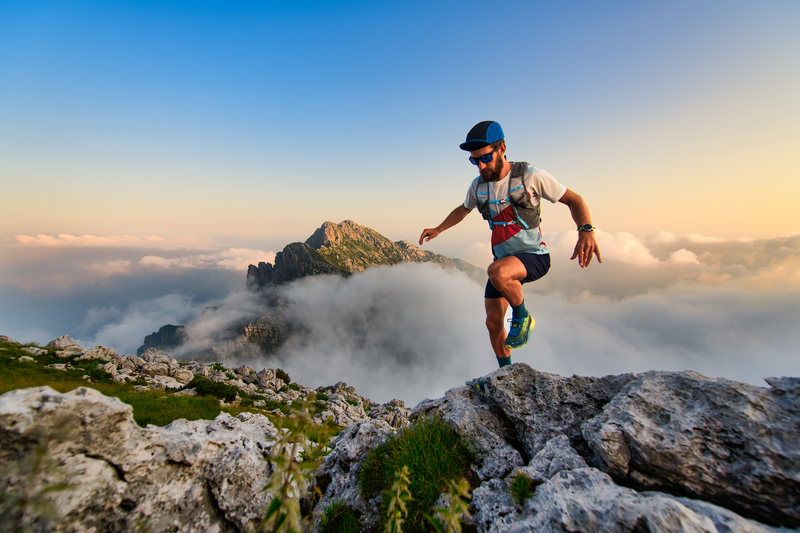
94% of researchers rate our articles as excellent or good
Learn more about the work of our research integrity team to safeguard the quality of each article we publish.
Find out more
ORIGINAL RESEARCH article
Front. Mar. Sci. , 28 March 2022
Sec. Marine Molecular Biology and Ecology
Volume 9 - 2022 | https://doi.org/10.3389/fmars.2022.841806
Caged salmon farming is increasingly undertaken in water bodies with strong hydrodynamics where hard and mixed substrate habitats are more prevalent. Yet, these structurally complex and heterogeneous habitats support diverse benthic communities including several cnidarians and sponges that remain poorly characterized. This study used a combination of respirometry measurements, gas chromatography and 16S rRNA metabarcoding to define the respiration rate, stable carbon (δ13C) and nitrogen isotopes (δ15N), fatty acid (FA) and microbial profiles, and assess the impact of salmon farming on four important epibenthic suspension-feeders along the western Norwegian coast: the sponges Craniella and Weberella, the soft coral Duva florida and the anemone Hormathia digitata. Our results showed striking differences in fatty acid profiles and host microbiome communities in terms of identity, functional capabilities and genetic properties across the suspension-feeders. We found evidence of increased mortality rate in specimens located near fish farm activities and of a species-specific effect on respiration rate, with D. florida showing increased activity under the farm. Effects of fish farming on the suspension feeders were also species-specific and particularly evidenced by functional microbial turnover and by alteration of overall FA profiles in the soft coral and sea anemone. In particular, D. florida showed reduced level of FAs close to the farm (0-350 m), with significant difference in composition along a distance gradient. Only H. digitata showed evidence of incorporation of organic material from the fish farm waste via fatty acids trophic markers (FATM) and stable isotope analysis. Overall, our study demonstrates that suspension feeders have taxon-specific sensitivity towards the effect of salmon farming, and identified several potential molecular indicators that could be used as surrogate of impact gradient upon further research and validation. It also provides a wealth of ecological and physiological information on some of the most common sessile epibenthic organisms within Arctic and sub-Arctic regions, enabling us to better understand their response and evaluate their resilience to environmental changes.
Worldwide salmon farming has increased substantially over the last decade and is projected to continue expanding substantially in the next 20 years (FAO, 2018). In countries with high coastal production density such as Norway, this growth, along with the search for optimal rearing settings, has led to the establishment of newer production sites in increasingly exposed environments (Holmer, 2010; Lekang et al., 2016; Lader et al., 2017).
Characterized by complex landscape, bathymetry and seafloor texture, these intricate habitats commonly support high, yet poorly characterized biodiversity (Sebens, 1991; Buhl-Mortensen et al., 2012; Dunlop et al., 2020). Such biodiversity is typically represented by sessile and hard-substrate dependent organisms such as sponges, bryozoans and corals. These animals are particularly vulnerable to environmental disturbances, including sedimentation and smothering, and have shown to be sensitive to salmon aquaculture activities (Sutherland et al., 2018). These taxa play an essential role in ecosystem functioning due to their ability to transfer energy between the benthic and pelagic compartments, and in providing habitats for a multitude of other organisms (Klitgaard, 1995; Gili and Coma, 1998; Bell, 2008; Maldonado et al., 2015). As such, they represent fitting candidates for evaluating the biological effects of salmonid aquaculture on benthic macro-organisms in exposed environments.
Mixed and hard substrates habitats are particularly challenging to monitor with traditional techniques such as sediment grabs due to the absence or sporadic distribution of soft sediment, the obstruction of the closing mechanisms by rocks or sideway landings of the grab on uneven seafloor (Dunlop et al., 2020). Video-surveys have been used as an alternative to monitor benthic habitats characterized by mix and hard substrates but typically require extensive observations and their effectiveness is considerably reduced over complex substrates where macro-benthic fauna distribution is patchy (Hamoutene et al., 2016; Hamoutene et al., 2018). Other alternatives include suction devices that could enable the characterization of physico-chemical properties and microbial community changes by collecting and analyzing particulate matter deposited on the seafloor. While preliminary results are encouraging (e.g., Keeley et al., 2021), this methodology would still not provide information as to how benthic macro-fauna are being affected by the environmental changes associated with salmon farming (e.g., organic enrichment, smothering, biocides from biofouling and parasite treatments). Awareness of the biological responses of these organisms is fundamental to the development of early warning indicators to avert substantial and irreparable damages to the ecosystem.
DNA metabarcoding and high-throughput sequencing represent powerful tools to rapidly and cost-effectively characterize microbial communities, including host-microbiomes (Yang et al., 2019). Microbiome turnovers are known to follow or prompt physiological alterations and pathological conditions in sponges and corals (Ribes et al., 2016; Ziegler et al., 2016; Gissi et al., 2019; Yang et al., 2019; Ziegler et al., 2019). Knowing how host-microbiomes are changing along an impact gradient could be helpful in evaluating the health of the hosts, better understand how they are being affected, and to identify stress indicators that could be incorporated into monitoring programs (Schirmer et al., 2010; Clerissi et al., 2020; Dittami et al., 2021).
Other common methods used to track aquaculture wastes in marine systems and with potential application on benthic sessile taxa include fatty acids (FAs) and stable isotopes (SIs) turnovers. Aquaculture feeds are mostly made of terrestrial plants and consequently, typically contain high levels of terrestrial fatty acids (FAs) such as oleic acid 18:1 (n-9), linoleic acid 18:2 (n-6) and a-linolenic acid 18:3 (n-3)), and display a stable isotope signature depleted in δ13C while enriched in δ15N compared to marine organic compounds (Sarà et al., 2006; Yokoyama et al., 2006; Fernandez-Jover et al., 2011; Woodcock et al., 2017a; White et al., 2019; Woodcock et al., 2019; Grego et al., 2020; Sun et al., 2020). By feasting on aquaculture pellets and wastes, marine animals in close proximity to salmon farms may therefore exhibit altered FA and SI profiles that could be used to monitor the impact gradient of fish farming.
Based on these grounds, we aimed to assess if, and potentially how, these changes are taking place in four sessile benthic organisms commonly found living on mixed and hard-substrate habitats of the Norwegian coast, namely the anemone Hormathia digitata, the soft coral Duva florida, and the sponges Craniella and Weberella (Dunlop et al., 2020). Several specimens from each taxon were translocated along a distance gradient from a salmon farm located in the Sognefjord region (mid-west coast of Norway), and exposed for a period of seven months prior to being retrieved and thoroughly analyzed. Specifically, specimens’ health and metabolism were assessed visually and through respirometry measurements, their epithelial microbiome characterized via 16S rRNA metabarcoding, their FA profile assessed via gas chromatography, and their carbon and nitrogen SI profile measured via mass spectrometry. We hypothesized that, for each of the benthic taxa, 1) specimens living further from the farm would present healthier physiology, 2) have a microbiome, FA and SI profile that significantly differs from those living in close proximity to the farm, and 3) that responses between benthic taxa would be substantially different due to their inherent specific physiology, ecology and reliance on their microbiome.
A translocation experiment was set up to exploit the organic enrichment gradient created by elevated levels of organic waste being discharged from a fully functioning high capacity salmon (Salmo salar) farm in a fjord in northern Norway (Figure 1). This study utilises samples from seven grow-out moorings positioned along a transect running parallel to the shoreline (i.e. along an isobath) in the prevailing down-current (ENE) direction: 0mA and 0mB (both beside cages), 110m, 360m and 605m (from cages) and at two reference stations: Ref1 and Ref2 (1,500m and 2,080m distant, respectively). Average near-bottom current speeds were 0.086 m/s (Dunlop et al., 2021). The seabed beneath the moorings was variably slopping and comprised of mixed- and hard-bottom habitats (including the culture species) interspersed with soft-sediment patches (Dunlop et al., 2021; Keeley et al., 2021).
Figure 1 Study site in Vargsundet, northern Norway (A) with arrangement of the seven translocation rigs in relation to the fish farm (B), and below, example photos of the four selected species, taken before being transferred to the grow-out frames.
Four sessile benthic epifauna species from the Vargsundet region in Northern Norway were selected for controlled in-situ exposure to farm waste and subsequent tests and detailed tissue analyses. Criteria for selection included: having a variety of percieved sesitivities to sedimentation and organic enrichment pressures, being relatively ubiquitous on hard substrates within the region, the selection having some contrasting physiological forms and feeding mechanisms, and finaly being sufficiently numerous and physically sampleable (by ROV) in the collection zone. The selected species were: two species of sponges (Craniella and Weberella), the common red anemone (Hormathia digitata) and soft coral (Duva florida) (Figure 1).
Specimens of the four target species were collected from a nearby reef approximately 2,800m from the farm in comparable depths (~80m) to the translocation sites (Figure 1) using an ROV with a specially designed flexible (soft grab) surface-controlled pincer arm. ‘Loose’ or ‘semi-loose’ specimens were selected and then placed in a reservoir in the ROV before being brought to the surface and transferred directly to an on-board holding tank. In order to avoid having to forcefully rip them from the substrate and creating an unkown amount of tissue damage and stress, the selected specimens were either lossely attached to loose substrates and therefore easily removed (Craniella), or attached to small rocks on soft sediment (mostly Weberella and D. florida), or in the case of H. digitata, attached to the shells of a mobile gastropod species. Once on board, the specimens were individually weighed, photographed and tagged before being transferred to a grow-out frame and attached while immersed in water to avoid exposure to air (i.e. transferred in buckets). The grow-out frame constituted four cicrular mesh discs (400 mm diameter) set 350 mm appart using precut polyethylene pipe on a central pillar (a threaded stainless steel rod), similar to those used as ‘lantern nets’ for bivalve culture. For this purpose, the outer side netting (or ‘sock’) was removed to ensure the culture surface was fully exposed to the sites hydrodynamics, setson and oxygen supply and biodeposition events, akin to what they would encounter on the seabed. The grow-out frames were deployed close to the seabed (ca. 2 – 3 m above bottom) on mooring lines along a comparable depth band. The fauna were cultured at the translocated positions for 7 months, from mid-March (15-18/03/2018) until retrieval in mid-October (15-23/10/2018).
The responses of the fauna to the translocation experiments were assessed from a variety of approaches. Firstly, a simple mortality rate for each species was calculated based on the number of intact ‘live’ specimens that were recovered when the frames were retrieved at the conclusion of the trial. Specimens were only considered dead if they had dissappeared altogether (assemmed decayed and fallen off) or only a residue was left and it was clearly expired. Secondly the respiratory rate of each individual was determined based on oxygen uptake. Finally the fauna were culled and dissected radially under semi-sterile conditions (new gloves and clean cutting board and scalpel between individuals) to provide approximately 10 g of tissue from a cross-section (segment) of the animal, which was then placed in pre-labelled Eppendorf tubes and snap frozen in liquid nitrogen. The sub-samples then underwent specialist analysis to assess the 1. microbiome, 2. fatty acid composition, and 3. carbon and nitrogen content (including stable isotope ratios δ15N and δ13C).
Upon retrieval, each fauna specimen was first carefully removed from the grow-out frame (under water) and transferred to flow-through holding tanks where they were left stationary and out of direct sunlight for a minimum of 12 hours before being carefully transferred to an appropriately sized respirometry core. Each core was given individual flow lines to allow the water to be gently exchanged while the specimen acclimatised to the new environment and recovered from the displacement. In the cases of the anenome and the soft coral, this was indicated by the opening out and extending of the animal, as both of these species can retrract to a small ball upon disturbance, during which time they are presumably dormant and not respiring at normal capacity. The water line to the core was then removed and an airtight lid was placed in to the top of the core that contained a digital oxygen sensor and a micropump which was initiated to keep the water moving to maintain mixing and prevent chemo-stratification. The oxygen concentration in the chamber was recorded using an 8-channel PresSense OXY-8 SMA (G3) fiber optic oxygen transmitter (with St10-YOP oxygen dipping probes and a Pt-100 temperartue probe) and the experiment was run until a consistent oxygen decay rate was observed and the concentration had been appreciably depleted. The experiments were concluded before the concentration got below 80% and restarted again if the animal visually closed down (e.g., if the anenome or soft coral retracted). Each fauna was subjected to two sequential trials, with the water in the core being refreshed inbetween. After the specimen was removed for dissection, the volume of the core was measured.
