- 1Oceanic Fisheries Programme, Fisheries Aquaculture and Marine Ecosystems Division, Pacific Community, Noumea, New Caledonia
- 2Centre for Conservation Ecology and Genomics, Institute for Applied Ecology, University of Canberra, Bruce, ACT, Australia
- 3Evaluation of Analyses and Forecasts Department, Mercator-Ocean International, Toulouse, France
- 4Marine Ecosystems Modeling and Monitoring by Satellites, Satellite Oceanography Division, Collecte Localisation Satellites, Ramonville, France
- 5Department of Agriculture, Water and the Environment, Australian Bureau of Agricultural and Resource Economics and Sciences, Canberra, ACT, Australia
- 6Faculty of Land and Food Systems, University of British Columbia, Vancouver, BC, Canada
- 7Department of Marine Sciences, Tjärnö Marine Laboratory, University of Gothenburg, Strömstad, Sweden
- 8Early Life History Group, Inter-American Tropical Tuna Commission, La Jolla, CA, United States
- 9ARC Centre of Excellence for Coral Reef Studies, James Cook University, Townsville, QLD, Australia
- 10School of Natural Sciences, Macquarie University, North Ryde, NSW, Australia
- 11Director’s Office, Fisheries Aquaculture and Marine Ecosystems Division, Pacific Community, Noumea, New Caledonia
The impacts of climate change are expected to have profound effects on the fisheries of the Pacific Ocean, including its tuna fisheries, the largest globally. This study examined the combined effects of climate change on the yellowfin tuna population using the ecosystem model SEAPODYM. Yellowfin tuna fisheries in the Pacific contribute significantly to the economies and food security of Pacific Island Countries and Territories and Oceania. We use an ensemble of earth climate models to project yellowfin populations under a high greenhouse gas emissions (IPCC RCP8.5) scenario, which includes, the combined effects of a warming ocean, increasing acidification and changing ocean chemistry. Our results suggest that the acidification impact will be smaller in comparison to the ocean warming impact, even in the most extreme ensemble member scenario explored, but will have additional influences on yellowfin tuna population dynamics. An eastward shift in the distribution of yellowfin tuna was observed in the projections in the model ensemble in the absence of explicitly accounting for changes in acidification. The extent of this shift did not substantially differ when the three-acidification induced larval mortality scenarios were included in the ensemble; however, acidification was projected to weaken the magnitude of the increase in abundance in the eastern Pacific. Together with intensive fishing, these potential changes are likely to challenge the global fishing industry as well as the economies and food systems of many small Pacific Island Countries and Territories. The modelling framework applied in this study provides a tool for evaluating such effects and informing policy development.
INTRODUCTION
Ocean ecosystems are some of the largest wilderness areas remaining on Earth. The Pacific Ocean, for example, at approximately 168 million square kilometres, is the planet’s largest single feature, covering one third of the Earth’s surface. In addition to their conservation value, pelagic fishes from these ecosystems support fisheries that account for a substantial portion of all-ocean seafood harvests (FAO, 2020). The gradual warming and acidification of the Earth’s oceans (e.g., Caldeira and Wickett, 2003; Feely et al., 2004; Barnett et al., 2005; Iida et al., 2021) due to anthropogenic carbon dioxide (CO2) emissions has the potential to affect the distribution and population dynamics of many marine organisms (Fabry et al., 2008; Doney et al., 2009; Kroeker et al., 2013; Poloczanska et al., 2013) including pelagic species. Moreover, the speed of change in marine ecosystems due to CO2 emissions is projected to be rapid (Pörtner et al., 2014; Pecl et al., 2017), potentially outpacing our ability to understand the complex climatic processes at play. Our knowledge is particularly limited for the ocean pelagic ecosystem, which represents a significant challenge to fishery resource managers and policymakers, who need to account for climate change (including ocean acidification) in addition to the impacts of fishing pressure on fish stocks and marine ecosystems (Rice and Garcia, 2011; Hobday et al., 2013; Salinger, 2013; Salinger et al., 2013).
Increased atmospheric CO2 is expected to alter global atmospheric temperatures with follow-on effects resulting in changes to ocean water temperatures, currents, ocean productivity and dissolved oxygen (Pörtner et al., 2019). Each of these variables influences the population dynamics of pelagic species (Lehodey et al., 2010a; Lehodey et al., 2010b; Lehodey et al., 2011; Dragon et al., 2015; Lehodey et al., 2015a; Dragon et al., 2017; Senina et al., 2020a). Increased CO2 absorption in our oceans shifts the carbonate chemical equilibrium of seawater towards a lower pH and lower calcium carbonate saturation states (Doney et al., 2009). These chemical changes are expected to impact many calcifying species, e.g., shell-forming marine organisms (Orr et al., 2005; Fabry et al., 2008; Doney et al., 2009; Gattuso and Hansson, 2011; Doney et al., 2020) and can also affect fishes through effects on acid-base regulation, internal calcification processes and respiration (Heuer et al., 2016; Stiasny et al., 2016; Grosell et al., 2019; Laubenstein et al., 2019; McMahon et al., 2020).
Global catches from near-shore and oceanic pelagic fisheries reached 9.5 million tonnes in the 2000s (Juan-Jorda et al., 2011) and provide a multitude of food, health and economic benefits to coastal and fishing nations. In the western and central Pacific Ocean, fish are necessary to meet the food security needs of island populations, and its tuna fisheries provide substantial income for most island economies (Gillet, 2009; Gillet and Cartwright, 2010; Bell et al., 2015; Bell et al., 2021). Beyond traditional food security and economic perspectives, tuna fisheries are a key component of the entire food system of Oceania, with cultural, societal and public health values and benefits (Bell et al., 2015; Pauwels and Fache, 2016). While several studies have predicted how ocean warming will affect Pacific tuna populations (Lehodey et al., 2013; Lehodey et al., 2015a; Dueri et al., 2016), there are only two studies that have examined how ocean acidification may affect these species and associated fisheries (Bromhead et al., 2015; Frommel et al., 2016). Despite substantial knowledge gaps on the expected impacts on tunas, fishery resource managers and policymakers will be increasingly challenged by the need to develop responses that mitigate the negative effects of a changing climate within the context of considerable uncertainty about ecosystem processes. Here, we use the SEAPODYM framework to parameterise an ensemble of models to summarise the population dynamics of yellowfin tuna, and to simulate the combined effects of ocean warming and acidification on yellowfin tuna distribution and abundance. SEAPODYM is a spatially explicit ecosystem dynamics model that integrates environmental, biological and fisheries data (Lehodey et al., 2008) to estimate population dynamics parameters (Senina et al., 2008; Senina et al., 2020b), and offers a framework for evaluating the combined effects of ocean warming and acidification on tuna populations in the Pacific Ocean.
METHODS
The modelling approach of SEAPODYM (see Note 1: Supplementary Material) has been extensively described (e.g., Lehodey et al., 2003; Lehodey et al., 2008; Senina et al., 2008; Senina et al., 2020b; Senina et al., 2022). The SEAPODYM model equations describe population dynamic processes (spawning, recruitment, movement, mortality) based on functional relationships with temperature, dissolved oxygen concentration and distributions of prey (micronektonic tuna forage). The model simulates age-structured population dynamics distinguishing between four life stages: larval, small juveniles of few months of age, young immature, and mature adult stages. At larval and juvenile phases, the biomass of fish is advected by currents and dispersed both with water masses and their random motions. When they become more autonomous at older ages, in addition to passive transport, their movement has active advective and diffusive components linked to their size and the habitat quality. The model accounts for fishing and predicts total catch and size frequencies of catch by fishery, the observed quantities of which are used to condition the parameterization and evaluate model fit. The spatial dynamics, relying on advection-diffusion-reaction equations, are fully explicit, meaning that biomass density of fish population and catch are computed in each cell of a grid defined for the model domain. The Maximum Likelihood Estimation approach developed for SEAPODYM (Senina et al., 2008; Senina et al., 2020b) takes advantage of this spatially explicit representation by integrating the numerous catch/effort, length frequencies of catch, and tagging data available (see Supplementary Material Table S1). In this work, the available tagging data were used only for the estimation of habitat parameters.
Integrating Ocean Acidification
Natural mortality is computed in SEAPODYM as a sum of functions describing the rates of population decay due to processes associated with predation and senescence with an added effect of environmental variability expressed through a habitat index to allow mortality at age to be variable in space and time (Senina et al., 2020b). The habitat index describes suitability of habitat for spawning success and larval survival as a function of sea-surface temperatures, primary production (a proxy for prey of larvae) and epipelagic micronekton that are larval predators (Senina et al., 2020b). The impact of acidification was incorporated by introducing an additional variable term to the larval (0-1 month of age) mortality rate, computed from the functional relationship describing the increase of larval mortality with increasing acidity (Figure 1). The spatial resolution of SEAPODYM allows the spatial and temporal variability of acidification in the Pacific Ocean (e.g. McNeil and Sasse, 2016; Figure 2) to be explicitly included rather than simply an average effect.
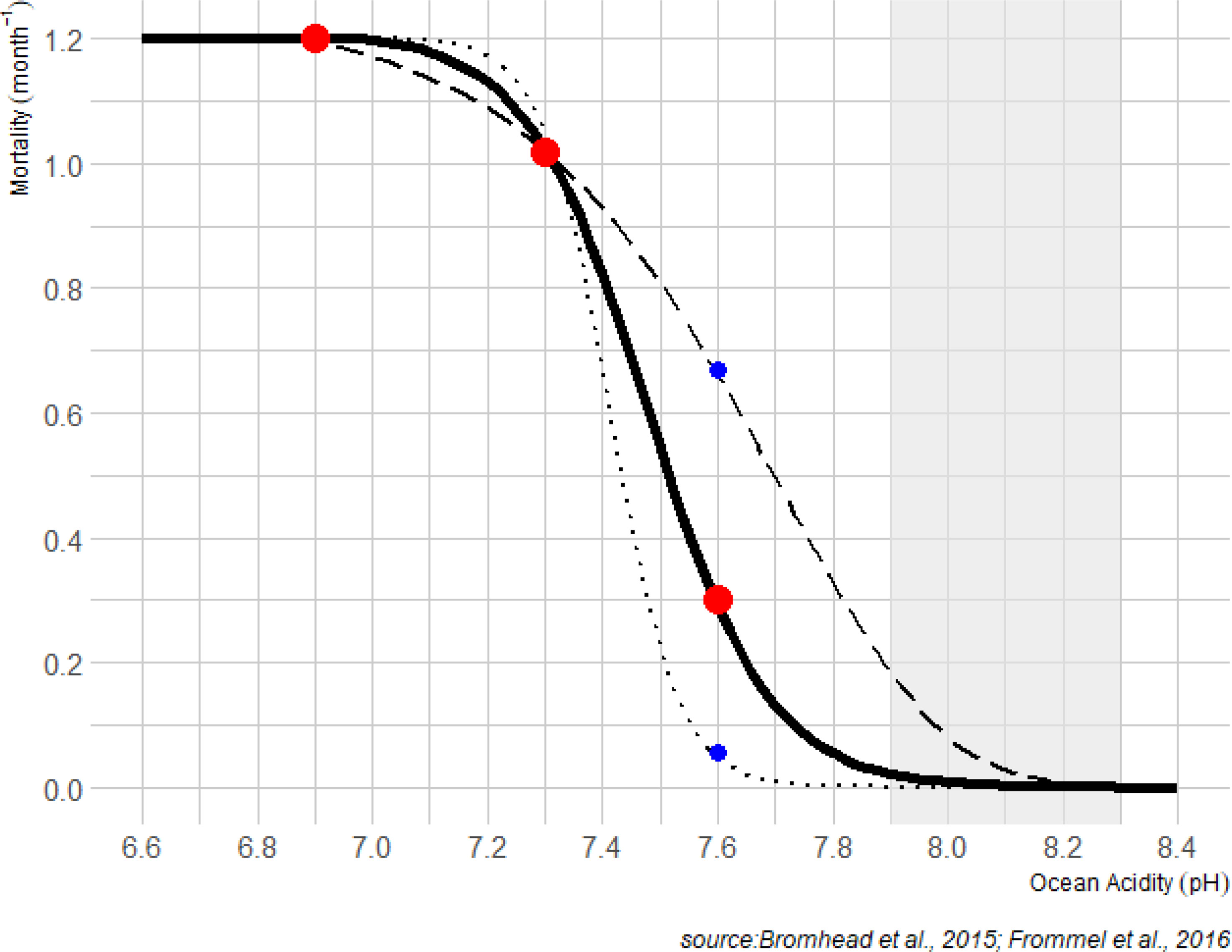
Figure 1 Change in additional monthly mortality rates of yellowfin larvae deduced from experimental results (Bromhead et al., 2015; Frommel et al., 2016) corresponding to 70%, 64% and 27% (red points) decrease in larvae survival during first 7 days of life at pH value of 6.9, 7.3, 7.6 respectively. The solid line shows the analytical function implemented in SEAPODYM to account the impact of acidification with these three mean values (scenario SOA1). Two other curves (dashed, SOA2 and dotted, SOA3) are high and low sensitivity scenarios to account uncertainty at higher levels of pH. The shaded area corresponds to the historical pH values estimated in the epipelagic layer of the ensemble models (see Figure 2).
