- 1Marine Biodiversity and Environmental Assessment Research Center, Research Institute for Global Change, Japan Agency for Marine-Earth Science and Technology (JAMSTEC), Yokosuka, Japan
- 2Marine Geology Research Group, Research Institute of Geology and Geoinformation, National Institute of Advanced Industrial Science and Technology, Tsukuba, Japan
- 3Research Laboratory On Environmentally-Conscious Developments and Technologies [E-Code], National Institute of Advanced Industrial Science and Technology (AIST), Tsukuba, Japan
- 4Biodiversity Division, National Institute for Environmental Studies, Tsukuba, Japan
- 5Japan Wildlife Research Center, Tokyo, Japan
- 6Department of Biology, Kyushu University, Fukuoka, Japan
- 7Faculty of Agriculture, University of Miyazaki, Miyazaki, Japan
- 8Coastal and Inland Fisheries Ecosystems Division, Fisheries Technology Institute, Japan Fisheries Research and Education Agency, Yokohama, Japan
- 9Department of Transdisciplinary Science and Engineering, School of Environment and Society, Tokyo Institute of Technology, Tokyo, Japan
- 10Graduate School of Agricultural and Life Sciences, The University of Tokyo, Tokyo, Japan
In this study we reviewed the use of genetic information in the Ecologically or Biologically Significant Marine Areas (EBSA) of Convention on Biological Diversity (CBD). We also evaluated genetic indicators for each criterion of important marine areas. We proposed five genetic indices, mainly based on microsatellite analysis (e.g., private allele frequency and number of cryptic species), then selected EBSAs in tropical and temperate zones of Japan based on eight coral species as a case study. Finally, we compared the results with the findings from conventional species-based EBSAs. In the EBSAs genetic information was mainly used in the Northern Hemisphere, particularly in the Baltic Sea; it was rarely applied in the Southern Hemisphere and Asian regions. Although typically applied to large organisms, genetic information is used to various organisms, including benthic and bacterial communities. Genetic data are used as indicators of diversity and endemism. Genetic indices were available for all seven EBSA criteria, but only five indices of three criteria were used. Examination of important areas of corals in the temperate zone using these indices showed that the indices without genetic indicators extracted a large number of important areas in the tropics; however, the use of genetic indicators identified important locations, including in temperate zones. Comparison with conventional, mainly species-based non-genetic methods showed less than 50% agreement, although particularly important sites in marine protected areas were identified by both methods. While there is still more work to be done, such as consideration of the number of survey sites or target species, one reason is that species-based methods tend to evaluate tropical areas higher. Therefore, these genetic indices are useful for examining important regions, particularly in temperate zones; they revealed cryptic lineages, indicating that many unknown marine taxa should be considered in vulnerable marine areas. Some indicators could be extracted with additional effort, such as population size estimation, immigration, or the use of next-generation sequencing, thus guiding future studies. Because limited genetic information was available in the early stages of EBSA selection, there is a need for systematic surveys and evaluations, particularly in the Southern hemisphere, Asian region, and in small organisms.
Introduction
The seven Ecologically or Biologically Significant Marine Areas (EBSA) criteria were introduced for identification of important areas for biodiversity at high sea under the Convention on Biological Diversity (CBD) (DFO, 2004; CBD Secretariat, 2008; Dunn et al., 2014). In 2010, numerical targets for protected areas were adopted in the CBD-COP10 as the Aichi Biodiversity Targets. Subsequently, discussions of important marine areas based on EBSA and other similar indicators have been underway in the open ocean, as well as the territorial waters and Exclusive Economic Zones of various countries (CBD, 2010; Bax et al., 2016; Asaad et al., 2017; Yamakita et al., 2017). Some of the indicators used for EBSA are based on genetic data. For example, the biodiversity criterion explicitly describes genetic diversity. In addition, the use of field genetic information (e.g., environmental DNA; eDNA) has become popular after the COP10. The application of such genetic methods is not limited to surveys of species distribution; it includes the collection of population genetic information (Ficetola et al., 2008; Tsuji et al., 2020; Miya et al., 2020). Therefore, the importance of managing genetic information for various types of ecosystem monitoring and assessment should be increase (Muller-Karger et al., 2018; Hoban et al., 2020).
Many marine taxa do not have clear correspondence between morphological species and genetic species. Recent genetic analyses of some common species have revealed cryptic species (Parsons, 1996; Bennetts et al., 1999; Krück et al., 2013; DeBiasse and Hellberg, 2015; Tyler et al., 2020). In particular, morphological identification of scleractinian corals (i.e., main constituents of coral reefs) is hampered by the paucity of species-specific morphological features and high morphological plasticity (Muko et al., 2000; Todd, 2008) accompanied by genetic complexes caused by hybridization, as well as incomplete lineage sorting (e.g., reticulate evolution) (Kenyon, 1997; Willis et al., 2006; Fukami, 2008; Richards and Hobbs, 2015). There may be many cryptic coral species in the Japanese temperate region (Fukami, 2008). Thus, it is difficult to identify species with high certainty, either using morphological features or individual-based phylogenetic analysis methods; it is essential to utilize population genetic information to evaluate hidden species boundaries and species diversity (Filatov et al., 2013; Yasuda et al., 2014; Kitano et al., 2015; Nakabayashi et al., 2019; Zawada et al., 2019). Considering the above complexity and the difficulty of using morphospecies as indicators, accurate estimation of coral biodiversity and important marine areas is difficult without geological genetic information. Therefore, we propose population genetic indicators of major coral species to fit the EBSA criteria; we performed a case study to identify important locations in the North West Pacific around the Japanese Archipelago (Yasuda et al., 2019). In addition, there is a lack of quantitative evaluations using genetic information to select important areas. To assess the utility and representativeness of genetic information as an indicator of important areas, case studies are needed to compare the results of genetic index-based methods and classical species or community-level methods. This would allow better identification of important areas for conservation from the perspective of land/seascapes and biogeography.
Here, we systematically review the use of genetic information in the reports of the EBSA regional workshops under the CBD. We focus on bias according to region, criteria, and organism. We also describe the genetic indices used for EBSA proposed by Yasuda et al. (2019), as well as the correspondence between genetic indices and the EBSA criteria; we perform a spatial comparison of the results. Finally, we compare the proposed genetic EBSA indicators and existing indicators, which are typically based on conventional biological community information.
Methods
Review of Studies Used for the EBSA Criteria, Including Genetic Indices
All 17 EBSA regional workshop reports published on the CBD website as of April 2020 (15 regions including two updates regarding the northeast Atlantic) were investigated (https://www.cbd.int/ebsa/). A list of target areas, criteria, and target organisms referring to “genetic*” was extracted. In particular, when a reference contained “genetic*,” we examined whether the genetic information was reflected in the text. We also extracted information regarding target ecosystems and organisms to assess potential target biases. To show regional differences concerning the relative number of areas that use genetic information, we conducted statistical tests using the Base and RVAideMemoire packages (v. 0.9-80) in R software. Fisher pairwise multiple comparison was applied to the comparison of each regional data. For hypothesis testing, we evaluated the difference between Asian regions and other areas, and the difference between the Northern and Southern Hemispheres. We performed Fisher’s exact test for this purpose. For the analysis of the regional difference, we limited the data only for the areas approved by CBD and recent candidate (OSPAR/North East Atlantic).
Potential Indicators and Proposed Important Marine Areas
We reanalyzed population genetics indicators to select EBSA of coral reefs on the Japanese coast (Yasuda et al., 2019). To understand the background of the indicators, we listed and ranked potentially applicable indicators based on expert discussion, then described the reasons for selecting specific indicators.
For the genetic indicators we used the number of unique alleles as criterion 1 (uniqueness or rarity). The cloning rate and the presence of environmental adaptive genes served as criterion 4 (vulnerability, fragility, sensitivity, or slow recovery). The number of cryptic species and the allelic richness constituted criterion 6 (biological diversity).
Specimens were collected in 31 regions from more than 4000 colonies, including 18 regions in temperate areas (Figure 1). Among these regions, Tatsukushi in Tosa-shimizu City, Kouchi in western Shikoku Island, and Sekisei Lagoon in Ishigaki City in western Okinawa were selected for restoration area of coral reefs based on the Law for the Promotion of Nature Restoration. Locations at the tip of the Japanese peninsula, on the Pacific side of Japan, have large coral reefs, even in temperate areas.