Oxygen consumption rates were calculated by initally plotting the oxygen timeseries and identifying the earliest stable and consistent section of the curve. This region was then randomly subsampled to obtain multiple meaurements of slope which was then concverted to mean oxygen consumption based on the seawater volume of the respective incubation cores.
Frozen tissue samples (-80°C) were ground and homogeneized in liquid nitrogen with a sterilized mortar and pestle soaked in 50% of commercial bleach solution (0.564 mol/L) for a minimum of 5 minutes and rinsed with double-distilled water (ddH2O) between each sample. For each sample, approximately 25 mg of powdered frozen tissue was lysed with the QIAshredder kit (QIAGEN, California, USA), and DNA/RNA co-extracted using the AllPrep DNA/RNA mini kit (QIAGEN, California, USA) following the manufacturer’s instruction. To assess potential contamination, an extraction blank filled with ddH2O was included for each batch of extraction.
To ensure the correct taxonomic identification of the collected benthic specimens, few individuals from each group was Sanger sequenced. Details of the methodology and results are provided in supplementary file 1 (Tables S1-S2 and Figures S1-S5).
DNA extracts were set at equimolar concentration (10 ng/μL) with 10 mM Tris for a total volume of 20 μL per sample and shipped on dry ice to the Norwegian Sequencing Center (NSC, Oslo, Norway) for 16S rRNA library preparation following the Fadrosh et al. (2014) protocol. Briefly, fusion primers that contained illumina overhang adaptors, a 12 base-pair (bp) sample index, a 0 to 7 bp heterogeneity spacer [to mitigate the issue of low diversity samples (Krueger et al., 2011)], and the forward 319F: 5’-ACT CCT ACG GGA GGC AGC AG’ or reverse 806R: 5’-GGA CTA CHV GGG TWT CTA AT-3’ 16S rRNA universal primers targeting the V3-V4 region (~469 bp) were used for polymerase chain reaction amplification (PCR). Amplicons were purified and normalised using the SequalPrep™ kit (Life Technologies, California, USA), pooled together and paired-end sequenced (2x300 bp) with the Illumina MiSeq platform (California, USA). To assess potential contamination, a PCR blank (ddH2O) was added during library preparation. Sequences are available from the NCBI Sequence Read Archive under project number PRJNA694725.
Fastq files were demultiplexed and primers trimmed using cutadapt (version 2.6; Martin, 2011), allowing no insertion or deletion, and requiring a minimum overlap of 15 bp. Sequences were then quality filtered and denoised with the default settings of the DADA2 R program (version 1.14; Callahan et al., 2016). The forward and reverse reads were merged using the default settings and a perfect minimum overlap of 10 bp. Chimera filtering was done using the consensus method where sequences found to be chimeric in most samples (default value = 90%) are discarded. Remaining sequences were taxonomically assigned using the RDP Naïve Bayesian Classifier algorithm (Wang et al., 2007) trained on the SILVA 16S rRNA database (version 132 clustered at 99% similarity). Non-bacterial sequences and those assigned to chloroplast were removed from the dataset. Additionally, only sequences with a minimum of 10 reads were kept for downstream analysis to remove potentially spurious reads. Sampling depth per sample was visualized with the ‘rarecurve’ function of the ‘vegan’ R package (version 2.5.6; Oksanen et al., 2019). Samples with less than 1,000 reads were discarded. The resulting samples and sequences were used for functional (Enzyme Commission numbers (ECs) and pathways) and genetic properties (i.e. mean genome size, mean G/C content, mean genome plasticity) profiling using the PAPRICA program (version 0.5.2; Bowman and Ducklow, 2015) with the default parameters. In addition, functional profiling was also performed with the TAX4FUN2 software (version 1.1.4; Wemheuer et al., 2020) and the KEGG Orthologs (KOs) database to cover pathways not present in the EC database.
A homogenized tissue sub-sample of each specimen was dried at 60°C for 24 hours and weighed out into a tin capsule (1.28 ± 0.15 mg). All samples were sent to the Stable Isotope Facility of the University of California (Davis Campus, California, USA). Samples were analyzed for δ15N and δ13C using a PDZ Europa 20-20 isotope ratio mass spectrometer (Sercon). Stable isotope ratios were expressed in delta notation per the following formula:
where X is the heavy isotope, Rsample is the ratio of heavy to light isotope in the sample, and Rstandard is the ratio of heavy to light isotope in the reference standard. The mean SD for reference materials replicates in this project is 0.06 ‰ for both δ15N and δ13C. In addition to giving the ratio of the two stable isotopes (δ15N and δ13C), the analysis also provides the total amount of carbon and nitrogen as percent of dry weight.
A sample of each specimen (1-2 g) was homogenized by scalpel and a subsample (50-150 mg) weighed in a 16 ml glass tube for fatty acids analysis. Additional subsamples of 1.5-2 g were weighed on aluminum dishes for measuring water and ash content. To enable quantification of the lipids relative to ash free dry weight (total sample weight – water – ash), grounded tissue material was dried over night at 110°C for determination of water content, thereafter the samples were burned at 450°C for 6 h to determine the ash weight. The lipids were thereafter quantified relative to ash free dry weight (total sample weight – water – ash).
All samples were methylated and the respective fatty acid methyl esters (FAME) were analysed on a HP-7890A gas chromatograph (Agilent, USA) with a flame ionization detector (GC-FID) according to a method described in (Meier et al., 2006) with the fatty acid 19:0 added as an internal standard. 2.5 M dry HCl in methanol was used as a methylation reagent. The FAMEs were extracted using 2×2 ml of hexane. The combined extract was diluted or concentrated to obtain a suitable concentration for gas chromatography before 1 µl was injected (splitless) on the GC.
The four different animals analyzed in this study have very different lipid composition and this demanded several individual modifications of the standard methods to analyze the fatty acids and fatty alcohols (FAOH) composition. Modifications applied to each taxon are summarized in Table 1 and described in detail in the text below.
As the sea anemone samples contain large amount of wax esters, the FAME and the fatty alcohols (FAOH) were fractionated on solid phase column (500 mg aminopropyl-SPE, Supelco). The FAME and FAOH fractions were analyzed separately on GC-FID to avoid co-elution. The sea anemone samples were first methylated and the resulting hexane extracts from the direct metanolysis were added nonadecanol (19:0 alcohol) as internal standard for the FAOHs. The hexane extract was loaded on to the SPE column and the FAME fraction was eluted with 3 ml hexane + 2 ml hexane:ethyl acetate (9:1 v/v) and the fatty alcohols were eluted with 4 ml chloroform. The two fractions were evaporated to dryness and dissolved in hexane before GC-FID analysis.
The soft coral contains large amount hydrocarbon (HC) which co-elute with FAME. To remove this problem, a saponification was performed after the first metanolysis to remove the HC, with back extraction of FFA followed by an additional metanolysis. The hexane extract after the first metanolysis were transferred to a 16 ml reaction tube, and the hexane were evaporated to dryness with N2 (g) and added 1 ml of 1 M KOH (aq). The reaction tube was heated at 90°C for 1 hour and after cooling, 2,5 ml H2O added prior to extraction of the HC with 2x2ml isooctane. The water fraction containing free fatty acids (FFA) were acidified by adding 2 ml 2 M HCl (aq) and the FA were extracted by 2x2 ml isooctane. The organic fraction was transferred to a new 16 ml reaction tube and evaporated to dryness with dryness with N2 (g) and the FFAs were methylated into FAMEs following the procedure afore mentioned.
The FAME/FAOH extracts from Weberella, Hormathia digitata and Duva florida were analyzed using a CP-Wax 52 CB column (25 m × 0.25 mm fused silica capillary, coated with polyethylene-glycol of 0.25 μm film thickness, Varian-Chrompack, Middelburg, Netherlands). The oven temperature was programmed to hold at 90°C for 2 minutes, then from 90°C to 150°C at 30°C/min and then to 225°C at 2.5°C/min and held there for 35 minutes, for a total analysis time of 75 min. Helium (99.9999%) was used as mobile phase at 1 mL/min for 45 min, followed by a flow increase to 3 mL/min which was held for 30 min. Injector and detector temperatures were 270°C and 300°C, respectively.
The FAME extract from Craniella were analysed on Agilent 122-5062 DB-5 (60 m x 0.25 mm x 0.25 μm) with helium as the carrier gas at a constant flow rate of 1 mL/min. The oven temperature was held at 60°C and ramped to 130°C at a rate of 60°C/min, then ramped to 325°C at 1°C/min rate and held at this temperature for 7 min. Injection and detection conditions were as described above. The chromatograms were integrated using the EZChrom Elite software (Agilent Technologies). Identification of FAME and other lipids were conducted by comparing retention times with a FAME standard (GLC-463 from Nu-Chek Prep. Elysian, MN, USA) performed under similar chromatographic conditions as the GC-FID (Wasta and Mjøs, 2013). Gas chromatography–mass spectrometry analysis of all samples was conducted using electron impact ionization (Agilent Technologies, 6890N/5975B GC–MS system, Santa Clara, CA, USA). A table with identification of FAME and other lipid from the GC-MS analysis is provided in supplementary file 2. The retention index maps and mass spectral libraries can be found at (GC-MS; http://www.chrombox.org/data).
The position of the double bounds of the non-methylene interrupted fatty acids (NMI-FAs) are not provided, since reference compounds are not available and the amounts were too small to apply special derivatization methods for structure elucidation (like picolinyl ester or dimethyl disulfide derivatives) that could confirm the positions. Letters (a/b) are applied to denote different NMI isomers. However, it is known that the dominating unsaturation of NMI-FA for Demospongiae are found in the Δ5,9 position, and it is likely that the two dominating NMI-FA in Weberella are 26:2 NMI (a) = 26:2 (Δ5,9) and 26:3 NMI (b) = 26:3 (Δ5,9,19) (Hahn et al., 1988; Kornprobst and Barnathan, 2010; de Kluijver et al., 2021).
In Craniella, the dominating NMI-PUFA were a branched C27. As several isomers are possible, we were not able from our analysis to estimate the double bound or branched position (de Kluijver et al., 2021). The position of the methyl-group of the branched saturated FAs in Craniella were obtained using retention index (equivalent chain length, ECL values) from Kubinec et al. (2011).
Taxonomic and functional microbial richness, and mean microbial genetic properties [i.e. mean and standard deviation of 16S rRNA sequences, number of genes, number of coding sequences (CDS), genome size, GC content and genome plasticity (phi) per taxa] were visualized and compared between benthic species using box plots and the ‘ggplot2’ R package (version 3.3; Wickham, 2016), and tested with analyses of variance (ANOVA; design: 4th root of distance from cage * benthic species) using the ‘stats’ function implemented in base R. In addition, values for sediment and floc (particulate organic matter deposited on hard mixed and hard substrates) samples collected along the same transect in the same year of the experiment (see Keeley et al., 2021) were added to enable comparison with the surrounding environment. The proportion of shared Amplicon Sequence Variants (ASVs) and ECs between taxa was assessed using Venn diagrams and the ‘eulerr’ R package (version 6.1.0; Larsson 2020).
Differences in microbial community composition and genetic properties between benthic species and across a distance gradient from the cage was visualised with Principal Component Analyses (PCOs) using Euclidean distance matrices of data transformed to relative abundance, and tested for significant differences using permutational analysis of variance (PERMANOVA; design: 4th root of distance from farm * benthic species) with the ‘adonis’ function of the ‘vegan’ R package. Similarly, differences in hosts’ microbiome genetic properties were assessed for significant differences between benthic species and across a distance gradient from the cage with a PEMANOVA (design: 4th root of distance from farm * benthic species). The overall within group dispersion across distance from farm was also investigated for the taxonomic and functional microbial profiles using the ‘betadisper’ function of the ‘vegan’ R package and visualized with boxplot.
Bacterial taxa and functions that associated with fish farm activities were assessed using the ‘DeSeq2’ R package (version 1.26.0; Love et al., 2014), with the 4th root of distance from cage as factor. Bacteria found significantly associated with the impact gradient were queried using the American Biological Safety Association (ABSA) database (https://my.absa.org/Riskgroups) to identify potential pathogenic taxa.
Fatty acids trophic markers (FATMs) were calculated for several primary and secondary producers; bacterial, microplankton (diatoms and flagellate), calanoid copepods and terrestrial markers according to Dalsgaard et al. (2003) (overview in Table S9). FATMs for demosponges were calculated using the sum of long chain NMI-PUFA (C24-C29) according to de Kluijver et al. (2021). The sums of important FA classes (e.g., saturated FAs (SFA), monosaturated FAs (MUFA), polyunsaturated FAs (PUFA), omega-3 PUFA (n-3 PUFA); omega-6 PUFA (n-6 PUFA) were also calculated to explore how sponge and coral FA content varied between species and conditions. The relationship of these main classes and FATMs with distance from fish farm activities (transformed to the 4th root) was explored with regression plot using the ‘ggscatter’ function of the ‘ggpubr’ R package (version 0.4.0; Kassambara, 2020). Differences in fatty acid composition between benthic species and across a distance gradient from the cage was visualised with Principal Component Analyses (PCAs) using Euclidean distance matrices of data transformed to relative abundance, and tested for significant differences using permutational analysis of variance (PERMANOVA; design: 4th root of distance from farm * benthic species) with the ‘adonis’ function of the ‘vegan’ R package.
