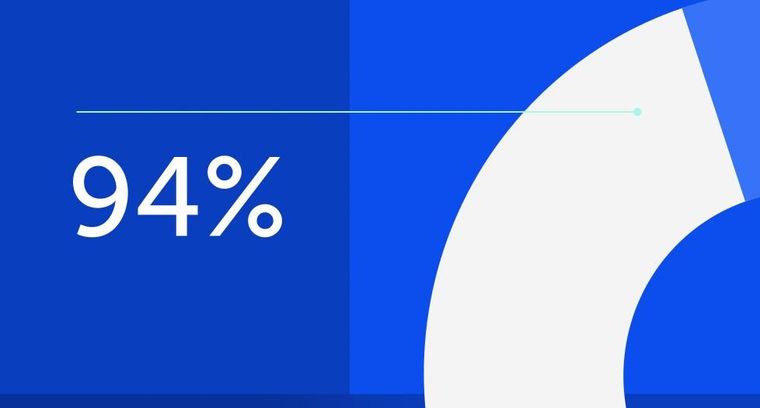
94% of researchers rate our articles as excellent or good
Learn more about the work of our research integrity team to safeguard the quality of each article we publish.
Find out more
ORIGINAL RESEARCH article
Front. Mar. Sci., 13 June 2022
Sec. Marine Conservation and Sustainability
Volume 9 - 2022 | https://doi.org/10.3389/fmars.2022.827590
This article is part of the Research TopicIdentifying and Comparing Important Areas for Marine Sustainable Use and ConservationView all 19 articles
Studies of mangrove population connectivity have focused primarily on global to regional scales and have suggested potential for long-distance connectivity, with archipelagos serving as stepping stones for trans-oceanic dispersal. However, the contribution of propagule dispersal to connectivity is still largely unknown, especially at local-scale. Identifying fine-scale propagule dispersal patterns unique to individual island systems is important to understand their contribution to global species distributions, and to select appropriate sizes and locations for mangrove conservation in archipelagos. Using population genetic methods and a release-recapture method employing GPS drifting buoys, we investigated the spatiotemporal scale of propagule dispersal of Rhizophora stylosa, one of the widely distributed mangrove species in the Indo-West Pacific. This study sought to quantify intra- and inter-island connectivity and to assess their contributions to oceanic scale dispersal of R. stylosa from the Ryukyu Archipelago, which spans over 545 km in southwestern Japan. Using 7 microsatellite markers, we tested 354 samples collected from 16 fringing populations on 4 islands. We identified 3 genetic populations, indicating distinct genetic structures comprising 3 distinguishable bioregions (genetic clusters). The western end of the archipelago receives relatively frequent migration (m > 0.1), but is genetically isolated from other sites. Based on genetic migration rates, we found that the central area of the archipelago serves as a stepping stone for southwestward, but not northeastward dispersal. On the other hand, with in-situ drifting buoys, we did not confirm prevailing dispersal directionality within the archipelago, instead confirming local eddies. Some buoys trapped in those eddies demonstrated potential for successful beaching from another island. A large portion of buoys were carried predominantly northeastward by the Kuroshio Current and drifted away from the coastal areas into the Pacific, contrary to local migrations. We found that the spatiotemporal scale of propagule dispersal is limited by the distance between islands (< 200km), propagule viability duration, and fecundity. Over all, recruitment does not occur frequently enough to unify the genetic structure in the archipelago, and the Ryukyu Archipelago is isolated in the center of the global mangrove distribution.
The spatiotemporal scale of mangrove propagule dispersal is foundational to understand demographic connectivity among islands that support fragmented, fringing mangrove populations. Demographic connectivity is defined as the relative contribution of propagule dispersal to population growth rate in comparison to local recruitment, and it is an important concept for conservation, especially when changes in propagule translocation result in a negative shift from stable and/or positive population growth (Lowe and Allendorf, 2010). Fragmented mangrove populations are vulnerable to environmental changes such as coastal development, climate change, and sea level rise. Mangrove populations at the edges of their distribution commonly exhibit low genetic diversity suggesting their higher risk to negative effects from monomorphism and inbreeding compared to mangrove habitats in the center of the species range. Although mangrove habitats are protected through international agreements in order to mitigate the impacts of changing climate on coastal ecosystems, conversion and degradation of mangroves are continuing, especially for smaller mangrove patches (Curnick et al., 2019). Complicated and spontaneous mangrove establishment at local- and fine-scales tends to occur in island systems (Triest et al., 2021a), and some islands facilitate global distribution of mangroves by acting as stepping stones for transoceanic dispersal (Van der Stocken et al., 2019). Thus loss of small mangrove habitats on islands may impact global distribution of mangroves in the long run, if not in the near term.
While demographic connectivity is important to sustain population sizes, to colonize unoccupied niches, and to assemble local communities from the meta-community (Levin et al., 2003; Levine and Murrell, 2003), the effects of population loss or degradation on other populations in the same island system are not well understood. Thus, understanding the spatiotemporal scale of propagule dispersal should help estimate demographic connectivity among islands, thereby helping to design effective protected areas.
Genetic methods have been used to understand spatial scales of population connectivity. Previous genetic studies have supported transoceanic connectivity, and have identified bioregions indicating genetic discontinuities among distant populations over biogeographic timescales (Dodd et al., 2002; Takayama et al., 2013; Lo et al., 2014). Spatial patterns of genetic discontinuity have been compared with ocean currents, prevailing winds, landscapes, and seascapes to understand mechanisms of dispersal that cause them. For example, the Central American Isthmus was suggested as a land barrier for dispersal of Rhizophora mangle and Rhizophora racemosa (Takayama et al., 2013), as was the Mexican coast for Avicenna germinans and R. mangle (Sandoval-Castro et al., 2012). Others have shown that mangrove connectivity is related to oceanic currents, particularly their direction along coastlines, and that migration routes follow major oceanic and coastal currents (Triest et al., 2021a).
On the other hand, mangrove propagule dispersal in island systems has received less attention than continental coastal dispersal. A few studies on mangrove genetic connectivity in island systems have suggested low genetic variation and limited genetic connectivity (Islam et al., 2012; Islam et al., 2014; Yahya et al., 2014; Al-Qthanin and Alharbi, 2020). Except for Yahya et al. (2014), a study on the Sunda Islands in Indonesia, archipelagos investigated in previous studies were located near the distributional edges of the studied species, where species are subjected to more genetic drift and environmental selection compared to those in central regions (Arnaud-Haond et al., 2006). Those studies also suggest that discreteness of island coastlines is a potential cause of limited genetic variation, regardless of distributional area. However, because of the paucity of studies in island systems, differences in studied species and different studied area sizes, more island studies with various species are needed to understand demographic connectivity in archipelagos.
Although genetic methods can detect the outcome of connectivity interference caused by physical processes such as hydrochory (dispersal via water), genetic information alone is insufficient to estimate spatiotemporal scales of connectivity (Lowe and Allendorf, 2010). Knowledge of propagule immigration revealed by genetic data, combined with physical oceanographic information obtained from numerical ocean modeling and/or empirical release-recapture methods, have been used to investigate demographic connectivity (Nathan and Muller-Landau, 2000; Nathan et al., 2008; Ngeve et al., 2017). In the past decade, with advances of computational power and modeling processes, numerical ocean modeling has been extensively incorporated into dispersal and connectivity studies (Fox-Kemper et al., 2019) including mangrove connectivity studies to interpret genetic results at regional and global scales (Pil et al., 2011; Wee et al., 2014; Ngeve et al., 2016; Geng et al., 2021). For example, a previous biophysical approach suggested an oceanic barrier between the Andaman Sea and the Malacca Strait around the Malay Peninsula for dispersal of Rhizophora mucronata by interpreting genetic outcomes with simulated ocean currents (Wee et al., 2014). Ngeve et al. (2016) identified an oceanic barrier at an oceanic conversion zone near the Cameroon Estuary Complex for dispersal of R. racemosa using a genetic approach with numerical particle tracking models. Van der Stocken et al. (2019) quantified potential for long-distance propagule dispersal at global scale using a Lagrangian particle tracking model. They found along-coast transport dominant over many continental coastlines, as well as stepping-stone dispersal via several Pacific island systems (the Galapagos Islands, Polynesia, Micronesia, and Melanesia) that allow for trans-oceanic dispersal. Their findings suggested potential effects of minimum and maximum floating periods of propagules in determining the extent of dispersal. However, detailed spatiotemporal studies of dispersal are not yet understood for island systems.
