- 1Key Laboratory of Sustainable Development of Marine Fisheries, Ministry of Agriculture and Rural Affairs, Yellow Sea Fisheries Research Institute, Chinese Academy of Fishery Sciences, Qingdao, China
- 2Laboratory for Marine Fisheries Science and Food Production Processes, Pilot National Laboratory for Marine Science and Technology (Qingdao), Qingdao, China
- 3College of Life Sciences, University of Qingdao, Qingdao, China
Laver is the most widely farmed seaweed with the largest culture area in China. The spatio-temporal variations in composition, diversity, and functional properties of bacteria in seawater as well as the environmental variables of seawater in a large-scale laver farm in China were studied. Both the community richness indices and Shannon index in the laver farming area remained at a relatively stable level during laver cultivation. Fifty-nine prokaryotic phyla were detected in all samples, however, only six of these phyla accounted for 98.84% of all sequences. Proteobacteria, Gammaproteobacteria, Rhodobacterales, Rhodobacteraceae, and Octadecabacter were the most predominant bacterial taxa at different levels of classification. The keystone bacterial taxa were Bacteroidetes, Pseudomonadales, Rhodobacterales, Flavobacteriales, Loktanella, and Pseudoruegeria based on network analysis. Members of representative bacterial biomarker taxa in November may be associated with degradation of algal cell wall polysaccharides. A significant increase in metabolic exchange and transformation nutrients occurred in the seawater during the early and late stage of laver cultivation, indicating that the laver reproductive activities (i.e. the formation/release activities of archeospores and zygotospores) probably drove the variation of metabolic functional diversity of bacterial communities. Based on Mantel test and redundancy analysis, we found the hydrographic parameters (e.g. salinity, temperature, DO, pH) as well as the key carbon (e.g. POC, DOC) and nitrogen parameters (e.g. nitrate, DIN, DON, TDN) were crucial environmental variables to shape the bacterial community composition in the surrounding seawater of laver farm. In a word, our results suggested that the microbial community structure and function significantly changed across the different succession stages during laver cultivation. This work provides new insights on the characteristics of bacterial communities in a large-scale laver farming system and solidifies the importance of laver farming in shaping seawater microbiomes.
Background
Microbes are the prevailing drivers of a variety of principal geochemical capacities, such as organic matter degradation, nutrient cycling and energy conversion (Zhao et al., 2018). The composition and diversity of bacterial communities is thought to have direct effects on a wide range of ecosystem processes (Fierer and Jackson, 2006). Knowledge of the distribution and abundance of bacteria and the environmental factors that control them is the key to understand ecosystem function (Suh et al., 2015). In recent years, a lot of studies have focused on aquatic microbial communities (Shafi et al., 2017; Callieri et al., 2019). Especially, studies on biodiversity and community composition of bacteria on the seaweed surface have been one of the scientists’ focus in the microbial ecology (Ahmed et al., 2021; Comba González et al., 2021; de Mesquita et al., 2019; Ghaderiardakani et al., 2020; Singh and Reddy, 2014; Zhang et al., 2020b).
Seaweed has attracted extensive attention worldwide due to its ecological and industrial value (Ren et al., 2022). Within the world aquaculture sector, the cultivation of seaweed is expanding by almost 8% per year over the past decade (FAO, 2016). The seaweed farm based on open sea culture system is a very dynamic environment that is affected by diverse biotic and abiotic factors, which will contribute to shaping the bacterial community associated with seaweed and surrounding seawater (Hu et al., 2017; Xie et al., 2017; Zhao et al., 2018; Wang et al., 2020). For instance, the large-scale cultivation of Gracilaria lemaneiformis increased dissolved oxygen and pH but decreased inorganic nutrients, which significantly changed the composition and structure of the microbial community in the surrounding environment (Hu et al., 2017; Xie et al., 2017). The microbial community structures and functions in seawater were significantly changed during the progression of Undaria pinnatifida aquaculture (Zhao et al., 2018). Moreover, Clasen and Shurin (2015) found that more extensive kelp forests are associated with larger changes in water column microbial communities; and they suggested that larger kelp forests produced more detritus and dissolved organic matter, which stimulate microbial activity. It was also reported that notable changes in the bacterial community structures were observed in a series of industrial algae production systems, and larger systems contained richer bacterial communities compared to smaller systems (Fulbright et al., 2018). However, characterization of the biotic component (communities) and the process component of an open, large, complex, and interacting ecosystem is a daunting task (Briones and Raskin, 2003; Butler and O’Dwyer, 2018). There is little information available about how the bacterial communities in surrounding seawater of seaweed farms vary on large time and spatial scales and what are the main drivers of this variation (Egan et al., 2013; Ren et al., 2022).
Laver is a popular seafood delicacy in many countries due to its high nutritional value and delicious flavor (Venkatraman and Mehta, 2019), and has the highest commercial value per unit mass when compared to other aquaculture species (Kim et al., 2017). Neopyropia yezoensis and Neoporphyra haitanensis are industrially farmed laver species in China. The culture area of laver is expanding over the past decade in China and it is still increasing, accounting for nearly 60% of all the farmed seaweeds (Wang et al., 2019; Liang et al., 2022). The relevant researches about the associations between microbial communities and laver mainly focused on the symbiotic microorganisms or pathogenic microorganisms of laver (Guan et al., 2018; Xiong et al., 2018; Yan et al., 2019; Liu et al., 2020; Wang et al., 2021). In terms of symbiotic role, the enriched microbial community might promote laver thallus morphogenesis and be beneficial for the growth and development of laver (Miranda et al., 2013; Wang et al., 2020). Reciprocally, the bacterial community diversity was affected by the thallus morphology, culture time and environmental factors during laver cultivation (Shen et al., 2013). In addition, a distinct shift in the microbial community composition has also been observed between seawater with and without N. haitanensis cultivation as well as between the algal heteromorphic generations (Wang et al., 2020). Nevertheless, a large number of comparative studies are still needed to reveal the interactions between laver-associated microbe and surrounding environments in the large-scale laver culture systems.
Therefore, a typical large-scale laver farm (approximately 40 ha) in Rizhao City, Shandong Province, China, were investigated in the present study. The temporal and spatial distribution characteristics of environmental factors and microbial communities in the seawater were investigated monthly during a production period of laver thallus stage in the study. The aim of this study was to characterize the dynamics of the bacterial community structure and function in the seawater from a laver farm and explore the crucial environmental factors in shaping them by means of metagenomics and physical-chemical analyses. These findings will enhance our understanding of the patterns and processes of microbial community assembly during laver cultivation.
Methods
Sample collection and water quality characteristics determination
Samples were collected in the sea area near Fuxin fishing port of Lanshan District in Rizhao, China, which has been sustained the laver farming (including species of N. yezoensis and N. haitanensis) more than 10 years and became a major laver farming site in Northern China. The cultivation time of laver thallus in the study area is generally from mid-October to early April of the next year. In order to obtain representative samples in the early (from October to November), middle (from January to February) and late (from March to April) stages respectively during a production period of laver thallus stage, we collected seawater samples from November 2016 to March 2017 in the laver farm (Table 1). The sampling sites were established from laver farming area (PF) (between latitudes of 35°14.04′N and 35°15.68′N and longitudes of 119°24.44′E and 119°26.44′E), near-shore non-farming sea area (CNS), and offshore non-farming sea area (COS), respectively (Figure 1). Each sampling area like CNS1 included six sampling sites in the study.
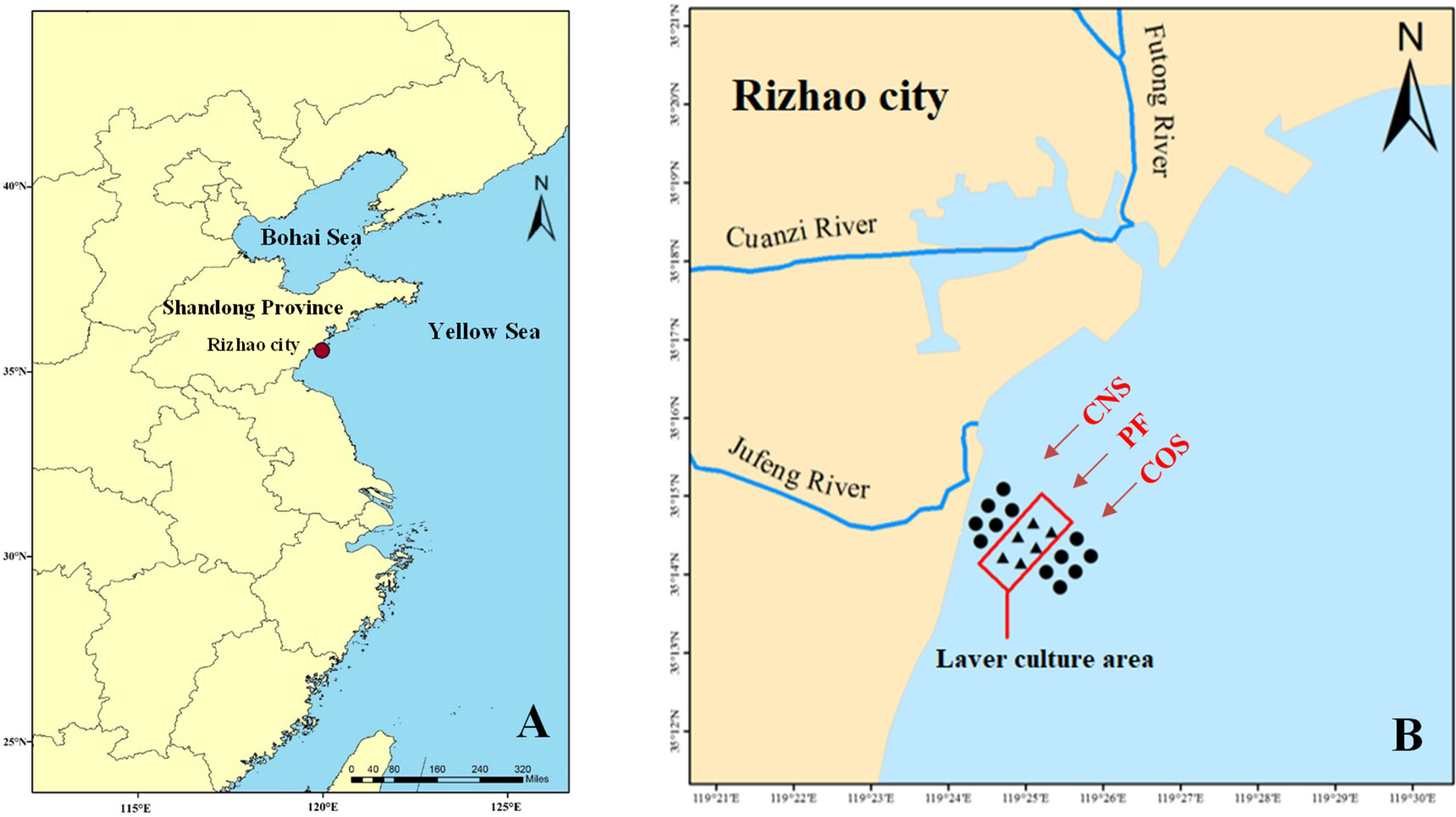
Figure 1 Map of the study area. (A) Location of the study area in Rizhao, a coastal city in East China’s Shandong province. The red dot indicates the study sea area near Fuxin fishing port of Lanshan District in Rizhao city. (B) The sampling points (shown by ▲ and ●), with Jufeng River estuary at the west and Futong River estuary at the north. The rectangle indicates a large-scale laver culture area (PF). The near-shore and off-shore non-farming areas approximately 1 km away from PF were represented as CNS and COS, respectively.
Each surface water sample (0.5-m depth) with volume of 3 liters was collected from all eighteen sampling sites monthly from November 2016 to March 2017. Then, the water samples were pre-filtered through nylon meshes (100-μm pore size) to remove debris and subsequently filtered through 0.22-μm mixed cellulose membranes (Merck Millipore, Burlington, MA) to collect the microbial biomass. The fiber membrane were preserved in the sterile centrifuge tube, stored in dry ice before transport to the laboratory, then stored at -80°C until further processing. Moreover, 50 mL of sample were filtered through glass microfiber filters (GF/F, pore size 0.45 μm, Whatman) and frozen immediately for later, shore-based phosphate , ammonium , nitrate , nitrite nitrogen , dissolved inorganic nitrogen (DIN, defined as sum of ammonia, nitrite and nitrate nitrogen) analyses. For particulate organic carbon (POC) determination, 200 mL of sample were filtered onto pre-combusted (450°C, 5 h) Whatman GF/F filters (pore size 0.7 μm) and then frozen in dry ice. In addition, for determinations of dissolved organic carbon (DOC) and total dissolved nitrogen (TDN), 100 mL of sample were put into glass bottles and were preserved with mercuric chloride (HgCl2, 0.5 μM) to stop microbial activity.
The temperature, pH, salinity, and dissolved oxygen (DO) were detected using the YSI-multiparameter instrument (600 QS-M-O, USA) at a depth of 0.5 m. The water transparency (Tra) was measured with a Secchi disc (20 cm in diameter). The concentration of total suspended solids (TSS) was determined by comparing the mass difference of filter paper (cellulose nitrate 0.45 μm) before and after filtering the 500 mL sample of water. The POC analyses were conducted according to Ray et al. (2018). The concentrations of , , and were determined using standard colorimetric techniques on an auto-analyzer (Auto Analyzer AA-3, Bran-Luebbe, Norderstadt, Germany). The concentrations of DOC and TDN were determined using a total organic carbon analyzer (TOC-V CSH, Shimadzu, Kyoto, Japan). TDN is comprised of DIN and dissolved organic nitrogen (DON), i.e. DON is calculated from measured TDN and DIN values (DON=TDNDIN).