Pearson correlations between the microbiome and fatty acid profiles were globally tested with a ‘protest’ using the ‘vegan’ R package, and individually tested per microbial function and fatty acid using the ‘cor’ function of base R.
The proportion of specimens that died during the experiment was highest for the soft coral (D. florida), followed by the two sponges Craniella and Weberella, and the anemone H. digitata (Figure 2). All taxa tended to have lower survival rate (R from 0.23 to 0.88) closer to the cage, with significant relationship with distance from cage for both H. digitata and Weberella (Figure 2).
Figure 2 Pie chart (A) of specimens per benthic taxa that either survived, died or are suspected lost, and scatter plot (B) with regression line and interval of confidence (shaded area) of survival rate (excluding lost specimens) across the 4th root of distance from the cage.
Craniella had a significantly (aov p.value < 0.001) higher oxygen consumption rate (average of 1.5 mg O2 L-1 individual-1) than the other three species, which values averaged between 0.66 – 0.80 mg O2 L-1 individual-1 (Figure S5). No clear trend could be observed based on distance from the cage.
When oxygen consumption rates were standardized to organism mass, the soft coral D. florida had significantly higher consumption rates relative to the other three species (average of 0.12 mg O2 l-1 g-1, compared to 0.012 – 0.032 mg O2 1-1 g-1) (Figure S5). With respect to distance from farm (i.e. Station), only D. florida showed a strong and significant negative correlation (R = -0.51, p.value = 0.006), indicating increased respiration rate near the cage.
Of a total of 142 samples (excluding blanks), 25 failed to yield any PCR product (Table S3). These samples belonged primarily to the anemone H. digitata (17 out of 35 specimens) and the soft coral D. florida (7 out of 34 specimens). A total of 13,553,694 reads (mean of 110,192 per sample) were sequenced. Of these, 48% were discarded after quality filtering, 1.1% after denoising, and 14.6% after merging and removing chimeric sequences. None of the blanks’ sequences remained after these pre-processing steps. Non-bacterial sequences and those either unidentified at kingdom level or assigned to chloroplast accounted for 23 and 14.3% of reads and ASV, and were removed from the dataset, along with rare ASVs (less than 10 reads), leaving a total of 4,303,153 reads and 1,688 ASVs. All samples with a minimum of 1,000 reads reached complete sequence coverage (Figure S7).
Phylum and family composition differed greatly between epibenthic taxa, and even between the two sponges (Figure 3). Additionally, strong variation in bacterial taxa relative abundance could be observed between benthic specimens of the same species/genus. Overall, Craniella specimens tended to be dominated by Proteobacteria such as Nitrosococcaceae and Nisaeaceae, by Chloroflexi such as the A4b, and by Actinobacteria of the Microtrichaceae family. On the other hand, Weberella specimens were primarily composed of Nitrospinaceae and Proteobacteria of the Thermopetrobacteraceae family. The anemone and soft coral also harboured very different microbiomes, with D. florida being largely composed of Endozoicomonadaceae (Proteobacteria) and Mycoplasmataceae (Tenericutes), and H. digitata of Flavobacteriaceae (Bacteroidetes) and various Proteobacteria.
Figure 3 Bar plots of the bacterial taxonomic composition (families and phyla) of samples per epibenthic taxa across distance from the fish farm.
Twelve families were unique to H. digitata including Ardenticatenaceae, Chitinophagaceae, Coxiellaceae, Ectothiorhodospiraceae, Fibrobacteraceae, Kiritimatiellaceae, Helicobacteraceae, Pseudomonadaceae and Staphylococcaceae (Figure 4). Several families were also unique to Craniella specimens, such as Desulfovibrionaceae, Leptotrichiaceae, Puniceispirillales and Rhodospirillaceae. D. florida specimens harboured three unique families, including Simkaniaceae and Spirosomaceae, while Weberella individuals were composed of two unique families, namely Thermopetrobacteraceae and UBAS12411.
Figure 4 Venn diagrams of the shared bacterial taxonomic (ASVs) and functional (ECs) communities between epibenthic taxa. Families and pathways unique to each taxon are also displayed. ASVs, Amplicon Sequence Variants; ECs, Enzyme Commission numbers.
In general, the core microbiome (ASVs shared by all specimens) for each epibenthic taxon only represented a very small fraction (from 0 for Weberella to 3.4% for Craniella) of the community (Figure 5). ASVs found in 75% of the specimens still constituted a small fraction (from 0.8% for both D. florida and H. digitata to 7.8% for Craniella) of the microbiome (Figure 5A). ASVs unique to individuals represented a relatively high proportion of the microbiome for Craniella, H. digitata, and Weberella with values of 19.3, 23.8 and 45%, respectively (Figure 5A). In D. florida, none of the ASVs was found to be specimen specific. Results at genus level were relatively similar. Indeed, very few genera were shared across all specimens (Figure 5B). Those shared included Nitrospira, PAUC26f, Sva0996 and Spirochaeta in Craniella, Endozoicomonas in D. florida, and Maribacter, Nitrosomonas and Phycisphaera in H. digitata. Few genera were also present in the majority (75%) of the specimens (from 1.3% in Weberella to 14.4% in Craniella), while high proportion of unique taxa were found in each epibenthic macro-faunal taxon (17.7, 23.7 and 33.9% for H. digitata, Craniella and Weberella, respectively; Figure 5B).
Figure 5 Percentage of ASVs (A) and genera (B) per epibenthic species that were found unique to or shared across all specimens (100%), most specimens (75%), half of the specimens (50%) or at least 2 specimens.
Interestingly, most bacterial genera found in benthic macrofaunal taxa were also detected in the surrounding benthic environment, and more so in the floc than in the sediment samples (Table 2).
Table 2 Mean percent of shared bacterial genera between epibenthic macrofaunal taxa with sediment and floc samples.
H. digitata specimens harboured several microbial metabolic pathways not found in the other epibenthic taxa (Figure 4). These included 5-hydroxybenzimidazole biosynthesis, adenine and adenosine salvage II, alpha-diglucosyldiacylglycerol biosynthesis, lactose and galactose degradation I, and staphylopine biosynthesis. Craniella and D. florida both possessed one unique microbial function, gallate degradation I and fatty acid beta-oxidation, respectively, while none were found unique to Weberella.
In terms of genetic properties, Weberella microbiome tended to have greater copy numbers of 16S rRNA genes, of genetic elements and of coding sequences, and larger genomes among the epibenthic taxa (Figure S7). Oppositely, Craniella specimens’ microbiome showed lowest copy numbers of 16S rRNA genes, of genetic elements, and tended to have comparatively low number of coding sequences and small genome size, while possessing relatively high proportions of GC bonds. Microbiome genomic plasticity was similar between taxa, but noticeably higher for D. florida specimens. In comparison, sediment bacterial communities tended to have higher number of coding elements and larger genomes than those of the hosts (Figure S7). None of the genetic properties showed significant association with distance from fish farm activities (data not shown).
Microbial taxonomic (ASV) and functional (EC) richness among epibenthic taxa was highest within H. digitata, followed by Craniella, D. florida and Weberella (Figures 4 and 6). Compared to bacterial communities found in the surrounding seafloor [from sediment and deposited organic particulate matter (floc) samples in Keeley et al. (2021)], host’s microbiomes were at least one order of magnitude lower in richness (Figure 6). None of the taxonomic nor functional microbial richness (Tables S4 and S5) appeared significantly influenced by fish farm activities.
Figure 6 Boxplot of bacterial taxonomic (ASV) and functional (EC) richness per epibenthic taxa and seafloor samples (sediment & floc) with pairwise Mann-Whitney-Wilcoxon results. The y axis of the functional richness has been truncated to better visualise values between taxa and seafloor samples. Significant differences between taxa were tested with analyses of variance and are displayed on top of the boxplots. ns = non-significant, * = 0.05 > adj. p.value ≥ 0.01, ** = 0.01 > adj. p.value ≥ 1e-3, *** = 1e-3 > adj. p.value ≥ 1e-4, **** = 1e-4 > adj. p.value ≥ 1e-5.
In terms of beta-diversity, strong and significant differences could be observed between epibenthic taxa, for all data types (Figure 7, Tables 3 and S6, S7, S8 of Supplementary File 1). Interestingly, Craniella microbial taxonomic community clustered in two distinct groups while only forming one group when based on functions or genetic properties (Figure 7).
Figure 7 Principal components analysis of the epibenthic taxa’ bacterial taxonomic (A) and functional (B) diversity, and microbiome genetic properties (C).
Table 3 Permutational analysis of variance (PERMANOVA) per dataset and Taxa with 4th root of distance from cage as factor.
Aquaculture activities appeared to have species specific effect on microbiota beta-diveristy (Figure 7 and Table 3). In particular, significant effect of fish farming was observed on H. digitata microbial taxonomic (R = 0.11, p.value < 0.001) and functional (R = 0.10, p.value = 0.02) profiles, and on the microbial functional profiles of D. florida (R = 0.10, p.value = 0.02) and Craniella (R = 0.05, p.value = 0.02) (Table 4). No significant difference in within group dispersion could be observed across distance from farm (betadisper analysis: p = 0.47 and 0.07 for ASVs and ECs, respectively), but overall dispersion was usually higher within the taxonomic (ASVs) microbial profile (Figure S9).
Table 4 Permutational analysis of variance (PERMANOVA) of microbiome taxonomic and functional beta-diversity per epibenthic species across the fourth root transformed distance from farm.
Few bacterial taxa associated significantly with distance from the cage. Among those seemingly positively affected by fish farm activities were ASVs belonging to Psychromonas (Craniella), Endozocomonas (D. florida), Polaribacter, Colwellia, Kordiimonas, Acanthopleuribacter and Cycloclasticus (H. digitata), and Sedimentitalea (Weberella) (Figure 8). Those negatively associated to fish farming included Mycoplasma and Candidatus Hepatoplasma (D. florida), Ulvibacter and Aurantivirga (H. digitata), and Ekhida and Endozoicomonas (Weberella) (Figure 8). The ASV belonging to the Mycoplasma genus was the only one found as potentially pathogenic within the ABSA database. Unfortunately, its species affiliation could not be ascertained using the SILVA nor the NCBI nt database (O’Leary et al., 2016).
Figure 8 Log2 fold change in relative abundance of bacterial ASV per species significantly associated with distance from the cage. Bacterial taxa with positive log2 fold change values were more abundant at the reference sites and vice-versa. Incidence of each bacterial taxa is indicated by the black dots. Percentages following bacterial genera names indicate the percent of ASVs within that genus that responded to a distance gradient from the fish farm.
In terms of metabolic functions based on the EC database, both D. florida and Weberella had pathways significantly associated with the impact gradient (Figure S9). Those were mostly negatively affected and notably included functions responsible for cobamide biosynthesis, alginate degradation, cadaverine biosynthesis, chondroitin sulfate and in biosynthesis of polyunsaturated long and very long chain fatty acids (PUFAs) in D. florida, and in taurine degradation and nitrogen fixation in Weberella (Figure S9). The same analysis performed on the KO database identified pathways for all epibenthic taxa except H. digitata (Figure S10). Again, most pathways were negatively associated with fish farming, with functions primarily responsible for the virulence factor and lipopolysaccharide biosynthesis in Craniella, for antimicrobial gene resistance, tight adherence proteins, nitrite reductase and tetrationate reductase (sulfur metabolism) in D. florida, and for DNA damage inducible proteins and type III secretion protein in Weberella (Figure S11). Functions that were significantly higher near the pen included lysine N6-hydroxilase, D−glycero−alpha−D−manno−heptose 1−phosphate guanylyltransferase and GDP/UDP−N,N’−diacetylbacillosamine 2−epimerase (hydrolysing) in Craniella, and type VI secretion protein and one pathway involved in antimicrobial gene resistance in Weberella (Figure S11).
Fatty acid profiles differed substantially between epibenthic taxa (Figure 9 and Table S9, 1-4 of Supplementary File 1), with FA diversity found highest in Craniella (106), followed by Weberella (87), H. digitata (86) and by D. florida (80). Craniella specimens had the highest proportion of unique FAs (77%) while most of H. digitata FAs (92%) were found within the other taxa (Figure 9). Especially, most of H. digitata FAs (88%) were found in D. florida specimens.