Therefore, this project was designed to investigate the spatiotemporal scale of propagule transport among islands. The model site was the southern half of the Ryukyu Archipelago (Figure 1A), a poleward peripheral region of the studied species, Rhizophora stylosa (Rhizophoraceae) in the northern hemisphere. The total area of mangrove in Japan is about 553 ha, from the Ryukyu Archipelago to Kagoshima Prefecture (31’20’N) of which 80% occurs on Iriomote island (Figure 1C) with 7 - 19 species (Minagawa, 2000). According to radiocarbon studies on one of the largest rivers on Iriomote Island (Urauchi River; 7.45 km), the present mangrove forests were established over 1,000 years ago (Fujimoto et al., 2015). Depending on locations in the archipelago, development of mangrove habitats varies between 400 years BP and 3,500 years BP (Fujimoto and Ohnuki, 1995; Fujimoto et al., 2015), which is relatively recent compared to global mangrove establishment. During the establishment period, the sea level was the highest between 1,700 and 5,100 years BP, and declined to the current sea level in the southern part of the archipelago about 1,000 years BP (Yamano et al., 2019). The archipelago is located 150~200 km to the east of the Kuroshio western boundary current flowing northeastward (Figure 1A). The previous oceanographic study regarding coral larval dispersal around the archipelago showed that most particles are carried southward by the Kuroshio Counter Current (Figure 1A) from Spring to Fall, and eastward transport of particles from the Kuroshio toward the western coast of Okinawa Island occurs primarily in warm seasons (Uchiyama et al., 2018). Additionally, the probability of long-distance dispersal (LDD) maybe largely influenced by oceanic conditions south of the archipelago, indicating complex surface hydrodynamics in the region (Uchiyama et al., 2018).
Figure 1 The Ryukyu Archipelago is located in southwestern Japan, spanning 545 km (A). Okinawa, Iriomote, Ishigaki, and Miyako Islands served as study sites. Each filled circle shows sampling sites with their location IDs. All sampling sites were natural, non-riverine coastal habitats (B), except for site OKI (C) which is located in a river mouth, and is the most northern site for R. stylosa. * shows the location of a deployment site for drifting buoys with the number of buoys deployed for in situ experiments. KCC (Kuroshio Counter Current).
Sampling sites for the current study were focused on fringing, non-riverine mangrove habitats (Figure 1B). We predicted that non-riverine sites proximal to the open ocean would facilitate island-to-island propagule transport, based on findings of previous studies (Kadoya and Inoue, 2015; Triest et al., 2021a). Rhizophora stylosa was chosen as the model species for this study, based on its propagule traits. Rhizophora stylosa propagules have higher dispersal capacity than other mangrove species in the Ryukyu Archipelago (e.g. Bruguiera gymnorhiza and Kandelia obovata), which is determined mainly by positive buoyancy upon detaching from parent trees, the length of the viable period while submerged in water, and root initiation time while floating (Kadoya and Inoue, 2015; Wang et al., 2019). By comparing those dispersal properties, Rhizophora appears to have great potential for LDD, especially R. mangle and R. stylosa because of their long floating periods (over 100 days in the laboratory) (Rabinowitz, 1978). They also have the capacity to tolerate high salinity and dryness (Clarke et al., 2001; Kadoya and Inoue, 2015, respectively), and to maintain a vertical orientation that is favorable for dispersal maneuvering between roots and landscapes of mangrove forests more easily compared to horizontal orientation (Van der Stocken et al., 2015). However, reproduction via propagules is significantly lower than those of other tropical and subtropical coastal species, such as seagrass and corals. Fecundity of mangrove species depends on the reproductive stage. Coupland et al. (2006) studied 20 reproductive shoots and studied 222 young buds from 10 trees. They showed a poor rate of pollination (0.5%), which is accomplished by wind (Yan et al., 2016), as well as a poor conversion rate (< 3%) from flowers to mature propagules, which resulted in an extremely low propagule fertility rate. Such low conversion rate of R.stylosa was found in a study on litter in mangrove forests in Okinawa Island as well (Sharma et al., 2011). Producing large propagules, like those of R.stylosa, is metabolically expensive; therefore, not only restricted pollination, but also resource limitation interferes with propagule reproduction (Coupland et al., 2006). Hence, the species propagule fecundity appears to be substantially low, however is yet understudied. It has also been argued that the efficiency of wind pollination is low in fragmented habitats (Ngeve et al., 2016). Hence, the primary method for mangrove gene exchange seems to be propagule dispersal.
We used 7 microsatellite markers for 354 samples collected from 16 populations on 4 islands, and obtained spatial information regarding genetic connectivity and migration rates to quantify potential for propagule transport between every site pair over several generations. To determine the temporal scale of dispersal trajectories and to quantify potential for direct dispersal within the archipelago, we used a release-recapture method employing GPS tracking-drifting buoys. These buoys float along the surface current and provide spatiotemporal information about geographic position until they beach or turn off due to battery exhaustion. Using ocean current data combined with genetic data and propagule ecological information, we estimated demographic connectivity among islands with detailed information about propagule dispersal.
Sampling sites included 16 sites on 4 islands spanning 545 km (Figure 1A). The area of individual sites ranged from 0.04 ha to 8 ha, small in comparison with other tropical mangrove populations. All sample sites were natural habitats where no mangrove reforestation had occurred, according to local records. Okinawa Island (Figure 1C) is R. stylosa’s most northern habitat (Spalding et al., 2010), and has been protected by the Ministry of the Environment in Japan. Iriomote Island (Figure 1C) has been protected since 1972 as a Japanese National Monument. All sampling sites, except for one riverine habitat in Okinawa Island are fringing habitats with tree heights from 1.5 m to 5m. We collected leaf samples from 20 to 40 trees that were 5 to10 meters apart in order to avoid sampling overlap. We cut leaves from their petioles, and immediately preserved them in sealable bags with silica gel to dehydrate them. In order to completely dehydrate them within 24 h, we discarded the main veins of leaves and cut the leaves into smaller pieces within 12 h after collection. After samples were brought back to the lab, they were kept in plastic bags with silica gel, sealed in a container, and kept at 25°C until DNA extraction.
For genomic DNA extraction, 0.03–0.05 g of each leaf was homogenized with zirconia beads using a cell disrupter (Tommy Micro Smash MS-100) at 2,500 rpm for two cycles of 29 s to achieve evenly homogenized specimens. Then, we used a QIAGEN DNeasy Plant Kit to extract DNA, and quantified collected DNA with a NanoDrop™ 1000 Spectrophotometer (Thermo Fisher Scientific, Waltham, MA, USA) before storing it at -20°C until use. We analyzed polymorphisms of isolated loci for 354 samples from 16 populations with 11 nuclear microsatellite markers (ncSSR) of which 8 ncSSR markers (Rhst01, Rhst02, Rhst13, Rhst15, Rhst16, Rhst19, Rhst20, Rhst27) were developed by Islam et al. (2004), and the other 3 ncSSR (RM110, RM107, RM121) were developed by Shinmura et al. (2012). Seven loci (Rhst01, Rhst15, Rhst19, Rhst20, Rhst27, RM107, RM121) were successfully amplified and showed polymorphisms under the following conditions.
Polymerase chain reactions (PCR) were performed in 10-µL reactions containing 10 ng/µL DNA template, QIAGEN Multiplex Master Mix, 0.2 µM of each primer pair, 0.2 µM of each fluorescence-labeled probe, and RNase-free H2O. PCR cycle conditions were 15 min at 94°C, followed by 35 cycles of 30 s at 94°C, 90 s at 60°C annealing temperature, and 60 s at 72°C in the final cycle. For samples that failed to amplify with the method above, each locus was amplified individually. PCR solution in this case was 10 µL which consisted of 1 µL DNA template, 5 µL of QIAGEN AmpliTaq Gold 360 Master Mix, 1.5 µL MilliQ water, and 0.2 mol primer pair and fluorescent-labeled probe. PCR cycle conditions for AmpliTaq included 5 min at 90°C initially, followed by 35 cycles of 30 s at 95°C, 30 s at 50°C annealing temperature, and 60 s at 72°C in the final cycle with a final extension at 72°C for 10 min. PCR products were then diluted 5x with MilliQ water and applied to a 3130 xl Genetic Analyzer by Applied Biosystems to analyze allelic variation. Fragment sizes were analyzed with Geneious 11.0.3 (Biomatters Ltd., Auckland, New Zealand) relative to internal lane size standards using a GeneScanTM 600 LIZ® (Thermo Fisher Scientific).