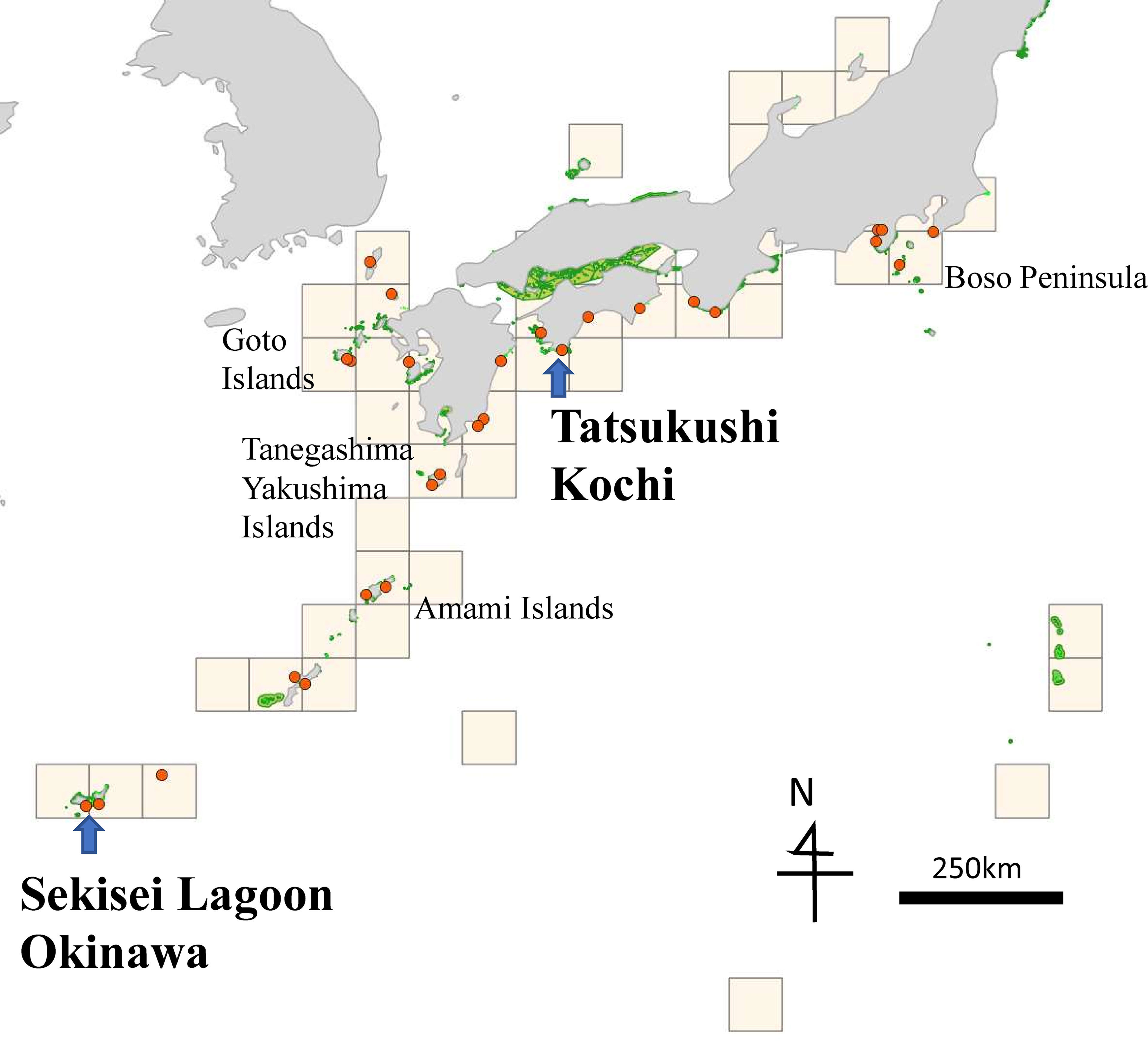
Figure 1 Study site and grid. Dots, sampling locations; arrows, areas for restoration of coral reefs; green, national parks and marine parks.
The genetic values of indicators based on microsatellite analysis were extracted for Acropora pruinosa (Brook, 1892), Acropora solitaryensis (Veron and Wallace, 1984), Acropora cf. glauca (Brook, 1893), Acropora hyacinthus complex (Dana, 1846) (Kitano et al., 2020), Pocillopora damicornis (Linnaeus, 1758), Pocillopora acuta (Lamarck, 1816), Pocillopora verrucosa (Ellis and Solander, 1786), and Heliopora coerulea (Pallas, 1766) (Published data from Yasuda et al., 2014; Kitano et al., 2015; Nakabayashi et al., 2019; Yasuda et al., 2019; Pipithkul et al., 2021, unpublished raw data also available at https://doi.org/10.5061/dryad.7pvmcvdv3 and orijinal values of estemated indicators are avirable on the Supplementary Table Data Sheet 1); most of these are dominant taxa, particularly in temperate areas.
To extract each indicator, we considered the mean number of unique alleles for each (newly identified) subspecies of the above taxa, except H. coerulea, which exists in a particularly limited area. We averaged the clone rate of two species with sufficient specimens—A. hyacinthus and A. pruinosa. We evaluated the presence/absence of allele G at C29226S281 which previously applied for Acropora species (Jin et al., 2016) for the species A. hyacinthus is the only one species with sufficient genetic information and most widely distributed species not limited to temperate like other species. We counted the number of taxa, including newly identified subspecies (cryptic species). We considered the mean allelic richness, standardized according to genus. Allelic richness was calculated as the percentage per genet in the sampled regions. We used FSTAT ver. 2.9.3.212 (Goudet, 2002) to calculate allergic richness for each population. This program uses rarefaction to standardize allelic richness to the smallest N in a comparison.
For the indicators which were not used genetic information we used latter variables (details of some indicators are explained in Supplementary Document Data Sheet 2). For Criterion 2 (special importance for life history stages of species), we used the center of connectivity networks according to ocean currents (Nakabayashi et al., 2019). For Criterion 3 (importance for threatened, endangered, or declining species and/or habitats), we used the number of endangered coral species habitat using range of each species (Ministry of the Environment Japan, 2009; Sugihara et al., 2015). For Criterion 5 (biological productivity), we used the mean of current coral area (Nature Conservation Bureau Environment Agency and Marine Parks Center of Japan, 1994) and predicted future coral area (Yamakita, 2018). For Criterion 7 (naturalness), we used the length of natural coast (Nature Conservation Bureau Environment Agency and Asia Air Survey Co. Ltd., 1994). To predict future coral area, we applied a model that used the generalized linear method for the areas of corals in an approximately 10-km mesh. As environmental variables, we used the winter sea-surface temperature, chlorophyll a, particulate inorganic carbon, water depth, coastline length, wave height, and tidal range (Kumagai et al., 2022). The predicted area was limited to existing areas of coral reefs and algal beds. The correlation of the model with the current status was 0.64.
EBSA extraction was conducted in two regions of the Japanese Archipelago (Figure 1). One region comprised all areas both temperate and tropical zones (areas with tropical zones hereafter); the other region comprised only temperate zones. This is because other studies have shown a biogeographic boundary due to the Kuroshio Current (Kai et al., 2022), and our results also show a boundary of genetic differences between temperate and tropical zones for several coral species. The values of each indicator were ranked as low, middle, or high, in accordance with the EBSA selection process by the calculation described in Yamakita et al. (2015). Although the highly evaluated places met the EBSA criteria, we conducted further prioritization using multiple criteria that have been proposed for systematic EBSA selection (Ardron et al., 2014; Yamakita et al., 2015; Yamakita et al., 2017). Data used for this calculation of was provided as Supplementary Table Data Sheet 1.
For prioritization we used the mean of seven criteria, number of highly evaluated criteria (count Max hereafter) among seven criteria, means of three criteria that used genetic valuables, and count Max among the three criteria. The result of prioritization was ranked as low, middle, or high. Other prioritization methods such as complementarity analysis using Maxan were not used (Ball and Possingham, 2000) because of the difficulty comparing result of areas with tropical zones and temperate zones only which has different number of no data places by this analysis. The analysis was conducted using 1° grids. For grids with multiple sample sites, the mean value after ranking was used for analysis.
We evaluated differences in the proportion of highly evaluated grids between tropical and temperate zones. Fisher’s exact test was conducted for each criterion, with Bonferroni correction for multiple comparisons. Next, the sums of the numbers of highly evaluated grids for criteria-based (Criteria 1, 4, and 6) or non-criteria-based (Criteria 2, 3, 5, and 7) genetic indicators were used to compare highly evaluated grids between tropical and temperate zones. We used the exact conditional test of independence, a test for three-way categorical table runs, with the Cochran-Mantel-Haenszel chi-squared test function in R software.
Comparison of Important Marine Areas According to Genetic and Species Diversity
Important areas selected by genetic indicators were compared with areas selected by conventional assessment based on species (Yamano et al. in preparation and partly published in the latter reports [Shirayama, 2016; Kitano et al., 2020]; compiled data also available in the appendix). The conventional species-based assessment used the following indices for important corals off the coast of Japan.
Criterion 1 (uniqueness or rarity) was ranked via complementarity analysis of endemic species. Criterion 2 (special importance for life history stages of species) was evaluated according to ocean current connectivity using the larvae transport model of Marine Geospatial Ecology Tools (Roberts et al., 2010; Treml et al., 2012). Criterion 3 (importance for threatened, endangered, or declining species and/or habitats) was based on the number of Red List species in Japan and ranked via complementarity analysis (https://www.env.go.jp/nature/kisho/sango_tokusei.html). Criterion 4 (vulnerability, fragility, sensitivity or slow recovery) was evaluated using coverage change after bleaching in 1998. Criterion 5 (biological production) was evaluated using coral reef development based on water temperature (18°C, 13°C, and 10°C of the coldest month). For Criterion 6 (biological diversity), the number of species was used. For Criterion 7 (naturalness), the ratio of natural coast was used (Nature Conservation Bureau Environment Agency and Asia Air Survey Co. Ltd., 1994). Although assessment based on species (Shirayama, 2016) considers two methods to integrate seven criteria (i.e., the use of different datasets and the use of distinct thresholds), we considered only the similar method of integration for this analysis (i.e., mean and count Max of seven criteria, and the mean and count Max of the three criteria that used for genetic variables).