Craniella specimens presented extremely complex profiles dominated by saturated FAs (SFA) [≈ 65% of total FAs and with abundance of branched isomers (> 50% of the total FAs)]. The mono-unsaturated FAs (MUFAs) contributed to 17% of the total FA and with relatively high levels of 16:1 (n-7) and 18:1 (n-7) (4-5%). The poly unsaturated (PUFAs) (20-26% of total FAs) were dominated by long chain non-methyl-interrupted (NMI) PUFA (C26-C28). Craniella specimens were also very high in bacteria FATMs (≈ 50% of total FAs) and the high complexity of their FA profile is illustrated by the fact that the 10 most dominating FAs contributed to only 44-45% of their total FAs (Table S9.1). In Weberella, specimens were characterized by high abundance of MUFAs (34-37% of total FAs) and (NMI) PUFA (38-41% of total FAs), with the 10 most important FAs representing 60% of total FA amount (Table S9.2). Weberella specimens also showed very high levels of Demospongic FATMs (≈ 40% of the total FAs). In D. florida, the FA profile was dominated by few FAs, with the 10 most abundant representing over 70% of the total profile. Notably, PUFA contained high levels of (n-6) PUFA; 20:4 (n-6) and 24:5 (n-6) (15-21% and 11-17% of the total FAs, respectively) (Table S9.3). The sea anemone H. digitata contained high concentration of wax esters and FAOHs composed 31-42% of the total amount of FAs. The FAOHs profile showed large amount of long chain monounsaturated FAOHs (20:1 and 22:1 FAOHs) and FAME with high amount of MUFA [20:1 (n-9) and 22:1 (n-11)] and (n-3) PUFA (20:5 (n-3) and 22:6 (n-3) (Table S9.4). The long chain MUFAs are typical calanus FATMs.
The total amount of fatty acids, in terms of µg per 100 µg of wet weight, also differed substantially between taxa and was found highest within H. digitata specimens (mean = 3.08), followed by Weberella (mean = 0.57), Craniella (mean = 0.48) and D. florida (mean = 0.46).
A PCA and PERMANOVA analysis confirmed that FA profiles differed substantially between taxa (R2 = 0.94), with effect of fish farming activities being species-specific (Figure 10). Especially, FA profiles changed significantly across the 4th root of distance from the farm for all taxa except Craniella, with R2 ranging from 0.32 (D. florida) to 0.11 (Weberella) (Figure 11). Interestingly, the sponges clustered more closely compared with the other taxa (Figure 10).
Figure 10 Principal component analysis of the fatty acid profiles colored by epibenthic taxa and shaped according to distance from the fish farm. Result of a permutational analysis of variance (PERMANOVA; 9,999 permutations) testing the difference between taxa and the effect of 4th root of distance from farm is displayed at the lower right corner, with significant relationship (p.value <= 0.05) highlighted in bold.
Figure 11 Redundancy analysis (RDA) of the fatty acid profiles per epibenthic species using the 4th root of distance from farm as explanatory variable. The five most important response variables (fatty acids) are overlaid as red arrows with shade corresponding to their contribution. Variables of particular interest, including distance from farm, were also fitted (blue arrows) using the ‘envfit’ function of the ‘vegan’ R package. Only the five most correlated variables to the ordination are displayed. Results of a permutational analysis of variance (PERMANOVA; 9,999 permutations) testing the effect of 4th root of distance from farm are displayed at the upper right corner of each facet, with significant relationship highlighted in bold.
D. florida was the only taxa to show significant changes in total FAs per µg of wet weight and ash free dry weight across a distance gradient from the farm, gradually increasing away from activities (Figure S12). Higher levels of (n-6)-PUFAs, SFA, bacterial and chlorophyl FAs, and lower levels of (n-3)-PUFA, MUFA, terrestrial and dinoflagellate FAs were also observed in D. florida specimens located under the farm compared to the reference sites (Figure 11 and S13). Oppositely, H. digitata specimen showed increased level of MUFA, (n-6)-PUFA, PUFA-NMI and terrestrial FAs, and decreased concentration of SFA, bacterial, chlorophyl and diatom FAs near the farm (Figure 11 and S13). Furthermore, 18:2 (n-6) was more abundant near the farm while 14:0, was more present further from away. In Weberella, FAs positively associated with fish farm activities included 24:1 (n-9) and MUFA in general, while 16:1 (n-11), 26:3 NMI-b, (n-3)-PUFA and dinoflagellate FAs were associated negatively (Figure 11 and S13). Craniella specimens appeared to be the least affected by fish farm activities and mostly showed increased concentrations of dinoflagellate FAs [18:3 (n-3) and 18:4 (n-3)] near the farm tended.
Correlation between the fatty acid and microbiome profiles were highly significant and substantially stronger with the functional community (r = 0.807) than with the taxonomic community (r = 0.525) (Table S10). Interestingly, the microbiome and FA composition of both sponges revealed two distinct clusters of the samples independently of the distances to the fish farm (Figure 9) and in both cases, samples part of cluster 1 and 2 were mostly similar between the microbial and fatty acids datasets (Figure S14), suggesting that changes in the microbiome were accompanied by changes in the fatty acid profile.
In terms of stable isotopes δ15N (‰) and δ13C (‰), only D. florida showed significant relationship with distance from the cage, with decreasing concentration of both δ15N and δ13C and increasing δ15N/δ13C ratio away from the farm (Figure S14).
Disentangling the effect of nutrient enrichment and smothering from aquaculture activities is difficult as they are induced by the same process and associated biodeposits. Nonetheless, it is generally recognized that nutrient enrichment near the farms can induce hypoxic or anoxic conditions, leading to reduced macro-benthic richness and the dominance of few tolerant opportunistic taxa including polychaetes and nematodes (Keeley, 2013; Price et al., 2014). Increased smothering on suspension-feeders is typically associated with increased mortality rate but the metabolic response varies substantially within the literature, with contrasting results. Several studies have reported reduced respiration activity in corals and sponges (Lohrer et al., 2006; Maldonado et al., 2008; Tompkins-MacDonald and Leys, 2008), while others found no effects (Cummings et al., 2020) or even heightened respiration rate in specimens under increased sedimentation (Dallmeyer et al., 1982; Riegl and Branch, 1995; Philipp and Fabricius, 2003; Bannister et al., 2012). Detrimental effects of increased turbidity and smothering are believed to arise primarily from the clogging of the aquiferous system in sponges or the feeding apparatus in corals, increasing energetic feeding cost associated with the need to produce more mucus for sediment clearance, and the reduction of ambient light hampering microbial photosynthetic activity (Erftemeijer et al., 2012; Leys, 2013). Divergence in the response between taxonomic groups is notably attributed to differences in size, morphology and physiological processes to avoid smothering (Erftemeijer et al., 2012; Leys, 2013; Junjie et al., 2014; Jones et al., 2019). In their reviews, Erftemeijer et al. (2012) and Leys (2013) found that fine sediment usually have a greater impact than coarse sediment, which may contribute to the differences in responses between studies. In addition, important smothering effects may cause organisms to physiologically shut down and reduce respiration rate while low to moderate smothering may reduce pumping activities due to clogging, but increase respiration rate due to the need to support higher mucus production (Leys, 2013; Biggerstaff et al., 2017). This bimodal response may further explain some of the divergences observed in the literature.
In this study, we found a gradual positive correlation of survival rate and distance from the farm for all epibenthic taxa, and especially for H. digitata and Weberella spp. This global trend suggests that the sessile epibenthic organisms were indeed being negatively affected by the environmental changes induced by fish farming. The soft coral D. florida also showed a strong increase in respiration rate near the farm, however, we found little evidence of altered oxygen consumption in the other three suspension-feeding species. It is possible that the relatively strong hydrodynamics at the study site (average near-bottom currents speed 0.086 m·s-1; Dunlop et al., 2021) mitigated the smothering effects to some extent, and with it the severity of the farm-related environmental stress gradient. These findings are nevertheless relevant as the fauna were deliberately cultured at a site that they naturally inhabited (beyond the farm footprint), and therefore meets their fundamental physical requirements. Removing the organisms from their natural habitat and culturing them under greater depositional loads at a non-dispersive site, where their physical requirements were not met, would only produce contrived results (stress upon stress) with limited applicability to the natural world.
Sponges and corals are known for hosting particularly dense and diverse microbial communities compared to other animals, likely due to their simple organization and reliance on symbionts for supporting various essential functions (Pita et al., 2016; van Oppen and Blackall, 2019). Several factors are thought to influence the diversity of their microbial consortia including host genotype, phenotype, physiological conditions (e.g., life stage, health), the presence of other symbionts and environmental factors (Easson and Thacker, 2014; Thomas et al., 2016; Cleary et al., 2019; van Oppen and Blackall, 2019). Accordingly, we found important differences in microbial diversity and community composition between each taxon. We also found marginal inter and intra-species overlap in ASV sequences, and despite relatively similar taxonomic diversity within epibenthic taxa, observed strong variations in the relative abundance of bacterial groups between specimens that appeared non-correlated to environmental conditions. These results support the presence of phylosymbiosis in sponges and corals, in which host microbiome diversity reflects the host’s phylogeny, and suggest a strong influence of biological factors (e.g., life stage, phenotype) that were not taken into account in this study.
In sponges, species can differ substantially with respect to their prokaryotic assemblages and are usually divided into two broad categories; high microbial abundance (HMA) sponges, also referred to as bacteriosponges, and low microbial abundance (LMA), referred to as nonsymbiont-harbouring sponges (Hentschel et al., 2003; Hentschel et al., 2012). Sponges that belong to the former group are typically dominated by Chloroflexi, Acidobacteria and Actinobacteria (van Soest et al., 2012; Moitinho-Silva et al., 2017), characterized by higher microbial richness (Weisz et al., 2007), and by a relatively stable consortia of sponge-enriched microbes (Kamke et al., 2010; Hentschel et al., 2012). Oppositely, LMA diversity is usually limited, restricted to few bacterial phyla such as Proteobacteria, Cyanobacteria and Chloroflexi (Weisz et al., 2007; Kamke et al., 2010; Giles et al., 2013; Bayer et al., 2014), relating closely to bacteria found in the surrounding seawater (Schmitt et al., 2007; Schmitt et al., 2011), with little overlap in bacterial taxa between specimens (Moitinho-Silva et al., 2014). Due to the higher intra and inter-species microbiome similarities within HMA sponges, it is also believed that vertical transmission (transfer of bacteria from parents to offspring) plays a more prominent role in HMA sponges than in LMA sponge, where horizontal transmission (bacterial acquisition from the environment) may be more prevalent (Giles et al., 2013; Oliveira et al., 2020). The microbiome profiles of the two sponge species that we examined differed substantially, both in terms of taxonomic and functional diversity, with Craniella harboring more phyla and over three times as much ASVs and ECs than Weberella. Few Craniella species have been identified as HMA sponges (Schöttner et al., 2013; Anbuchezhian and Li, 2015) but no previous information could be obtained for Weberella. While we did not perform quantitative analysis of microbial density, our results suggest that Weberella belongs to LMA sponges, with important dominance of Proteobacteria and Nitrospinae, and richness values similar to those previously reported for LMA sponges (Giles et al., 2013). This assumption is also supported by the smaller proportion of core ASVs in Weberella, and higher percent of shared bacterial genera with sediment and floc samples, suggesting a more prominent role of horizontal transfer in bacterial acquisition.
Coral and sponge-associated bacteria are believed to play an important role in their host’s nutrition and chemical defense mechanisms by contributing to carbon fixation, nitrogen metabolism, sulfur cycling and production of antimicrobial compounds (Thompson et al., 2014; Li, 2019; van Oppen and Blackall, 2019). As such, and despite important differences in microbial taxonomic composition between taxa, the functional microbial profile usually converges in hosts, with enrichment of specialized bacteria compared to the surrounding environment (Bayer et al., 2014; Gantt et al., 2019; Fiore et al., 2020). While clear distinction could still be observed between the functional profiles of the different suspension-feeding taxa, this convergence was exemplified in the Craniella specimens, which appeared to be represented by two different species or sub-species based on their microbial ASV communities but could not be discriminated based on their microbial functional capabilities. The higher functional versus taxonomic richness ratio between the free-living communities and host microbiomes also support the confluence of metabolic pathways in hosts.
Interestingly, each epibenthic taxon could also be differentiated by the overall genetic properties of their microbiomes (i.e., mean number of 16S rRNA copies, mean genome size, mean GC content, mean genome plasticity, mean number of coding sequences (ncds) and mean number of genetic elements). In this case, the mean genome size and ncds were found highest within the seafloor samples, closely followed by Weberella’s microbiome. Free-living and generalist bacteria are usually characterized by larger genomes and higher number of coding sequences than specialized, symbiotic ones as they tend to perform a more diverse range of metabolic activities. The fact that Weberella’s microbiomes had values close to that of the sediment and floc samples is another indication that it may rely more heavily on horizontal transfer for bacterial acquisition. In Craniella, microbiomes tended to have elevated GC content compared to other taxa while D. florida’s microbiome showed higher genome plasticity. GC pairs are linked by three hydrogen bonds versus two for Adenine-thyamine (AT), making DNA with high GC content more thermo-stable (Yakovchuk et al., 2006). GC content in prokaryotes have also been found to positively correlate with genome size (Almpanis et al., 2018) which, with the exception of Craniella, can be observed in our data. Mutations in prokaryote genomes are naturally biased toward AT bonds (Hershberg and Petrov, 2010; Bohlin et al., 2018) and as such, elevated GC content is believed to be driven by increased selective pressure (Raghavan et al., 2012). While factors influencing GC content are still up to debate, the consensus is that multiple not mutually exclusive elements related to both evolutionary history and the environment are involved (Mann and Chen, 2010; Almpanis et al., 2018). Notably, there is evidence of association between GC content and optimal growth temperature (Musto et al., 2004; Wang et al., 2006; Zheng and Wu, 2010), and positive correlation with nitrogen-fixing capabilities (McEwan et al., 1998) and aerobiosis (Naya et al., 2002). As for the phi metric, it represents an indication of how ‘plastic’ genomes are, and therefore, how likely they are to lose or integrate new genes through horizontal transfer (Bowman and Ducklow, 2015). Genome plasticity facilitates adaptation to novel environments or conditions (Woodcock et al., 2017b) and have been linked to increased microbial resistance in Vibrio (Verma et al., 2019). Hypothetically, these differences in microbial genetic properties could influence the resilience of the microbiomes and hence of the host to environmental changes. Further research in this area could provide interesting insights as to the climate resilience of sponges and corals in a changing ocean.