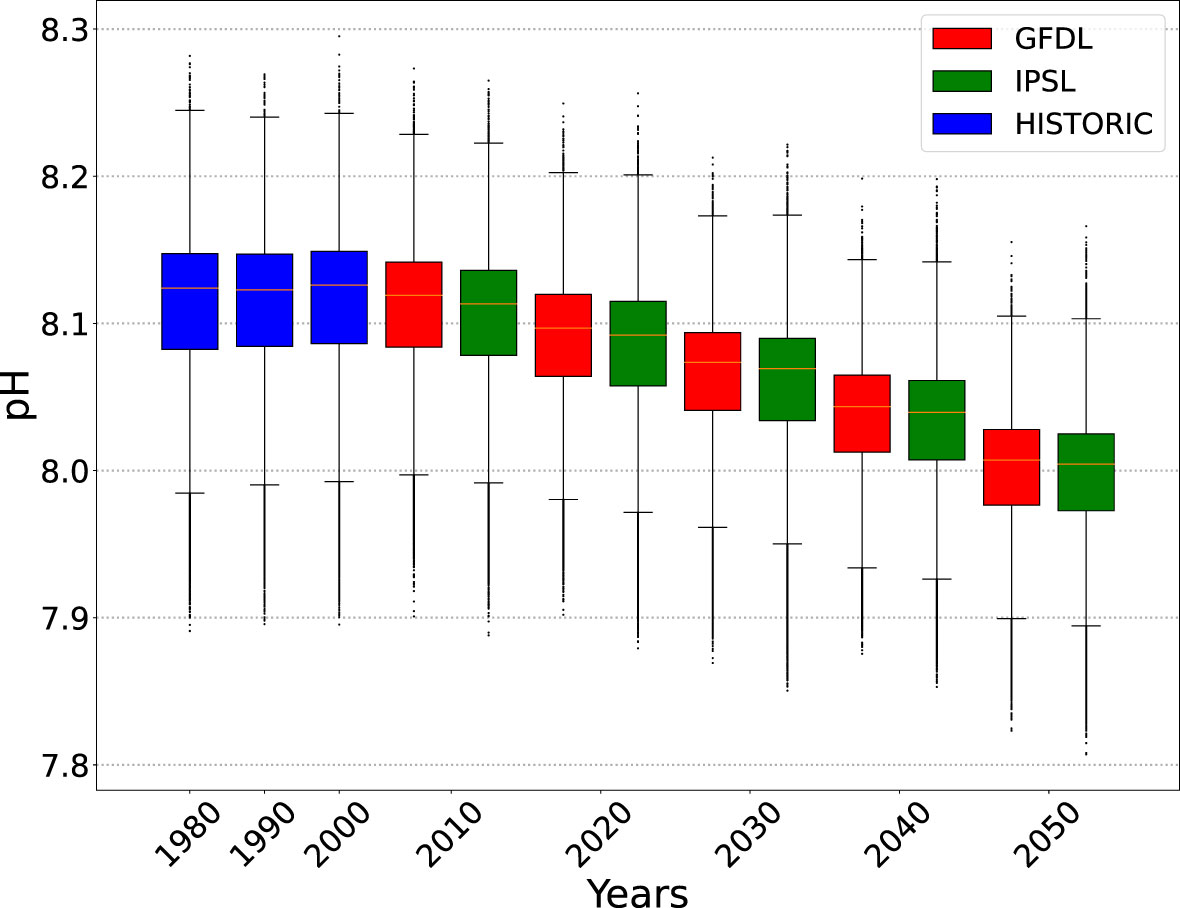
Figure 2 Boxplots on the distribution of pH in the epipelagic layer where yellowfin tuna larvae are predicted to occur (density threshold is set to 0.1). Data aggregated by decade (1981-1990; 1991-2000; etc). Historical values of pH (HISTORIC) are from the ERA40 INTERIM renanalysis forcings. Future values of pH are from the GFDL and IPSL CMIP6 earth climate models used in the ensemble (see Table 1).
The parameterization of the response to pH was based on the experimental study described in Bromhead et al. (2015) and Frommel et al. (2016). Lower pH was found to significantly reduce larval survival by 64% and 70% (pH 7.3 and 6.9 respectively) during the first 7 days compared with ambient controls (pH 8.1) (Frommel et al., 2016). For the less extreme pH reduction tested (pH=7.6) the mean reduction in survival was 27%, although this result was not statistically significant. A logistic function (Figure 1) was fitted to the estimates of Frommel et al. (2016) assuming maximum mortality (due to ocean acidification) at a pH of 6.9 and zero mortality (due to ocean acidification) at a pH =8.1. The other mean estimates from Frommel et al. (2016) at pH values of 7.3 and 7.6 were used to shape the analytical function. To test how sensitive results may be to this analytical function, the logistic curve was also shaped using the highest and lowest mortality values estimated for pH=7.6 in Frommel et al. (2016) (Figure 1).
The early life stages of fishes can be particularly vulnerable to ocean acidification, due to a small body size and high surface to volume ratio, as well as immature acid-base regulatory organs (Brauner et al., 2019). To convert our analytical function describing acidification induced mortality to a monthly mortality coefficient for application in SEAPODYM, we assumed that there was no additional mortality due to ocean acidification after 7 days (i.e. when the tuna have completed organogenesis and are able to effectively regulate acid-base balance). This was derived from the observed reduction in survival rate due to pH conditions. For instance, a 70% decrease in larvae survival at pH 6.9 results in an additional monthly mortality coefficient of 1.2 (Figure 1). The simulated acidification effect did not consider impacts on lower trophic orders within the SEAPODYM framework.
Simulating the Combined Effects of Ocean Warming and Acidification
A 2° regular grid at monthly time step was used to solve numerically the optimal solution of SEAPODYM. The age structure of yellowfin was discretized between 0 and agemax = 10 (yr) into monthly cohorts for the first five years and a last cohort aggregating oldest individuals. Age-length and age-weight relationships were derived from the estimates reported in Langley et al. (2011). The species was assumed to be an opportunistic spawner with a local spawning success proportional to the spawning habitat index and the larvae stock-recruitment (Beverton and Holt) function computed at the cell level. The initial conditions at the beginning of the simulation (1979) were obtained using the previous reference configuration for yellowfin tuna (Senina et al., 2015).
Parameter Optimization
The SEAPODYM model parameters were estimated for yellowfin tuna for the historical period 1979-2010. Environmental forcings (water temperature and currents, dissolved oxygen concentrations, total vertically-integrated primary production and euphotic depths) were provided by the ocean model NEMO (www.nemo-ocean.eu/) coupled to the biogeochemical model PISCES (Pelagic Interaction Scheme for Carbon and Ecosystem Studies; Aumont et al., 2015). Ocean Acidification (pH) was not included in the environmental forcings for the optimization. The ocean physical model was driven by the ERA40-INTERIM atmospheric reanalysis (atmospheric temperature, zonal and meridional wind speeds, radiative heat fluxes, relative humidity, and precipitation) which has been corrected using satellite data (Dee et al., 2011). All forcing variables are interpolated on the same regular grid and time (Aumont et al., 2015). The coupled NEMO-PISCES model outputs were first used to simulate the micronekton functional groups (Lehodey et al., 2010a; Lehodey et al., 2015b). SEAPODYM forcing fields were then complete and model parameters were estimated by fitting model predictions to fisheries catch, CPUE and length frequency data (Supplementary Material Table S1) using a maximum likelihood estimation approach (Senina et al., 2008; Senina et al., 2020b).
Once the optimal parameterization was achieved and validated by statistical fits to the data, one more simulation was produced without fisheries data. In this analysis without fishing mortality, the stocks rapidly increased and reached an equilibrium state in a time that is defined by the life span and the estimated stock-recruitment relationship. We assume that at the end of the 30-year reanalysis, the population was at its virgin (unfished) state and influenced by environmental variability only. The population state in December 2010, the last time step of the reanalysis, was saved to serve as the initial condition for the projections starting in 2011. The simulation ensembles were then produced without fishing and thus highlight the impact of climate change only.
Projections of Ocean Futures
Previous studies have shown that each earth climate model has its own biases and errors that can propagate in the projections of tuna populations (e.g., Lehodey et al., 2013). To overcome these biases a projection ensemble approach to simulations in model forcings was applied to account for the uncertainty (Bell et al., 2021; Receveur et al., 2021). The ensemble included four different earth climate models (Table 1) obtained from the Coupled Model Inter-comparison Project Phase 5 (CMIP5). The models selected were those that captured cycles similar to El Niño Southern Oscillation (ENSO) in their simulations (Bellenger et al., 2014). The scenario used was the RCP8.5 (business as usual) as defined by IPCC for the 5th assessment exercise.
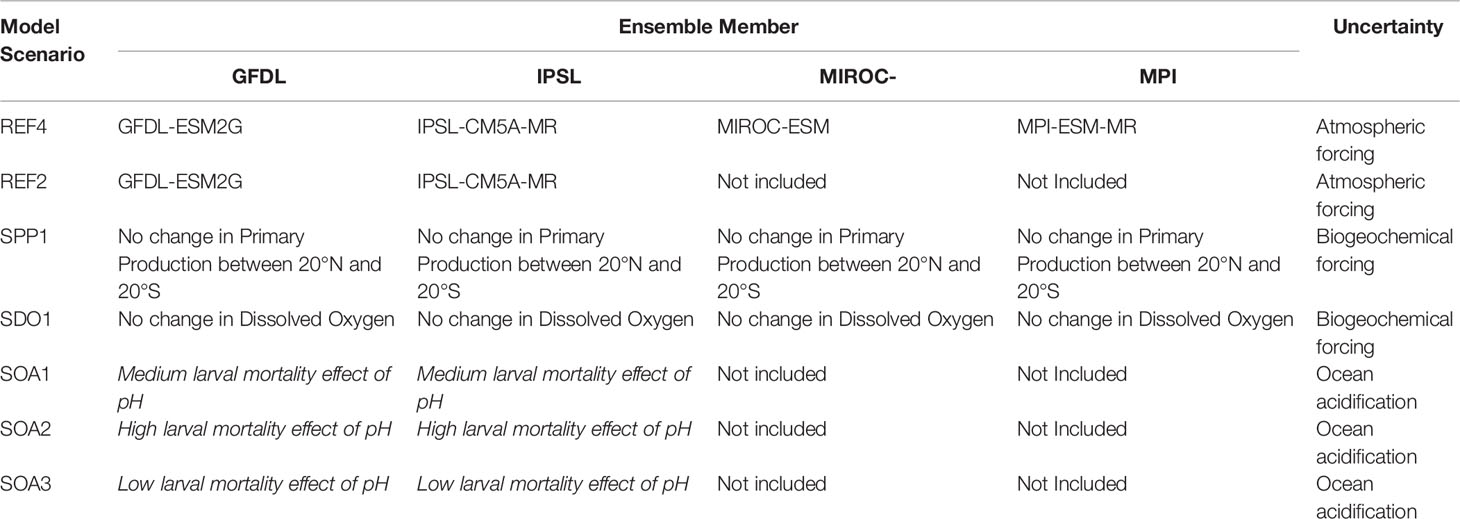
Table 1 Description of the ensemble of 18 simulations used in this study. The last column provides a descriptor of the model uncertainty considered in each scenario.
We applied the ensemble model described in Bell et al. (2021) and Receveur et al. (2021) to project ocean futures. Briefly, each earth climate model was coupled to NEMO-PISCES to generate SEAPODYM environmental forcings for the period 2011-2100. The low frequency trends from the climate model atmospheric variables were extracted and smoothed with a 31-year wide Hann filter. They were then added to three successive cycles of 30 years of the atmospheric INTERIM reanalysis variables prior to coupling with NEMO-PISCES. The results were 90-year atmospheric forcing sets that span the period 2011-2100 and mix the past observed variability with long-term trends due to climate change. A control simulation (three successive repetitions of the reanalysis) with the coupled physical-biogeochemical model was produced to verify that there was no long-term trend generated that was not associated with the climate change forcing. This approach has the advantage of applying one single and best optimal solution over the historical period with all future projections consistent with the historical period since they share the same mean state. A simulation of this ensemble with the effect of ocean warming alone defined our reference (REF4) for comparing scenarios that included either the additional effects of altered primary productivity or availability of dissolved oxygen.
Three scenarios were tested using the functional relationship between pH and larval mortality described in Figure 1: SOA1 was the intermediate scenario based on the three mean values of mortality observed in Frommel et al. (2016); SOA2 was a high mortality scenario; and SOA3 was a low mortality scenario as a function of pH as in Figure 1. A climatological distribution for pH was only available for both the MROC and MPI forcings and they were not included in the ensemble for these ocean acidification scenarios. As such, the ensemble for projected changes in ocean acidification only included the GFDL and IPSL environmental forcings. Both scenarios show median pH level of 8.13 for larval habitat during the model optimization period, with decreasing trends from 2010 (Figure 2). The pH values used were the mean values for the epipelagic layer (in time and space). As the ensemble was reduced to two environmental forcing for these scenarios examining the additional effects of ocean acidification the REF4 ensemble was also revised to only include the GFDL and IPSL forcings (REF2) for the purpose of comparing changes due to acidification. Note in the biogeochemical models used, pH was computed using a relationship to the CO2 concentration without any interaction with primary production. Hence any feedback between changing pH and primary production was not included in the projections.
Population dynamics of tropical tunas have been shown to be sensitive to the projected decrease in primary production in the western equatorial Pacific, and to declines in dissolved oxygen concentration in the eastern equatorial Pacific (Lehodey et al., 2013; Lehodey et al., 2015a). Long-term trends projected with coarse resolution, coupled physical-biogeochemical models are uncertain for these two variables. Warming induced increases in phytoplankton growth, intensification of the microbial loop, or stronger mixing that is not well represented in climate models using coarse resolution, can counter-balance classical expected nutrient limitation due to increased stratification at low latitude (Laufkötter et al., 2015; Matear et al., 2015). For example, Matear et al. (2015) showed that an enhanced-resolution model projected enhanced vertical shear mixing, increased vertical supply of nutrients to the photic zone, and increased sub-surface phytoplankton concentrations which resulted in an increase in sub-surface phytoplankton concentrations that offset the decline in surface phytoplankton concentrations and results in a projection of almost no change in the western tropical Pacific primary productivity. In contrast, the low-resolution model projected in Matear et al. (2015) showed a substantial reduction in phytoplankton concentrations and primary productivity. To account for this uncertainty, one additional member in the simulation ensemble (SPP1) includes a gradual increase in primary production in warm waters of the tropics, defined by SST > 27°C, to a level approximating the historical average level (i.e. pre-2010).
Decreasing trends in dissolved oxygen concentration have also been detected from historical datasets (Schmidtko et al., 2017), although their origin (observation errors, natural variability or climate change) is not yet clear. To evaluate uncertainty to dissolved oxygen, an additional member of the ensemble (SDO1) used climatological distribution of dissolved oxygen concentration instead of model projection.
Taken together, these different simulations produce an ensemble of 18 members (Table 1). Impact of these futures was analyzed by extracting the mean and standard errors of biomass of yellowfin tuna for the adults and recruits from each of the simulation ensembles. The yellowfin tuna population is simulated in the absence of fishing because (i) it is difficult to predict how the distribution of fishing effort would respond to distributional changes in the population; and (ii) estimated unexploited population trends would more clearly isolate the impacts of climate change, including ocean acidification effects. We examined the effect of these climate change impacts by comparing changes in biomass between those predicted in 2050 (calculated as the average between 2045-2055) and 2015 (calculated as the average between 2011-2020).