Prior to population genetic analysis, we tested the probability of identity (PI) and linkage disequilibrium of genetic data. PI was tested to determine whether any two individuals accidentally shared an identical multilocus genotype, to derive a cumulative probability of identity for all polymorphic loci in each population using GenAlEx 6.5 (Peakall and Smouse, 2012), and gave a total value of 2.9 x 10-4 to 5.4 x 10-1 for all polymorphic loci in each site. Linkage disequilibrium for each locus pair across all populations was tested using Fisher’s method on GENEPOP on the web (Raymond and Rousset, 1995; Rousset, 2008), and detected significant linkage disequilibrium (P < 0.01) for Rhst15 & Rhst20 (MYKc, ISGa, IRMe), Rhst01 & Rhst15 (IRMa, IRMc), Rhst01 & Rhst19 (MYKd), Rhst15 & Rhst27 (IRMd), Rhst20 & Rhst27 (IRMd), Rhst20 & RM107 (ISGa), and Rhst27 & RM107 (IRMc). In the majority of population, paired loci with significant linkage disequilibrium were not observed.
To analyze genetic diversity, we computed expected heterozygosity (He), observed heterozygosity (Ho), and pairwise population genetic differentiation (FSTP) using GenAlEx (Peakall and Smouse, 2012). FSTP < 0.05 was set as the threshold for insignificant genetic differentiation between paired sites (Frankham et al., 2010). The number of alleles (Ar) per locus and per population, and the inbreeding coefficient (FIS) for each population were analyzed using FSTAT 2.9.3 (Goudet, 2001). Allelic richness was rarefied to the smallest population size of 9 (MYKb) based on a rarefaction method (Hurlbert, 1971). To evaluate population differentiation, analysis of molecular variance (AMOVA) was conducted within and among populations using GenAlEx 6.5 (Peakall and Smouse, 2012).
To identify genetic structures of individual samples, we used a Bayesian clustering model, STRUCTURE 2.3 (Pritchard et al., 2000; Hubisz et al., 2009). Each run assigned K (1 to 18) clusters to each sample. All parameters were set to default. With a Bayesian Markov chain Monte Carlo (MCMC) resampling method, each run underwent 500,000 iterations, after discarding 80,000 as burn-in for 20 replicates. The optimal K for this dataset was determined with Structure Harvester (Earl and Holdt, 2012) using results from STRUCTURE, and the likelihood of multiple values of K was tested using the Evanno method (Evanno et al., 2005). With CLUMPAK (Kopelman et al., 2015), the entire set of runs obtained from STRUCTURE was merged for each K value, based on similarity matrices, and visualized.
To test the effect of geographic distance on genetic connectivity, we compared pairwise genetic differentiation (FSTP) and geographic distance between every population pair using Mantel’s test in GenAlEx 6.5 (Peakall and Smouse, 2012). All FSTP values were linearized (LinFST = FSTP/1-FSTP), and we measured the Euclidean distance between populations using Google Earth Pro.
To identify differences in contemporary patterns of migration (a few generations) from historical patterns of population connectivity, we estimated recent migration rates between site pairs with BayesAss 3.0.4 (Wilson and Rannala, 2003), which uses a MCMC resampling method. BayesAss assumes that migration does not change allelic frequencies over two generations, and that output migration rates reflect the most recent several generations. We computed five replicates with different random starting seeds for an MCMC run with 10,000,000 iterations, discarding the first 1,000,000 as burn-in in each run. Samples were collected every 100 observations with default parameter settings. Sample site MYKb was excluded from this analysis due to its inadequate sample size. We examined posterior mean migration rates (m) for gene flows between sites. We followed (Faubet et al., 2007) for performance analysis of the test.
31 drifting buoys were deployed in order to estimate effects of ocean hydrodynamics on propagule dispersal. Drifter deployment was carried out with one drifter per day for 31 consecutive days (except when weather or sea conditions made it unsafe to conduct field work) starting on August 7, 2018, which is the middle of fruiting season on Okinawa Island (Sharma et al., 2011), and a little after its peak in July for Iriomote Island (Kadoya and Inoue, 2015). To also avoid major typhoon season, August was reasonable time of the year for deployment. Buoys were deployed from just off the reef of IRMg on Iriomote Island (* on Figure 1C), where clear genetic isolation was detected despite the proximity of neighboring populations (< 5km to the closest population). In order to obtain ocean surface current vector information, we used Microstar drifters developed by Pacific Gyre. Microstar drifters have two parts, a surface float (20 cm in diameter) and an underwater corner-radar-reflector-type drogue (Ohlmann et al., 2005). The float maintains its drogue at a constant depth of 1 m below the surface to capture the surface current, and minimizes the effects of wind and surface waves with its spherical shape. Drogues were calibrated to provide about < 2 cm/s velocity differences from the “tagged” water parcel with little slip measurement under calm wind (< 12 m) (Ohlmann et al., 2005). Direct effects of high wind on Microstar drifters are not yet well understood. Drifter positions were determined by GPS at near-real time, using the Iridium satellite data network with user-specified up-linking intervals, which allowed us to collect spatiotemporal information of dispersal trajectories from all deployed buoys. We set the intervals so that positioning information with higher resolution would be obtained near the coast. Intervals were set from 5 min to 12 h depending on the distance from the nearest coast. Drifters can be positioned with an accuracy of < 7m (Ohlmann et al., 2005). Collected spatiotemporal data were analyzed using visualized using MATLAB.
The average number of alleles (allelic richness) per population varied depending on sites from 1.2 (OKI) to 2.7 (IRMc) (Table 1). This study showed little to no heterozygosity (Ho) in any population, and at all loci, and expected heterozygosity (He) consistently exceeded Ho for all sampling sites. The inbreeding coefficient (FIS) exceeded 0.8 (p < 0.01) for all sites, while pairwise genetic differentiation (FSTP) varied depending on sites ranging from 0.017 (IRMe-IRMf) to 0.828 (IRMg-OKI) (Figure 2). We found four site pairs (three neighboring pairs, IRMf-IRMe, ISGa-ISGc, MYKb-MYKd and one inter-island pair, IRMd-MYKc) with insignificant pairwise genetic differentiation (FSTP < 0.05) (Figure 3). The hierarchical analysis of molecular variance (AMOVA) (Excoffier et al., 1992) showed 78% of genetic variation occurred among individuals within sites, 12% of variation occurred among sites, and 10% of variation occurred among islands (p < 0.01) (Table 2).
Figure 2 Pairwise genetic differentiation values (FSTP) revealed that strong connectivity exists among islands < 200km apart in the Ryukyu Archipelago. Of 25 site pairs with FSTP < 0.1 (bold grids), 8 pairs exhibited intra-island connectivity, whereas OKI and IRMg are almost completely isolated. X-and Y-axes show site names, and the figure shows no directionality. P-values < 0.01 for all pairs, except for grids with *, ** and NS indicating p > 0.1, 0.05 < p < 0.1, and not-significant, respectively.
Figure 3 Population pairs with FSTP < 0.1 are projected over the map, and bold lines indicate pairs with FSTP < 0.05, a threshold value indicating significant connectivity.
Table 2 AMOVA summary shows that genetic variation is highest among individuals within populations, and that fixation indices are all significant with a tendency toward genetic isolation.
Mantel tests revealed that distance accounts for < 5% of genetic differentiation between sites in the Yaeyama region (R2 = 0.045, p < 0.1), < 10% in the Sakishima region (R2 = 0.076, p < 0.05), and > 30% in the entire archipelago (R2 = 0.341, p < 0.01), which indicates isolation-by-distance over the archipelago, but not at a given island. We also found that the westernmost site in the archipelago, IRMg, revealed linearized FSTP values (LinFSTP), which are consistently above average regardless of Euclidean distance between IRMg and other sites (Figure 4). However, IRMg was not the cause of the lower fit, according to the sensitivity analysis.