Several studies have reported effects of anthropogenic activities on coral and sponge microbiomes (Meron et al., 2011; Morrow et al., 2012; Jessen et al., 2013; Gissi et al., 2019; Ziegler et al., 2019) (but see Pineda et al., 2017), with evidence of reduced evenness and increased richness and beta diversity from stress (see review from McDevitt-Irwin et al., 2017). Indeed, it is believed that environmental stress weakens the top-down control in the holobiont, inducing dysbiosis (microbial imbalance) and increasing prevalence of opportunistic microbes to the detriment of beneficial ones (van Oppen and Blackall, 2019). In our study, we did not detect alteration of bacterial richness due to fish farming but did find evidence of species-specific effect on host microbiome beta-diversity. Changes associated with fish farming were particularly observed in H. digitata, with significant turnover of both taxonomic and functional profiles. Weak but significant effect on the functional profiles were also found in the other epibenthic taxa, with the exception of Weberella. Stochasticity in community composition was generally higher for ASVs than ECs. As such, it is likely that the lower responsivity of the taxonomic profile is due to different but closely related taxa performing equivalent metabolic functions associated to fish farm activities between specimens from same stations.
Few ASVs could be identified as potential indicators of organic enrichment, including Psychromonas in Craniella, several Endozoicomonas ASVs in D. florida, Polaribacter, Kordiimonas, Acanthopleuribacter, Cycloclasticus and Colwellia in H. digitata, and Sedimentalea in Weberella. Several studies have reported enrichment of Colwellia, Polaribacter and Psychromonas in mariculture systems (Verhoeven et al., 2018; Wang et al., 2018; Molina and Fernández, 2020). As for Endozoicomonas, they often represent an important part of the microbiome in marine eukaryotes (Bayer et al., 2013; Nishijima et al., 2013; Fiore et al., 2015; Ziegler et al., 2016; Bartz et al., 2018), but their functional role remains largely unknown (Neave et al., 2017). In sponges and corals, they are believed to contribute to nutrient acquisition and cycling, playing an increasing role in oligotrophic environments (Rossbach et al., 2019). Conversely, Endozoicomonas were found to have a detrimental effect on fish hosts in mariculture systems, notably causing epitheliocystis and inducing mortality (Mendoza et al., 2013; Katharios et al., 2015). Here, several members of Endozoicomonas were associated with fish farming in D. florida, but to reference conditions in Weberella. These results align with that of Ziegler et al. (2016) where different Endozocoimonas strains within coral hosts responded oppositely to anthropogenic impacts, suggesting that members of this family may be playing very distinct roles in their eukaryotic hosts.
Numerous microbial metabolic functions were found significantly correlated with fish farming, but few positively associated with organic enrichment per se. These included lysine N6-hydroxylase and D-glycero-alpha-manno-heptose 1-phosphate guanylyltransferase in Craniella and type VI secretion system protein, and an antimicrobial resistance function in Weberella. Secretion systems of type IV function primarily as an interbacterial antagonisms to ward off competition (García-Bayona and Comstock, 2018). Its increased abundance in near-field Weberella specimens, along with antimicrobial resistance pathways, may indicate an increased effort of the holobiont to keep microbial diversity under control in the nutrient-enriched environment or a side effect of increased mucus production and associated antimicrobial compounds produced by resident bacteria (Ritchie, 2006; Nissimov et al., 2009). Several of the pathways negatively associated with fish farming were related to nutrient and transport metabolisms (e.g., nitrogen fixation, sulfite oxidation, fructose and sucrose transport and degradation). While this observation links to the difference in nutrient concentration and provenance between the cage and reference sites, it contrasts with findings of Kelly et al. (2014) and Ziegler et al. (2016) where higher nutrient availability was associated with increased nutrient-related metabolisms. This discordance is possibly due to the effect of smothering near the pen, reducing nutrient acquisition in both sponges and corals caused by the obstruction of their feeding mechanism. Notwithstanding, these observations both support the hypothesis that microbial community structure is partly determined by available food sources and the nutritional needs of the host.
Fatty acid profiles in corals and sponges are known to be taxa-specific and strongly influenced by their symbionts and feed sources (Rod’kina, 2005; Imbs et al., 2010; Salvo et al., 2018). As such, they can be useful chemotaxonomic and ecological biomarkers (Núñez-Pons et al., 2012; Imbs et al., 2019). They have also been used as a proxy to estimate the biomass of microorganisms in sponge host (Rod’kina, 2003). In this study, fatty acid profiles were highly specific to each epibenthic taxa, with highest similarity between the two sponges. Craniella specimens were notably characterized with the most complex FA profiles of all taxa, and a strong dominance of branched FAs likely originating from their microbiomes. This coincides with Craniella’s high bacterial diversity, about three times higher than that of Weberella. Oppositely, Weberella specimens showed simpler FA profiles, mostly dominated by NMI PUFAs (32-40%), MUFAs (35-37%) and SFA (19-20%). These results further support Craniella as HMA sponge, depending heavily on its microbiome for FA synthesis, and Weberella as an LMA, with lesser contribution from its microbiome. Interestingly, both Craniella and Weberella datasets showed clustering of the specimens into two distinct groups, regardless of their site location. Additionally, clustered specimens based on FA profiles mostly concorded with those based on host microbial profiles. This observation provides further evidence of having sampled two distinct but closely related Craniella and Weberella species or sub-species, and further highlight the value of FAs as chemotaxonomic indicators.
The soft coral D. florida specimens were characterized by an important concentration of (n-6)-PUFA, a peculiarity of octocorals (Imbs, 2013). As for the anemone H. digitata, specimens were found with large concentration of wax-esters, which are particularly useful for storing energy and typically found in high concentration in marine animals feeding on phyto and zooplankton (Dalsgaard et al., 2003). H. digitata also had a particularly high total FA weight compared to the other epibenthic taxa (up to six times higher), while possessing the simplest FA profile of all taxa. The high levels of long chain MUFA (20:1 and 22:1) in both FAOHs and the FAs suggest that the sea anemone’s diet was mainly focused on Calanus, an abundant marine copepod especially rich in these wax esters (Lee et al., 2006).
Overall, the fatty acid profiles were highly taxa-specific and correlated strongly with the host’s microbiome, and especially with the functional microbial community (r≥0.8). This strong association suggest an important influence of the metabolic capabilities of the microbiome in structuring the fatty acids in these epibenthic organisms.
Corals and sponges have highly diverse FA profiles compared to other marine animals, and because these organisms derive most of their FAs from their symbionts and/or from feed sources, these can be particularly useful as ecological biomarkers, notably to track changes in environmental conditions (Imbs, 2013; de Kluijver et al., 2021). Constituting the main component of total lipid in both corals and sponges, fatty acids have also been used as a measure of energy reserve and to assess the potential resilience of taxa to stress (Imbs and Yakovleva, 2012; Bennett et al., 2018). In our study, only D. florida showed significant relationship between normalized total FA weight and fish farming, displaying reduced amount near the cage. Interestingly, D. florida was also the only epibenthic taxon to show significant increase of respiration rate near the farm, and the one with lowest survival rate. This suggest that D. florida specimens located near the farm may have been particularly active in de-clogging their feeding apparatus, which, combined with potentially reduced nutrient intake, may have led to reduced lipid storage and resilience.
Looking at the effect of fish farm activities on the overall FA profiles, D. florida appeared to be the most responsive taxa, followed by H. digitata and Weberella. In particular, D. florida specimens located under the farm showed decreased concentration of (n-3) PUFA and MUFA but increased (n-6) PUFA compared to reference conditions. While Weberella also showed decreased (n-3) PUFA under farming conditions, both Weberella and H. digitata specimens showed elevated MUFA concentration at the cage. Omega-3 and 6 PUFA are believed to provide increased stress resistance across a range of taxa and depletion of these PUFA in sponges and corals has been associated with deteriorating health and increased disease and mortality rate (Bachok et al., 2006; Bennett et al., 2018). As for MUFA, they represent a promptly available source of energy (Tocher, 2003) and have been found to be reduced in stressed sponges to compensate for energy deficit (Bennett et al., 2018). The apparent feeding difficulty experienced by D. florida specimens under farming condition may have induced the catabolism of MUFA and (n-3) PUFA and explain the lower concentrations found in these organisms. Oppositely, H. digitata and Weberella specimens did not appear to experience compromised feeding activities and may have adapted a different strategy to increased sedimentation rate, such as elevating their metabolization of MUFA.
Salmon feeds are primarily made of terrestrial plants that contain high concentration of terrestrial fatty acids and stable isotope δ13C and δ15N which have been successfully used as proxy of fish farming activities in sediment (White et al., 2019; Woodcock et al., 2019). As such, we anticipated that terrestrial FAs and the stable isotope δ13C would become more prominent in sponges and corals located near the cage while δ15N would decrease. However, only H. digitata showed significant increase of terrestrial FAs near the fish farm, while D. florida showed opposite response. It is possible that this contrasting observation in D. florida was caused by the clogging of its feeding apparatus near the farm, and hence of its capacity to assimilate organic material. However, we also acknowledge that the fatty acid profile of the feed was not performed in this study and may not have been as strongly dominated by terrestrial fatty acid as anticipated.
In regard to stable isotopes, only D. florida showed significant association of δ13C and δ15C with farming activities. Taken together, the fatty acid and stable isotope profiles indicate that specifically targeting terrestrial FAs and stable isotopes in the tissue of epibenthic sessile organism may not represent a sensible method to detect fish farming impact, and may instead be better assessed through overall FA profile turnover.
Overall, our results indicate that fish farming do impact sessile epibenthic suspension feeders but that these impacts are specific to each taxonomic group. We found that fish farming impacts, including organic enrichment and smothering, were associated with increased mortality rate in epifauna but apart from D. florida, had little to no effect on respiration rate. While this suggest mortality rate of translocated epifauna could be used as proxy of impact gradient, finding early-sings of health degradation is vital to intervene before irreparable damage is done. In this study, we found evidence of altered functional microbial and fatty acid profiles in epifauna associated to farming activities but also that the degree of response was species-specific, particularly affecting H. digitata and D. florida. We also identified several microbial taxa and functions, and FAs that significantly associated with the exposure gradient that could, upon further research validation, serve as proxies of benthic health to monitor conditions over mixed and hard substrates, where traditional monitoring techniques are unsuitable. Future research should aim at better understanding the underlying mechanisms causing microbiome and FA alterations using a combination of omics approaches (e.g. metagenomics and metatranscriptomics), and confirm associations made between the identified putative indicators and impact gradient originating from fish farming.
The datasets presented in this study can be found in NCBI’s online repositories (https://www.ncbi.nlm.nih.gov/) under project number PRJNA694725.
NK conceptualised the study. NK conducted field work and sampling. OL, SMe and SMj generated the data and performed analyses, and OL wrote the manuscript with intellectual contributions from all co-authors. NK provided grant and equipment support. All authors contributed to the article and approved the submitted version.
Support and funding for this project was provided by the Norwegian Research Council under the project 267829 SUSTAINable AQUAculture in the North: identifying thresholds, indicators and tools for future growth.
The authors declare that the research was conducted in the absence of any commercial or financial relationships that could be construed as a potential conflict of interest.
All claims expressed in this article are solely those of the authors and do not necessarily represent those of their affiliated organizations, or those of the publisher, the editors and the reviewers. Any product that may be evaluated in this article, or claim that may be made by its manufacturer, is not guaranteed or endorsed by the publisher.
We thank Raymond Bannister and Astrid Harenza for valuable assistance setting up the experiment and the captain and crew of Fangst for assistance at sea. We thank the Norwegian Academic HPC Services for access and support to their infrastructure to perform bioinformatics, and the Stable Isotope Facility of the University of California (Davis Campus, California, USA) for analysing the stable isotopes. A special thank you goes to Chris Yesson (ZSL) for the mitogenomic information of Nephtheid soft corals.