DNA extraction, PCR amplification, illumina Hiseq sequencing, and data processing
The bacterial community DNA was extracted from the sample using HiPureSoil DNA Kits (Megan, Guangzhou, China) according to the manufacturer’s instructions. The hypervariable region V3-V4 of the 16S rRNA gene was selected to construct a bacterial community library by Illumina sequencing. The specific primer set, 341F: 5’-CCTACGGGNGGCWGCAG-3’ and 806R: 5’-GGACTACHVGGGTATCTAAT-3’, was used for amplification. PCR amplifications were performed according to Liang et al. (2019a). The amplicons were extracted by agarose gel electrophoresis, purified using the AxyPrep DNA Gel Extraction Kit (Axygen Biosciences, Union City, CA, USA) and quantified using QuantiFluor-ST (Promega, USA) according to the manufacturer’s instructions. Purified amplicons were pooled in equimolar and paired-end sequenced (2×250) by Illumina HiSeq2500 PE250 technology. Sequencing of the 16S rRNA gene was performed in Gene Denovo Biotechnology Co., Ltd. (Guangzhou, China). Then, the data processing, including quality control and reads assembly as well as OTU cluster and taxonomic classification, were carried out according to the method of Gu et al. (2021). Raw-reads data were deposited in the NCBI Sequence Read Archive (SRA) with accession number of PRJNA841061.
Alpha diversity and beta diversity analysis
Alpha diversities including the abundance-based coverage estimator (ACE), bias-corrected Chao 1 richness estimator, Shannon’s diversity index (H’) and Simpson’s diversity index (D) were calculated in QIIME software package (Caporaso et al., 2010). As D increases, diversity decreases and therefore Simpson’s index is usually expressed as Simpson’s complementary diversity (1−D) or Simpson’s reciprocal index (1/D) (Goswami et al., 2017). In this study, the former expression (i.e. 1−D) was used. Community richness and diversity comparisons among groups were computed by Duncan multiple comparisons test using the SPSS 19.0 statistical software packages. The statistical significance was set at p< 0.05. The coverage percentage was estimated by Good’s method (Good, 1953). The Shannon rarefaction curve was plotted using Mothur (v.1.34.0) to evaluate whether the sequencing data amount was enough to cover all of the sample species.
Analysis of spatial and temporal variations using PCA, cluster, and LEfSe method
The principal component analysis (PCA), reflecting the microbial community structures differences between samples, was analyzed based on the expression profile of OTUs using R software package. Moreover, according to the OTU expression profile, the distance between samples was calculated and then cluster analysis was carried out using R software package pvclust (http://www.is.titech.ac.jp/~shimo/prog/pvclust/) to predict the sample similarity on OTU level. Two kinds of p-value including AU (Approximately Unbiased) and BP (Bootstrap Probability) were provided to evaluate the reliability of cluster.
Linear discriminant analysis (LDA) effect size (LEfSe) method was used to identify the most differentially abundant taxa/pathways between groups (Segata et al., 2011). Differentially abundant KEGG pathways at level 2 were compared using LEfSe with default parameter (alpha value for Kruskal Wallis and Wilcoxon test was set to 0.05 and LDA threshold value of > 2). Whereas, to identify differentially abundant taxa at all taxonomical level (from Phylum to Species level), LEfSe analysis was carried out using a LDA threshold value of > 3.5 and the taxonomic cladograms representative of the structure of microbial community were drawn.
Predicted functional analysis and correlation analysis with environmental factors
Phylogenetic Investigation of Communities by Reconstruction of Unobserved States (PICRUSt) was used to predict potential functional gene abundances of the bacterial communities (Douglas et al., 2018). A Pearson’s correlation analysis was calculated to determine the interaction between environmental factors. Moreover, in order to assess the relationship between environmental factors and taxonomic/functional composition, a Mantel test was performed (Sunagawa et al., 2015). Redundancy analysis was then conducted to further evaluate the effects of water quality variables on the bacterial communities using R software. Moreover, network analysis was conducted on bacterial OTUs and water quality variables according to Reshef et al. (2011) and Li et al. (2017).
Results
After filtering chimeric sequences and mismatches, the total number of V3-V4 region of the 16S rRNA gene reads obtained from the all samples was 4,614,926, which was clustered into 426,599 OTUs at a cut-off of 97% sequence similarity. The Shannon rarefaction curves of all samples tend to be flat (Additional File 1: Figure S1), suggesting that the sequencing data amount was enough to cover all of the sample species.
Bacterial community richness and community diversity
As shown in Figure 2, both the community richness indices (ACE and Chao 1) and Shannon index (H’) were the highest in near-shore non-farming area (CNS) in November. The values of ACE, Chao 1 and H’ in CNS decreased from November to February (during the first two stages) and then increased in March (the late stage). However, ACE, Chao 1 and H’ in the laver farming area (PF) remained at a relatively stable level during laver cultivation. Furthermore, PF in January showed higher (p< 0.05) bacterial equitability (i.e. Simpson 1-D index) compared to that in November, and then remained at a steadily high level in the last two stages during laver cultivation. The community richness indices remained at a stable level in offshore non-farming area (COS) during laver cultivation, but equitability index decreased significantly (p< 0.05) in COS in March.
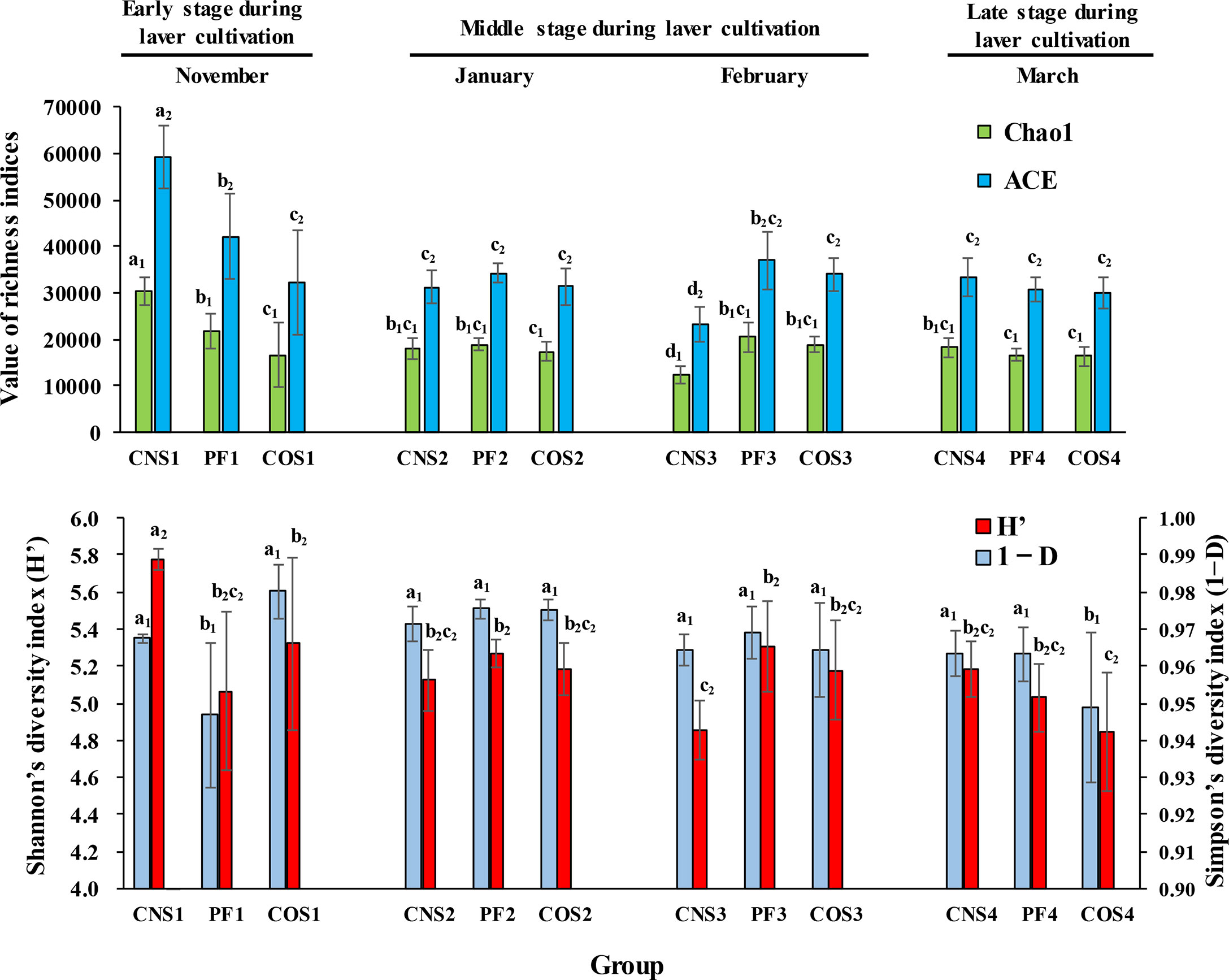
Figure 2 The water bacterial community richness and community diversity at different sea areas in different months during laver cultivation. Data were separated into three categories representing three stages during laver cultivation: early stage (November), middle stage (from January to February), and late stage (March). ACE (abundance-based coverage estimator) and Chao1 indices were used to evaluate the community richness, while Shannon’s diversity index (H’) and Simpson’s complementary diversity index (1−D) were used to assess the community diversity. The sample abbreviations are as in Table 1. The groups with the same subscript were compared. The different letters represent significant differences. Data are the mean ± SD (n = 6).
Relationships among the bacterial communities in the different sampling groups
The principal component analysis (PCA) was carried out to reveal the relationships among bacterial communities in the different sampling groups at the order level (Figure 3A) and genus level (Figure 3B). The first (PC1) and second (PC2) principal components explained 52.28% and 32.21% of total variation, respectively, at the order level. In addition, PC1 and PC2 explained 50.98% and 33.98% of total variation, respectively, at the genus level. The PCA diagrams illustrate that samples are not clearly grouped by spatial variations, but rather separated by temporal variation. Nonetheless, more distinct spatial partitioning at early stage (November) was observed compared to the other stages. Clear partitioning of the samples collected in November (i.e. CNS1, PF1, COS1) from the samples collected in January and February (i.e. CNS2, PF2, COS2, CNS3, PF3, and COS3) along the PC2 (Figure 3A). The cluster dendrogram (Figure 3C) shows that the CNS and PF samples tended to cluster together, except the samples collected in February, that is CNS3 and COS3 samples were merged into a cluster in February.
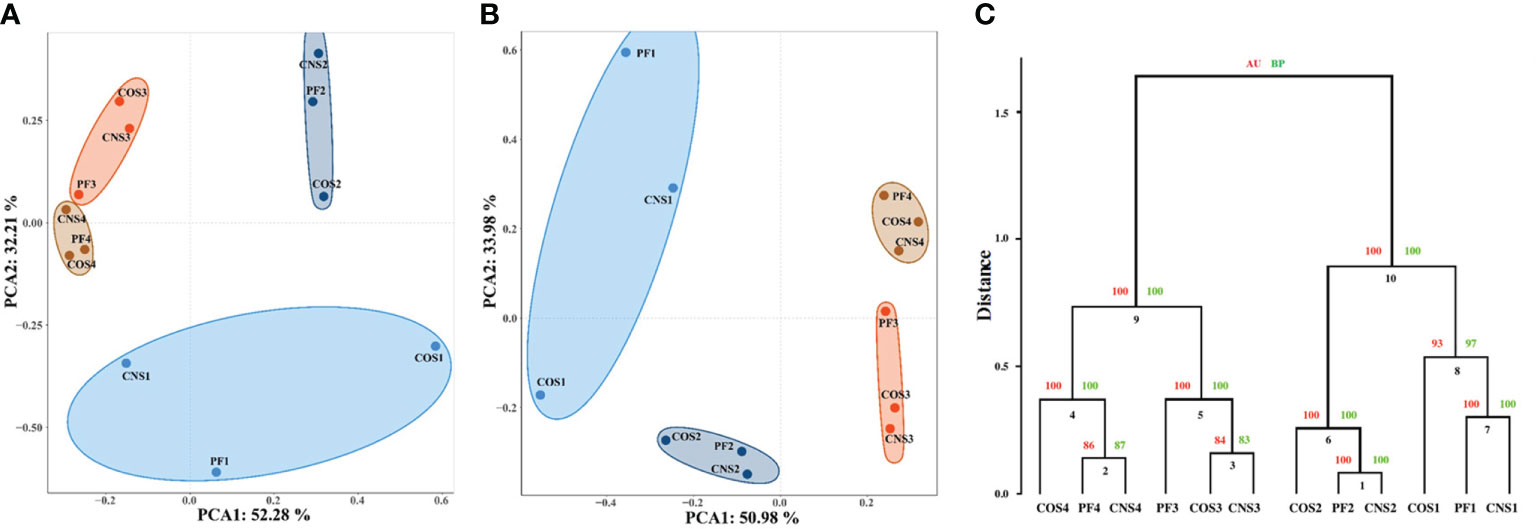
Figure 3 Relationships between individual datasets illustrated by principal component analysis (PCA) at the order level (A) and genus level (B) as well as cluster analysis at the genus level (C). In the PCA diagram, the axis labels indicate the percentages of variance explained by each principal component. In the cluster dendrogram, AU and BP indicate approximately unbiased and bootstrap probability values (%), respectively. The sample abbreviations are as in Table 1.