To quantify spatial changes in distribution of biomass, the Pacific was subdivided into five regions (North Pacific Ocean (NPO), Western Tropical Pacific Ocean (WTPO) and Central Tropical Pacific Ocean (CTPO), Eastern Tropical Pacific Ocean (ETPO) and South Pacific Ocean (SPO); see Figure S1 in Supplementary Material). When aggregating biomass to represent the Western and Central Pacific Ocean (WCPO) and Eastern Pacific Ocean (EPO) the Pacific was divided at the 150°W line of longitude.
RESULTS
Reference Simulation From Historical Hindcast (1980-2010)
The SEAPODYM solution for Pacific yellowfin tuna was achieved with the full catch-and-effort dataset being raised to the total nominal catch (see Table S2; Figures S2; S3; S4 in Supplementary Material). There was contrast in the final solution for larval distribution with areas of less dense concentrations in the central equatorial region, and higher densities in the eastern and western regions (Figure 3). The effect of fishing was predicted to have reduced the adult (spawning) biomass from unfished estimates in 2010 by 45% from 6.4 mt to 3.6 mt (Figure S5a in Supplementary Material). This fishing impact was highest in the WTPO and ETPO for both the immature (purse-seine caught) and mature (purse-seine and longline caught) components of the population and less prevalent in the CTPO (Figures S5b; S5c in Supplementary Material).
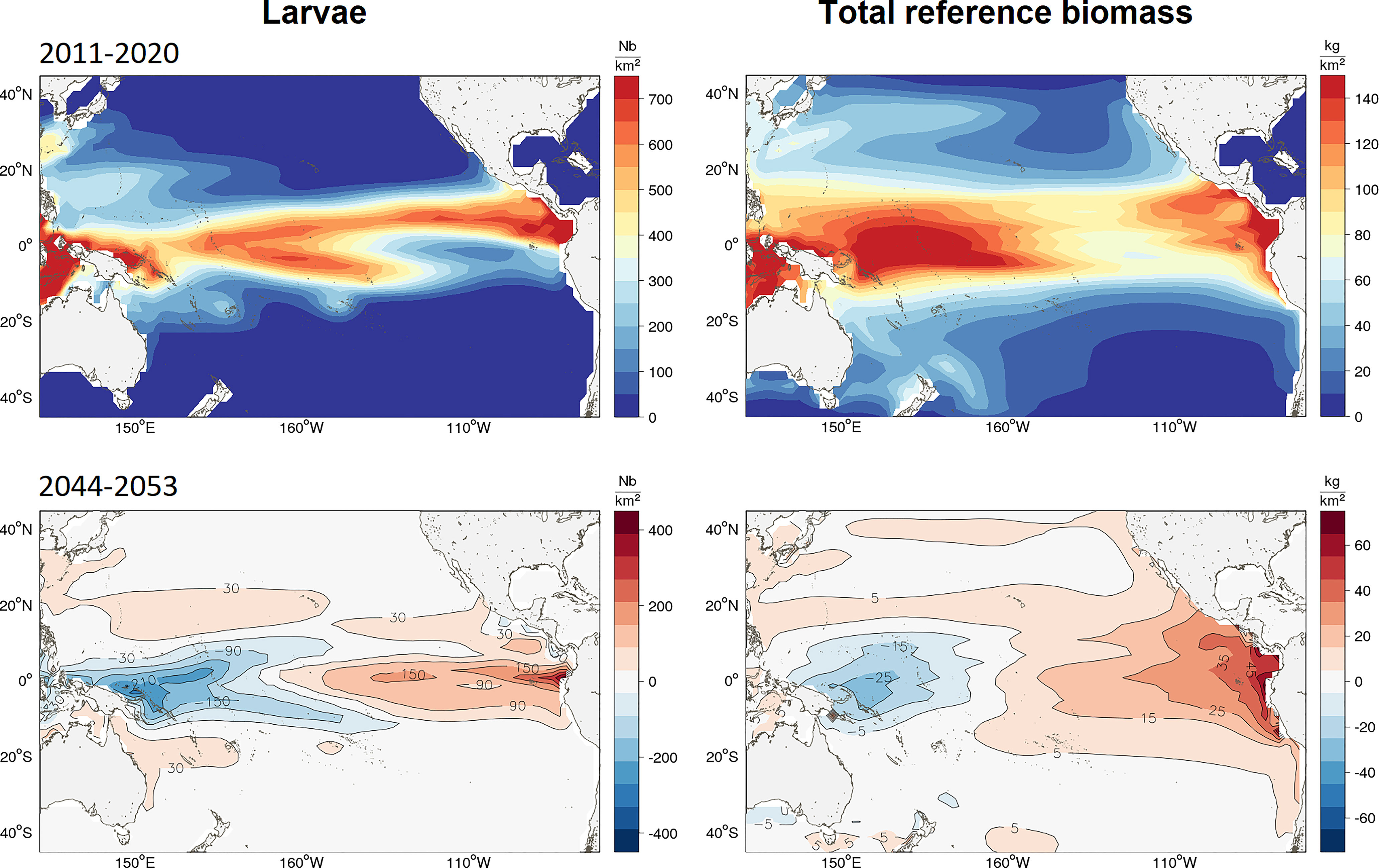
Figure 3 Maps of average distribution from the ensemble simulation of (top-left) yellowfin larvae in Nb/sq. km and (top-right) total unexploited (without fishing) biomass (top-right) in mt/sq.km predicted by SEAPODYM in 2001-10. The color maps for 2050 (2044-53) show the average biomass change since 2015 (in respective density units) projected to occur under the high emissions scenario RCP8.5.
Projection of Climate Change Effects Under Scenario RCP8.5
The predicted impact of climate change on the yellowfin tuna population was mainly driven by the change in the spawning habitat and subsequent larval recruitment in the equatorial region of the Pacific Ocean (20°N to 20°S). The projections of the REF4 ensemble showed opposite anomalies forming between the ETPO and WTPO by 2050 (Table 2 and Figure 3). A mean decrease of 10% was predicted in larval biomass for the WTPO and 5% for the CTPO respectively, whereas a mean increase of 30% was predicted for the ETPO (Figure 3, 4). The total reference biomass of yellowfin tuna (immature and mature combined) was projected to be stable at the basin-scale, but there was a clear shift in population density from the WTPO (Figure 3, 4). A 6% mean decrease (range -2 to -12%) by 2050 was predicted in total biomass for the WTPO whereas mean increases of 26% (range 7 to 49%) was predicted for the ETPO, 7% (range 4 to 9%) for the NPO and 10% (range 1 to 24%) for the SPO respectively (Table 2). No mean change (0%) was estimated for total biomass in the CTPO however the range varied between -7 to 7% (Table 2).
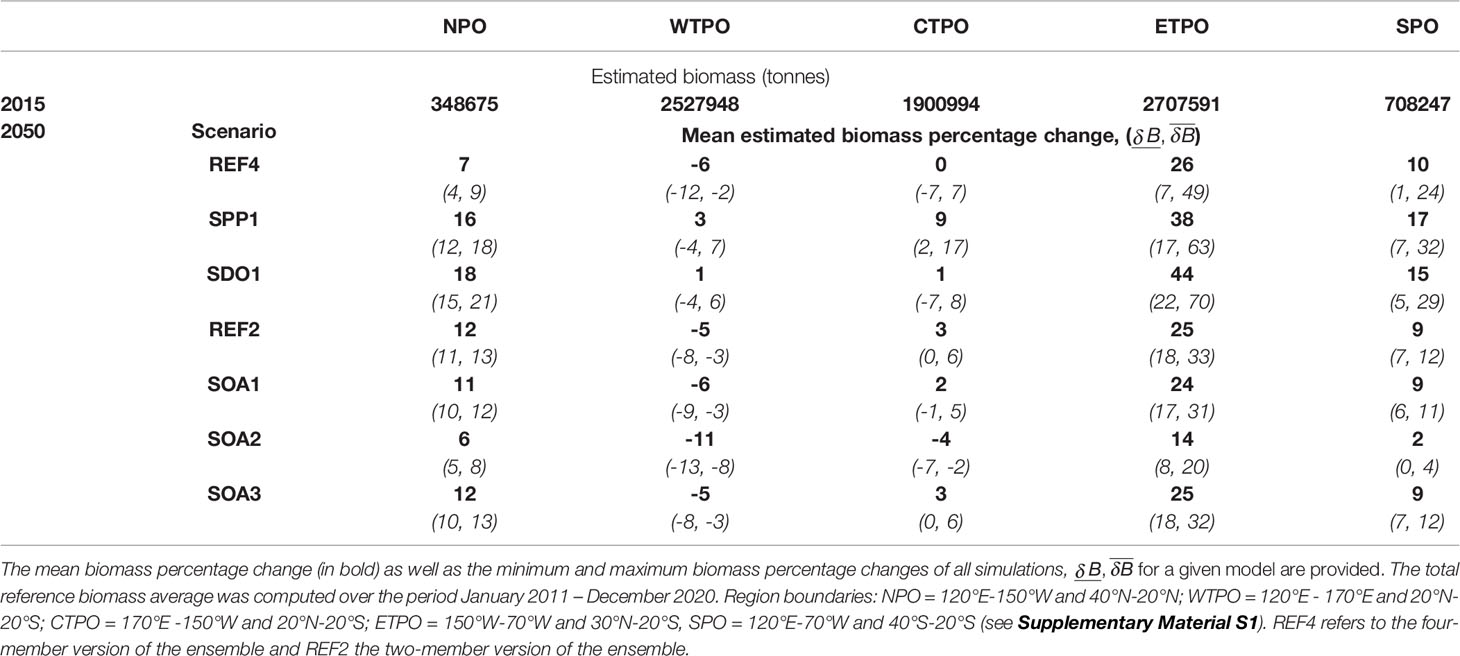
Table 2 The variability of projected changes (defined as the difference between the projected and reference biomass relative to the reference biomass) by large oceanic region and each model forcing for the yellowfin tuna stock in 2050 (average over January 2046 – December 2055) from the ensemble simulations for Pacific regions.
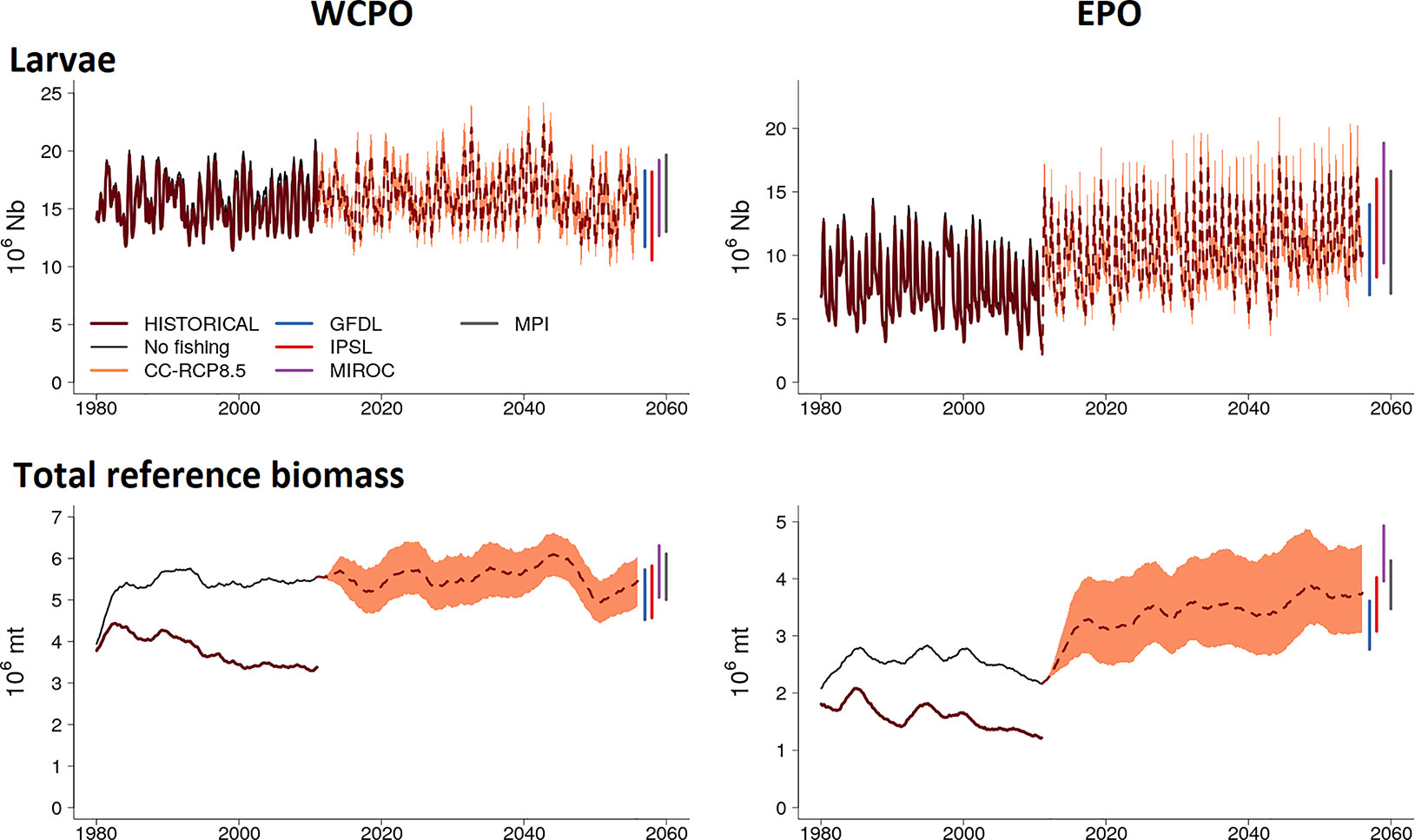
Figure 4 Envelope of predictions of larvae and total biomass computed from simulation ensembles under IPCC RCP8.5 scenario for the western central (WCPO) and eastern (EPO) Pacific Ocean. The change in larvae and total biomass, from 2011 onwards, is presented with the average (dotted line) and its envelope bounded by the 5% and 95% quantile values of the simulation ensembles.