The Supplementary Material for this article can be found online at: https://www.frontiersin.org/articles/10.3389/fmars.2022.841806/full#supplementary-material
Almpanis A., Swain M., Gatherer D., McEwan N. (2018). Correlation Between Bacterial G+C Content, Genome Size and the G+C Content of Associated Plasmids and Bacteriophages. Microb. Genomics 4 (4), 0–7. doi: 10.1099/mgen.0.000168
Anbuchezhian R., Li Z. (2015). “Marine Sponge Craniella Australiensis-Associated Bacterial Diversity,” in Encyclopedia of Metagenomics (Springer US), 319–326. doi: 10.1007/978-1-4899-7475-4_604
Bachok Z., Mfilinge P., Tsuchiya M. (2006). Characterization of Fatty Acid Composition in Healthy and Bleached Corals From Okinawa, Japan. Coral Reefs 25 (4), 545–554. doi: 10.1007/s00338-006-0130-9
Bartz J. O., Blom J., Busse H. J., Mvie J. B., Hardt M., Schubert P., et al. (2018). Parendozoicomonas Haliclonae gen. nov. sp. nov. Isolated From a Marine Sponge of the Genus Haliclona and Description of the Family Endozoicomonadaceae fam. nov. Comprising the Genera Endozoicomonas, Parendozoicomonas, and Kistimonas. Syst. Appl. Microbiol. 41 (2), 73–84. doi: 10.1016/j.syapm.2017.11.004
Bannister R. J., Battershill C. N., de Nys R. (2012). Suspended Sediment Grain Size and Mineralogy Across the Continental Shelf of the Great Barrier Reef: Impacts on the Physiology of a Coral Reef Sponge. Cont. Shelf Res. 32, 86–95. doi: 10.1016/j.csr.2011.10.018
Bayer T., Neave M. J., Alsheikh-Hussain A., Aranda M., Yum L. K., Mincer T., et al. (2013). The Microbiome of the Red Sea Coral Stylophora pistillata Is Dominated by Tissue-Associated Endozoicomonas Bacteria. Appl. Environ. Microbiol. 79 (15), 4759–4762. doi: 10.1128/AEM.00695-13
Bayer K., Moitinho-Silva L., Brümmer F., Cannistraci C. V., Ravasi T., Hentschel U. (2014). GeoChip-Based Insights Into the Microbial Functional Gene Repertoire of Marine Sponges (High Microbial Abundance, Low Microbial Abundance) and Seawater. FEMS Microbiol. Ecol. 90 (3), 832–843. doi: 10.1111/1574-6941.12441
Bell J. J. (2008). The Functional Roles of Marine Sponges. Estuar. Coast. Shelf Sci. 79 (3), 341–353. doi: 10.1016/j.ecss.2008.05.002
Bennett H., Bell J. J., Davy S. K., Webster N. S., Francis D. S. (2018). Elucidating the Sponge Stress Response; Lipids and Fatty Acids can Facilitate Survival Under Future Climate Scenarios. Global Change Biol. 24 (7), 3130–3144. doi: 10.1111/gcb.14116
Biggerstaff A., Smith D. J., Jompa J., Bell J. J. (2017). Metabolic Responses of a Phototrophic Sponge to Sedimentation Supports Transitions to Sponge-Dominated Reefs. Sci. Rep. 7 (1), 1–11. doi: 10.1038/s41598-017-03018-y
Bohlin J., Eldholm V., Brynildsrud O., Petterson J. H. O., Alfsnes K. (2018). Modeling of the GC Content of the Substituted Bases in Bacterial Core Genomes. BMC Genomics 19 (1), 1–6. doi: 10.1186/s12864-018-4984-3
Bowman J. S., Ducklow H. W. (2015). Microbial Communities can be Described by Metabolic Structure: A General Framework and Application to a Seasonally Variable, Depth-Stratified Microbial Community From the Coastal West Antarctic Peninsula. PLoS One 10 (8), 1–18. doi: 10.1371/journal.pone.0135868
Buhl-Mortensen L., Buhl-Mortensen P., Dolan M. F. J., Dannheim J., Bellec V., Holte B. (2012). Habitat Complexity and Bottom Fauna Composition at Different Scales on the Continental Shelf and Slope of Northern Norway. Hydrobiologia 685 (1), 191–219. doi: 10.1007/s10750-011-0988-6
Callahan B. J., McMurdie P. J., Rosen M. J., Han A. W., Johnson A. J. A., Holmes S. P. (2016). DADA2: High-Resolution Sample Inference From Illumina Amplicon Data. Nat. Methods 13 (7), 581–583. doi: 10.1038/nmeth.3869
Cleary D. F. R., Swierts T., Coelho F. J. R. C., Polónia A. R. M., Huang Y. M., Ferreira M. R. S., et al. (2019). The Sponge Microbiome Within the Greater Coral Reef Microbial Metacommunity. Nat. Commun. 10 (1), 1–12. doi: 10.1038/s41467-019-09537-8
Clerissi C., de Lorgeril J., Petton B., Lucasson A., Escoubas J.-M., Gueguen Y., et al. (2020). Microbiota Composition and Evenness Predict Survival Rate of Oysters Confronted to Pacific Oyster Mortality Syndrome. Front. Microbiol. 11. doi: 10.3389/fmicb.2020.00311
Cummings V. J., Beaumont J., Mobilia V., Bell J. J., Tracey D., Clark M. R., et al. (2020). Responses of a Common New Zealand Coastal Sponge to Elevated Suspended Sediments: Indications of Resilience. Mar. Environ. Res. 155, 104886. doi: 10.1016/j.marenvres.2020.104886
Dallmeyer D. G., Porter J. W., Smith G. J. (1982). Effects of Particulate Peat on the Behavior and Physiology of the Jamaican Reef-Building Coral Montastrea Annularis. Mar. Biol. 68 (3), 229–233. doi: 10.1007/BF00409589
Dalsgaard J., St. John M., Kattner G., Müller-Navarra D., Hagen W. (2003). Fatty Acid Trophic Markers in the Pelagic Marine Environment, Vol. 4. 225–340. doi: 10.1016/S0065-2881(03)46005-7
de Kluijver A., Nierop K. G. J., Morganti T. M., Bart M. C., Slaby B. M., Hanz U., et al. (2021). Bacterial Precursors and Unsaturated Long-Chain Fatty Acids are Biomarkers of North-Atlantic Deep-Sea Demosponges. PLoS One , 16 (1 January 2021), 1–18. doi: 10.1371/journal.pone.0241095
Dittami S. M., Arboleda E., Auguet J.-C., Bigalke A., Briand E., Cárdenas P., et al. (2021). A Community Perspective on the Concept of Marine Holobionts: Current Status, Challenges, and Future Directions. Peer J. 9, e10911. doi: 10.7717/peerj.10911
Dunlop K., Harendza A., Bannister R., Keeley N. (2021). Spatial Response of Hard- and Mixed-Bottom Benthic Epifauna to Organic Enrichment From Salmon Aquaculture in Northern Norway. Aquac. Environ. Interact. 13 (December), 455–475. doi: 10.3354/aei00419
Dunlop K., Harendza A., Plassen L., Keeley N. (2020). Epifaunal Habitat Associations on Mixed and Hard Bottom Substrates in Coastal Waters of Northern Norway. Front. Mar. Sci. 7 (November). doi: 10.3389/fmars.2020.568802
Easson C. G., Thacker R. W. (2014). Phylogenetic Signal in the Community Structure of Host-Specific Microbiomes of Tropical Marine Sponges. Front. Microbiol. 5 (OCT). doi: 10.3389/fmicb.2014.00532
Erftemeijer P. L. A., Riegl B., Hoeksema B. W., Todd P. A. (2012). Environmental Impacts of Dredging and Other Sediment Disturbances on Corals: A Review. Mar. Pollut. Bull. 64 (9), 1737–1765. doi: 10.1016/j.marpolbul.2012.05.008
Fadrosh D. W., Ma B., Gajer P., Sengamalay N., Ott S., Brotman R. M., et al. (2014). An Improved Dual-Indexing Approach for Multiplexed 16S rRNA Gene Sequencing on the Illumina MiSeq Platform. Microbiome 2 (1), 6. doi: 10.1186/2049-2618-2-6
FAO (2018) The State of World Fisheries and Aquaculture 2018-Meeting the Sustainable Development Goals. Available at: https://linkinghub.elsevier.com/retrieve/pii/S1056617119320537.
Fernandez-Jover D., Arechavala-Lopez P., Martinez-Rubio L., Tocher D., Bayle-Sempere J., Lopez-Jimenez J., et al. (2011). Monitoring the Influence of Marine Aquaculture on Wild Fish Communities: Benefits and Limitations of Fatty Acid Profiles. Aquac. Environ. Interact. 2 (1), 39–47. doi: 10.3354/aei00029
Fiore C. L., Jarett J. K., Steinert G., Lesser M. P. (2020). Trait-Based Comparison of Coral and Sponge Microbiomes. Sci. Rep. 10 (1), 2340. doi: 10.1038/s41598-020-59320-9
Fiore C. L., Labrie M., Jarett J. K., Lesser M. P. (2015). Transcriptional activity of the giant barrel sponge, Xestospongia muta Holobiont: Molecular evidence for metabolic interchange. Front. Microbiol. 6 (APR). doi: 10.3389/fmicb.2015.00364
Gantt S. E., McMurray S. E., Stubler A. D., Finelli C. M., Pawlik J. R., Erwin P. M. (2019). Testing the Relationship Between Microbiome Composition and Flux of Carbon and Nutrients in Caribbean Coral Reef Sponges. Microbiome 7 (1), 1–13. doi: 10.1186/s40168-019-0739-x
García-Bayona L., Comstock L. E. (2018). Bacterial Antagonism in Host-Associated Microbial Communities. Science 361 (6408), 1–11. doi: 10.1126/science.aat2456
Giles E. C., Kamke J., Moitinho-Silva L., Taylor M. W., Hentschel U., Ravasi T., et al. (2013). Bacterial Community Profiles in Low Microbial Abundance Sponges. FEMS Microbiol. Ecol. 83 (1), 232–241. doi: 10.1111/j.1574-6941.2012.01467.x
Gili J.-M. M., Coma R. (1998). Benthic Suspension Feeders: Their Paramount Role in Littoral Marine Food Webs. Trends Ecol. Evol. 13 (8), 316–321. doi: 10.1016/S0169-5347(98)01365-2
Gissi F., Reichelt-Brushett A. J., Chariton A. A., Stauber J. L., Greenfield P., Humphrey C., et al. (2019). The Effect of Dissolved Nickel and Copper on the Adult Coral Acropora Muricata and its Microbiome. Environ. Pollut. 250, 792–806. doi: 10.1016/j.envpol.2019.04.030
Grego M., Malej A., De Troch M. (2020). The Depleted Carbon Isotopic Signature of Nematodes and Harpacticoids and Their Place in Carbon Processing in Fish Farm Sediments. Front. Mar. Sci. 7 (July). doi: 10.3389/fmars.2020.00572
Hahn S., Stoilov I. L., Ha T. B. T., Raederstorff D., Doss G. A., Li H. T., et al. (1988). Biosynthetic Studies of Marine Lipids. 17. The Course of Chain Elongation and Desaturation in Long-Chain Fatty Acids of Marine Sponges. J. Am. Chem. Soc. 110 (24), 8117–8124. doi: 10.1021/ja00232a025
Hamoutene D., Salvo F., Cross S., Dufour S. C., Donnet S. (2018). Linking the Presence of Visual Indicators of Aquaculture Deposition to Changes in Epibenthic Richness at Finfish Sites Installed Over Hard Bottom Substrates. Environ. Monit. Assess. 190 (12), 750. doi: 10.1007/s10661-018-7108-2
Hamoutene D., Salvo F., Donnet S., Dufour S. C. (2016). The Usage of Visual Indicators in Regulatory Monitoring at Hard-Bottom Finfish Aquaculture Sites in Newfoundland (Canada). Mar. Pollut. Bull. 108 (1–2), 232–241. doi: 10.1016/j.marpolbul.2016.04.028
Hentschel U., Fieseler L., Wehrl M., Gernert C., Steinert M., Hacker J., et al. (2003). Microbial Diversity of Marine Sponges. Prog. Mol. Subcell. Biol. 37, 59–88. doi: 10.1007/978-3-642-55519-0_3
Hentschel U., Piel J., Degnan S. M., Taylor M. W. (2012). Genomic Insights Into the Marine Sponge Microbiome. Nat. Rev. Microbiol. 10 (9), 641–654. doi: 10.1038/nrmicro2839
Hershberg R., Petrov D. A. (2010). Evidence That Mutation is Universally Biased Towards AT in Bacteria. PLoS Genet. 6 (9), e1001115. doi: 10.1371/journal.pgen.1001115
Holmer M. (2010). Environmental Issues of Fish Farming in Offshore Waters: Perspectives, Concerns and Research Needs. Aquac. Environ. Interact. 1 (1), 57–70. doi: 10.3354/aei00007
Imbs A. B. (2013). Fatty Acids and Other Lipids of Corals: Composition, Distribution, and Biosynthesis. Russian J. Mar. Biol. 39 (3), 153–168. doi: 10.1134/S1063074013030061
Imbs A. B., Dang L. P. T., Nguyen K. B. (2019). Comparative Lipidomic Analysis of Phospholipids of Hydrocorals and Corals From Tropical and Cold-Water Regions. PLoS One 14 (4), e0215759. doi: 10.1371/journal.pone.0215759
Imbs A. B., Latyshev N. A., Dautova T. N., Latypov Y. Y. (2010). Distribution of Lipids and Fatty Acids in Corals by Their Taxonomic Position and Presence of Zooxanthellae. Mar. Ecol. Prog. Ser. 409 (June 2019), 65–75. doi: 10.3354/meps08622
Imbs A. B., Yakovleva I. M. (2012). Dynamics of Lipid and Fatty Acid Composition of Shallow-Water Corals Under Thermal Stress: An Experimental Approach. Coral Reefs 31 (1), 41–53. doi: 10.1007/s00338-011-0817-4
Jessen C., Villa Lizcano J. F., Bayer T., Roder C., Aranda M., Wild C., et al. (2013). In-Situ Effects of Eutrophication and Overfishing on Physiology and Bacterial Diversity of the Red Sea Coral Acropora Hemprichii. PLoS One 8 (4), e62091. doi: 10.1371/journal.pone.0062091
Jones R., Fisher R., Bessell-Browne P. (2019). Sediment Deposition and Coral Smothering. PLoS One 14 (6), 1–24. doi: 10.1371/journal.pone.0216248
Junjie R. K., Browne N. K., Erftemeijer P. L. A. A., Todd P. A. (2014). Impacts of Sediments on Coral Energetics: Partitioning the Effects of Turbidity and Settling Particles. PLoS One 9 (9), e107195. doi: 10.1371/journal.pone.0107195
Kamke J., Taylor M. W., Schmitt S. (2010). Activity Profiles for Marine Sponge-Associated Bacteria Obtained by 16S rRNA vs 16S rRNA Gene Comparisons. ISME J. 4 (4), 498–508. doi: 10.1038/ismej.2009.143
Kassambara A. (2020) Ggpubr: Ggplot2 Based Publication Ready Plots. Available at: https://rpkgs.datanovia.com/ggpubr/.