Bacterial community composition
Fifty-nine prokaryotic phyla were detected in all samples, however, only six of these phyla accounted for 98.84% of all sequences. The predominant phyla were Proteobacteria (78.04%), Bacteroidetes (10.58%), Firmicutes (3.92%), Actinobacteria (3.53%), Cyanobacteria (1.57%), and Verrucomicrobia (1.21%) in all sequences (Figure 4A). Gammaproteobacteria, Rhodobacterales, Rhodobacteraceae, and Octadecabacter were the most predominant class, order, family, and genus with proportions of 39.00%, 27.49%, 27.47% and 12.89% respectively. Moreover, Alphaproteobacteria (31.34%), Oceanospirillales (13.65%), Halomonadaceae (6.40%), and Candidatus Portiera (6.31%) were the second most predominant class, order, family, and genus respectively.
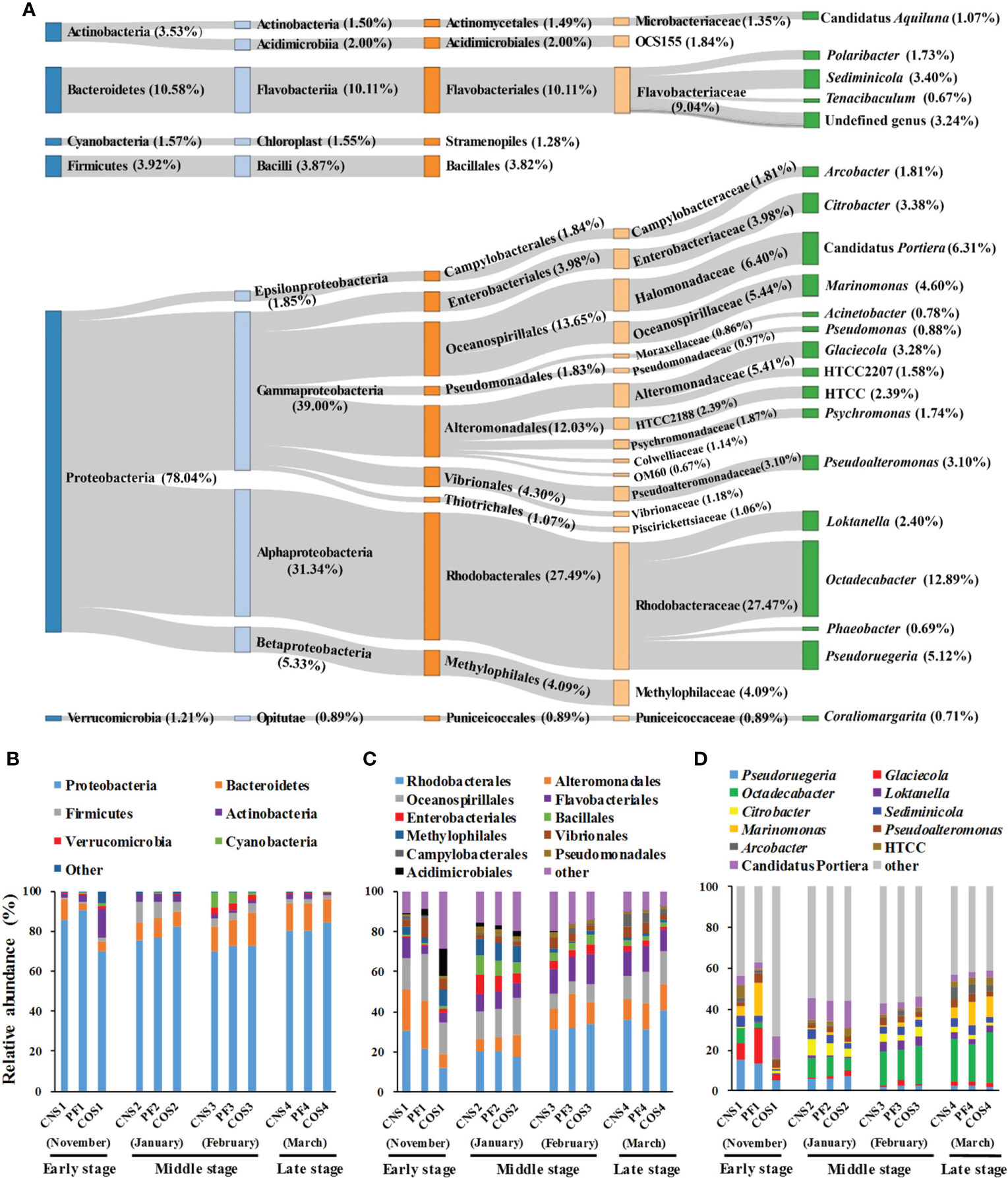
Figure 4 The taxonomy and average relative abundance of core-mircobiome in all samples (A) and the relative abundance of predominant bacteria at different sampling areas during laver cultivation at three classification levels. (B) at the phylum level; (C) at the order level; (D) at the genus level. The sample abbreviations are as in Table 1.
The relative abundance of bacteria showed marked spatial variations in the early stage (November) during laver cultivation (Figures 4B–D). The abundance of Proteobacteria, Rhodobacterales, Alteromonadales, Pseudoruegeria, Glaciecola, Octadecabacter, and Marinomonas in COS1 were all significantly lower compared with those in CNS1 and PF1 (p< 0.05). Conversely, Actinobacteria, Pseudomonadales (belonging to Actinobacteria), Firmicutes, Bacillales (belonging to Firmicutes), and Citrobacter in COS1 were all significantly higher compared to those in CNS1 and PF1 (p< 0.05). In the middle stage during laver cultivation (from January to February), there were significant differences (p< 0.05) in the abundance of Bacteroidetes, Firmicutes, Alteromonadales, and Oceanospirillales among the groups of CNS2, PF2, and COS2, and the abundance of Alteromonadales in PF3 increased significantly (p< 0.05) compared to that in CNS3 and COS3. In addition, the abundance of Cyanobacteria in COS3 was significantly lower (p< 0.05) compared to that in CNS3 and PF3. In the late stage during laver cultivation (March), there were significant differences (p< 0.05) in the abundance of Marinomonas and Pseudoalteromonas among the groups of CNS4, PF4, and COS4.
In general, the relative abundance of most bacteria also showed marked temporal variations during laver cultivation (Figures 4B–D). For example, the abundance of Bacteroidetes in PF2 and COS2 increased obviously (p< 0.05) compared to that in PF1 and COS1 respectively, then remained at high levels during subsequent cultivation. The abundance of Firmicutes was significantly higher (p< 0.05) in the middle stage (from January to February) compared with that in the early or late stage during laver cultivation. The abundance of Cyanobacteria was significantly higher in February compared to that in other sampling times. Furthermore, both the abundance of Pseudoruegeria and Glaciecola (both belonging to Gammaproteobacteria) in PF2 and CNS2 decreased obviously (p< 0.05) compared to those in PF1 and CNS1 respectively, then Pseudoruegeri abundance continued to decrease and Glaciecola abundance remained at low levels during subsequent cultivation. In addition, the abundance of Octadecabacter and Loktanella (both belonging to Alphaproteobacteria) were significantly lower in the early stage compared the other stages during laver cultivation.
Specific bacterial taxa with statistically significant differences based on LEfse analysis
LEfSe was also used to assess the effect size of each differentially abundant taxon (Figure 5). Fifteen, nineteen, and fifteen biomarkers with a LDA score greater than 4 were identified in the spatial comparison groups of CNS-PF (Figure 5A), COS-PF (Figure 5B), and CNS-COS (Figure 5C), respectively. The microbiota of PF was characterized by a preponderance of microorganisms from the class Gammaproteobacteria including families of Oceanospirillaceae and Alteromonadaceae as well as genera of Marinomonas and Glaciecola. Moreover, the microbiota of COS was characterized by the class Acidimicrobiia including order Acidimicrobiales and family OCS155 as well as the order Oceanospirillales including family Halomonadaceae and genus Candidatus Portiera. In addition, the genera of Pseudoruegeria and Sediminicola were considerably more abundant in CNS than those in COS.
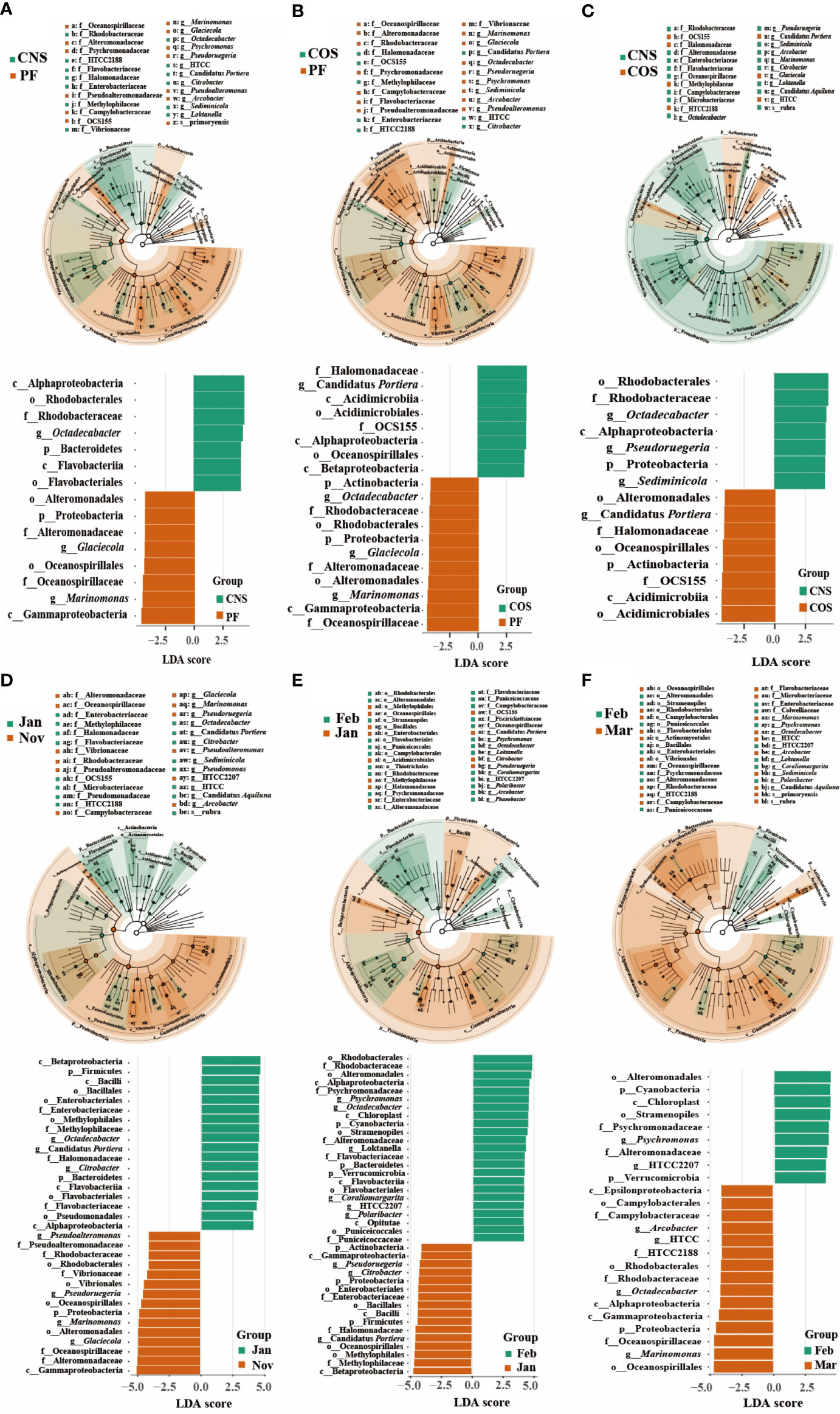
Figure 5 Distinct bacterial taxa identified by linear discriminant analysis (LDA) coupled with effect size (LEfSe) in different spatial comparison groups (CNS-PF (A), COS-PF (B), and CNS-COS (C)) as well as temporal comparison groups in the laver farming area (“January-November” (D), “February-January” (E), and “March-February” (F)). For each comparison group, the LDA value distribution histogram (bottom of each sub figure) shows bacterial communities or species (biomarker) with a LDA score greater than 4. The taxonomic cladogram was visualized with LDA values higher than 3.5. The significantly distinct taxon nodes are colored in blue-green and brown circles. The nonsignificant bacterial taxa are indicated with white circles. PF, CNS, and COS indicate the laver farming area, near-shore non-farming area, and off-shore non-farming area, respectively.
Thirty-four, thirty-nine, and twenty-four biomarkers with a LDA score greater than 4 were identified in the temporal comparison groups of “January-November” (Figure 5D), “February-January” (Figure 5E), and “March-February” (Figure 5F), respectively. The class Gammaproteobacteria was significantly more abundant in November compared to January, with representative biomarkers of genera of Marinomonas, Glaciecola, Pseudoruegeria, and Pseudoalteromonas in November as well as genera of Octadecabacter, Candidatus Portiera, and Citrobacter in January. Compared to January, the classes of Alphaproteobacteria, Flavobacteriia, and Opitutae were evidently more abundant and characterized by taxonomic biomarkers of genera of Psychromonas, Octadecabacter, Loktanella, Coraliomargarita, and Polaribacter in February. Compared to February, the classes of Gammaproteobacteria, Alphaproteobacteria, and Epsilonproteobacteria were markedly more abundant in March, with representative biomarkers of genera of Marinomonas, Arcobacter, and HTCC.
