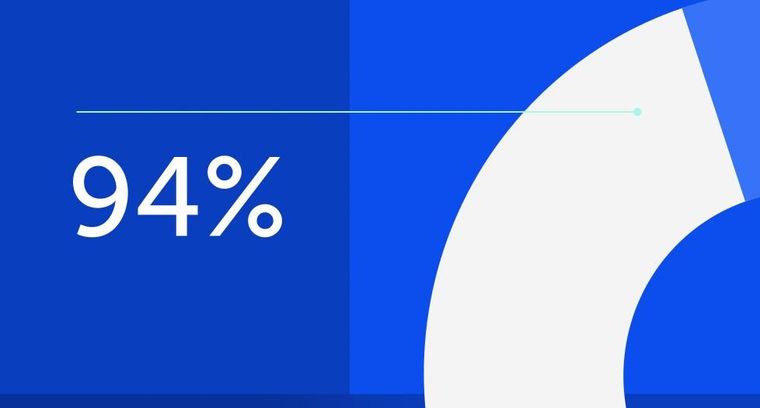
94% of researchers rate our articles as excellent or good
Learn more about the work of our research integrity team to safeguard the quality of each article we publish.
Find out more
EDITORIAL article
Front. Mar. Sci., 20 October 2022
Sec. Marine Conservation and Sustainability
Volume 9 - 2022 | https://doi.org/10.3389/fmars.2022.1050548
This article is part of the Research TopicHabitat and Distribution Models of Marine and Estuarine Species: Advances for a Sustainable FutureView all 15 articles
Editorial on the Research Topic
Habitat and distribution models of marine and estuarine species: Advances for a sustainable future
The physical and biological characterization of suitable habitats and species-specific models to estimate their extent are valuable for conservation and fisheries management. As exploited species and habitats face challenges from anthropogenic influences, such as fishing and climate change, the identification and protection of habitats becomes increasingly important. Most of the papers within this special topic issue used some form of species distribution model (SDM) to identify habitats used by fishes (Asch et al.; Crear et al.; Fabrizio et al.; Freidland et al.; Zydlewski et al.), marine mammals (Astarloa et al.), nearshore invertebrates (Cristiani et al.; Behan et al.), or deep-sea communities (Bowden et al.; Saunders et al.). A few papers focused on developing methods to better describe habitats (Griffin et al.; Henderson et al.; Cecino et al.), while other papers investigated model performance and incorporation of new statistical methods to improve model accuracy (Asch et al.; Behan et al.; Bowden et al.). Below we provide a synthesis of these papers under the topics of data sources used for analyses, statistical methods, stationarity and model performance, connectivity, and management implications; we conclude with a consideration of opportunities for advancing this field of study.
Most SDMs used presence/absence information to describe relationships between taxa and habitat features; only a few SDMs were informed by estimates of relative abundance, density, or biomass (Astarloa et al.; Behan et al.; Fabrizio et al.; Freidland et al.). Occurrence and abundance information were commonly derived from stratified random surveys using bottom trawls, dredges, or epibenthic sleds. Transect sampling with ROV video and still cameras was used in three papers, suggesting that new methods to automate image analysis allow researchers to obtain rich datasets from habitats that are otherwise poorly sampled. Two papers in this issue used acoustic telemetry (Crear et al.; Griffin et al.) to develop habitat models for marine fishes.
Data sources to describe spatio-temporal variation in environmental conditions comprised outputs from a variety of numerical models, including ocean circulation models, coupled physical-biogeochemical models, and earth system models (Asch et al.; Behan et al.; Cecino et al.; Crear et al.; Cristiani et al.; Fabrizio et al.; Henderson et al.). A few of these authors noted the need to rescale (simplify or summarize) biotic data to be consistent with the spatial resolution of oceanographic models (Asch et al.; Cecino et al.; Cristiani et al.). Physico-chemical models were also used to project habitat conditions under one or more future climate scenarios that typically included the ‘status quo.’ Resource-specific attributes (e.g., life history, distribution, length of time series, and so forth) appeared to influence the choice of projection years, as these varied among studies. Remotely sensed environmental conditions and chlorophyll-a concentrations were also incorporated into habitat models (Freidland et al.). In most papers, however, SDMs were often informed by readily available habitat descriptors (e.g., depth, temperature, salinity, dissolved oxygen) that were typically collected at the time of sampling of the biota. Biotic variables, such as prey abundance and primary productivity, were sometimes used to describe habitats (Asch et al.; Astarloa et al.; Freidland et al.). When relatively long time series (> 35 years) of observations of marine biota were available, atmospheric indices such as the PDO, AMO, and NAO were considered as climate indicators (Asch et al.; Astarloa et al.).
Statistical modelling corresponds to an ensemble of steps that considers the sampling design, the covariates and their quality, the model type, and the fitting procedure. All these aspects were addressed by the papers in this special topic issue. In particular, presence-only data are a problem for model fitting (Winship et al.) so analysts are often forced to use pseudo-absences generated by random resampling across the area (Griffin et al.). Zero-inflated data may require hurdle models to estimate the probability of occurrence and the conditional positive catch component (Astarloa et al.; Lowman et al.).