Figure 4 The tendency of IBD (isolation by distance) became more apparent over the Sakishima region (B) and over the entire Ryukyu Archipelago (A), which indicates decreasing gene flow affected by the absence of coastline over a certain distance. Within the Yaeyama region (C), Euclidean and genetic distance did not show a correlation. All outliers in the plots are associated with IRMg indicating that the site is isolated by other environmental factors beside geographical distance or ocean connectivity. The Y-axis shows linearized genetic differentiation values, and the X-axis shows the Euclidean distance between paired sites. All filled markers in (B, C) are paired sites with IRMg.
Bayesian analyses suggested 3 genetically homogeneous groups of individuals (genetic clusters) in the Ryukyu Archipelago (Figure 5): western Iriomote Island (WI), North Miyako Island (NM), and Okinawa Island (OKN). The majority of individuals (> 75%) on the west coast of Iriomote Island (WCI) had > 90% inferred ancestry from a local genetic cluster (WI), particularly at the westernmost site, IRMg, (> 95%). Site IRMf in the WCI area showed one individual with > 90% inferred ancestry from OKN. Most individuals (> 92%) at site OKI had > 90% inferred ancestry from a local cluster (OKN). OKI and IRMg exhibited homogeneous population genetic structures, highlighting the lack of common inferred ancestry between them. On the other hand, the remaining sampling sites showed heterogenous genetic structure within each site. Miyako, Ishigaki, and the east coast of Iriomote Island (ECI) (Figure 5) exhibited individuals with > 80% of inferred ancestry from NM admixed with individuals having inferred ancestry from OKN and WI. For example, 27 individuals from the northern site of Iriomote Island (IRMa) included 2 individuals with inferred ancestry (> 80% here after) from OKN, 4 individuals with inferred ancestry from NM, 9 individuals with inferred ancestry from WI, and the remaining 12 individuals with mixed ancestries. We also found that very few inferred ancestries were shared within an island, particularly between WCI and ECI.
Figure 5 The optimal number of genetic clusters was 3 (K= 3); clustered with inferred ancestry from the west coast of Iriomote Island (WCI) (purple), from Miyako Island (orange), from Okinawa mainland (blue). Each bar represents an individual sample. The cluster from OKI was distributed throughout the Archipelago, but its ratio drastically declined at WCI (IRMe-g), while the cluster from WCI revealed very limited distribution, even among neighboring sites on Iriomote Island. Heterogeneous genetic structures were found at most sampling sites, except for IRMg and OKI, and such patterns were consistent from the results with K= 4, but not with K=2 where both clusters are distributed throughout the archipelago.
Contemporary migration rates (m) represent the frequency of genetic immigration in the last few generations between all pairs of sites in the archipelago, which ranged from m = 0.008 to m = 0.154 (m = 0.01 implies that on average 1 individual among a sample of 100 per generation would be a migrant). The highest migration rate (m = 0.154) was found from Ishigaki Island (ISGa) to east of Iriomote Island (IRMb), followed by pairs among neighboring sites along the west coast of Iriomote Island (i.e., m=0.149 from IRMg to IRMf, and m=0.114 from IRMg to IRMe). However, migration rates in the opposite direction were substantially lower (Figure 6). The analysis also identified ISGa as the site with the highest frequency of emigrants to other sites (ΣmISGa(Source) = 0.489). Furthermore, the analysis identified the potential for long distance (> 200km) propagule immigration from Okinawa Island (OKI) to Iriomote Island (IRMc) with relatively higher migration rates (m = 0.052) (Figure 7). The majority of migration rates were too low (m < 0.05) (Meirmans, 2014) to confirm genetic exchange between sites.
Figure 6 Bayesian assignment test identified contemporary migration patterns in the Ryukyu Archipelago with posterior mean migration rates (m = 0.01 implying that 1 individual among a sample of 100 per generation is a migrant). The majority of site pairs showed lower m values (x-axis: target sites, y-axis: origin sites), and a few with higher, sporadic migration rates.
Figure 7 All migration patterns with m > 0.05 were projected over a map with arrows pointing at target sites. The higher migration rates (m > 0.1) for R. stylosa in the Ryukyu Archipelago were unidirectional (bold arrows). The majority of site pairs with m > 0.05 were found between Iriomote and Ishigaki Islands, and site ISGa was identified as the strongest source population for gene flows among study sites. Black arrows have higher accuracy (FST > 0.25).
Of 31 GPS drifting buoys released from a location just off the west coast of Iriomote Island, 6 drifters beached on the same island after an average of 23 days with high retention rates around the release points for the first 20 days (Figure 8), while one drifter took > 130 days to beach on the natal island coastline (Figure 8, Table 3). We confirmed 4 island-to-island dispersal beaching events, including one each on the coasts of Ishigaki Island (58 km in 12 days), Kuroshima Island (35 km in 9 days), Miyako Island (183 km in 58 days), and Yakabi Island (414 km in 109 days). We found that northeastward was the dominant direction of drifter movements north of the Yaeyama region (Figure 9). We also found that 60% of drifters were taken by the strong western boundary current, the Kuroshio Current, of which 76% were carried out into the mid Pacific Ocean and were eventually lost due to battery failure (Figure 10). We also observed sporadic counter-current drifting southwestward with various trajectories. Frequent local eddies were also observed in the archipelago, causing dispersal periods unrelated to Euclidean distance from the deployment site. For example, we identified a drifter traveling > 1,000 km in 50 days while another traveled 183 km in 59 days.
Figure 8 9 buoys beached within the Sakishima region, one of which drifted for over 100 days while others beached < 60 days, identifying a temporal scale of the trajectories. Deployment site is shown in *, and beaching locations are shown in red. Local beaching events highlighted the absence of trajectories within Sekisei Lagoon, between Iriomote and Ishigaki Islands. Color bars shows temporal data in days for each position of buoys.
Table 3 In situ drifter experiments showed about 30% of local beaching (Bold letters) with a wide range of temporal variation from 0 to 132 days.
Figure 9 The Ryukyu Archipelago, showing differences in concentration of trajectories within the island system, especially between west and east ends of the archipelago. Particles deployed from western Iriomote Island tend to stay on the west side of the archipelago, but showed frequent mixing within the Yaeyama region. The color bar shows temporal data in days for each position of the buoys.
Figure 10 Trajectories of 31 GPS drifting buoys over 250 days are shown. The retention period of buoys in the Ryukyu Archipelago is < 100 days on average and only a few stayed as long as 200 days. The Kuroshio Current is involved in trajectories of particles released from western Iriomote Island. Potential to travel as far as the mid Pacific Ocean was confirmed. The color bar shows temporal data in days for each position of buoys, and a red point shows the deployment location.
Significant genetic disequilibrium found in this study may be due to high monomorphism caused by high rate of inbreeding expected in naturally fragmented population (Provan et al., 2008) or/and by selfing due to pollinator limitation (Bawa, 1990) both of which are common to Rhizophora species. The genetic variance (Na), inbreeding coefficient (FIS) and limited area of fringing mangroves in the current study all exhibited characteristics of habitats at the species range limit. The Ryukyu Archipelago is located at the northern edge of mangrove global distribution, and site OKI, showing the lowest genetic variance in the study (Ar=1.2), is the northernmost habitat of R. stylosa. Compared to a genetic connectivity study of R. stylosa in South China (Geng et al., 2021), the reduction of genetic diversity in this study area was apparent. Hence, R. stylosa in the Ryukyu Archipelago maybe subject to genetic drift and environmental selection due to population sizes limited by extreme and/or variable environments (Brown et al., 1996) at the edge of the species distribution. Based on genetic structures indicating infrequent gene flow among sites (Figure 5), we suggest that the genetic variation at the time of establishment in the archipelago may already have been limited.
