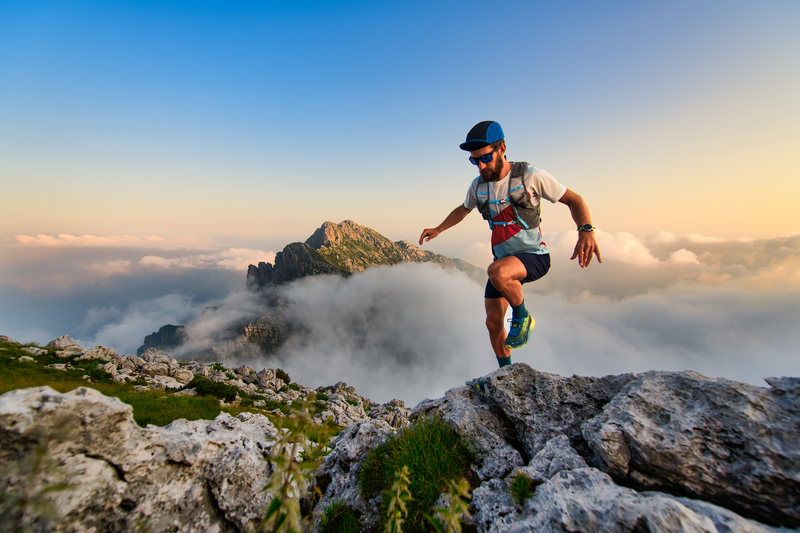
94% of researchers rate our articles as excellent or good
Learn more about the work of our research integrity team to safeguard the quality of each article we publish.
Find out more
ORIGINAL RESEARCH article
Front. Mar. Sci. , 10 March 2022
Sec. Aquatic Microbiology
Volume 9 - 2022 | https://doi.org/10.3389/fmars.2022.782456
Benthic sediment bacteria are important drivers for material circulation and energy flow in aquatic ecosystem, and they are sensitive to environmental changes. Large scale suspended mariculture in coastal waters induces high organic loading to the sediment through biodeposition, and causes changes in sediment biogeochemical features. However, the impacts of mariculture on sediment bacterial communities are not fully understood. In the present study, sediment samples were collected from three coastal semi-enclosed bays of China, i.e., Sanggou Bay, Daya Bay, and Maniao Bay, where large scale mariculture were carried out since the 1980s. High-throughput sequencing was used to examine the spatial and seasonal variations of bacterial communities. The results indicated that the dominant phyla of three bays were Proteobacteria (39.18–47.21%), Bacteroidetes (9.91–19.25%), and Planctomycetes (7.12–13.88%). Spatial variations played a greater role in shaping the bacterial communities than seasonal variation. The bacterial diversity indices (Chao1, Pielou’s evenness, and Shannon-Wiener index) of Sanggou Bay were significantly lower than those of Daya Bay and Maniao Bay. For seasonal variation, bacterial diversity indices in spring were significantly lower than that in autumn. Five keystone taxa belonging to Planctomycetes, Alphaproteobacteria, and Acidobacteria were identified in Sanggou Bay. Temperature, particulate organic carbon, pH, and salinity were the most important environmental factors shaping the spatial and seasonal variations of bacterial communities in the studied areas. The abundances of bacteria, particularly Bacteroidetes, Gammaproteobacteria, and Deltaproteobacteria, were significantly correlated with the mariculture-driven chemical properties of the sediment. These results indicated that intensive mariculture could induce profound and diverse impacts on the bacterial communities, and therefore modify their role in marine ecosystem. The interactions between mariculture and sediment bacterial communities should be considered in regard to mariculture management and carrying capacity.
Bacteria are the most abundant and diverse organisms in marine ecosystems. They are major drivers of biogeochemical cycles in aquatic ecosystems, and facilitate the cycling of organic matter and nutrients by maintaining the marine ecosystem’s health, balance, and ability of recovering from degradation (DeLong, 1992; Pedrós-Alió, 2006). Their essential role in organic matter decomposition and recycling has been well recognized (Faulwetter et al., 2013; Ibekwe et al., 2016). The community composition, biomass, and activity of these organisms have been shown to critically influence carbon fluxes of marine ecosystems (Mou et al., 2008; Xia et al., 2015).
With increased understanding of the bacterial importance in energy and organic matter cycling, knowledge of the structure and diversity of bacterial communities has become essential for understanding the relationship between ecosystem functions and the environment (Kolukirik et al., 2011; Olsen et al., 2017). Various environmental factors (salinity, temperature, organic matter, etc.) in marine environments have been shown to influence several aspects of bacterial communities such as abundance, activity, and exoenzyme profiles (Hou et al., 2017; Türetken, 2021). Large scale suspended aquaculture, e.g., floating cage farming of fish, longline culture of bivalves (e.g., oyster, mussel, and scallop, etc.), and seaweeds (e.g., kelp Saccharina spp. and Gracilaria spp.), in coastal waters have rapidly developed in many regions of the world, particularly in southeast Asia, like in China, where intensive aquaculture has been carried out since 1980s. Intensive mariculture induce high organic loading to the sediment through biodeposition (Gray and Langdon, 2019). The organic enrichment has various influences on sediment biogeochemical features, and could be an important aspect for the bacterial community structure and sediment metabolic capacity.
To date, most of the studies investigating the spatial variation of bacterial communities have been focused on a certain region, e.g., in one bay (Leon et al., 2017; Olsen et al., 2017; Zhu et al., 2018) or in one river (Ibekwe et al., 2016; Jordaan and Bezuidenhout, 2016; Zhang et al., 2019). However, if we want a more comprehensive understanding on the spatial distribution of bacterial communities and their relationship with environmental factors, the specific existing regional data is insufficient. Studies across a relatively larger spatial scale are needed. Comparison of different regions may provide a broader view and better understanding (Kolukirik et al., 2011; Beaulne et al., 2020; Yi et al., 2021). Therefore, in the present study, we investigated the spatial distribution characteristics of bacterial communities in three mariculture bays, i.e., Sanggou Bay (in the Yellow Sea, northern China, a temperate zone), and Daya Bay and Maniao Bay (in the South China Sea, southern China, subtropical and tropical zones).
High-throughput sequencing was used to examine the temporal and spatial variation of bacteria in sediments from the three bays. The objectives were: (1) to investigate the seasonal patterns of the bacteria inhabiting the sediment of Sanggou Bay and (2) to examine the spatial variation and key environmental factors driving the spatial heterogeneities of bacteria in sediments of the three bays. We aimed to obtain useful information for further understanding about the mariculture and environment interactions, and provide implications for mariculture management in coastal waters.