To compare the genetic and conventional evaluations, we applied Cohen’s kappa statistic, which represents coincidence on a scale of -1 to 1; a value of > 0.6 is considered significant coincidence and a higher value indicates a stronger relationship (Yamakita et al., 2019).
Results
Review of Studies Using Genetic Indices in EBSAs
Among the 16 regional reports, genetic information was included in 14 (Table 1). Genetic information was used 40 cases for seven criteria in 347 EBSAs and candidate areas (2%). The percentage was also 2% using five criteria as the denominator, excluding two criteria (Criteria 5 [biological productivity] and 7 [naturalness]) that were not based on genetic information.
Thirty-five areas had genetic information (10% of 347 EBSAs) (Table 1 and Figure 2). Red shapes indicate areas where genetic indicators were used. Areas with genetic information were in the Baltic Sea, northeast Atlantic, northwest Atlantic (Arctic, Caribbean Sea/western mid-Atlantic), and eastern tropical and temperate Pacific. The differences were significant for Baltic Sea vs. East Asia and Baltic Sea vs. southeastern Atlantic (p < 0.05; pairwise comparisons using Fisher’s exact test with Hochberg adjustment for multiple comparisons. Note that the 16 regions were regarded as different groups). A significant difference was also detected between the Asia and other regions; Northern and Southern Hemispheres (p = 0.01 and p = 0.05; Fisher’s exact test).
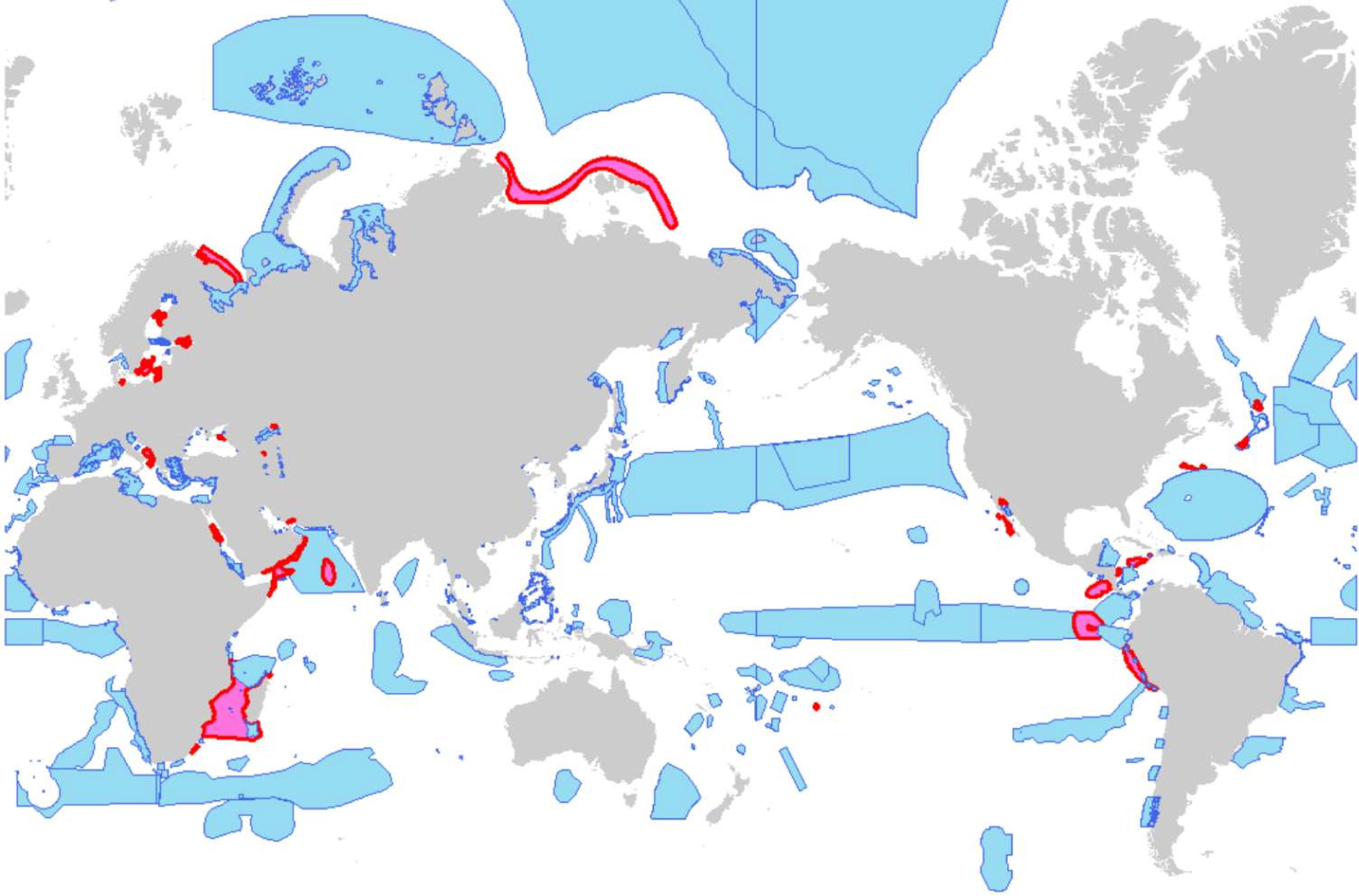
Figure 2 EBSAs in the CBD repository (added information about OSPAR/North East Atlantic) and distribution of use of genetic information. Red and blue, areas that did and did not, respectively, use genetic information.
As target organisms, cetaceans (whales and dolphins) were used most frequently (15 times). Invertebrates (except coral) and fish were used 14 and 12 times, respectively; and general statements about genetic diversity that do not refer to any particular species was tied with birds (9 times). Bacterial communities were used as the target organisms twice, as representatives of hydrothermal and deep habitats. The same study or series of studies by the same authors tended to be cited for a particular region, such as birds in the northeast Atlantic. This is presumably because of insufficient data or because regional workshops are not required to review all genetic research.
Corals were used as target organisms of genetic evaluation seven times including two locations which did not appeared on the CBD approved area. These included three deep sea corals and two studies that used different criteria in the same areas. The studies demonstrated the distribution of endangered species, genetic isolation, human impact, genetic connectivity, and discontinuity (Le Goff-Vitry and Rogers, 2005; Miller et al., 2008; Magalon et al., 2011; Morrison et al., 2011; Ross et al., 2017).
Genetic evaluation was performed via phylogenetic tree construction using mitochondrial 16S DNA and intraspecific analyses of diversity and connectivity that involved microsatellite markers (Le Goff-Vitry and Rogers, 2005; Morrison et al., 2011; Ross et al., 2017). Although most analyses were population-based, two focused on invertebrate communities. No study focused on genetic data for multiple species.
Case Study of Important Marine Areas: Selection of Indicators
The following genetic indicators are listed in Table 2: 1) genetic diversity; 2) genetic structure, determined by simple clustering or structure analysis; 3) inter- and intra-genetic structures of populations; 4) phylogenetic analysis; 5) almost-fixed model of migration; 6) and 7) advanced flexible simulations (e.g., migration and branching); 8) population history; and 9) adaptation, determined by simple detection or using statistics. Use of the zooxanthellae genetic structure was excluded based on data availability and analysis complexity.
Next, we identified indicators that represented the EBSA criteria and indicators that were readily measurable; these were used to assess genetic indicators of EBSA. Table 3 shows criteria representativeness from one (low) to five (high).
With respect to genetic diversity, the number of private alleles was considered; this potentially represents Criteria 1 and 6. The number of private alleles increases in the presence of genetic structure or local adaptation. Therefore, it was considered an appropriate indicator of diversity or uniqueness.
Allelic richness was also considered as an indicator of Criteria 6 and 4. A higher allelic richness value indicates a larger population and significantly greater population structure. However, when the degree of population structure is high, local diversity may be low despite high overall diversity. Furthermore, harmful alleles may accumulate when the population size decreases.
With respect to genetic structure, genetic structure analysis and identification of hidden lineages was considered. The discovery of hidden species is likely to fall under Criteria 1 and 6. Hidden lineages fell under Criterion 3. The reliability of other analysis methods will be affected by these results because they assume a uniform genetic structure within the local population.
With respect to structure among and within populations, genetic distance (Fst) was regarded as an indicator of gene flow. By examining the centrality of the network based on the genetic distance, this value indicates Criterion 2 over long periods. In addition, the edge of the network contains habitat for endangered species (i.e., Criterion 3). Isolation in the network could be an indicator of Criterion 4 in some instances. Criterion 5 represents the inverse of diversity. A high genetic flow reduces the number of locally specific characteristics but increases overall adaptation in the target area.
Fis, the inbreeding coefficient, was used as an indicator of structure among and within populations. If the inbreeding percentage is high, the target population is likely to have high vulnerability (Criterion 4). A high rate of inbreeding corresponded to Criteria 3 (endangered species), 5, and 6.
