation in depth extension and annual production of kelp in Greenland. Glob. Chang. Biol. 18, 2981–2994. doi: 10.1111/j.1365-2486.2012.02765.x
Krause-Jensen, D., Marbà, N., Sanz-Martin, M., Hendriks, I. E., Thyrring, J., Carstensen, J., et al. (2016). Long photoperiods sustain high pH in arctic kelp forests. Sci. Adv. 2:e1501938. doi: 10.1126/sciadv.1501938
Krause-Jensen, D., Sejr, M. K., Bruhn, A., Rasmussen, M. B., Christensen, P. B., Hansen, J. L. S., et al. (2019). Deep penetration of kelps offshore along the west coast of Greenland. Front. Mar. Sci. 6:375.
Lalumière, R., Messier, D., Fournier, J. J., and Peter McRoy, C. (1994). Eelgrass meadows in a low arctic environment, the northeast coast of James Bay. Québec. Aquatic Bot. 47, 303–315.
Lantuit, H., Overduin, P. P., Couture, N., Wetterich, S., Aré, F., Atkinson, D., et al. (2012). The arctic coastal dynamics database: a new classification scheme and statistics on arctic permafrost coastlines. Estuar. Coasts 35, 383–400.
Martins, M. R., Assis, J., and Abecasis, D. (2021). Biologically meaningful distribution models highlight the benefits of the Paris agreement for demersal fishing targets in the North Atlantic Ocean. Glob. Ecol. Biogeogr. 30, 1643–1656.
Marbà, N., Krause-Jensen, D., Masqué, P., and Duarte, C. M. (2018). Expanding Greenland seagrass meadows contribute new sediment carbon sinks. Sci. Rep. 8:14024. doi: 10.1038/s41598-018-32249-w
Melo-Merino, S. M., Reyes-Bonilla, H., and Lira-Noriega, A. (2020). Ecological niche models and species distribution models in marine environments: a literature review and spatial analysis of evidence. Ecol. Model. 415:108837. doi: 10.1016/j.envpol.2018.10.032
Mudryk, L. R., Derksen, C., Howell, S., Laliberté, F., Thackeray, C., and Sospedra-Alfonso, R. (2018). Canadian snow and sea ice: historical trends and projections. Cryosphere 12, 1157–1176.
Müller, R., Laepple, T., Bartsch, I., Wiencke, C., Müller, R., Laepple, T., et al. (2009). Impact of oceanic warming on the distribution of seaweeds in polar and cold-temperate waters. Bot. Mar. 52, 617–638.
Olesen, B., Krause-Jensen, D., Marbà, N., and Christensen, P. B. (2015). Eelgrass Zostera marina in subarctic Greenland: dense meadows with slow biomass turnover in cold waters. Mar. Ecol. Progr. Ser. 518, 107–121.
Ortega, A., Geraldi, N. R., Alam, I., Kamau, A. A., Acinas, S. G., Logares, R., et al. (2019). Important contribution of macroalgae to oceanic carbon sequestration. Nat. Geosci. 12, 748–754. doi: 10.1016/j.biortech.2016.03.060
Pessarrodona, A., Filbee-Dexter, K., Alcoverro, T., Boada, J., Feehan, C. J., Fredriksen, S., et al. (2021). Homogenization and miniaturization of habitat structure in temperate marine forests. Glob. Change Biol. 2720, 5262–5275. doi: 10.1111/gcb.15759
Poloczanska, E. S., Brown, C. J., Sydeman, W. J., Kiessling, W., Schoeman, D. S., Moore, P. J., et al. (2013). Global imprint of climate change on marine life. Nat. Clim. Change 3, 919–925.
Riahi, K., Rao, S., Krey, V., Cho, C., Chirkov, V., Fischer, G., et al. (2011). RCP 8.5-A scenario of comparatively high greenhouse gas emissions. Clim. Change 109, 33–57.
Spalding, M. D., Fox, H. E., Allen, G. R., Davidson, N., Finlayson, M. A. X., Halpern, B. S., et al. (2007). Marine ecoregions of the world: a bioregionalization of coastal and shelf areas. Bioscience 57, 573–583. doi: 10.1111/cla.12453
Spurkland, T., and Iken, K. (2011). Salinity and irradiance effects on growth and maximum photosynthetic quantum yield in subarctic Saccharina latissima (Laminariales, Laminariaceae). Bot. Mar. 54, 355–365.