This collection of papers exemplifies the now widely accepted use of machine learning procedures that go beyond classical statistical methods. In particular, random forest approaches and regression trees (boosted by an iterative procedure) were used to identify covariates and their ranges of values and interactions that best explained observed species distributions (Cecino et al.; Fabrizio et al.; Freidland et al.; Griffin et al.; Henderson et al.; Saunders et al.). Suitable ranges for covariates can be envisaged using physiological principles (Crear et al.) or inferred from a histogram approach (Fabrizio et al.). Approaches to model species occurrence probability included logistic regression (Henderson et al.), resource selection functions (Griffin et al.), generalized linear or additive models, and generalized linear mixed-effects models (GLMM; Astarloa et al.; Lowman et al.). GLMMs were used to model space-time interactions and thus evidence of change in spatial distributions across time.
Multiple papers used recently developed numerical computing methods to efficiently incorporate spatial and temporal autocorrelation with a Bayesian approach. For example, two papers used Vector Autoregressive Spatio-Temporal (VAST) models to examine changes in animal densities and distributions through time (Astarloa et al.; Lowman et al.). VAST models treat the space-time correlation function as a time-varying spatial component so the remaining variance can be estimated as a fixed effect (Thorson and Barnett, 2017). A similar approach was used to account for spatial autocorrelation in an analysis of associations between fish and deep-sea corals (Henderson et al.).
Because SDMs are based on correlative relationships between species occurrence and covariates, model projections outside the range of values, time frame, or area used to fit the SDM must assume that the correlative relationships are valid under conditions that were not considered in model fitting. Thus, stationarity of relationships is a critical assumption when making projections under future climate scenarios. Asch et al. questioned the temporal stationarity assumption by fitting SDMs for multiple time periods and Behan et al. address the spatial stationarity assumption by fitting models for multiple sub-areas. For some species, the assumption of stationarity is questionable and depends on the covariates.
Model performance refers to the ability of the model to reliably predict species distributions using values within the fitting conditions. In most of the papers in this special topic issue, data were split into a set for model fitting and another for model testing. Model performance is assessed by cross-validation using the test set. Yet, uncertainty in the covariates is seldom considered. Bowden et al. tackle this question and show that current cross-validation procedures may lead to overestimation of model performance. They also show that in general, presence/absence is estimated more reliably than abundance, and that model performance relies on the precision and spatio-temporal resolution of covariates.
The importance of considering connectivity when evaluating habitat occupancy has been long discussed (Bryan-Brown et al., 2017). The transport and movement abilities of individuals, combined with the spatial distribution of habitats, determine the dispersal patterns of key life stages of many coastal and marine species (Cowen and Sponaugle, 2009). Closing the life cycle by linking post-settlement larvae to juveniles and adults is valuable and informs a species’ spatial distribution (Beck et al., 2001). Connectivity also plays a critical role in the theme of “seascapes” (Pittman, 2017). Two papers in this special topic issue (Cecino et al., Cristiani et al.) specifically focused on connectivity.
The effect of connectivity on predictions of species distributions can be quantified explicitly by incorporating connectivity metrics as covariates in SDMs. Cecino et al. offered a new approach to bridge standard SDMs with methods that assess connectivity. They found that centrality measures, which characterize connectivity, influenced the geographic structure of predicted habitat quality. Using a particle-tracking approach and network analysis, Cristiani et al. reported that topography acted to limit dispersal of benthic larvae among seagrass beds, and that subregions with limited exchange could be identified.
Effective management and conservation require knowledge of species habitat use and the ecosystem effects of anthropogenic change. Multiple papers raised the issue of using SDMs to identify habitats that could be targeted for protection and species conservation, particularly with the potential for habitat degradation as the climate changes (Griffin et al.; Fabrizio et al.; Cecino et al.). In addition to using SDMs to identify suitable habitats, other papers within this issue used SDMs to assess anthropogenic impacts from dams, fisheries, and wind farms (Zydlewski et al.; Lowman et al.; Freidland et al.). A common message across these papers was the importance of reporting accurate measures of uncertainty alongside predictions to ensure proper interpretation.
(1) A theme common to all papers in this special topic issue is the necessity of merging multiple datasets from unrelated sources to assemble a more complete understanding of the modeled system. Most habitat assessments involve the merging of information from physical oceanography models, biogeochemistry models, and marine ecological observations including information on trophic interactions and connectivity. To the extent practical, resolution of differences in spatial and temporal scales required to address ecological questions and those typical of hydrodynamic models would allow the exploration and application of habitat models to additional species and systems (e.g., Behan et al.).
(2) Habitat assessments, especially those for mobile life stages, should incorporate concepts and results from the rapidly expanding field of movement ecology (Nathan et al., 2008; Abrahms et al., 2021). In particular, estimates of connectivity can be improved by considering movements of organisms, which are often elicited in response to the key constituents of habitat quality. Movement and connectivity information can be valuable for assessing the actual use (i.e., realized habitat) of potential habitats predicted by SDMs.