Lack of riverine inputs may also have effects on low genetic variation. The current samples were collected at fringing mangrove habitats in proximity to the open ocean, where there were no inputs from rivers. On the other hand, samples used by Islam et al. (2014) were collected from river basins located further inland than our sampling sites. Over all, FIS of this study was substantially higher and Ho was also significantly lower than values reported by Islam et al. (2014) (Table 1). Proximity to open ocean has been suggested to influence inbreeding and genetic diversity of mangrove populations, in that the most seaward habitats should have a higher likelihood of propagule dispersal and should exhibit higher genetic diversity (Triest et al., 2021a). However, genetic comparisons with those of Islam et al. (2014) revealed the opposite, and suggested very low likelihood of propagule dispersal among fringing mangroves in the island system via ocean currents, despite being directly exposed to ocean hydrodynamics. In general, riverine systems provide more suitable habitats for mangroves because the constant sediment supply from upstream expands available habitat areas for new propagules (Kadoya and Inoue, 2015). This, in turn, supports larger population sizes resulting in greater genetic variation within habitats (Kadoya and Inoue, 2015). Additionally, gene flow in riverine systems may be bidirectional because of interacting river flow with tidal fluxes, coastal currents, and wind, which supports genetic diversity within fluvial systems (Ngeve et al., 2017). On the other hand, fringing habitats lack terrestrial input and fluvial interactions with rivers. Thus, habitat areas are often limited, and demographic connectivity must rely predominantly on ocean currents, which limit genetic diversity due to a lack of connectivity among populations on island systems. Over all, genetic heterozygosity (Ho > He) and inbreeding coefficients (FIS) in the current study indicated inbreeding/selfing as the dominant method to sustain population sizes, which suggests that propagule dispersal in the archipelago is restricted to local scale. Genetic variation revealed that fringing habitats of the Ryukyu Archipelago are naturally fragmented with higher potential for genetic isolation within the island system, making them vulnerable to environmental changes.
Considering ecological characteristics of propagules that contribute to LDD, in addition to direct ocean access at each sampling site, we hypothesized a uniform level of genetic connectivity throughout the archipelago. However, contrary to our hypothesis, we found genetic structures indicating limited connectivity. The majority of sites in the Sakishima region (Iriomote, Ishigaki and Miyako Islands) displayed heterogeneous genetic structures in each population with inferred ancestries from all three bioregions (OKN, NM, WI) (Figure 5). Since the wind-pollination success rate of R. stylosa is low, structural heterogeneity may indicate that propagule immigration from other sites is consistently sporadic over many generations, and that the frequency of inbreeding/selfing is too high to achieve homogeneity. On the other hand, at the edges of the archipelago, both OKI and IRMg exhibited genetic structure with one dominant inferred ancestry (OKN and WI, respectively) indicating their genetic isolation. Overall, genetic structures and FIS showed that inbreeding/selfing has consistently been the major reproductive method and population connectivity even within islands is extremely limited.
Directionality and spatial range of historical genetic connectivity were interpreted based on shared inferred ancestries in populations. With > 80% of OKN inferred ancestry in an individual throughout the Ryukyu Archipelago, genetic exchange southwestward is suggested. On the other hand, inferred ancestry of WI is absent in Miyako and Okinawa islands, which suggests that northeastward genetic exchange declines drastically beyond Ishigaki Island (Figure 5). The decline of genetic exchange showed its correlation with distance between islands, according to the Mantel test between genetic differentiation and Euclidean distance (Figure 3). Hence, in the Ryukyu Archipelago, spatial range of > 100 km between islands in northeastward direction is identified as threshold for genetic discontinuity, and no population connectivity among islands was frequent enough to homogenize genetic structure within the archipelago. However, significantly high inbreeding coefficients from all study sites must be carefully considered since significant FIS may cause additional populations to be inferred (Falush et al., 2003). Additionally, high FIS and estimated selfing rates in the studied populations have been explained for R.mucronata (Triest et al., 2021b).The optimal K was 3 in this study, based on the Evanno method (Evanno et al., 2005), but according to the genetic structure with K=2, all islands are well admixed within the archipelago; however, not enough connectivity among islands was found to homogenize the genetic structure (Figure 5). On the other hand, at the edges of the archipelago, both OKI and IRMg exhibited genetic structure with one dominant inferred ancestry (OKN and WI, respectively) indicating their genetic isolation. Over all, genetic structures and FIS showed that inbreeding/selfing has consistently been the major reproductive method and population connectivity even within islands is limited.
Reliable migrant ancestry assignment values (m > 0.05) of the last few generations between populations were found between 14 site pairs, mostly from Ishigaki to Iriomote Island representing an influx of immigrant alleles among populations via propagule dispersal. This southwestward pattern of propagule immigration from Ishigaki Island to ECI was also found in a locally abundant broadcast-spawning coral species, Acropora tenuis (Zayasu et al., 2016). Low frequency of influx of alleles in recent generations was suggested over the archipelago, and inter-island influx was only found in a southwestward direction, as suggested from genetic structure. However, we must remain tentative with our results derived from only 7 loci, which is the lower limit of sample size suitable for BayesAss assignment (Wilson and Rannala, 2003). With a small number of loci (5 in Wilson and Rannala, 2003), accuracy of assignments is reasonable if migration is low (m < 0.2) and genetic differentiation is high (FST > 0.25) (Wilson and Rannala, 2003). Hence, migration ancestries with higher accuracy in this study were found only between 6 pairs (Figure 6). With obtained high FIS values in the archipelago, this suggests that the influx of propagules from other populations is extremely low, but still contributes to maintain genetic diversity within populations in the archipelago.
Overall, in this study, contemporary gene flow patterns identified with higher migration rates (m > 0.05) seem to correspond well to the results of STRUCTURE, indicating that those sites with m > 0.05 have undergone consistent, sporadic gene flow over a historical timescale (Wilson and Rannala, 2003). Based on the “one-migrant-per-generation” rule (Mills and Allendorf, 1996), one immigrant per generation is enough to avoid harmful effects of local inbreeding in a population (Lowe and Allendorf, 2010). Thus, populations with m > 0.05 and population size > 20 in the archipelago indicate that those populations may avoid harmful effects of local inbreeding/selfing, despite low genetic variation and limited genetic heterozygosity.
Numerical ocean modeling and Lagrangian particle tracking methods have been widely accepted to estimate larvae dispersal of marine organisms. A previous mangrove study utilizing a numerical modeling method identified predominant coastal propagule transport along several continental coastlines, and island systems that are stepping stones to transpacific connectivity for mangrove species (Van der Stocken et al., 2019). However, the process of detachment from and settlement in nearshore areas before and after dispersal have not yet been examined. Despite the lower statistical power compared to numerical ocean modeling, the release-recapture method utilizing drifting buoys shows beaching events as part of settlement. Rhizophora stylosa’s propagules are about 23 cm in length, weigh about 35 grams, tend to maintain an efficient vertical floating orientation in higher salinity while fully submerged at the surface (Clarke et al., 2001). Hence, drifting buoys are suitable surrogates for mangrove propagules to simulate a subset of dispersal trajectories to estimate potential connectivity via propagule dispersal among islands, and to interpret genetic results at a current time scale.
Of 31 drifters deployed from just outside the reef at WCI, 4 trajectories revealed potential for inter-island dispersal with various destinations in the archipelago (Ishigaki, Kuroshima, Miyako, and Yakabi Islands), and 6 trajectories showed within-island dispersal (Table 3). Mangrove sites that are < 100 km apart were indicated as neighboring sites based on genetic analyses; thus, we suggest that beaching events on Ishigaki Island (~58km in 12 days) and Kuroshima Island (~35 km in 9 days) represent the potential extent of local dispersal, and those on Miyako Island (~180 km in 59 days) and Yakabi Island (~ 400 km in 109 days) represent potential for LDD. Those potentials for dispersal are further supported by the estimated minimum and maximum floating periods of propagules considering that they play significant roles restricting dispersal range (Van der Stocken et al., 2019). Although there have been no studies to our knowledge on R. stylosa’s maximum floating and viability periods, we assume that R. stylosa shares propagule traits with R. mucronata, morphology of which is almost indistinguishable from that of R. stylosa (Spalding et al., 2010). Hence, the potential for northeastward LDD among islands is supported by R. stylosa’s maximum dispersal viability (150 days, median: 70 days), even though the survival rate of propagules is also suggested to decrease as the dispersal period increases (Drexler, 2001). However, no genetic result confirmed long-distance connectivity from WCI to other islands. Hence, northeastward LDD in the archipelago was not documented by this study. On the other hand, the retention period of buoys in the Yaeyama region was below the maximum floating period (Figure 10), which may increase the potential for beaching at local scale and may explain the admixed genetic structures in this region (Figure 5).
The genetic isolation of OKI is probably influenced by the Kuroshio western boundary current. The Kuroshio current flows northeastward about 150~200km west of the Ryukyu Archipelago, and current velocity and location are not only seasonal, but also highly variable year by year (Uchiyama et al., 2018; Takeda et al., 2021). The majority of observed trajectories beyond Ishigaki Island following the Kuroshio current confirmed a tendency for fast, long-distance (< 100 days) northeastward dispersal without reaching any coast in the archipelago (Table 3). The limited distribution of the WI cluster on Miyako and Okinawa Islands maybe due to the Kuroshio current, with a temporal limitation imposed by propagule viability. These limited trajectories were also found with numerical ocean modeling in a previous study on the Ryukyu Archipelago (Uchiyama et al., 2018).