Katharios P., Seth-Smith H. M. B., Fehr A., Mateos J. M., Qi W., Richter D., et al. (2015). Environmental Marine Pathogen Isolation Using Mesocosm Culture of Sharpsnout Seabream: Striking Genomic and Morphological Features of Novel Endozoicomonas Sp. Sci. Rep. 5 (1), 17609. doi: 10.1038/srep17609
Keeley N. (2013) Litterature Review of Ecological Effects of Aquaculture. Available at: https://www.mpi.govt.nz/dmsdocument/3751/direct.
Keeley N., Laroche O., Birch M., Pochon X. (2021). A Substrate-Independent Benthic Sampler (Sibs) for Hard and Mixed-Bottom Marine Habitats: A Proof-of-Concept Study. Front. Mar. Sci. 8. doi: 10.3389/fmars.2021.627687
Kelly L. W., Williams G. J., Barott K. L., Carlson C. A., Dinsdale E. A., Edwards R. A., et al. (2014). Local Genomic Adaptation of Coral Reef-Associated Microbiomes to Gradients of Natural Variability and Anthropogenic Stressors. Proc. Natl. Acad. Sci. U. S. A. 111 (28), 10227–10232. doi: 10.1073/pnas.1403319111
Klitgaard A. B. (1995). The Fauna Associated With Outer Shelf and Upper Slope Sponges (Porifera, Demospongiae) at the Faroe Islands, Northeastern Atlantic. Sarsia 80 (1), 1–22. doi: 10.1080/00364827.1995.10413574
Kornprobst J. M., Barnathan G. (2010). Demospongic Acids Revisited. Mar. Drugs 8 (10), 2569–2577. doi: 10.3390/md8102569
Krueger F., Andrews S. R., Osborne C. S. (2011). Large Scale Loss of Data in Low-Diversity Illumina Sequencing Libraries can be Recovered by Deferred Cluster Calling. PLoS One 6 (1), 4–10. doi: 10.1371/journal.pone.0016607
Kubinec R., Blaško J., Górová R., Addová G., Ostrovský I., Amann A., et al. (2011). Equivalent Chain Lengths of All C4-C23 Saturated Monomethyl Branched Fatty Acid Methyl Esters on Methylsilicone OV-1 Stationary Phase. J. Chromatogr. A 1218 (13), 1767–1774. doi: 10.1016/j.chroma.2011.01.065
Lader P., Kristiansen D., Alver M., Bjelland H. V., Myrhaug D. (2017). Classification of Aquaculture Locations in Norway With Respect to Wind Wave Exposure. Ocean Space Util. 6, 1–10. doi: 10.1115/OMAE2017-61659
Lee R. F., Hagen W., Kattner G. (2006). Lipid Storage in Marine Zooplankton. Mar. Ecol. Prog. Ser. 307 (1863), 273–306. doi: 10.3354/meps307273
Lekang O. I., Salas-Bringas C., Bostock J. C. (2016). Challenges and Emerging Technical Solutions in on-Growing Salmon Farming. Aquac. Int. 24 (3), 757–766. doi: 10.1007/s10499-016-9994-z
Leys S. P. (2013). Effects of Sediment on Glass Sponges (Porifera, Hexactinellida) and Projected Effects on Glass Sponge Reefs. Can. Sci. Advis. Secr. Res. Document 074 (November), 23.
Li Z. (2019). “Sponge and Coral Microbiomes,” in Symbiotic Microbiomes of Coral Reefs Sponges and Corals (Dordrecht: Springer Netherlands), 17–28. doi: 10.1007/978-94-024-1612-1_2
Lohrer A., Hewitt J., Thrush S. (2006). Assessing Far-Field Effects of Terrigenous Sediment Loading in the Coastal Marine Environment. Mar. Ecol. Prog. Ser. 315, 13–18. doi: 10.3354/meps315013
Love M. I., Anders S., Huber W. (2014). Differential Analysis of Count Data - the DESeq2 Package. Genome Biol. 15 (12), 550. doi: 10.1186/s13059-014-0550-8
Maldonado M., Aguilar R., Bannister R. J., Bell J. J., Conway K. W., Dayton P. K., et al. (2015). “Sponge Grounds as Key Marine Habitats: A Synthetic Review of Types, Structure, Functional Roles, and Conservation Concerns,” in Marine Animal Forests (Cham: Springer International Publishing), 1–39. doi: 10.1007/978-3-319-17001-5_24-1
Maldonado M., Giraud K., Carmona C. (2008). Effects of Sediment on the Survival of Asexually Produced Sponge Recruits. Mar. Biol. 154 (4), 631–641. doi: 10.1007/s00227-008-0956-5
Mann S., Chen Y. P. P. (2010). Bacterial Genomic G + C Composition-Eliciting Environmental Adaptation. Genomics 95 (1), 7–15. doi: 10.1016/j.ygeno.2009.09.002
Martin M. (2011). Cutadapt Removes Adapter Sequences From High-Throughput Sequencing Reads. EMBnet J. 17 (1), 10. doi: 10.14806/ej.17.1.200
McDevitt-Irwin J. M., Baum J. K., Garren M., Vega Thurber R. L. (2017). Responses of Coral-Associated Bacterial Communities to Local and Global Stressors. Front. Mar. Sci. 4 (AUG). doi: 10.3389/fmars.2017.00262
McEwan C. E. A., Gatherer D., McEwan N. R. (1998). Nitrogen-Fixing Aerobic Bacteria Have Higher Genomic GC Content Than non-Fixing Species Within the Same Genus. Hereditas 128 (2), 173–178. doi: 10.1111/j.1601-5223.1998.00173.x
Meier S., Mjøs S. A., Joensen H., Grahl-Nielsen O. (2006). Validation of a One-Step Extraction/Methylation Method for Determination of Fatty Acids and Cholesterol in Marine Tissues. J. Chromatogr. A 1104 (1–2), 291–298. doi: 10.1016/j.chroma.2005.11.045
Mendoza M., Güiza L., Martinez X., Caraballo X., Rojas J., Aranguren L., et al. (2013). A Novel Agent (Endozoicomonas Elysicola) Responsible for Epitheliocystis in Cobia Rachycentrum Canadum Larvae. Dis. Aquat. Org. 106 (1), 31–37. doi: 10.3354/dao02636
Meron D., Atias E., Iasur Kruh L., Elifantz H., Minz D., Fine M., et al. (2011). The Impact of Reduced pH on the Microbial Community of the Coral Acropora Eurystoma. ISME J. 5 (1), 51–60. doi: 10.1038/ismej.2010.102
Moitinho-Silva L., Bayer K., Cannistraci C. V., Giles E. C., Ryu T., Seridi L., et al. (2014). Specificity and Transcriptional Activity of Microbiota Associated With Low and High Microbial Abundance Sponges From the Red Sea. Mol. Ecol. 23 (6), 1348–1363. doi: 10.1111/mec.12365
Moitinho-Silva L., Nielsen S., Amir A., Gonzalez A., Ackermann G. L., Cerrano C., et al. (2017). The Sponge Microbiome Project. GigaScience 6 (10), 1–7. doi: 10.1093/gigascience/gix077
Molina V., Fernández C. (2020). Bacterioplankton Response to Nitrogen and Dissolved Organic Matter Produced From Salmon Mucus. MicrobiologyOpen 9 (12), 1–11. doi: 10.1002/mbo3.1132
Morrow K. M., Moss A. G., Chadwick N. E., Liles M. R. (2012). Bacterial Associates of Two Caribbean Coral Species Reveal Species-Specific Distribution and Geographic Variability. Appl. Environ. Microbiol. 78 (18), 6438–6449. doi: 10.1128/AEM.01162-12
Musto H., Naya H., Zavala A., Romero H., Alvarez-Valín F., Bernardi G. (2004). Correlations Between Genomic GC Levels and Optimal Growth Temperatures in Prokaryotes. FEBS Lett. 573 (1–3), 73–77. doi: 10.1016/j.febslet.2004.07.056
Naya H., Romero H., Zavala A., Alvarez B., Musto H. (2002). Aerobiosis Increases the Genomic Guanine Plus Cytosine Content (Gc%) in Prokaryotes. J. Mol. Evol. 55 (3), 260–264. doi: 10.1007/s00239-002-2323-3
Neave M. J., Michell C. T., Apprill A., Voolstra C. R. (2017). Endozoicomonas Genomes Reveal Functional Adaptation and Plasticity in Bacterial Strains Symbiotically Associated With Diverse Marine Hosts. Sci. Rep. 7 (October 2016), 1–12. doi: 10.1038/srep40579
Nishijima M., Adachi K., Katsuta A., Shizuri Y., Yamasato K. (2013). Endozoicomonas Numazuensis sp. nov., a Gammaproteobacterium Isolated From Marine Sponges, and Emended Description of the Genus Endozoicomonas Kurahashi and Yokota 2007. Int. J. Syst. Evol. Microbiol. 63 (Pt_2), 709–714. doi: 10.1099/ijs.0.042077-0
Nissimov J., Rosenberg E., Munn C. B. (2009). Antimicrobial Properties of Resident Coral Mucus Bacteria of Oculina Patagonica. FEMS Microbiol. Lett. 292 (2), 210–215. doi: 10.1111/j.1574-6968.2009.01490.x
Núñez-Pons L., Carbone M., Paris D., Melck D., Ríos P., Cristobo J., et al. (2012). Chemo-Ecological Studies on Hexactinellid Sponges From the Southern Ocean. Naturwissenschaften 99 (5), 353–368. doi: 10.1007/s00114-012-0907-3
Oksanen J., Blanchet F. G., Friendly M., Kindt R., Legendre P., McGlinn D., et al. (2019) Vegan: Community Ecology Package. Available at: https://cran.r-project.org/package=vegan.