(3) Increasing refinement of the biological characteristics of individuals represented in particle-tracking and movement models would improve predictions and allow for species and regional differences to emerge. For example, Cristiani et al. defaulted to using a single mortality rate for their community-level analysis because species-specific information was lacking.
(4) Studies that consider habitat and connectivity effects at the population and ecosystem levels and over the entire life cycle of organisms can provide insight on the cumulative life-time effects of directional changes in habitat such as loss of spawning and rearing areas (e.g., Zydlewski et al.). Agent-based modeling approaches offer a viable approach to explore such effects.
(5) Results from habitat models, and SDMs in particular, should be expressed in a manner to ensure proper interpretation. Two major considerations are the proper reporting of the confidence in model predictions (e.g., confidence intervals), and distinguishing between habitat capacity, potential habitat, and realized habitat when interpreting model results. Additionally, all data and code from these analyses should be made available to aid in reproducibility.
(6) Habitat models could be improved by considering stakeholder and local knowledge (e.g., Lowman et al.). Such information could increase the understanding of past and present conditions, movement and migration patterns of organisms, and locations and environmental conditions under which organisms were previously found.
(7) Finally, practitioners should identify and examine key assumptions of models and data used to describe relationships between habitats and biota, including stationarity (e.g., Asch et al.). Additional comparison of methods and sensitivities of models to input data would also be valuable (e.g., Bowden et al.).
All authors listed have made a substantial, direct, and intellectual contribution to the work and approved it for publication.
The authors declare that the research was conducted in the absence of any commercial or financial relationships that could be construed as a potential conflict of interest.
All claims expressed in this article are solely those of the authors and do not necessarily represent those of their affiliated organizations, or those of the publisher, the editors and the reviewers. Any product that may be evaluated in this article, or claim that may be made by its manufacturer, is not guaranteed or endorsed by the publisher.
Abrahms B., Aikens E. O., Armstrong J. B., Deacy W. W., Kauffman M. J., Merkle J. A. (2021). Emerging perspectives on resource tracking and animal movement ecology. Trends Ecol. Evol. 36 (4), 308–320. doi: 10.1016/j.tree.2020.10.018
Beck M. W., Heck K. L., Able K. W., Childers D. L., Eggleston D. B., Gillanders B. M., et al. (2001). The identification, conservation, and management of estuarine and marine nurseries for fish and invertebrates: a better understanding of the habitats that serve as nurseries for marine species and the factors that create site-specific variability in nursery quality will improve conservation and management of these areas. Bioscience 51 (8), 633–641. doi: 10.1641/0006-3568(2001)051[0633:TICAMO]2.0.CO;2
Bryan-Brown D. N., Brown C. J., Hughes J. M., Connolly R. M. (2017). Patterns and trends in marine population connectivity research. Mar. Ecol. Prog. Ser. 585, 243–256. doi: 10.3354/meps12418
Cowen R. K., Sponaugle S. (2009). Larval dispersal and marine population connectivity. Annu. Rev. Mar. Sci. 1 (1), 443–466. doi: 10.1146/annurev.marine.010908.163757
Nathan R., Getz W. M., Revilla E., Holyoak M., Kadmon R., Saltz D., et al. (2008). A movement ecology paradigm for unifying organismal movement research. Proc. Natl. Acad. Sci. 105 (49), 19052–19059. doi: 10.1073/pnas.0800375105
Keywords: SDM, habitat, ecology, spatial, models, aquatic
Citation: Fabrizio MC, Henderson MJ, Rose K and Petitgas P (2022) Editorial: Habitat and distribution models of marine and estuarine species: Advances for a sustainable future. Front. Mar. Sci. 9:1050548. doi: 10.3389/fmars.2022.1050548
Received: 21 September 2022; Accepted: 07 October 2022;
Published: 20 October 2022.
Edited and Reviewed by:
Laura Airoldi, University of Padova Chioggia Hydrobiological Station, ItalyCopyright © 2022 Fabrizio, Henderson, Rose and Petitgas. This is an open-access article distributed under the terms of the Creative Commons Attribution License (CC BY). The use, distribution or reproduction in other forums is permitted, provided the original author(s) and the copyright owner(s) are credited and that the original publication in this journal is cited, in accordance with accepted academic practice. No use, distribution or reproduction is permitted which does not comply with these terms.
*Correspondence: Mary C. Fabrizio, bWZhYnJpemlvQHZpbXMuZWR1
Disclaimer: All claims expressed in this article are solely those of the authors and do not necessarily represent those of their affiliated organizations, or those of the publisher, the editors and the reviewers. Any product that may be evaluated in this article or claim that may be made by its manufacturer is not guaranteed or endorsed by the publisher.
Research integrity at Frontiers
Learn more about the work of our research integrity team to safeguard the quality of each article we publish.