Local beaching events within 6 days on Iriomote Island were confirmed, except for one drifter that took > 131 days to beach on the coast 18 km from its deployment site. Drifter experiments highlighted the wide range of temporal variation in dispersal affected by local eddies. We suggest that the higher migration rate in WCI (IRMg-IRMf, IRMg-IRMe) and homogeneous genetic structure in the area (Figure 5) may be explained by the higher retention rate around the deployment site along the reef of WCI during the first 20 days (Figure 9). Retention by currents in WCI and a high proportion of self-recruitment were also hypothesized in a previous ocean-modeling study for coral dispersal in the archipelago (Uchiyama et al., 2018). Moreover, the absence of a trajectory from WCI to ECI indicated a potential local oceanic barrier, which may explain the genetic discontinuities we found between those areas. Using a numerical model, Uchiyama et al. (2018) also indicated lower particle density reaching ECI from WCI, which suggests a consistent local oceanic pattern.
The difficulties of propagule dispersal among sites were further suggested by observed alongshore currents. More than half the drifters (19) did not beach during our experiments, and their closest approaches to coastlines while drifting ranged from 20 m to ~1 km (Table 3). Most drifters reached reef edges, and drifted with the alongshore current without entering the reefs. It is important to note that shallow reefs even interfere with genetic exchange between close sites for coral species such as Pocillopora damicornis (Miller and Ayre, 2004) despite their floating capacities and higher abundance of larvae compared to mangrove propagules. Hence, we suggest that tendency of localized dispersal of R.stylosa is mainly due to its low propagule fecundity, despite the long floating and viable periods of propagules. Stokes drift, contrary to alongshore currents, also affects onshore transport (Monismith et al., 2018). However, these drifters were designed to eliminate effects of waves (Stokes drift); thus, representation of propagule dispersal with drifting buoys may have underestimated the success rate of actual propagules. It is also worth noting that the present in-situ results represent subset of entire dispersal trajectories that are specific to seasons and release locations.
In this study, with a single empirical dataset with genetic results, we discussed how ocean currents may affect fine-scale connectivity among islands. We interpreted genetic discontinuities within and among islands with current patterns, the distance between islands, and propagule characteristics such as fecundity and floating periods; however, further statistical approaches are needed for a more robust understanding of genetic discontinuities in the archipelago. Fine-scale numerical models may be suitable to estimate tidal amplitude affecting inundation of mangrove habitats and cross-shore currents at the reef edge to predict propagule dispersal from distant habitats. However, including wave-current interactions and obtaining the right combination of environmental parameters, such as topological factors as parameters for the model to achieve a finer-scale evaluation for near shore currents is difficult (Kamidaira et al., 2017; Uchiyama et al., 2018). Overall, in this study we showed that island-to-island propagule dispersal of R. stylosa in the Ryukyu Archipelago is possible even without stepping stones, although in reality, it is not frequent enough to unify population genetic structure. We also found that the archipelago is isolated from global distribution. Our findings are based on mangrove habitats with little or no human destruction, which should serve as a warning for habitats on island systems that are subject to ongoing threats from deforestation and environmental changes. It is important to identify the spatiotemporal scale of propagule movement specific to each island system to accurately understand demographic connectivity among populations.
The datasets presented in this study can be found in online repositories. The names of the repository/repositories and accession number(s) can be found below: https://datadryad.org/stash, doi:10.5061/dryad.bcc2fqzdh.
MT designed and conducted the majority of the project, including field sampling, main experiments, data analysis, and manuscript writing. YN provided assistance in genetic experiments and analysis and contributed to manuscript writing. SM contributed to the designing in situ experiments and provided guidance for manuscript writing. All authors contributed to the discussion and interpretation of data, and contributed to the article and approved the submitted version.
This work was supported by the Marine Biophysics Unit from Okinawa Institute of Science and Technology Graduate University (OIST).
The authors declare that the research was conducted in the absence of any commercial or financial relationships that could be construed as a potential conflict of interest.
All claims expressed in this article are solely those of the authors and do not necessarily represent those of their affiliated organizations, or those of the publisher, the editors and the reviewers. Any product that may be evaluated in this article, or claim that may be made by its manufacturer, is not guaranteed or endorsed by the publisher.
We thank NPO Manglobal for helping us select study sites by giving us local information of each habitat and providing us access and assistance for field work. We also thank Akinori Murata and Kirk Sato for their assistances in the deployment of drifting buoys, and further thank Kazumi Inoha for her assistance in obtaining and managing in situ data. We also thank the OIST Sequencing Section for providing resources and maintenance for the sequencer. We thank Steven D. Arid for editing and commenting on the manuscript.
Al-Qthanin R. N., Alharbi S. A. (2020). Spatial Structure and Genetic Variation of a Mangrove Species (Avicennia Marina (Forssk.) Vierh) in the Farasan Archipelago. Forests 11, 1287–1307. doi: 10.3390/f11121287
Arnaud-Haond S., Teixeira S., Massa S., Billot C., Saenger P., Coupland G., et al (2006). Genetic Structure at Range Edge: Low Diversity and High Inbreeding in Southeast Asian Mangrove (Avicennia Marina) Populations. Mol. Ecol. 15, 3515–3525. doi: 10.1111/j.1365-294X.2006.02997.x
Bawa K. S. (1990). Plant-Pollinator Interactions in Tropical Rain Forests. Annu. Rev. Ecol. Syst. 21, 399–422. doi: 10.1146/annurev.es.21.110190.002151
Brown J., Stevens G., Kaufman D. (1996). The Geographic Range: Size, Shape, Boundaries, and Internal Structure. Annu. Rev. Ecol. Syst. 27, 597–623. doi: 10.1146/annurev.ecolsys.27.1.597
Clarke P. J., Kerrigan R. A., Westphal C. J. (2001). Dispersal Potential and Early Growth in 14 Tropical Mangroves: Do Early Life History Traits Correlate With Patterns of Adult Distribution? J. Ecol. 89, 648–659. doi: 10.1046/j.0022-0477.2001.00584.x
Coupland G. T., Paling E. I., Mcguinness K. A. (2006). Floral Abortion and Pollination in Four Species of Tropical Mangroves From Northern Australia. Aquat. Bot. 84, 151–157. doi: 10.1016/j.aquabot.2005.09.003
Curnick D. J., Pettorelli N., Amir A. A., Balke T., Barbier E. B., Crooks S., et al (2019). The Value of Small Mangrove Patches. Science 363, 239. doi: 10.1126/science.aaw0809
Dodd R. S., Afzal-Rafii Z., Kashani N., Budrick J. (2002). Land Barriers and Open Oceans: Effects on Gene Diversity and Population Structure in Avicennia Germinans L. (Avicenniaceae). Mol. Ecol. 11, 1327–1338. doi: 10.1046/j.1365-294X.2002.01525.x
Drexler J. Z. (2001). Maximum Longevities of Rhizophora Apiculata and R. Mucronata Propagules. Pac. Sci. 55, 17–22. doi: 10.1353/psc.2001.0004
Earl D., Holdt B. (2012). STRUCTURE HARVESTER: A Website and Program for Visualizing STRUCTURE Output and Implementing the Evanno Method. Conserv. Genet. Resour. 4, 359–361. doi: 10.1007/s12686-011-9548-7
Evanno G., Regnaut S., Goudet J. (2005). Detecting the Number of Clusters of Individuals Using the Software Structure: A Simulation Study. Mol. Ecol. 14, 2611–2620. doi: 10.1111/j.1365-294X.2005.02553.x
Excoffier L., Smouse P. E., Quattro J. M. (1992). Analysis of Molecular Variance Inferred From Metric Distances Among DNA Haplotypes: Application to Human Mitochondrial DNA Restriction Data. Genetics 131, 479–491. doi: 10.1093/genetics/131.2.479
Falush D., Stephens M., Pritchard J. K. (2003). Inference of Population Structure Using Multilocus Genotype Data: Linked Loci and Correlated Allele Frequencies. Genetics 164, 1567–1587. doi: 10.1093/genetics/164.4.1567
Faubet P., Waples R., Gaggiotti O. (2007). Evaluating the Performance of a Multilocus Bayesian Method for the Estimation of Migration Rates. Mol. Ecol. 16, 1149–1166. doi: 10.1111/j.1365-294X.2007.03218.x
Fox-Kemper B., Adcroft A., Böning C. W., Chassignet E. P., Curchitser E., Danabasoglu G., et al (2019). Challenges and Prospects in Ocean Circulation Models. Front. Mar. Sci. 6. doi: 10.3389/fmars.2019.00065
Frankham R., Ballou J. D., Briscoe D. A. (2010). Introduction to Conservation Genetics (Cambridge, UK, New York: Cambridge University Press).