Sediment samples were collected from the three mariculture bays of Sanggou Bay, Daya Bay, and Maniao Bay. Sanggou Bay is a typical semi-enclosed bay in the northern part of the Yellow Sea, located on the eastern side of Shandong Peninsula. Sanggou Bay covers an area of 150.3 km2, with an average water depth of 7.5 m. The minimum sea surface temperature of Sanggou Bay occurs in winter (1.8°C), and the maximum in summer (23.3°C). In the bay, suspended cultures of kelp (Saccharina japonica), bivalves, e.g., oyster (Crassostrea gigas), and scallops (Chlamys farreri and Mizuhopecten yessoensis) have been established on a large scale from the 1980s (see Jiang et al. (2015), Zhu et al. (2017), Sui et al. (2020) for more detailed information). Daya Bay is a 650 km2 semi-enclosed subtropical embayment, located in the northeast South China Sea. The minimum sea surface temperature of Daya Bay occurs in winter (15°C), and the maximum in summer and autumn (30°C) [see Wang et al. (2006) for more detailed information]. Maniao Bay is located in northern Hainan Province. The minimum sea surface temperature of the bay occurs in autumn and winter (22.5°C), and the maximum in summer (32.5°C). Suspended fish cage cultures have been established in Daya Bay and Maniao Bay, the famed fish species include Paralichthys olivaceus, Trachinotus ovatus, and Lutjanus erythropterus [for more detailed information see Qi et al. (2019)]. These three bays were chosen because the seasonal differences in temperature in Sanggou Bay made it suitable for comparing the seasonal variation in the bacterial community, and the distances among the three bays could provide a spatial comparison.
Surface sediments were collected using an Ekman-Birge type bottom sampler in spring (April 2020), summer (July 2020), autumn (October 2020), and winter (December 2020) from 21 stations in Sanggou Bay (Figure 1). Samples from Daya Bay and Maniao Bay were obtained in summer (August and July 2020, respectively) from 15 stations in each bay.
Triplicate sediment samples were collected from each site. Samples were gently placed on a white enamel tray (30 cm × 60 cm), and the top 2 cm of sediment was collected and blended using a stainless-steel ruler. Sediment samples were homogenized and further separated into two subsamples: one was stored at −80°C for DNA extraction, and another was stored at −20°C for physical and chemical analyses. During the sampling period, the temperature, pH, dissolved oxygen (DO), and salinity of the water were measured with a multiprobe sonde (Yellow Springs Instrument Co., Dayton, OH, United States).
For particulate organic carbon (POC) and particulate organic nitrogen (PON) analysis, sediment samples (∼6 g) were weighed and then dried at 60°C for 24 h until a constant weight was obtained. After that, samples were homogenized and ground to fine powder in an agate ball mill, and were immersed into 30 mL HCl (1M) and oscillated for 2 h to remove carbonates. Solid samples were then rinsed with deionized water for several times until the pH of washing fluid became neutral. After drying at 60°C for 24 h, the samples were ground for POC and PON analysis using elementary analyzer (EuroVector, Italy).
Sediment samples (∼100 g) were centrifuged at 3,000 × g for 10 min, and supernatant was then filtered through membrane (0.45 μm, 47 mm). The NO3–, NO2–, NH4+ and PO43– concentrations of porewater from the sediments were measured according to marine monitoring specifications method (GB/T 12763.4-2007, 2007) with a spectrophotometer (Shanghai Precision Science Instrument Co., Ltd., Shanghai, China).
Samples (0.25 g, wet weight) were used for DNA extraction with the E.Z.N.A.® soil DNA Kit (Omega Bio-Tek, Norcross, GA, United States) following the manufacturer’s instructions. The DNA concentration was determined by NanoDrop 2000 UV-vis spectrophotometer (Thermo Fisher Scientific, Wilmington, MA, United States), and DNA quality was checked by 1% agarose gel electrophoresis. The V4-V5 hypervariable regions of the bacterial 16S rRNA gene were amplified with the universal bacterial primers 515F (5′-GTGYCAGCMGCCGCGGTAA-3′) and 926R (5′-CCGYCAATTYMTTTRAGTTT-3′) by a GeneAmp 9700 (ABI, United States) thermocycler (Parada et al., 2015). PCR reactions were performed in triplicate 20 μL mixture containing 4 μL of 5 × FastPfu Buffer, 2 μL of 2.5 mM dNTPs, 0.8 μL of each primer (5 μM), 0.4 μL of FastPfu Polymerase, 0.2 μL of BSA and 10 ng of template DNA. The PCR reactions were conducted using the following program: 3 min of denaturation at 95°C, 30 cycles of 30 s at 95°C, 30 s for annealing at 53°C, and 45 s for elongation at 72°C, and a final extension at 72°C for 10 min. The resulted PCR products were extracted from a 2% agarose gel and further purified using the AxyPrep DNA Gel Extraction Kit (Axygen Biosciences, Union City, CA, United States) and quantified using QuantiFluor™-ST (Promega, United States) according to the manufacturer’s protocol. Purified amplicons were pooled in equimolar and paired-end sequenced (2 × 300) on an Illumina MiSeq platform (Illumina, San Diego, CA, United States) according to the standard protocols by Shanghai Majorbio Bio-Pharm Technology Co., Ltd. (Shanghai, China). All the sequences used in this study are publicly available at the NCBI Sequence Read Archive1 under BioProject accession number PRJNA734373.
The raw 16S rRNA gene sequencing reads were demultiplexed, quality-filtered by fastp version 0.20.0 (Chen et al., 2018) and merged by FLASH version 1.2.7 (Magoč and Salzberg, 2011) with the following criteria: (i) the 300 bp reads were truncated at any site receiving an average quality score of <20 over a 50 bp sliding window, and the truncated reads shorter than 50 bp were discarded, reads containing ambiguous characters were also discarded; (ii) only overlapping sequences longer than 10 bp were assembled according to their overlapped sequence. The maximum mismatch ratio of overlap region is 0.2. Reads that could not be assembled were discarded; (iii) samples were distinguished according to the barcode and primers, and the sequence direction was adjusted, exact barcode matching, 2 nucleotide mismatch in primer matching. Operational taxonomic units (OTUs) with 97% similarity cutoff were clustered using UPARSE version 7.1 (Edgar, 2013), and chimeric sequences were identified and removed. The sequences of samples were rarefied based on the minimum sequence (20393) to standardize uneven sequence depth, and the taxonomy of each OTU representative sequence was analyzed by RDP Classifier version 2.2 against the 16S rRNA database (Silva v138) using confidence threshold of 0.7 (Wang et al., 2007).