Functional predictions for bacterial communities
Based on PICRUSt analysis, 5068 KEGG Orthology IDs, including 139 KEGG pathways, 25 KEGG modules and 6 KEGG categories were generated in all sampling groups (Figure 6A). The most dominant KEGG modules were global and overview, carbohydrate metabolism, membrane transport, signal transduction, amino acid metabolism, nucleotide metabolism, and energy metabolism (Figure 6B). The samples collected in the middle stage during laver cultivation (January and February) showed a significant higher abundance of metabolism of cofactors and vitamins compared to that in the early (November) and late (March) stages (p< 0.05), and those collected in January had a significant higher level of nucleotide metabolism compared to other groups (p< 0.05) (Figure 6C).
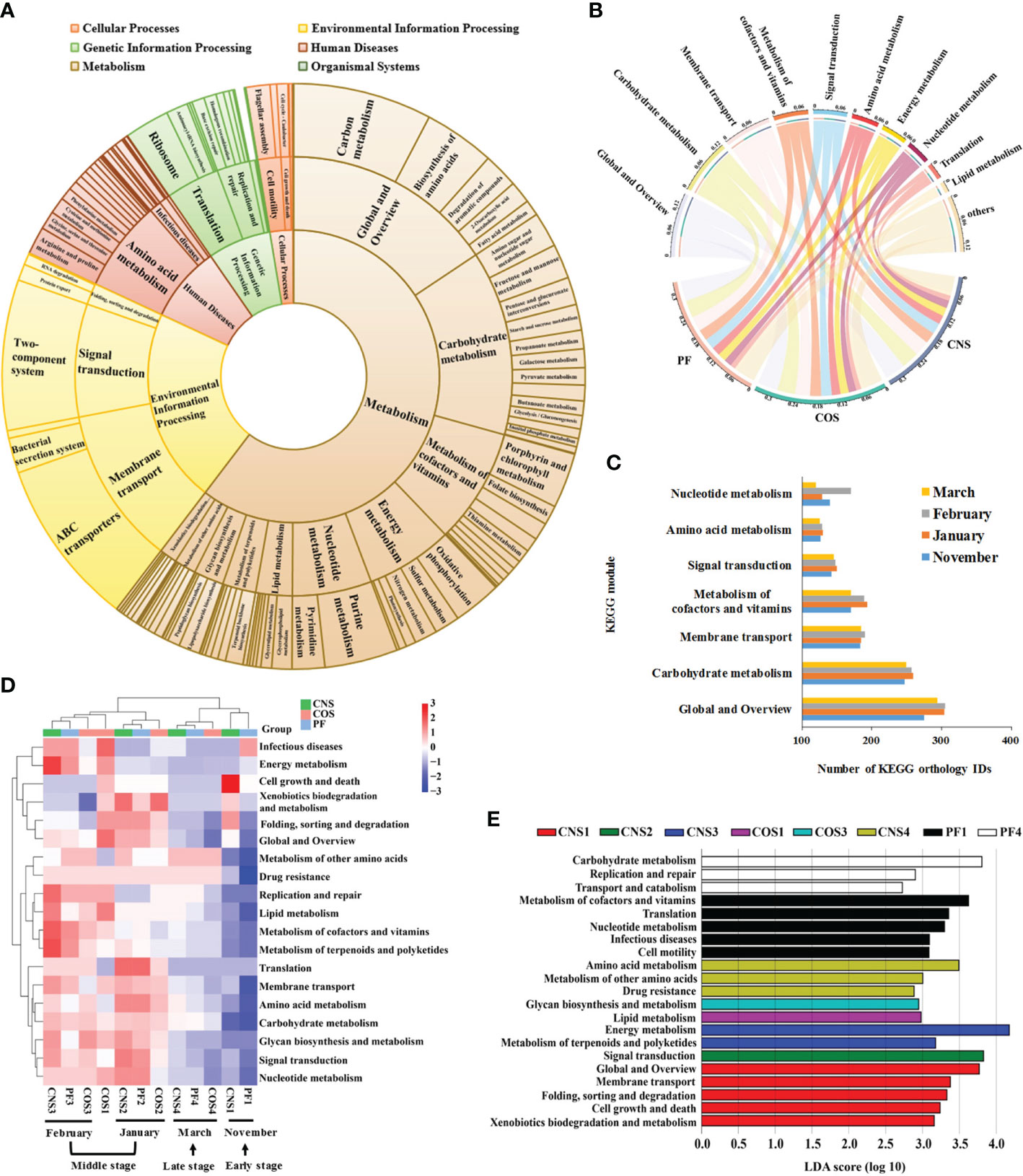
Figure 6 Predictive functional profiling of the bacterial communities in all samples. (A) Pie chart showing the distribution of the Kyoto Encyclopedia of Genes and Genomes (KEGG) orthology groups at three levels in the bacterial microbiota. (B) Relative abundance of the top 10 predicted KEGG modules in different sampling groups (PF, CNS, and COS). The length of the bars of the outer-ring on the above semicircle represented the percentage of the KEGG modules and the width of ends of the colored stripes in the middle circle represented the relative abundance among different samples. (C) Average number of the predicted KEGG orthology IDs in seven dominant KEGG modules for the sampling groups collected in November, January, February, and March, respectively. (D) Heat map showing the hierarchical clustering of KEGG orthology groups at level 2 in the bacterial microbiota. Heat map is color-coded based on row z-scores. (E) Linear discriminative analysis (LDA) effect size (LEfSe) analyses of statistically significant KEGG modules with a LDA score cut-off of 2. The sample abbreviations are as in Table 1.
The heat map of the dominant KEGG modules (Figure 6D) showed distinct differences in functional potentials of bacterial communities between early and middle stages, and between middle and late stages across the laver cultivation period. Nevertheless, the samples collected in the middle stage during laver cultivation (i.e. January (CNS2, COS2, PF2) and February (CNS3, COS3, PF3)) were tend to clustered together, and the samples collected in the early stage (CNS1 and PF1, except COS1) and late stage (CNS4, PF4, and COS4) were closely related (Figure 6D). The LEfSe analysis showed that metabolism of cofactors and vitamins, translation, nucleotide metabolism, and cell motility were representative biomarkers in PF1; carbohydrate metabolism, replication and repair, and transport and catabolism were representative biomarkers in PF4 (Figure 6E). Furthermore, KEGG modules of global and overview, membrane transport, cell growth and death, xenobiotics biodegradation and metabolism as well as folding, sorting and degradation were significantly more abundant in CNS1 (Figure 6E). In addition, signal transduction, lipid metabolism, glycan biosynthesis and metabolism, and amino acid metabolism were representative biomarkers of CNS2, COS1, COS3, and COS4, respectively (Figure 6E).
Correlation among environmental factors and bacterial community
The spatio-temporal variations of seawater environmental factors were shown in Additional File 2 (Table S1). Water temperature varied from maxima of 9.97–11.20°C in November to minima of 5.03–5.53°C in February. Obvious seasonal variation in DO concentration was observed and maxima in mean DO were recorded in February (ranging from 11.67 to 13.17 mg L-1). Salinity varied geographically with an increasing trend from near-shore to off-shore sea areas, which was likely to be caused by the river water runoff. Generally, the spatial horizontal distribution of inorganic nitrogen and phosphate concentrations showed a decreasing trend from the nearshore to offshore sea areas. The concentrations of nitrate, phosphate, and DIN were significantly different in the different months. The concentrations of ammonium in PF2 group were significantly higher than that in PF1 group. The concentrations of nitrate and ammonium were the lowest in February, while the concentration of phosphate was the lowest in March. Nitrate was the main form of inorganic nitrogen, accounting for 79.76% of DIN. DOC, DON, and TDN concentrations were significantly higher in November than those in other months.
The links between taxonomic/functional community composition and environmental factors were detected using the Mantel test (Figures 7A–C). The taxonomic community composition was significantly correlated (Mantel’s p< 0.05) with some environmental factors, i.e. salinity in CNS (Mantel’s r = 0.832, Figure 7A), TDN (Mantel’s r = 0.649) and POC (Mantel’s r = 0.519) in PF (Figure 7B), and TDN (Mantel’s r = 0.767) in COS (Figure 7C). Moreover, the functional community composition also had significant correlations (Mantel’s p< 0.05) with some environmental factors, i.e. DO (Mantel’s r = 0.845) and TSS (Mantel’s r = 0.917) in CNS (Figure 7A), temperature (Mantel’s r = 0.979) and DOC (Mantel’s r = 0.756) in PF (Figure 7B), and phosphate (Mantel’s r = 0.979) in COS (Figure 7C).
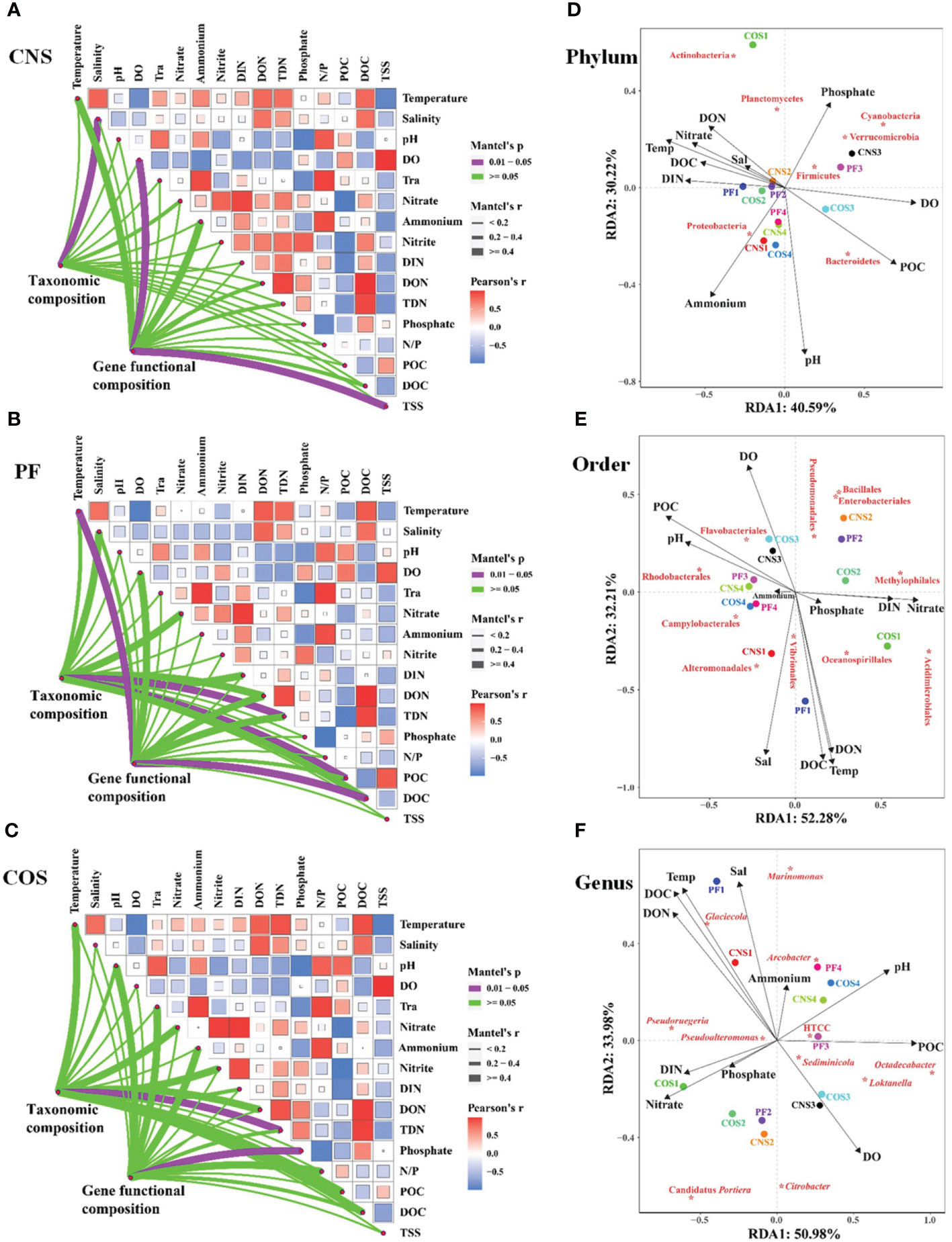
Figure 7 Correlation analysis between environmental factors and bacterial community. (1) Pairwise comparisons of environmental factors are shown with a color gradient denoting Pearson’s correlation coefficients in CNS (A), PF (B), and COS (C). Taxonomic (based on OTUs) and functional (based on KEGG modules) community composition were related to each environmental factor by partial Mantel tests. Mantel’s r represents the coefficient of correlation, in which thickness of the line represents the level of correlation and the color of the lines represents the Mantel’s p-value (purple line indicates p< 0.05). (2) Redundancy analysis (RDA) biplot of bacterial community in relation to environmental data at the phylum level (D), order level (E), and genus level (F). Species are labeled with asterisk. The closeness of these indicates correlation with each other and existence in similar environment. Only the environmental variables that significantly explained variability in bacterial community structures are fitted to the ordination and are shown by arrows; the relative length is positive correlation with the importance in influencing bacterial community structure. The angle between the arrow and the ordination axis suggests the variable response with respect to the RDA gradient. The sample abbreviations are as in Table 1. The abbreviations for the environmental variables are translated as follows: DO, dissolved oxygen; Tra, transparency; DIN, dissolved inorganic nitrogen; DON, dissolved organic nitrogen; TDN, total dissolved nitrogen; N/P, ratio of nitrogen to phosphorus; POC, particulate organic carbon; DOC, dissolved organic carbon; TSS, total suspended solids; Temp, temperature; Sal, salinity.