The initial increase in larval and total reference biomass observed in the ensemble envelope immediately at the start of the simulation period is principally in response to the forcings of the MIROC and MPI ensemble members (Figure 4 and Figure S6a in Supplementary Material). Both MIROC and MPI predict much higher primary productivity for the period 2010-2045 which corresponds to high estimates for all forage groups through this period in comparison to the IPSL and GFDL ensemble members (Figure S6b and Figure S6c in Supplementary Material; Bell et al., 2021). The four ensemble members show convergence after 2045 (Figures S6a in Supplementary Material).
The addition of ocean acidification scenarios resulted in detectable impacts on the total reference biomass (Table 2 and Figure 5). A small negative effect (~1% change in biomass) was evident under the intermediate SOA1 scenario on the trends projected due to ocean warming in REF2. The predicted increases in the ETPO were weakened and the predicted decreases in biomass in the CTPO and WTPO were strengthened (Table 2). A larger effect of acidification was predicted for the higher mortality SOA2 scenario. This scenario reduced the REF2 predicted ETPO increase in biomass by 11% and strengthened the decrease in biomass in the CTPO and WTPO by 7% and 6% respectively (Table 2). There was no discernable effect for the low mortality SOA3 effect (Table 2). The total reference biomass in 2050 was reduced by 6% and 10% in the WCPO (Figure 5A) and EPO (Figure 5B) respectively, under SOA2 from the biomasses estimated in the REF2 simulation. Negative effects on larval density were evident by 2050 in the EPO for the intermediate scenario SOA1 (Figure 6). The higher mortality scenario SOA2 showed a stronger negative impact on larval density in the CTPO and ETPO. The impact of the higher mortality scenario due to acidification was visible at the end of the historical period (Figure 6). The projected decreases in pH to 2050 increased the magnitude and spatial extent of the negative impact on larval density in the CTPO and ETPO (Figure 6).
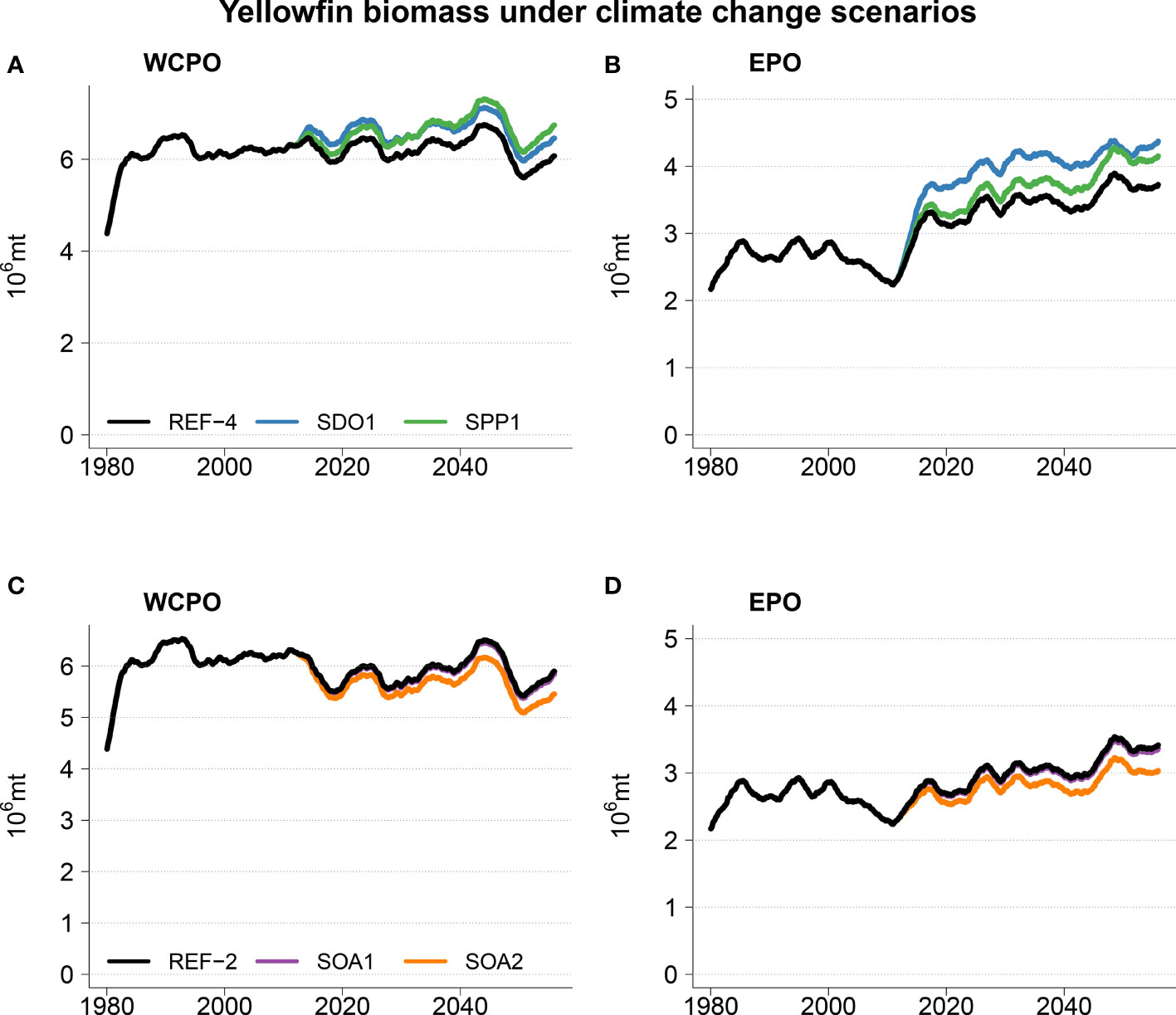
Figure 5 Top panels (A, B) projection mean of total yellowfin biomass for scenarios REF4 (reference), SPP1 (primary production), and SDO1 (no change in dissolved oxygen concentration). Bottom panels (C, D) projection mean of total yellowfin biomass for scenarios REF2 (reference), SOA1 (intermediate impact of ocean acidification on larval mortality) and SOA2 (high impact of ocean acidification on larval mortality). Note that SOA1 and REF2 are indistinguishable.
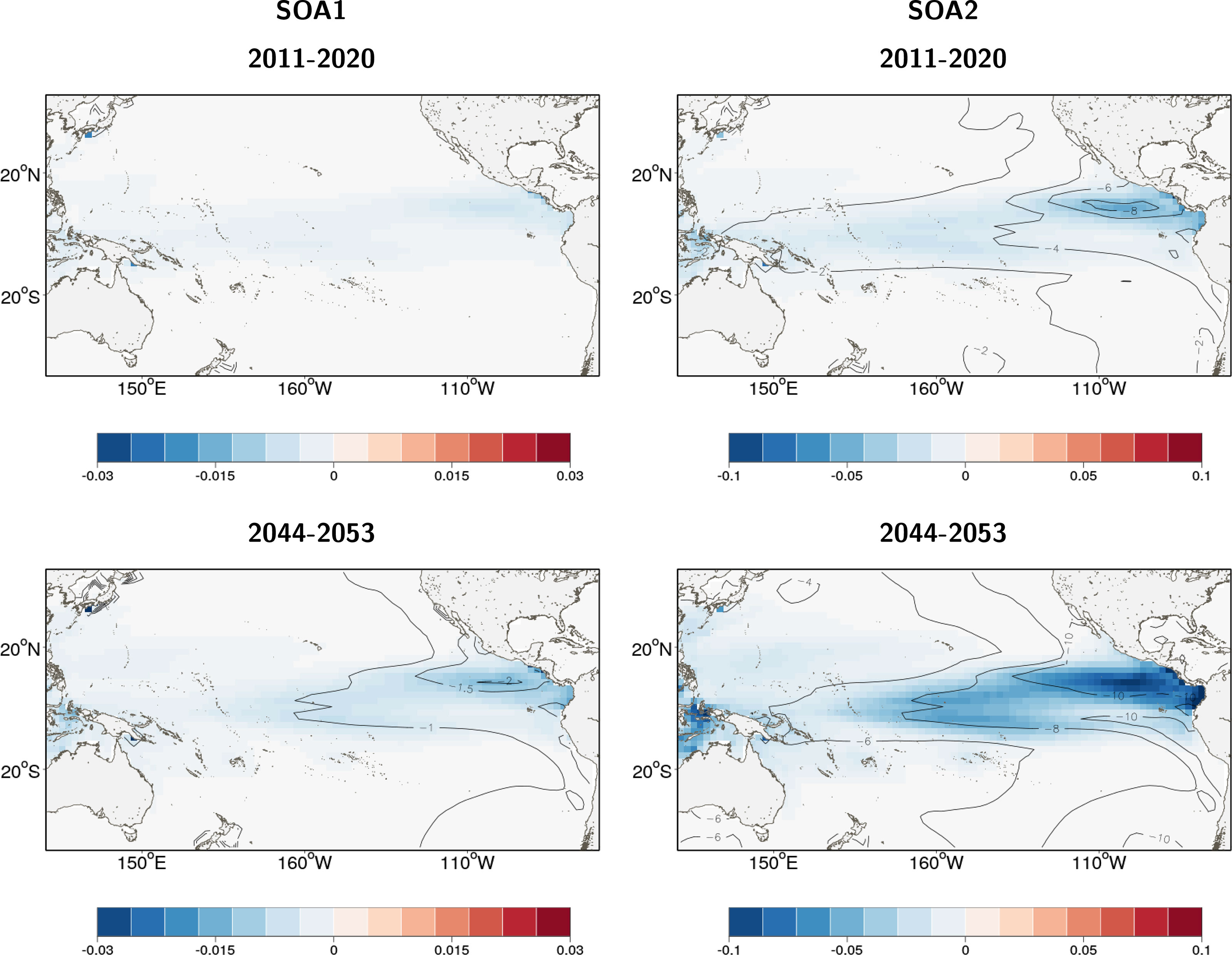
Figure 6 Change in density of yellowfin tuna larval recruits due to ocean acidification effects included in SEAPODYM, in 2015 (average of 2011-2020) and 2050 (average 2044-2053) for intermediate scenario SOA1 and high sensitivity scenario SOA2. There is no change for scenario SOA3. The color maps show the average density (thous.Nb/sq.km) change, and isopleths give the relative percentage change in density with respect to the density in respective year projected to occur without ocean acidification effect under a high emission scenario (RCP8.5) with 2 atmospheric forcings derived from Earth Climate models.
Yellowfin tuna dynamics were also sensitive to the other potential changes in oceanography (Figure 6). Decreases in dissolved oxygen predicted by earth climate models resulted in lower total reference biomass for those models, when compared to the scenario with no decrease in dissolved oxygen (SDO1), with this effect particularly apparent in the NPO, SPO and ETPO where biomasses were respectively, 11%, 5% and 18% higher under the SDO1 scenario (Table 2 and Figure 5). The scenario correcting for a possible underestimation of primary production in the tropical ocean had a positive effect. Under this scenario (when primary production gradually increases up to 10% at the end of the simulation in waters above 27°C), the mean total reference biomass of yellowfin tuna increased up to 9%, 9%, 9%, 7% and 12% by 2050 in the NPO, WTPO, CTPO, SPO and ETPO respectively, when compared to the REF4 scenario (Table 2 and Figure 5).
DISCUSSION
While the ocean is warming, it is also absorbing about a quarter of the anthropogenic CO2 released into the atmosphere, changing the chemistry of the seawater to more acidic conditions. These changes are expected to have consequences for many marine organisms, leading to changes in population abundances and species distributions, trophic network organization, and patterns of biodiversity (Hoegh-Guldberg et al., 2014; Pörtner et al., 2014; Stiasny et al., 2016; Sunday et al., 2017). Understanding the effects of multiple climate drivers acting simultaneously is difficult, and yet better understanding of these effects is needed (Boyd et al., 2018). The approach developed in this study provides a general framework to investigate the future of tuna populations under the impact of changes from multiple oceanic variables. It integrated key relationships between fish population dynamics and the environmental conditions of their marine ecosystem in a spatially explicit representation, with a robust estimation approach of population dynamics and fisheries parameters. The modelling framework allowed for the combined effects of ocean warming, acidification and changing ecosystem function to be evaluated at the population level.
Our analyses represent a significant step forward for evaluating the impacts of climate change on tuna resources in the Pacific Ocean. In previous modelling studies, the IPCC climate change AR4-A2 scenario (“business as usual”) was used with this model to project the future of different Pacific tuna populations (Lehodey et al., 2010b; Bell et al., 2013; Lehodey et al., 2013; Lehodey et al., 2015a). The potential for ocean acidification impact was not included as little knowledge was available to model the effect on tuna biology and thus population dynamics. Recent experimental studies on yellowfin tuna (Bromhead et al., 2015; Frommel et al., 2016) provided the first information making the inclusion of ocean acidification tractable. This information has been used in this study to introduce potential impacts of ocean acidification on the most critical and environmentally sensitive stage (larvae) of the fish life cycle. The ensemble approach adopted with additional effects of ocean acidification and potential changes in ocean dynamics provides a more representative evaluation of the variation in response by yellowfin tuna that can be expected under future ocean conditions.
An eastward shift in the distribution of yellowfin biomass was observed in the projections of the model ensemble in the absence of explicitly accounting for changes in acidification, primary productivity and dissolved oxygen. A similar impact of ocean warming (by 2050) has been predicted for skipjack and bigeye tuna in the Pacific Ocean (Bell et al., 2021). The extent of this shift for yellowfin tuna did not substantially differ when the three acidification scenarios were included in the ensemble; however, acidification was projected to weaken the magnitude of the increase in abundance projected in the ETPO in the absence of fishing. While the acidification impacts were predicted to be small in comparison to the ocean warming impact in the ETPO for our low and intermediate mortality scenarios, they were substantially larger for the highest mortality scenario explored.