Starko, S., Wilkinson, P. D., and Bringloe, T. T. (2021). Recent global model underestimates the true extent of Arctic kelp habitat. Biol. Conserv. 257:109082.
Stekoll, M. S. (2019). The seaweed resources of Alaska. Bot. Mar. 62, 227–235. doi: 10.1515/bot-2018-0064
Stroeve, J. C., Kattsov, V., Barrett, A., Serreze, M., Pavlova, T., Holland, M., et al. (2012). Trends in Arctic sea ice extent from CMIP5, CMIP3 and observations. Geophys. Res. Lett. 39:L16502.
Teagle, H., Hawkins, S. J., Moore, P. J., and Smale, D. A. (2017). The role of kelp species as biogenic habitat formers in coastal marine ecosystems. J. Exp. Mar. Biol. Ecol. 492, 81–98.
United Nations Framework Convention on Climate Change (2015). Paris Agreement to the United Nations Framework Convention on Climate Change, Dec. 12, 2015, T.I.A.S. No. 16-1104.
van Vuuren, D. P., Edmonds, J., Kainuma, M., Riahi, K., Thomson, A., Hibbard, K., et al. (2011). The representative concentration pathways: an overview. Clim. Change 109:5.
Vergés, A., Doropoulos, C., Malcolm, H. A., Skye, M., Garcia-Pizá, M., Marzinelli, E. M., et al. (2016). Long-term empirical evidence of ocean warming leading to tropicalization of fish communities, increased herbivory, and loss of kelp. Proc. Natl. Acad. Sci. U.S.A. 113, 13791–13796. doi: 10.1073/pnas.1610725113
Vilas, D., Coll, M., Pedersen, T., Corrales, X., Filbee-Dexter, K., Pedersen, M. F., et al. (2020). Kelp-carbon uptake by Arctic deep-sea food webs plays a noticeable role in maintaining ecosystem structural and functional traits. J. Mar. Syst. 203:103268.
Wang, F., Tokarska, K. B., Zhang, J., Ge, Q., Hao, Z., Zhang, X., et al. (2018). Climate warming in response to emission reductions consistent with the Paris agreement. Adv. Meteorol. 2018, 1–9.
Weslawski, J. M., Wiktor, J., and Kotwicki, L. (2010). Increase in biodiversity in the arctic rocky littoral, Sorkappland, Svalbard, after 20 years of climate warming. Mar. Biodiver. 40, 123–130. doi: 10.1007/s12526-010-0038-z
Wilson, K. L., and Lotze, H. K. (2019). Climate change projections reveal range shifts of eelgrass Zostera marina in the Northwest Atlantic. Mar. Ecol. Progr. Ser. 620, 47–62. doi: 10.3354/meps12973
Young, A. P., and Carilli, J. E. (2019). Global distribution of coastal cliffs. Earth Surf. Process. Landf. 44, 1309–1316. doi: 10.1002/esp.4574
Keywords: Arctic, marine forests, macrophytes, climate change, Paris Agreement, range shifts
Citation: Assis J, Serrão EA, Duarte CM, Fragkopoulou E and Krause-Jensen D (2022) Major Expansion of Marine Forests in a Warmer Arctic. Front. Mar. Sci. 9:850368. doi: 10.3389/fmars.2022.850368
Received: 07 January 2022; Accepted: 07 February 2022;
Published: 01 March 2022.
Edited by:
Mariana Mayer Pinto, University of New South Wales, AustraliaReviewed by:
Beth Crase, Charles Darwin University, AustraliaJan Marcin Weslawski, Institute of Oceanology (PAN), Poland
Copyright © 2022 Assis, Serrão, Duarte, Fragkopoulou and Krause-Jensen. This is an open-access article distributed under the terms of the Creative Commons Attribution License (CC BY). The use, distribution or reproduction in other forums is permitted, provided the original author(s) and the copyright owner(s) are credited and that the original publication in this journal is cited, in accordance with accepted academic practice. No use, distribution or reproduction is permitted which does not comply with these terms.
*Correspondence: Jorge Assis, am9yZ2VtZmFAZ21haWwuY29t; Ester A. Serrão, ZXNlcnJhb0B1YWxnLnB0