Redundancy analysis (RDA) was used to further discern the vital environmental factors shaping bacterial community structure. The RDA results showed that the first two RDA axes explained 70.81%, 84.49%, and 84.96% of the accumulated variance in the species-environment relation at the phylum level (Figure 7D), order level (Figure 7E), and genus level (Figure 7F), respectively. Of all the environmental factors examined, pH, DO, POC, DOC, temperature and key nutrient parameters (i. e. ammonium, phosphate, nitrate, DIN, DON) were the crucial environmental variables in shaping the bacterial community composition at the phylum level (Figure 7D). Similarly, temperature, salinity, pH, DO, DOC, POC, DON, nitrate, and DIN were the key factors affecting bacterial community composition at the order level (Figure 7E) and genus level (Figure 7F).
At the phylum level, Proteobacteria exhibited positive correlation with ammonium and pH, and negative correlation with phosphate. Three phyla (Firmicutes, Verrucomicrobia, and Cyanobacteria) correlated positively with phosphate and DO and negatively correlated with ammonium and pH (Figure 7D). Moreover, Bacteroidetes correlated positively with POC, pH, and DO, and negatively with temperature, DOC, and nutrient parameters (i. e. phosphate, nitrate, DIN, DON). Both DON and phosphate had significantly positive effect on Actinobacteria and Planctomycetes. At the order level (Figure 7E), the most dominant order Rhodobacterales exhibited positive relationship with POC and pH, and negative correlation with nutrient parameters (i. e. nitrate, DIN, DON and phosphate). Furthermore, the second and third dominant order (i. e. Alteromonadales and Oceanospirillales) showed positive associations with salinity, temperature, DOC, and DON. At the genus level (Figure 7F), the most dominant genus Pseudoruegeria was positively correlated with DIN, nitrate, phosphate, DOC, and DON, and negatively correlated with DO, POC and pH. The second dominant genus Glaciecola correlated negatively with DO and positively with temperature, DOC, DON, salinity, and ammonium. Besides, Sediminicola correlated positively with DO.
The associations among dominant bacterial taxa and environmental factors were further analyzed in the network diagram (Figure 8). The network comprised 109 significant associations (edges) of 36 nodes. Based on betweenness centrality scores, the keystone members of the bacterial communities were phylum Bacteroidetes, orders of Pseudomonadales, Rhodobacterales, and Flavobacteriales, and genera of Loktanella and Pseudoruegeria. POC showed strong positive associations with Rhodobacterales, Flavobacteriales, and Loktanella. Nitrate had the closest relationships with Actinobacteria, Methylophilales, and Pseudoruegeria. Temperature showed strong positive associations with Proteobacteria and Oceanospirillales. Both POC and TSS had significant influence on Bacteroidetes, and both DO and TSS exhibited significantly positive correlations with Loktanella. Moreover, TDN had significantly positive effect on Oceanospirillales and Pseudoruegeria.
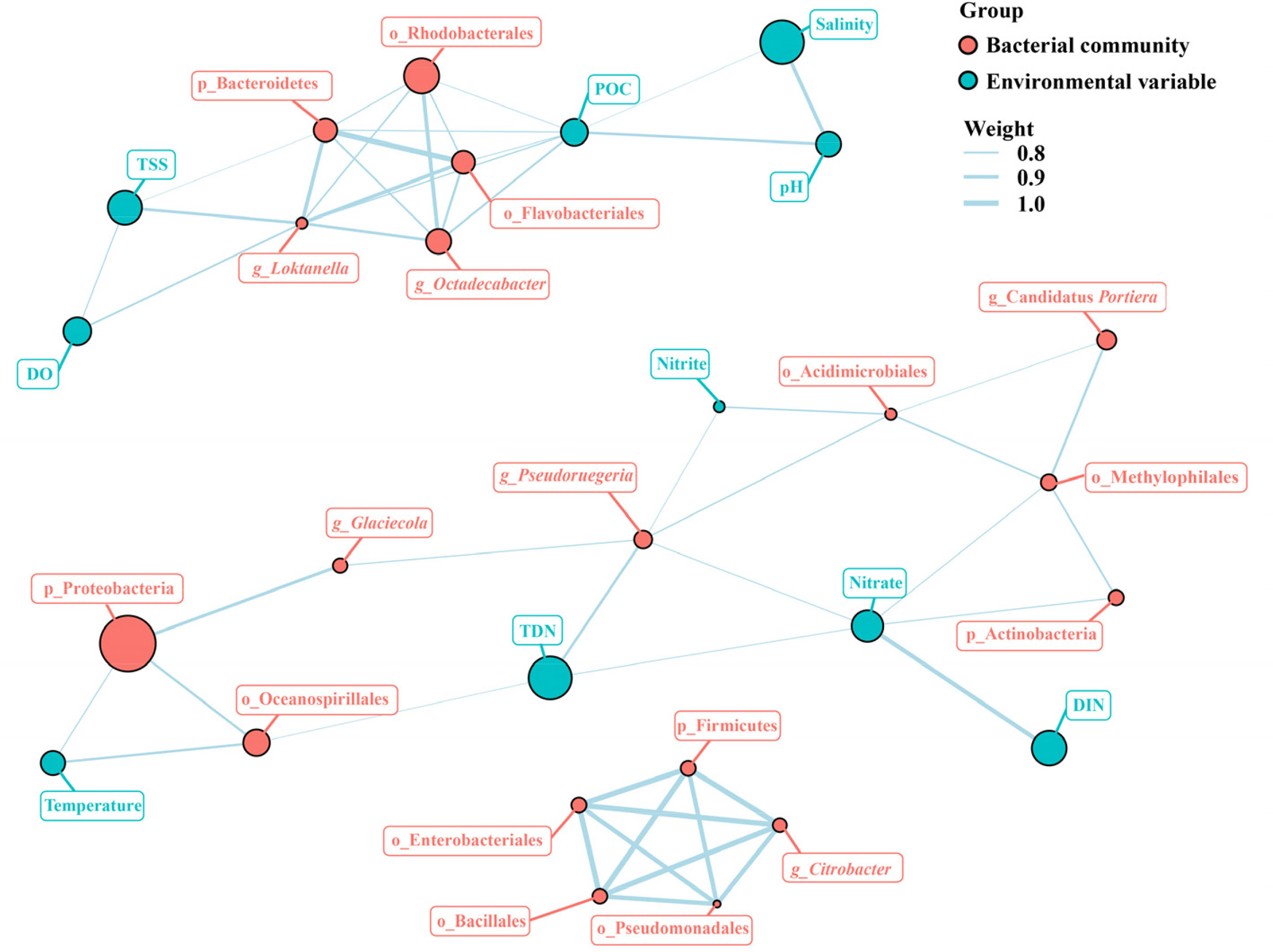
Figure 8 Network analysis of the major bacterial taxa and environmental factors. POC, particulate organic carbon; DO, dissolved oxygen; TSS, total suspended solids; DIN, dissolved inorganic nitrogen; DOC, dissolved organic carbon.
Discussion
Understanding characteristic changes in aquatic bacterial community composition over space and time can provide insight into the processes driving community assembly (Jones et al., 2012). Given the large-scale cultivation of laver and its ecological value, we used Illumina Miseq sequencing technology to analyze the spatio-temporal dynamics of environmental factors as well as bacterial communities and functions in the seawater in a typical laver farm (locating in Rizhao City, China), so that it would allow us to advance the comprehension of laver farming practices shaping microbial structures and functions.
Bacterial community richness and diversity
Biodiversity enhances ecosystem stability and productivity (Maron et al., 2018). The diversity-stability hypothesis states that systems with high diversity are more stable than systems with low diversity upon environmental fluctuation (McCann, 2000). Recent studies have shown that the microbial diversity in aquatic ecosystems is strongly dependent on physicochemical and anthropogenic factors (Shafi et al., 2017). The rapid growth of seaweed blades could provide new surface area for microbial colonization from the surrounding seawater, or other microbial reservoirs (Weigel and Pfister, 2019). Furthermore, Selvarajan et al. (2019) concluded that the diversity of epiphytic bacteria on seaweed surfaces is greatly influenced by algal organic exudates. The algal organic exudates would trigger chemical signaling and transportation responses from the microbial community (Ahmed et al., 2021). Some studies have found divergent microbial communities between seaweed cultivation zones and non-aquaculture zones (Hu et al., 2017; Xie et al., 2017; Zhao et al., 2018; Wang et al., 2020). For instance, Wang et al. (2020) found the microbial alpha-diversity (diversity, evenness, and richness) of seawater in laver cultivation area was significantly greater than that of non-cultivation seawater.
We found that both the bacterial community richness indices (ACE and Chao 1) and Shannon index (H’) of seawater in the near-shore non-farming area had obvious dynamic changes over time, while those in the laver farming area remained at relatively stable levels during the entire monitoring period (Figure 2). Moreover, bacterial equitability in the laver farming area also remained at a steadily high level from January to March, but that in offshore non-farming area decreased significantly in March. The physicochemical structures of water can result abiotically from microscale gradients in nutrients or chemicals (e.g. oxygen or light), or can be established biotically through the metabolic activity (Fenchel and Finlay, 2008; Schreiber and Ackermann, 2020; Liu et al., 2022). Structured environments would lead to more environmental gradients (i.e., habitats) (Fenchel and Finlay, 2008; Esteban et al., 2015; Quinn et al., 2018; Junkins et al., 2022). Therefore, the observed stable microbial diversity in the seawater may be a result of microbial community adaptations to the diverse physical-chemical habitats in the laver farm.
Bacterial community composition
The samples are not clearly grouped by spatial variations, but rather separated by temporal variation based on the PCA and cluster analysis in this study (Figure 3). The relative abundance of most bacteria showed generally marked temporal variations during laver cultivation. Lachnit et al. (2011) investigated the epiphytic bacterial patterns and their spatio-temporal variability on host algae, and demonstrate that macroalgae harbor species-specific and temporally adapted epiphytic bacterial biofilms on their surfaces. Proteobacteria, Bacteroidetes, Firmicutes, Actinobacteria, Cyanobacteria, and Verrucomicrobia were the dominant phyla in all seawater samples (Figure 4). Similarly, Hollants et al. (2013) pointed out that bacteria described from seaweed surfaces or within algal thalli generally belong to the phyla Proteobacteria, Actinobacteria, Bacteroidetes, Cyanobacteria, Firmicutes, Planctomycetes, Verrucomicrobia, Chloroflexi, Fusobacteria, etc. Epibiotic bacteria could be influenced directly by the surrounding water body (He et al., 2014). Meanwhile, macroalgae could modify the microbiota in their surroundings through the dispersal of epibiotic microbes directly into the water column or on particles of degrading algal tissue (Chen and Parfrey, 2018). It was reported that some members of Rhodobacteraceae, Gammaproteobacteria and Bacteriodetes displayed considerable temporal variation, often with similar trends in the seawater and the surface of the kelp (Michelou et al., 2013). Besides, Ahmed et al. (2021) also found that the profile of the bacterial community on laver thallus and the surrounding seawater showed spatial resemblance and variation at the same time.
In this study, Proteobacteria had the highest contributions in the seawater samples, with the dominance of Gammaproteobacteria and Alphaproteobacteria (Figure 4). Gammaproteobacteria are the most common bacterial clade associated with seaweeds (Hollants et al., 2013). They play an important role in the degradation and absorption of organic compounds, ammonia and sulfide (Zhang et al., 2020a). Members of Alphaproteobacteria contribute significantly to global sulphur cycling (Malmstrom et al., 2004). In addition, a wide variety of bacterial species isolated from seaweeds are capable of assimilating algal cell wall sugars, and these algal cell wall degrading bacteria mainly belong to the Alphaproteobacteria, Gammaproteobacteria, and Bacteroidetes (Hollants et al., 2013). Moreover, Rhodobacterales and Rhodobacteraceae (both belonging to Alphaproteobacteria) were the most predominant order and family respectively in this study (Figure 4). Rhodobacteraceae are generally aquatic bacteria that frequently thrive in marine environments (Pujalte et al., 2014).
Temporal changes of bacterial communities and specific bacterial taxa
Biotic and/or abiotic factors have been extensively reported to affect community assembly and stability to different extents (Langenheder and Lindström, 2019). Seasonal alteration is one of the most representative environmental variations (e.g., temperature and precipitation) that affect microbial communities (Liu et al., 2022). The competitive advantage between microorganisms changes under conditions such as temperature changes (Zhang et al., 2020a). The maximum seawater temperatures (9.97–11.20°C) occurred in November, and the minimum seawater temperatures (5.03–5.53°C) in February in the study (Table S1). The abundance of Octadecabacter and Loktanella were significantly higher from January to March (Figure 4). Compared to January, the genera of Coraliomargarita, Psychromonas, Octadecabacter, Loktanella, and Polaribacter were taxonomic biomarkers in February (Figure 5). It is interesting to note that members of Psychromonas, Octadecabacter, Loktanella, and Polaribacter are known for their psychrophilic characters (Morita and Moyer, 2001; Auman et al., 2010; Angelaccio et al., 2012; Vollmers et al., 2013; Pujalte et al., 2014; Alvarado and Leiva, 2017). Psychrophilic bacteria synthesize cold-active enzymes to sustain their cell cycle (Feller, 2013). Moreover, the function of samples collected in February exhibited a significant higher abundance of metabolism of cofactors and vitamins (Figure 6). These findings suggest that a wide range of enzyme-catalyzed reactions may occur in the psychrophilic bacteria to drive their microbial metabolism for adapting to the cold environment in the laver farm system.