Statistical analyses were performed using R software (R Core Team, 2018). Three diversity indices including Chao1 which is referred as to estimator of species richness based on abundance, Pielou’s evenness which is referred as to equitability of species and Shannon-Wiener indices which is referred as to estimator of both species richness and evenness, were calculated by mothur (Schloss et al., 2009). Multiple Comparisons of Alpha diversity indices were generated by the package “multcomp” of R (Hothorn et al., 2008). Non-metric multidimensional scaling (NMDS) of the bacterial community structure was run in package “vegan” of R based on Bray-Curtis distance and followed by ANOSIM with 999 permutations (Oksanen et al., 2020). NMDS was used to visualize the beta diversity of the bacterial communities among the bays and across seasons and ANOSIM was used to compare the difference between and within groups. Correlation coefficients between environmental factors and bacterial α-diversity indices, dominant bacterial lineages in three bays were visualized in heatmaps generated by package “pheatmap” of R (Kolde, 2019). The influence of environmental factors on bacterial community structure from sediments was shown in RDA generated by package “vegan” of R, and in order to find a reduced form model and to avoid collinearity among environmental factors, forward selection was used to select a parsimonious set of significant environmental variables based on permutation test. Co-occurrence network were conducted by the packages of “psych” and “igraph” in R software and based on the OTUs level with mean proportions >0.01% in all samples to simplify the network (Csardi and Nepusz, 2006; Revelle, 2021). Co-occurrence pairs with a Spearman’s correlation coefficient >0.7 or <−0.7 and a p-value < 0.01 (Benjamini and Hochberg adjusted) were considered as a valid co-occurrence event (Benjamini et al., 2006; Ma et al., 2016). Network topological features including degree (the number of adjacent edges) and betweenness centrality (the number of shortest paths going through a node) for each node in the network were calculated with package “igraph” in R software. Nodes with high degree and low betweenness centrality values are recognized as keystone species in co-occurrence networks (Berry and Widder, 2014; Banerjee et al., 2018; Tian et al., 2020). The difference significance tests (ANOVA) of bacterial distribution and adjusted p-values (Benjamini and Hochberg false discovery rate) were calculated using STAMP (Parks et al., 2014). In addition, a two-way ANOVA analysis was applied to compare the variances among environmental factors by package “multcomp” of R.
A two-way analysis of variance revealed that seasonal variation had significant effects on the temperature, salinity, DO, NO2–, NO3–, NH4+, and POC in Sanggou Bay (p < 0.05, Table 1). The temperature varied significantly among the four seasons; however, DO and POC values in spring were significantly higher than those in autumn and winter (p < 0.05). The NO2– and NO3– contents in spring and winter were higher than those in summer and autumn, but the NH4+ content was lowest in spring.
Temperature, salinity, pH, PO43–, PON, and POC were significantly different among three bays (p < 0.05). The temperatures in Daya Bay and Maniao Bay were significantly higher than that in Sanggou Bay, the pH and PO43– were highest in Daya Bay, but the salinity in Sanggou Bay was significantly higher (p < 0.05). In addition, PON and POC contents were significantly higher in Daya Bay, followed, in descending order, by Maniao Bay and Sanggou Bay (p < 0.05).
The 16S rRNA sequences obtained from sediment samples were clustered into 18,085 OTUs, which belong to 63 different phyla. The dominant phyla of the three bays were similar and included Proteobacteria (relative abundance ranged from 39.18 to 47.21% for the six groups), Bacteroidetes (9.91–19.25%), and Planctomycetes (7.12–13.88%), and the identified members of Proteobacteria mainly belonged to Gammaproteobacteria, Deltaproteobacteria, and Alphaproteobacteria (Supplementary Figure 1 and Supplementary Table 1).
In Sanggou Bay, the abundances of Planctomycetes, Acidobacteria, Chloroflexi, Firmicutes, Nitrospinae, and Actinobacteria were generally lower in spring and then slightly increased thereafter (p < 0.01). On the other hand, the abundances of Gammaproteobacteria, Bacteroidetes, Verrucomicrobia, and Cyanobacteria showed an inverse trend. The abundances of Deltaproteobacteria, Kiritimatiellaeota, Latescibacteria, Schekmanbacteria, Spirochaetes, and Calditrichaeota were highest in summer, significantly higher than the other seasons (p < 0.01, Figure 2). In summer, the abundances of Planctomycetes, Acidobacteria, Alphaproteobacteria, Actinobacteria, and Nitrospirae were significantly higher in Maniao Bay than the two other bays, especially the abundances of Alphaproteobacteria and Nitrospirae which were significantly higher in Maniao Bay than Daya Bay (p < 0.01). Whereas, the abundances of Gammaproteobacteria, Bacteroidetes, Verrucomicrobia, Kiritimatiellaeota, Schekmanbacteria, and Deinococcus Thermus were highest in Sanggou Bay. The abundances of Deltaproteobacteria Chloroflexi, Latescibacteria, Firmicutes, Cyanobacteria, Spirochaetes, Calditrichaeota, Patescibacteria, Zixibacteria, and Nitrospinae were significantly higher in Daya Bay than in the two other bays (p < 0.01, Figure 3).
Figure 2. Boxplots of top 20 phyla and the dominant classes of Proteobacteria in all samples whose proportions were significantly different among the four seasons of Sanggou Bay (adjusted p < 0.01). The “star” and “+” represented the mean value and abnormal value, respectively. The abnormal values refer to outliers of the boxplot.
Figure 3. Boxplots of top 20 phyla and the dominant classes of Proteobacteria in all samples whose proportions were significantly different among Sanggou Bay, Daya Bay, and Maniao Bay in the summer (adjusted p < 0.01). The “star” and “+” represented the mean value and abnormal value, respectively. The abnormal values refer to outliers of the boxplot.
For seasonal variations in Sanggou Bay, its Chao1 index was lowest in spring and highest in autumn and summer, with a significant difference observed between the spring and autumn (Figure 4A1). The values of Shannon-Wiener index and Pielou’s evenness index were lowest in spring and continuously increased thereafter, with highest values observed in autumn and winter, significantly higher than that in spring (p < 0.05, Figures 4B1,C1).