With respect to branching relationships between populations or individuals, we did not consider specific indicators for each location. Such analysis involves the visualization of phylogenetic relationships, which are calculated from genetic distances (e.g., Fst). Although the result was used to create clustered zones, it was not a sufficient indicator to show the status of each location. In terms of population differentiation, the numbers of overlapping genetic boundaries between multiple and cryptic species were considered. Because of the plethora of boundaries, we included in the analysis only boundaries between temperate and tropical zones.
The migration rate was calculated by assignment test. Based on the migration rate, the centrality of the network was calculated as an indicator of Criterion 2 (importance in life history). Furthermore, the percentage of self-seeding was regarded as an indicator of biological productivity in the target taxon. The assignment test result is also related to the origin of diversity and the hierarchical structure of differentiation. Therefore, exchange of diversity (overall or local) should be considered when analyzing diversity. Calculation of this index based on Fst results in significant variance and is dependent on the model. Therefore, it is important to specify the target of analysis and establish appropriate assumptions prior to the analysis. Because of these reasons migration rate was not used in our case study.
With respect to population history, clonal structure and effective population size were considered as suitable indicators. Clonal rate was presumed to decrease the value (score) of Criterion 6 (genetic diversity) and increase the score of Criterion 4 (vulnerability). It could also be an indicator of Criterion 5 (productivity) for specific clades such as staghorn corals in a local area. However, most species targeted in this study do not reproduce often. Therefore, regardless of whether corals are quantitatively increased, productivity does not contribute to their reproduction. Thus, productivity may not be an effective indicator. Some taxa that propagate by fragmentation (e.g., staghorn corals) or a regional colony of large populations may also correspond to Criterion 1 or Criterion 2 in addition to Criterion 5. However, such cases will be specific to species or region. Although fragmentation may increase the clonal rate in corals, sexual reproduction is the main mechanism to maintain their heterozygosity or genetic diversity (Ayre and Hughes, 2004). In addition, the vulnerability of clonal populations to environmental change is situation-dependent (Lasker and Coffroth, 1999).
Notably, the effective population size can be estimated based on heterozygosity. Although it is difficult to set the generation time for corals, whole-genome analysis enabled estimation of past population history. Estimated population size can be used as an indicator of Criterion 5. The population history and differentiation size can be used as indicators of Criterion 6. In addition, past changes in the population may enable assessments of vulnerability and susceptibility (Criterion 4). We considered the presence or absence of environment-adaptive genes. We examined stress-tolerance genes (Jin et al., 2016), particularly for high water temperatures, as an indicator of Criterion 4 and uniqueness (Criterion 1). This aspect also might be related to Criterion 5, in terms of adaptation. If different alleles are adaptive in different populations, the diversity (Criterion 6) of the population might also be increased.
We applied five genetic indicators for EBSA Criteria 1, 4, and 6 on the Japanese coast. The number of private alleles was used for Criterion 1. The clonal rate and the presence of environmental adaptive genes were used for Criteria 4. The number of cryptic species and the allelic diversity were used for Criterion 6. We also considered genetic indicators for Criteria 2, 3, and 5; however, we did not use any of these indicators. For Criterion 2, only a weak correlation was observed between genetic distance and the distance matrix based on ocean currents. In addition, similar geographical distribution were observed between genetic distance and other genetic indices. Therefore, we did not adopt this genetic connectivity indicator; we used a distance matrix based on ocean currents. However, genetic distance was used as a reference to separate areas for analysis (i.e. separation of temperate and tropical was supported by genetic distance). For Criterion 3, we considered allele heterozygosity and the number of cryptic species. However, accuracy was low for allele heterozygosity because of the small sample size; the number of cryptic species was more appropriate as an indicator of biodiversity. For Criterion 5 (productivity), the estimated population according to genetic analysis was not used because availability of data on coral reef area, the inadequate accuracy of heterozygosity-based estimation, and variation in the estimated population, which depends on the model assumptions.
Case Study of Important Marine Areas: Results
Figure 3 shows the results of the evaluation of indicators for EBSA criteria. The raw values were ranked high, middle, or low, as instructed in the EBSA protocol (Figures S-1-3-1A in Supplementary Document Data Sheet 2). If there were two locations in the same grid, the values were averaged. The tropical zone was highly rated for some indicators. For Criteria 5, 6, and 7, high values were rare in the temperate zone. For Criteria 2 and 4, high values tended to be found in the temperate zone. Comparison of the ratio of highly evaluated grids between the temperate and tropical zones yielded a significant difference for Criterion 5 (p < 0.007; significance level after Bonferroni adjustment for multiple comparisons). Furthermore, the numbers of highly evaluated grids significantly differed in the temperate and tropical zones between the genetic (Criteria 1, 4, and 6) and non-genetic (Criteria 2, 3, 5, and 7) criteria (p = 0.005, exact conditional test of independence in 2 × 2 × 2 tables). Therefore, the degree of bias in tropical zones differed according to whether criteria were based on genetic information.
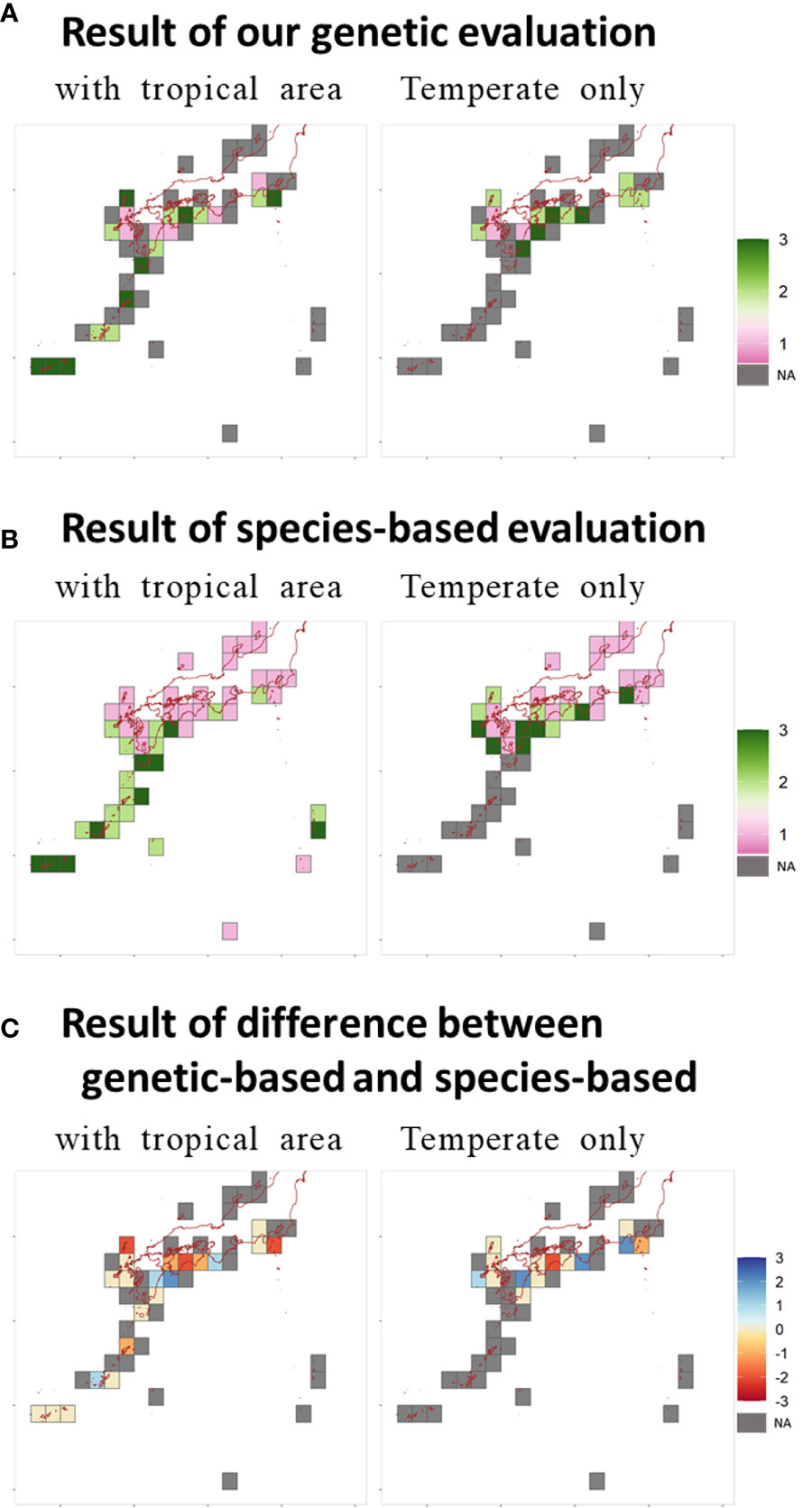
Figure 3 Distribution of results for (A) integrated result for all criteria, (B) integrated result for all criteria for species-based evaluation, and (C) difference between genetic and species-based evaluation. NA, not available.