Compared to January, the genera of Marinomonas, Glaciecola, Pseudoruegeria, and Pseudoalteromonas appeared as biomarkers in November (Figure 5). It is interesting to found that most of these bacterial biomarker taxa might be associated with degradation of algal cell wall polysaccharides (e.g. agar, cellulose, xylan). For example, many Pseudoalteromonas strains have been described to have agarolytic activities (Vera et al., 1998; Holmström and Kjelleberg, 1999; Romanenko et al., 2003b; Khalifa and Aldayel, 2019). Some Glaciecola strains exhibited agar-digesting activities (Romanenko et al., 2003a; Yong et al., 2007) and was capable of efficiently hydrolyzing cellulose and xylan (Klippel et al., 2011). Yu et al. (2020) reported that a strain of Marinomonas exhibited agar-degrading activity. Based on these studies, we deduce that laver was a rich source of agarase-producing bacteria.
The abundance of Firmicutes increased significantly in the middle stage during laver cultivation (from January to February) (Figure 4). Genera of Marinomonas and Arcobacter were representative biomarkers in March as compared to February (Figure 5). Compared to November, genera of Octadecabacter and Citrobacter appeared as the representative biomarkers in January (Figure 5). These bacterial taxa exhibit important ecological functions. For example, Firmicutes from a coastal marine sediment fermented almost all the high quality carbon substrates (glucose and amino acids) (Chen et al., 2017); Members of Marinomonas are known for their environmental adaptation and metabolically versatility, with an abundance of antifreeze proteins, osmotic pressure resistance, carbohydrase, and a variety of secondary metabolites (Xue et al., 2022); Arcobacter possess genes encoding enzymes to catabolize dimethylsulfoniopropionate (DMSP), which is a secondary metabolite produced in large quantities by marine algae and is a key component of the global geochemical sulfur cycle (Wesley, 2014; Zeng, 2019); Some members of Octadecabacter are psychrophilic (Vollmers et al., 2013) and also possess genes for DMSP degradation (Zeng and Qiao, 2019); Citrobacter species are strictly aerobic, and some of them are aerobic denitrifiers, which could convert nitrate or ammonium ion to nitrite, thereby playing important role in the nitrogen cycle (Huang and Tseng, 2001; Ko et al., 2015). Moreover, we found that the most abundant functional categories of the bacterial communities were related to carbohydrate metabolism, membrane transport, signal transduction, amino acid metabolism, nucleotide metabolism, and energy metabolism in all samples (Figure 6). Similarly, Ahmed et al. (2021) showed that the complex and diverse associations of microbial communities with laver and surrounding seawater displayed an exceptional transport, metabolic, and biosynthetic functions. In view of these findings, the bacterial biomarker taxa among different months were likely to function in the carbon, nitrogen, or sulfur cycles through their diverse metabolic activities in the laver culture system.
Spatial changes of bacterial communities and specific bacterial taxa
The relative abundance of bacteria showed marked spatial variations in November (Figure 4). Membrane transport, cell growth and death, as well as xenobiotics biodegradation and metabolism were the representative functional biomarkers in CNS1 group (Figure 6). This finding indicated that the water runoff was likely to bring a large amount of potential pollutants (e.g. pesticides, metals) into the near-shore sea area in November, which may trigger chemotaxis responses from bacteria with the requisite genes to metabolise these substrates. One of the most relevant factors to bacterial growth and reproduction is inorganic nutrition and algae biomass in aquatic ecosystems (Zhang et al., 2020b). For example, a unique shift in the kelp surface microbiome was associated with changes of kelp growth (Minich et al., 2018). Hence, we propose that the large increase in laver biomass was the important biotic factor contributing to spatial variation of microbial community composition in November in this study.
The LEfSe analysis showed metabolism of cofactors and vitamins, translation, nucleotide metabolism, cell motility, carbohydrate metabolism, replication and repair, and transport and catabolism were representative biomarkers in groups of PF1 and PF4 (Figure 6). This finding suggest that a significant increase in metabolic exchange and transformation nutrients occurred in the seawater in the laver farm during the early and late stage of laver cultivation. Cells at the edge of an intact laver thallus can differentiate into archeospores during early growth stage of N. yezoensis (Chen et al., 2020). The mature N. yezoensis may release zygotospores during late stage of cultivation. After reproduction, a lot of tissue at the edge of laver thallus would release into the seawater. Hence, we speculate that the laver reproductive activities (i.e. the formation/release activities of archeospores and zygotospores during the early and late stage of laver cultivation respectively) were probably accompanied by decomposition of a large amount of laver tissue, thus providing a large influx of carbon/nitrogen to the seawater. This process may promote the assimilation and utilization of nutrition by bacteria and driving the variation of metabolic functional diversity of bacterial communities. But more evidence is needed to support this assumption.
The abundance of Alteromonadales in the laver farming area increased significantly compared to that in the two non-farming areas in February (Figure 4). The families Oceanospirillaceae and Alteromonadaceae (both belonging to Gammaproteobacteria) were found as biomarkers in the laver farming area (Figure 5), which was in agreement with the result of Wang et al. (2020). Both members of Alteromonadales and Oceanospirillaceae have been shown to support algal growth through the synthesis of vitamin B12 (Delmont et al., 2015; Joglar et al., 2021). Ecologically, Alteromonadaceae are often associated to nutrient-rich environments (López-Pérez and Rodriguez-Valera, 2014). Alteromonadales also responded rapidly to changes in DOM (Shi et al., 2011). Furthermore, it was reported that some marine bacteria from the family Alteromonadaceae exhibited an ability of degradation metabolism of plant cell wall polysaccharides (López-Pérez and Rodriguez-Valera, 2014; Wietz et al., 2015; Mitulla et al., 2016). The polysaccharides (agar, alginate, carrageenan, cellulose, etc.) that compose the bulk of macroalgal biomass are rich sources of energy and carbon that can promote epibiont settlement and growth (Steinberg and De Nys, 2002; Lachnit et al., 2011). For example, Saccharophagus (belonging to Alteromonadaceae) has been shown to utilize a large set of sugars and polysaccharides as carbon and energy source (Shin et al., 2010; Fraiberg et al., 2011). Wietz et al. (2015) showed that the addition of soluble alginate to seawater induced a strong increase in Alteromonadaceae. Together these findings hint that the mutualistic relationship between laver and bacteria probably has a strong influence on the spatial changes of representative biomarkers.
Correlation among environmental factors and bacterial community
The concentrations of inorganic nitrogen and phosphorus in the near-shore waters were higher and varied monthly in the study (Table S1), which were probably be attributed to the anthropogenic flux of nutrients from the estuary and the monthly changes in discharge of estuary. Inorganic carbon, nitrogen and phosphorus are the main elements required by seaweeds for photosynthesis and growth (Roleda and Hurd, 2019). Changes in discharge flow would led to shifts in the nutrient and stoichiometric balance in an estuary, which could influence the laver production (Kim et al., 2019). The representative biomarkers in CNS group (e.g. Pseudoruegeria and Sediminicola, Figure 5) were found to be significantly associated with DO concentration based on RDA (Figure 7). Correspondingly, DO was one of the main environmental drivers for functional community composition in CNS group by Mantel test (Figure 7). As excessive nitrogen, aromatic compounds and other pollutants enter the aquatic environment, bacteria consume more oxygen to participate in nitrogen transformation and organic matter degradation (Liu et al., 2021). Furthermore, we found salinity was the main environmental driver for taxonomic community composition in CNS group based on Mantel test (Figure 7). The salinity varied geographically with an increasing trend from near-shore to off-shore sea areas (Table S1), likely owing to the influence of discharge of Jufeng estuary. The salinity gradient is one of the main features characteristic of any estuarine ecosystem (Telesh and Khlebovich, 2010) and plays a unique and fundamental role in structuring spatial patterns of physical properties, biota, and biogeochemical processes (Cloern et al., 2017). Taken together, it is reasonable to assume that microbial community composition in the near-shore sea area were affected significantly by water runoff.
The organic matter is a carrier of large quantities of carbon and nitrogen (Ye et al., 2018). Both algae and riverine are also key organic matter sources in the coastal zone (Ray et al., 2018). Higher concentrations of DOC and DON were observed in November in the study (Table S1), which were probably derived from the releasing laver tissue or may be partly owing to the cumulative influence of changes in phytoplankton abundance and discharge of estuary (Liang et al., 2019b). Algae might excrete a portion of photosynthetic organic matter as DOC, and heterotrophic bacteria assimilate and decompose the algal DOC for growth (Egan et al., 2013; Clasen and Shurin, 2015; Kouzuma and Watanabe, 2015). Based on Mantel test, we found the taxonomic/functional community compositions were significantly correlated with TDN, POC, and DOC in group PF (Figure 7), indicating that the bacterial community composition was significantly affected by nitrogen and carbon availability in the laver farm. Besides, we aslo found pH, DO, POC, DOC, temperature and key nutrient parameters (i. e. ammonium, phosphate, nitrate, DIN, DON) were the crucial environmental variables at the phylum level based on RDA (Figure 7). The most dominant phylum Proteobacteria exhibited positive correlation with ammonium and pH, and negative correlation with phosphate. These RDA results further indicated the environmental variables such as key nitrogen parameters (e.g. nitrate, DIN, DON, TDN) and pH, DO, POC, DOC, and temperature were crucial in shaping the bacterial community composition in the surrounding seawater of laver farm.
Based on network analysis, the keystone members of the bacterial communities were phylum Bacteroidetes, orders of Pseudomonadales, Rhodobacterales, and Flavobacteriales, and genera of Loktanella and Pseudoruegeria (Figure 8). Keystone taxa are the species playing crucial roles in a community and their removal can lead to a notable variance in community structure and functioning (Mouquet et al., 2013). It was suggested that seasonal variations of environmental factors could influence microbial networks and community stability via the regulation of keystone taxa (Liu et al., 2022).
Conclusion
The results of our investigation indicated that large-scale laver aquaculture affects taxonomic diversity, composition, and function of seawater bacteria communities. Laver cultivation was likely to be beneficial to maintain seasonal stability of microbial community richness and diversity in the laver farm ecosystem. Many bacterial biomarker taxa with psychrophilic characters or with an ability of degrading algal cell wall polysaccharides were found in the samples. The bacterial biomarkers were probably closely associated with temperature, concentrations of DO and ammonium, as well as the secondary metabolite produced by laver. The variation of metabolic functional diversity of bacterial communities in the seawater were proposed to be promoted by the laver reproductive activities during the early and late stage of large-scale laver cultivation. However, further long-term temporal observations will be needed to develop a general understanding of inter-annual bacterial community composition variation. Future work should also focus on the ecology and metabolic functions of the predominant species or bacterial biomarker taxa in the laver farm.
Data availability statement
The datasets presented in this study can be found in online repositories. The names of the repository/repositories and accession number(s) can be found below: https://www.ncbi.nlm.nih.gov/, PRJNA841061.
Author contributions
ZL: Writing - Original Draft, Conceptualization, Investigation, Visualization. RJ: Writing - Original Draft, Methodology, Validation. TS: Investigation, Validation, Visualization. WW: Supervision, Project administration, Writing - Review & Editing. CW: Resources, Writing - Review & Editing. XL: Methodology, Resources. All authors contributed to the article and approved the submitted version.
Funding
This work was supported by the National Key R&D Program of China ‘Scientific and Technological Innovation of Blue Granary’ (2018YFD0901504), the earmarked fund for CARS-50, Shandong Province Key Research and Development Program (2021LZGC004), Shandong Agricultural Good Seed Project (South to North) (2017LZN013), Primary Research & Development Plan of Shandong Province (2016GSF115038), Central Public-interest Scientific Institution Basal Research Fund (2020TD27).
Conflict of interest
The authors declare that the research was conducted in the absence of any commercial or financial relationships that could be construed as a potential conflict of interest.
Publisher’s note
All claims expressed in this article are solely those of the authors and do not necessarily represent those of their affiliated organizations, or those of the publisher, the editors and the reviewers. Any product that may be evaluated in this article, or claim that may be made by its manufacturer, is not guaranteed or endorsed by the publisher.