Figure 4. Boxplot of α-diversity indices on OTUs level (A–C). Data of α-diversity indices in each group with the same letters were not significantly different among four seasons and among three bays (p > 0.05). SSp, spring of Sanggou Bay; SSu, summer of Sanggou Bay; SAu, Autumn of Sanggou Bay; SWi, winter of Sanggou Bay; DSu, summer of Daya Bay; MSu, summer of Maniao Bay.
For spatial variations in summer, the three diversity indices were lowest in Sanggou Bay, and were comparable in Daya Bay and Maniao Bay. The Chao1 index was significantly lower in Sanggou Bay than in Daya Bay (Figure 4A2), while the Shannon-Wiener and Pielou’s evenness indices were significantly lower in Sanggou Bay than in the two other bays (p < 0.05, Figures 4B2,C2).
Spatial distributions of bacterial communities at the OTU level using NMDS were shown in Figure 5A. The results indicated that the bacterial community structure varied gradually throughout the four seasons in Sanggou Bay (Figures 5B,C). There were bigger between-group variance in bacterial community structure among the three bays than among four seasons (0.93 > 0.40), indicated that the differences between bacterial communities were more attributed to spatial separation rather than seasonal variations.
Figure 5. NMDS (A) and ANOSIM plots (B,C) on OTUs level. The gray arrow of (A) represents the variation trend of bacterial community structure from spring to winter of Sanggou Bay. SSp, spring of Sanggou Bay; SSu, summer of Sanggou Bay; SAu, Autumn of Sanggou Bay; SWi, winter of Sanggou Bay; DSu, summer of Daya Bay; MSu, summer of Maniao Bay.
A co-occurrence network was created for bacteria at the OTU level using a Spearman’s coefficient threshold of ±0.7 and a p-value threshold of 0.01. Based on a high degree (>90) and low betweenness centrality (<5,000) in the co-occurrence networks, five OTUs were identified as keystone taxa three of them belong to the Planctomycetes phylum (two Rubripirellula sp., one Blastopirellula sp.), one of them belongs to Alphaproteobacteria, and one belongs to Subgroup 10 of Acidobacteria (Table 2). In addition, the maximal relative abundances of all keystone OTUs were lower than 2% and the max values were all detected in the winter (Table 2).
As exhibited in Supplementary Table 2, six environmental factors were selected to obtain reduced form models of RDA. Temperature, POC, pH, NO3–, salinity, and NH4+ were selected based on p-values < 0.05 to analyze the bacteria distribution of four seasons in Sanggou Bay (Figure 6A). The RDA plot showed that the variances in bacterial community structure in the winter were positively correlated with salinity and NH4+. In addition, temperature, POC, pH, salinity, PO43–, and DO were selected to analyze the bacteria distribution of three regions in summer (Figure 6B). The RDA plot showed that the bacterial communities in Sanggou Bay were positively correlated with salinity and DO, and the bacterial communities in Daya Bay and Maniao Bay were positively correlated with temperature, POC, pH, and PO43–. In summary, temperature, POC, pH, and salinity were the most important environmental factors influencing the spatial and seasonal variations of bacterial communities, but the influences of environmental factors on bacterial spatial variations were stronger than bacterial seasonal variations (Figure 6).
Figure 6. RDA of environmental factors and bacterial community structure from sediments of Sanggou Bay in four seasons (A) and from sediments of Sanggou Bay, Daya Bay, and Maniao Bay in summer (B).
The values of Chao1, Shannon-Wiener, and Pielou’s evenness indices were significantly positively correlated with PON, POC, PO43–, and temperature (Figure 7), but were negatively correlated with DO and salinity (p < 0.01). The abundances of Latescibacterota, Spirochaetes, Calditrichaeota, Deltaproteobacteria, Chloroflexi and Zixibacteria were significantly positively correlated with PON, POC, PO43–, and temperature, but negatively correlated with DO, salinity and NO3– (p < 0.01). The abundances of Gammaproteobacteria, Verrucomicrobia and Alphaproteobacteria were significantly negatively correlated with PON, POC, PO43–, and temperature, but positively correlated with DO and salinity (p < 0.05).
Figure 7. Correlation coefficients between environmental factors and bacterial α-diversity indices (A), between environmental factors and dominant bacterial lineages [phylum and classes for Proteobacteria, (B)] in three bays. * and ** represent significant correlations (p < 0.05) and extremely significant correlations (p < 0.01), respectively. PON, particle organic nitrogen; POC, particle organic carbon; T, temperature; DO, dissolved oxygen.
It has been well demonstrated that extensive suspended culture of fish and/or bivalves has various impacts on the physicochemical of sediments including increased organic loading under and around the farms (Gray and Langdon, 2019; Sanz-Lazaro et al., 2021). It is reasonable that since the mariculture activities and physiological parameters of cultured species vary among seasons, the environment and microbial communities influenced by them would also vary correspondingly. This has been proved by previous studies which showed that sediment microbial communities are subject to seasonal changes (Vetterli et al., 2015; Fu et al., 2020; Yi et al., 2021). Therefore, it is necessary to examine the seasonal variations of microbial communities and the corresponding environmental parameters, so that to reveal the possible impacts of mariculture activities. Furthermore, spatial comparison among mariculture areas is also necessary to achieve an insight into the underlying mechanisms of how mariculture exerts influences on microbial communities.
In the present study, we examined the bacterial communities characteristics in three typical mariculture bays, i.e., Sanggou Bay, Daya Bay, and Maniao Bay, located from Northern to Southern China. The seasonal variations of bacterial communities in Sanggou Bay and their relationship with key environmental factors were analyzed. Bacterial communities among the three bays were also compared. It is possible that the geographical distance may contribute to a geographical isolation of bacteria species and community dissimilarity. Even though, however, the spatial comparison is feasible Firstly, the differentiation in habitat in ocean is much less evident than the terrestrial differentiation, since the ocean current and sea shippings cause mixing, transition and exchange among different seas, the km scale dispersal effects in the oceans should not be an obstacle as on land. Secondly, large scale mariculture activities have been carried out since 1980s in all of the three bays. There are frequently “culture products exchange” among them, such as buying or selling of fish fry, bivalves (e.g., oyster, clam, and scallop, etc.) seedlings, and seaweeds/macroalgae seedlings, between each other. For example, the seedlings of oyster C. gigas, clam Ruditapes philippinarum, and Gracilaria lemaneiformis, etc., cultured in Sanggou Bay and Maniao Bay were bought from Fujian province and Guangdong province (where the Daya Bay located). Another example is the “relay race” culture mode of abalone Haliotis discus hannai Ino. The abalone are cultured in Sanggou Bay during summer and autumn (from June to late October), and then transported to subtropical waters like Fujian province which is adjacent to Guangdong province, and cultured there during winter and spring (from November to May). By doing this, abalone can reach to a commercial market size in a shorter time. It is reasonable that these frequently exchange improved communication and transplantation of bacteria species among these bays, and to some extent, weakened the dispersal limitation. In this regard, the bacterial communities among these three bays are comparable.