These simulations demonstrate that the differing disturbances climate change imposes on marine ecosystems is not an “either or” scenario: both warming and acidification are expected to impact tuna populations into the future, and therefore the finding that acidification can reduce tuna biomass over and above the effects due to warming, is an important consideration for fisheries management. The ensemble for the acidification scenarios was reduced to the IPSL and GFDL earth climate models as only climatology pH values were available for the other two ensemble members. Both predicted lower primary production to 2050 than the MIROC and MPI earth climate models. Consequently, our simulations represent the more pessimistic futures of our ensemble. We also only modelled the effects of a mean decrease in pH in the epipelagic layer at a coarse spatial and temporal resolution (2 degrees by month). However, both the magnitude and range of pH reductions due to ocean acidification are expected to increase in the future at local scales (McNeil and Matsumoto, 2019). For example, the pH in the EPO in the vicinity of the Achotines Laboratory in the northern Panama Bight, where the experiments of Bromhead et al. (2015) were undertaken, is often at levels between 7.7 and 7.8 (internal water monitoring records of the Inter-American Tropical Tuna Commission Achotines Laboratory). These values are lower than the range of values in our forcings ensemble. Exposure to (naturally) fluctuating low pH conditions can have very different effects to those in constant pH reductions (e.g. Onitsuka et al., 2018; Jiang et al., 2019). Thus, it is possible that the integrated effects of frequent peaks of low pH have a stronger impact than that computed with the mean at lower frequency. This possibility could be tested in future SEAPODYM simulation with environmental forcings configured for higher temporal and spatial resolution. Such forcings would need to modulate the local-scale chemical, biological and physical processes that generate acidification hotspots (McNeil and Matsumoto, 2019). Ideally these processes need to be integrated within the next generation of CMIP models to ensure coherence between local and basin scale processes.
The changes in biomass distribution observed in our analyses are driven largely by the projected weakening of equatorial upwelling and current systems, and the warming of waters and associated increase of water stratification in the western region leading to lower primary production (Steinacher et al., 2010; Bopp et al., 2013). The favorable effects of SST warming on recruitment in the EPO produced a strong effect on larvae to the extent that it compensated for the reduced primary production observed in the GFDL and IPSL models. This observation emphasizes the benefits of examining the impacts of climate on tuna population dynamics within the SEAPODYM framework where non-linear and complex interactions between variables can be integrated. In general, tuna populations are thought to be sensitive to changes in productivity of the food web that supports them, ranging from changes in primary productivity and associated zooplankton on which tuna larvae are feeding to the abundance of micronekton prey for juvenile and adult tuna (Lehodey et al., 2011). In particular, decreases in micronekton are likely to increase natural mortality of tuna and lower the overall production of tuna fisheries in the region (Lehodey et al., 2011). Therefore, improved prediction of changes in ocean productivity under climate change is particularly important. Recent analyses suggest that the failure to include the effects of natural temperature variations in the phytoplankton responses used in CMIP5 models could result in substantial overestimation of actual phytoplankton production rates (Zaiss et al., 2021; Tittensor et al., 2021).
The impacts of acidification on ocean productivity were not considered in our analyses. There is no consensus on the effect of ocean acidification on ocean productivity with various and opposite effects observed from increased resilience (Valenzuela et al., 2018) to growth increase (Monteiro et al., 2016; Tortell et al., 2008) or abundance decrease and loss of diversity (Nagelkerken and Connell, 2015). Many calcareous zooplankton species may be susceptible to dissolution in acidic waters (Flynn et al., 2012; Uthicke et al., 2013; Cripps et al., 2014). Potential changes in the abundance or diversity of zooplankton species may have far reaching consequences in pelagic ecosystems since the connection to zooplankton by higher trophic orders is often the primary trophic pathway (Choy et al., 2015; Hunt et al., 2015; Griffiths et al., 2019) and food limitation has been shown to exacerbate negative effects of climate change on larval fishes (Stiasny et al., 2019). The consequences of changes in zooplankton composition and abundance could result in a general decrease or increase in system productivity without cascading impacts on ecosystem structure (Caron and Hutchins, 2013; Sswat et al., 2018). Alternatively, it may result in large structural changes to ecosystems, such as recent examples of jellyfish eruptions (Richardson et al., 2009). SEAPODYM utilises a Holling-III function to describe the relationship between prey density (zooplankton or primary production as a proxy) and the favorability of the spawning/larvae habitat controlling recruitment. Incorporating the impacts of acidification on trophic processes into SEAPODYM is potentially feasible through increasing the density of prey before the Holling-III function asymptotes with increasing acidity (i.e. effectively adding a third dimension to the Holling-III function).
Ocean warming effects on tuna larvae were integrated into SEAPODYM through an index describing suitability of habitat for spawning success and larval survival as a function of sea-surface temperatures, primary production (a proxy for prey of larvae) and epipelagic micronekton that are larval predators (Senina et al., 2020b).The temperature effect on larvae (modeled by a Gaussian function) had estimated optimal and standard deviation values of 28.94°C and 1.85°C respectively (Table S2). We did not include any threshold value for lethal effects of warming itself on larvae as noted to occur in laboratory experiments (Watson et al., 2018) for yellowtail kingfish (Seriola lalandi) (a coastal pelagic species). Future ocean temperatures were observed in that laboratory study to have a greater effect than predicted future ocean acidification conditions (Watson et al., 2018). Interestingly, in contrast to the laboratory experiments on yellowfin tuna (Frommel et al., 2016), no statistically significant sub-lethal effects were detected through histological examination of organs from samples taken during the same yellowtail kingfish experiments (Frommel et al., 2019), indicating that sub-lethal effects may not be as prevalent in more realistic experiments, although behavioral effects were detected (Jarrold et al., 2020). Conversely, it may be that sub-lethal physiological responses of larvae to acidification may be species-specific. Our mortality functions were based on single factor experiments of pH without crossing the impacts of temperature or parental acclimation (Bromhead et al., 2015). As such we had no observational evidence to parameterize the effect of potential interactions between warming and acidification. Moreover, the habitat index we applied in SEAPODYM is restricted to the epipelagic layer (Senina et al., 2020b). If yellowfin larvae utilize deeper ocean habitat layers, then pH values of these layers would need to be considered. Yellowfin larvae are predominately reported to be found between 0-30 m in the water column (Boehlert and Mundy, 1994; Lauth and Olson, 1996).
We did not include additional larval mortality due to acidification after day 7 when the empirical evidence of Bromhead et al. (2015) and Frommel et al. (2016) ended. Under optimal rearing/experimental conditions, approximately 80-95% of mortality during feeding stages occurs in the first week, however an additional 5-20% of mortality also occurs through juvenile metamorphosis (Margulies et al., 2016). The sublethal effects on larvae detected by Frommel et al. (2016) may also give reason to consider that the mortality may occur beyond day 7. The histological analyses conducted by Frommel et al. (2016) identified clear tissue damage to the kidney, liver and pancreas. These damages are irreversible and additional cumulative mortality would therefore plausibly be extended beyond 7 days of larval feeding. We accounted for this potential for higher mortality by the SOA2 scenario where we fitted the logistic curve through the upper (but not statistically significant) bound of mortality reported at pH=7.6 in Frommel et al. (2016). That is, we accounted for potential mortality after day 7 by increasing the mortality within the first 7 days. If the experiments of Bromhead et al. (2015) and Frommel et al. (2016) were to be repeated, it would be sensible to not only implement a cross design between parental acclimation, temperature, and acidity but also to extend the study duration to 30 days.
Significant sublethal changes in otolith morphology in yellowfin tuna were also observed at pH 7.6 (Wexler et al., 2021) in the experiment undertaken by Bromhead et al. (2015), similar to the effects reported for Mahi-Mahi (Coryphaena hippurus) (Bignami et al., 2014), a species which occupies similar habitats to yellowfin tuna. Altered metabolism, growth and swimming behavior were also reported for this species (Bignami et al., 2014; Pimentel et al., 2014) as was observed for yellowtail kingfish (Jarrold et al., 2020). In a separate study, Heuer et al. (2021) also reported a reduction in metabolic rate of first-feeding yellowfin larvae under increased acidification conditions (1900 μatm CO2). The parameterization of SEAPODYM did not include any changed behavior or growth associated with differing acidification levels.
The projected changes in distribution of tuna are likely to have consequences for fishing operations in the Pacific Ocean, including industrial, artisanal and subsistence fisheries. Changes in the location of fishing grounds and the catchability of tuna by surface and longline fisheries are expected to affect the sector in a similar way to that observed during El Niño events. In particular, fishing grounds are likely to be displaced further eastward along the equator and at higher latitudes, increasing access to tuna resources in international waters (Lehodey et al., 2011; Bell et al., 2013; Bell et al., 2021). Climate driven redistribution of tuna is an ongoing concern for fisheries management in the WCPO with tuna fisheries providing government revenue and supporting food security for the numerous island nations and territories in the region (Bell et al., 2013; Gilman et al., 2016; Bell et al., 2018; Bell et al., 2021). Annual catches of tuna in the WCPO have varied between 2.5-2.9 million mt since 2012 (OFP et al., 2021) of which approximately 57% is harvested annually from the EEZ of these nations (Bell et al., 2021). Access fees paid by tuna-fishing fleets provide between 4 and 84% of all government revenue to these nations (Bell et al., 2021). Yellowfin tuna has also been identified as a priority species to meet future food security needs in the region given its accessibility for artisanal fisheries (Bell et al., 2018). Potential climate adaptation in fisheries policies and management that facilitate access to tuna resources for these nations will need to consider not only the distribution change but also the cumulative negative impacts of ocean acidification and changing productivity.
Our simulations suggest that losses of 15-25 kg/km2 of yellowfin tuna in the exclusive economic zones of Papua New Guinea, Solomon Islands, Nauru, Federated States of Micronesia and the Republic of the Marshall Islands can be expected by 2050 (i.e. a reduction of approximately 75,000-149,000 t across their combined EEZ area of 9.28 Million km2). While the effects of and on fishing were not included in our simulations it is likely that these losses will impact the industrial tuna fisheries operating in these exclusive economic zones and on the supporting food systems of each country. Yellowfin tuna comprises 25% of the 940,000 t of the recent average total annual tuna catch for these EEZs (WCPFC, 2020; Bell et al., 2021). This total tuna catch is expected to decrease to approximately 670,000 t by 2050 due to the effects of ocean warming (Bell et al., 2021) with yellowfin estimated to contribute approximately 177,000 t to this total in 2050. Catches of yellowfin however in near-shore and archipelagic sub-regions have recently contributed approximately 16% to the total yellowfin catch (SPC held catch data). The fisheries in these sub-regions include artisanal and subsistence fishers that supply local markets and value chains in addition to industrial fisheries. Yellowfin tuna catches in these sub-regions could be expected to decrease to approximately 29,000 t by 2050, assuming current fishery gears and effort patterns are maintained. Regionally, the leaders of the Pacific Islands have committed to increase the availability of fish from tuna fisheries for local consumption by 40,000 t per year to meet the food security needs of their populations (FFA and SPC, 2015). While developing opportunities to access fish from tuna fisheries through offloading of catch from the industrial fisheries is an important component to this supply chain (James et al., 2018) the development of robust systems through artisanal and subsistence fishers are also necessary for rural and urban areas away from ports used by industrial vessels (Gillett, 2016). Yellowfin tuna is a priority species for artisanal and subsistence fisheries in the western Pacific as their distribution includes both oceanic and near-coastal habitats (Bell et al., 2018). Industrial and artisanal fisheries already overlap in some regions of the western Pacific (e.g., Tremblay-Boyer, 2013; Leroy et al., 2016). Reduced access to yellowfin tuna either through scarcity of the resource or increased catch variability are likely to increase interactions between these fisheries. Moreover, reduced access is likely to disproportionally impact the value chains provided by artisanal and subsistence fishers as they do not use vessels with the capability to safely fish open ocean and distant habitats (Remola and Gudmundsson, 2018). Much of the focus on preparing the region for the impacts of climate change have centered on the industrial tuna fisheries including the urgent need to reduce green-house gas emissions (Bell et al., 2021). Our simulations suggest that attention is also warranted towards understanding the potential impact on the food systems provided by artisanal and subsistence fishers. Developing methods to reliably project the distribution and extent of future fishing effort will be important for quantifying the impacts on regional food security and artisanal/subsistence fisheries in addition to the impacts on industrial fisheries.
As in many other published studies on the impact of climate change on marine resources and biodiversity (e.g. Dueri et al., 2014; Lehodey et al., 2015a; Bianucci et al., 2016; Gailbraith et al., 2017), we have used projections of physical and biogeochemical forcings derived from earth climate models such as those associated with the World Climate Research Programme’s Coupled Model Intercomparison Project (Meehl, 1995; Meehl et al., 2000). However, the CMIP models have known biases when applied at local and regional scales (Li et al., 2019; Mckenna et al., 2020; Tian and Dong, 2020; Samuels et al., 2021; Tang et al., 2021; Zhu et al., 2021) and without corrections these biases can lead to spurious correlations when estimating habitat parameters over the historical period (Lehodey et al., 2013). Although we applied both bias-correction (as described in Bell et al., 2021) and ensemble simulations (Semenov and Stratonovitch, 2010; Tittensor et al., 2018; Eddy, 2019), there remains considerable uncertainty in our understanding of how the dynamics of the Western Pacific warm-pool will be altered due to climate change (Brown et al., 2014) and how dissolved oxygen availability within the Pacific will change (Ganachaud et al., 2013). This uncertainty may not have been fully captured by the ensemble of models used. Our inclusion of alternate scenarios for primary production and dissolved oxygen demonstrates this sensitivity. Since the Western Pacific warm pool is a key feature that influences the ecology of tuna in the Pacific (Lehodey et al., 2020), we chose four earth climate models that captured the historical dynamics of the Western Pacific warm pool, particularly its association with the El Nino Southern Oscillation phenomenon. Further comparative analyses by adding models, including biogeochemical ones, to this ensemble would aid with identifying and removing bias associated with Western Pacific warm pool dynamics and more fully capture plausible levels of variation. This will be an important next step for modelling the effects of climate on tuna, including longer-term forecast where both CMIP5 and CMIP6 models predict decreases in primary productivity after 2060 (Tittensor et al., 2021; Zaiss et al., 2021). Preliminary longer-term forecasts for Pacific tunas indicate that decreases in primary production are expected to be associated with potential decreases in tuna abundances at basin scales (Bell et al., 2013; Senina et al., 2018; Chang et al., 2021).
The model ensemble used in this study provided a representative evaluation of the variation in ocean conditions that can be expected under future ocean conditions. The models included were chosen on the basis that they captured cycles similar to ENSO in their simulations (Bellenger et al., 2014). However, their dynamics produce divergent regional trends for important ecosystem drivers such as primary productivity. The methods used to construct the ensemble are particularly beneficial for the evaluation of climate effects within SEAPODYM. The method applied projects the historical ocean conditions into the future with the addition of the climate trend from each of the included CMIP5 models. This has the distinct advantage of ensuring that the optimized parameters of SEAPODYM, generated from the historical time-series are consistent for the ensemble. The alternative approach of optimizing SEAPODYM to the historical time-series of each CMIP5 model would result in estimation of different population dynamics for the historical period. This would confound the ability to differentiate the impact of climate from the impacts of these different population dynamics. The ensemble members provide robust representations of the global atmospheric conditions (Bellenger et al., 2014), and although they were corrected for known regional biases in the Pacific Ocean, it is possible that other biases, albeit unknown, remain in the ensemble. Such biases may contribute to the higher and increasing variability of primary production observed in the EPO between the climate models for example. Identifying and correcting for regional and sub-regional biases will be an important task to appropriately migrate the model ensemble used in this study from CMIP5 to CMIP6 models.