Fujimoto K., Miura M., Haruyama S. (2015). Mangrove Habitat Dynamics and Seismic Crustal Movement During the Late Holocene on the Nakama River Delta, Iriomote Island, Southwestern Japan. Mangrove Sci. 9, 3–15. doi: 10.1016/j.ecss.2019.03.001
Fujimoto K., Ohnuki Y. (1995). Developmental Processes of Mangrove Habitat Related to Relative Sea-Level Changes at the Mouth of the Urauchi River, Iriomote Island, Southwestern Japan. Q. J. Geogr. 47, 1–12. doi: 10.5190/tga.47.1
Geng Q., Wang Z., Tao J., Kimura M., Liu H., Hogetsu T., et al (2021). Ocean Currents Drove Genetic Structure of Seven Dominant Mangrove Species Along the Coastlines of Southern China. Front. Genet. 12. doi: 10.3389/fgene.2021.615911
Goudet J. (2001). FSTAT, a Program to Estimate and Test Gene Diversities and Fixation Indices (Version 2.9.3), Updated from Goudet (1995)", Switzerland: Université de Lausanne, Dorigny. Avaliable at: http://www2.unil.ch/popgen/softwares/fstat.htm
Hubisz M., Falush D., Stephens M., Pritchard J. (2009). Inferring Weak Population Structure With the Assistance of Sample Group Information. Mol. Ecol. Resour. 9, 1322–1332. doi: 10.1111/j.1755-0998.2009.02591.x
Hurlbert S. H. (1971). Nonconcept of Species Diversity - Critique and Alternative Parameters. Ecology 52, 577. doi: 10.2307/1934145
Islam M. S., Lian C., Kameyama N., Hogetsu T. (2012). Analyses of Genetic Population Structure of Two Ecologically Important Mangrove Tree Species, Bruguiera Gymnorrhiza and Kandelia Obovata From Different River Basins of Iriomote Island of the Ryukyu Archipelago, Japan. Tree Genet. Genomes 8, 1247–1260. doi: 10.1007/s11295-012-0512-9
Islam M. S., Lian C. L., Kameyama N., Hogetsu T. (2014). Low Genetic Diversity and Limited Gene Flow in a Dominant Mangrove Tree Species (Rhizophora Stylosa) at its Northern Biogeographical Limit Across the Chain of Three Sakishima Islands of the Japanese Archipelago as Revealed by Chloroplast and Nuclear SSR Analysis. Plant Syst. Evol. 300, 1123–1136. doi: 10.1007/s00606-013-0950-3
Islam M. S., Lian C., Kameyama N., Wu B., Hogetsu T. (2004). Development of Microsatellite Markers in Rhizophora Stylosa Using a Dual-Suppression-Polymerase Chain Reaction Technique. Mol. Ecol. Notes 4, 110–112. doi: 10.1046/j.1471-8286.2003.00585.x
Kadoya T., Inoue T. (2015). Spatio-Temporal Pattern of Specific Gravity of Mangrove Diaspore: Implications for Upstream Dispersal. Ecography 38, 472–479. doi: 10.1111/ecog.01074
Kamidaira Y., Uchiyama Y., Mitarai S. (2017). Eddy-Induced Transport of the Kuroshio Warm Water Around the Ryukyu Islands in the East China Sea. Cont. Shelf Res. 143, 206–218. doi: 10.1016/j.csr.2016.07.004
Kopelman N., Mayzel J., Jakobsson M., Rosenberg N., Mayrose I. (2015). Clumpak: A Program for Identifying Clustering Modes and Packaging Population Structure Inferences Across K. Mol. Ecol. Resour. 15, 1179–1191. doi: 10.1111/1755-0998.12387
Levine J. M., Murrell D. J. (2003). The Community-Level Consequences of Seed Dispersal Patterns. Annu. Rev. Ecol. Evol. Syst. 34, 549–574. doi: 10.1146/annurev.ecolsys.34.011802.132400
Levin S. A., Muller-Landau H. C., Nathan R., Chave J. (2003). The Ecology and Evolution of Seed Dispersal: A Theoretical Perspective. Annu. Rev. Ecol. Evol. Syst. 34, 575–604. doi: 10.1146/annurev.ecolsys.34.011802.132428
Lo E. Y., Duke N. C., Sun M. (2014). Phylogeographic Pattern of Rhizophora (Rhizophoraceae) Reveals the Importance of Both Vicariance and Long-Distance Oceanic Dispersal to Modern Mangrove Distribution. BMC Evol. Biol. 14, 83. doi: 10.1186/1471-2148-14-83
Lowe W. H., Allendorf F. W. (2010). What can Genetics Tell Us About Population Connectivity? Mol. Ecol. 19, 3038–3051. doi: 10.1111/j.1365-294X.2010.04688.x
Meirmans P. G. (2014). Nonconvergence in Bayesian Estimation of Migration Rates. Mol. Ecol. Resour. 14, 726–733. doi: 10.1111/1755-0998.12216
Miller K. J., Ayre D. J. (2004). The Role of Sexual and Asexual Reproduction in Structuring High Latitude Populations of the Reef Coral Pocillopora Damicornis. Heredity 92, 557–568. doi: 10.1038/sj.hdy.6800459
Mills L. S., Allendorf F. W. (1996). The One-Migrant-Per-Generation Rule in Conservation and Management. Conserv. Biol. 10, 1509–1518. doi: 10.1046/j.1523-1739.1996.10061509.x
Minagawa M. (2000). "Japan: Mangrove Areas and Their Utilization" Primavera J. H., Garcia L. M. B., Castaños M. T., Surtida M. B. (Eds.), Mangrove-Friendly Aquaculture: Proceedings of the Workshop on Mangrove-Friendly Aquaculture organized by the SEAFDEC Aquaculture Department, January 11-15, 1999 (Tigbauan, Iloilo, Philippines: Southeast Asian Fisheries Development Center, Aquaculture Department), 35–40.
Monismith S. G., Barkdull M. K., Nunome Y., Mitarai S. (2018). Transport Between Palau and the Eastern Coral Triangle: Larval Connectivity or Near Misses. Geophys. Res. Lett. 45, 4974–4981. doi: 10.1029/2018GL077493
Nathan R., Muller-Landau H. C. (2000). Spatial Patterns of Seed Dispersal, Their Determinants and Consequences for Recruitment. Trends Ecol. Evol. 15, 278–285. doi: 10.1016/S0169-5347(00)01874-7
Nathan R., Schurr F., Spiegel O., Steinitz O., Trakhtenbrot A., Tsoar A. (2008). Mechanisms of Long-Distance Seed Dispersal. Trends Ecol. Evol. 23, 638–647. doi: 10.1016/j.tree.2008.08.003
Ngeve M., Van der Stocken T., Menemenlis D., Koedam N., Triest L. (2016). Contrasting Effects of Historical Sea Level Rise and Contemporary Ocean Currents on Regional Gene Flow of Rhizophora Racemosa in Eastern Atlantic Mangroves. PloS One 11, 1–24. doi: 10.1371/journal.pone.0150950
Ngeve M., Van der Stocken T., Sierens T., Koedam N., Triest L. (2017). Bidirectional Gene Flow on a Mangrove River Landscape and Between-Catchment Dispersal of Rhizophora Racemosa (Rhizophoraceae). Hydrobiologia 790, 93–108. doi: 10.1007/s10750-016-3021-2
Ohlmann J., White P., Sybrandy A., Miler P. (2005). GPS-Cellular Drifter Technology for Coastal Ocean Observing Systems. J. Atmos. Ocean. Technol. 22, 1381–1388. doi: 10.1175/JTECH1786.1
Peakall R., Smouse P. (2012). GenAlEx 6.5: Genetic Analysis in Excel. Population Genetic Software for Teaching and Research – an Update. Bioinformatics 28, 2537–39. doi: 10.1093/bioinformatics/bts460
Pil M. W., Boeger M. R. T., Muschner V. C., Pie M. R., Ostrensky A., Boeger W. A. (2011). Postglacial North – South Expansion of Populations of Rhizophora Mangle (Rhizophoraceae) Along the Brazilian Coast Revealed by Microsatellite Analysis. Am. J. Bot. 98, 1031–39. doi: 10.3732/ajb.1000392
Pritchard J., Stephens M., Donnelly P. (2000). Inference of Population Structure Using Multilocus Genotype Data. Genetics 155, 945–959. doi: 10.1093/genetics/155.2.945
Provan J., Beatty G. E., Hunter A. M., Mcdonald R. A., Mclaughlin E., Preston S. J., et al (2008). Restricted Gene Flow in Fragmented Populations of a Wind-Pollinated Tree. Conserv. Genet. 9, 1521–1532. doi: 10.1007/s10592-007-9484-y
Rabinowitz D. (1978). Dispersal Properties Of Mangrove Propagules. Biotropica 10, 47–57. doi: 10.2307/2388105
Raymond M., Rousset F. (1995). GENEPOP (Version 1.2): Population Genetics Software for Exact Tests and Ecumenicism. J. Heredity 86, 248–249.