In Sanggou Bay, the bacteria abundances did not change drastically among the four seasons (the values were highest in winter, whereas, they were only about 1.5–3 times higher than the lowest values observed in spring). By comparing with Daya Bay and Maniao Bay, the comprehensive results suggested that the bacterial communities were more impacted by spatial factors than by seasonal variations in the present study. The mariculture activities and their influences on the environment among the three study regions are different. For example, POC content in sediment from Daya Bay and Maniao Bay was significantly higher than that in Sanggou Bay (p < 0.05). This is because the main mariculture species are fish in Daya Bay and Maniao Bay, which lead to a much higher POC and PON enrichment on sediment (Qi et al., 2019). On the other hand, the main mariculture species in Sanggou Bay are bivalves, such as scallops and oysters, which are non-feeding species and deposit much fewer particulate matters onto the sediment (Xia et al., 2019). Additionally, the high quantity of farmed seaweeds like kelp in Sanggou Bay acted as biological carbon pump and sequestrated carbon (Jiang et al., 2015). Since carbon and nitrogen are the crucial elements for bacteria, the different POC and PON profile might be a possible reason for the significant differences in bacterial community structure observed among the three bays. The diversity and evenness of bacterial communities in Daya Bay and Maniao Bay were significantly higher than those in Sanggou Bay, probably due to the abundant POC that provided carbon and/or energy to the bacteria (Burton and Johnston, 2010; Xie et al., 2016).
The chemical properties of organic matters in sediment are important factors for bacterial degradation (Koho et al., 2013). The organic matter mineralization rate is usually limited by extracellular enzyme-associated hydrolysis of macromolecules, because their biological structure might too large to be readily transported into bacterial cells (Arnosti and Holmer, 2003; Koho et al., 2013). Under this circumstance, Bacteroidetes have an advantage in organic decomposition. They can hydrolyze insoluble organics into soluble macromolecular organics, and further convert them into fatty acids and alcohols, which are preferential substrates for most microorganisms (Erik et al., 1995; Ibekwe et al., 2016; Jordaan and Bezuidenhout, 2016). Therefore, in this respect, Bacteroidetes have a comparative advantage in sediments with a higher proportion of structural complicated organic maters, which are difficult for degradation by other species. However, for the three bays in the present study, all the suspended culture species, e.g., fish, bivalves and seaweed deposited considerable amount of organic matters to the surface sediments in the mariculture areas. Most of these organic matters are chemical labile and biological degradable for microorganisms. Under this circumstance, Bacteroidetes did not have competitive edge in POC utilization. This could be a possible explanation for why there is no significant negative correlation between the abundance of Bacteroidetes and POC content observed in the present study. The abundance of Bacteroidetes was negatively correlated with temperature, thus compared to Daya Bay and Maniao Bay, which are located in subtropical and tropical regions, respectively, Bacteroidetes were the most abundant in Sanggou Bay.
In regard to Gammaproteobacteria, their abundances showed a similar variation trend with Bacteroidetes, which was negatively correlated with PO43– content in the sediment. This could be due to Gammaproteobacteria species are mostly affiliated with S2– oxidization and prefer surface sediment-harbored O2 or Mn/Fe oxides (Julies Elsabé et al., 2012). When there are sufficient O2 or Mn oxides available, they can oxidize Fe2+ into Fe3+, which could couple with PO43– and precipitate into the sediment. This could explain why the abundance of Gammaproteobacteria was negatively correlated with PO43– content, and positively correlated with DO concentration in all of the three bays. Regarding another dominant class of Proteobacteria, the relative abundance of Deltaproteobacteria was the highest in the suspended mariculture areas of fish and oysters in Daya Bay. This could be due to the fact that there is a higher POC deposition rate in this area, as the aerobic oxidation of POC resulted in a lower DO concentration in porewater (Castine et al., 2009; Faulwetter et al., 2013). Since members of Deltaproteobacteria are mostly belong to strict anaerobic genera and are known sulfate-reducing bacteria, it is reasonable that their abundances were highest in Daya Bay. These results were in accordance with previous findings showing that the abundances of bacterial communities in POC-driven coastal sediments are linked to their functions, such as sulfur and carbon cycling (Alongi, 2005; Muyzer and Stams, 2008; Zhu et al., 2018).
Co-occurrence pattern, based on statistical correlations among bacterial community, provide a new insight into potential biotic interactions and reveal niche spaces shared by community members (Kara et al., 2013; Ma et al., 2016; Tian et al., 2020). In the present study, the co-occurrence network showed that there were 3,457 positive correlations, whereas, 76 negative ones only. This indicated that the predominant relationships between bacterial populations were mutualistic interactions, rather than competitive ones for substrates or habitats. For instance, aerobic nitrogen – fixing bacteria use organic acids produced by cellulose-decomposing bacteria as their carbon source, and in return they provide fixed organic nitrogen compounds for cellulose-decomposing bacteria (Jensen and Swaby, 1941). Similarly, sulfate-reducing bacteria such as Desulfuromonas acetoxidans decompose organic matter and provide substrates for photosynthetic bacteria such as Chlorobium sp., and in return the S2– produced by photosynthetic bacteria can be used by sulfate-reducing bacteria. These results together with the statements on bacterial functions mentioned above suggested that the functions of bacteria and their inter-species mutualistic interactions were strongly linked with important geochemical processes in sediments. In addition, the network method could help identify which species play the most important role in maintaining the structure and interactions of microbial communities in sediments (Lupatini et al., 2014). The most widely used definition for keystone taxa is “whose impact on its community or ecosystem is huge and disproportionately large relative to its abundance” (Power et al., 1996). Therefore, OTUs with high degree and low betweenness centrality values are recognized as keystone taxa in co-occurrence networks (Berry and Widder, 2014; Banerjee et al., 2018; Tian et al., 2020). In the present study, five OTUs were identified as keystone taxa based on a high degree (>90) and low betweenness centrality (<5,000). Three of them belong to phylum of Planctomycetes phylum (two Rubripirellula sp., and one Blastopirellula sp.). It was reported that aquatic bacteria belonging to the Planctomycetes phylum could oxidize organic substrates through nitrate reduction and some are also capable of anaerobic ammonium oxidation (Kallscheuer et al., 2020). In the present study, we found that Planctomycetes was significantly positively correlated with NH4+ in all of the three bays, indicating that Planctomycetes probably play a major role in carbon and nitrogen circulations.