Developing capacity to evaluate the combined effects of multiple drivers of climate change will be necessary for the development of robust policies that account for future ocean conditions. The potential for evolutionary adaptation to future ocean warming and acidification will also be an important consideration in policy development (Munday et al., 2013; Reusch, 2014; Sunday et al., 2014). Heritable genetic and epigenetic variation in thermal tolerance has been identified in some reef fishes (Munday et al., 2017; Ryu et al., 2018), however even fishes with short generation turnover see low potential for evolutionary rescue (Morgan et al., 2020). While the speed and spatial scale at which adaptation is likely to occur in yellowfin and other tuna species is not known, given their generation times it is unlikely that evolutionary adaptation will occur quickly enough to offset the effects observed in our simulations (Bell, 2013). Developing a better understanding of sub-regional and local oceanographic effects and the likely prevalence of refuge habitats remaining in the EEZ of the Pacific Island Nations is likely to be more fruitful for policy formation. The adaptive capacity however will be an important consideration for understanding the resilience of yellowfin tuna populations beyond 2050. Moreover, as we did not consider adaptive capacity in our simulations it is possible that inherent adaptive traits may mitigate against some of the impacts we observed in the period leading up to 2050. The preparation of CMIP6 earth climate models at 1 degree resolution or higher (Eyring et al., 2016) provides a first opportunity to examine how refuge habitats may offset the broader level impacts of climate change. Similarly, the simulations only considered one bio-geochemical model and coupling other models would allow the sensitivities to these processes to be evaluated. The modelling framework applied in this study provides a tool for evaluating such effects and informing policy development.
Data Availability Statement
Publicly available datasets were analyzed in this study. This data can be found here: The executable files for SEAPODYM, together with the input files, the outputs and the SEAPODYM manual, are available on a repository at https://osf.io/qa8w4/. The 3D ocean data from the ESMs in netcdf format used to inform the SEAPODYM modelling are available at http://data.umr-lops.fr/pub/AFCM85/.
Ethics Statement
Ethical review and approval was not required for the animal study because no animals were captured, handled or observed in this study. All data used was accessed from the literature or from commercial fisheries landings.
Author Contributions
SN, PL, DB, AYF, DM, JEW, PLM, JHav, and NS conceived the idea for the research and manuscript. All authors contributed to the development of the functional relationship between mortality and ocean acidification. IS undertook the modelling and data analyses. All authors contributed to manuscript preparation and revision and approved the submitted version.
Funding
This work was supported by the Pacific Community and Collecte Localisation Satellites, with financial assistance from the Australian Department of Foreign Affairs and Trade, the New Zealand Ministry of Foreign Affairs and Trade, the GEF OFMP II Project, and the Government of Monaco. The Western and Central Pacific Commission and Inter American Tropical Tuna Commission provided access to non-public domain data for the purposes of implementing the work programme.
Conflict of Interest
The authors declare that the research was conducted in the absence of any commercial or financial relationships that could be construed as a potential conflict of interest.
Publisher’s Note
All claims expressed in this article are solely those of the authors and do not necessarily represent those of their affiliated organizations, or those of the publisher, the editors and the reviewers. Any product that may be evaluated in this article, or claim that may be made by its manufacturer, is not guaranteed or endorsed by the publisher.
Acknowledgments
We are grateful to Peter Williams and Sylvain Caillot for their support in processing fishing and tagging data. Darren Parsons, Tommy Moore and Michael Gillings participated in a workshop where the initials concept around inclusion of ocean acidification within the SEAPODYM framework were first discussed. Their inputs to this discussion are acknowledged as are the contributions of Jeanne Wexler and Maria Stein to the implementation of the experiments conducted at the Achotines Laboratory (as described in Bromhead et al., 2015). Graham Pilling is thanked for his comments on a final draft of this manuscript. We thank the reviewers and editor for their thoughtful and constructive comments.
Supplementary Material
The Supplementary Material for this article can be found online at: https://www.frontiersin.org/articles/10.3389/fmars.2022.816772/full#supplementary-material
References
Aumont O., Ethé C., Tagliabue A., Bopp L., Gehlen M. (2015). PISCES-V2: An Ocean Biogeochemical Model for Carbon and Ecosystem Studies. Geosci. Model Dev. 8, 2465–2513. doi: 10.5194/gmd-8-2465-2015
Barnett T. P., Pierce D. W., Achuta Rao K. M., Gleckler P. J., Santer B. D., Gregory J. M., et al. (2005). Penetration of Human-Induced Warming Into the World’s Oceans. Science 309, 284–287. doi: 10.1126/science.1112418
Bell G. (2013). Evolutionary Rescue and the Limits of Adaptation. Philos. Trans. R. Soc Lond. B Biol. Sci. 368, 20120080. doi: 10.1098/rstb.2012.0080
Bell J. D., Allain V., Allison E. H., Andréfouët S., Andrew N. L., Batty M. J., et al. (2015). Diversifying the Use of Tuna to Improve Public Health in Pacific Island Countries and Territories. Mar. Pol. 51, 584–591. doi: 10.1016/j.marpol.2014.10.005
Bell J. D., Cisneros A., Crowder L., Hanich Q., Johnson J., Lehodey P., et al. (2018). Adaptations to Maintain the Contributions of Small-Scale Fisheries to Food Security in the Pacific Islands. Mar. Pol. 88, 303–314. doi: 10.1016/j.marpol.2017.05.019
Bellenger H., Guilyardi E., Leloup J., Lengaigne M., Vialard J. (2014). ENSO Representation in Climate Models: From CMIP3 to CMIP5. Clim. Dyn. 42, 1999–2018. doi: 10.1007/s00382-013-1783-z
Bell J. D., Ganachaud A., Gehrke P. C., Griffiths S. P., Hobday A. J., Hoegh-Guldberg O., et al. (2013). Tropical Pacific Fisheries and Aquaculture Will Respond Differently to Climate Change. Nat. Clim. Change 3, 591–599. doi: 10.1038/nclimate1838
Bell J. D., Senina I., Adams T., Aumont O., Calmettes B., Clark S., et al. (2021). Pathways to Sustaining Tuna-Dependent Pacific Island Economies During Climate Change. Nat. Sustain. 4, 900–910. doi: 10.1038/s41893-021-00745-z
Bianucci L., Fennel K., Chabot D., Shackell N., Lavoie D. (2016). Ocean Biogeochemical Models as Management Tools: A Case Study for Atlantic Wolffish and Declining Oxygen. ICES J. Mar. Sci. 73, 263–274. doi: 10.1093/icesjms/fsv220
Bignami S., Sponaugle S., Cowen R. K. (2014). Effects of Ocean Acidification on the Larvae of a High-Value Pelagic Fisheries Species, Mahi-Mahi Coryphaena Hippurus. Aquat. Biol. 21, 249–260. doi: 10.3354/ab00598
Boehlert G. W., Mundy B. C. (1994). Vertical and Onshore-Offshore Distributional Patterns of Tuna Larvae in Relation to Physical Habitat Features. Mar. Ecol. Prog. Ser. 107, 1–13. doi: 10.3354/meps107001
Bopp L., Resplandy L., Orr J. C., Doney S. C., Dunne J. P., Gehlen M., et al. (2013). Multiple Stressors of Ocean Ecosystems in the 21st Century: Projections With CMIP5 Models. Biogeosciences 10, 6225–6245. doi: 10.5194/bg-10-6225-2013
Boyd P. W., Collins S., Dupont S., Fabricius K., Gattuso J. P., Havenhand J., et al. (2018). Experimental Strategies to Assess the Biological Ramifications of Multiple Drivers of Global Ocean Change—A Review. Glob. Change Biol. 24, 2239–2261. doi: 10.1111/gcb.14102
Brauner C. J., Shartau R. B., Damsgaard C., Esbaugh A. J., Wilson R. W., Grosell M. (2019). “Acid-Base Physiology and CO2 Homeostasis: Regulation and Compensation in Response to Elevated Environmental CO2,” in Fish Physiology Volume 37: Carbon Dioxide. Eds. Grosell M., Munday P. L., Farrell A. P., Brauner C. J. (Cambridge USA: Academic Press), 69–132.
Bromhead D., Scholey V., Nicol S., Margulies D., Wexler J., Stein M., et al. (2015). The Potential Impact of Ocean Acidification Upon Eggs and Larvae of Yellowfin Tuna (Thunnus Albacares). Deep Sea Res. Part II 113, 268–279. doi: 10.1016/j.dsr2.2014.03.019
Brown J. N., Langlais C., Maes C. (2014). Zonal Structure and Variability of the Western Pacific Dynamic Warm Pool Edge in CMIP5. Clim. Dyn. 42, 3061–3076. doi: 10.1007/s00382-013-1931-5
Caldeira K., Wickett M. E. (2003). Anthropogenic Carbon and Ocean pH. Nature 425, 365. doi: 10.1038/425365a
Caron D. A., Hutchins D. A. (2013). The Effects of Changing Climate on Microzooplankton Grazing and Community Structure: Drivers, Predictions and Knowledge Gaps. J. Plankton Res. 35, 235–252. doi: 10.1093/plankt/fbs091
Chang Y.-J., Hsu J., Lai P.-K., Lan K.-W., Tsai W.-P. (2021). Evaluation of the Impacts of Climate Change on Albacore Distribution in the South Pacific Ocean by Using Ensemble Forecast. Front. Mar. Sci. 8. doi: 10.3389/fmars.2021.731950
Choy C. A., Popp B. N., Hannides C., Drazen J. C. (2015). Trophic Structure and Food Resources of Epipelagic and Mesopelagic Fishes in the North Pacific Subtropical Gyre Ecosystem Inferred From Nitrogen Isotopic Compositions. Limnol. Oceanogr. 60, 1156–1171. doi: 10.1002/lno.10085
Cripps G., Lindeque P., Flynn K. J. (2014). Have We Been Underestimating the Effects of Ocean Acidification in Zooplankton? Glob. Change Biol. 20, 3377–3385. doi: 10.1111/gcb.12582
Dee D. P., Uppala S. M., Simmons A. J., Berrisford P., Poli P., Kobayashi S., et al. (2011). The ERA-Interim Reanalysis: Configuration and Performance of the Data Assimilation System. Q. J. R. Meteorol. Soc 137553, – 597. doi: 10.1002/qj.828
Doney S. C., Busch D. S., Cooley S. R., Kroeker K. J. (2020). Acidification on Marine Ecosystems and Reliant Human Communities. Annu. Rev. Environ. Resour. 45, 83–112. doi: 10.1146/annurev-environ-012320-083019
Doney S. C., Fabry V. J., Feely R. A., Kleypas J. A. (2009). Ocean Acidification: The Other CO2 Problem. Annu. Rev. Mar. Sci. 1, 169–192. doi: 10.1146/annurev.marine.010908.163834
Dragon A.-C., Senina I., Hintzen N., Lehodey P. (2017). A South-Pacific Basin-Scale Modelling of Jack Mackerel Under Combined Impacts of Fishing and Climate Variability. Fish. Oceanogr. 27, 97–113. doi: 10.1111/fog.12234
Dragon A.-C., Senina I., Titaud O., Calmettes B., Conchon A., Arrizabalaga H., et al. (2015). An Ecosystem-Driven Model for Spatial Dynamics and Stock Assessment of North Atlantic Albacore Tuna. Can. J. Fish. Aquat. Sci. 72, 864–878. doi: 10.1139/cjfas-2014-0338
Dueri S., Bopp L., Maury O. (2014). Projecting the Impacts of Climate Change on Skipjack Tuna Abundance and Spatial Distribution. Glob. Chang. Biol. 20, 742–753. doi: 10.1111/gcb.12460
Dueri S., Guillotreau P., Jiménez-Toribio R., Oliveros-Ramos R., Bopp L., Maury O. (2016). Food Security or Economic Profitability? Projecting the Effects of Climate and Socioeconomic Changes on Global Skipjack Tuna Fisheries Under Three Management Strategies. Glob. Environ. Change 41, 1–12. doi: 10.1016/j.gloenvcha.2016.08.003
Eddy T. D. (2019). “Building Confidence in Projections of Future Ocean Capacity, in Predicting Future Oceans,” in Predicting Future Oceans: Sustainability of Ocean and Human Systems Amidst Global Environmental Change. Eds. Cisneros-Montemayor A. M., Cheung W. W. L., Ota Y. (Netherlands: Elsevier).
Eyring V., Bony S., Meehl G. A., Senior C. A., Stevens B., Stouffer R. J., et al. (2016). Overview of the Coupled Model Intercomparison Project Phase 6 (CMIP6) Experimental Design and Organization. Geosci. Model Dev. 9, 1937–1958. doi: 10.5194/gmd-9-1937-2016
Fabry V. J., Seibel B. A., Feely R. A., Orr J. C. (2008). Impacts of Ocean Acidification on Marine Fauna and Ecosystem Processes. –. ICES J. Mar. Sci. 65, 414–432. doi: 10.1093/icesjms/fsn048
FAO (2020). The State of World Fisheries and Aquaculture 2020. Sustainability in Action (Rome: Food and Agriculture Organization of the United Nations). doi: 10.4060/ca9229en
Feely R. A., Sabine C. L., Lee K., Berelson W., Kleypas J., Fabry V. J., et al. (2004). Impact of Anthropogenic CO2 on the CaCO3 System in the Oceans. Science 305, 362–366. doi: 10.1126/science.1097329
FFA, and SPC (2015). Future of Fisheries: A Regional Roadmap for Sustainable Pacific Fisheries (Noumea, New Caledonia: Pacific Islands Forum Fisheries Agency, Honiara, Solomon Islands and Pacific Community).