Supplementary material
The Supplementary Material for this article can be found online at: https://www.frontiersin.org/articles/10.3389/fmars.2022.1056199/full#supplementary-material
References
Ahmed A., Khurshid A., Tang X., Wang J., Khan T. U., Mao Y. (2021). Structural and functional impacts of microbiota on Pyropia yezoensis and surrounding seawater in cultivation farms along coastal areas of the yellow Sea. Microorganisms 9, 1291. doi: 10.3390/microorganisms9061291
Alvarado R., Leiva S. (2017). Agar-degrading bacteria isolated from Antarctic macroalgae. Folia Microbiol. (Praha). 62, 409–416. doi: 10.1007/s12223-017-0511-1
Angelaccio S., Florio R., Consalvi V., Festa G., Pascarella S. (2012). Serine hydroxymethyltransferase from the cold adapted microorganism Psychromonas ingrahamii: a low temperature active enzyme with broad substrate specificity. Int. J. Mol. Sci. 13, 1314–1326. doi: 10.3390/ijms13021314
Auman A. J., Breezee J. L., Gosink J. J., Schumann P., Barnes C. R., Kämpfer P., et al. (2010). Psychromonas boydii sp. nov., a gas-vacuolate, psychrophilic bacterium isolated from an Arctic sea-ice core. Int. J. Syst. Evol. Microbiol. 60, 84–92. doi: 10.1099/ijs.0.007773-0
Briones A., Raskin L. (2003). Diversity and dynamics of microbial communities in engineered environments and their implications for process stability. Curr. Opin. Biotechnol. 14, 270–276. doi: 10.1016/S0958-1669(03)00065-X
Butler S., O’Dwyer J. P. (2018). Stability criteria for complex microbial communities. Nat. Commun. 9, 2970. doi: 10.1038/s41467-018-05308-z
Callieri C., Eckert E. M., Di Cesare A., Bertoni F. (2019). “Microbial communities,” in Encyclopedia of ecology (New York, Elsevier), 126–134. doi: 10.1016/B978-0-12-409548-9.11222-9
Caporaso J. G., Kuczynski J., Stombaugh J., Bittinger K., Bushman F. D., Costello E. K., et al. (2010). QIIME allows analysis of high-throughput community sequencing data. Nat. Methods 7, 335–336. doi: 10.1038/nmeth.f.303
Chen J., Hanke A., Tegetmeyer H. E., Kattelmann I., Sharma R., Hamann E., et al. (2017). Impacts of chemical gradients on microbial community structure. ISME. J. 11, 920–931. doi: 10.1038/ismej.2016.175
Chen M. Y., Parfrey L. W. (2018). Incubation with macroalgae induces large shifts in water column microbiota, but minor changes to the epibiota of co-occurring macroalgae. Mol. Ecol. 27, 1966–1979. doi: 10.1111/mec.14548
Chen N. C., Tang L., Guan X. W., Chen R., Cao M., Mao Y. X., et al. (2020). Thallus sectioning as an efficient monospore release method in Pyropia yezoensis (Bangiales, rhodophyta). J. Appl. Phycol. 32, 2195–2200. doi: 10.1007/s10811-019-01992-6
Clasen J. L., Shurin J. B. (2015). Kelp forest size alters microbial community structure and function on Vancouver island, Canada. Ecology 96, 862–872. doi: 10.1890/13-2147.1
Cloern J. E., Jassby A. D., Schraga T. S., Nejad E., Martin C. (2017). Ecosystem variability along the estuarine salinity gradient: examples from long-term study of San Francisco bay. Limnol. Oceanogr. 62, 272–291. doi: 10.1002/lno.10537
Comba González N. B., Niño Corredor A. N., López Kleine L., Montoya Castaño D. (2021). Temporal changes of the epiphytic bacteria community from the marine macroalga Ulva lactuca (Santa Marta, Colombian-Caribbean). Curr. Microbiol. 78, 534–543. doi: 10.1007/s00284-020-02302-x
Delmont T. O., Eren A. M., Vineis J. H., Post A. F. (2015). Genome reconstructions indicate the partitioning of ecological functions inside a phytoplankton bloom in the amundsen Sea, Antarctica. Front. Microbiol. 6, 1090. doi: 10.3389/fmicb.2015.01090
de Mesquita M. M. F., Crapez M. A. C., Teixeira V. L., Cavalcanti D. N. (2019). Potential interactions bacteria-brown algae. J. Appl. Phycol. 31, 867–883. doi: 10.1007/s10811-018-1573-4
Douglas G. M., Beiko R. G., Langille M. G. I. (2018). “Predicting the functional potential of the microbiome from marker genes using PICRUSt,” in Microbiome analysis: methods and protocols methods in molecular biology. Eds. Beiko R. G., Hsiao W., Parkinson J. (New York, NY: Springer), 169–177. doi: 10.1007/978-1-4939-8728-3_11
Egan S., Harder T., Burke C., Steinberg P., Kjelleberg S., Thomas T. (2013). The seaweed holobiont: Understanding seaweed–bacteria interactions. FEMS Microbiol. Rev. 37, 462–476. doi: 10.1111/1574-6976.12011
Esteban D. J., Hysa B., Bartow-McKenney C. (2015). Temporal and spatial distribution of the microbial community of winogradsky columns. PloS One 10, e0134588. doi: 10.1371/journal.pone.0134588
FAO (2016). The state of world fisheries and aquaculture 2016 (Rome, Food and Agriculture Organization of the United Nations).
Feller G. (2013). Psychrophilic enzymes: from folding to function and biotechnology. Scientifica 2013, 512840. doi: 10.1155/2013/512840
Fenchel T., Finlay B. (2008). Oxygen and the spatial structure of microbial communities. Biol. Rev 83, 553–569. doi: 10.1111/j.1469-185X.2008.00054.x
Fierer N., Jackson R. B. (2006). The diversity and biogeography of soil bacterial communities. Proc. Natl. Acad. Sci. 103, 626–631. doi: 10.1073/pnas.0507535103
Fraiberg M., Borovok I., Bayer E. A., Weiner R. M., Lamed R. (2011). Cadherin domains in the polysaccharide-degrading marine bacterium Saccharophagus degradans 2-40 are carbohydrate-binding modules. J. Bacteriol. 193, 283–285. doi: 10.1128/JB.00842-10
Fulbright S. P., Robbins-Pianka A., Berg-Lyons D., Knight R., Reardon K. F., Chisholm S. T. (2018). Bacterial community changes in an industrial algae production system. Algal. Res. 31, 147–156. doi: 10.1016/j.algal.2017.09.010
Ghaderiardakani F., Quartino M. L., Wichard T. (2020). Microbiome-dependent adaptation of seaweeds under environmental stresses: A perspective. Front. Mar. Sci. 7, 575228. doi: 10.3389/fmars.2020.575228
Good I. J. (1953). The population frequencies of species and the estimation of population parameters. Biometrika 40, 237–264. doi: 10.1093/biomet/40.3-4.237
Goswami M., Bhattacharyya P., Mukherjee I., Tribedi P. (2017). Functional diversity: an important measure of ecosystem functioning. Adv. Microbiol. 7, 82–93. doi: 10.4236/aim.2017.71007
Guan X. Y., Zhou W., Hu C. M., Zhu M. X., Ding Y., Gai S. S., et al. (2018). Bacterial community temporal dynamics and disease-related variations in the seawater of Pyropia (laver) seedling pools. J. Appl. Phycol. 30, 1217–1224. doi: 10.1007/s10811-017-1272-6
Gu Y., Wang J. H., Cai W. J., Li G. L., Mei Y., Yang S. H. (2021). Different amounts of nitrogen fertilizer applications alter the bacterial diversity and community structure in the rhizosphere soil of sugarcane. Front. Microbiol. 12. doi: 10.3389/fmicb.2021.721441
He D., Ren L., Wu Q. L. (2014). Contrasting diversity of epibiotic bacteria and surrounding bacterioplankton of a common submerged macrophyte, Potamogeton crispus, in freshwater lakes. FEMS Microbiol. Ecol. 90, 551–562. doi: 10.1111/1574-6941.12414
Hollants J., Leliaert F., De Clerck O., Willems A. (2013). What we can learn from sushi: A review on seaweed-bacterial associations. FEMS Microbiol. Ecol. 83, 1–16. doi: 10.1111/j.1574-6941.2012.01446.x
Holmström C., Kjelleberg S. (1999). Marine pseudoalteromonas species are associated with higher organisms and produce biologically active extracellular agents. FEMS Microbiol. Ecol. 30, 285–293. doi: 10.1111/j.1574-6941.1999.tb00656.x
Huang H. K., Tseng S. K. (2001). Nitrate reduction by Citrobacter diversus under aerobic environment. Appl. Microbiol. Biotechnol. 55, 90–94. doi: 10.1007/s002530000363
Hu X., Wen G., Cao Y., Gong Y., Li Z., He Z., et al. (2017). Metabolic and phylogenetic profiles of microbial communities from a mariculture base on the Chinese guangdong coast. Fish. Sci. 83, 465–477. doi: 10.1007/s12562-017-1073-5
Joglar V., Pontiller B., Martínez-García S., Fuentes-Lema A., Pérez-Lorenzo M., Lundin D., et al. (2021). Microbial plankton community structure and function responses to vitamin B12 and B1 amendments in an upwelling system. Appl. Environ. Microbiol. 87, e0152521. doi: 10.1128/AEM.01525-21
Jones S. E., Cadkin T. A., Newton R. J., McMahon K. D. (2012). Spatial and temporal scales of aquatic bacterial beta diversity. Front. Microbiol. 3. doi: 10.3389/fmicb.2012.00318
Junkins E. N., McWhirter J. B., McCall L. I., Stevenson B. S. (2022). Environmental structure impacts microbial composition and secondary metabolism. ISME. Commun. 2, 15. doi: 10.1038/s43705-022-00097-5
Khalifa A., Aldayel M. (2019). Isolation and characterisation of the agarolytic bacterium Pseudoalteromonas ruthenica. Open Life Sci. 14, 588–594. doi: 10.1515/biol-2019-0066
Kim J. K., Yarish C., Hwang E. K., Park M., Kim Y. (2017). Seaweed aquaculture: cultivation technologies, challenges and its ecosystem services. Algae 32, 1–13. doi: 10.4490/algae.2017.32.3.3
Kim S., Yoon S. C., Yoo M. H., Park K. W., Park S. R., Youn S.-H. (2019). Physiological responses of cultured seaweed Pyropia yezoensis to phosphorous limitation in the nakdong river estuary, Korea. Ocean. Sci. J. 54, 129–139. doi: 10.1007/s12601-018-0065-4
Klippel B., Lochner A., Bruce D. C., Davenport K. W., Detter C., Goodwin L. A., et al. (2011). Complete genome sequence of the marine cellulose- and xylan-degrading bacterium glaciecola sp. strain 4H-3-7+YE-5. J. Bacteriol. 193, 4547–4548. doi: 10.1128/JB.05468-11
Ko K. S., Choi J. Y., Kim J., Park M. K. (2015). Citrobacter bitternis sp. nov. isolated from bitterns. Curr. Microbiol. 70, 894–897. doi: 10.1007/s00284-015-0810-1
Kouzuma A., Watanabe K. (2015). Exploring the potential of algae/bacteria interactions. Curr. Opin. Biotechnol. 33, 125–129. doi: 10.1016/j.copbio.2015.02.007
Lachnit T., Meske D., Wahl M., Harder T., Schmitz R. (2011). Epibacterial community patterns on marine macroalgae are host-specific but temporally variable: epiphytic communities on macroalgae. Environ. Microbiol. 13, 655–665. doi: 10.1111/j.1462-2920.2010.02371.x
Langenheder S., Lindström E. S. (2019). Factors influencing aquatic and terrestrial bacterial community assembly. Environ. Microbiol. Rep. 11, 306–315. doi: 10.1111/1758-2229.12731
Liang Z. R., Liu F. L., Wang W. J., Zhang P. Y., Sun X. T., Wang F. J., et al. (2019a). High-throughput sequencing revealed differences of microbial community structure and diversity between healthy and diseased Caulerpa lentillifera. BMC Microbiol. 19, 225. doi: 10.1186/s12866-019-1605-5
Liang Z. R., Sun T. Q., Wang W. J., Liu F. L., Cao Y., Liang Q. D., et al. (2019b). The temporal and spatial distribution characteristics of nutrient and community structure of phytoplankton and their relationship in the Pyropia culture area in rizhao. Prog. Fish. Sci. 40, 78–88. doi: 10.19663/j.issn2095-9869.20180702001
Liang Z. R., Wang W. J., Liu L. L., Li G. L., Xia B. (2022). Influence of commercial-scale seaweed cultivation on water quality: A case study in a typical laver culture area of the yellow Sea, north China. J. Mar. Sci. Eng. 10, 681. doi: 10.3390/jmse10050681
Li F., Chen L., Zhang J. B., Yin J., Huang S. M. (2017). Bacterial community structure after long-term organic and inorganic fertilization reveals important associations between soil nutrients and specific taxa involved in nutrient transformations. Front. Microbiol. 8. doi: 10.3389/fmicb.2017.00187
Liu X. Y., Hu S. H., Sun R., Wu Y. G., Qiao Z. X., Wang S. C., et al. (2021). Dissolved oxygen disturbs nitrate transformation by modifying microbial community, co-occurrence networks, and functional genes during aerobic-anoxic transition. Sci. Total. Environ. 790, 148245. doi: 10.1016/j.scitotenv.2021.148245
Liu S. W., Yu H., Yu Y. H., Huang J., Zhou Z., Zeng J. X., et al. (2022). Ecological stability of microbial communities in lake donghu regulated by keystone taxa. Ecol. Indic. 136, 108695. doi: 10.1016/j.ecolind.2022.108695
Liu Q. Q., Zhi Y. J., He Y. Y., Ren Z. M., Chen H. M., Yang R. (2020). Changes in phycospheric and environmental microbes associated with an outbreak of yellow spot disease on Pyropia yezoensis. Aquaculture 529, 735651. doi: 10.1016/j.aquaculture.2020.735651
López-Pérez M., Rodriguez-Valera F. (2014). “The family alteromonadaceae,” in The prokaryotes. Eds. Rosenberg E., DeLong E. F., Lory S., Stackebrandt E., Thompson F. (Berlin, Heidelberg: Springer Berlin Heidelberg), 69–92. doi: 10.1007/978-3-642-38922-1_233