This study revealed the characteristics of bacterial communities in three typical mariculture bays. We found that the bacterial communities were influenced by both seasonal and spatial variation, with the spatial variation has a greater impact. The abundances of bacteria, particularly Bacteroidetes, Gammaproteobacteria, and Deltaproteobacteria, were significantly correlated with the mariculture-driven chemical properties of the sediment. The extent and pattern of mariculture derived impacts on sediment bacteria communities varied among regions, and were correlated with culture pattern and culture species. Our findings suggest that intensive mariculture could induce various impacts on the bacterial communities, and therefore modify their role in marine ecosystem, such as drive elements (e.g., carbon, nitrogen, and phosphorus) biogeochemical circulations and sediment metabolic capacity. The interactions between aquaculture and sediment bacterial communities should be considered in regard to aquaculture management and carrying capacity. Further studies are in need.
The datasets presented in this study can be found in online repositories. The names of the repository/repositories and accession number(s) can be found below: https://www.ncbi.nlm.nih.gov/, PRJNA734373.
RS and ZQ conceived the original ideas for the manuscript and collected sample from three mariculture areas. RS developed the outline, analyzed the data, prepared the figures and tables, and wrote the manuscript with some contributions to drafting from ZK and TH and critical refinement by HH. All authors contributed to the article and approved the submitted version.
This work was supported by the National Key Research and Development Program of China (2018YFD0900703 and 2018YFD0900704), National Natural Science Foundation of China (31900094 and 41976149), Guangdong Basic and Applied Basic Research Foundation (2021A1515011377), Central Public-Interest Scientific Institution Basal Research Fund, South China Sea Fisheries Research Institute, CAFS (2020YJ02 and 2021SD03), Central Public-Interest Scientific Institution Basal Research Fund, CAFS (2020TD15), Financial Fund of the Ministry of Agriculture and Rural Affairs, China (NFZX2021), and Key Special Project for Introduced Talents Team of Southern Marine Science and Engineering Guangdong Laboratory (Guangzhou) (ML2019ZD0402).
The authors declare that the research was conducted in the absence of any commercial or financial relationships that could be construed as a potential conflict of interest.
All claims expressed in this article are solely those of the authors and do not necessarily represent those of their affiliated organizations, or those of the publisher, the editors and the reviewers. Any product that may be evaluated in this article, or claim that may be made by its manufacturer, is not guaranteed or endorsed by the publisher.
We are thankful for the comments and suggestions of the reviewers and the editor that helped to improve the manuscript.
The Supplementary Material for this article can be found online at: https://www.frontiersin.org/articles/10.3389/fmars.2022.782456/full#supplementary-material
Alongi, D. M. (2005). “Mangrove-microbe-soil relations,” in Interactions Between Macro- and Microorganisms in Marine Sediments, eds E. Kristensen, R. R. Haese, and J. E. Kostka (Washington, DC: American Geophysical Union), 85–103. doi: 10.1029/ce060p0085
Arnosti, C., and Holmer, M. (2003). Carbon cycling in a continental margin sediment: contrasts between organic matter characteristics and remineralization rates and pathways. Estuar. Coast. Shelf Sci. 58, 197–208.
Banerjee, S., Schlaeppi, K., and Van der Heijden, M. G. A. (2018). Keystone taxa as drivers of microbiome structure and functioning. Nat. Rev. Microbiol. 16, 567–576. doi: 10.1038/s41579-018-0024-1
Beaulne, J., Mishra, S. R., Suar, M., Panda, A. N., Rastogi, G., Pattnaik, A. K., et al. (2020). Spatial analysis of bacteria in brackish lake sediment. Int. J. Sediment Res. 35, 227–236. doi: 10.1016/j.ijsrc.2020.01.003
Benjamini, Y., Krieger, A. M., and Yekutieli, D. (2006). Adaptive linear step-up procedures that control the false discovery rate. Biometrika 93, 491–507. doi: 10.1093/biomet/93.3.491
Berry, D., and Widder, S. (2014). Deciphering microbial interactions and detecting keystone species with co-occurrence networks. Front. Microbiol. 5:219. doi: 10.3389/fmicb.2014.00219
Burton, G. A., and Johnston, E. L. (2010). Assessing contaminated sediments in the context of multiple stressors. Environ. Toxicol. Chem. 29, 2625–2643. doi: 10.1002/etc.332
Castine, S. A., Bourne, D. G., Trott, L. A., and McKinnon, D. A. (2009). Sediment microbial community analysis: establishing impacts of aquaculture on a tropical mangrove ecosystem. Aquaculture 297, 91–98.
Chen, S., Zhou, Y., Chen, Y., and Gu, J. (2018). fastp: an ultra-fast all-in-one FASTQ preprocessor. Bioinformatics 34, i884–i890. doi: 10.1093/bioinformatics/bty560
Csardi, G., and Nepusz, T. (2006). The igraph software package for complex network research. Inter. J. Complex Systems. doi: 10.1186/1471-2105-12-455
DeLong, E. F. (1992). Archaea in coastal marine environments. Proc. Natl. Acad. Sci. U S A. 89, 5685–5689. doi: 10.1073/pnas.89.12.5685
Edgar, R. C. (2013). UPARSE: highly accurate OTU sequences from microbial amplicon reads. Nat. Methods 10, 996–998. doi: 10.1038/nmeth.2604
Erik, K., Ahmed, S. I., and Devol, A. H. (1995). Aerobic and anaerobic decomposition of organic matter in marine sediment: which is fastest? Limnol. Oceanogr. 40, 1430–1437. doi: 10.4319/lo.1995.40.8.1430
Faulwetter, J. L., Burr, M. D., Parker, A. E., Stein, O. R., and Camper, A. K. (2013). Influence of season and plant species on the abundance and diversity of sulfate reducing bacteria and ammonia oxidizing bacteria in constructed wetland microcosms. Environ. Microbiol. 65, 111–127. doi: 10.1007/s00248-012-0114-y
Fu, D., Wu, X., Qiu, Q., Duan, C., and Jones, D. L. (2020). Seasonal variations in soil microbial communities under different land restoration types in a subtropical mountains region. Southwest China. Appl. Soil Ecol. 153:103634. doi: 10.1016/j.apsoil.2020.103634
GB/T 12763.4-2007 (2007). Specifications for Oceanographia Survey—Part 4: Survey of Chemical Parameters in Sea Water. Beijing: Standards Press of China.