Integration of the seven criteria is shown in Figure 3A. Areas around the Sekisei Lagoon, Amami Oshima Island, and Yakushima–Tanegashima Island were stably selected in the tropical zone. Upon integration of temperate-zone data alone, Miyazaki and Kochi Prefectures (the southernmost areas affected by the Kuroshio Warm Current) were identified as important. Sekisei Lagoon and Kochi were selected as coral restoration areas (Figure 1). Integration of the results of genetic indicators only (Figures S-1-3-1C) identified several major discrete coral reef areas from the Boso Peninsula (northern limit of the Pacific Ocean) to the Goto Islands (northwest Kyushu).
Comparison of Proposed Important Marine Areas Based on Genetic and Species Diversities
Figure 3B shows the integrated results for species-based assessment and the difference in the results for species-based and genetic evaluation. Positive values indicate that genetic information was highly valued. Species-based criteria tended to yield a larger number of highly evaluated areas in the tropical zone (Figures 3B and S-1-3-2A). Comparison of the rates of highly evaluated grids between temperate and tropical zones yielded a significant difference for Criteria 3, 5, 6, and 7 (p < 0.007; significance level after Bonferroni adjustment for multiple comparisons). There was also a significant difference in the number of highly evaluated grids in temperate and tropical zones between criteria that were (Criteria 1, 4, 6) and were not (Criteria 2, 3, 5, and 7) used on genetic indicators for the genetic analysis (p < 0.001, exact conditional test of independence in 2 × 2 × 2 tables).
The results of integrating the seven criteria ware compared as the rates of the number of highly evaluated grids identified by both methods (i.e., agreement). For the areas with tropical zone, the rates of agreement were 5/12 and 5/6, κ = 0.3 based on the mean (agreement divided by highly evaluated grids by genetic method, the agreement by species based method, and kappa statistic successively); they were 4/6 and 4/8, κ = 0.4 based on the count Max (Figures 3C and S-1-3-2C). For the temperate zone, the rates of agreement were 3/6 and 3/4, κ = 0.4 based on the mean; they were 2/6 and 2/3, κ = 0.2 based on the count Max (Figures 3C and S-1-3-2C). The agreement of highly evaluated grids was higher for locations evaluated for the areas with tropical zone. However, the kappa value did not indicate high correspondence.
Integration of the three criteria (Criteria 1, 4, 6) using genetic indicators in the areas with tropical zone yielded agreement rates of 3/7 and 3/6, κ = 0.2 based on the mean; the rates were 6/13 and 6/10, κ < 0.1 based on the count Max (Figures S-1-3-2D). For the temperate zone, the rates of agreement were 3/5 and 3/4, κ = 0.5 based on the mean, they were 3/7 and 3/5, κ = 0.3 based on the count Max.
There were no significant negative or positive relationships between the results of genetic and species-based indicators. This means that there are some differences between methods, and the two are complementary especially in temperate zone. However, important areas such as areas for restoration of coral reefs (Tatsukushi in Kochi and Sekisei Lagoon in Okinawa), were selected in all cases.
Discussion
Biases in EBSA Selection and Potential Use of Genetic Variables
Most of the criteria lacked genetic information; few of the areas used such information. The geographical bias of the evaluation toward the Northern Hemisphere and non-Asian regions, and toward larger and/or iconic animals, suggest that research bias is linked to the use of genetic information (Mayer et al., 2007; Webb et al., 2010; Hughes et al., 2021). However, there are cases that consider variety in the types of organisms, suggesting feasibility for the use of such genetic methods in analyses of other organisms and/or ecosystems.
Collection of the species-distribution data used to select EBSAs is facilitated by global databases such as the Ocean Biodiversity Information System (OBIS) and the Global Biodiversity Information Facility (GBIF). In the case of tropical Asia, for which genetic information was lacking, > 49,000 occurrence data were recently updated (Yamakita et al., 2017; Sudo and Nakaoka, 2020; Sudo et al., 2021; Takeuchi et al., 2021). These contributed increasing-use cases for the analysis of important areas (Beazley et al., 2016; Guijarro et al., 2016; Yamakita et al., 2017; Furushima et al., 2019) and revealed global biodiversity patterns/dynamics (Chaudhary et al., 2016). Several works referred to national/regional spatial planning either directly or indirectly (Gormley et al., 2013; Nature Conservation Bureau Ministry of the Environment Government of Japan, 2014; Shirayama, 2016). Therefore, databases, species distribution data, and species distribution modelling influence the identification of important marine areas, particularly in the context of insufficient data.
For the collection of the genetic data, GenBank and the European Nucleotide Archive were established in 1982; the DNA Data Bank of Japan was established in 1986 (Benson et al., 2013) which is more than 10 years earlier than OBIS and GBIF. Data linked to a geographical location and standardized for a particular marker can be used to identify geographical or temporal differences (e.g., farm crops) (Hijmans and Spooner, 2001; Gratton et al., 2017). Although databases lack useful information regarding marine species that do not use the same marker, the recent increase in the number of readable genes will enable standardization. For example, genome-wide genotyping by MIG-seq (Suyama and Matsuki, 2015) enables the identification of single nucleotide polymorphisms using a universal primer set. Therefore, it can be used to detect cryptic species and other genetic differences as a future perspective (Hoban et al., 2020).
The use of coral DNA is hampered by the lack of variability in mitochondrial DNA (Hellberg, 2007; Shearer and Coffroth, 2008), which is typically used to estimate inter- and intra-species differences (Avise, 2000; Bowen et al., 2014). However, next-generation sequencing enables the assessment of genome-wide genetic variation (Iguchi et al., 2019; Takata et al., 2019). eDNA analysis, which targets mitochondrial DNA, is difficult to apply to corals because of low resolution to identify coral species; nonetheless, trials involving nuclear DNA regions with high resolution are underway (Alexander et al., 2020). eDNA analysis is also capable of detecting adaptive genetic markers, coralline algae that promote coral settlement, and macro algae that compete with corals, thereby enabling the evaluation of coral distribution in terms of interspecies interactions. eDNA analysis also can provide the detailed biodiversity information of bacteria and plankton communities in coral ecosystems, and probably it would be a useful tool to evaluate the healthy and preferable environments for coral propagation.
In addition to the lack of genetic information, the structure of EBSA workshops may lead to bias. The proposed area of each EBSA initially relied on the opinions of a small number of experts. Although the proposal was made after review of potential EBSAs in each geographic unit, there were limited time and information available for the review. This was partly because completion of the EBSA identification work was required soon after the CBD-COP10. The possibility of providing more global data and systematic information was suggested after the first round of the workshop (Ardron et al., 2014; Bax et al., 2016). Because the aim was to establish protected areas by negotiation after the identification of scientifically important marine areas, strong evidence, high resolution, and geographically wide genetic and species factors were needed. A flame work for the data accumulation and integrative analysis of such data (especially including genetic data) is needed to elaborate a new strategic plan (CBD Secretariat, 2020; Hoban et al., 2020; Laikre et al., 2020).
Usefulness of the Proposed Indicators and Perspectives
At least three of seven EBSA criteria can be evaluated using population genetic indicators. Genetic indicators of connectivity, productivity, and naturalness had different feasibilities. Connectivity can be evaluated immediately using pre-existing numerical values. In the future, productivity may be evaluated using genetic measures if an appropriate survey design and analysis of local population structures are employed. Naturalness may not be evaluated using genetic measures. It may need to combine with other measures, such as the use of coral growth rings or human activity itself.
Although it is not essential to evaluate all criteria, nor is it required to use genetic indicators for all criteria, the use of genetic information is expected to increase over time. Genetic data have not been used for indicators of EBSA, other than biodiversity and endemism, partly because of insufficient examples. Each EBSA criterion has an assumed levels of ecological hierarchy; most criteria are considered compatible with community- and species-level indicators (Asaad et al., 2017). However, there were levels in which genetic data contributed to indicators as well as species-level and community-level indicators. In addition, the adaptability of indicators to multiple criteria (Table 3) and the similarity of the criteria suggests that prioritization including weighting of the criteria, or visual representation used in integrated evaluations may be preferable [e.g., ecosystem health or services (Halpern et al., 2013; Yamakita et al., 2020)]. For such representation more amount of systematic sampling will be needed in the future. Recently, the world wide application of eDNA surveys has been considered in future planning for the Asia–Pacific and marine components of The Group on Earth Observations, Biodiversity Observation Network (AP-BON and MBON) (Muller-Karger et al., 2018; Takeuchi et al., 2021). Such efforts have already begun in some places, such as the ANEMONE project in Japan. A much more ideal approach would be to collect specimens directly in many locations. However, water sampling is probably a more easily accessible way to obtain genetic data on dominant corals on a global scale.
The application of genetic indices to the major taxa, including dominant species, allowed the identification of important areas of coral reefs, particularly in the temperate zone. The biogeographic boundary between the Amami Islands of Kagoshima Prefecture and the Tanegashima and Yakushima Islands, which are separated by the Kuroshio Current, has been established (Sato, 1969). Although this boundary is not definitive for marine organisms (Sakai et al., 2009), it was consistent with several genetic boundaries detected in the present study and a previous study (Nakabayashi et al., 2019).