O’Leary N. A., Wright M. W., Brister J. R., Ciufo S., Haddad D., McVeigh R., et al. (2016). Reference Sequence (RefSeq) Database at NCBI: Current Status, Taxonomic Expansion, and Functional Annotation. Nucleic Acids Res. 44 (D1), D733–D745. doi: 10.1093/nar/gkv1189
Oliveira B. F. R., Lopes I. R., Canellas A. L. B., Muricy G., Dobson A. D. W., Laport M. S. (2020). Not That Close to Mommy: Horizontal Transmission Seeds the Microbiome Associated With the Marine Sponge Plakina Cyanorosea. Microorganisms 8 (12), 1978. doi: 10.3390/microorganisms8121978
Philipp E., Fabricius K. (2003). Photophysiological Stress in Scleractinian Corals in Response to Short-Term Sedimentation. J. Exp. Mar. Biol. Ecol. 287 (1), 57–78. doi: 10.1016/S0022-0981(02)00495-1
Pineda M. C., Strehlow B., Sternel M., Duckworth A., Jones R., Webster N. S. (2017). Effects of Suspended Sediments on the Sponge Holobiont With Implications for Dredging Management. Sci. Rep. 7 (1), 1–15. doi: 10.1038/s41598-017-05241-z
Pita L., Fraune S., Hentschel U. (2016). Emerging Sponge Models of Animal-Microbe Symbioses. Front. Microbiol. 7 (DEC). doi: 10.3389/fmicb.2016.02102
Price C., Black K. D., Hargrave B. T., Morris J. A. (2014). Marine Cage Culture and the Environment: Effects on Water Quality and Primary Production. Aquac. Environ. Interact. 6 (2), 151–174. doi: 10.3354/aei00122
Raghavan R., Kelkar Y. D., Ochman H. (2012). A Selective Force Favoring Increased G+C Content in Bacterial Genes. Proc. Natl. Acad. Sci. U. S. A. 109 (36), 14504–14507. doi: 10.1073/pnas.1205683109
Ribes M., Calvo E., Movilla J., Logares R., Coma R., Pelejero C. (2016). Restructuring of the Sponge Microbiome Favors Tolerance to Ocean Acidification. Environ. Microbiol. Rep. 8 (4), 536–544. doi: 10.1111/1758-2229.12430
Riegl B., Branch G. M. (1995). Effects of Sediment on the Energy Budgets of Four Scleractinian (Bourne 1900) and Five Alcyonacean (Lamouroux 1816) Corals. J. Exp. Mar. Biol. Ecol. 186 (2), 259–275. doi: 10.1016/0022-0981(94)00164-9
Ritchie K. (2006). Regulation of Microbial Populations by Coral Surface Mucus and Mucus-Associated Bacteria. Mar. Ecol. Prog. Ser. 322, 1–14. doi: 10.3354/meps322001
Rod’kina S. A. (2003). Comparative Characterization of the Microbial Community in Two Species of Sponges From Sea of Japan Using Fatty Acid Markers. Russian J. Mar. Biol. 29 (4), 255–258. doi: 10.1023/A:1025441111489
Rod’kina S. A. (2005). Fatty Acids and Other Lipids of Marine Sponges. Russian J. Mar. Biol. 31 (SUPPL. 1), S49–S60. doi: 10.1007/s11179-006-0015-3
Rossbach S., Cardenas A., Perna G., Duarte C. M., Voolstra C. R. (2019). Tissue-Specific Microbiomes of the Red Sea Giant Clam Tridacna Maxima Highlight Differential Abundance of Endozoicomonadaceae. Front. Microbiol. 10 (November). doi: 10.3389/fmicb.2019.02661
Salvo F., Hamoutene D., Hayes V. E. W., Edinger E. N., Parrish C. C. (2018). Investigation of Trophic Ecology in Newfoundland Cold-Water Deep-Sea Corals Using Lipid Class and Fatty Acid Analyses. Coral Reefs 37 (1), 157–171. doi: 10.1007/s00338-017-1644-z
Sarà G., Scilipoti D., Milazzo M., Modica A. (2006). Use of Stable Isotopes to Investigate Dispersal of Waste From Fish Farms as a Function of Hydrodynamics. Mar. Ecol. Prog. Ser. 313 (May), 261–270. doi: 10.3354/meps313261
Schirmer K., Fischer B. B., Madureira D. J., Pillai S. (2010). Transcriptomics in Ecotoxicology. Anal. Bioanal. Chem. 397 (3), 917–923. doi: 10.1007/s00216-010-3662-3
Schmitt S., Deines P., Behnam F., Wagner M., Taylor M. W. (2011). Chloroflexi Bacteria are More Diverse, Abundant, and Similar in High Than in Low Microbial Abundance Sponges. FEMS Microbiol. Ecol. 78 (3), 497–510. doi: 10.1111/j.1574-6941.2011.01179.x
Schmitt S., Wehrl M., Lindquist N., Weisz J. B. (2007). “Morphological and Molecular Analyses of Microorganisms in Caribbean Reef Adult Sponges and in Corresponding Reproductive Material,” in Porifera Research Biodiversity, Innovation and Sustainability. Eds. Custodio M., Lobo-Hajdu G., Hajdu E., Muricy G. (Rio de Janeiro: Museu Nacional), 561–568. Available at: http://oceanrep.geomar.de/29359/.
Schöttner S., Hoffmann F., Cárdenas P., Rapp H. T., Boetius A., Ramette A. (2013). Relationships Between Host Phylogeny, Host Type and Bacterial Community Diversity in Cold-Water Coral Reef Sponges. PLoS One 8 (2), 1–11. doi: 10.1371/journal.pone.0055505
Sebens K. P. (1991). “Habitat Structure and Community Dynamics in Marine Benthic Systems,” in Habitat Structure: The Physical Arrangement of Objects in Space. Eds. Bell S. S., McCoy E. D., Mushinsky H. R. (Dordrecht: Springer Netherlands), 211–234. doi: 10.1007/978-94-011-3076-9_11
Sun J., Hamel J. F., Gianasi B. L., Graham M., Mercier A. (2020). Growth, Health and Biochemical Composition of the Sea Cucumber Cucumaria Frondosa After Multi-Year Holding in Effluent Waters of Land-Based Salmon Culture. Aquac. Environ. Interact. 12 (April), 139–151. doi: 10.3354/aei00356
Sutherland T. F., Sterling A. M., Ou M. (2018). Influence of Salmonid Aquaculture Activities on a Rock-Cliff Epifaunal Community in Jervis Inlet, British Columbia. Mar. Pollut. Bull. 127 (March 2017), 297–309. doi: 10.1016/j.marpolbul.2017.12.005
Thomas T., Moitinho-Silva L., Lurgi M., Björk J. R., Easson C., Astudillo-García C., et al. (2016). Diversity, Structure and Convergent Evolution of the Global Sponge Microbiome. Nat. Commun. 7 (May), 11870. doi: 10.1038/ncomms11870
Thompson J. R., Rivera H. E., Closek C. J., Medina M. (2014). Microbes in the Coral Holobiont: Partners Through Evolution, Development, and Ecological Interactions. Front. Cell. Infect. Microbiol. 4 (DEC). doi: 10.3389/fcimb.2014.00176
Tocher D. R. (2003). Metabolism and Functions of Lipids and Fatty Acids in Teleost Fish. Rev. Fisheries Sci. 11 (2), 107–184. doi: 10.1080/713610925
Tompkins-MacDonald G. J., Leys S. P. (2008). Glass Sponges Arrest Pumping in Response to Sediment: Implications for the Physiology of the Hexactinellid Conduction System. Mar. Biol. 154 (6), 973–984. doi: 10.1007/s00227-008-0987-y
van Oppen M. J. H., Blackall L. L. (2019). Coral Microbiome Dynamics, Functions and Design in a Changing World. Nat. Rev. Microbiol. 17 (9), 557–567. doi: 10.1038/s41579-019-0223-4
van Soest R. W. M. M., Boury-Esnault N., Vacelet J., Dohrmann M., Erpenbeck D., de Voogd N. J., et al. (2012). Global Diversity of Sponges (Porifera). PLoS One 7 (4), e35105. doi: 10.1371/journal.pone.0035105
Verhoeven J. T. P., Salvo F., Knight R., Hamoutene D., Dufour S. C. (2018). Temporal Bacterial Surveillance of Salmon Aquaculture Sites Indicates a Long Lasting Benthic Impact With Minimal Recovery. Front. Microbiol. 9 (DEC). doi: 10.3389/fmicb.2018.03054
Verma J., Bag S., Saha B., Kumar P., Ghosh T. S., Dayal M., et al. (2019). Genomic Plasticity Associated With Antimicrobial Resistance in Vibrio Cholerae. Proc. Natl. Acad. Sci. U. S. A. 116 (13), 6226–6231. doi: 10.1073/pnas.1900141116
Wang Q., Garrity G. M., Tiedje J. M., Cole J. R. (2007). Naive Bayesian Classifier for Rapid Assignment of Rrna Sequences Into the New Bacterial Taxonomy. Appl. Environ. Microbiol. 73 (16), 5261–5267. doi: 10.1128/AEM.00062-07
Wang J. H., Lu J., Zhang Y. X., Wu J., Zhang C., Yu X., et al. (2018). High-Throughput Sequencing Analysis of the Microbial Community in Coastal Intensive Mariculture Systems. Aquac. Eng. 83 (June), 93–102. doi: 10.1016/j.aquaeng.2018.10.001
Wang H.-C., Susko E., Roger A. J. (2006). On the Correlation Between Genomic G+C Content and Optimal Growth Temperature in Prokaryotes: Data Quality and Confounding Factors. Biochem. Biophys. Res. Commun. 342 (3), 681–684. doi: 10.1016/j.bbrc.2006.02.037
Wasta Z., Mjøs S. A. (2013). A Database of Chromatographic Properties and Mass Spectra of Fatty Acid Methyl Esters From Omega-3 Products. J. Chromatogr. A 1299, 94–102. doi: 10.1016/j.chroma.2013.05.056
Weisz J. B., Hentschel U., Lindquist N., Martens C. S. (2007). Linking Abundance and Diversity of Sponge-Associated Microbial Communities to Metabolic Differences in Host Sponges. Mar. Biol. 152 (2), 475–483. doi: 10.1007/s00227-007-0708-y
Wemheuer F., Taylor J. A., Daniel R., Johnston E., Meinicke P., Thomas T., et al. (2020). Tax4Fun2: Prediction of Habitat-Specific Functional Profiles and Functional Redundancy Based on 16S rRNA Gene Sequences. Environ. Microbiomes 15 (1). doi: 10.1186/s40793-020-00358-7
White C. A., Woodcock S. H., Bannister R. J., Nichols P. D. (2019). Terrestrial Fatty Acids as Tracers of Finfish Aquaculture Waste in the Marine Environment. Rev. Aquac. 11 (1), 133–148. doi: 10.1111/raq.12230
Wickham H. (2016). Ggplot2: Elegant Graphics for Data Analysis. Available at: http://ggplot2.org.
Woodcock D. J., Krusche P., Strachan N. J. C., Forbes K. J., Cohan F. M., Méric G., et al. (2017b). Genomic Plasticity and Rapid Host Switching can Promote the Evolution of Generalism: A Case Study in the Zoonotic Pathogen Campylobacter. Sci. Rep. 7 (1), 1–13. doi: 10.1038/s41598-017-09483-9
Woodcock S., Troedsson C., Strohmeier T., Balseiro P., Skaar K., Strand Ø (2017a). Combining Biochemical Methods to Trace Organic Effluent From Fish Farms. Aquac. Environ. Interact. 9, 429–443. doi: 10.3354/aei00242
Woodcock S. H., Meier S., Keeley N. B., Bannister R. J. (2019). Fate and Longevity of Terrestrial Fatty Acids From Caged Fin-Fish Aquaculture in Dynamic Coastal Marine Systems. Ecol. Indic. 103 (March), 43–54. doi: 10.1016/j.ecolind.2019.03.057
Yakovchuk P., Protozanova E., Frank-Kamenetskii M. D. (2006). Base-Stacking and Base-Pairing Contributions Into Thermal Stability of the DNA Double Helix. Nucleic Acids Res. 34 (2), 564–574. doi: 10.1093/nar/gkj454
Yang Q., Franco C. M. M. M., Lin H.-W. W., Zhang W. (2019). Untapped Sponge Microbiomes: Structure Specificity at Host Order and Family Levels. FEMS Microbiol. Ecol. 95 (9), 1–16. doi: 10.1093/femsec/fiz136
Yang Q., Zhang W., Franco C. M. M. (2019). “Response of Sponge Microbiomes to Environmental Variations,” in Symbiotic Microbiomes of Coral Reefs Sponges and Corals (Dordrecht: Springer Netherlands), 181–247. doi: 10.1007/978-94-024-1612-1_11
Yokoyama H., Abo K., Ishihi Y. (2006). Quantifying Aquaculture-Derived Organic Matter in the Sediment in and Around a Coastal Fish Farm Using Stable Carbon and Nitrogen Isotope Ratios. Aquaculture 254 (1–4), 411–425. doi: 10.1016/j.aquaculture.2005.10.024
Zheng H., Wu H. (2010). Gene-Centric Association Analysis for the Correlation Between the Guanine-Cytosine Content Levels and Temperature Range Conditions of Prokaryotic Species. BMC Bioinf. 11 (S11), S7. doi: 10.1186/1471-2105-11-S11-S7
Ziegler M., Grupstra C. G. B., Barreto M. M., Eaton M., BaOmar J., Zubier K., et al. (2019). Coral Bacterial Community Structure Responds to Environmental Change in a Host-Specific Manner. Nat. Commun. 10 (1), 3092. doi: 10.1038/s41467-019-10969-5
Keywords: metabarcoding, 16S rRNA, organic enrichment, functional profiling, metataxonomics
Citation: Laroche O, Meier S, Mjøs SA and Keeley N (2022) Suspension-Feeding Benthic Species’ Physiological and Microbiome Response to Salmon Farming and Associated Environmental Changes. Front. Mar. Sci. 9:841806. doi: 10.3389/fmars.2022.841806
Received: 22 December 2021; Accepted: 03 March 2022;
Published: 28 March 2022.
Edited by:
Oliver Nicholas Shipley, University of New Mexico, United StatesReviewed by:
Michael Phillips, WorldFish, MalaysiaCopyright © 2022 Laroche, Meier, Mjøs and Keeley. This is an open-access article distributed under the terms of the Creative Commons Attribution License (CC BY). The use, distribution or reproduction in other forums is permitted, provided the original author(s) and the copyright owner(s) are credited and that the original publication in this journal is cited, in accordance with accepted academic practice. No use, distribution or reproduction is permitted which does not comply with these terms.
*Correspondence: Olivier Laroche, T2xpdmllci5sYXJvY2hlQGNhd3Rocm9uLm9yZy5ueg==
Disclaimer: All claims expressed in this article are solely those of the authors and do not necessarily represent those of their affiliated organizations, or those of the publisher, the editors and the reviewers. Any product that may be evaluated in this article or claim that may be made by its manufacturer is not guaranteed or endorsed by the publisher.
Research integrity at Frontiers
Learn more about the work of our research integrity team to safeguard the quality of each article we publish.