Gray, M. W., and Langdon, C. (2019). Particle processing by Olympia oysters Ostrea lurida and Pacific oysters Crassostrea gigas. Estuar. Coast. 42, 779–791. doi: 10.1007/s12237-018-0480-x
Hothorn, T., Bretz, F., and Westfall, P. (2008). Simultaneous inference in general parametric models. Biometrical J. 50, 346—-363. doi: 10.1002/bimj.200810425
Hou, D., Huang, Z., Zeng, S., Liu, J., Wei, D., Deng, X., et al. (2017). Environmental factors shape water microbial community structure and function in shrimp cultural enclosure ecosystems. Front. Microbiol. 8:2359. doi: 10.3389/fmicb.2017.02359
Ibekwe, A. M., Ma, J., and Murinda, S. E. (2016). Bacterial community composition and structure in an urban river impacted by different contaminant sources. Sci. Total Environ. 566– 567, 1176–1185. doi: 10.1016/j.scitotenv.2016.05.168
Jensen, H. L., and Swaby, R. J. (1941). Association between nitrogen-fixing and cellulose-decomposing microorganisms. Nature 147, 147–148. doi: 10.1038/147147a0
Jiang, Z., Li, J., Qiao, X., Wang, G., Bian, D., Xu, J., et al. (2015). The budget of dissolved inorganic carbon in the shellfish and seaweed integrated mariculture area of Sanggou Bay, Shandong, China. Aquaculture 446, 167–174. doi: 10.1016/j.aquaculture.2014.12.043
Jordaan, K., and Bezuidenhout, C. C. (2016). Bacterial community composition of an urban river in the north west province, South Africa, in relation to physico-chemical water quality. Environ. Sci. Pollut. Res. 23, 5868–5880. doi: 10.1007/s11356-015-5786-7
Julies Elsabé, J. M., Brüchert, V., and Fuchs, B. M. (2012). Vertical shifts in the microbial community structure of organic-rich Namibian shelf sediments. Afr. J. Microbiol. Res. 6, 3887–3897. doi: 10.5897/ajmr12.146
Kallscheuer, N., Wiegand, S., Heuer, A., Rensink, S., Jogler, C., Boedeker, C., et al. (2020). Blastopirellula retiformator sp. nov. isolated from the shallow-sea hydrothermal vent system close to Panarea Island. Anton. Leeuw. 113, 1811–1822. doi: 10.1007/s10482-019-01377-2
Kara, E. L., Hanson, P. C., Hu, Y. H., Winslow, L., and McMahon, K. D. (2013). A decade of seasonal dynamics and co-occurrences within freshwater bacterioplankton communities from eutrophic Lake Mendota, WI, USA. ISME J. 7, 680–684. doi: 10.1038/ismej.2012.118
Koho, K. A., Nierop, K. G. J., Moodley, L., Middelburg, J. J., Pozzato, L., Soetaert, K., et al. (2013). Microbial bioavailability regulates organic matter preservation in marine sediments. Biogeosciences 10, 1131–1141. doi: 10.5194/bg-10-1131-2013
Kolde, R. (2019). Pheatmap: Pretty Heatmaps. R Package Version 1.0.12. Available online at: https://CRAN.R-project.org/package=pheatmap (accessed January 4, 2019).
Kolukirik, M., Ince, O., Cetecioglu, Z., Celikkol, S., and Ince, B. K. (2011). Spatial and temporal changes in microbial diversity of the Marmara Sea Sediments. Mar. Pollut. Bull. 62, 2384–2394. doi: 10.1016/j.marpolbul.2011.08.033
Leon, S. M. S. S., Nuñal, S. N., Wei, H., Yoshikawa, T., and Maeda, H. (2017). Bacterial community composition shifts in sediments influenced by fish feeds. Aquac. Res. 48, 4380–4389. doi: 10.1111/are.13261
Lupatini, M., Suleiman, A. K. A., Jacques, R. J. S., Antoniolli, Z. I., Ferreira, A. S., Kuramae, E. E., et al. (2014). Network topology reveals high connectance levels and few key microbial genera with in soils. Front. Env. Sc. 2:10. doi: 10.3389/fenvs.2014.00010
Ma, B., Wang, H., Dsouza, M., Lou, J., He, Y., Dai, Z., et al. (2016). Geographic patterns of co-occurrence network topological features for soil microbiota at continental scale in eastern China. ISME J. 10, 1891–1901. doi: 10.1038/ismej.2015.261
Magoč, T., and Salzberg, S. L. (2011). FLASH: fast length adjustment of short reads to improve genome assemblies. Bioinformatics 27, 2957–2963. doi: 10.1093/bioinformatics/btr507
Mou, X., Sun, S., Edwards, R. A., Hodson, R. E., and Moran, M. A. (2008). Bacterial carbon processing by generalist species in the coastal ocean. Nature 451, 708–711. doi: 10.1038/nature06513
Muyzer, G., and Stams, A. J. M. (2008). The ecology and biotechnology of sulphate-reducing bacteria. Nat. Rev. Microbiol. 6, 441–454. doi: 10.1038/nrmicro1892
Oksanen, J., Blanchet, F. G., Friendly, M., Kindt, R., Legendre, P., McGlinn, D., et al. (2020). vegan: Community Ecology Package. R Package Version 2.5-7. Available online at: https://CRAN.R-project.org/package=vegan (accessed November 28, 2020).
Olsen, L. M., Hernández, K. L., Ardelan, M. V., Iriarte, J. L., Bizsel, K. C., and Olsen, Y. (2017). Responses in bacterial community structure to waste nutrients from aquaculture: an in situ microcosm experiment in a Chilean fjord. Aquacult. Environ. Interact. 9, 21–32. doi: 10.3354/aei00212
Parada, A. E., Needham, D. M., and Fuhrman, J. A. (2015). Every base matters: assessing small subunit rRNA primers for marine microbiomes with mock communities, time series and global field samples. Environ. Microbiol. 18, 1403–1414. doi: 10.1111/1462-2920.13023
Parks, D. H., Tyson, G. W., Hugenholtz, P., and Beiko, R. G. (2014). STAMP: statistical analysis of taxonomic and functional profiles. Bioinformatics 30, 3123–3124. doi: 10.1093/bioinformatics/btu494
Pedrós-Alió, C. (2006). Marine microbial diversity: can it be determined? Trends Microbiol. 14, 257–263. doi: 10.1016/j.tim.2006.04.007
Power, M. E., Tilman, D., Estes, J. A., Menge, B. A., Bond, W. J., Mills, L. S., et al. (1996). Challenges in the quest for keystones. Bioscience 46, 609–620. doi: 10.2307/1312990
Qi, Z., Shi, R., Yu, Z., Han, T., Li, C., Xu, S., et al. (2019). Nutrient release from fish cage aquaculture and mitigation strategies in Daya Bay, southern China. Mar. Pollut. Bull. 146, 399–407. doi: 10.1016/j.marpolbul.2019.06.079
R Core Team (2018). R: A Language and Environment for Statistical Computing. Vienna: R Foundation for Statistical Computing.