Flynn K. J., Blackford J. C., Baird M. E., Raven J. A., Clark D. R., Beardall J., et al. (2012). Changes in pH at the Exterior Surface of Plankton With Ocean Acidification. Nat. Clim. Change 2, 510–513. doi: 10.1038/nclimate1489
Frommel A. Y., Brauner C. J., Allan B. J. M., Nicol S., Parsons D. M., Pether S. M. J., et al. (2019). Organ Health and Development in Larval Kingfish are Unaffected by Ocean Acidification and Warming. PeerJ 7, e8266. doi: 10.7717/peerj.8266
Frommel A., Margulies D., Wexler J. B., Stein M. S., Scholey V. P., Williamson J. E., et al. (2016). Ocean Acidification has Lethal and Sub-Lethal Effects on Larval Development of Yellowfin Tuna, Thunnus Albacares. J. Exp. Mar. Biol. Ecol. 482, 18–24. doi: 10.1016/j.jembe.2016.04.008
Galbraith E., Carozza D., Bianchi D. (2017). A Coupled Human-Earth Model Perspective on Long-Term Trends in the Global Marine Fishery. Nat. Commun. 8, 14884. doi: 10.1038/ncomms14884
Ganachaud A., Sen Gupta A., Brown J. N., Evans K., Maes C., Muir L., et al. (2013). Projected Changes in the Tropical Pacific Ocean of Importance to Tuna Fisheries. Clim. Change 119, 163–169. doi: 10.1007/s10584-012-0631-1
Gillet R. (2009). Fisheries in the Economies of Pacific Island Countries and Territories (Manila: Asian Development Bank).
Gillet R., Cartwright I. (2010). The Future of Pacific Island Fisheries (Noumea: Secretariat of the Pacific Community).
Gillett R. (2016). Fisheries in the Economies of Pacific Island Countries and Territories (Noumea, New Caledonia: Pacific Community).
Gilman E., Allain V., Collette B., Hampton J., Lehodey P. (2016). “Effects of Ocean Warming on Pelagic Tunas, a Review,” in Explaining Ocean Warming: Causes, Scale, Effects and Consequences. Eds. Laffole D., Baxter J. (Gland: International Union for the Conservation of Nature), 254–270.
Griffiths S. P., Allain V., Hoyle S. D., Lawson T. A., Nicol S. J. (2019). Just a FAD? Ecosystem Impacts of Tuna Purse-Seine Fishing Associated With Fish Aggregating Devices in the Western Pacific Warm Pool Province. Fish. Oceanogr. 28, 94–112. doi: 10.1111/fog.12389
Grosell M., Munday P. L., Farrell A. P., Brauner C. J. (2019). Fish Physiology Volume 37: Carbon Dioxide (Amsterdam: Elsevier).
Heuer R., Wang Y., Pasparakis C., Zhang W., Scholey V. P., Margulies D., et al. (2021). Effects of Elevated CO2 on Metabolic Rate, Nitrogenous Waste Handling, and Specific Gravity in the Early Life Stages of Yellowfin Tuna (Thunnus Albacares) (Miami: U Miami internal document). Available at:cmhldWVyQHJzbWFzLm1pYW1pLmVkdQ==.
Heuer R., Welch M. J., Rummer J. L., Munday P. L., Grosell M. (2016). Altered Brain Ion Gradients Following Compensation for Elevated CO2 are Linked to Behavioural Alterations in a Coral Reef Fish. Sci. Rep. 6, 33216. doi: 10.1038/srep33216
Hobday A. J., Young J. W., Abe O., Costa D. P., Cowen R. K., Evans K., et al. (2013). Climate Impacts and Oceanic Top Predators: Moving From Impacts to Adaptation in Oceanic Systems. Rev. Fish Biol. Fish. 23, 537–546. doi: 10.1007/s11160-013-9311-0
Hoegh-Guldberg O., Cai R., Poloczanska E. S., Brewer P. G., Sundby S., Hilmi K., et al. (2014). “The Ocean,” in Climate Change 2014: Impacts, Adaptation and Vulnerability. Contribution of Working Group II to the Fifth Assessment Report of the Intergovernmental Panel on Climate Change. Eds. Field C. B., Barros V. R., Dokken D. J., Mach K. J., Mastrandrea M. D., Bilir T. E., et al. (Cambridge: Cambridge University Press), 1655–1731.
Hunt B. P. V., Allain V., Menkes C., Lorrain A., Graham B., Rodier M., et al. (2015). A Coupled Stable Isotope-Size Spectrum Approach to Understanding Pelagic Food-Web Dynamics: A Case Study From the Southwest Sub-Tropical Pacific. Deep Sea Res. Part II 113, 208–224. doi: 10.1016/j.dsr2.2014.10.023
Iida Y., Takatani Y., Kojima A., Ishii M. (2021). Global Trends of Ocean CO2 Sink and Ocean Acidification: An Observation-Based Reconstruction of Surface Ocean Inorganic Carbon Variables. J. Oceanogr. 77, 323–358. doi: 10.1007/s10872-020-00571-5
James P. A. S., Tidd A., Kaitu L. P. (2018). The Impact of Industrial Tuna Fishing on Small-Scale Fishers and Economies in the Pacific. Mar. Pol. 95, 189–198. doi: 10.1016/j.marpol.2018.03.021
Jarrold M. D., Welch M. J., McMahon S. J., McArley T., Allan B. J. M., Watson S. A., et al. (2020). Elevated CO2 Affects Anxiety But Not a Range of Other Behaviours in Juvenile Yellowtail Kingfish. Mar. Environ. Res. 157, 104863. doi: 10.1016/j.marenvres.2019.104863
Jiang L., Guo Y.-J., Zhang F., Zhang Y.-Y., McCook L. J., Yuan X.-C., et al. (2019). Diurnally Fluctuating Pco2 Modifies the Physiological Responses of Coral Recruits Under Ocean Acidification. Front. Physiol. 9, 1952. doi: 10.3389/fphys.2018.01952
Juan-Jorda M., Mosqueira I., Cooper A. B., Freire J., Dulvy N. K. (2011). Global Population Trajectories of Tunas and Their Relatives. Proc. Natl. Acad. Sci. U.S.A. 108, 20650–20655. doi: 10.1073/pnas.1107743108
Kroeker K. J., Kordas R. L., Crim R., Hendriks I. E., Ramajo L., Singh G. S., et al. (2013). Impacts of Ocean Acidification on Marine Organisms: Quantifying Sensitivities and Interaction With Warming. Glob. Change Biol. 19, 1884–1896. doi: 10.1111/gcb.12179
Langley A., Hoyle S., Hampton J. (2011). Working Paper WCPFC-SC7-2011/SA-WP-03: Stock Assessment of Yellowfin Tuna in the Western and Central Pacific Ocean (Pohnpei: Western and Central Pacific Fisheries Commission). Available at: https://www.wcpfc.int/meetings/7th-regular-session-scientific-committee.
Laubenstein T. D., Rummer J. L., McCormick M. I., Munday P. L. (2019). A Negative Correlation Between Behavioural and Physiological Performance Under Ocean Acidification and Warming. Sci. Rep. 9, 4265. doi: 10.1038/s41598-018-36747-9
Laufkötter C., Vogt M., Gruber N., Aita-Noguchi M., Aumont O., Bopp L., et al. (2015). Drivers and Uncertainties of Future Global Marine Primary Production in Marine Ecosystem Models. Biogeosciences 12, 6955–6984. doi: 10.5194/bg-12-6955-2015
Lauth R. R., Olson R. J. (1996). Distribution and Abundance of Larval Scombridae in Relation to the Physical Environment in the Northwestern Panama Bight. Inter-Amer. Trop. Tuna Commun. Bull. 21, 127–167. Available at: https://www.iattc.org/BulletinsENG.htm.
Lehodey P., Bertrand A., Hobday A. J., Kiyofuji H., McClatchie S., Menkès C. E, et al. (2020). “ENSO Impact on Marine Fisheries and Ecosystems,” in El Niño Southern Oscillation in a Changing. Eds. McPhaden M. J., Santoso A., Cai W.. Geophysical Monograph 253 (Washington D.C.: American Geophysical Union). doi: 10.1002/9781119548164.ch19
Lehodey P., Chai F., Hampton J. (2003). Modelling Climate-Related Variability of Tuna Populations From a Coupled Ocean-Biogeochemical-Populations Dynamics Model. Fish. Oceanogr. 12, 483–494. doi: 10.1046/j.1365-2419.2003.00244.x
Lehodey P., Conchon A., Senina I., Domokos R., Calmettes B., Jouanno J., et al. (2015b). Optimization of a Micronekton Model With Acoustic Data. ICES J. Mar. Sci. 72, 1399–1412. doi: 10.1093/icesjms/fsu233
Lehodey P., Hampton J., Brill R. W., Nicol S., Senina I., Calmettes B., et al. (2011). “Vulnerability of Oceanic Fisheries in the Tropical Pacific to Climate Change,” in Vulnerability of Tropical Pacific Fisheries and Aquaculture to Climate Change. Eds. Bell J., Johnson J. E., Hobday A. J. (Noumea: Secretariat of the Pacific Community), 433–492.
Lehodey P., Murtugudde R., Senina I. (2010a). Bridging the Gap From Ocean Models to Population Dynamics of Large Marine Predators: A Model of Midtrophic Functional Groups. Prog. Oceanogr. 84, 69–84. doi: 10.1016/j.pocean.2009.09.008
Lehodey P., Senina I., Calmettes B., Hampton J., Nicol S. (2013). Modelling the Impact of Climate Change on Pacific Skipjack Tuna Population and Fisheries. Clim. Change. 119, 95–109. doi: 10.1007/s10584-012-0595-1
Lehodey P., Senina I., Murtugudde R. (2008). A Spatial Ecosystem And Populations Dynamics Model (SEAPODYM) - Modelling of Tuna and Tuna-Like Populations. Prog. Oceanogr. 78, 304–318. doi: 10.1016/j.pocean.2008.06.004
Lehodey P., Senina I., Nicol S., Hampton J. (2015a). Modelling the Impact of Climate Change on South Pacific Albacore Tuna. Deep Sea Res. Part II, 113, 246–259. doi: 10.1016/j.dsr2.2014.10.028
Lehodey P., Senina I., Sibert J., Bopp L., Calmettes B., Hampton J., et al. (2010b). Preliminary Forecasts of Population Trends for Pacific Bigeye Tuna Under the A2 IPCC Scenario. Prog. Oceanogr. 86, 302–315. doi: 10.1016/j.pocean.2010.04.021
Leroy B., Peatman T., Usu T., Caillot S., Moore B., Williams A., et al. (2016). Interactions Between Artisanal and Industrial Tuna Fisheries: Insights From a Decade of Tagging Experiments. Mar. Pol. 65, 11–19. doi: 10.1016/j.marpol.2015.12.001
Li G., Jian Y., Yang S., Du Y., Wang Z., Li Z., et al. (2019). Effect of Excessive Equatorial Pacific Cold Tongue Bias on the El Niño-Northwest Pacific Summer Monsoon Relationship in CMIP5 Multi-Model Ensemble. Clim. Dyn. 52, 6195–6212. doi: 10.1007/s00382-018-4504-9
Margulies D., Scholey V., Wexler J., Stein M. (2016). “Chapter 5 Research on the Reproductive Biology and Early Life History of Yellowfin Tuna Thunnus albacares in Panama,” in Advances in Tuna Aquaculture. Eds. Benetti D. D., Partridge G. J., Buentello A. (Cambridge: Academic Press), 77–114.
Matear R. J., Chamberlain M. A., Sun C., Feng M. (2015). Climate Change Projection for the Western Tropical Pacific Ocean Using a High-Resolution Ocean Model: Implications for Tuna Fisheries. Deep Sea Res. Part II 113, 22–46. doi: 10.1016/j.dsr2.2014.07.003
McKenna S., Santoso A., Gupta A. S., Taschetto A. S., Cai W. (2020). Indian Ocean Dipole in CMIP5 and CMIP6: Characteristics, Biases, and Links to ENSO. Sci. Rep. 10, 11500. doi: 10.1038/s41598-020-68268-9
McMahon S. J., Parsons D., Donelson J. M., Pether S. J. M., Munday P. L. (2020). Elevated CO2 and Heatwave Conditions Affect the Aerobic and Swimming Performance of Juvenile Australasian Snapper. Mar. Biol. 167, 1–12. doi: 10.1007/s00227-019-3614-1
McNeil B. I., Matsumoto K. (2019). “The Changing Ocean and Freshwater CO2 System,” in Fish Physiology Volume 37: Carbon Dioxide. Eds. Grosell M., Munday P. L., Farrell A. P., Brauner C. J. (Cambridge USA: Academic Press), 1–32.
McNeil B., Sasse T. (2016). Future Ocean Hypercapnia Driven by Anthropogenic Amplification of the Natural CO2 Cycle. Nature 529, 383–386. doi: 10.1038/nature16156
Meehl G. A. (1995). Global Coupled General Circulation Models. Bull. Amer. Meteor. Soc 76, 951–957. doi: 10.1175/1520-0477-76.6.951
Meehl G. A., Boer G. J., Covey C., Latif M., Stouffer R. J. (2000). The Coupled Model Intercomparison Project (CMIP). Bull. Amer. Meteor. Soc 81, 313–318. doi: 10.1175/1520-0477(2000)081<0313:TCMIPC>2.3.CO;2
Monteiro F. M., Bach L. T., Brownlee C., Brown P., Rickaby R. E. M., Poulton A. J., et al. (2016). Why Marine Phytoplankton Calcify. Sci. Adv. 2, e150182. doi: 10.1126/sciadv.1501822
Morgan R., Finnøen M. H., Jensen H., Pélabon C., Jutfelt F. (2020). Low Potential for Evolutionary Rescue From Climate Change in a Tropical Fish. Proc. Natl. Acad. Sci. U. S. A. 117, 33365–33372. doi: 10.1073/pnas.2011419117
Munday P. L., Donelson J. M., Domingos J. A. (2017). Potential for Adaptation to Climate Change in a Coral Reef Fish. Glob. Change Biol. 23, 307–317. doi: 10.1111/gcb.13419
Munday P. L., Warner R. R., Monro K., Pandolfi J. M., Marshall D. J. (2013). Predicting Evolutionary Responses to Climate Change in the Sea. Ecol. Lett. 16, 1488–1500. doi: 10.1111/ele.12185
Nagelkerken I., Connell S. D. (2015). Global Alteration of Ocean Ecosystem Functioning Due to Increasing Human CO2 Emissions. Proc. Natl. Acad. Sci. U. S. A. 112, 13272–13277. doi: 10.1073/pnas.1510856112
OFP (2021). Estimates of Annual Catches in the WCPFC Statistical Area. WCPFC-SC17-2021/ST IP-1 (Pohnpei: Western and Central Pacific Fisheries Commission). Available at: https://www.wcpfc.int/meetings/sc17-2021.