The two types of assessments yielded similar results on a broad spatial scale especially for nature restoration area and important areas for conservation efforts are identified as well as selection based on species. However, they did not show sufficiently high agreement with the species-based indicators. Therefore, a more detailed study is needed. There is a need to determine whether the proposed indicators adequately reflect the biodiversity of the target taxa. The small number of sites, limitation of used species, and criteria; use of means for integration; and lack of information regarding rare species are the weaknesses of the present study. In addition, some issues remain, such as understanding the regional historical changes in biodiversity (e.g., temporal change and biogeography of species distributions). A notable finding of this study was the selected EBSAs for corals in the temperate zone, which will inform future conservation planning. Integrated assessment of species-based and genetic methods will be complementary and useful for investigations of important marine areas.
In conclusion, genetic surveys have become easier to conduct; species distribution and biodiversity can be assessed. However, the CBD targets did not consider enough for genetic diversity (CBD Secretariat, 2020; Hoban et al., 2020; Laikre et al., 2020). Using the EBSA report of CBD as an example, we quantified the shortfalls in marine biodiversity assessments using genetic information in terms of geographic area, species, and criteria. We also conducted a case study concerning the application of genetic indicators in assessments of important areas using the EBSA criteria and revealed important areas in the temperate zone, particularly for coral reefs. Our findings will enhance assessments based on macro ecological and biogeographic analysis using genetic information.
Data Availability Statement
The datasets presented in this study can be found in online repositories or Supplementary Material. The names of the repository/repositories and accession number(s) can be found in the article/Supplementary Material.
Author Contributions
TY, NY, AI, and YK conceived the idea. FS, YK, TY, AN, AS, HA, NY, TN, KT, and SN compiled the data. TY analyzed the compiled data. TY, NY, AI, YK, FS, KT, TN, and SN write and/or commented on the paper. All authors contributed to the article and approved the submitted version.
Funding
We would like to thank the support by the members and/or funding of the Environmental Research and Technology Development Fund (4RF–1501[JPMEERF20154R01], 4–1304, S9, and S15 PANCES[JPMEERF16S11530]) of the Ministry of the Environment; a Grant-in-Aid for Young Scientists (A) (17H04996), JSPS Bilateral Joint Research (JPJSBP120209929), JSPS KAKENHI Grant Number 20H00653, by Tenure Track Facilitation and Fixing Project of Miyazaki University and support of the Research Laboratory on Environmentally-conscious Developments and Technologies (E-code) at the National Institute of Advanced Industrial Science and Technology (AIST).
Conflict of Interest
The authors declare that the research was conducted in the absence of any commercial or financial relationships that could be construed as a potential conflict of interest.
Publisher’s Note
All claims expressed in this article are solely those of the authors and do not necessarily represent those of their affiliated organizations, or those of the publisher, the editors and the reviewers. Any product that may be evaluated in this article, or claim that may be made by its manufacturer, is not guaranteed or endorsed by the publisher.
Acknowledgments
We acknowledge the support of the Research Laboratory on Environmentally-conscious Developments and Technologies (E-code) at the National Institute of Advanced Industrial Science and Technology (AIST), and the Tenure Track Facilitation and Fixing Project of Miyazaki University. We also would like to thank Hiroya Yamano for providing the information of the part of comparison to conventional way of analysis.
Supplementary Material
The Supplementary Material for this article can be found online at: https://www.frontiersin.org/articles/10.3389/fmars.2022.823009/full#supplementary-material
References
Alexander J. B., Bunce M., White N., Wilkinson S. P., Adam A. A. S., Berry T., et al. (2020). Development of a Multi-Assay Approach for Monitoring Coral Diversity Using eDNA Metabarcoding. Coral Reefs 39, 159–171. doi: 10.1007/s00338-019-01875-9
Ardron J. A., Clark M. R., Penney A. J., Hourigan T. F., Rowden A. A., Dunstan P. K., et al. (2014). A Systematic Approach Towards the Identification and Protection of Vulnerable Marine Ecosystems. Mar. Policy 49, 146–154. doi: 10.1016/j.marpol.2013.11.017
Asaad I., Lundquist C. J., Erdmann M. V., Costello M. J. (2017). Ecological Criteria to Identify Areas for Biodiversity Conservation. Biol. Conserv. 213, 309–316. doi: 10.1016/j.biocon.2016.10.007
Avise J. C. (2000). Phylogeography: The History and Formation of Species. Cambridge: Harvard University Press. doi: 10.5860/choice.37-5647
Ayre D. J., Hughes T. P. (2004). Climate Change, Genotypic Diversity and Gene Flow in Reef-Building Corals. Ecol. Lett. 7, 273–278. doi: 10.1111/j.1461-0248.2004.00585.x
Ball I. R., Possingham H. P. (2000). “MARXAN (V1. 8.2)”, in Marine Reserve Design Using Spatially Explicit Annealing, a Manual. Brisbane: University of Queensland.
Bax N. J., Cleary J., Donnelly B., Dunn D. C., Dunstan P. K., Fuller M., et al. (2016). Results of Efforts by the Convention on Biological Diversity to Describe Ecologically or Biologically Significant Marine Areas. Conserv. Biol. 30, 571–581. doi: 10.1111/cobi.12649
Beazley L., Kenchington E., Murillo F. J., Lirette C., Guijarro J., McMillan A., et al. (2016). Species Distribution Modelling of Corals and Sponges in the Maritimes Region for Use in the Identification of Significant Benthic Areas. Canadian Technical Report of Fisheries and Aquatic Sciences. 3172 Nova Scotia: Fisheries and Oceans Canada.
Bennetts R. Q., Grady J. M., Rohde F. C., Quattro J. M. (1999). Discordant Patterns of Morphological and Molecular Change in Broadtail Madtoms (Genus Noturus). Mol. Ecol. 8, 1563–1569. doi: 10.1046/j.1365-294x.1999.00709.x
Benson D. A., Cavanaugh M., Clark K., Karsch-Mizrachi I., Lipman D. J., Ostell J., et al. (2013). GenBank. Nucleic Acids Res. 41, D36–D42. doi: 10.1093/nar/gks1195
Bowen B. W., Shanker K., Yasuda N., Maria M. C., Von Der Heyden S., Paulay G., et al. (2014). Phylogeography Unplugged: Comparative Surveys in the Genomic Era. Bull. Mar. Sci. 90, 13–46. doi: 10.5343/bms.2013.1007
CBD (2010). “Strategic Plan for Biodiversity 2011–2020,” in Montreal: Secretariat of the Convention on Biological Diversity (Canada: Secretariat of the Convention on Biological Diversity Montreal), 10–11.
CBD Secretariat (2008). “COP 9 Decision IX/20. Marine and Coastal Biodiversity,” in Ninth Meet. Conf. Parties to Conv. Biol. Divers, 19 - 30 May 2008. 113–124. Available at: https://www.cbd.int/decisions/cop/?m=cop-09.
CBD Secretariat (2020) Zero Draft of the Post-2020 Global Biodiversity Framework, CBD/Wg2020/2/3. Available at: https://www.cbd.int/doc/c/efb0/1f84/a892b98d2982a829962b6371/wg2020-02-03-en.pdf.
Chaudhary C., Saeedi H., Costello M. J. (2016). Bimodality of Latitudinal Gradients in Marine Species Richness. Trends Ecol. Evol. 31, 670–676. doi: 10.1016/j.tree.2016.06.001
DeBiasse M. B., Hellberg M. E. (2015). Discordance Between Morphological and Molecular Species Boundaries Among Caribbean Species of the Reef Sponge Callyspongia. Ecol. Evol. 5, 663–675. doi: 10.1002/ece3.1381
DFO (2004). “Identification of Ecologically and Biologically Significant Areas,” in Can. Sci. Advis. Sec. Ecosystem Status Rep. 2004/006 [Canda: Fisheries and Oceans Canada (DFO)], 15.
Dunn D. C., Ardron J., Bax N., Bernal P., Cleary J., Cresswell I., et al. (2014). The Convention on Biological Diversity’s Ecologically or Biologically Significant Areas: Origins, Development, and Current Status. Mar. Policy 49, 137–145. doi: 10.1016/j.marpol.2013.12.002
Ficetola G. F., Miaud C., Pompanon F., Taberlet P. (2008). Species Detection Using Environmental DNA From Water Samples. Biol. Lett. 4, 423–425. doi: 10.1098/rsbl.2008.0118
Filatov M. V., Frade P. R., Bak R. P. M., Vermeij M. J. A., Kaandorp J. A. (2013). Comparison Between Colony Morphology and Molecular Phylogeny in the Caribbean Scleractinian Coral Genus Madracis. PloS One 8, e71287. doi: 10.1371/journal.pone.0071287
Ellis J., Solander D. (1786). The Natural History of Many Curious and Uncommon Zoophytes. London: Benjamin White and Son. 208 pp.