Rousset F. (2008). Genepop'007: A Complete Reimplementation of the Genepop Software for Windows and Linux. Mol. Ecol. Res. 8, 103–106.
Sandoval-Castro E., Muniz-Salazar R., Manuel Enriquez-Paredes L., Riosmena-Rodriguez R., Dodd R. S., Tovilla-Hernandez C., et al (2012). Genetic Population Structure of Red Mangrove (Rhizophora Mangle L.) Along the Northwestern Coast of Mexico. Aquat. Bot. 99, 20–26. doi: 10.1016/j.aquabot.2012.01.002
Sharma S., Kamruzzaman M., Hoque A. T. M., Analuddin K., Hagihara A. (2011). Vegetative and Reproductive Phenology, and Litterfall Production of Rhizophora Stylosa in Okinawa Island, Japan. Int. J. Environ. 1, 21–27. doi: 10.1007/s00468-012-0816-2
Shinmura Y., Wee A. K. S., Takayama K., Meenakshisundaram S. H., Asakawa T., Onrizal, et al (2012). Isolation and Characterization of 14 Microsatellite Markers for Rhizophora Mucronata (Rhizophoraceae) and Their Potential Use in Range-Wide Population Studies. Conserv. Genet. Resour. 4, 951–954. doi: 10.1007/s12686-012-9681-y
Spalding M. D., Kainuma M., Collins L. (2010). World Atlas of Mangrove Earthscan. Earthscan and James & James.
Takayama K., Tamura M., Tateishi Y., Webb E. L., Kajita T. (2013). Strong Genetic Structure Over the American Continents and Transoceanic Dispersal in the Mangrove Genus Rhizophora (Rhizophoraceae) Revealed by Broad-Scale Nuclear and Chloroplast DNA Analysis. Am. J. Bot. 100, 1191–1201. doi: 10.3732/ajb.1200567
Takeda N., Kashima M., Odani S., Uchiyama Y., Kamidaira Y., Mitarai S. (2021). Identification of Coral Spawn Source Areas Around Sekisei Lagoon for Recovery and Poleward Habitat Migration by Using a Particle-Tracking Model. Sci. Rep. 11, 6963. doi: 10.1038/s41598-021-86167-5
Triest L., Del Socorro A., Gado V. J., Mazo A. M., Sierens T. (2021a). Avicennia Genetic Diversity and Fine-Scaled Structure Influenced by Coastal Proximity of Mangrove Fragments. Front. Mar. Sci. 8. doi: 10.3389/fmars.2021.643982
Triest L., Van der Stocken T., De Ryck D., Kochzius M., Lorent S., Ngeve M., et al (2021b). Expansion of the Mangrove Species Rhizophora Mucronata in the Western Indian Ocean Launched Contrasting Genetic Patterns. Sci. Rep. 11, 4987. doi: 10.1038/s41598-021-84304-8
Uchiyama Y., Odani S., Kashima M., Kamidaira Y., Mitarai S. (2018). Influences of the Kuroshio on Interisland Remote Connectivity of Corals Across the Nansei Archipelago in the East China Sea. J. Geophys. Res. Oceans 123, 9245–9265. doi: 10.1029/2018JC014017
Van der Stocken T., Cerroll D., Menemenlis D., Simard M., Koedam N. (2019). Global-Scale Dispersal and Connectivity in Mangroves. Proc. Natl. Acad. Sci. U. S. A. 116, 915–922. doi: 10.1073/pnas.1812470116
Van der Stocken T., De Ryck D. J. R., Vanschoenwinkel B., Deboelpaep E., Bouma T. J., Dahdouh-Guebas F., et al (2015). Impact of Landscape Structure on Propagule Dispersal in Mangrove Forests. Mar. Ecol. Prog. Ser. 524, 95–106. doi: 10.3354/meps11206
Wang W., Li X., Wang M. (2019). Propagule Dispersal Determines Mangrove Zonation. Forests 10, 245. doi: 10.3390/f10030245
Wee A. K. S., Takayama K., Asakawa T., Thompson B., Onrizal, Sungkaew S., et al (2014). Oceanic Currents, Not Land Masses, Maintain the Genetic Structure of the Mangrove Rhizophora Mucronata Lam. (Rhizophoraceae) in Southeast Asia. J. Biogeogr. 41, 954–964. doi: 10.1111/jbi.12263
Wilson G., Rannala B. (2003). Bayesian Inference of Recent Migration Rates Using Multilocus Genotypes. Genetics 163, 1177–1191. doi: 10.1093/genetics/163.3.1177
Yahya A. F., Hyun J. O., Lee J. H., Kim Y. Y., Lee K. M., Hong K. N., et al (2014). Genetic Variation and Population Genetic Structure of Rhizophora Apiculata (Rhizophoraceae) in the Greater Sunda Islands, Indonesia Using Microsatellite Markers. J. Plant Res. 127, 287–297. doi: 10.1007/s10265-013-0613-z
Yamano H., Inoue T., Adachi H., Tsukaya K., Adachi R., Baba S. (2019). Holocene Sea-Level Change and Evolution of a Mixed Coral Reef and Mangrove System at Iriomote Island, Southwest Japan. Estuar. Coast. Shelf Sci. 220, 166–175. doi: 10.1016/j.ecss.2019.03.001
Yan Y., Duke N., Sun M. (2016). Comparative Analysis of the Pattern of Population Genetic Diversity in Three Indo-West Pacific Rhizophora Mangrove Species. Front. Plant Sci. 7. doi: 10.3389/fpls.2016.01434
Keywords: mangrove, population connectivity, stepping stones, island system, biophysical approach, propagule dispersal, drifting buoy tracking
Citation: Thomas M, Nakajima Y and Mitarai S (2022) Extremely Stochastic Connectivity of Island Mangroves. Front. Mar. Sci. 9:827590. doi: 10.3389/fmars.2022.827590
Received: 02 December 2021; Accepted: 09 May 2022;
Published: 13 June 2022.
Edited by:
Piers Dunstan, Oceans and Atmosphere (CSIRO), AustraliaReviewed by:
Ludwig Triest, Vrije University Brussel, BelgiumCopyright © 2022 Thomas, Nakajima and Mitarai. This is an open-access article distributed under the terms of the Creative Commons Attribution License (CC BY). The use, distribution or reproduction in other forums is permitted, provided the original author(s) and the copyright owner(s) are credited and that the original publication in this journal is cited, in accordance with accepted academic practice. No use, distribution or reproduction is permitted which does not comply with these terms.
*Correspondence: Maki Thomas, bWFraS50aG9tYXNAb2lzdC5qcA==
Disclaimer: All claims expressed in this article are solely those of the authors and do not necessarily represent those of their affiliated organizations, or those of the publisher, the editors and the reviewers. Any product that may be evaluated in this article or claim that may be made by its manufacturer is not guaranteed or endorsed by the publisher.
Research integrity at Frontiers
Learn more about the work of our research integrity team to safeguard the quality of each article we publish.