Onitsuka T., Takami H., Muraoka D., Matsumoto Y., Nakatsubo A., Kimura R., et al. (2018). Effects of Ocean Acidification With Pco2 Diurnal Fluctuations on Survival and Larval Shell Formation of Ezo Abalone, Haliotis Discus Hannai. Mar. Environ. Res. 134, 28–36. doi: 10.1016/j.marenvres.2017.12.015
Orr J. C., Fabry V. J., Aumont O., Bopp L., Doney S. C., Feely R. A., et al. (2005). Anthropogenic Ocean Acidification Over the Twenty-First Century and its Impact on Calcifying Organisms. Nature 437, 681–686. doi: 10.1038/nature04095
Pauwels S., Fache E. (Eds.) (2016). Fisheries in the Pacific: The Challenges of Governance and Sustainability (Marseille, France: pacific-credo Publications). doi: 10.4000/books.pacific.395
Pecl G. T., Araujo M. B., Bell J., Blanchard J., Bonebrake T. C., Chen I., et al. (2017). Biodiversity Redistribution Under Climate Change: Impacts on Ecosystems and Human Well-Being. Science 355, 1–9. doi: 10.1126/science.aai9214
Pimentel M., Pegado M., Repolho T., Rosa R. (2014). Impact of Ocean Acidification in the Metabolism and Swimming Behavior of the Dolphinfish (Coryphaena Hippurus) Early Larvae. Mar. Biol. 161, 725–729. doi: 10.1007/s00227-013-2365-7
Poloczanska E. S., Brown C. J., Sydeman W. J., Kiessling W., Schoeman D. S., Moore P. J., et al. (2013). Global Imprint of Climate Change on Marine Life. Nat. Clim. Change 3, 919–925. doi: 10.1038/nclimate1958
Pörtner H. O., Karl D. M., Boyd P. W., Cheung W., Lluch-Cota S., Nojiri Y., et al. (2014). “Ocean Systems,” in Climate Change 2014: Impacts, Adaptation and Vulnerability. Contribution of Working Group II to the Fifth Assessment Report of the Intergovernmental Panel on Climate Change. Eds. Field C. B., Barros V. R., Dokken D. J., Mach K. J., Mastrandrea M. D., Bilir T. E., et al. (Cambridge: Cambridge University Press), 411–484.
Pörtner H. O., Roberts D. C., Masson-Delmotte V., Zhai P., Tignor M., Poloczanska E., et al (2019). “Technical Summary,” in IPCC Special Report on the Ocean and Cryosphere in a Changing Climate. Eds. Pörtner H. O., Roberts D. C., Masson-Delmotte V., Zhai P., Tignor M., Poloczanska E., et al. (Cambridge: Cambridge University Press) pp. 39–69. Available at: https://www.ipcc.ch/srocc/.
Receveur A., Dutheil C., Gorgues T., Menkes C., Lengaigne M., Nicol S., et al. (2021). Exploring the Future of the Coral Sea Micronekton. Prog. Oceanogr. 195, 102593. doi: 10.1016/j.pocean.2021.102593
Remolà A. O., Gudmundsson A. (2018). Global Review of Safety at Sea in the Fisheries Sector. FAO Fisheries and Aquaculture Circular No. 1153 (Rome, Italy: Food and Agriculture Organization of the United Nations).
Reusch T. B. H. (2014). Climate Change in the Oceans: Evolutionary Versus Phenotypically Plastic Responses of Marine Animals and Plants. Evol. Appl. 7, 104–122. doi: 10.1111/eva.12109
Rice J. C., Garcia S. M. (2011). Fisheries, Food Security, Climate Change, and Biodiversity: Characteristics of the Sector and Perspectives on Emerging Issues. ICES J. Mar. Sci. 68, 1343–1353. doi: 10.1093/icesjms/fsr041
Richardson A. J., Bakun A., Hays G. C., Gibbons M. J. (2009). The Jellyfish Joyride: Causes, Consequences and Management Responses to a More Gelatinous Future. Trends Ecol. Evol. 24, 312–322. doi: 10.1016/j.tree.2009.01.010
Ryu T., Veilleux H. D., Donelson J. M., Munday P. L., Ravasi T. (2018). The Epigenetic Landscape of Transgenerational Acclimation to Ocean Warming. Nat. Clim. Change 8, 504–509. doi: 10.1038/s41558-018-0159-0
Salinger M. J. (2013). A Brief Introduction to the Issue of Climate and Marine Fisheries. Clim. Change 119, 23–35. doi: 10.1007/s10584-013-0762-z
Salinger M. J., Bell J. D., Evans K., Hobday A. J., Allain V., Brander K., et al. (2013). Climate and Oceanic Fisheries: Recent Observations and Projections and Future Needs. Clim. Change. 119, 213–221. doi: 10.1007/s10584-012-0652-9
Samuels M., Adam O., Gildor H. (2021). A Shallow Thermocline Bias in the Southern Tropical Pacific in CMIP5/6 Models Linked to Double-ITCZ Bias. Geophys. Res. Lett. 48, e2021GL093818. doi: 10.1029/2021GL093818
Schmidtko S., Stramma L., Visbeck M. (2017). Decline in Global Oceanic Oxygen Content During the Past Five Decades. Nature 542, 335–339. doi: 10.1038/nature21399
Semenov M. A., Stratonovitch P. (2010). Use of Multi-Model Ensembles From Global Climatemodels for Assessment of Climate Change Impacts. Clim. Res. 41, 1–14. doi: 10.3354/cr00836
Senina I., Lehodey P., Calmettes B., Dessert M., Hampton J., Smith N., et al. (2018). Working Paper WCPFC-SC14-2018/EB-WP-01: Impact of Climate Change on Tropical Pacific Tuna and Their Fisheries in Pacific Islands Waters and High Seas Areas (Pohnpei: Western and Central Pacific Fisheries Commission). Available at: https://meetings.wcpfc.int/node/10666.
Senina I., Lehodey P., Calmettes B., Nicol S., Caillot S., Hampton J., et al. (2015). Information Paper WCPFC-SC11-2015/EB-IP-01: SEAPODYM Application for Yellowfin Tuna in the Pacific Ocean (Pohnpei: Western and Central Pacific Fisheries Commission). Available at: https://www.wcpfc.int/meetings/11th-regular-session-scientific-committee.
Senina I., Lehodey P., Hampton J., Sibert J. (2020a). Quantitative Modeling of the Spatial Dynamics of South Pacific and Atlantic Albacore Tuna Populations. Deep Sea Res. Part II 175, 104667. doi: 10.1016/j.dsr2.2019.104667
Senina I., Lehodey P., Sibert J., Hampton J. (2020b). Integrating Tagging and Fisheries Data Into a Spatial Population Dynamics Model to Improve its Predictive Skills. Can. J. Fish. Aquat. Sci. 77, 576–593. doi: 10.1139/cjfas-2018-0470
Senina I., Sibert J., Lehodey P. (2008). Parameter Estimation for Basin-Scale Ecosystem-Linked Population Models of Large Pelagic Predators: Application to Skipjack Tuna. Prog. Oceanogr. 78, 319–335. doi: 10.1016/j.pocean.2008.06.003
Senina I., Titaud O., Briand G., Lehodey L. (2022). SEAPODYM (Spatial Ecosystem and POpulation DYnamics Model for Migratory Age-Structured Stocks). User Manual Version 4 (Noumea, New Caledonia: Pacific Community).
Sswat M., Stiasny M. H., Taucher J., Algueró-Muñiz M., Bach L. T., Jutfelt F., et al. (2018). Food Web Changes Under Ocean Acidification Promote Herring Larvae Survival. Nat. Ecol. Evol. 2, 836–840. doi: 10.1038/s41559-018-0514-6
Steinacher M., Joos F., Frolicher T. L., Bopp L., Cadule P., Cocco V., et al. (2010). Projected 21st Century Decrease in Marine Productivity: A Multi-Model Analysis. Biogeosciences 7, 979–1005. doi: 10.5194/bg-7-979-2010
Stiasny M. H., Mittermayer F. H., Sswat M., Voss R., Jutfelt F., Chierici M., et al. (2016). Ocean Acidification Effects on Atlantic Cod Larval Survival and Recruitment to the Fished Population. PloS One 11, e0155448. doi: 10.1371/journal.pone.0155448
Stiasny M. H., Sswat M., Mittermayer F. H., Falk-Petersen I.-B., Schnell N. K., Puvanendran V., et al. (2019). Divergent Responses of Atlantic Cod to Ocean Acidification and Food Limitation. Glob. Change Biol. 25, 839–849. doi: 10.1111/gcb.14554
Sunday J. M., Calosi P., Dupont S., Munday P. L., Stillman J. H., Reusch T. B. (2014). Evolution in an Acidifying Ocean. Trends Ecol. Evol. 29, 117–125. doi: 10.1016/j.tree.2013.11.001
Sunday J. M., Fabricius K. E., Kroeker K. J., Anderson K. M., Brown N. E., Barry J. P., et al. (2017). Ocean Acidification can Mediate Biodiversity Shifts by Changing Biogenic Habitat. Nat. Clim. Change 7, 81–85. doi: 10.1038/nclimate3161
Tang T., Luo J.-J., Peng K., Qi L., Tang S. (2021). Over-Projected Pacific Warming and Extreme El Niño Frequency Due to CMIP5 Common Biases. Natl. Sci. Rev. 8, nwab056. doi: 10.1093/nsr/nwab056
Tian B., Dong X. (2020). The Double-ITCZ Bias in CMIP3, CMIP5 and CMIP6 Models Based on Annual Mean Precipitation. Geophys. Res. Lett. 47, e2020GL087232. doi: 10.1029/2020GL087232
Tittensor D. P., Eddy T. D., Lotze H. K., Galbraith E. D., Cheung W., Barange M., et al. (2018). A Protocol for the Intercomparison of Marine Fishery and Ecosystem Models: Fish-MIP V1.0. Geosci. Model Dev. 11, 1421–1442. doi: 10.5194/gmd-11-1421-2018
Tittensor D. P., Novaglio C., Harrison C. S., Heneghan R. F., Barrier N., Bianchi D., et al. (2021). Next-Generation Ensemble Projections Reveal Higher Climate Risks for Marine Ecosystems. Nat. Clim. Change 11, 973–981. doi: 10.1038/s41558-021-01173-9
Tortell P. D., Payne C. D., Li Y., Trimborn S., Rost B., Smith W. O., et al. (2008). CO2 Sensitivity of Southern Ocean Phytoplankton. Geophys. Res. Lett. 35, L04605. doi: 10.1029/2007GL032583
Tremblay-Boyer L. (2013). Potential for Interactions Between the Industrial and Artisanal Fleets in French Polynesia. Technical Report (Noumea, New Caledonia: Secretariat for the Pacific Communities). 59pp.
Uthicke S., Momigliano P., Fabricius K. E. (2013). High Risk of Extinction of Benthic Foraminifera in This Century Due to Ocean Acidification. Sci. Rep. 3, 1769. doi: 10.1038/srep01769
Valenzuela J. J., López García de Lomana A., Lee A., Armbrust E. V., Orellana M. V., Baliga N. S. (2018). Ocean Acidification Conditions Increase Resilience of Marine Diatoms. Nat. Commun. 9, 2328. doi: 10.1038/s41467-018-04742-3
Watson S.-A., Allan B. J. M., McQueen D. E., Nicol S., Parsons D. M., Pether S. M. J., et al. (2018). Ocean Warming has a Greater Effect Than Acidification on the Early Life History Development and Swimming Performance of a Large Circumglobal Pelagic Fish. Glob. Change Biol. 24, 4368–4385. doi: 10.1111/gcb.14290
WCPFC (2020) Part 1 Annual Reports of Members of the Western and Central Pacific Fisheries Commission. Available at: https://meetings.wcpfc.int/meetings/type/11.
Wexler J. B., Margulies D., Scholey V., Lennert-Cody C. E., Bromhead D., Nicol S., et al. (2021). The Effect of Ocean Acidification on Otolith Morphology in Larvae of a Tropical, Epipelagic Fish Species, Yellowfin Tuna (Thunnus Albacares) (La Jolla: IATTC internal document). Available at:ZG1hcmd1bGllc0BpYXR0Yy5vcmc=.
Zaiss J., Boyd P. W., Doney S. C., Havenhand J. N., Levine N. M. (2021). Impact of Lagrangian Sea Surface Temperature Variability on Southern Ocean Phytoplankton Community Growth Rates. Global Biogeochem. Cycles 35, e2020GB006880. doi: 10.1029/2020GB006880
Keywords: SEAPODYM, climate change, greenhouse gas, Pacific Ocean, fisheries
Citation: Nicol S, Lehodey P, Senina I, Bromhead D, Frommel AY, Hampton J, Havenhand J, Margulies D, Munday PL, Scholey V, Williamson JE and Smith N (2022) Ocean Futures for the World’s Largest Yellowfin Tuna Population Under the Combined Effects of Ocean Warming and Acidification. Front. Mar. Sci. 9:816772. doi: 10.3389/fmars.2022.816772
Received: 17 November 2021; Accepted: 22 March 2022;
Published: 22 April 2022.
Edited by:
Kenneth Alan Rose, University of Maryland Center for Environmental Science (UMCES), United StatesReviewed by:
John Patrick Dunne, Geophysical Fluid Dynamics Laboratory (GFDL), United StatesSibylle Dueri, Institut National de recherche pour l’agriculture, l’alimentation et l’environnement (INRAE), France
Copyright © 2022 Nicol, Lehodey, Senina, Bromhead, Frommel, Hampton, Havenhand, Margulies, Munday, Scholey, Williamson and Smith. This is an open-access article distributed under the terms of the Creative Commons Attribution License (CC BY). The use, distribution or reproduction in other forums is permitted, provided the original author(s) and the copyright owner(s) are credited and that the original publication in this journal is cited, in accordance with accepted academic practice. No use, distribution or reproduction is permitted which does not comply with these terms.
*Correspondence: Simon Nicol, c2ltb25uQHNwYy5pbnQ=
†These authors have contributed equally to this work and share first authorship