Fukami H. (2008). Short Review: Molecular Phylogenetic Analyses of Reef Corals. Galaxea J. Coral Reef Stud. 10, 47–55. doi: 10.3755/galaxea.10.47
Furushima Y., Yamakita T., Miwa T., Lindsay D., Fukushima T., Shirayama Y. (2019). “New Techniques for Standardization of Environmental Impact Assessment,” in Environmental Issues of Deep-Sea Mining (Cham: Springer International Publishing), 275–313. doi: 10.1007/978-3-030-12696-4_11
Gormley K. S. G., Porter J. S., Bell M. C., Hull A. D., Sanderson W. G. (2013). Predictive Habitat Modelling as a Tool to Assess the Change in Distribution and Extent of an OSPAR Priority Habitat Under an Increased Ocean Temperature Scenario: Consequences for Marine Protected Area Networks and Management. PloS One 8, e68263. doi: 10.1371/journal.pone.0068263
Gratton P., Marta S., Bocksberger G., Winter M., Trucchi E., Kühl H. (2017). A World of Sequences: Can We Use Georeferenced Nucleotide Databases for a Robust Automated Phylogeography? J. Biogeogr. 44, 475–486. doi: 10.1111/jbi.12786
Guijarro J., Beazley L., Lirette C., Kenchington E., Wareham V., Gilkinson K., et al. (2016). Species Distribution Modelling of Corals and Sponges From Research Vessel Survey Data in the Newfoundland and Labrador Region for Use in the Identification of Significant Benthic Areas. Canadian Technical Report of Fisheries and Aquatic Sciences 3171, (Nova Scotia: Fisheries and Oceans Canada).
Halpern B. S., Longo C., Hardy D., McLeod K. L., Samhouri J. F., Katona S. K., et al. (2013). An Index to Assess the Health and Benefits of the Global Ocean. Nature 488, 615–620. doi: 10.1038/nature11397
Hellberg M. E. (2007). Footprints on Water: The Genetic Wake of Dispersal Among Reefs. Coral Reefs 26, 463–473. doi: 10.1007/s00338-007-0205-2
Hijmans R. J., Spooner D. M. (2001). Geographic Distribution of Wild Potato Species. Am. J. Bot. 88, 2101–2112. doi: 10.2307/3558435
Hoban S., Bruford M., D’Urban Jackson J., Lopes-Fernandes M., Heuertz M., Hohenlohe P. A., et al. (2020). Genetic Diversity Targets and Indicators in the CBD Post-2020 Global Biodiversity Framework Must be Improved. Biol. Conserv. 248, 108654. doi: 10.1016/j.biocon.2020.108654
Hughes A. C., Orr M. C., Ma K., Costello M. J., Waller J., Provoost P., et al. (2021). Sampling Biases Shape Our View of the Natural World. Ecography 4, 1259–1269. doi: 10.1111/ecog.05926
Iguchi A., Yoshioka Y., Forsman Z. H., Knapp I. S. S., Toonen R. J., Hongo Y., et al. (2019). RADseq Population Genomics Confirms Divergence Across Closely Related Species in Blue Coral (Heliopora Coerulea). BMC Evol. Biol. 19, 187. doi: 10.1186/s12862-019-1522-0
Jin Y. K., Lundgren P., Lutz A., Raina J. B., Howells E. J., Paley A. S., et al. (2016). Genetic Markers for Antioxidant Capacity in a Reef-Building Coral. Sci. Adv. 2, 1500842. doi: 10.1126/sciadv.1500842
Kai Y., Motomura H., Matsuura K. (2022). Fish Diversity of Japan:Evolution, Zoogeography, and Conservation. Eds. Kai Y., Motomura H., Matsuura K. (Singapore: Springer Singapore). doi: 10.1007/978-981-16-7427-3
Kenyon J. C. (1997). Models of Reticulate Evolution in the Coral Genus Acropora Based on Chromosome Numbers: Parallels With Plants. Evol. (N. Y) 51, 756–767. doi: 10.2307/2411152
Kitano Y. F., Hongo C., Yara Y., Sugihara K., Kumagai N. H., Yamano H. (2020). Data on Coral Species Occurrences in Japan Since 1929. Ecol. Res. 35, 975–985. doi: 10.1111/1440-1703.12136
Kitano Y. F., Nagai S., Ueno M., Yasuda N. (2015). Most Pocillopora Damicornis Around Yaeyama Islands are Pocillopora Acuta According to Mitochondrial ORF Sequences. Galaxea J. Coral Reef Stud. 17, 21–22. doi: 10.3755/galaxea.17.21
Krück N. C., Tibbetts I. R., Ward R. D., Johnson J. W., Loh W. K. W., Ovenden J. R. (2013). Multi-Gene Barcoding to Discriminate Sibling Species Within a Morphologically Difficult Fish Genus (Sillago). Fish. Res. 143, 39–46. doi: 10.1016/j.fishres.2013.01.007
Kumagai J., Wakamatsu M., Hashimoto S., Saito O., Yoshida T., Yamakita T., et al. (2022). Natural Capitals for Nature’s Contributions to People: The Case of Japan. Sustain. Sci 17, 919–954. doi: 10.1007/s11625-020-00891-x
Laikre L., Hoban S., Bruford M. W., Segelbacher G., Allendorf F. W., Gajardo G., et al. (2020). Post-2020 Goals Overlook Genetic Diversity. Sci. (80-. ) 367, 1083–1085. doi: 10.1126/science.abb2748
Lasker H. R., Coffroth M. A. (1999). Responses of Clonal Reef Taxa to Environmental Change. Am. Zool. 39, 92–103. doi: 10.1093/icb/39.1.92
Le Goff-Vitry M. C., Rogers A. D. (2005). “Molecular Ecology of Lophelia Pertusa in the NE Atlantic,” in Cold-Water Corals and Ecosystems (Berlin: Springer), 653–662. doi: 10.1007/3-540-27673-4_32
Magalon H., Faure B., Bigot L., Guillaume M., Bruggemann H. (2011). “Connectivity of the Coral Pocillopora Meandrina in the Eparses and Réunion Islands,” in 7th Western Indian Ocean Marine Science Association Symposium, Oct 2011 Kenya. (Accession No. hal-00852811)
Mayer P. M. R., McCutchen S. K., Canfield M. D., Timothy J. (2007). Meta-Analysis of Nitrogen Removal in Riparian Buffers. J. Environ. Qual. 36, 1172. doi: 10.2134/jeq2006.0462
Miller M. W., Halley R. B., Gleason A. C. R. (2008). “Reef Geology and Biology of Navassa Island,” in Coral Reefs of the USA (Dordrecht: Springer), 407–433. doi: 10.1007/978-1-4020-6847-8_10
Ministry of the Environment Japan (2009). Environmental Characteristics of Red List Corals (Species), (Tokyo: Ministry of the Environment Japan).
Miya M., Gotoh R. O., Sado T. (2020). MiFish Metabarcoding: A High-Throughput Approach for Simultaneous Detection of Multiple Fish Species From Environmental DNA and Other Samples. Fish. Sci. 86, 939–970. doi: 10.1007/s12562-020-01461-x
Morrison C. L., Ross S. W., Nizinski M. S., Brooke S., Järnegren J., Waller R. G., et al. (2011). Genetic Discontinuity Among Regional Populations of Lophelia Pertusa in the North Atlantic Ocean. Conserv. Genet. 12, 713–729. doi: 10.1007/s10592-010-0178-5
Muko S., Kawasaki K., Sakai K., Takasu F., Shigesada N. (2000). Morphological Plasticity in the Coral Porites Sillimaniani and its Adaptive Significance. Bull. Mar. Sci. 66, 225–239.
Muller-Karger F. E., Miloslavich P., Bax N. J., Simmons S., Costello M. J., Sousa Pinto I., et al. (2018). Advancing Marine Biological Observations and Data Requirements of the Complementary Essential Ocean Variables (EOVs) and Essential Biodiversity Variables (EBVs) Frameworks. Front. Mar. Sci. 5. doi: 10.3389/fmars.2018.00211
Nakabayashi A., Yamakita T., Nakamura T., Aizawa H., Kitano Y. F., Iguchi A., et al. (2019). The Potential Role of Temperate Japanese Regions as Refugia for the Coral Acropora Hyacinthus in the Face of Climate Change. Sci. Rep. 9, 1892. doi: 10.1038/s41598-018-38333-5
Nature Conservation Bureau Environment Agency and Asia Air Survey Co. Ltd (1994). Coastal Survey Report, the 4th National Survey on the Natural Environment, Tokyo: Environment Agency.
Nature Conservation Bureau Environment Agency and Marine Parks Center of Japan (1994). The Report of the Marine Biotic Environment Survey in the 4th National Survey on the Natural Environment (Coral Reefs) Vol. 3 (Tokyo: Environment Agency).