Revelle, W. (2021). psych: Procedures for Personality and Psychological Research. Evanston, ILL: Northwestern University.
Sanz-Lazaro, C., Casado-Coy, N., Calderero, E. M., and Villamar, U. A. (2021). The environmental effect on the seabed of an offshore marine fish farm in the tropical Pacific. J. Environ. Manage. 300:113712. doi: 10.1016/j.jenvman.2021.113712
Schloss, P. D., Westcott, S. L., Ryabin, T., Hall, J. R., Hartmann, M., Hollister, E. B., et al. (2009). Introducing mothur: open-source, platform-independent, community-supported software for describing and comparing microbial communities. Appl. Environ. Microbiol. 75, 7537–7541. doi: 10.1128/AEM.01541-09
Sui, Q., Zhang, L., Xia, B., Chen, B., and Qu, K. (2020). Spatiotemporal distribution, source identification and inventory of microplastics in surface sediments from Sanggou Bay. China. Sci. Total Environ. 723:138064. doi: 10.1016/j.scitotenv.2020.138064
Tian, W., Xiang, X., Ma, L., Evers, S., and Wang, H. (2020). Rare species shift the structure of bacterial communities across sphagnum compartments in a subalpine peatland. Front. Microbiol. 10:3138. doi: 10.3389/fmicb.2019.03138
Türetken, P. S. (2021). Environment variables, composition and metabolic characteristics of culturable sediment bacteria isolated around Gökçeada Island, Aegean Sea, Turkey. Reg. Stud. Mar. Sci. 42:101613. doi: 10.1016/j.rsma.2021.101613
Vetterli, A., Hyytiäinen, K., Ahjos, M., Auvinen, P., Paulin, L., Hietanen, S., et al. (2015). Seasonal patterns of bacterial communities in the coastal brackish sediments of the Gulf of Finland, Baltic Sea. Estuar. Coast. Shelf S. 165, 86–96. doi: 10.1016/j.ecss.2015.07.049
Wang, Q., Garrity, G. M., Tiedje, J. M., and Cole, J. R. (2007). Naive Bayesian classifier for rapid assignment of rRNA sequences into the new bacterial taxonomy. Appl. Environ. Microbiol. 73, 5261–5267. doi: 10.1128/AEM.00062-07
Wang, Y. S., Lou, Z. P., Sun, C. C., Wu, M. L., and Han, S. H. (2006). Multivariate statistical analysis of water quality and phytoplankton characteristics in Daya Bay, China, from 1999 to 2002. Oceanologia 48, 193–211.
Xia, B., Han, Q., Chen, B., Sui, Q., Jiang, T., Sun, X., et al. (2019). Influence of shellfish biodeposition on coastal sedimentary organic matter: a case study from Sanggou Bay. China. Cont. Shelf Res. 172, 12–21. doi: 10.1016/j.csr.2018.11.002
Xia, X., Guo, W., and Liu, H. (2015). Dynamics of the bacterial and archaeal communities in the Northern South China Sea revealed by 454 pyrosequencing of the 16SrRNA gene. Deep-Sea Res. Pt. II 117, 97–107. doi: 10.1016/j.dsr2.2015.05.016
Xie, Y., Wang, J., Wu, Y., Ren, C., Song, C., Yang, J., et al. (2016). Using in situ bacterial communities to monitor contaminants in river sediments. Environ. Pollut. 212, 348–357. doi: 10.1016/j.envpol.2016.01.031
Yi, Y., Lin, C., Wang, W., and Song, J. (2021). Habitat and seasonal variations in bacterial community structure and diversity in sediments of a Shallow lake. Ecol. Indic. 120:106959. doi: 10.1016/j.ecolind.2020.106959
Zhang, M., Wu, Z., Sun, Q., Ding, Y., Ding, Z., Sun, L., et al. (2019). The spatial and seasonal variations of bacterial community structure and influencing factors in river sediments. J. Environ. Manage. 248:109293. doi: 10.1016/j.jenvman.2019.109293
Zhu, P., Wang, Y., Shi, T., Zhang, X., Huang, G., and Gong, J. (2018). Intertidal zonation affects diversity and functional potentials of bacteria in surface sediments: a case study of the Golden Bay mangrove, China. Appl. Soil Ecol. 130, 159–168. doi: 10.1016/j.apsoil.2018.06.003
Keywords: mariculture, spatial and seasonal variation, sediment, bacterial community, keystone taxa
Citation: Shi R, Han T, Huang H, Kuang Z and Qi Z (2022) The Extent and Pattern of Mariculture Impacts on Spatial and Seasonal Variations of Sediment Bacterial Communities Among Three Coastal Waters. Front. Mar. Sci. 9:782456. doi: 10.3389/fmars.2022.782456
Received: 24 September 2021; Accepted: 16 February 2022;
Published: 10 March 2022.
Edited by:
Daniel P. R. Herlemann, Estonian University of Life Sciences, EstoniaReviewed by:
Dandan Izabel-Shen, Stockholm University, SwedenCopyright © 2022 Shi, Han, Huang, Kuang and Qi. This is an open-access article distributed under the terms of the Creative Commons Attribution License (CC BY). The use, distribution or reproduction in other forums is permitted, provided the original author(s) and the copyright owner(s) are credited and that the original publication in this journal is cited, in accordance with accepted academic practice. No use, distribution or reproduction is permitted which does not comply with these terms.
*Correspondence: Zhanhui Qi, cWl6aGFuaHVpQHNjc2ZyaS5hYy5jbg==
Disclaimer: All claims expressed in this article are solely those of the authors and do not necessarily represent those of their affiliated organizations, or those of the publisher, the editors and the reviewers. Any product that may be evaluated in this article or claim that may be made by its manufacturer is not guaranteed or endorsed by the publisher.
Research integrity at Frontiers
Learn more about the work of our research integrity team to safeguard the quality of each article we publish.