Malmstrom R. R., Kiene R. P., Kirchman D. L. (2004). Identification and enumeration of bacteria assimilating dimethylsulfoniopropionate (DMSP) in the north Atlantic and gulf of Mexico. Limnol. Oceanogr. 49, 597–606. doi: 10.4319/lo.2004.49.2.0597
Maron P. A., Sarr A., Kaisermann A., Lévêque J., Mathieu O., Guigue J., et al. (2018). High microbial diversity promotes soil ecosystem functioning. Appl. Environ. Microbiol. 84, e02738–e02717. doi: 10.1128/AEM.02738-17
Michelou V. K., Caporaso J. G., Knight R., Palumbi S. R. (2013). The ecology of microbial communities associated with Macrocystis pyrifera. PloS One 8, e67480. doi: 10.1371/journal.pone.0067480
Minich J. J., Morris M. M., Brown M., Doane M., Edwards M. S., Michael T. P., et al. (2018). Elevated temperature drives kelp microbiome dysbiosis, while elevated carbon dioxide induces water microbiome disruption. PloS One 13, e0192772. doi: 10.1371/journal.pone.0192772
Miranda L. N., Hutchison K., Grossman A. R., Brawley S. H. (2013). Diversity and abundance of the bacterial community of the red macroalga Porphyra umbilicalis: Did bacterial farmers produce macroalgae? PloS One 8, e58269. doi: 10.1371/journal.pone.0058269
Mitulla M., Dinasquet J., Guillemette R., Simon M., Azam F., Wietz M. (2016). Response of bacterial communities from California coastal waters to alginate particles and an alginolytic alteromonas macleodii strain. Environ. Microbiol. 18, 4369–4377. doi: 10.1111/1462-2920.13314
Morita R. Y., Moyer C. L. (2001). “Psychrophiles,” in Encyclopedia of biodiversity (Second edition). Ed. Levin S. A. (Waltham: Academic Press), 298–303. doi: 10.1016/B978-0-12-384719-5.00176-3
Mouquet N., Gravel D., Massol F., Calcagno V. (2013). Extending the concept of keystone species to communities and ecosystems. Ecol. Lett. 16, 1–8. doi: 10.1111/ele.12014
Pujalte M. J., Lucena T., Ruvira M. A., Arahal D. R., Macián M. C. (2014). “The family rhodobacteraceae,” in The prokaryotes. Eds. Rosenberg E., DeLong E. F., Lory S., Stackebrandt E., Thompson F. (Berlin, Heidelberg: Springer Berlin Heidelberg), 439–512. doi: 10.1007/978-3-642-30197-1_377
Quinn R. A., Comstock W., Zhang T., Morton J. T., da Silva R., Tran A., et al. (2018). Niche partitioning of a pathogenic microbiome driven by chemical gradients. Sci. Adv. 4, eaau1908. doi: 10.1126/sciadv.aau1908
Ray R., Michaud E., Aller R. C., Vantrepotte V., Gleixner G., Walcker R., et al. (2018). The sources and distribution of carbon (DOC, POC, DIC) in a mangrove dominated estuary (French Guiana, south America). Biogeochemistry 138, 297–321. doi: 10.1007/s10533-018-0447-9
Ren C. G., Liu Z. Y., Wang X. L., Qin S. (2022). The seaweed holobiont: from microecology to biotechnological applications. Microb. Biotechnol. 15, 738–754. doi: 10.1111/1751-7915.14014
Reshef D. N., Reshef Y. A., Finucane H. K., Grossman S. R., McVean G., Turnbaugh P. J., et al. (2011). Detecting novel associations in large data sets. Science 334, 1518–1524. doi: 10.1126/science.1205438
Roleda M. Y., Hurd C. L. (2019). Seaweed nutrient physiology: application of concepts to aquaculture and bioremediation. Phycologia 58, 552–562. doi: 10.1080/00318884.2019.1622920
Romanenko L. A., Zhukova N. V., Rohde M., Lysenko A. M., Mikhailov V. V., Stackebrandt E. (2003a). Glaciecola mesophila sp. nov., a novel marine agar-digesting bacterium. Int. J. Syst. Evol. Microbiol. 53, 647–651. doi: 10.1099/ijs.0.02469-0
Romanenko L. A., Zhukova N. V., Rohde M., Lysenko A. M., Mikhailov V. V., Stackebrandt E. (2003b). Pseudoalteromonas agarivorans sp. nov., a novel marine agarolytic bacterium. Int. J. Syst. Evol. Microbiol. 53, 125–131. doi: 10.1099/ijs.0.02234-0
Schreiber F., Ackermann M. (2020). Environmental drivers of metabolic heterogeneity in clonal microbial populations. Curr. Opin. Biotechnol. 62, 202–211. doi: 10.1016/j.copbio.2019.11.018
Segata N., Izard J., Waldron L., Gevers D., Miropolsky L., Garrett W. S., et al. (2011). Metagenomic biomarker discovery and explanation. Genome Biol. 12, R60. doi: 10.1186/gb-2011-12-6-r60
Selvarajan R., Sibanda T., Venkatachalam S., Ogola H. J. O., Christopher Obieze C., Msagati T. A. (2019). Distribution, interaction and functional profiles of epiphytic bacterial communities from the rocky intertidal seaweeds, south Africa. Sci. Rep. 9, 19835. doi: 10.1038/s41598-019-56269-2
Shafi S., Kamili A. N., Shah M. A., Parray J. A., Bandh S. A. (2017). Aquatic bacterial diversity: Magnitude, dynamics, and controlling factors. Microb. Pathog. 104, 39–47. doi: 10.1016/j.micpath.2017.01.016
Shen M. L., Yang R., Luo Q. J., Wang S. G., Ren J. R. (2013). Microbial diversity of Pyropia haitanensis phycosphere during cultivation. Acta Microbiol. Sin. 53, 1087–1102. doi: 10.13343/j.cnki.wsxb.2013.10.007
Shin M. H., Lee D. Y., Skogerson K., Wohlgemuth G., Choi I. G., Fiehn O., et al. (2010). Global metabolic profiling of plant cell wall polysaccharide degradation by Saccharophagus degradans. Biotechnol. Bioeng. 105, 477–488. doi: 10.1002/bit.22557
Shi Y., Tyson G. W., Eppley J. M., DeLong E. F. (2011). Integrated metatranscriptomic and metagenomic analyses of stratified microbial assemblages in the open ocean. ISME. J. 5, 999–1013. doi: 10.1038/ismej.2010.189
Singh R. P., Reddy C. R. K. (2014). Seaweed-microbial interactions: key functions of seaweed-associated bacteria. FEMS Microbiol. Ecol. 88, 213–230. doi: 10.1111/1574-6941.12297
Steinberg P. D., De Nys R. (2002). Chemical mediation of colonization of seaweed surfaces. J. Phycol. 38, 621–629. doi: 10.1046/j.1529-8817.2002.02042.x
Suh S. S., Park M., Hwang J., Kil E.-J., Jung S. W., Lee S., et al. (2015). Seasonal dynamics of marine microbial community in the south Sea of Korea. PloS One 10, e0131633. doi: 10.1371/journal.pone.0131633
Sunagawa S., Coelho L. P., Chaffron S., Kultima J. R., Labadie K., Salazar G., et al. (2015). Structure and function of the global ocean microbiome. Science 348, 1261359. doi: 10.1126/science.1261359
Telesh I. V., Khlebovich V. V. (2010). Principal processes within the estuarine salinity gradient: a review. Mar. pollut. Bull. 61, 149–155. doi: 10.1016/j.marpolbul.2010.02.008
Venkatraman K. L., Mehta A. (2019). Health benefits and pharmacological effects of Porphyra species. Plant Foods. Hum. Nutr. 74, 10–17. doi: 10.1007/s11130-018-0707-9
Vera J., Alvarez R., Murano E., Slebe J. C., Leon O. (1998). Identification of a marine agarolytic pseudoalteromonas isolate and characterization of its extracellular agarase. Appl. Environ. Microbiol. 64, 4378–4383. doi: 10.1128/AEM.64.11.4378-4383.1998
Vollmers J., Voget S., Dietrich S., Gollnow K., Smits M., Meyer K., et al. (2013). Poles apart: Arctic and Antarctic Octadecabacter strains share high genome plasticity and a new type of xanthorhodopsin. PloS One 8, e63422. doi: 10.1371/journal.pone.0063422
Wang W. J., Li X. L., Sun T. Q., Liang Z. R., Liu F. L., Sun X. T., et al. (2019). Effects of periodical drying and non-drying on nutrient content and desiccation tolerance of an intertidal Pyropia yezoensis strain subject to farming conditions. J. Appl. Phycol. 31, 1897–1906. doi: 10.1007/s10811-018-1684-y
Wang J. H., Mao Y. X., Du G. Y., Li X. J., Tang X. H. (2021). On microbial community of Pyropia haitanensis by metagenomic analysis. J. Oceanol. Limnol. 39, 1091–1102. doi: 10.1007/s00343-020-0189-0
Wang W. L., Wu L., Xu K., Xu Y., Ji D. H., Chen C. S., et al. (2020). The cultivation of Pyropia haitanensis has important impacts on the seawater microbial community. J. Appl. Phycol. 32, 2561–2573. doi: 10.1007/s10811-020-02068-6
Weigel B. L., Pfister C. A. (2019). Successional dynamics and seascape-level patterns of microbial communities on the canopy-forming kelps Nereocystis luetkeana and Macrocystis pyrifera. Front. Microbiol. 10. doi: 10.3389/fmicb.2019.00346
Wesley I. V. (2014). “Arcobacter,” in Encyclopedia of food microbiology (second edition). Eds. Batt C. A., Tortorello M. L. (Oxford: Academic Press), 61–68. doi: 10.1016/B978-0-12-384730-0.00008-2
Wietz M., Wemheuer B., Simon H., Giebel H.-A., Seibt M. A., Daniel R., et al. (2015). Bacterial community dynamics during polysaccharide degradation at contrasting sites in the southern and Atlantic oceans. Environ. Microbiol. 17, 3822–3831. doi: 10.1111/1462-2920.12842
Xie X. F., He Z. L., Hu X. J., Yin H. Q., Liu X. D., Yang Y. F. (2017). Large-Scale seaweed cultivation diverges water and sediment microbial communities in the coast of nan’ao island, south China Sea. Sci. Total. Environ. 598, 97–108. doi: 10.1016/j.scitotenv.2017.03.233
Xiong Y. Q., Yang R., Sun X. X., Yang H. T., Chen H. M. (2018). Effect of the epiphytic bacterium Bacillus sp. WPySW2 on the metabolism of Pyropia haitanensis. J. Appl. Phycol. 30, 1225–1237. doi: 10.1007/s10811-017-1279-z
Xue J. H., Zhang B. N., Zhang F., Liu Y. Y., Wu W.-J., Wu Z. M., et al. (2022). Comparative genomic analysis of the genus Marinomonas and taxonomic study of marinomonas algarum sp. nov., isolated from red algae Gelidium amansii. Arch. Microbiol. 204, 586. doi: 10.1007/s00203-022-03215-y
Yan Y. W., Yang H. C., Tang L., Li J., Mao Y. X., Mo Z. L. (2019). Compositional shifts of bacterial communities associated with Pyropia yezoensis and surrounding seawater co-occurring with red rot disease. Front. Microbiol. 10. doi: 10.3389/fmicb.2019.01666
Ye F., Guo W., Wei G. J., Jia G. D. (2018). The sources and transformations of dissolved organic matter in the pearl river estuary, China, as revealed by stable isotopes. J. Geophys. Res. Oceans. 123, 6893–6908. doi: 10.1029/2018JC014004
Yong J. J., Park S. J., Kim H. J., Rhee S. K. (2007). Glaciecola agarilytica sp. nov., an agar-digesting marine bacterium from the East Sea, Korea. Int. J. Syst. Evol. Microbiol. 57, 951–953. doi: 10.1099/ijs.0.64723-0
Yu W. N., Du Z. Z., Chang Y. Q., Mu D. S., Du Z. J. (2020). Marinomonas agarivorans sp. nov., an agar-degrading marine bacterium isolated from red algae. Int. J. Syst. Evol. Microbiol. 70, 100–104. doi: 10.1099/ijsem.0.003723
Zeng Y. X. (2019). Phylogenetic diversity of dimethylsulfoniopropionatedependent demethylase gene dmdA in distantly related bacteria isolated from Arctic and Antarctic marine environments. Acta Oceanol. Sin. 38, 64–71. doi: 10.1007/s13131-019-1393-7
Zeng Y. X., Qiao Z. Y. (2019). Diversity of dimethylsulfoniopropionate degradation genes reveals the significance of marine Roseobacter clade in sulfur metabolism in coastal areas of Antarctic Maxwell bay. Curr. Microbiol. 76, 967–974. doi: 10.1007/s00284-019-01709-5
Zhang R., Chang L. R., Xiao L. Y., Zhang X., Han Q., Li N., et al. (2020b). Diversity of the epiphytic bacterial communities associated with commercially cultivated healthy and diseased Saccharina japonica during the harvest season. J. Appl. Phycol. 32, 2071–2080. doi: 10.1007/s10811-019-02025-y
Zhang L., Shen T. T., Cheng Y., Zhao T. T., Li L., Qi P. F. (2020a). Temporal and spatial variations in the bacterial community composition in lake bosten, a large, brackish lake in China. Sci. Rep. 10, 304. doi: 10.1038/s41598-019-57238-5
Keywords: seaweed, bacterial community, coastal waters, seasonal variations, environmental factor, cultivation
Citation: Liang Z, Jia R, Sun T, Wang W, Wang C and Lu X (2022) Insights into the spatio-temporal composition, diversity and function of bacterial communities in seawater from a typical laver farm. Front. Mar. Sci. 9:1056199. doi: 10.3389/fmars.2022.1056199
Received: 28 September 2022; Accepted: 11 October 2022;
Published: 21 October 2022.
Edited by:
Wenlei Wang, Jimei University, ChinaReviewed by:
Zengling Ma, Wenzhou University, ChinaLien Yang, Jiangsu Institute of Oceanology and Marine Fisheries, China
Copyright © 2022 Liang, Jia, Sun, Wang, Wang and Lu. This is an open-access article distributed under the terms of the Creative Commons Attribution License (CC BY). The use, distribution or reproduction in other forums is permitted, provided the original author(s) and the copyright owner(s) are credited and that the original publication in this journal is cited, in accordance with accepted academic practice. No use, distribution or reproduction is permitted which does not comply with these terms.
*Correspondence: Wenjun Wang, d2p3YW5nQHlzZnJpLmFjLmNu
†These authors have contributed equally to this work and share first authorship