Nature Conservation Bureau Ministry of the Environment Government of Japan (2014) Ecologically or Biologically Significant Marine Area (EBSAs) Identified by Japan. Available at: http://www.env.go.jp/en/nature/biodic/kaiyo-hozen/kaiiki/index.html. [Accessed April 10, 2022]
Parsons K. E. (1996). Discordant Patterns of Morphological and Genetic Divergence in the “Austrocochlea Constricta” (Gastropoda:Trochidae) Species Complex. Mar. Freshw. Res. 47, 981–990. doi: 10.1071/MF9960981
Pipithkul S., Ishizu S., Shimura A., Yokochi H., Nagai S., Fukami H., et al. (2021). High Clonality and Geographically Separated Cryptic Lineages in the Threatened Temperate Coral, Acropora pruinosa. Front. Mar. Sci. doi: 10.3389/fmars.2021.668043
Richards Z. T., Hobbs J. P. A. (2015). Hybridisation on Coral Reefs and the Conservation of Evolutionary Novelty. Curr. Zool. 61, 132–145. doi: 10.1093/czoolo/61.1.132
Roberts J. J., Best B. D., Dunn D. C., Treml E. A., Halpin P. N. (2010). Marine Geospatial Ecology Tools: An Integrated Framework for Ecological Geoprocessing With ArcGIS, Python, R, MATLAB, and C++. Environ. Model. Software 25, 1197–1207. doi: 10.1016/j.envsoft.2010.03.029
Ross R. E., Nimmo-Smith W. A. M., Howell K. L. (2017). Towards ‘Ecological Coherence’: Assessing Larval Dispersal Within a Network of Existing Marine Protected Areas. Deep. Res. Part I Oceanogr. Res. Pap. 126, 128–138. doi: 10.1016/j.dsr.2017.06.004
Sakai Y., Kadota T., Shimizu N., Tsuboi M., Yamaguchi S., Nakaguchi K., et al. (2009). Fish Fauna on Reefs of Tokara Islands, Southern Japan, Surveyed By Underwater Census During 2002-2007. J. Grad. Sch. Biosph. Sci. Hiroshima Univ. 48, 19–35. doi: 10.15027/39872
Shearer T. L., Coffroth M. A. (2008). Barcoding Corals: Limited by Interspecific Divergence, Not Intraspecific Variation. Mol. Ecol. Resour. 8, 247–255. doi: 10.1111/j.1471-8286.2007.01996.x
Shirayama Y. (2016) Project Final Reports:S-9-5 Quantitative Assessment and Future Projection of Biodiversity Loss in Marine Ecosystems. Available at: https://www.env.go.jp/policy/kenkyu/suishin/kadai/kadai_ichiran/completed_projects.html.
Sudo K., Nakaoka M. (2020). Fine-Scale Distribution of Tropical Seagrass Beds in Southeast Asia. Ecol. Res. 35, 994–1000. doi: 10.1111/1440-1703.12137
Sudo K., Quiros T. E. A. L., Prathep A., Luong C., Lin H. J., Bujang J. S., et al. (2021). Distribution, Temporal Change, and Conservation Status of Tropical Seagrass Beds in Southeast Asia: 2000–2020. Front. Mar. Sci. 8. doi: 10.3389/fmars.2021.637722
Sugihara K., Nomura K., Yokochi H., Shimoike K., Kajiwara K., Suzuki G., et al. (2015). Zooxanthellate Scleractinian Corals of Tanegashima Island, Japan, (Tsukuba: National Institute for Environmental Studies).
Suyama Y., Matsuki Y. (2015). MIG-Seq: An Effective PCR-Based Method for Genome-Wide Single-Nucleotide Polymorphism Genotyping Using the Next-Generation Sequencing Platform. Sci. Rep. 5, 16963. doi: 10.1038/srep16963
Takata K., Taninaka H., Nonaka M., Iwase F., Kikuchi T., Suyama Y., et al. (2019). Multiplexed ISSR Genotyping by Sequencing Distinguishes Two Precious Coral Species (Anthozoa: Octocorallia: Coralliidae) That Share a Mitochondrial Haplotype. PeerJ 2019, e7769. doi: 10.7717/peerj.7769
Takeuchi Y., Muraoka H., Yamakita T., Kano Y., Nagai S., Bunthang T., et al. (2021). The Asia-Pacific Biodiversity Observation Network: 10-Year Achievements and New Strategies to 2030. Ecol. Res. 36, 232–257. doi: 10.1111/1440-1703.12212
Todd P. A. (2008). Morphological Plasticity in Scleractinian Corals. Biol. Rev. 83, 315–337. doi: 10.1111/j.1469-185X.2008.00045.x
Treml E. A., Roberts J. J., Chao Y., Halpin P. N., Possingham H. P., Riginos C. (2012). “Reproductive Output and Duration of the Pelagic Larval Stage Determine Seascape-Wide Connectivity of Marine Populations,” in Integrative and Comparative Biology (Oxford: Oxford University Press), 525–537. doi: 10.1093/icb/ics101
Tsuji S., Maruyama A., Miya M., Ushio M., Sato H., Minamoto T., et al. (2020). Environmental DNA Analysis Shows High Potential as a Tool for Estimating Intraspecific Genetic Diversity in a Wild Fish Population. Mol. Ecol. Resour. 20, 1248–1258. doi: 10.1111/1755-0998.13165
Tyler J., Bonfitto M. T., Clucas G. V., Reddy S., Younger J. L. (2020). Morphometric and Genetic Evidence for Four Species of Gentoo Penguin. Ecol. Evol. 10, 13836–13846. doi: 10.1002/ece3.6973
Veron J. E. N., Wallace C. C. (1984). Scleractinia of Eastern Australia. Part V. Familiy Acroporidae. Monogr. Ser. Aust. Inst. Mar. Sci. 6, 1–422.
Webb T. J., vanden Berghe E., O’Dor R. (2010). Biodiversity’s Big Wet Secret: The Global Distribution of Marine Biological Records Reveals Chronic Under-Exploration of the Deep Pelagic Ocean. PloS One 5, e10223. doi: 10.1371/journal.pone.0010223
Willis B. L., Van Oppen M. J. H., Miller D. J., Vollmer S. V., Ayre D. J. (2006). The Role of Hybridization in the Evolution of Reef Corals. Annu. Rev. Ecol. Evol. Syst. 37, 489–517. doi: 10.1146/annurev.ecolsys.37.091305.110136
Yamakita T. (2018). Change of the Ocean After the Great East Japan Earthquake: Utilization of Geographic Information System and GIScience. e-Research Species Biol. 2, 20181016. Available at: http://www.speciesbiology.org/eShuseibutsu/evol2/front.html [Accessed April 10, 2022]
Yamakita T., Nakaoka M., Yamano H., Nanami A., Ishikawa Y., Sudo K., et al. (2020). PANCES Policy Brief, No. 3 (Tsukuba: NIES). Available at: https://www.nies.go.jp/pances/en/policybrief. [Accessed April 10, 2022]
Yamakita T., Sodeyama F., Whanpetch N., Watanabe K., Nakaoka M. (2019). Application of Deep Learning Techniques for Determining the Spatial Extent and Classification of Seagrass Beds, Trang, Thailand. Bot. Mar. 62, 291–307. doi: 10.1515/bot-2018-0017
Yamakita T., Sudo K., Jintsu-Uchifune Y., Yamamoto H., Shirayama Y. (2017). Identification of Important Marine Areas Using Ecologically or Biologically Significant Areas (EBSAs) Criteria in the East to Southeast Asia Region and Comparison With Existing Registered Areas for the Purpose of Conservation. Mar. Policy 81, 273–284. doi: 10.1016/j.marpol.2017.03.040
Yamakita T., Yamamoto H., Nakaoka M., Yamano H., Fujikura K., Hidaka K., et al. (2015). Identification of Important Marine Areas Around the Japanese Archipelago: Establishment of a Protocol for Evaluating a Broad Area Using Ecologically and Biologically Significant Areas Selection Criteria. Mar. Policy 51, 136–147. doi: 10.1016/j.marpol.2014.07.009
Yasuda N., Shimura A., Nakabayashi A., Yamakita T., Nakamura T., Aizawa H., et al. (2019). Does Temperate Japanese Region Provide Refugia for Reef-Building Corals in Face of Climate Change? DNA Polymorph. 27, 37–42.
Yasuda N., Taquet C., Nagai S., Fortes M., Fan T.-Y., Phongsuwan N., et al. (2014). Genetic Structure and Cryptic Speciation in the Threatened Reef-Building Coral Heliopora Coerulea Along Kuroshio Current. Bull. Mar. Sci. 90, 233–255. doi: 10.5343/bms.2012.1105
Keywords: marine protected area (MPA), phylogeny, coral, spatial planning, Aichi 2020 target, biogeography, macro ecology
Citation: Yamakita T, Sodeyama F, Iguchi A, Kitano YF, Teshima KM, Shimura A, Nakabayashi A, Nagai S, Nakamura T, Aizawa H and Yasuda N (2022) Consideration of Genetic Structure in the Ecologically or Biologically Significant Marine Areas Criteria: A Review of Convention on Biological Diversity Regional Workshops and A Case Study of Coral Reef Conservation Planning. Front. Mar. Sci. 9:823009. doi: 10.3389/fmars.2022.823009
Received: 26 November 2021; Accepted: 04 April 2022;
Published: 27 May 2022.
Edited by:
Andrew M Fischer, University of Tasmania, AustraliaReviewed by:
Ana Carolina de Azevedo Mazzuco, Federal University of Espirito Santo, BrazilCong Zeng, Shanghai Jiao Tong University, China
Copyright © 2022 Yamakita, Sodeyama, Iguchi, Kitano, Teshima, Shimura, Nakabayashi, Nagai, Nakamura, Aizawa and Yasuda. This is an open-access article distributed under the terms of the Creative Commons Attribution License (CC BY). The use, distribution or reproduction in other forums is permitted, provided the original author(s) and the copyright owner(s) are credited and that the original publication in this journal is cited, in accordance with accepted academic practice. No use, distribution or reproduction is permitted which does not comply with these terms.
*Correspondence: Takehisa Yamakita, yamakitat@jamstec.go.jp